- 1Department of Dermatology, Chongqing Dangdai Plastic Surgery Hospital, Chongqing, China
- 2Department of Dermatology, The Third Affiliated Hospital of Chongqing Medical University, Chongqing, China
- 3Department of Dermatology, Chongqing Huamei Plastic Surgery Hospital, Chongqing, China
Background: The emergence of immunotherapy has changed the treatment modality for melanoma and prolonged the survival of many patients. However, a handful of patients remain unresponsive to immunotherapy and effective tools for early identification of this patient population are still lacking. Researchers have developed machine learning algorithms for predicting immunotherapy response in melanoma, but their predictive accuracy has been inconsistent. Therefore, the present systematic review and meta-analysis was performed to comprehensively evaluate the predictive accuracy of machine learning in melanoma response to immunotherapy.
Methods: Relevant studies were searched in PubMed, Web of Sciences, Cochrane Library, and Embase from their inception to July 30, 2022. The risk of bias and applicability of the included studies were assessed using the Prediction Model Risk of Bias Assessment Tool (PROBAST). Meta-analysis was performed on R4.2.0.
Results: A total of 36 studies consisting of 30 cohort studies and 6 case-control studies were included. These studies were mainly published between 2019 and 2022 and encompassed 75 models. The outcome measures of this study were progression-free survival (PFS), overall survival (OS), and treatment response. The pooled c-index was 0.728 (95%CI: 0.629–0.828) for PFS in the training set, 0.760 (95%CI: 0.728–0.792) and 0.819 (95%CI: 0.757–0.880) for treatment response in the training and validation sets, respectively, and 0.746 (95%CI: 0.721–0.771) and 0.700 (95%CI: 0.677–0.724) for OS in the training and validation sets, respectively.
Conclusion: Machine learning has considerable predictive accuracy in melanoma immunotherapy response and prognosis, especially in the former. However, due to the lack of external validation and the scarcity of certain types of models, further studies are warranted.
1 Introduction
Melanoma is a potentially fatal and highly malignant melanocytic tumor with the highest metastasis and mortality rates among all skin cancers (1). Melanoma accounts for < 5% of all skin cancers but is responsible for 80% of skin cancer deaths. The incidence and mortality of melanoma continue to rise every year (2). Melanoma commonly develops in the skin but can also occur in the mucosa and organs. Confirmed risk factors for cutaneous melanoma include ultraviolet radiation and subsequent sunburn (3). For primary melanoma without lymph node metastases, the 5-year relative survival is 98% for stage I melanoma and 90% for stage II melanoma (4). However, melanoma often metastasizes to the lymph nodes first, and metastatic melanoma has a very poor prognosis. Melanomas that are identified early are generally removed by extensive local resection and staged by sentinel lymph node biopsy. Advanced melanoma, on the other hand, requires a multimodal approach consisting of systemic treatments such as targeted therapy (e.g., targeted BRAF inhibition (BRAFi)) and immune checkpoint inhibitors (ICIs) (5).
Immunotherapy exerts antitumor activities by enhancing immune responses in the body. However, hyperactivation of the immune system can damage normal cells. The advent of cytotoxic T-lymphocyte-associated protein 4 inhibitors (CTLA4 inhibitors) and programmed death ligand 1 inhibitors (PD-1 inhibitors) has completely revolutionized the management and treatment of various cancers and especially improved the systemic treatment of advanced melanoma (6). ICIs are superior to chemotherapy alone in the treatment of melanoma, but ICI combination therapy is even more effective than ICIs alone. As a result, nivolumab plus ipilimumab has been approved for the treatment of metastatic melanoma (7). A previous study showed that ipilimumab can increase the long-term survival rate (3 years or longer) of metastatic melanoma patients by about 20% (8). Although ICIs can improve the survival time of melanoma patients and maintain remission in responders, there are still a substantial number of patients who do not respond to ICIs. It was reported that less than 50% of patients respond to ICI monotherapy (9). Similarly, about 60%-70% of melanoma patients are unresponsive to anti-PD-1 monotherapy (nivolumab or pembrolizumab), and 40%-50% of patients are unresponsive to anti-CTLA-4 combination therapy (10). Therefore, early identification of the survival outcomes of melanoma patients receiving ICIs can aid in the development of rehabilitation and follow-up strategies and is of great significance in clinical practice. Unfortunately, there is currently a lack of such highly effective and accurate prediction tools.
With the rapid development of intelligent computing in recent years, machine learning has gained wide application in various fields and has been integrated into clinical practice. In particular, machine learning is commonly used for disease diagnosis and prognosis prediction (11). As machine learning continues to be improved and refined, challenges that were previously faced by researchers and clinicians are now easily resolved, and these models demonstrate great potentials in medical research, refinement of clinical care, and prediction of treatment and cancer prognosis. In fact, several studies have developed machine learning models for the early prediction of melanoma immunotherapy response and prognosis. However, these models were constructed using different methods and prediction variables such as clinical features, radiomics features, and a combination of both features. As a result, the predictive accuracy of these models has been inconsistent, which hinders the further development of artificial intelligence (AI) for the early prediction of immunotherapy response in melanoma. Here, we conducted a meta-analysis to systematically evaluate the predictive accuracy of machine learning in immunotherapy response and prognosis of melanoma in order to provide new insights into AI development in melanoma.
