- 1Department of Breast Surgery, Fujian Medical University Union Hospital, Fuzhou, Fujian, China
- 2Department of General Surgery, Fujian Medical University Union Hospital, Fuzhou, Fujian, China
- 3Breast Cancer Institute, Fujian Medical University, Fuzhou, Fujian, China
- 4Administration Department of Nosocomial Infection, Fujian Medical University Union Hospital, Fuzhou, Fujian, China
- 5Department of Pathology, Fujian Medical University Union Hospital, Fuzhou, Fujian, China
Introduction: The programmed cell death (PCD) pathway plays an important role in restricting cancer cell survival and proliferation. However, limited studies have investigated the association between genetic variants in the 3′-untranslated region of the PCD pathway genes and breast cancer outcomes.
Methods: In this study, we genotyped 28 potentially functional single nucleotide polymorphisms (SNPs) in 23 PCD pathway genes in 1,177 patients with early-stage breast cancer (EBC) from a Han Chinese population. The median follow-up period was 174 months.
Results: Among all the candidate SNPs, four independent SNPs (rs4900321 and rs7150025 in ATG2B, rs6753785 in BCL2L11, and rs2213181 in c-Kit) were associated with invasive disease-free survival (iDFS), distant disease-free survival (DDFS), breast cancer-specific survival (BCSS) and overall survival (OS), respectively. Further combined genotypes of these four SNPs revealed that the survival decreased as the number of unfavorable genotypes increased (Ptrend = 1.0 × 10−6, 8.5 × 10−8, 3.6 × 10−4, and 1.3 × 10−4 for iDFS, DDFS, BCSS, and OS, respectively). Receiver operating characteristic curve analysis demonstrated that incorporating unfavorable genotypes and clinicopathological variables improved the ability to predict EBC survival (P = 0.006, 0.004, 0.029, and 0.019 for iDFS, DDFS, BCSS, and OS, respectively). Additionally, rs6753785 and rs2213181 were associated with BCL2L11 and c-Kit mRNA expression, respectively.
Conclusions: Our results suggest that these four SNPs may act as novel biomarkers for EBC survival, possibly by modulating the expression of the corresponding genes.
Introduction
Breast cancer is one of the major causes of cancer-related deaths among women in China and many other countries worldwide (1, 2). Notably, this disease is fundamentally and clinically heterogeneous, with variable survival outcomes. Although most patients with early-stage breast cancer are curable with current treatments, approximately 34% of patients who receive neoadjuvant or adjuvant chemotherapy die of breast cancer within 15 years (3). Biomarkers are required to predict which patients are at high risk of recurrence or metastasis. Moreover, inherited genetic variations may play a role in the prognosis of breast cancer, which has been verified by many candidate genes and genome-wide association studies (4–7).
MicroRNAs (miRNAs) are small, single-stranded, non-coding RNAs that modulate gene expression by binding to partially complementary sequences in the 3′-untranslated regions (3′-UTRs) of target mRNAs (8). Single nucleotide polymorphisms (SNPs), the most common genetic variations in the human genome, occur once every 100–300 base pairs. Recently, an increasing number of studies have demonstrated that SNPs within miRNA-binding regions contribute to cancer risk and outcomes by altering miRNA–mRNA binding affinities and miRNA-targeted gene expression (9–11).
Programmed cell death (PCD) is defined as regulated cell death executed by an intracellular program that includes several classic modalities, namely, apoptosis, autophagy, and programmed necrosis (12). Over the past decade, mounting evidence has suggested that these three types of programmed cell death are involved in cancer initiation and progression, making them promising pharmacological targets (12–14). miRNAs play important roles in regulating the PCD pathway and cancer progression (15, 16). However, few studies have investigated the association between the SNPs in the 3′-UTR of PCD-related genes and the susceptibility and prognosis of cancer (17–20).
Because of the requirements for sequence complementarity and stable thermodynamics around the miRNA–mRNA binding site, sequence variations within these regions are regarded as strong candidates for functional SNPs. Here, we hypothesized that genetic variations in miRNA–mRNA binding sites contribute to breast cancer recurrence and death. To test this hypothesis, we analyzed 28 SNPs in 3′-UTR of several key genes involved in PCD pathways with breast cancer prognosis in a large Han Chinese breast cancer cohort from Fuzhou, China.
Materials and methods
Study population
The patients were recruited from Fujian Medical University Union Hospital, Fujian, China, between July 2000 and October 2014. All 1,177 participants were Han Chinese women from Southeast China. All patients were diagnosed with early-stage (I–III) breast cancer and underwent curative surgical resection. Adjuvant treatments were chosen based on the surgical approach, patient’s menopausal status, and disease stage, in accordance with the relevant guidelines. Demographic and clinicopathological data were obtained from medical records. The estrogen receptor (ER), progesterone receptor (PR), and human epidermal growth factor-2 (HER2) statuses of each patient were evaluated using immunohistochemistry (IHC). All patients were followed up by personal or family contacts from the time of enrollment until death or the last follow-up (December 2016). The median follow-up period was 174 months. This study was approved by the Ethics Committee of the Fujian Medical University Union Hospital. All the participants provided written informed consent for inclusion in the study.
SNP selection
Candidate genes of the PCD pathway were selected from the KEGG (http://www.genome.jp/kegg/) and miRDeathDB (http://rna-world.org/mirdeathdb/) databases. The 3′-UTR of candidate genes were selected from the UCSC genome browser (http://genome.ucsc.edu/). Furthermore, 28 SNPs in the 3′-UTR of PCD-related genes were extracted from miRBase (http://www.mirbase.org/), miRanda (http://www.microrna.org/), and TargetScans (http://www.targetscan.org/) with three filtering criteria: (1) minor allele frequency (MAF) must be ≥0.10 in the Han Chinese population in Beijing (CHB) from 1000 Genomes Project; (2) pairwise linkage disequilibrium between the eligible SNPs calculated by Haploview 4.1 software must be <0.8 (r2 < 0.8); and (3) SNPs were scored on the basis of the ΔΔG values, and only those that caused a change in the |ΔΔGtot| ≥ 2 kcal/mol (upper tertile) were considered biologically relevant and included in the study (21, 22). Information on eligible SNPs is presented in Supplementary Table S1.
Genotyping
Blood samples were collected in tubes with ethylenediaminetetraacetic acid (EDTA) anticoagulant and stored at −80°C. Genomic DNA was extracted from EDTA anti-coagulated whole blood using a Whole-Blood DNA Extraction Kit (Bioteke, Beijing, China) according to the manufacturer’s protocol. DNA quality was assessed by agarose gel electrophoresis. Genotype analysis was performed using SNPScan, a high-throughput SNP genotyping tool (Genesky Biotechnologies Inc., Shanghai, China). Finally, raw data were analyzed using GeneMapper software (version 4.0; Applied Biosystems, Foster City, CA, USA). Five percent of the samples were randomly selected as blinded duplicates for quality assessment, and 100% concordance was obtained.
