- 1Division of Rheumatology, The First Affiliated Hospital of Nanjing Medical University, Nanjing, Jiangsu, China
- 2Division of Rheumatology, Wuxi People’s Hospital, Wuxi, Jiangsu, China
- 3Division of Rheumatology, Huai’an First People’s Hospital, Huai’an, Jiangsu, China
- 4Division of Rheumatology, The First People’s Hospital of Changzhou, Changzhou, Jiangsu, China
- 5Division of Rheumatology, Affiliated Hospital of Nantong University, Nantong, Jiangsu, China
- 6Division of Rheumatology, Northern Jiangsu People’s Hospital, Yangzhou, Jiangsu, China
- 7Division of Rheumatology, Changzhou No.2 People’s Hospital, Changzhou, Jiangsu, China
- 8Division of Rheumatology, Affiliated Hospital of Xuzhou Medical University, Xuzhou, Jiangsu, China
- 9Division of Rheumatology, The First Affiliated Hospital of Soochow University, Suzhou, Jiangsu, China
- 10Division of Rheumatology, Jiangsu Province Hospital of Chinese Medicine, Nanjing, Jiangsu, China
- 11Division of Rheumatology, Nanjing First Hospital, Nanjing, Jiangsu, China
- 12Division of Rheumatology, The Second Affiliated Hospital of Soochow University, Suzhou, Jiangsu, China
- 13Division of Rheumatology, Xuzhou Central Hospital, Xuzhou, Jiangsu, China
- 14Division of Rheumatology, Yancheng No.1 People’s Hospital, Yancheng, Jiangsu, China
- 15Division of Rheumatology, Zhongda Hospital Southeast University, Nanjing, Jiangsu, China
- 16Division of Rheumatology, The Affiliated Suqian First People’s Hospital of Nanjing Medical University, Suqian, Jiangsu, China
- 17Division of Rheumatology, The First People’s Hospital of Lianyungang, Lianyungang, Jiangsu, China
- 18Division of Rheumatology, Wuxi No.2 People’s Hospital, Wuxi, Jiangsu, China
- 19Research Institute of Clinical Medicine, The First Affiliated Hospital of Nanjing Medical University, Nanjing, Jiangsu, China
- 20Division of Cardiology, The First Affiliated Hospital of Nanjing Medical University, Nanjing, Jiangsu, China
Background: The prognosis of anti-melanoma differentiation-associated gene 5 positive dermatomyositis (anti-MDA5+DM) is poor and heterogeneous. Rapidly progressive interstitial lung disease (RP-ILD) is these patients’ leading cause of death. We sought to develop prediction models for RP-ILD risk in anti-MDA5+DM patients.
Methods: Patients with anti-MDA5+DM were enrolled in two cohorts: 170 patients from the southern region of Jiangsu province (discovery cohort) and 85 patients from the northern region of Jiangsu province (validation cohort). Cox proportional hazards models were used to identify risk factors of RP-ILD. RP-ILD risk prediction models were developed and validated by testing every independent prognostic risk factor derived from the Cox model.
Results: There are no significant differences in baseline clinical parameters and prognosis between discovery and validation cohorts. Among all 255 anti-MDA5+DM patients, with a median follow-up of 12 months, the incidence of RP-ILD was 36.86%. Using the discovery cohort, four variables were included in the final risk prediction model for RP-ILD: C-reactive protein (CRP) levels, anti-Ro52 antibody positivity, short disease duration, and male sex. A point scoring system was used to classify anti-MDA5+DM patients into moderate, high, and very high risk of RP-ILD. After one-year follow-up, the incidence of RP-ILD in the very high risk group was 71.3% and 85.71%, significantly higher than those in the high-risk group (35.19%, 41.69%) and moderate-risk group (9.54%, 6.67%) in both cohorts.
Conclusions: The CROSS model is an easy-to-use prediction classification system for RP-ILD risk in anti-MDA5+DM patients. It has great application prospect in disease management.
1 Background
Anti-melanoma differentiation-associated gene 5 positive (anti-MDA5+) dermatomyositis (DM) is a subtype of DM characterized by distinct cutaneous lesions, little or no muscle involvement, and interstitial lung disease (ILD) (1). ILD is the most important clinical feature of anti-MDA5+DM affecting approximately 60–100% of patients; importantly, over 40% (20–75%) of them tend to develop the rapidly progressive ILD phenotype (RP-ILD), especially in the Asian population (2–5). Anti-MDA5+DM patients with RP-ILD are generally resistant to glucocorticoid and immunosuppressant therapy, leading to a 6-month mortality rate as high as 50-70% (6). Risk stratification to predict patients who will develop fatal RP-ILD at the early stage of the disease is very important for discussing patient expectations and supporting therapy decision-making in anti-MDA5+DM patients.
In the past decade, a great effort has been attempted to identify risk factors that predict ILD progression and mortality in anti-MDA5+DM. Old age, skin ulceration, and lack of myositis are suggested as risk factors for RP-ILD (7). Elevated serum CRP, ferritin level, and Krebs von den Lungen-6 (KL-6) levels have been linked to poor outcomes in RP-ILD patients (8, 9). Most recently, forced vital capacity has been validated as a predictor for the survival of ILD in anti-MDA5+DM (10). However, the accuracy of this single risk factor for prognosis estimation is limited by small size, lack of validation, and disease heterogeneity, hindering them from being genuinely applied in the clinic.
Recently, a clinical prediction model of FLAIR score that combines five clinical items, including ferritin, lactate dehydrogenase, anti-MDA5 antibody, CT imaging score, and RP-ILD, has been developed (11). The FLAIR risk score model could help to predict survival in amyopathic DM with ILD. However, the availability of certain items, such as the HRCT scoring system and anti-MDA5 antibody titers, limited the FLAIR model from being quickly adopted in daily clinical practice (12–14).
The current study aims to establish a simple-to-use risk prediction model for RP-ILD based on easily available clinical variables. Using a discovery cohort and an independent validation cohort, we identified and validated that the CROSS model is a readily available risk classification system for predicting RP-ILD in anti-MDA5+DM patients.
2 Methods
2.1 Study cohort and participants
Our cohort study was registered (ClinicalTrials.gov NCT04747652). Adult patients with DM meeting the European NeuroMusclar Center (ENMC) criteria or Sontheimer criteria were recruited into the cohort after excluding other autoimmune diseases or other causes of ILD (15, 16). Consecutive anti-MDA5+DM collected from ten tertiary hospitals in southern Jiangsu province from March 2019 to March 2021 were included in the discovery cohort. To externally verify the derived prediction model, 85 eligible consecutive anti-MDA5+DM patients were recruited into the validation cohort from eight tertiary hospitals in the northern region of Jiangsu province between March 2019 and March 2021 (Figure 1).