2 Materials and methods
Intervention studies involving animals or humans, and other studies that require ethical approval, must describe the authority that provided approval and the corresponding ethical approval code. This systematic review and meta-analysis was performed according to the Preferred Reporting Items for Systematic Reviews and Meta-Analyses (PRIMSA) guidelines (12).
2.1 Article search strategy
Relevant articles were systematically searched in PubMed, Cochrane Library, Embase, and Web of Science from their inception to July 30, 2022, without restrictions on country, language, or publication type. Search keywords included “Melanoma”, “machine learning”, and “Immune Checkpoint Inhibitors”. The complete search strategy for all databases is shown in Supplementary Table 1.
2.2 Inclusion and exclusion criteria
Inclusion criteria:
1. Patients who have been diagnosed with melanoma and received ICI treatment;
2. A complete machine learning predictive model for immunotherapy response or prognosis of melanoma;
3. Studies that only trained but did not validate the model using an independent validation set were included;
4. Different machine learning models based on the same dataset were included;
5. Studies published in English;
6. Randomized-controlled trials (RCTs), case-control studies, cohort studies, nested case-control studies, and case-cohort studies were included.
Exclusion criteria:
1. Studies that did not clearly describe ICI for the treatment of melanoma;
2. Only risk factors analysis was performed without the construction of a complete risk model;
3. Lack of metrics (receiver operating characteristic (ROC) curves, c-index, sensitivity, specificity, accuracy, recovery, precision, confusion matrix, diagnostic 2x2 table, F1 score, and calibration curve) that evaluate the accuracy of the machine learning model;
4. Meeting abstract without full text.
2.3 Article screening
The identified articles were imported into Endnote X20, and duplications were automatically and manually identified and removed. Titles and abstracts were screened, and the full texts of potentially eligible studies were downloaded for further review. Article screening was independently conducted and cross-checked by two researchers (Juan Li and Kena Dan). Any disagreement was resolved with the help of another researcher (Jun Ai).
2.4 Data extraction
A data extraction form was designed based on the modified Critical Appraisal and Data Extraction for Systematic Reviews of Prediction Modelling Studies (CHARMS) (13). Data that were extracted include:
1. Characteristics of included studies: Title, first author, year of publication, country, study type, and source of patients.
2. Characteristics of immunotherapy: Immunotherapy drug and duration of follow-up.
3. Characteristics of machine learning: Prediction goals, validation set, training set, method of variable screening/feature selection, model type, modeling variables, and model evaluation measures.
Data extraction was independently performed and cross-checked by two researchers (Juan Li and Kena Dan). Any disagreement was resolved with the help of another researcher (Jun Ai).
2.5 Risk of bias assessment
The risk of bias and applicability of the included studies were evaluated using the Prediction Model Risk of Bias Assessment Tool (PROBAST) (14). This tool includes 4 domains, namely participants, predictors, outcome, and analysis. The risk of bias in each included study was assessed as “high risk”, “low risk” or “unclear risk”. This process was independently completed by two researchers (Juan Li and Kena Dan), and any disagreement was resolved with the help of a third researcher (Jun Ai).
2.6 Outcome measures
The outcome measures for this systematic review were overall survival (OS), progression-free survival (PFS), and objective response rate (ORR) of ICI-treated melanoma patients.
2.7 Data analysis
A meta-analysis was performed on the c-index and accuracy of machine learning models. If the c-index lacks a 95% confidence interval (IC) and standard error, the standard error was estimated using the methods by Debray TP et al (15). Given the differences in variables and parameters used in various machine learning models, a random effects model was used for the meta-analysis of c-index. In addition, sensitivity and specificity were meta-analyzed using a bivariate mixed effects model. Subgroup analysis was performed based on each type of modeling variables (genomics, radiomics, and clinical characteristics. All meta-analyses were completed on R4.2.0 (R development Core Team, Vienna, http://www.R-project.org).
3 Results
3.1 Process of article screening
A total of 2,759 records were identified from the four databases, and 1,062 duplicate records were deleted. After title and abstract screening, 1,624 records were excluded, and the remaining 72 records were assessed for eligibility. After reviewing the full texts, 36 records were further excluded, and a final total of 36 records were included in the meta-analysis. The study selection process is shown in Figure 1.
3.2 General characteristics of included studies
The year of publication of the 36 included studies was primarily between 2019 and 2022. Of the 36 studies, 18 were conducted in China (16–33) and 5 were conducted in Germany (34–38). The primary outcome measures were treatment response, OS, and PFS. The included studies encompassed 75 models, including 45 OS prediction models, 21 treatment response models, and 6 PFS prediction models. In terms of modeling variables, there were 17 clinical features-based models, 27 radiomics-based risk models, and 31 genomics-based models. The specific characteristics of the included studies (16–50) are summarized in Table 1.
3.3 Risk of bias assessment
All included studies evaluated the OS, treatment response, and PFS of melanoma patients after ICI treatment. Patients used for OS and PFS evaluation mainly originated from the respective cohort studies in registered databases and single-center electronic case databases. PROBAST assessment indicated that studies from the registered databases had a low risk of bias while those from the single-center electronic case database had a high risk of bias. Patients used for treatment response evaluation originated from case-control studies in registered databases or single-center electronic case databases. Most studies did not have >20 events per variable (EPV) or >100 patients in the independent validation set. Therefore, these two parameters constituted the primary sources of high bias in the included models (Figure 2).