Bioinformatics analysis
Expression quantitative trait locus (eQTL) analysis was performed for different tissue samples using the GTEx project portal (https://www.gtexportal.org/). Significant results were evaluated further. RegulomeDB (http://www.regulomedb.org) and HaploReg v4.2 (https://pubs.broadinstitute.org/mammals/haploreg/haploreg.php) were used to annotate potential functions of the selected SNPs. Differential gene expression analysis was performed using paired t-tests for paired samples. RNA-seq data of breast cancer tissues and corresponding adjacent normal tissues were downloaded from The Cancer Genome Atlas (TCGA) database (https://portal.gdc.cancer.gov). The Kaplan–Meier Plotter breast cancer microarray database (https://kmplot.com/analysis/index.php?p=service&cancer=breast) was used to assess the association between gene expression and breast cancer survival probability. Samples were divided into two groups according to median gene expression, and the JetSet best probe set was used for survival analysis.
Statistical analysis
Overall survival (OS) and breast-cancer-specific survival (BCSS) were the primary endpoints of our study and were defined as the time from the date of cancer diagnosis to the date of all-cause mortality and death from breast cancer, respectively. Invasive disease-free survival (iDFS) and distant disease-free survival (DDFS) were the secondary endpoints and were calculated separately as the time from the date of diagnosis to the date of any invasive recurrence and distant relapse (23). Survival data were analyzed using the Kaplan–Meier (KM) method with the log-rank test and multivariate Cox stepwise regression analysis at the end of the follow-up (31 December 2016). Age at diagnosis, tumor size, lymph node involvement, histological grade, hormone receptor (HR) status, and HER-2/neu expression were adjusted. The hazard ratios (HRs) and 95% confidence intervals (CIs) for each factor in the multivariate analyses were calculated using a Cox regression model. Receiver operating characteristic (ROC) curve analysis was performed to estimate the area under the curve (AUC) of the logistic regression model. The Delong test was performed to compare the AUCs across different models. All tests were two-sided, and p-values <0.05 were considered statistically significant. SAS 9.4 (SAS Institute Inc., Cary, NC, USA) was used for all statistical analyses.
Results
Patients’ characteristics and clinical features
The clinical characteristics and survival information of the patients are summarized in Table 1. For the early-stage breast cancer (EBC) cohort, all cases were female patients, and their mean age was 47.0 ± 10.3 years at breast cancer diagnosis. During the follow-up period, 446 patients experienced recurrence (446 locoregional and 410 distant) and 343 died (333 died of BC and 10 from other diseases).
Patients with tumor size >2 cm, lymph node positivity, clinical stage II + IIIs, or grade III had worse survival (log-rank p < 0.001). HER2 positivity was also associated with a short survival time, whereas ER/HR-positive and luminal A subtypes were associated with improved survival (log-rank p <0.001). However, no association was observed between the age at diagnosis and DDFS, BCSS, or OS (log-rank p = 0.087, 0.420, and 0.402, respectively).
Effects of SNPs in 3′-UTR of PCD-related pathway genes on EBC survival
The effects of different genotype distributions for each SNP on the survival of patients with early-stage breast cancer were evaluated using the log-rank test. Three SNPs (rs4900321, rs7150025, and rs6753785) in the dominant model were significantly associated with iDFS, DDFS, BCSS, and OS in patients with EBC (all log-rank p < 0.05, Table 2).
Multivariate Cox regression analyses revealed that four SNPs (rs4900321 and rs7150025 in ATG2B, rs6753785 in BCL2L11, and rs2213181 in c-Kit) were significantly associated with EBC prognosis in the different genetic models (Table 3). Among these four SNPs, rs4900321and rs2213181 were associated with worse EBC survival (rs4900321 in the dominant model: aHR = 1.330, p = 0.004 for iDFS; 1.444, 0.0003 for DDFS; and 1.268, 0.034 for OS; rs2213181 in the recessive model: aHR = 3.034, p = 0.004 for iDFS; 3.118, 0.003 for DDFS; 3.088, 0.007 for BCSS; and 2.919, 0.010 for OS), while rs6753785 and rs7150025 were associated with better survival (rs7150025 in dominant model: aHR = 0.719, p = 0.0009 for iDFS; 0.706, 0.008 for DDFS; 0.751, 0.013 for BCSS; and 0.741, 0.009 for OS; rs6753785 in dominant model: aHR = 0.748, p = 0.006 for iDFS; 0.729, 0.002 for DDFS; 0.755, 0.013 for BCSS; and 0.757, 0.013 for OS).
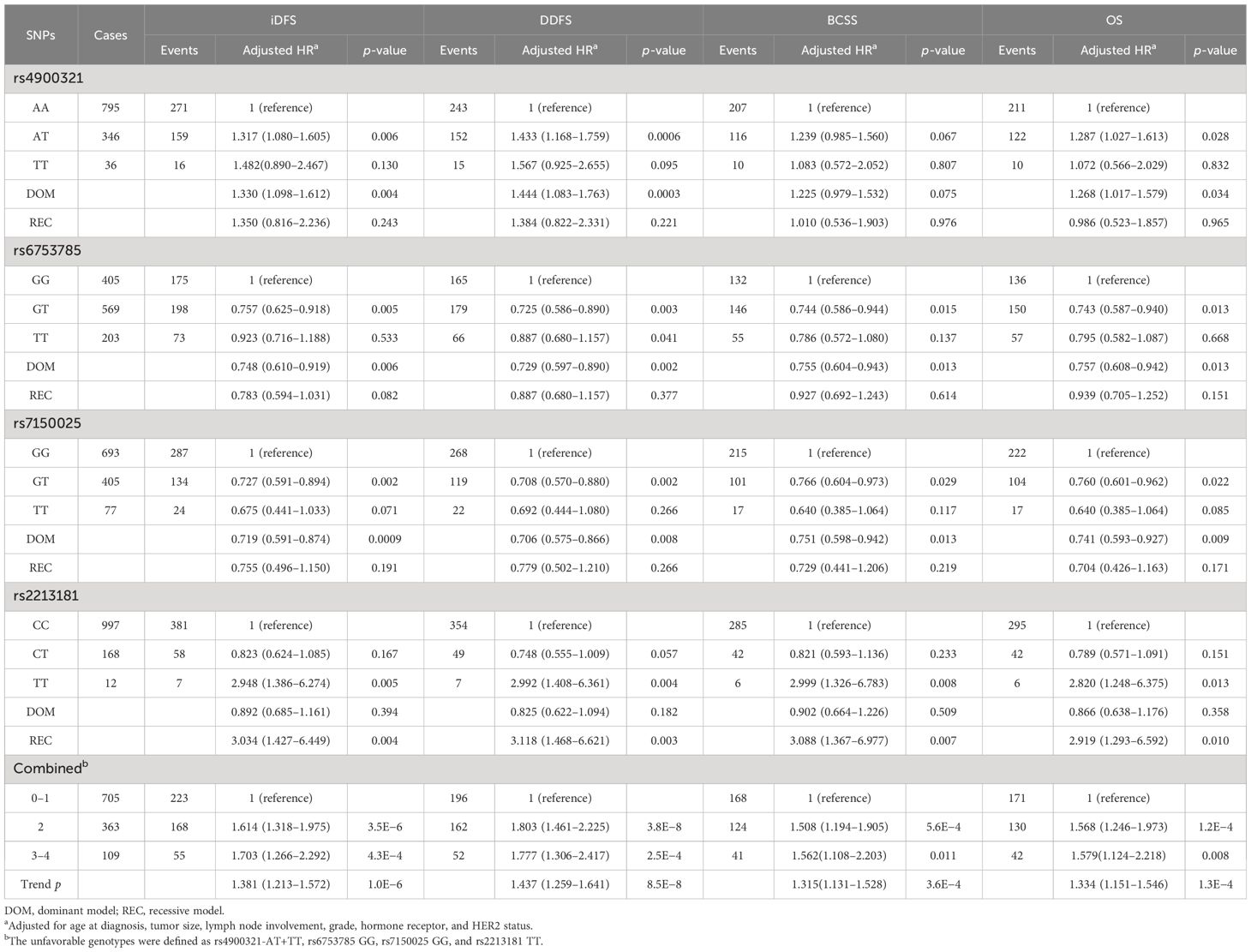
Table 3 Association between the SNPs genotypes and early breast cancer survival (multivariate cox proportional hazard model).
