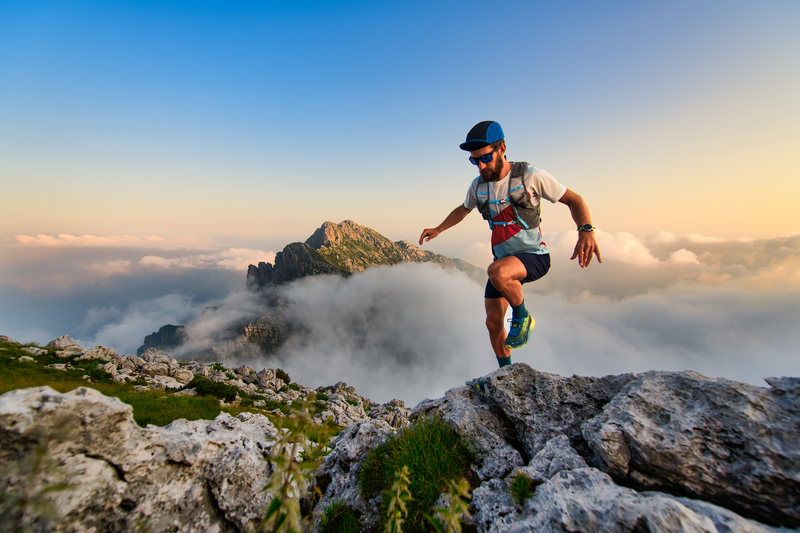
94% of researchers rate our articles as excellent or good
Learn more about the work of our research integrity team to safeguard the quality of each article we publish.
Find out more
ORIGINAL RESEARCH article
Front. Immunol. , 13 February 2024
Sec. Autoimmune and Autoinflammatory Disorders : Autoimmune Disorders
Volume 15 - 2024 | https://doi.org/10.3389/fimmu.2024.1321507
This article is part of the Research Topic Prognostic and Predictive Factors in Autoimmune Connective Tissue Disorders View all 13 articles
Background: Lupus nephritis (LN) is a common disease with diverse clinical and pathological manifestations. A major challenge in the management of LN is the inability to predict its treatment response at an early stage. The objective of this study was to determine whether the density of tubulointerstitial macrophage infiltration can be used to predict treatment response in LN and whether its addition to clinicopathological data at the time of biopsy would improve risk prediction.
Methods: In this retrospective cohort study, 430 patients with LN in our hospital from January 2010 to December 2017 were included. We used immunohistochemistry to show macrophage and lymphocyte infiltration in their biopsy specimens, followed by quantification of the infiltration density. The outcome was the treatment response, defined as complete or partial remission at 12 months of immunosuppression.
Results: The infiltration of CD68+ macrophages in the interstitium increased in patients with LN. High levels of CD68+ macrophage infiltration in the interstitium were associated with a low probability of treatment response in the adjusted analysis, and verse vice. The density of CD68+ macrophage infiltration in the interstitium alone predicted the response to immunosuppression (area under the curve [AUC], 0.70; 95% CI, 0.63 to 0.76). The addition of CD68+cells/interstitial field to the pathological and clinical data at biopsy in the prediction model resulted in an increased AUC of 0.78 (95% CI, 0.73 to 0.84).
Conclusion: The density of tubulointerstitial macrophage infiltration is an independent predictor for treatment response in LN. Adding tubulointerstitial macrophage infiltration density to clinicopathological data at the time of biopsy significantly improves risk prediction of treatment response in LN patients.
Lupus nephritis (LN) is a common and important manifestation of systemic lupus erythematosus (SLE). About 60% of patients with SLE experience renal involvement, and 10% to 20% progress to end-stage kidney disease (ESKD) (1, 2). Chronic kidney disease (CKD) and ESKD caused by LN are the leading causes of mortality among patients with SLE. Although the precise pathogenesis of LN is not very clear at present, it is widely believed that immune and autoimmune activation plays an important role. Pathologically, LN is characterized by immune complex deposition and inflammation in glomeruli and the tubulointerstitium (3). Therefore, glucocorticoid steroids combined with other immunosuppressants are recommended to treat LN. With the rapid progress in the treatment of LN, several new immunosuppression strategies that target different pathways in the pathogenesis of LN have recently emerged (4). Despite these advancements, a subset of patients remains unresponsive to current therapeutic interventions.