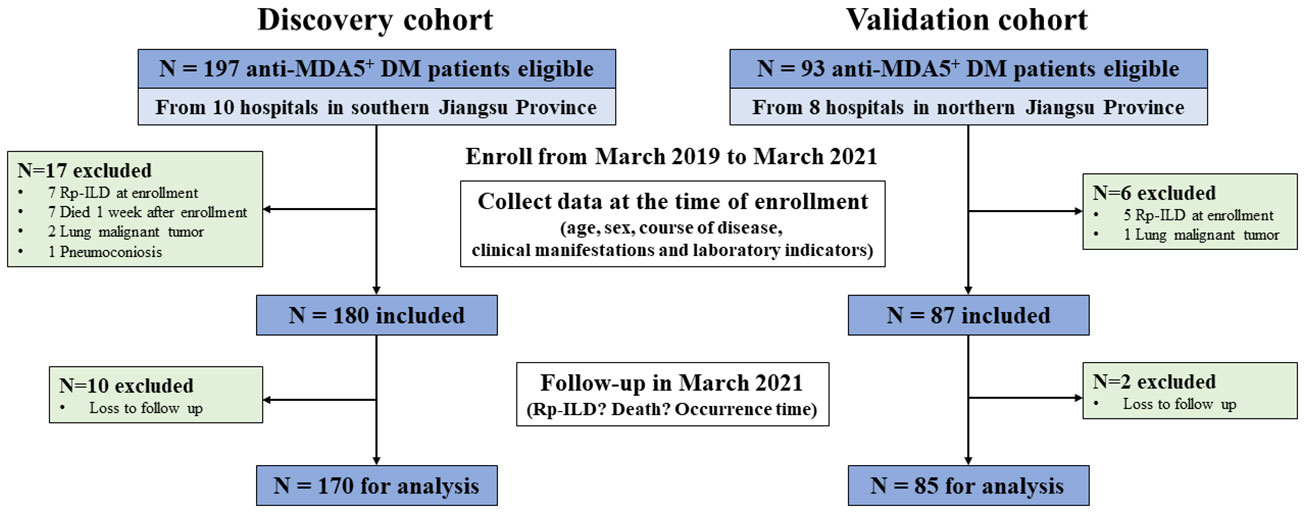
Figure 1 Flow chart of study enrollment. Patients who were diagnosed with dermatomyositis and had positive serum anti-MDA5 antibodies were included in this study. Among them, patients who presented with RP-ILD at enrollment and died within one week of enrollment, as well as those with lung malignant tumor, pneumoconiosis, and loss to follow-up were excluded. Anti-MDA5+DM, anti-melanoma differentiation-associated gene 5 positive dermatomyositis; RP-ILD, rapidly progressive interstitial lung disease.
All clinical data were collected at baseline (initially diagnosed as DM), to death or the last follow-up visit. Clinical parameters of all subjects include the demographic information (including age at onset, gender, disease duration (defined as from disease onset to the cohort enrollment), initial symptoms associated with the disease), clinical manifestation (including muscle weakness, rash, periungual erythema, arthritis, mechanic’s hand, skin ulcer, and interstitial lung disease) and laboratory indicators (including alanine transaminase [ALT], aspartate aminotransferase [AST], lactic dehydrogenase [LDH], creatine kinase [CK], erythrocyte sedimentation rate [ESR], C-reactive protein [CRP], serum ferritin [SF], autoantibodies [including MDA5, ANA and Ro-52]). CT scans were obtained at 1- to 3-month intervals. Two expert thoracic radiologists re-reviewed all available HRCT imaging from baseline to death or the last follow-up visit.
The definition of RP-ILD was according to the descriptions in previous literature with some modifications (17, 18). Briefly, RP-ILD was defined as the presence of any of the following four conditions within one month after the onset of respiratory symptoms: 1) acute and progressive worsening of dyspnea requiring hospitalization or supplementary oxygen; 2) lung function including forced vital capacity (FVC) decreases by more than 10%, or diffusion capacity for carbon monoxide of the Lung (DLCO) falls over 15% with the decreased FVC; 3) high-resolution CT (HRCT) of the chest demonstrates that the extent of interstitial abnormalities increased more than 20%; 4) arterial blood gas analysis suggests respiratory failure or the oxygen partial pressure reduction is more significant than 10mmHg.
2.2 Statistical strategy and modeling
Medical records were reviewed retrospectively to collect clinical, laboratory, and imaging data, and all data were collected using an Electronic Data Capture (EDC) System explicitly designed for the cohort. If a patient developed RP-ILD during follow-up, it will be recorded as an endpoint event for poor outcomes.
In data processing, some continuous variables were transformed into dichotomies. Patients’ median age and disease duration at baseline were used as the cutoff value to distinguish elderly patients and short course of disease. The abnormal thresholds of laboratory indicators used the upper end of their normal reference range. Anti-MDA5 antibodies were tested by immunoblot testing (Euroimmun, Lubeck, Germany) in the same central laboratory for all subjects, and the high titer positivity is defined as +++. ANA is detected using the indirect immunofluorescence assay, and a titre greater than 1:40 is considered positive.
SPSS 23.0, GraphPad Prism 8.4.2, and R version 3.6.3 were used in the statistical analysis. Differences between the different groups (whether an RP-ILD and death endpoint event occurred in the discovery cohort) were calculated with the Mann-Whitney U test and Pearson’s chi-square test in measurement and categorical data, respectively. P < 0.05 was considered statistically significant, and all statistical tests were two-tailed probability tests.
A discovery cohort was used to develop a prediction model of RP-ILD in anti-MDA5+DM patients. All transformed dichotomous variables were tested by univariate Cox analysis to screen whether it is a related factor to the occurrence of RP-ILD. Some continuous variables were dichotomized based on the median, including age, course of the disease (short disease duration is defined as ≤ 3 months after anti-MDA5+DM onset), follow-up time, etc. In contrast, other continuous variables were dichotomized based on the upper limit of 95% confidence interval of clinical tests, including ALT, AST, LDH, CK, ESR, CRP and SF. Univariate and multivariable Cox analyses were then performed to identify the independent risk factors for developing RP-ILD in anti-MDA5+DM patients. Variables with p < 0.2 in the univariate analysis were included in the multivariable Cox analysis as the covariates. To identify independent prognostic risk factors and calculate their weightiness, β regression coefficient and integer estimation were used to form an integral model for predicting the occurrence of RP-ILD.
Time-dependent receiver operating characteristic (ROC) curve analysis was used to determine whether the two score models were the optimal clinical significance threshold. Kaplan-Meier method was used to calculate the cumulative poor prognosis rates during follow-up, and the logarithmic rank test was used to compare different risk groups. Finally, the incidence of RP-ILD and mortality at 3 months, 6 months, and 1 year in anti-MDA5+DM patients with varying levels of risk was accurately measured and demonstrated. The above verification methods are carried out in the external validation cohort to verify the prediction models’ reliability further.