Stratified analysis for association between SNPs and EBC survival
To further explore whether the association between SNP genotypes and EBC survival was modified by other variables, an analysis stratified according to age at diagnosis, tumor size, lymph node involvement, grade, hormone receptor status, and HER2 status was performed. As shown in Supplementary Table S2, we observed the association between rs4789560 and worse survival among young patients (rs4789560 for age at diagnosis ≤ 35: aHR = 1.464, 95% CI = 0.863–2.484 for DDFS, 1.722, 0.909–3.262 for BCSS, and 1.871, 0.988–3.544 for OS). Furthermore, analysis stratified by HER2 status revealed that rs2285332 and rs205107 were associated with survival in patients with higher-grade tumors (rs2285332 for grade III: aHR = 2.364, 95% CI = 1.275–4.383 for iDFS; 2.383, 1.249–4.548 for DDFS; 2.477, 1.215–5.050 for BCSS; and 2.391, 1.174–4.869 for OS; rs205107 for grade III: aHR = 2.258, 95% CI = 1.043–4.889 for iDFS, 1.844, 0.848–4.011 for DDFS; 2.621, 1.186–5.790 for BCSS, and 2.479, 1.126–5.459 for OS). Moreover, rs4900321 in ATG2B was found to have a detrimental effect, while rs7150025 in ATG2B had a beneficial effect on survival in HER2-positive patients (rs4900321 for HER2 positive: aHR = 1.747, 95% CI = 1.266–2.413 for iDFS; 2.013, 1.442–2.809 for DDFS; 1.827, 1.269–2.630 for BCSS; and 1.860, 1.296–2.669 for OS; rs7150025 for HER2 positive: aHR = 0.472, 95% CI = 0.330–0.676 for iDFS; 0.496, 0.341–0.721 for DDFS; 0.493, 0.327–0.744 for BCSS; and 0.500, 0.333–0.751 for OS). However, no obvious differences in the effects of the SNPs on EBC survival were observed among the other strata.
Prognostic significance of risk genotypes in different molecular subtypes of EBC
Next, we performed a subgroup analysis according to the IHC-based breast cancer subtypes. Several variants were found to be strongly associated with HER2 enriched and Luminal B EBC outcomes (Table 4). HER2-enriched patients with the AT + TT genotype for rs4900321 had worse survival (aHR = 1.870, p = 0.006 for iDFS; 2.115, 0.001 for DDFS; 2.076, 0.003 for BCSS; and 2.076, 0.003 for OS) than did patients carrying the AA genotype. In contrast, rs7150025 in the dominant model was significantly correlated with better prognosis (aHR = 0.394, p = 0.0005 for iDFS; 0.422, 0.002 for DDFS; 0.388, 0.001 for BCSS; and 0.388, 0.001 for OS). Moreover, we found that rs2213181 was markedly associated with survival in Luminal B early-stage breast cancer. The TT carriers for rs2213181 had worse survival than did the CC/CT carriers (aHR = 3.920, p = 0.008 for iDFS; 5.017, 0.002 for DDFS; 8.541, 3.7×10−5 for BCSS; and 7.428, 0.0001 for OS).
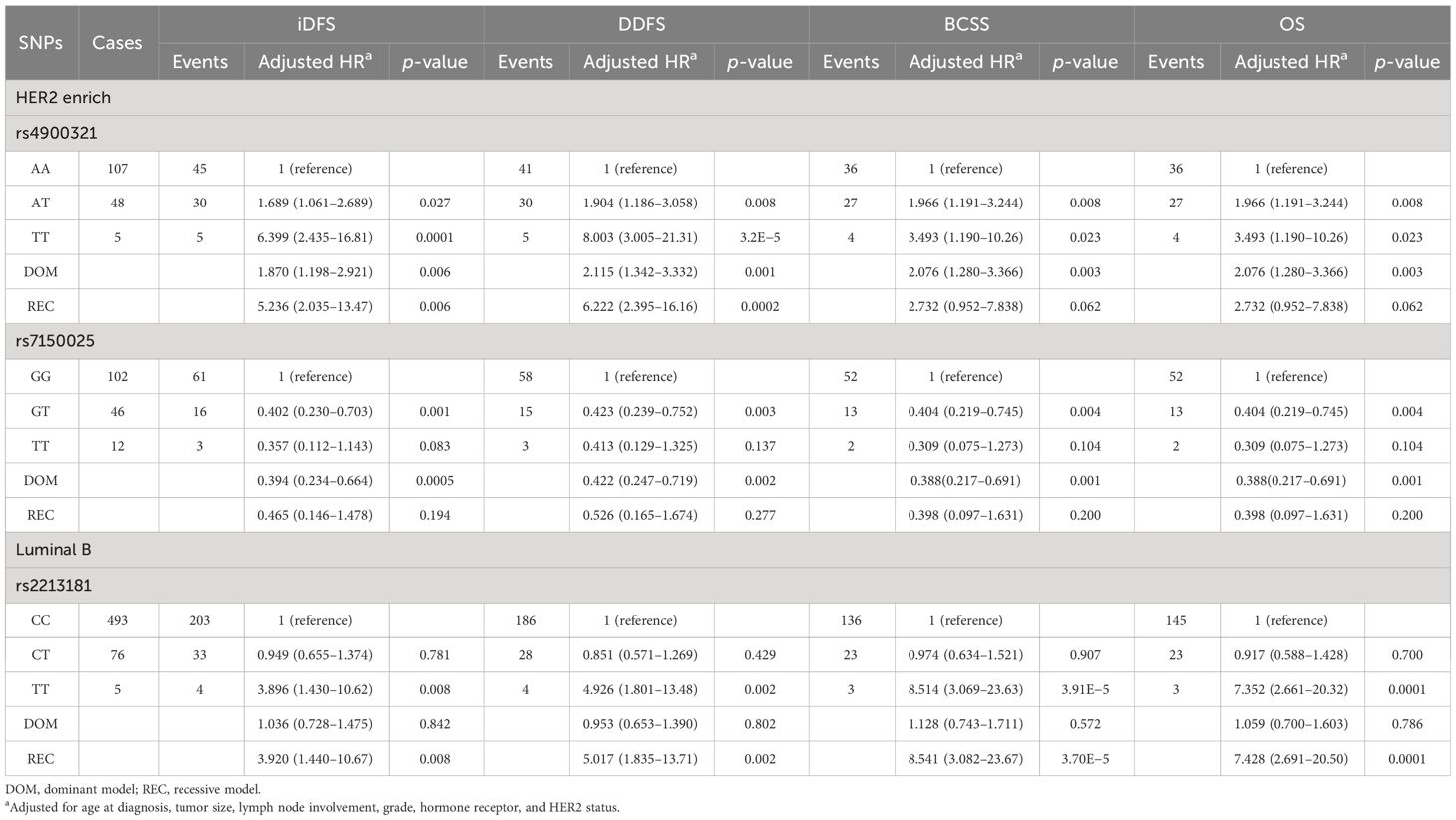
Table 4 Association between the SNPs genotypes and breast cancer survival based on subtypes (multivariate cox proportional hazard model).