3.4 Meta-analysis
The OS, treatment response, and PFS data were pooled from the 36 studies to determine the c-index (95% CI) of each outcome measure predicted by different models and modeling variables.
Genomics-based prediction models showed the best performance in OS prediction. The pooled c-index was 0.805 (95%CI: 0.731–0.879) in the training set and 0.694 (95%CI: 0.670–0.718) in the validation set, and the logistic regression model performed best with a c-index of 0.882 (95%CI: 0.835–0.929). Radiomics-based models ranked second in OS prediction (pooled c-index: 0.733 (95%CI: 0.722–0.745) in the training set), followed by clinical features-based models [pooled c-index: 0.719 (95%CI: 0.664–0.774) in the training set and 0.650 (95%CI: 0.641–0.659) in the validation set]. The overall c-index for OS was 0.746 (95%CI: 0.721–0.771) in the training set and 0.700 (95%CI: 0.677–0.724) in the validation set (Table 2).
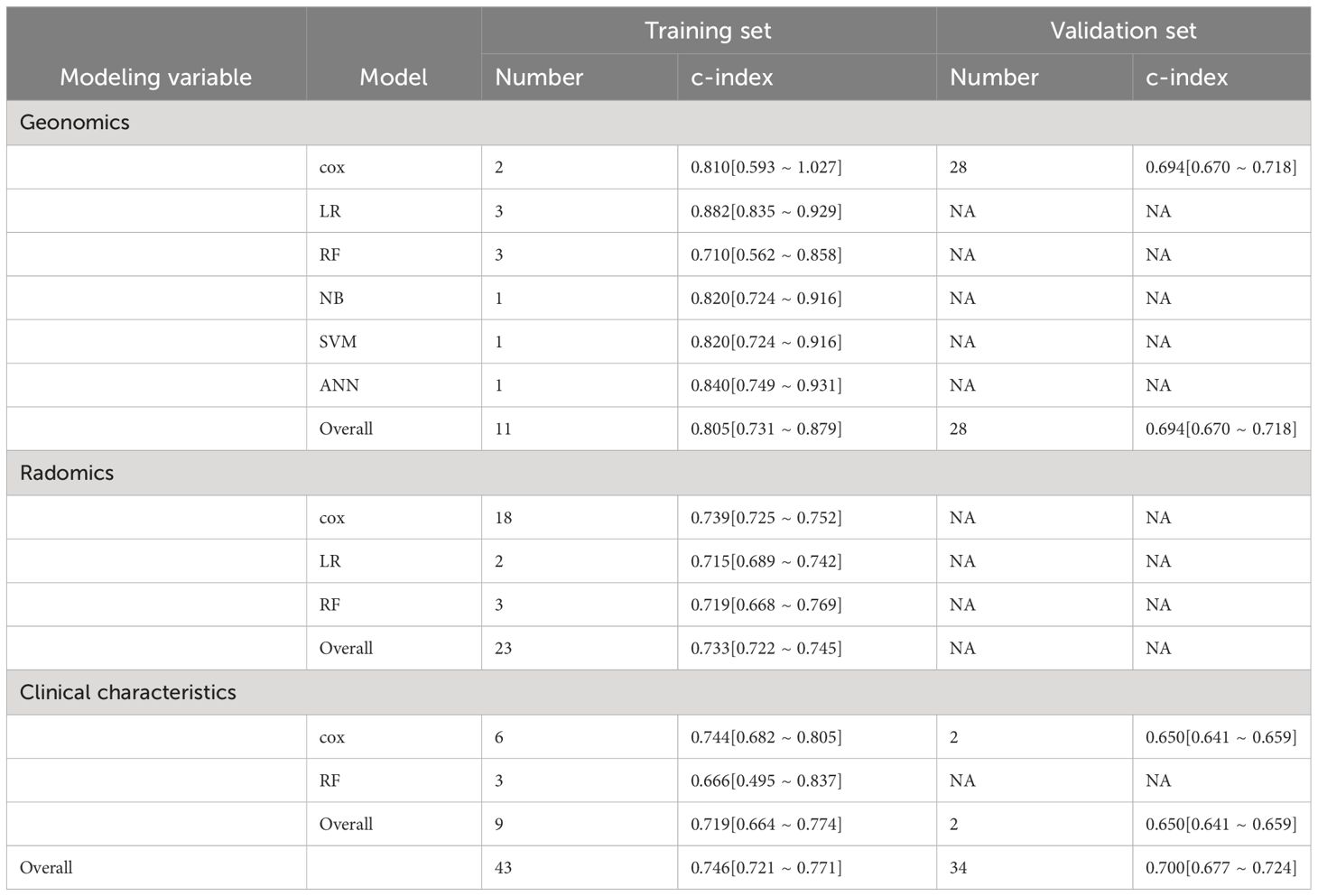
Table 2 Meta-analysis of c-index of machine learning in OS prediction for ICI-treated melanoma patients.