It has been desired that a physician can predict the treatment response of patients with LN based on the biopsy. Unfortunately, there is a lack of good biomarkers that predict the response to treatment. Previous studies have shown that hypertension, serum creatinine (SCr), chronicity index score (CIs), and other indicators can serve as predictors of treatment response (5–8). However, the prognostic value of the conventional clinicopathological features remains low. Immune cells, including T lymphocytes, B lymphocytes, and macrophages, play roles in the pathogenesis of LN. The density of the immune cell infiltration, especially macrophages, correlates with the severity of renal histologic lesions and the level of SCr at biopsy (9, 10). Previous studies have demonstrated that an increased number of macrophages is associated with impaired kidney function. After activation, macrophages can secrete proinflammatory cytokines and activate other immune cells (9, 11). Additionally, macrophages exhibit compromised phagocytic activity and a diminished ability to eliminate apoptotic cells, resulting in the excessive generation of autoantibodies in LN patients.
Based on the above studies, macrophages have been suggested to be closely associated with LN. Therapeutic interventions targeting macrophage function in LN patients have been explored, and ongoing studies are investigating the potential benefits of macrophage depletion (12, 13). Nevertheless, it is not known whether the density of macrophage infiltration can serve as a predictor for treatment response in LN. Therefore, we aimed to determine whether the extent of macrophage infiltration in kidney can predict the response to immunosuppression in LN patients.
This retrospective cohort study was conducted with the approval of the Institutional Review Board of Nanjing Jinling Hospital (2021NZKY-021-01). A total of 1,297 patients with biopsy-proven LN in the hospital from January 2010 to December 2017 were screened for the cohort. Their renal biopsy samples were assessed following the classification criteria established by the International Society of Nephrology/Renal Pathology Society (ISN/RPS) (14). The patients who met at least four of the criteria for SLE in the 1997 revised American College of Rheumatology classification were selected for the cohort. Baseline and follow-up data of the LN patients were obtained from the database of the National Clinical Research Center of Kidney Diseases, Jinling Hospital.
All the patients received regular immunosuppressive therapy for one year. Patients with incomplete information of CD4+, CD8+ lymphocytes, and CD68+ macrophages in kidney tissue and follow-up records were excluded (Figure 1). Ultimately, a total of 430 patients were enrolled in the study.
Baseline data collection and follow-up visits were conducted by research personnel. Data on demographic characteristics, clinicopathological features, treatment modalities, and treatment response were obtained. Anti-nuclear antibodies (ANA) and anti-double stranded DNA antibodies (anti-dsDNA) were described as positive or negative. The estimated glomerular filtration rate (eGFR) was calculated by the Chronic Kidney Disease Epidemiology Collaboration (CKD-EPI) (15). Disease activity was scored according to the Systemic Lupus Erythematous Disease Activity Index (SLEDAI). Treatment response included complete remission (urinary protein quantitation (UPRO) < 0.4 g/24 hours and normal SCr) and partial remission (≥ 50% reduction in proteinuria and UPRO < 3.5 g/24 hours, serum albumin (ALB) level ≥ 30 g/L, and normal or ≤ 25% increase in SCr level from baseline) at 12 months.
All renal biopsy specimens were obtained by percutaneous needle biopsy and were routinely examined by light microscopy, immunofluorescence, and electron microscopy. Two experienced pathologists independently examined the kidney biopsy specimens. They had access to the patient’s clinical information, including vital signs, blood test results, urinalysis results, and clinical diagnoses. Pathological parameters such as activity index score (AIs) and chronicity index score (CIs) were evaluated as described previously (16).
Infiltrating macrophages and lymphocytes in renal tissue were identified using immunohistochemical staining. Paraffin-embedded tissue sections (2 µm) were incubated with the following primary antibodies: mouse anti-human CD68 mAb (M0876; DAKO, Glostrup, Denmark), rabbit antihuman CD3 antibody (SP7; LEICA), rabbit antihuman CD4 antibody (NCL-L-368; LEICA) and rabbit antihuman CD8 (NCL-L-295). The slides were then incubated with the secondary antibody and visualized using a LEICA System Kit and counterstained with hematoxylin. The stained sections were scanned with a Digital Pathology Slide Scanner (Leica, Wetzlar, Germany). Positive-stained cells were automatically counted in all nonglobally sclerotic glomeruli at 400 magnifications using Aperio eSlide Manager (Leica)(Figure 2).