Combined analysis of four independent SNPs on survival of EBC
Subsequently, to investigate the potential combined effects of the four independent SNPs on EBC survival, we combined the risk genotypes of rs4900321 AT+TT, rs6753785 GG, rs7150025 GG, and rs2213181 TT into a single variable as the number of unfavorable genotypes. We divided the combined unfavorable genotypes into three groups: low risk (0 unfavorable genotypes), middle risk (1–2 unfavorable genotypes), and high risk (3–4 unfavorable genotypes). Kaplan–Meier analysis revealed that survival, including iDFS, DDFS, BCSS, and OS, decreased as the number of risk genotypes increased (log-rank p = 0.0003 for iDFS; 0.0001 for DDFS; 0.0071 for BCSS; and 0.0051 for OS; Figure 1). Compared with the low-risk group in the multivariate analysis, the middle- and high-risk groups were associated with decreasing risks of recurrence and death in a dose-dependent manner (aHR = 1.381, adjusted ptrend = 1.0×10−6 for iDFS; 1.437, 8.5×10−8 for DDFS; 1.315, 3.6×10−4 for BCSS; and 1.334, 1.3×10−4 for OS; Table 3).
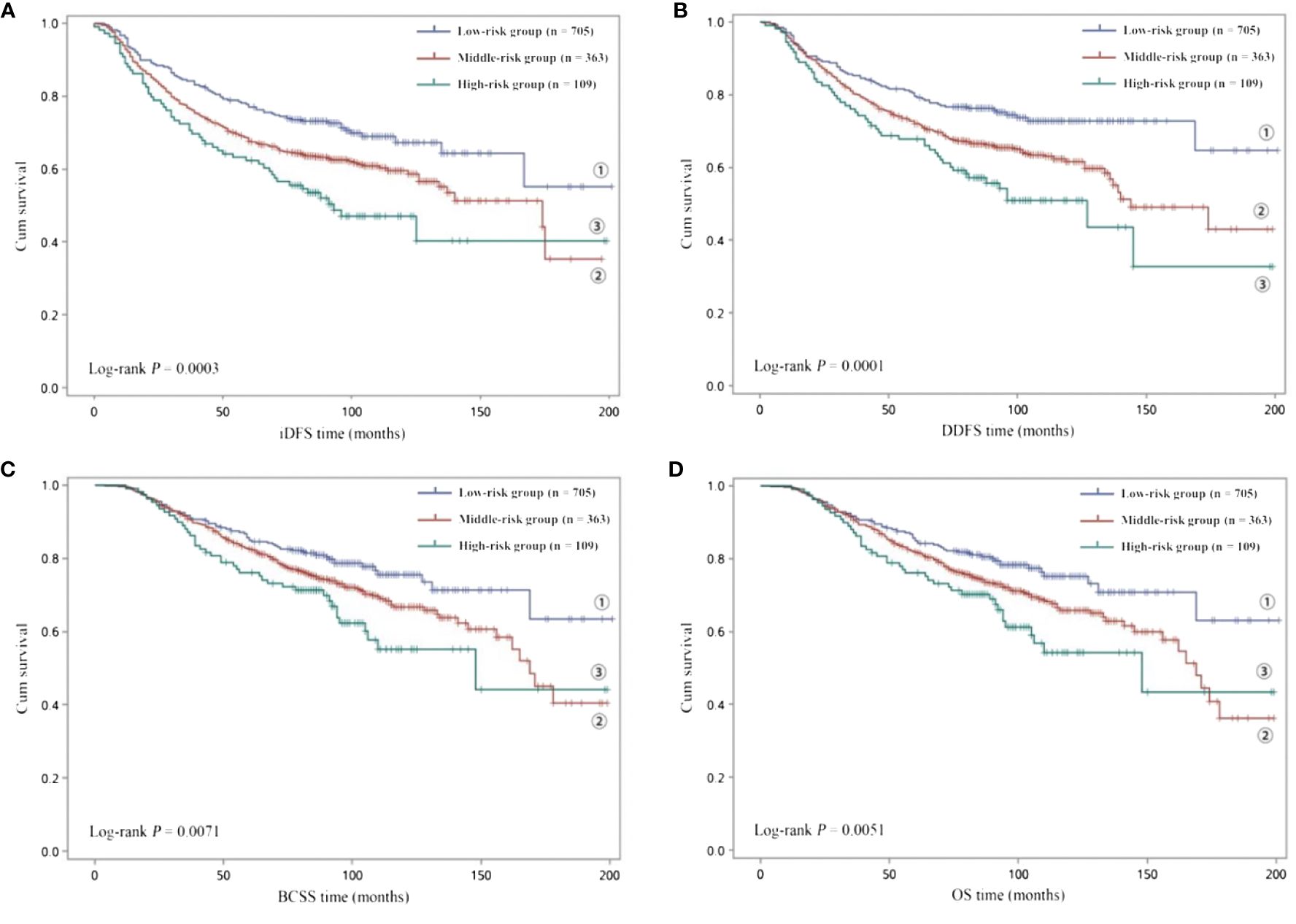
Figure 1 Kaplan–Meier analysis of (A) iDFS and (B) DDFS and (C) BCSS and (D) OS for low-risk (0 unfavorable genotype), middle-risk (1–2 unfavorable genotypes) and high-risk (3–4 unfavorable genotypes) EBC patients.