For the prediction of treatment response, the pooled c-index of radiomics-based models was 0.802 (95%CI: 0.739–0.866) in the training set and 0.813 (95%CI: 0.711–0.915) in the validation set. The pooled c-index of genomics-based models was 0.760 (95%CI: 0.668–0.853) in the training set and 0.857 (95%CI: 0.698–1.000) in the validation set. The pooled c-index of clinical characteristics-based models was 0.723 (95%CI: 0.699–0.747). The overall c-index for treatment response was 0.760 (95%CI: 0.728–0.792) in the training set and 0.819 (95%CI: 0.757–0.880) in the validation set (Table 3).
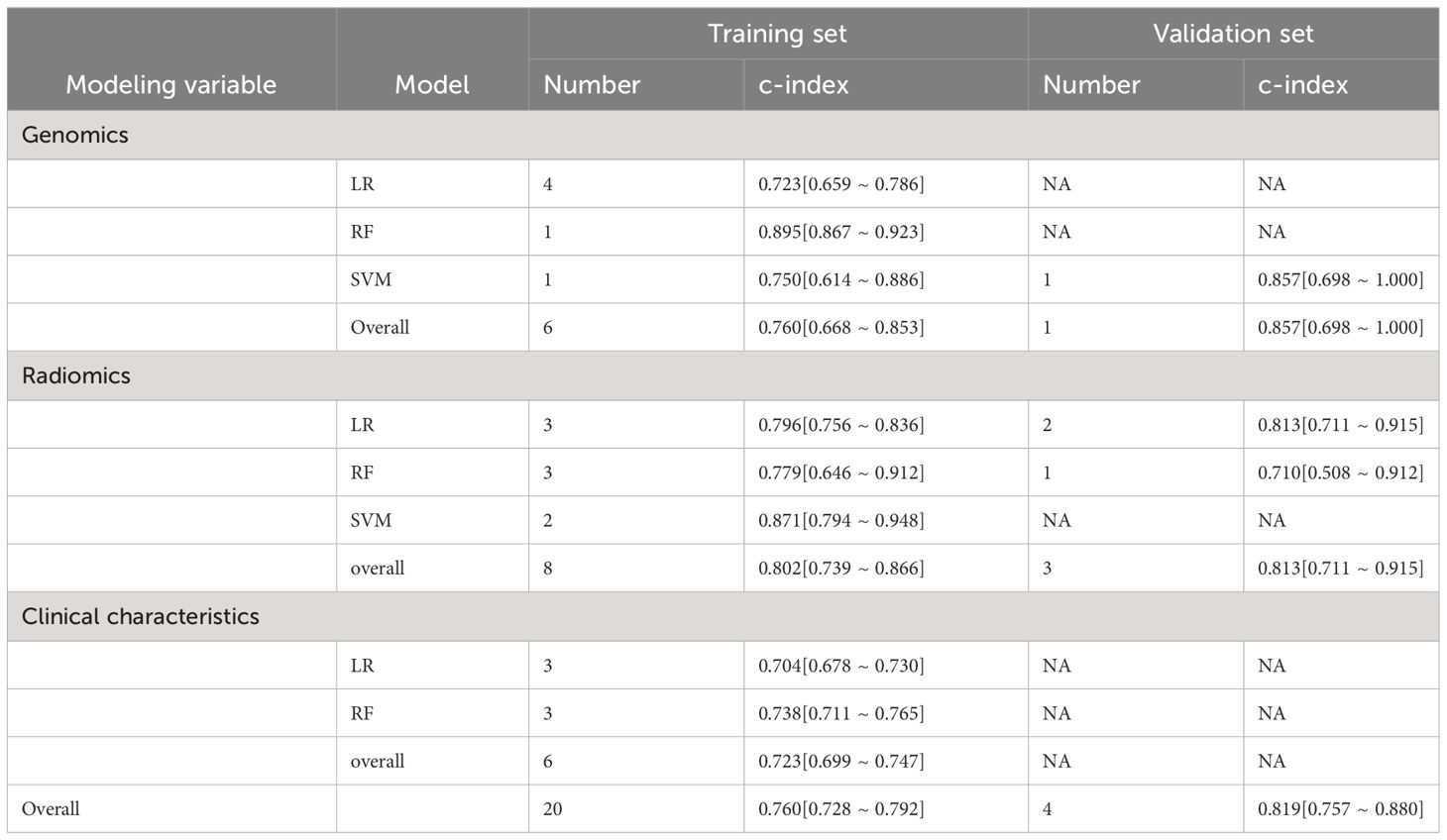
Table 3 Meta-analysis of c-index of machine learning in treatment response prediction for ICI-treated melanoma patients.
Only genomics-based machine learning models were included for PFS prediction, but a validation set was lacking for the calculation of c-index. The overall c-index for PFS was 0.728 (95%CI: 0.629–0.828) in the training set (Table 4).