Figure 2 Characteristics of infiltrating cells in two groups. (A, B) Representative images of immunostaining for CD4 of tubulointerstitial (×200), (C, D) immunostaining for CD8 of tubulointerstitial(×200), (E, F) immunostaining for CD68 of glomerular (×400), (G,H) immunostaining for CD68 of tubulointerstitial(×200).
The density of glomerular and tubulointerstitial infiltration between the response and nonresponse groups was compared using the Mann–Whitney test and the Spearman correlation between the density of glomerular and tubulointerstitial infiltration and clinicopathologic variables was calculated. In addition, logistic regression analyses were used to examine the association between different levels of glomerular and tubulointerstitial infiltration and the treatment response, with and without adjustment for gender, age, UPRO, SCr, C4, LN duration, hypertension, repeat biopsy, AIs, CIs, and ISN/RPS classification. Then all patients included in this study were randomly categorized into a training cohort or validation cohort in a 3:1 ratio. Three prediction models were used: a univariable model with the level of infiltration in glomeruli or tubulointerstitium separately; a model with CD4+ cells/interstitial field, CD8+ cells/interstitial field, and CD68+ cells/interstitial field together; and a model using combinations of the level of infiltration in glomerulus or tubulointerstitium, other pathological and clinical data. We also used stepwise regression with forward selection and backward elimination to obtain the model that minimizes the Alkaike information criterion (AIC). Performance of three logistic regression models in predicting the treatment response in both the training set and the validation set was reported and the predictive accuracy of these models was assessed by discrimination as measured by AUC.
Data analysis was performed using RStudio version 2023.03.0, an integrated development environment for R version 4.2.3 (R Core Team, Vienna, Austria). The difference was considered statistically significant as a two-sided P-value < 0.05.
Included in this study were 430 biopsy-proven LN patients, with the female gender predominating (84.65%) and a mean age of 30.31 ± 11.14 years (Table 1). The median SCr level at presentation was 0.83 (0.63–1.31) mg/dl. The median UPRO was 3.10 (1.72-5.58) g/24h. The median C3 and C4 levels were 0.41 (0.10-1.28) g/L and 0.08 (0.04-0.12) g/L, respectively. Most patients in our study had proliferative LN, of which 209 patients (48.60%) had pure proliferative LN (n = 35 for class III and n = 174 for class IV), 167 patients (38.83%) mixed proliferative LN (n = 44 for class III + V and n = 123 for class IV + V), 2 (0.47%) lupus podocytopathy, 5 (1.16%) class II LN, and 47 (10.93%) membranous LN. At the time of biopsy, the median AIs and CIs were evaluated by modified NIH activity and chronicity index of 7.00 (4.00-9.00) and 2.00 (1.00-3.00), respectively. The induction therapy was based on the histological lesions shown by renal biopsy. All the patients received treatment with corticosteroids without contraindications. Additionally, 126 (29.30%) received multi-target therapy, of which 120 cases of mycophenolate mofetil (MMF) combined with tacrolimus and 6 MMF combined with cyclosporine. There were 112 (26.05%) cyclophosphamide (CYC), 74 (17.21%) MMF, 66 (15.35%) calcineurin inhibitors (including 60 tacrolimus and 6 cyclosporine), 16 (3.72%) Triptergium wilfordii (TW), 7 (1.63%) rituximab (RTX), 6 (1.40%) azathioprine (AZA), and 5 (1.16%) autologous hematopoietic stem cell transplantation (AHSCT), respectively. 18 (4.19%) patients were treated with corticosteroids alone (Table 1).
Our analysis encompassed four patients who underwent repeat renal biopsies. The characteristics of the patients at the time of kidney biopsy were stratified by response to immunosuppression and are presented in Tables 1, 2.
There were 69.07% of patients (n = 297) in the response group and the rest patients (n = 133) in the nonresponse group. The proportion of women in the response group was significantly higher than in the nonresponse group (P < 0.01). Patients in the response group were more likely to have higher SLEDAI scores (P = 0.013). However, patients in the nonresponse group were more likely to have comorbid hypertension, a longer course of SLE and LN, and a higher frequency of cardiac involvement (P < 0.05). Regarding laboratory parameters, patients in the response group were more likely to have better renal function, fewer immunological indexes, and more numbers of lymphocyte subsets than the nonresponse group (P < 0.05). There were no significant differences in age, diabetes, SS, induction therapy, and baseline level of leukocyte counts, platelets (PLT), hemoglobin (Hb), ALB, UPRO, Anti-Complement 1q antibodies (anti-C1q), CD3+ cell counts (CD3), and CD8+ cell counts (CD8) between the two groups (P > 0.05).