Survival predictive model based on clinical factors combined with risk genotypes
We constructed a prognostic model that combined risk genotypes and clinical characteristics for survival. The predictive value of the model was assessed using an ROC curve analysis. Compared with the reference model of clinical factors, including age at diagnosis, tumor size, lymph node involvement, grade, hormone receptor, and HER2 status, the model with the addition of unfavorable genotypes improved the predictive capability for iDFS (AUC = 0.732, 95% CI = 0.702–0.761, p = 0.006, Figure 2A), DDFS (AUC = 0.735, 95% CI = 0.705–0.764, p = 0.004, Figure 2B), BCSS (AUC = 0.742, 95% CI = 0.710–0.773, p = 0.029, Figure 2C), and OS (AUC = 0.733, 95% CI = 0.702–0.765, p = 0.019, Figure 2D).
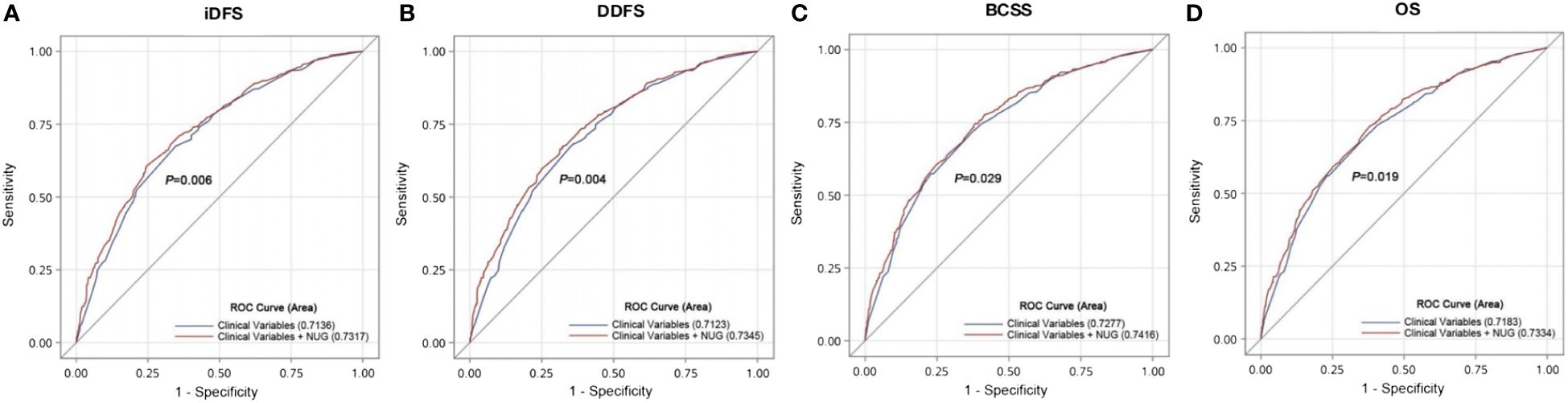
Figure 2 Validation of the prognostic model that combines risk genotypes and clinical characteristics to predict EBC survival. ROC curves and AUCs were used to assess the prognostic accuracy for (A) iDFS and (B) DDFS and (C) BCSS and (D) OS.
eQTL analysis and functional prediction
Next, we sought to better understand the potential mechanisms by which these SNPs influenced the prognosis of EBC. The GTEx Portal eQTL Browser was used to evaluate the correlations between these four SNPs and the mRNA expression of their corresponding genes (Supplementary Table S3). We found that the rs2213181C allele correlated with heightened expression levels of c-Kit in normal breast tissues (p = 0.023). The rs6753785T allele was significantly associated with increased BCL2L11 expression levels in brain tissues (p < 0.001) but not in normal breast tissues (p = 0.9). rs4900321 and rs7150025 statuses had no significant correlation with ATG2B mRNA expression levels (p = 0.81 and 0.47, respectively).
We then performed functional predictions for these four identified SNPs using the online bioinformatics databases RegulomeDB and Haploreg. The results showed that these four SNPs affected promoter histone marks, enhancer histone marks, DNase, and motifs. The details of the biological function predictions are summarized in Supplementary Table S4.
Differential mRNA expression analysis
Finally, we analyzed the mRNA expression of ATG2B, BCL2L11, and c-Kit in 113 pairs of breast tumor and adjacent normal tissue samples obtained from the TCGA database. Kaplan–Meier survival analysis was used to assess the association between the expression levels of these genes and the probability of breast cancer survival. Survival data were obtained from the Kaplan–Meier plotter database. Compared to adjacent normal tissues, breast cancer tissues had lower mRNA expression levels of ATG2B and c-Kit (p <0.001 for both; Supplementary Figures S1A, C). Moreover, lower expression levels of ATG2B and c-Kit mRNA were significantly associated with worse breast cancer recurrence-free survival (RFS) and OS (all log-rank p <0.001; Supplementary Figures S1D, F, G, I). Although the expression levels of BCL2L11 were higher in breast cancer tissues compared to normal breast tissues (p < 0.001, Supplementary Figure S1B), patients with higher BCL2L11 expression within the breast cancer cohort exhibited better RFS and OS compared to those with lower BCL2L11 expression (log-rank p = 0.0016 and 0.013, respectively; Supplementary Figures S1E, H).
Discussion
In this study, we conducted a clinical follow-up to systematically investigate the correlation between miRNA-binding site polymorphisms in PCD pathway genes and disease progression and death in patients with EBC. We found that the variant genotypes ATG2B rs4900321AT+TT and rs7150025 GG, BCL2L11 rs6753785 GG, and c-Kit rs2213181 TT or a combination of these genotypes was significantly associated with an increased risk of EBC survival. Additionally, we discovered that several polymorphisms might play important roles in the progression of HER2 enriched (ATG2B rs4900321 and rs7150025) and Luminal B (c-Kit rs2213181) early breast cancer. Further bioinformatics analyses suggested that the rs6753785T and rs2213181C alleles were correlated with higher BCL2L11 and c-Kit expression.
ATG2B is a core member of the autophagy-related gene (ARG). Autophagy has been reported to play a dual role in tumor development. ATG2B is a direct target of miR-130a, which inhibits autophagy and promotes cell death and chemosensitivity by downregulating ATG2B expression in chronic lymphocytic leukemia and gastrointestinal stromal tumor (GIST) (24, 25). Conversely, ATG2B has been identified as a potential protective biomarker in Ewing’s sarcoma (26). In a recent study by Park et al., ATG2B was shown to inhibit cancer stemness in TNBC (27). Moreover, Zhang et al. found that decreased transcription and expression of ATG2B was related to ER/PR-negative breast cancer, and methylation of ATG2B was also correlated with high-grade and TNM stage in invasive ductal carcinomas (28). According to the KM plotter database, higher ATG2B mRNA expression is associated with better RFS and OS in patients with breast cancer. Our genetic association study indicated that putative miRNAs binding site rs4900321 and rs7150025, located at the 3′-UTR of ATG2B, had an independent impact on the risk of EBC recurrence and death, particularly in HER2-positive breast cancer. We speculate that the rs4900321T and rs7150025G alleles could modify EBC outcomes by influencing the binding affinity of miRNAs and altering ATG2B expression. Further biological and functional studies are needed to determine the role of rs4900321 and rs7150025 in ATG2B during EBC progression.