3 Results
3.1 Baseline characteristics of anti-MDA5+DM patients in the discovery and validation cohort
Table 1 shows the baseline characteristics of the discovery and validation cohorts. Patients in the discovery and validation cohorts do not show marked differences at baseline. As one group, anti-MDA5+DM patients are predominantly women (69.8%) with a median age of 53.0 (47.0-63.0) years old. The median follow-up time is 10.0 (3.0-14.0) and 12.0 (3.0-14.0) months in the discovery and validation cohorts, respectively. The overall incidence of RP-ILD in anti-MDA5+DM patients was 36.86% (94/255), and the mortality was 24.71% (63/255).
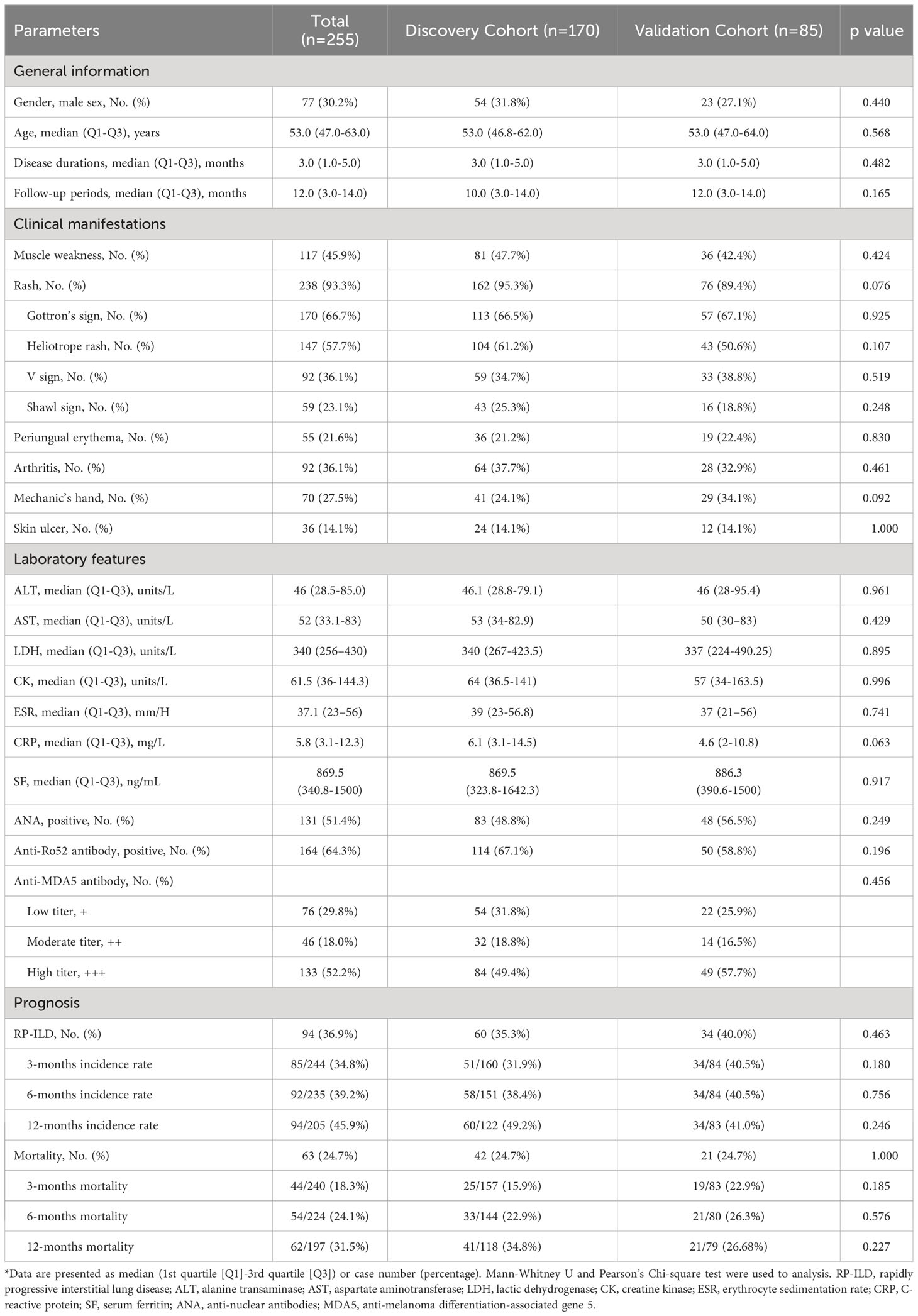
Table 1 Comparison of clinical manifestations and laboratory features between discovery and validation cohorts at baseline.
3.2 Development of the CROSS model to predict the risk of RP-ILD
After univariate Cox analysis, variables with p < 0.2 were included in the multivariable Cox analysis as the covariates, including male sex, short disease duration, abnormal laboratory parameters (including AST, LDH, CK, CRP, and SF), anti-Ro52 antibody positivity and high titer positivity of anti-MDA5 antibody defined as +++.
After COX regression, four risk factors were finally determined as the independent risk factors for the development of RP-ILD, including CRP abnormal (defined as exceed the upper limit of the normal detection range), anti-Ro52 antibody positivity, male sex, and short disease duration (Figure 2). We then selected these four independent risk factors to create a new RP-ILD risk prediction model of the CROSS score (CRP abnormal, anti-Ro52 antibody, sex [male sex], and short disease duration). These four variables were weighted according to the ratio of the β coefficient. When calculating the CROSS score, anti-Ro52 positivity and short disease duration scored 2 points, respectively, and abnormal CRP and male sex scored one point. The Alignment Diagram also shows the weighted relationship between these four predictors and the occurrence of RP-ILD, and the concordance index reached 0.825 (Supplementary Figure 1).