Histologically, there was no significant difference in pathological classification between the two groups. The patients in the nonresponse group had higher CIs compared with those in the response group (P < 0.05). Most importantly, the level of inflammatory infiltration including CD4+, CD8+ T cells, and CD68+ macrophages in tubulointerstitium was significantly higher in the nonresponse group. However, infiltrated CD68+ macrophages in glomeruli were increased in the response group.
We estimated the levels of CD4+, CD8+ lymphocytes, and CD68+ macrophages in glomeruli and tubulointerstitium in 430 patients with LN. As shown in Figure 3, Table 3, infiltrated CD4+, CD8+ lymphocytes, and CD68+ macrophages in tubulointerstitium were increased in patients with proliferative LN compared with those with pure SLE ISN/RPS class V. The number of CD68+ macrophages in glomeruli was also increased in patients with proliferative LN, but the statistical difference was not significant.
Figure 3 Correlations between ISN/RPS classification in LN with CD4+ lymphocyte infiltration in tubulointerstitium (A), CD8+ lymphocyte infiltration in tubulointerstitium (B), CD68+ macrophage infiltration in tubulointerstitium (C), and CD68+ macrophage infiltration in glomeruli (D). *P < 0.05, **P < 0.01, ***P < 0.001, ****P < 0.0001. Boxplot: boxplot medians (center lines), interquartile ranges (box ranges), whisker ranges; LP, lupus podocytopathy. ns, not significant.
The density of infiltrated CD68+ macrophages in glomeruli correlated with age, SLEDAI, SLE duration, LN duration, PLT, Hb, blood urea nitrogen (BUN), UA, C3, C4, CD3, CD8, AIs, and CIs, with the highest Spearman correlation coefficients (r) being 0.41. The density of tubulointerstitial infiltration, including CD4+ cells, CD8+ cells, and CD68+ cells, positively correlated with BUN, SCr, and CIs, and negatively correlated with Hb and eGFR. The absolute values of r ranged from 0.31 to 0.51 (Figure 4, Table 4).
Figure 4 The heatmap shows correlations between parameters relevant for LN among the 430 patients enrolled. Only significant p values (P < 0.05) are shown. Red and blue colors represent significant negative and positive correlations. Darker color represents stronger correlations. *P < 0.05, **P < 0.01, ***P < 0.001.WBC, white blood cell; PLT, blood platelet; Hb, hemoglobin; ALB, albumin; SCr, serum creatinine; UA, blood uric acid; UPRO, urinary protein quantitation; eGFR, estimated glomerular filtration rate; C3, complement 3; C4, complement 4; CD4, CD4+ cell count; CD8, CD8+ cell count; AIs, activity index score; CIs, chronicity index score; GN, glomerulus number.
The logistic regression analyses indicated that the tertile groups with more infiltration had a lower probability of responding to treatment in the crude model when they were compared with the groups with fewer CD4+, CD8+, and CD68+ cells infiltrated in the tubulointerstitium. The association between the density of CD68+ cell infiltration in the glomeruli and the treatment response disappeared even without adjustment (Table 5).
After adjusting for gender, age, UPRO, UA, SCr, and C4, the density of interstitium infiltration, expressed as CD4+, CD8+, and CD68+ cells in the tubulointerstitium, remained significantly associated with treatment response, although this association was attenuated. The association between the density of CD4+ and CD8+ cells in the tubulointerstitium and treatment response disappeared after further adjusting for gender, age, UPRO, SCr, C4, LN duration, hypertension, repeat biopsy, AIs, CIs, and ISN/RPS classification. However, the density of CD68+ cells in the tubulointerstitium remained significantly associated with treatment response after further adjustment. Among different level groups, the density of CD68+ cells in the tubulointerstitium was significantly associated with treatment response in a high-level group (452–1600) (adjusted HR, 0.451; 95% CI, 0.231, 0.878) (Table 5).
Among the univariable models, inflammatory cell infiltration in tubulointerstitium had good performance in predicting the response to treatment with AUCs of 0.6672, 0.6592, and 0.6973 for CD4+, CD8+, and CD68+ cells, respectively (Table 6).
Table 6 Performance of inflammatory cell infiltration, clinical data, or other renal pathological features for predicting response to treatment.