c-Kit, also known as CD117, is a type III transmembrane receptor protein kinase that regulates cellular proliferation, differentiation, adhesion, and apoptosis. Notably, c-Kit-positive ovarian cancer cells showed higher autophagy levels (29), and c-Kit has been identified as an oncogenic driver and prognostic risk factor for various malignancies, including GIST, osteosarcoma, and ovarian cancer (30–32); however, its role in breast cancer remains unclear. C-Kit overexpression, assessed by immunohistochemistry, has been proven to be an independent indicator of worse prognosis in breast carcinomas and basal-like breast cancer (33, 34). However, high c-Kit expression levels occur infrequently in breast cancer, and c-Kit somatic gene mutations are absent in breast cancer (35). Tsutsui et al. reported that low c-Kit expression is significantly correlated with lymph node metastasis and worse survival in invasive breast cancer (36). Another study demonstrated that TNBC patients with at least one positive expression of three biomarkers (c-Kit, CK5, and EGFR) had a longer OS (37). With respect to common variants in c-Kit gene, a few studies have revealed that some SNPs in c-Kit may be predisposed to melanoma and GIST (38, 39). In our study, we found that the rs2213181T allele was associated with low c-Kit mRNA expression levels and worse prognosis in EBC, especially Luminal B EBC. Additionally, survival data from the KM plotter database indicated a favorable effect of c-Kit expression on breast cancer survival. Similarly, rs2213181 was associated with non-small-cell lung cancer (NSCLC) survival and acute myeloid leukemia susceptibility (40, 41). We speculated that rs2213181 might regulate the expression of c-Kit genes and influence EBC progression. However, the molecular mechanisms by which c-KIT regulates breast cancer cell proliferation remain unclear.
BCL2L11 (also known as BIM) is an essential proapoptotic BH3-only protein that initiates the intrinsic apoptotic pathway and plays an important role in promoting apoptosis in many cancer cells (42). Specifically, BCL2L11 is a tumor suppressor that regulates cell proliferation, metastasis, and chemotherapeutic resistance in breast cancer (43, 44). Genetic variations in BCL2L11 mediate the heterogeneity of responses to tyrosine kinase inhibitors in NSCLC and chronic myeloid leukemia (45, 46). In addition, rs71801447, which lies in the 3′-UTR of BCL2L11, was associated with BCL2L11 expression and anti-tumor immune response in breast cancer (47). The above experimental evidence suggests that variants in BCL2L11 may be of great importance to the susceptibility and treatment response of some cancers. In our study, the rs6753785G allele was a poor prognostic indicator of EBC and was significantly associated with lower BCL2L11 mRNA expression in some specific tissues. These results support the hypothesis that rs6753785 influences EBC prognosis by altering BCL2L11 expression.
However, some potential limitations should be taken into consideration. First, there were geographical limitations because all participants were recruited from the same hospital. Second, it is difficult to explore gene–environment interactions in the development of breast cancer because of the absence of relevant information. Finally, although several genetic variants in the 3′-UTR of PCD-related genes were found to be associated with EBC survival based on in silico analyses, the biological mechanisms underlying the observed association between these SNPs and the development and progression of EBC remain unclear.
In conclusion, this study revealed that rs4900321 and rs7150025 in ATG2B, rs6753785 in BCL2L11, and rs2213181 in c-Kit are associated with early breast cancer prognosis in a Chinese population. These potential functional loci could be used as biomarkers to identify patients with EBC at a high risk of recurrence or death who require more aggressive treatment. Further studies will focus on the evaluation of the 3′-UTR SNP-induced miRNA targeted gene regulation and the functional role of these genes in breast cancer.
Data availability statement
The original contributions presented in the study are included in the article/Supplementary Material, further inquiries can be directed to the corresponding author/s.
Ethics statement
The studies involving humans were approved by Research Ethics Committee of Fujian Medical University Union Hospital. The studies were conducted in accordance with the local legislation and institutional requirements. Written informed consent was obtained from the patients/participants.
Author contributions
HC: Conceptualization, Investigation, Resources, Writing – original draft. MC: Funding acquisition, Investigation, Resources, Validation, Writing – original draft. BZ: Methodology, Writing – review & editing. LT: Resources, Validation, Writing – review & editing. QN: Resources, Writing – review & editing. XJ: Investigation, Writing – review & editing. WG: Writing – review & editing. LC: Funding acquisition, Writing – review & editing. YL: Conceptualization, Resources, Supervision, Writing – review & editing. CW: Funding acquisition, Project administration, Supervision, Writing – review & editing. FF: Conceptualization, Funding acquisition, Project administration, Supervision, Writing – review & editing.
Funding
The author(s) declare that financial support was received for the research, authorship, and/or publication of this article. This work was supported by grants from the Joint Funds for the Innovation of Science and Technology, Fujian Province (2018Y9055 and 2019Y9103), Natural Science Foundation of Fujian Province (2020J01995), and Fujian Provincial Health Technology Project (2020QNA039).
Acknowledgments
We would like to acknowledge the staff of the Department of Breast Surgery, Fujian Medical University Union Hospital, for their support in collecting samples and clinical data.
Conflict of interest
The authors declare that the research was conducted in the absence of any commercial or financial relationships that could be construed as a potential conflict of interest.
Publisher’s note
All claims expressed in this article are solely those of the authors and do not necessarily represent those of their affiliated organizations, or those of the publisher, the editors and the reviewers. Any product that may be evaluated in this article, or claim that may be made by its manufacturer, is not guaranteed or endorsed by the publisher.