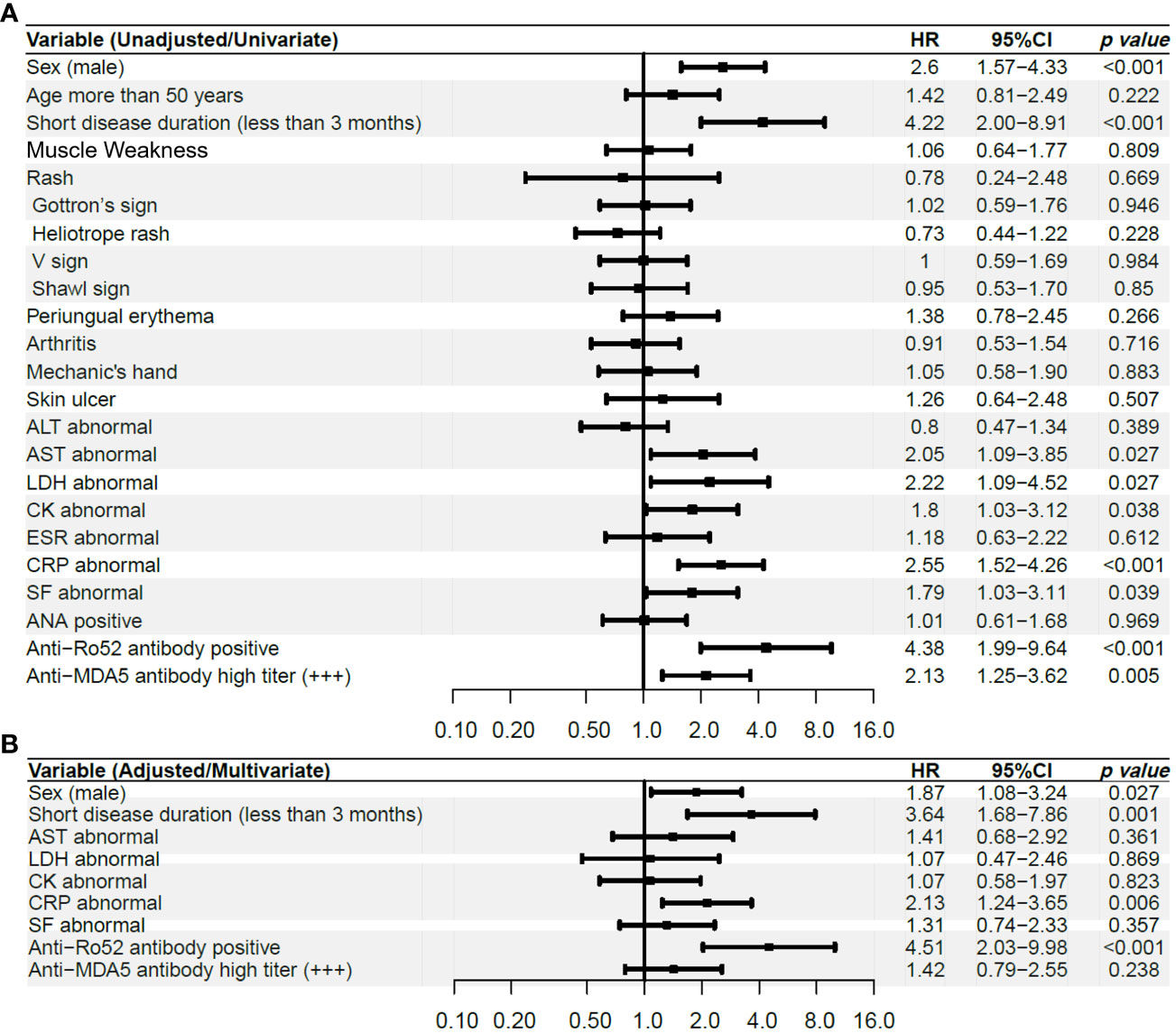
Figure 2 Univariate and Multivariate COX analysis of RP-ILD influenced by baseline manifestations in the discovery cohort (A) Univariate COX analysis, (B) Multivariate COX analysis. ALT, alanine transaminase; AST, aspartate aminotransferase; LDH, lactic dehydrogenase; CK= creatine kinase; ESR, erythrocyte sedimentation rate; CRP, C-reactive protein; SF, serum ferritin; ANA, anti-nuclear antibodies; MDA5, anti-melanoma differentiation-associated gene 5; RP-ILD, rapidly progressive interstitial lung disease; HR, Hazard ratio.
Based on the CROSS score with a range from 0 to 6, anti-MDA5+DM patients were classified as moderate risk (CROSS score = 0-2), high risk (CROSS score = 3-4), and very high risk (CROSS score = 5-6) for developing of RP-ILD, respectively (Table 2).
3.3 Efficiency validation of the CROSS model
To verify the accuracy of the predictive model, the CROSS score was calculated in both cohorts as the internal validation and external validation datasets, respectively. Compared with patients without RP-ILD, the CROSS score at baseline was significantly higher in anti-MDA5+DM patients with RP-ILD in both cohorts (Supplementary Figure 2). Then, time-dependent ROC curves were performed to evaluate the forecast effects of the CROSS model. The area under the curve (AUC) of the CROSS model in both cohorts are more than 0.8 at each time point within one year, which indicates excellent differentiation efficiency of the CROSS model (Supplementary Figure 3).
Next, Kaplan-Meier analysis was used to assess whether the percentage of adverse events in patients at different risk levels differed significantly over time. The proportion of anti-MDA5+DM patients developing RP-ILD over time increases considerably as the risk level assessed based on the CROSS model increases in both cohorts (p< 0.0001). In the discovery cohort, the 1-year non-RP-ILD survival rate of the moderate-, high-, and very high-risk groups were 90.46%, 64.81%, and 28.7%, respectively (Figure 3A). In the validation cohort, the 1-year non-RP-ILD survival rate of the moderate-, high-, and very high-risk groups were 93.33%, 58.31%, and 14.29%, respectively (p< 0.0001) (Figure 3B). At the same time, we further analyzed the time-dependent mortality of these three groups of anti-MDA5+DM patients. The prognostic grouping based on the CROSS model also has an excellent predictive effect on the risk of death (p=0.0003 in the discovery cohort and p< 0.0001 in the validation cohort). The one-year survival rates of very high-risk patients were 54.28% and 30.86%, significantly lower than those in moderate- and high-risk patients (Figures 3C, D).
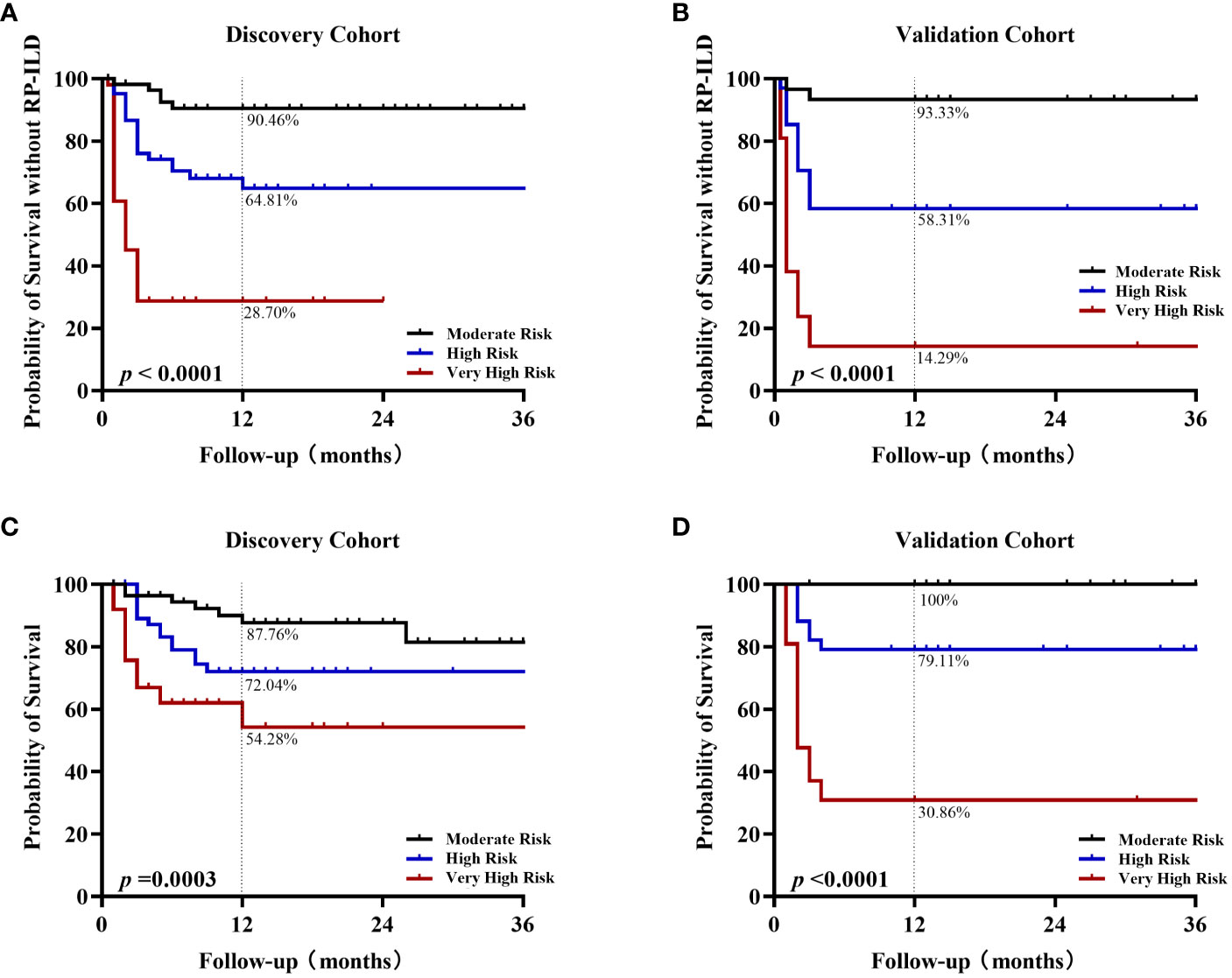
Figure 3 Kaplan-Meier analysis of different risk grades in survival rate and RP-ILD-free survival rate Kaplan-Meier non-RPILD survival curves for the different risk stratification groups according to the CROSS model in the discovery cohort (A) or validation cohort (B). Kaplan-Meier survival curves in the discovery cohort (C) or validation cohort (D).