Multivariable models incorporating infiltrations of all the inflammatory cells in tubulointerstitium, including CD4+, CD8+, and CD68+ cells, did not improve the predictive performance as compared with the single density of CD68+ macrophages in tubulointerstitium. To select a model that is convenient for clinical use, we used stepwise regression to select the variables with a minimized Alkaike information criterion. The final model included eight variables, including sex, SLEDAI, SCr, ANA, C4, CD4, CIs, and the density of CD68+ macrophage in the tubulointerstitium. The model was logit (P)= -0.4844 + 0.6953 (if male) +0.0887*SLEDAI -0.5931*SCr -4.1868*C4 + 1.4451 (if ANA positive) +0.0010*CD4 -0.2040*CIs -0.0017*the density of CD68+ macrophages in tubulointerstitium, where p is the probability of being responsive to treatment. This model produced an AUC of 0.7812 (95% CI, 0.7266 to 0.8358) in the training cohort and 0.7315 (95% CI, 0.6010 to 0.8620) in the validation cohort, respectively (Table 6).
Compared with the model containing CD68+ macrophages only in tubulointerstitium, the final model significantly improved the risk reclassification, and net reclassification improved by 4.5%.
Therefore, our subsequent study was focused on the infiltration of CD68+ macrophages in the tubulointerstitium. The relationship between CD68+ macrophages and response to immunosuppression was further stratified by gender, age, SLEDAI, SCr, UA, eGFR, UPRO, anti-dsDNA, C3, C4, CD4, PLN (proliferative lupus nephritis), AIs, and CIs (Table 7).
Table 7 Relationship between the density of CD68+ macrophage in tubulointerstitium and response to treatment under different stratification.
Our study has indicated that the density of CD68+ macrophage infiltration in the tubulointerstitium can predict the treatment response with high accuracy. Among the inflammatory cells tested, only the density of CD68+ macrophage infiltration in the tubulointerstitium at the time of renal biopsy was associated with response in the treatment for one year. The density of CD68+ macrophage infiltration was shown to be a more effective predictor of treatment outcome compared to conventional histological assessment. Furthermore, we have developed a simple prediction model that incorporates clinicopathological variables and CD68+ cell infiltration and has an AUC of 0.78.
In the majority of previous studies, several variables such as SCr, CIs, age, sex, Hb, urine protein-to-creatinine ratio (UPCR) or UPRO, LN duration, ISN/RPS classification, interstitial fibrosis, and tubular atrophy, have been identified as independent risk factors for poor treatment response in LN patients. In this study, eight factors were included in the prediction model, namely SCr, CIs, the density of CD68+ macrophages in the tubulointerstitium, sex, SLEDAI, ANA, C4, and CD4. Notably, the density of CD68+ macrophage infiltration in the tubulointerstitium demonstrated exceptional predictive ability for treatment response, even superior over the established factors such as SCr and CIs. Indeed, it has previously been suggested that urine macrophages could be a promising marker for identifying patients with active kidney disease in childhood-onset systemic lupus erythematosus (cSLE) (17). Also, one study showed that the number of CD68+ macrophages in the tubulointerstitium was an independent variable associated with poor renal outcomes in proliferative LN patients with a mean follow-up of 45 months (18).
Previous studies showed that macrophage infiltration density was closely associated with renal function decline in both transplanted kidneys and immunocomplex nephritis. Jan Hinrich Brasen et al. showed that the density of macrophages predicted future renal transplant function and improved the prognostic value of early renal transplant biopsies (19). Consistently, one study showed that tubulointerstitial macrophage infiltration was correlated with renal interstitial fibrosis and significant reduction of eGFR in patients with IgA Nephropathy (IgAN) (20), suggesting that renal tubulointerstitial macrophage infiltration was a potential hallmark of chronic kidney injury. Macrophages were shown to be necessary for the development of immune glomerulonephritis mediated by pathogenic antibodies in mouse models of LN, thus being a potential therapeutic target for LN (12, 21–23). In mouse models of lupus, functional impairment or depletion of macrophages resulted in attenuation of kidney injury (24, 25). Consistently, the reduction of macrophage recruitment to the kidney by disrupting the action of the related chemokines or their receptors also alleviated kidney injury in the lupus mice (26, 27). Similarly, macrophage injection or macrophage infiltration stimulation in lupus-prone mice aggravated nephritis and kidney damage (28).