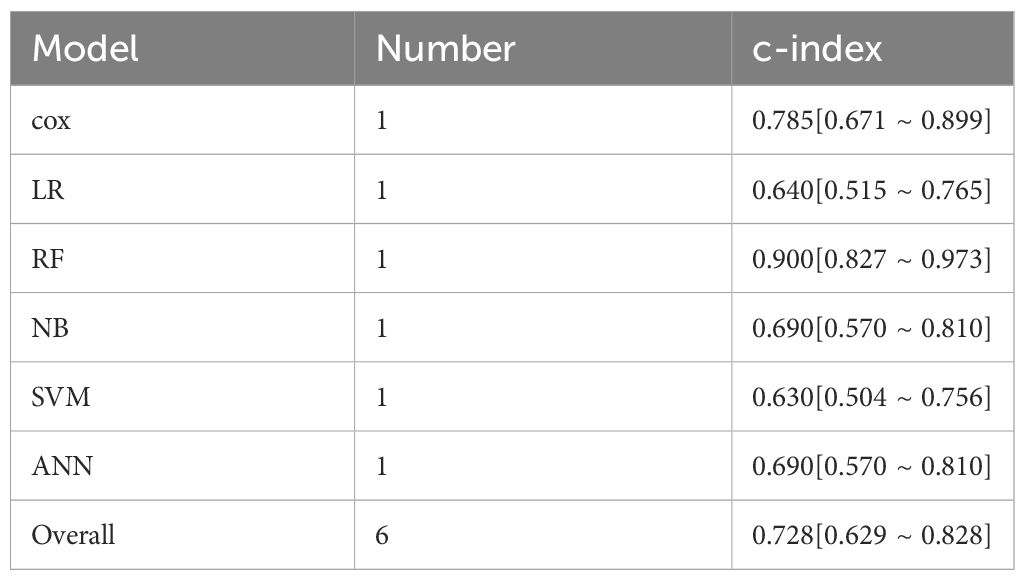
Table 4 Meta-analysis of c-index of machine learning in PFS prediction for ICI-treated melanoma patients.
4 Discussion
4.1 Summary of the main findings
We performed a meta-analysis of the outcome measures of melanoma patients receiving ICI from 36 studies, and the pooled c-index showed that machine learning exhibits considerable predictive accuracy in melanoma immunotherapy response and prognosis. Our results showed that the genomics-based logistic regression model had the best performance in OS prediction [c-index 0.882 (95%CI: 0.835–0.929)], while the genomics-based random forest plot model performed best in treatment response and PS prediction [c-index 0.895 (95%CI: 0.867–0.923) and 0.900 (95%CI: 0.827–0.973), respectively]. Overall, we found that machine learning had the highest predictive accuracy for immunotherapy response in melanoma patients [0.760 (95%CI: 0.728–0.792) in the training set and 0.819 (95%CI: 0.757–0.880) in the validation set].
4.2 Comparison with previous reviews
Several studies have investigated the application of AI in the treatment and management of advanced melanoma. A review by Guerrisi et al. has discussed the application significance and challenges of AI in melanoma treatment but failed to assess the risk of bias and accuracy of existing machine learning models (51). Valenti and colleagues have provided an overview of the application of multi-omics (genomics, transcriptomics, proteomics, metabolomics and radiomics) in evaluating the immunotherapy response of melanoma. Many of these multi-omics models were highly dependent on machine learning, and their accuracy was not systematically described (52). Furthermore, another systematic review by Guerrisi et al. revealed that radiomics is a new robust approach for predicting targeted therapy and immunotherapy of melanoma (53). Though, none of these reviews has quantitatively assessed the predictive accuracy of the various machine learning models in the immunotherapy response and prognosis of melanoma. To fill in this gap, our systematic review has quantitatively determined the predictive accuracy of relevant radiomics-, genomics-, and clinical features-based machine learning models in order to provide valuable insights into subsequent development of AI tools.
4.3 Modeling variables
The selection of modeling variables is critical for machine learning. Common modeling variables for cancer prediction models are genomics, transcriptomics, proteomics, metabolomics, radiomics, and clinical features. In particular, clinical features often play an important role in model construction due to their ease of acquisition and good interpretability. Therefore, clinical features-based models are viewed as highly valuable by clinicians in predicting tumor treatment response or prognosis, especially in solid tumor prognosis. Moreover, machine learning models that integrate tumor stages have been shown to demonstrate superior predictive accuracy than other omics-based models. The emergence of ICIs has completely changed the treatment modality and prolonged the PFS and OS of melanoma patients (54). Despite the success of immunotherapy, treatment response and prognosis still vary greatly among patients, rendering many patients unable to benefit from these treatments. At present, PFS and OS predictions are still primarily based on clinical features. Our results showed that clinical features-, radiomics- and genomics-based machine learning models all exhibit favorable predictive accuracy in melanoma treatment response and prognosis. In particular, radiomics-based models have demonstrated promising application prospects in treatment response prediction in cancer patients, which is consistent with the findings by Zhang et al (55).
4.5 Limitations
There are several limitations to this study. First, due to practical reasons and the lack of external validation, the c-index of the training set was relatively high. Second, the low number of certain types of models in the included studies impeded the assessment of their predictive accuracy. Last, only a few of the included studies reported a diagnostic continency table or sensitivity and specificity for predicting treatment response, OS and PFS. Therefore, sensitivity and specificity will need to be further analyzed in subsequent studies.