3.4 Incidence rate of poor prognosis in various risk grades evaluated by the models
At last, we calculated the rates of RP-ILD in anti-MDA5+DM patients with moderate, high, and very high-risk stratification at different time points. As the risk level rises, the incidence rate of RP-ILD based on the CROSS model was gradually raised in both cohorts. Of note, the incidence rate of RP-ILD in very high-risk patients is over 70%, high-risk patients are around 25%-50%, and less than 15% in moderate-risk patients (Figure 4).
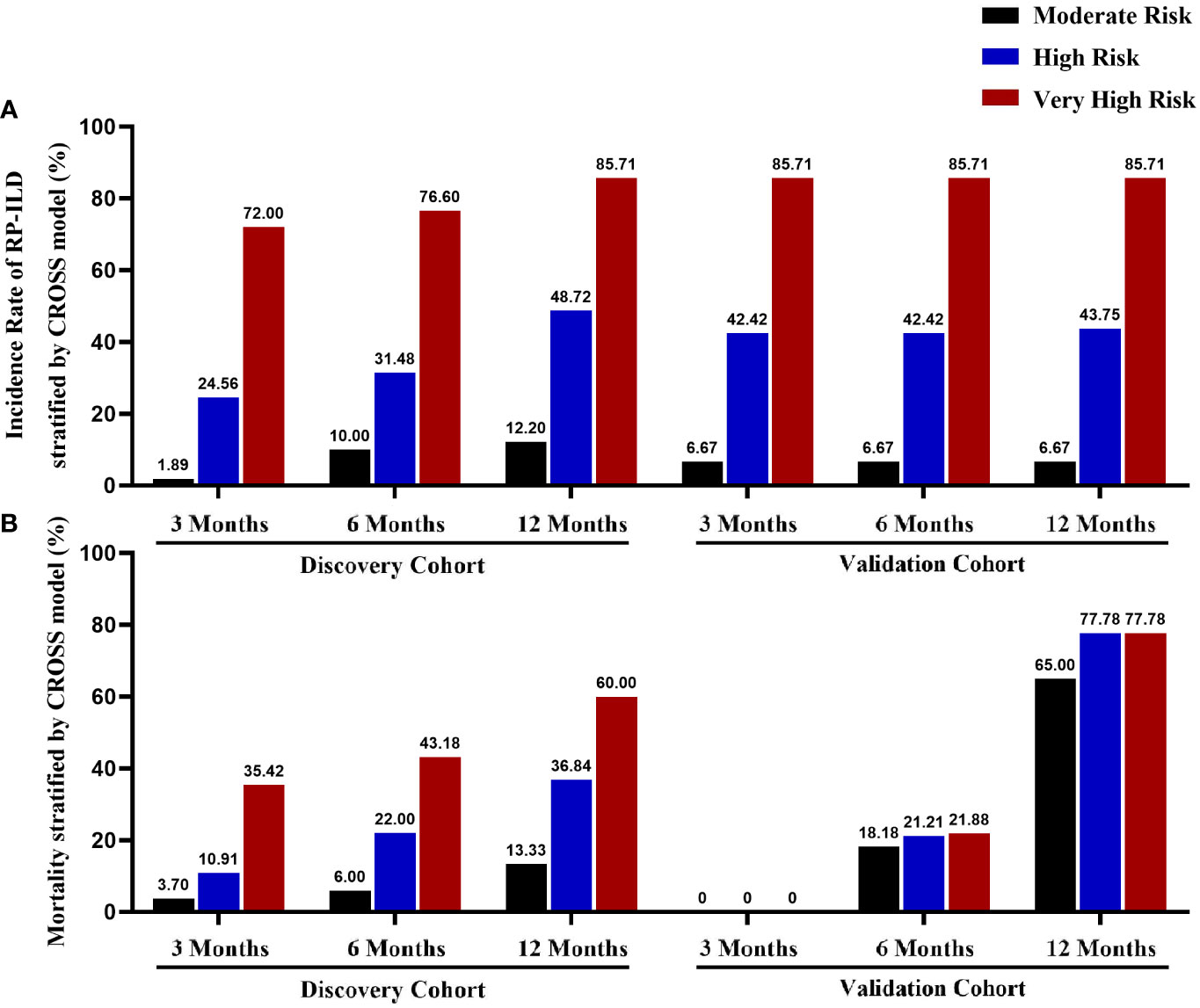
Figure 4 The incidence rate of RP-ILD and mortality according to the CROSS model Comparison of 3-months, 6-months, and 1-year incidence rates of RP-ILD (A) and mortality (B) in the discovery and validation cohorts with different risk stratification.
4 Discussion
RP-ILD is a common and potentially fatal complication of anti-MDA5+DM. Risk stratification to predict patients who will develop fatal RP-ILD at the early stage of the disease is very important for discussing patient expectations and supporting therapy decision-making in anti-MDA5+DM patients. Based on the current largest reported anti-MDA5+DM cohort containing 255 consecutive anti-MDA5+DM patients, we developed and validated a CROSS model that successfully predicts RP-ILD and mortality risk.