At present, there is no panel of biomarkers or modeling approach for LN that exhibits superiority in predicting the response after treatment for one year. Quantification of tubulointerstitial macrophage infiltration is simple and robust, which may help improve the treatment decision for LN patients, particularly those who are at high risk of disease progression. After comparing the predictive performance of various types of inflammatory cell infiltration, we have developed a model combining tubulointerstitial macrophage infiltration and clinicohistological data in the present study. The results of response prediction to immunosuppression were consistent between training and validation cohorts. For simplicity and convenience, the final model uses only eight variables, nevertheless, it still shows good predictive performance. In addition, we conducted internal verification in the Pred+MMF+CNIs, Pred+CYC, Pred+MMF, and Pred+CNIs groups, and the AUC were 0.758, 0.719, 0.747, and 0.722 respectively, and there were no statistical differences between the groups.
This study has several limitations. Firstly, we excluded the patients whose data of CD4+, CD8+ lymphocytes, and CD68+ macrophages in the kidney biopsy sample and follow-up records were lacking, as noted earlier in the Methods. This exclusion might have resulted in both selection bias and confounding bias. Secondly, it was a pity that we did not have the quantitative data about the level of anti-dsDNA antibody, so we were unable to analyze the association between the level of anti-dsDNA antibody and response to treatment. Thirdly, the retrospective nature of this study prevented the collection of data on cumulative immunosuppressive agent dosage. Future prospective studies should be considered analyzing the correlation between cumulative immunosuppressive agent dosage and the level of CD68+ macrophage infiltration. Lastly, this is a single-center study conducted and validated in a Chinese population.
In conclusion, we have identified the density of macrophage infiltration in tubulointerstitium as an independent and robust predictor of response to immunosuppression in patients with LN. Our prediction model that combines the density of CD68+ macrophage in tubulointerstitium with clinicopathologic data can improve early treatment decisions for patients with LN.
The raw data supporting the conclusions of this article will be made available by the authors, without undue reservation.
The studies involving humans were approved by Institutional Review Board of Nanjing Jinling Hospital. The studies were conducted in accordance with the local legislation and institutional requirements. Written informed consent for participation was not required from the participants or the participants’ legal guardians/next of kin in accordance with the national legislation and institutional requirements.
JW: Data curation, Investigation, Methodology, Writing – original draft, Writing – review & editing, Project administration, Validation. WL: Data curation, Investigation, Writing – original draft. MZ: Data curation, Investigation, Writing – original draft. YT: Data curation, Investigation, Writing – original draft. DC: Data curation, Investigation, Writing – original draft. DQ: Data curation, Investigation, Writing – original draft. FX: Methodology, Writing – original draft. DL: Methodology, Writing – original draft. ZC: Conceptualization, Supervision, Writing – review & editing. HZ: Conceptualization, Supervision, Writing – review & editing.
The author(s) declare financial support was received for the research, authorship, and/or publication of this article. This work was supported by the National Key Research and Development Project of China (Grant number 2021YFC2501302) and Jinling Hospital (2023LCZLXB049, 2023JCYJYB126, 2022LCZLXJS7).
The authors thank Professor Shi Shaolin for his help in revising and polishing the manuscript.
The authors declare that the research was conducted in the absence of any commercial or financial relationships that could be construed as a potential conflict of interest.
All claims expressed in this article are solely those of the authors and do not necessarily represent those of their affiliated organizations, or those of the publisher, the editors and the reviewers. Any product that may be evaluated in this article, or claim that may be made by its manufacturer, is not guaranteed or endorsed by the publisher.