Supplementary material
The Supplementary Material for this article can be found online at: https://www.frontiersin.org/articles/10.3389/fimmu.2024.1284579/full#supplementary-material
References
1. Chen W, Zheng R, Baade PD, Zhang S, Zeng H, Bray F, et al. Cancer statistics in China, 2015. CA Cancer J Clin. (2016) 66:115–32. doi: 10.3322/caac.21338
2. Sung H, Ferlay J, Siegel RL, Laversanne M, Soerjomataram I, Jemal A, et al. Global cancer statistics 2020: GLOBOCAN estimates of incidence and mortality worldwide for 36 cancers in 185 countries. CA Cancer J Clin. (2021) 71:209–49. doi: 10.3322/caac.21660
3. Early Breast Cancer Trialists’ Collaborative Group (EBCTCG). Long-term outcomes for neoadjuvant versus adjuvant chemotherapy in early breast cancer: meta-analysis of individual patient data from ten randomised trials. Lancet Oncol. (2018) 19:27–39. doi: 10.1016/S1470-2045(17)30777-5
4. Pirie A, Guo Q, Kraft P, Canisius S, Eccles DM, Rahman N, et al. Common germline polymorphisms associated with breast cancer-specific survival. Breast Cancer Res. (2015) 17:58. doi: 10.1186/s13058-015-0570-7
5. Adedokun B, Du Z, Gao G, Ahearn TU, Lunetta KL, Zirpoli G, et al. Cross-ancestry GWAS meta-analysis identifies six breast cancer loci in African and European ancestry women. Nat Commun. (2021) 12:4198. doi: 10.1038/s41467-021-24327-x
6. Morra A, Escala-Garcia M, Beesley J, Keeman R, Canisius S, Ahearn TU, et al. Association of germline genetic variants with breast cancer-specific survival in patient subgroups defined by clinic-pathological variables related to tumor biology and type of systemic treatment. Breast Cancer Res. (2021) 23:86. doi: 10.1186/s13058-021-01450-7
7. Escala-Garcia M, Guo Q, Dörk T, Canisius S, Keeman R, Dennis J, et al. Genome-wide association study of germline variants and breast cancer-specific mortality. Br J Cancer. (2019) 120:647–57. doi: 10.1038/s41416-019-0393-x
8. Farh KK, Grimson A, Jan C, Lewis BP, Johnston WK, Lim LP, et al. The widespread impact of mammalian MicroRNAs on mRNA repression and evolution. Science. (2005) 310:1817–21. doi: 10.1126/science.1121158
9. Song F, Zheng H, Liu B, Wei S, Dai H, Zhang L, et al. An miR-502-binding site single-nucleotide polymorphism in the 3’-untranslated region of the SET8 gene is associated with early age of breast cancer onset. Clin Cancer Res. (2009) 15:6292–300. doi: 10.1158/1078-0432.CCR-09-0826
10. Zhang L, Ye Y, Tu H, Hildebrandt MA, Zhao L, Heymach JV, et al. MicroRNA-related genetic variants in iron regulatory genes, dietary iron intake, microRNAs and lung cancer risk. Ann Oncol. (2017) 28:1124–9. doi: 10.1093/annonc/mdx046
11. Zhu Y, Tian J, Peng X, Wang X, Yang N, Ying P, et al. A genetic variant conferred high expression of CAV2 promotes pancreatic cancer progression and associates with poor prognosis. Eur J Cancer. (2021) 151:94–105. doi: 10.1016/j.ejca.2021.04.008
12. Koren E, Fuchs Y. Modes of regulated cell death in cancer. Cancer Discovery. (2021) 11:245–65. doi: 10.1158/2159-8290.CD-20-0789
13. Tang R, Xu J, Zhang B, Liu J, Liang C, Hua J, et al. Ferroptosis, necroptosis, and pyroptosis in anticancer immunity. J Hematol Oncol. (2020) 13:110. doi: 10.1186/s13045-020-00946-7
14. Chen X, Zeh HJ, Kang R, Kroemer G, Tang D. Cell death in pancreatic cancer: from pathogenesis to therapy. Nat Rev Gastroenterol Hepatol. (2021) 18:804–23. doi: 10.1038/s41575-021-00486-6
15. Di Leva G, Garofalo M, Croce CM. MicroRNAs in cancer. Annu Rev Pathol. (2014) 9:287–314. doi: 10.1146/annurev-pathol-012513-104715
16. Shirjang S, Mansoori B, Asghari S, Duijf PHG, Mohammadi A, Gjerstorff M, et al. MicroRNAs in cancer cell death pathways: Apoptosis and necroptosis. Free Radic Biol Med. (2019) 139:1–15. doi: 10.1016/j.freeradbiomed.2019.05.017
17. Deng Q, Hu H, Yu X, Liu S, Wang L, Chen W, et al. Tissue-specific microRNA expression alters cancer susceptibility conferred by a TP53 noncoding variant. Nat Commun. (2019) 10:5061. doi: 10.1038/s41467-019-13002-x
18. Liang D, Meyer L, Chang DW, Lin J, Pu X, Ye Y, et al. Genetic variants in MicroRNA biosynthesis pathways and binding sites modify ovarian cancer risk, survival, and treatment response. Cancer Res. (2010) 70:9765–76. doi: 10.1158/0008-5472.CAN-10-0130
19. Liu Z, Wei S, Ma H, Zhao M, Myers JN, Weber RS, et al. A functional variant at the miR-184 binding site in TNFAIP2 and risk of squamous cell carcinoma of the head and neck. Carcinogenesis. (2011) 32:1668–74. doi: 10.1093/carcin/bgr209
20. Shi TY, Cheng X, Yu KD, Sun MH, Shao ZM, Wang MY, et al. Functional variants in TNFAIP8 associated with cervical cancer susceptibility and clinical outcomes. Carcinogenesis. (2013) 34:770–8. doi: 10.1093/carcin/bgt001
21. Gong J, Tong Y, Zhang HM, Wang K, Hu T, Shan G, et al. Genome-wide identification of SNPs in microRNA genes and the SNP effects on microRNA target binding and biogenesis. Hum Mutat. (2012) 33:254–63. doi: 10.1002/humu.21641
22. Crocco P, Montesanto A, Passarino G, Rose G. Polymorphisms falling within putative miRNA target sites in the 3’UTR region of SIRT2 and DRD2 genes are correlated with human longevity. J Gerontol A Biol Sci Med Sci. (2016) 71:586–92. doi: 10.1093/gerona/glv058
23. Gourgou-Bourgade S, Cameron D, Poortmans P, Asselain B, Azria D, Cardoso F, et al. Guidelines for time-to-event end point definitions in breast cancer trials: results of the DATECAN initiative (Definition for the Assessment of Time-to-event Endpoints in CANcer trials). Ann Oncol. (2015) 26:2505–6. doi: 10.1093/annonc/mdv478
24. Kovaleva V, Mora R, Park YJ, Plass C, Chiramel AI, Bartenschlager R, et al. miRNA-130a targets ATG2B and DICER1 to inhibit autophagy and trigger killing of chronic lymphocytic leukemia cells. Cancer Res. (2012) 72:1763–72. doi: 10.1158/0008-5472.CAN-11-3671
25. Zhang J, Chen K, Tang Y, Luan X, Zheng X, Lu X, et al. LncRNA-HOTAIR activates autophagy and promotes the imatinib resistance of gastrointestinal stromal tumor cells through a mechanism involving the miR-130a/ATG2B pathway. Cell Death Dis. (2021) 12:367. doi: 10.1038/s41419-021-03650-7
26. Wen J, Wan L, Dong X. The prognostic value of autophagy related genes with potential protective function in Ewing sarcoma. BMC Bioinf. (2022) 23:306. doi: 10.1186/s12859-022-04849-x