There are three essential differences between the FLAIR model (11) mentioned in introduction and our own. First, all patients included in our cohort are anti-MDA5+DM patients. Second, unlike the FLAIR model for predicting death risk, the CROSS model represents the first prognostic tool for RP-ILD in anti-MDA5+DM patients. RP-ILD is linked to high mortality. Given that only about 1/3 of anti-MDA5+DM may develop RP-ILD after disease onset in our cohort and the previous report, early recognition of patients with high RP-ILD risk is particularly important in halting disease progression and improving prognosis in anti-MDA5+DM. Third, the inconvenience of obtaining HRCT scoring and anti-MDA5 titers data limits the FLAIR model’s widespread adoption in daily clinical practice (12–14). To overcome these limitations, we developed a simpler prognostic model.
We compared 26 clinical or laboratory parameters from 255 patients (94 RP-ILD and 161 non-RP-ILD; 192 survivors and 63 non-survivors). Amongst all parameters investigated, we found four parameters to be independent risk factors for RP-ILD in the discovery cohort. We then developed and externally validated a CROSS (CRP, anti-Ro52 antibody, short disease duration, Sex[male sex]) for predicting RP-ILD risk in two different anti-MDA5+DM cohorts. The CROSS model is an easy-to-use risk prediction system based on commonly used and easily obtained variables in the clinical setting. In both discovery and validation cohorts, very high-risk patients had significantly higher RP-ILD rates than moderate- and high-risk patients. Interestingly, the risk of death in anti-MDA5+DM patients was also well stratified based on the predictive model (Figures 3C, D). However, there was no significant difference in overall mortality among the three groups based on the CROSS model in the validation cohort (Figure 4B).
According to the CROSS model, 51 patients (30.0%) were classified as at very high risk of developing RP-ILD in the discovery cohort, and 76.6% of them eventually developed RP-ILD in the first 6 months. Similar accuracy was confirmed in the validation cohort and achieved 85.7%. Stratifying anti-MDA5+DM into different risk categories allows for closer monitoring of very high-risk patients and guiding management decisions.
In our cohort, 34.84% of anti-MDA5+DM patients will develop RP-ILD during the first 3 months after disease onset. Actually we find significant, time- time-dependent changes in RP-ILD and mortality risk in MDA5+ DM patients in our cohort. More than 90% RP-ILD and 84% mortality occurs in the first 6-months after disease onset. Notably, the first 3-months is a particularly high-risk period, with 50% RP-ILD and 46% death occurring. We proposed the first 6-months, especially the first 3-months, is a risk window for the poor outcome in anti-MDA5+ DM patients (19). We recommend calculating the CROSS score initially after the patient is diagnosed with anti-MDA5+DM. Then, the CROSS risk classification system can be used sequentially, particularly in the first 3~6 months.
Among the variables in the CROSS model, the increased CRP levels imply high disease activity and hyperinflammatory state in anti-MDA5+DM. We previously identified 3 distinct phenotypes with significantly different prognoses in patients with anti-MDA5+DM. The most prominent feature in anti-MDA5+DM with RP-ILD is the high inflammatory status (20), supporting CRP played an important role in the CROSS score. CRP is a dynamic index. Besides its association with an inflammatory state, CRP levels are also linked to potential infection, which might warn differential diagnosis during disease progression. Based on the requirement of simplification of the clinical model, all quantitative parameters were qualified based on whether they were abnormal, which may even weaken the impact of CRP in predicting the prognosis of anti-MDA5+DM patients.
In our CROSS model, anti-Ro52 is a strong prognostic factor for RP-ILD. Anti-Ro52 is the most common autoantibody detected in polymyositis and the anti-synthetase syndrome. Previous studies have reported that anti-Ro52 antibodies significantly correlated with ILD in DM, juvenile myositis, primary Sjögren syndrome, and connective tissue diseases, suggesting anti-Ro52 is an intended risk factor for ILD (21–25). Consistent with our findings, recent studies have found that the coexistence of anti-MDA5 and anti-Ro52 correlates with an increased frequency of RP-ILD and poor prognosis in anti-MDA5 DM patients (25). Thus, the presence of anti-Ro52 might help to distinguish a subgroup of anti-MDA5+DM patients with more aggressive phenotypes.
There are limitations to this study. First, all data are obtained from a multicenter retrospective study, and missing data could not be avoided, which might be a bias of the analysis. Second, FVC, DLCO values, and hypoxemia have been reported as risk factors for RP-ILD and poor prognosis in patients with anti-MDA5+DM (7). Approximately36.9% of patients develop RP-ILD during the follow-up period in our cohort, and most patients have no markedly respiratory symptoms at the beginning of disease onset. Our goal is to create an easy-to-use prediction model that could be applied at the initial visit of a patient with anti-MDA5 DM. Therefore, we did not include lung function and arterial blood gas in our risk classification system. Third, the treatment regimen was not analyzed in the current study, especially lacking the relationship between changes in CROSS score over time with treatment response. Fourth, the worldwide coronavirus disease 2019 (COVID-19) pandemic indicated a similar cytokine storm in anti-MDA5+DM with RP-ILD. CROSS score did not take into account blood cytokine profiles with clinical outcomes. Given that the development and validation of the CROSS model were conducted with patients from various hospitals in Jiangsu Province, China, its applicability to populations in other regions or ethnic groups beyond Asians has yet to be established. This necessitates additional validation to confirm its effectiveness in diverse settings. Despite its limitations, based on the most significant reported study populations, our CROSS score provides a simple and accurate model for predicting RP-ILD onset and mortality risk in anti-MDA5+DM patients. Further prospective studies are needed to validate its accuracy in risk prediction further, facilitating the truly clinical decision-making support in anti-MDA5+DM patients.
Recently, Jacqueline So et al. also revealed a FLAW model that based on fever, LDH, age, and white cell count maybe useful to predict the risk of RP-ILD in anti-MDA5+DM patients (26). Similar to our CROSS model, FLAW provide a simple pragmatic model for predicting RP-ILD. Although the variables are different, both these two models showed good predictive effects. Future prospective studies are needed to compare the clinical application value of these two models. Using the largest reported cohort, we developed and validated a prediction model for RP-ILD risk in anti-MDA5+DM patients. The strength of this model is the use of clinical variables that could be easily obtained during the routine clinic visit. This simple predictive model could aid in the early detection of anti-MDA5+DM patients without RP-ILD at poor prognosis risk, guiding treatment and improving outcomes.
5 Conclusions
The CROSS model could help to identify anti-MDA5+DM patient who are at high risk of RP-ILD. It provides a simple and easy-used mothed to early warning and early detection of anti-MDA5+DM patients with poor outcomes in in daily clinical practice.
Data availability statement
The raw data supporting the conclusions of this article will be made available by the authors, without undue reservation.