1. Croca SC, Rodrigues T, Isenberg DA. Assessment of a lupus nephritis cohort over a 30-year period. Rheumatol (Oxford) (2011) 50(8):1424–30. doi: 10.1093/rheumatology/ker101
2. Maroz N, Segal MS. Lupus nephritis and end-stage kidney disease. Am J Med Sci (2013) 346(4):319–23. doi: 10.1097/MAJ.0b013e31827f4ee3
3. Giannakakis K, Faraggiana T. Histopathology of lupus nephritis. Clin Rev Allergy Immunol (2011) 40(3):170–80. doi: 10.1007/s12016-010-8207-1
4. Mok CC, Teng Y, Saxena R, Tanaka Y. Treatment of lupus nephritis: consensus, evidence and perspectives. Nat Rev Rheumatol (2023) 19(4):227–38. doi: 10.1038/s41584-023-00925-5
5. Moroni G, Gatto M, Tamborini F, Quaglini S, Radice F, Saccon F, et al. Lack of EULAR/ERA-EDTA response at 1 year predicts poor long-term renal outcome in patients with lupus nephritis. Ann Rheum Dis (2020) 79(8):1077–83. doi: 10.1136/annrheumdis-2020-216965
6. Chen YE, Korbet SM, Katz RS, Schwartz MM, Lewis EJ. Value of a complete or partial remission in severe lupus nephritis. Clin J Am Soc Nephrol (2008) 3(1):46–53. doi: 10.2215/CJN.03280807
7. Ioannidis JP, Boki KA, Katsorida ME, Drosos AA, Skopouli FN, Boletis JN, et al. Remission, relapse, and re-remission of proliferative lupus nephritis treated with cyclophosphamide. Kidney Int (2000) 57(1):258–64. doi: 10.1046/j.1523-1755.2000.00832.x
8. Ichinose K, Kitamura M, Sato S, Eguchi M, Okamoto M, Endo Y, et al. Complete renal response at 12 months after induction therapy is associated with renal relapse-free rate in lupus nephritis: a single-center, retrospective cohort study. Lupus (2019) 28(4):501–9. doi: 10.1177/0961203319829827
9. Tucci M, Stucci S, Strippoli S, Silvestris F. Cytokine overproduction, T-cell activation, and defective T-regulatory functions promote nephritis in systemic lupus erythematosus. J BioMed Biotechnol (2010) 2010:457146. doi: 10.1155/2010/457146
10. Olmes G, Büttner-Herold M, Ferrazzi F, Distel L, Amann K, Daniel C. CD163+ M2c-like macrophages predominate in renal biopsies from patients with lupus nephritis. Arthritis Res Ther (2016) 18:90. doi: 10.1186/s13075-016-0989-y
11. Biermann MH, Veissi S, Maueröder C, Chaurio R, Berens C, Herrmann M, et al. The role of dead cell clearance in the etiology and pathogenesis of systemic lupus erythematosus: dendritic cells as potential targets. Expert Rev Clin Immunol (2014) 10(9):1151–64. doi: 10.1586/1744666X.2014.944162
12. Ahamada MM, Jia Y, Wu X. Macrophage polarization and plasticity in systemic lupus erythematosus. Front Immunol (2021) 12:734008. doi: 10.3389/fimmu.2021.734008
13. Yoo EJ, Oh KH, Piao H, Kang HJ, Jeong GW, Park H, et al. Macrophage transcription factor TonEBP promotes systemic lupus erythematosus and kidney injury via damage-induced signaling pathways. Kidney Int (2023) 104(1):163–80. doi: 10.1016/j.kint.2023.03.030
14. Weening JJ, D'Agati VD, Schwartz MM, Seshan SV, Alpers CE, Appel GB, et al. The classification of glomerulonephritis in systemic lupus erythematosus revisited. Kidney Int (2004) 65(2):521–30. doi: 10.1111/j.1523-1755.2004.00443.x
15. Levey AS, Stevens LA, Schmid CH, Zhang YL, Castro AF3, Feldman HI, et al. A new equation to estimate glomerular filtration rate. Ann Intern Med (2009) 150(9):604–12. doi: 10.7326/0003-4819-150-9-200905050-00006
16. Bajema IM, Wilhelmus S, Alpers CE, Bruijn JA, Colvin RB, Cook HT, et al. Revision of the International Society of Nephrology/Renal Pathology Society classification for lupus nephritis: clarification of definitions, and modified National Institutes of Health activity and chronicity indices. Kidney Int (2018) 93(4):789–96. doi: 10.1016/j.kint.2017.11.023
17. Inthavong H, Vanarsa K, Castillo J, Hicks MJ, Mohan C, Wenderfer SE. Urinary CD163 is a marker of active kidney disease in childhood-onset lupus nephritis. Rheumatol (Oxford) (2023) 62(3):1335–42. doi: 10.1093/rheumatology/keac465