4.6 Future prospects
(1) Various adverse events (AEs) have been reported in melanoma patients receiving ICIs. Hence, early prediction of ICI-related AEs has important clinical implications in treatment decision-making. However, very few published studies have investigated the predictive performance of machine learning in AEs. Therefore, the performance of machine learning models in predicting the risk of immunotherapy-related AEs in melanoma should be further examined in future work (2). The selection of modeling variables is critical for machine learning. We hope to identify more efficient and convenient predictors in our subsequent studies (3). Although our study showed that radiomics-based models have favorable predictive accuracy in immunotherapy response and prognosis of melanoma, radiomics faces many drawbacks such as over-configuration of imaging equipment, segmentation specificity of regions of interest, diversity in the modeling process, and lack of external validation. Thus, the development of a rational guideline for standardizing the implementation of radiomics research is warranted.
5 Conclusion
Machine learning models, especially those based on radiomics, have promising predictive accuracy in melanoma immunotherapy response and prognosis. Radiomics has gained wide interest in the research community in recent years, and hence standardization of radiomics research is critical for minimizing the risks of heterogeneity and bias.
Data availability statement
The original contributions presented in the study are included in the article/Supplementary material. Further inquiries can be directed to the corresponding author.
Author contributions
JL: Data curation, Formal analysis, Investigation, Methodology, Writing – original draft, Writing – review & editing. KD: Data curation, Formal analysis, Investigation, Methodology, Writing – original draft, Writing – review & editing. JA: Conceptualization, Formal analysis, Writing – original draft, Writing – review & editing.
Funding
The author(s) declare that no financial support was received for the research, authorship, and/or publication of this article.
Acknowledgments
We would like to thank the researchers and study participants for their contributions.
Conflict of interest
The authors declare that the research was conducted in the absence of any commercial or financial relationships that could be construed as a potential conflict of interest.
Publisher’s note
All claims expressed in this article are solely those of the authors and do not necessarily represent those of their affiliated organizations, or those of the publisher, the editors and the reviewers. Any product that may be evaluated in this article, or claim that may be made by its manufacturer, is not guaranteed or endorsed by the publisher.
Supplementary material
The Supplementary Material for this article can be found online at: https://www.frontiersin.org/articles/10.3389/fimmu.2024.1281940/full#supplementary-material
References
1. Ascierto PA, Eggermont AMM. Neoadjuvant therapy in melanoma: the next step? Lancet Oncol. (2018) 19:151–3. doi: 10.1016/s1470–2045(18)30016–0
2. Bertolotto C. Melanoma: from melanocyte to genetic alterations and clinical options. Scientifica. (2013) 2013:635203. doi: 10.1155/2013/635203
3. Gandini S, Autier P, Boniol M. Reviews on sun exposure and artificial light and melanoma. Prog biophys Mol Biol. (2011) 107:362–6. doi: 10.1016/j.pbiomolbio.2011.09.011
4. Miller KD, Siegel RL, Lin CC, Mariotto AB, Kramer JL, Rowland JH, et al. Cancer treatment and survivorship statistics, 2016. CA: Cancer J Clin. (2016) 66:271–89. doi: 10.3322/caac.21349
5. Ward WH, Farma JM. Cutaneous melanoma: etiology and therapy. Brisbane (AU: Codon Publications (2017). doi: 10.15586/codon.cutaneousmelanoma.2017
6. Carlino MS, Larkin J, Long GV. Immune checkpoint inhibitors in melanoma. Lancet (London England). (2021) 398:1002–14. doi: 10.1016/S0140-6736(21)01206-X
7. SChadendorf D, van Akkooi ACJ, Berking C, Griewank KG, Gutzmer R, Hauschild A, et al. Melanoma. Lancet (London England). (2018) 392:971–84. doi: 10.1016/s0140–6736(18)31559–9
8. SChadendorf D, Hodi FS, Robert C, Weber JS, Margolin K, Hamid O, et al. Pooled analysis of long-term survival data from phase ii and phase iii trials of ipilimumab in unresectable or metastatic melanoma. J Clin Oncol. (2015) 33:1889–94. doi: 10.1200/jco.2014.56.2736
9. Lee KA, Thomas AM, Bolte LA, Björk JR, de Ruijter LK, Armanini F, et al. Cross-cohort gut microbiome associations with immune checkpoint inhibitor response in advanced melanoma. Nat Med. (2022) 28:535–44. doi: 10.1038/s41591–022-01695–5
10. Ugurel S, SChadendorf D, Horny K, Sucker A, Schramm S, Utikal J, et al. Elevated baseline serum pd-1 or pd-L1 predicts poor outcome of pd-1 inhibition therapy in metastatic melanoma. Ann Oncol. (2020) 31:144–52. doi: 10.1016/j.annonc.2019.09.005
11. Handelman GS, Kok HK, Chandra RV, Razavi AH, Lee MJ, Asadi H. Edoctor: machine learning and the future of medicine. J Internal Med. (2018) 284:603–19. doi: 10.1111/joim.12822