Ethics statement
The studies involving humans were approved by the ethical committee of the First Affiliated Hospital of Nanjing Medical University: 2020-SR-265. The studies were conducted in accordance with the local legislation and institutional requirements. The participants provided their written informed consent to participate in this study. Written informed consent was obtained from the individual(s) for the publication of any potentially identifiable images or data included in this article.
Author contributions
LW: Conceptualization, Data curation, Investigation, Writing – original draft, Formal Analysis, Methodology, Project administration, Software, Visualization. CL: Conceptualization, Supervision, Validation, Writing – review & editing. HY: Writing – review & editing, Data curation, Supervision, Validation. LX: Data curation, Writing – review & editing, Supervision, Validation. FY: Writing – review & editing, Investigation. JLi: Investigation, Writing – review & editing. MW: Investigation, Writing – review & editing. SZ: Investigation, Writing – review & editing. ZD: Investigation, Writing – review & editing. JQ: Investigation, Writing – review & editing. HW: Investigation, Writing – review & editing. WY: Investigation, Writing – review & editing. LZ: Investigation, Writing – review & editing. YW: Investigation, Writing – review & editing. SY: Investigation, Writing – review & editing. DZ: Investigation, Writing – review & editing. JW: Investigation, Writing – review & editing. YL: Investigation, Writing – review & editing. DS: Investigation, Writing – review & editing. ZL: Investigation, Writing – review & editing. LL: Investigation, Writing – review & editing. LM: Investigation, Writing – review & editing. XX: Investigation, Writing – review & editing. YZ: Investigation, Writing – review & editing. HL: Investigation, Writing – review & editing. TR: Investigation, Writing – review & editing. JLiu: Writing – review & editing, Data curation. FW: Writing – review & editing, Funding acquisition. MZ: Writing – review & editing, Data curation. WT: Data curation, Conceptualization, Funding acquisition, Investigation, Supervision, Writing – original draft.
Funding
The author(s) declare financial support was received for the research, authorship, and/or publication of this article. This work was supported by the National Natural Science Foundation of China (NSFC) [grant numbers: 81971532, 81971533].
Acknowledgments
We thank all authors for their continuous and excellent support with patient data collection, data analysis, statistical analysis, and valuable suggestions for the article.
Conflict of interest
The authors declare that the research was conducted in the absence of any commercial or financial relationships that could be construed as a potential conflict of interest.
Publisher’s note
All claims expressed in this article are solely those of the authors and do not necessarily represent those of their affiliated organizations, or those of the publisher, the editors and the reviewers. Any product that may be evaluated in this article, or claim that may be made by its manufacturer, is not guaranteed or endorsed by the publisher.
Supplementary material
The Supplementary Material for this article can be found online at: https://www.frontiersin.org/articles/10.3389/fimmu.2024.1286973/full#supplementary-material
Supplementary Figure 1 | A nomogram predicting RP-ILD risk of anti-MDA5+DM patients Each variable’s value was given a score on the point scale axis. A total score could be calculated by adding every single score, and by projecting the complete score to the lower full-point scale, we could estimate the probability of RP-ILD.
Supplementary Figure 2 | Differences in CROSS scores in anti-MDA5+DM patients The violin diagram shows the difference in CROSS scores between patients who progressed to RP-ILD and those who did not in both discovery (A) and validation cohort (B).
Supplementary Figure 3 | AUC value of time-dependent ROC curve in CROSS model The area under the curve (AUC) value of the time-dependent ROC curve shows that the CROSS model has excellent differentiation efficiency in both cohorts within different time points.
Abbreviations
MDA5, Melanoma Differentiation-associated Gene 5; DM, Dermatomyositis; RP-ILD, Rapidly Progressive Interstitial Lung Disease; CRP, C-reactive Protein; KL-6, Krebs von den Lungen-6; ENMC, European NeuroMusclar Center; ALT, Alanine Transaminase; AST, Aspartate Aminotransferase; LDH, Lactic Dehydrogenase; CK, Creatine Kinase; ESR, Erythrocyte Sedimentation Rate; SF, Serum Ferritin; ANA, Anti-nuclear Antibodies; FVC, Forced Vital Capacity; DLCO, Diffusion Capacity for Carbon Monoxide of the Lung; HRCT, High-resolution CT; EDC, Electronic Data Capture; ROC, Receiver Operating Characteristic; AUC, The Area Under the Curve; COVID-19, Coronavirus Disease 2019; HR, Hazard Ratio.
References
1. Kurtzman DJB, Vleugels RA. Anti-melanoma differentiation-associated gene 5 (MDA5) dermatomyositis: A concise review with an emphasis on distinctive clinical features. J Am Acad Dermatol (2018) 78(4):776–85. doi: 10.1016/j.jaad.2017.12.010
2. Koga T, Fujikawa K, Horai Y, Okada A, Kawashiri SY, Iwamoto N, et al. The diagnostic utility of anti-melanoma differentiation-associated gene 5 antibody testing for predicting the prognosis of Japanese patients with DM. Rheumatol (Oxford) (2012) 51(7):1278–84. doi: 10.1093/rheumatology/ker518
3. Moghadam-Kia S, Oddis CV, Sato S, Kuwana M, Aggarwal R. Antimelanoma differentiation-associated gene 5 antibody: expanding the clinical spectrum in north american patients with dermatomyositis. J Rheumatol (2017) 44(3):319–25. doi: 10.3899/jrheum.160682
4. Allenbach Y, Uzunhan Y, Toquet S, Leroux G, Gallay L, Marquet A, et al. Different phenotypes in dermatomyositis associated with anti-MDA5 antibody: Study of 121 cases. Neurology (2020) 95(1):e70–8. doi: 10.1212/WNL.0000000000009727
5. Fujimoto M, Watanabe R, Ishitsuka Y, Okiyama N. Recent advances in dermatomyositis-specific autoantibodies. Curr Opin Rheumatol (2016) 28(6):636–44. doi: 10.1097/BOR.0000000000000329
6. Wu W, Guo L, Fu Y, Wang K, Zhang D, Xu W, et al. Interstitial lung disease in anti-MDA5 positive dermatomyositis. Clin Rev Allergy Immunol (2021) 60(2):293–304. doi: 10.1007/s12016-020-08822-5
7. Motegi SI, Sekiguchi A, Toki S, Kishi C, Endo Y, Yasuda M, et al. Clinical features and poor prognostic factors of anti-melanoma differentiation-associated gene 5 antibody-positive dermatomyositis with rapid progressive interstitial lung disease. Eur J Dermatol (2019) 29(5):511–7. doi: 10.1684/ejd.2019.3634
8. Gono T, Masui K, Nishina N, Kawaguchi Y, Kawakami A, Ikeda K, et al. Risk prediction modeling based on a combination of initial serum biomarker levels in polymyositis/dermatomyositis-associated interstitial lung disease. Arthritis Rheumatol (2021) 73(4):677–86. doi: 10.1002/art.41566
9. Zhu Y, Wang L, Sun Y, Wang J, Lv C, You H, et al. Serum Krebs von den Lungen-6 concentrations reflect severity of anti-melanoma differentiation-associated protein 5 antibody positive dermatomyositis associated interstitial lung disease. Clin Exp Rheumatol (2022) 40(2):292–7. doi: 10.55563/clinexprheumatol/zmn18h
10. Wu W, Xu W, Sun W, Zhang D, Zhao J, Luo Q, et al. Forced vital capacity predicts the survival of interstitial lung disease in anti-MDA5 positive dermatomyositis: a multi-centre cohort study. Rheumatology (2022) 61(1):230–9.