18. Chen J, Cui L, Ouyang J, Wang J, Xu W. Clinicopathological significance of tubulointerstitial CD68 macrophages in proliferative lupus nephritis. Clin Rheumatol (2022) 41(9):2729–36. doi: 10.1007/s10067-022-06214-y
19. Bräsen JH, Khalifa A, Schmitz J, Dai W, Einecke G, Schwarz A, et al. Macrophage density in early surveillance biopsies predicts future renal transplant function. Kidney Int (2017) 92(2):479–89. doi: 10.1016/j.kint.2017.01.029
20. Soares MF, Genitsch V, Chakera A, Smith A, MacEwen C, Bellur SS, et al. Relationship between renal CD68(+) infiltrates and the Oxford Classification of IgA nephropathy. Histopathology (2019) 74(4):629–37. doi: 10.1111/his.13768
21. Luan J, Fu J, Chen C, Jiao C, Kong W, Zhang Y, et al. LNA-anti-miR-150 ameliorated kidney injury of lupus nephritis by inhibiting renal fibrosis and macrophage infiltration. Arthritis Res Ther (2019) 21(1):276. doi: 10.1186/s13075-019-2044-2
22. Ding T, Yi T, Li Y, Zhang W, Wang X, Liu J, et al. Luteolin attenuates lupus nephritis by regulating macrophage oxidative stress via HIF-1α pathway. Eur J Pharmacol (2023) 953:175823. doi: 10.1016/j.ejphar.2023.175823
23. Jing C, Castro-Dopico T, Richoz N, Tuong ZK, Ferdinand JR, Lok L, et al. Macrophage metabolic reprogramming presents a therapeutic target in lupus nephritis. Proc Natl Acad Sci U.S.A. (2020) 117(26):15160–71. doi: 10.1073/pnas.2000943117
24. Chalmers SA, Chitu V, Herlitz LC, Sahu R, Stanley ER, Putterman C. Macrophage depletion ameliorates nephritis induced by pathogenic antibodies. J Autoimmun (2015) 57:42–52. doi: 10.1016/j.jaut.2014.11.007
25. Zhang W, Zhou Q, Xu W, Cai Y, Yin Z, Gao X, et al. DNA-dependent activator of interferon-regulatory factors (DAI) promotes lupus nephritis by activating the calcium pathway. J Biol Chem (2013) 288(19):13534–50. doi: 10.1074/jbc.M113.457218
26. Devarapu SK, Kumar Vr S, Rupanagudi KV, Kulkarni OP, Eulberg D, Klussmann S, et al. Dual blockade of the pro-inflammatory chemokine CCL2 and the homeostatic chemokine CXCL12 is as effective as high dose cyclophosphamide in murine proliferative lupus nephritis. Clin Immunol (2016) 169:139–47. doi: 10.1016/j.clim.2016.07.003
27. Liu H, Diao C, Wu C, Xia L, Duan H, Fang F, et al. Anti-macrophage-derived chemokine antibody relieves murine lupus nephritis. Rheumatol Int (2011) 31(11):1459–64. doi: 10.1007/s00296-010-1499-x
Keywords: lupus nephritis, macrophage infiltration, treatment response, predictor, predictive models
Citation: Wang J, Lou W, Zhu M, Tu Y, Chen D, Qiu D, Xu F, Liang D, Cheng Z and Zhang H (2024) Prediction of treatment response in lupus nephritis using density of tubulointerstitial macrophage infiltration. Front. Immunol. 15:1321507. doi: 10.3389/fimmu.2024.1321507
Received: 14 October 2023; Accepted: 29 January 2024;
Published: 13 February 2024.
Edited by:
Francesca Wanda Rossi, University of Naples Federico II, ItalyReviewed by:
Filippo Fagni, University of Erlangen Nuremberg, GermanyCopyright © 2024 Wang, Lou, Zhu, Tu, Chen, Qiu, Xu, Liang, Cheng and Zhang. This is an open-access article distributed under the terms of the Creative Commons Attribution License (CC BY). The use, distribution or reproduction in other forums is permitted, provided the original author(s) and the copyright owner(s) are credited and that the original publication in this journal is cited, in accordance with accepted academic practice. No use, distribution or reproduction is permitted which does not comply with these terms.
*Correspondence: Zhen Cheng, Y2hlbmd6aGVuMzNAaG90bWFpbC5jb20=; Haitao Zhang, aHR6aGFuZzE2M0AxNjMuY29t
Disclaimer: All claims expressed in this article are solely those of the authors and do not necessarily represent those of their affiliated organizations, or those of the publisher, the editors and the reviewers. Any product that may be evaluated in this article or claim that may be made by its manufacturer is not guaranteed or endorsed by the publisher.
Research integrity at Frontiers
Learn more about the work of our research integrity team to safeguard the quality of each article we publish.