27. Park JW, Kim Y, Lee SB, Oh CW, Lee EJ, Ko JY, et al. Autophagy inhibits cancer stemness in triple-negative breast cancer via miR-181a-mediated regulation of ATG5 and/or ATG2B. Mol Oncol. (2022) 16:1857–75. doi: 10.1002/1878-0261.13180
28. Zhang X, Li C, Wang D, Chen Q, Li CL, Li HJ, et al. Aberrant methylation of ATG2B, ATG4D, ATG9A and ATG9B CpG island promoter is associated with decreased mRNA expression in sporadic breast carcinoma. Gene. (2016) 590:285–92. doi: 10.1016/j.gene.2016.05.036
29. Pagotto A, Pilotto G, Mazzoldi EL, Nicoletto MO, Frezzini S, Pastò A, et al. Autophagy inhibition reduces chemoresistance and tumorigenic potential of human ovarian cancer stem cells. Cell Death Dis. (2017) 8:e2943. doi: 10.1038/cddis.2017.327
30. Corless CL, Fletcher JA, Heinrich MC. Biology of gastrointestinal stromal tumors. J Clin Oncol. (2004) 22:3813–25. doi: 10.1200/JCO.2004.05.140
31. Adhikari AS, Agarwal N, Wood BM, Porretta C, Ruiz B, Pochampally RR, et al. CD117 and Stro-1 identify osteosarcoma tumor-initiating cells associated with metastasis and drug resistance. Cancer Res. (2010) 70:4602–12. doi: 10.1158/0008-5472.CAN-09-3463
32. Mazzoldi EL, Pavan S, Pilotto G, Leone K, Pagotto A, Frezzini S, et al. A juxtacrine/paracrine loop between C-Kit and stem cell factor promotes cancer stem cell survival in epithelial ovarian cancer. Cell Death Dis. (2019) 10:412. doi: 10.1038/s41419-019-1656-4
33. Charpin C, Giusiano S, Charfi S, Secq V, Carpentier S, Andrac L, et al. Quantitative immunohistochemical expression of c Kit in breast carcinomas is predictive of patients’ outcome. Br J Cancer. (2009) 101:48–54. doi: 10.1038/sj.bjc.6605113
34. Kashiwagi S, Yashiro M, Takashima T, Aomatsu N, Kawajiri H, Ogawa Y, et al. c-Kit expression as a prognostic molecular marker in patients with basal-like breast cancer. Br J Surg. (2013) 100:490–6. doi: 10.1002/bjs.9021
35. Simon R, Panussis S, Maurer R, Spichtin H, Glatz K, Tapia C, et al. KIT (CD117)-positive breast cancers are infrequent and lack KIT gene mutations. Clin Cancer Res. (2004) 10:178–83. doi: 10.1158/1078-0432.ccr-0597-3
36. Tsutsui S, Yasuda K, Suzuki K, Takeuchi H, Nishizaki T, Higashi H, et al. A loss of c-kit expression is associated with an advanced stage and poor prognosis in breast cancer. Br J Cancer. (2006) 94:1874–8. doi: 10.1038/sj.bjc.6603183
37. Schulmeyer CE, Fasching PA, Häberle L, Meyer J, Schneider M, Wachter D, et al. Expression of the immunohistochemical markers CK5, CD117, and EGFR in molecular subtypes of breast cancer correlated with prognosis. Diagnostics (Basel). (2023) 13:372. doi: 10.3390/diagnostics13030372
38. Bourillon A, Hu HH, Hetet G, Lacapere JJ, André J, Descamps V, et al. Genetic variation at KIT locus may predispose to melanoma. Pigment Cell Melanoma Res. (2013) 26:88–96. doi: 10.1111/pcmr.12032
39. Ravegnini G, Serrano C, Simeon V, Sammarini G, Nannini M, Roversi E, et al. The rs17084733 variant in the KIT 3’ UTR disrupts a miR-221/222 binding site in gastrointestinal stromal tumour: a sponge-like mechanism conferring disease susceptibility. Epigenetics. (2019) 14:545–57. doi: 10.1080/15592294.2019.1595997
40. Sun J, Zhu M, Shen W, Wang C, Dai J, Xu L, et al. A potentially functional polymorphism in ABCG2 predicts clinical outcome of non-small cell lung cancer in a Chinese population. Pharmacogenomics J. (2017) 17:280–5. doi: 10.1038/tpj.2016.2
41. Walgren R, McLaughlin E, Peterson E, Crosswhite M, Province M, McLeod H, et al. Identification of polymorphisms associated with susceptibility to therapy-related MDS and AML. Blood. (2007) 110:15. doi: 10.1182/blood.V110.11.15.15
42. Akiyama T, Dass CR, Choong PF. Bim-targeted cancer therapy: a link between drug action and underlying molecular changes. Mol Cancer Ther. (2009) 8:3173–80. doi: 10.1158/1535-7163.MCT-09-0685
43. Merino D, Best SA, Asselin-Labat ML, Vaillant F, Pal B, Dickins RA, et al. Pro-apoptotic Bim suppresses breast tumor cell metastasis and is a target gene of SNAI2. Oncogene. (2015) 34:3926–34. doi: 10.1038/onc.2014.313
44. Sun WL, He LY, Liang L, Liu SY, Luo J, Lv ML, et al. Ambra1 regulates apoptosis and chemosensitivity in breast cancer cells through the Akt-FoxO1-Bim pathway. Apoptosis. (2022) 27:329–41. doi: 10.1007/s10495-022-01718-z
45. Ng KP, Hillmer AM, Chuah CT, Juan WC, Ko TK, Teo AS, et al. A common BIM deletion polymorphism mediates intrinsic resistance and inferior responses to tyrosine kinase inhibitors in cancer. Nat Med. (2012) 18:521–8. doi: 10.1038/nm.2713
46. Marum JE, Yeung DT, Purins L, Reynolds J, Parker WT, Stangl D, et al. ASXL1 and BIM germ line variants predict response and identify CML patients with the greatest risk of imatinib failure. Blood Adv. (2017) 1:1369–81. doi: 10.1182/bloodadvances.2017006825
Keywords: breast cancer, survival, single nucleotide polymorphism, programmed cell death, prognosis
Citation: Chen H, Chen M, Zeng B, Tang L, Nie Q, Jin X, Guo W, Chen L, Lin Y, Wang C and Fu F (2024) Additional prognostic value of polymorphisms within the 3′-untranslated region of programmed cell death pathway genes in early-stage breast cancer. Front. Immunol. 15:1284579. doi: 10.3389/fimmu.2024.1284579
Received: 28 August 2023; Accepted: 25 March 2024;
Published: 16 April 2024.
Edited by:
Mohammad Hojjat-Farsangi, Karolinska Institutet (KI), SwedenReviewed by:
Milad Khorasani, Neyshabur University of Medical Sciences, IranKhawla S. Al-Kuraya, King Faisal Specialist Hospital and Research Centre, Saudi Arabia
Copyright © 2024 Chen, Chen, Zeng, Tang, Nie, Jin, Guo, Chen, Lin, Wang and Fu. This is an open-access article distributed under the terms of the Creative Commons Attribution License (CC BY). The use, distribution or reproduction in other forums is permitted, provided the original author(s) and the copyright owner(s) are credited and that the original publication in this journal is cited, in accordance with accepted academic practice. No use, distribution or reproduction is permitted which does not comply with these terms.
*Correspondence: Yuxiang Lin, bHl4MDUyMEBmam11LmVkdS5jbg==; Chuan Wang, ZHJfY2h1YW53YW5nQGZqbXUuZWR1LmNu; Fangmeng Fu, ZmZtQGZqbXUuZWR1LmNu
†These authors have contributed equally to this work and share first authorship