11. Lian X, Zou J, Guo Q, Chen S, Lu L, Wang R, et al. Mortality risk prediction in amyopathic dermatomyositis associated with interstitial lung disease: the FLAIR model. Chest (2020) 158(4):1535–45. doi: 10.1016/j.chest.2020.04.057
12. Baker MC, Chung L, Fiorentino DF. A mortality risk score model for clinically amyopathic dermatomyositis-associated interstitial lung disease: will it have the necessary "FLAIR" to improve clinical outcomes? Chest (2020) 158(4):1307–9. doi: 10.1016/j.chest.2020.06.001
13. Waki D, Nishimura K. Impact of the combined immunosuppressive therapy on different mortality score groups in amyopathic dermatomyositis-associated interstitial lung disease and the problem of sample splitting. Chest (2021) 159(2):882–3. doi: 10.1016/j.chest.2020.07.099
14. Wang M, Fan C. Mortality risk prediction in amyopathic dermatomyositis associated with interstitial lung disease: perhaps some potential details to consider. Chest (2021) 159(4):1686–7. doi: 10.1016/j.chest.2020.10.096
15. Hoogendijk JE, Amato AA, Lecky BR, Choy EH, Lundberg IE, Rose MR, et al. 119th ENMC international workshop: trial design in adult idiopathic inflammatory myopathies, with the exception of inclusion body myositis, 10-12 October 2003, Naarden, The Netherlands. Neuromuscul Disord (2004) 14(5):337–45. doi: 10.1016/j.nmd.2004.02.006
16. Euwer R, Sontheimer R. Dermatologic aspects of myositis. Curr Opin Rheumatol (1994) 6(6):583–9. doi: 10.1097/00002281-199411000-00006
17. Sato S, Hirakata M, Kuwana M, Suwa A, Inada S, Mimori T, et al. Autoantibodies to a 140-kd polypeptide, CADM-140, in Japanese patients with clinically amyopathic dermatomyositis. Arthritis Rheum (2005) 52(5):1571–6. doi: 10.1002/art.21023
18. Abe Y, Kusaoi M, Tada K, Yamaji K, Tamura N. Successful treatment of anti-MDA5 antibody-positive refractory interstitial lung disease with plasma exchange therapy. Rheumatol (Oxford) (2020) 59(4):767–71. doi: 10.1093/rheumatology/kez357
19. You H, Wang L, Wang J, Lv C, Xu L, Yuan F, et al. Time-dependent changes in RPILD and mortality risk in anti-MDA5+ DM patients: a cohort study of 272 cases in China. Rheumatol (Oxford) (2023) 62(3):1216–26. doi: 10.1093/rheumatology/keac450
20. Xu L, You H, Wang L, Lv C, Yuan F, Li J, et al. Identification of three different phenotypes in anti-MDA5 antibody-positive dermatomyositis patients: implications for rapidly progressive interstitial lung disease prediction. Arthritis Rheumatol (2022).
21. Xing X, Li A, Li C. Anti-Ro52 antibody is an independent risk factor for interstitial lung disease in dermatomyositis. Respir Med (2020) 172:106134.
22. Vojinovic T, Cavazzana I, Ceruti P, Fredi M, Modina D, Berlendis M, et al. Predictive features and clinical presentation of interstitial lung disease in inflammatory myositis. Clin Rev Allergy Immunol (2021) 60(1):87–94.
23. Sabbagh S, Pinal-Fernandez I, Kishi T, Targoff IN, Miller FW, Rider LG, et al. Anti-Ro52 autoantibodies are associated with interstitial lung disease and more severe disease in patients with juvenile myositis. Ann Rheum Dis (2019) 78(7):988–95.
24. Buvry C, Cassagnes L, Tekath M, Artigues M, Pereira B, Rieu V, et al. Anti-Ro52 antibodies are a risk factor for interstitial lung disease in primary Sjogren syndrome. Respir Med (2020) 163:105895.
25. Xu A, Ye Y, Fu Q, Lian X, Chen S, Guo Q, et al. Prognostic values of anti-Ro52 antibodies in anti-MDA5-positive clinically amyopathic dermatomyositis associated with interstitial lung disease. Rheumatol (Oxford) (2021) 60(7):3343–51.
26. So J, So H, Wong V, Ho R, Wu T, Wong P, et al. Predictors of rapidly progressive interstitial lung disease and mortality in patients with autoantibodies against melanoma differentiation-associated protein 5 dermatomyositis. Rheumatol (Oxford) (2022) 61(11):4437–44. doi: 10.1093/rheumatology/keac094
Keywords: anti-melanoma differentiation-associated gene 5, dermatomyositis, rapidly progressive interstitial lung disease, predict models, easy-to-use
Citation: Wang L, Lv C, You H, Xu L, Yuan F, Li J, Wu M, Zhou S, Da Z, Qian J, Wei H, Yan W, Zhou L, Wang Y, Yin S, Zhou D, Wu J, Lu Y, Su D, Liu Z, Liu L, Ma L, Xu X, Zang Y, Liu H, Ren T, Liu J, Wang F, Zhang M and Tan W (2024) Rapidly progressive interstitial lung disease risk prediction in anti-MDA5 positive dermatomyositis: the CROSS model. Front. Immunol. 15:1286973. doi: 10.3389/fimmu.2024.1286973
Received: 01 September 2023; Accepted: 19 January 2024;
Published: 01 February 2024.
Edited by:
Francesca Wanda Rossi, University of Naples Federico II, ItalyReviewed by:
Zhengping Huang, Guangdong Second Provincial General Hospital, ChinaJosé Jiram Torres-Ruiz, National Institute of Medical Sciences and Nutrition Salvador Zubirán, Mexico
Frédéric Coutant, Université de Lyon, France
Copyright © 2024 Wang, Lv, You, Xu, Yuan, Li, Wu, Zhou, Da, Qian, Wei, Yan, Zhou, Wang, Yin, Zhou, Wu, Lu, Su, Liu, Liu, Ma, Xu, Zang, Liu, Ren, Liu, Wang, Zhang and Tan. This is an open-access article distributed under the terms of the Creative Commons Attribution License (CC BY). The use, distribution or reproduction in other forums is permitted, provided the original author(s) and the copyright owner(s) are credited and that the original publication in this journal is cited, in accordance with accepted academic practice. No use, distribution or reproduction is permitted which does not comply with these terms.
*Correspondence: Wenfeng Tan, dGFud2VuZmVuZzIwMDVAMTI2LmNvbQ==