12. Liberati A, Altman DG, Tetzlaff J, Mulrow C, Gøtzsche PC, Ioannidis JP, et al. The prisma statement for reporting systematic reviews and meta-analyses of studies that evaluate healthcare interventions: explanation and elaboration. BMJ. (2009) 339:b2700. doi: 10.1136/bmj.b2700
13. Moons KG, de Groot JA, Bouwmeester W, Vergouwe Y, Mallett S, Altman DG, et al. Critical appraisal and data extraction for systematic reviews of prediction modelling studies: the charms checklist. PloS Med. (2014) 11:e1001744. doi: 10.1371/journal.pmed.1001744
14. Wolff RF, Moons KGM, Riley RD, Whiting PF, Westwood M, Collins GS, et al. Probast: A tool to assess the risk of bias and applicability of prediction model studies. Ann Internal Med. (2019) 170:51–8. doi: 10.7326/m18–1376
15. Debray TP, Damen JA, Riley RD, Snell K, Reitsma JB, Hooft L, et al. A framework for meta-analysis of prediction model studies with binary and time-to-event outcomes. Stat Methods Med Res. (2019) 28:2768–86. doi: 10.1177/0962280218785504
16. Hu G, Jiang Y, Ma J, Zhan H. Necroptosis-associated classification combined with tumor microenvironment characteristic analysis of cutaneous melanoma. Sci Rep. (2022) 12:8752. doi: 10.1038/s41598–022-12676–6
17. Tian L, Long F, Hao Y, Li B, Li Y, Tang Y, et al. A cancer associated fibroblasts-related six-gene panel for anti-pd-1 therapy in melanoma driven by weighted correlation network analysis and supervised machine learning. Front Med. (2022) 9:880326. doi: 10.3389/fmed.2022.880326
18. Xie K, Peng Y, Zhong W, Liu X. Kmt2c is a potential biomarker of anti-pd-1 treatment response in metastatic melanoma. Front bioscience (Landmark edition). (2022) 27:103. doi: 10.31083/j.fbl2703103
19. Xie Y, Zhang J, Li M, Zhang Y, Li Q, Zheng Y, et al. Identification of lactate-related gene signature for prediction of progression and immunotherapeutic response in skin cutaneous melanoma. Front Oncol. (2022) 12:818868. doi: 10.3389/fonc.2022.818868
20. Mao R, Yang F, Zhang T, Li J. Landscape and clinical significance of immune checkpoint in cutaneous melanoma. Front Immunol. (2021) 12:756282. doi: 10.3389/fimmu.2021.756282
21. Wu L, Hu X, Dai H, Chen K, Liu B. Identification of an M6a regulators-mediated prognosis signature for survival prediction and its relevance to immune infiltration in melanoma. Front Cell Dev Biol. (2021) 9:718912. doi: 10.3389/fcell.2021.718912
22. Xu Z, Xie Y, Mao Y, Huang J, Mei X, Song J, et al. Ferroptosis-related gene signature predicts the prognosis of skin cutaneous melanoma and response to immunotherapy. Front Genet. (2021) 12:758981. doi: 10.3389/fgene.2021.758981
23. Chen X, Zhou M, Wang Z, Lu S, Chang S, Zhou Z. Immunotherapy treatment outcome prediction in metastatic melanoma through an automated multi-objective delta-radiomics model. Comput Biol Med. (2021) 138:104916. doi: 10.1016/j.compbiomed.2021.104916
24. Zhou S, Sun Y, Chen T, Wang J, He J, Lyu J, et al. The landscape of the tumor microenvironment in skin cutaneous melanoma reveals a prognostic and immunotherapeutically relevant gene signature. Front Cell Dev Biol. (2021) 9:739594. doi: 10.3389/fcell.2021.739594
25. Li G, Zhu X, Liu C. Characterization of immune infiltration and construction of a prediction model for overall survival in melanoma patients. Front Oncol. (2021) 11:639059. doi: 10.3389/fonc.2021.639059
26. Zeng Y, Zeng Y, Yin H, Chen F, Wang Q, Yu X, et al. Exploration of the immune cell infiltration-related gene signature in the prognosis of melanoma. Aging. (2021) 13:3459–82. doi: 10.18632/aging.202279
27. Wang X, Xu W, Chi Z, Si L, Sheng X, Kong Y, et al. Chemotherapy combined with antiangiogenic drugs as salvage therapy in advanced melanoma patients progressing on pd-1 immunotherapy. Trans Oncol. (2021) 14:100949. doi: 10.24112/ijccpm.191949
28. Sheng Y, Tong L, Geyu L. An immune risk score with potential implications in prognosis and immunotherapy of metastatic melanoma. Int Immunopharmacol. (2020) 88:106921. doi: 10.1016/j.intimp.2020.106921
29. Tian M, Yang J, Han J, He J, Liao W. A novel immune checkpoint-related seven-gene signature for predicting prognosis and immunotherapy response in melanoma. Int Immunopharmacol. (2020) 87:106821. doi: 10.1016/j.intimp.2020.106821
30. Kang K, Xie F, Mao J, Bai Y, Wang X. Significance of tumor mutation burden in immune infiltration and prognosis in cutaneous melanoma. Front Oncol. (2020) 10:573141. doi: 10.3389/fonc.2020.573141
31. Wang ZL, Mao LL, Zhou ZG, Si L, Zhu HT, Chen X, et al. Pilot study of ct-based radiomics model for early evaluation of response to immunotherapy in patients with metastatic melanoma. Front Oncol. (2020) 10:1524. doi: 10.3389/fonc.2020.01524
32. Wang X, Chai Z, Li Y, Long F, Hao Y, Pan G, et al. Identification of potential biomarkers for anti-pd-1 therapy in melanoma by weighted correlation network analysis. Genes. (2020) 11:435. doi: 10.3390/genes11040435
33. Li D, Duan H, Jiang P, Jiang X, He Z, Guo C, et al. Trend and socioeconomic disparities in survival outcome of metastatic melanoma after approval of immune checkpoint inhibitors: A population-based study. Am J Trans Res. (2020) 12:3767–79.
34. Filipski K, Scherer M, Zeiner KN, Bucher A, Kleemann J, Jurmeister P, et al. DNA methylation-based prediction of response to immune checkpoint inhibition in metastatic melanoma. J ImmunoTher Cancer. (2021) 9:e002226. doi: 10.1136/jitc-2020–002226
35. Schraag A, Klumpp B, Afat S, Gatidis S, Nikolaou K, Eigentler TK, et al. Baseline clinical and imaging predictors of treatment response and overall survival of patients with metastatic melanoma undergoing immunotherapy. Eur J Radiol. (2019) 121:108688. doi: 10.1016/j.ejrad.2019.108688
36. Brendlin AS, Peisen F, Almansour H, Afat S, Eigentler T, Amaral T, et al. A machine learning model trained on dual-energy ct radiomics significantly improves immunotherapy response prediction for patients with stage iv melanoma. J immunother Cancer. (2021) 9:e003261. doi: 10.1136/jitc-2021–003261
37. Peisen F, Hänsch A, Hering A, Brendlin AS, Afat S, Nikolaou K, et al. Combination of whole-body baseline ct radiomics and clinical parameters to predict response and survival in a stage-iv melanoma cohort undergoing immunotherapy. Cancers. (2022) 14:2992. doi: 10.3390/cancers14122992
38. Almansour H, Afat S, Serna-Higuita LM, Amaral T, Schraag A, Peisen F, et al. Early Tumor Size Reduction of at Least 10% at the First Follow-up Computed Tomography Can Predict Survival in the Setting of Advanced Melanoma and Immunotherapy. Acad Radiol. (2022) 29:514–22. doi: 10.1016/j.acra.2021.04.015
39. Marsavela G, Lee J, Calapre L, Wong SQ, Pereira MR, McEvoy AC, et al. Circulating tumor DNA predicts outcome from first-, but not second-line treatment and identifies melanoma patients who may benefit from combination immunotherapy. Clin Cancer Res. (2020) 26:5926–33. doi: 10.1158/1078–0432.CCR-20–2251
40. Da Silva IP, Ahmed T, McQuade JL, Nebhan CA, Park JJ, Versluis JM, et al. Clinical models to define response and survival with anti-pd-1 antibodies alone or combined with ipilimumab in metastatic melanoma. J Clin Oncol. (2022) 40:1068–80. doi: 10.1200/JCO.21.01701
41. Diem S, Kasenda B, Martin-Liberal J, Lee A, Chauhan D, Gore M, et al. Prognostic score for patients with advanced melanoma treated with ipilimumab. Eur J Cancer. (2015) 51:2785–91. doi: 10.1016/j.ejca.2015.09.007
42. Flaus A, Habouzit V, De Leiris N, Vuillez JP, Leccia MT, Simonson M, et al. Outcome prediction at patient level derived from pre-treatment 18f-fdg pet due to machine learning in metastatic melanoma treated with anti-pd1 treatment. Diagnostics. (2022) 12:388. doi: 10.3390/diagnostics12020388
43. Ungan G, Lavandier AF, Rouanet J, Hordonneau C, Chauveau B, Pereira B, et al. Metastatic melanoma treated by immunotherapy: discovering prognostic markers from radiomics analysis of pretreatment ct with feature selection and classification. Int J Comput Assisted Radiol Surg. (2022) 17:1867–77. doi: 10.1007/s11548–022-02662–8
44. Madonna G, Masucci GV, Capone M, Mallardo D, Grimaldi AM, Simeone E, et al. Clinical categorization algorithm (Clical) and machine learning approach (Srf-clical) to predict clinical benefit to immunotherapy in metastatic melanoma patients: real-world evidence from the istituto nazionale tumori irccs fondazione pascale, napoli, Italy. Cancers. (2021) 13:4164. doi: 10.3390/cancers13164164
45. Indini A, Di Guardo L, Cimminiello C, Prisciandaro M, Randon G, De Braud F, et al. Developing a score system to predict therapeutic outcomes to anti-pd-1 immunotherapy in metastatic melanoma. Tumori. (2019) 105:465–73. doi: 10.1177/0300891619868009
46. Basler L, Gabryś HS, Hogan SA, Pavic M, Bogowicz M, Vuong D, et al. Radiomics, tumor volume, and blood biomarkers for early prediction of pseudoprogression in patients with metastatic melanoma treated with immune checkpoint inhibition. Clin Cancer Res. (2020) 26:4414–25. doi: 10.1158/1078–0432.CCR-20–0020
47. Burgermeister S, Gabryś HS, Basler L, Hogan SA, Pavic M, Bogowicz M, et al. Improved survival prediction by combining radiological imaging and S-100b levels into a multivariate model in metastatic melanoma patients treated with immune checkpoint inhibition. Front Oncol. (2022) 12:830627. doi: 10.3389/fonc.2022.830627
48. Dercle L, Zhao B, Gönen M, Moskowitz CS, Firas A, Beylergil V, et al. Early readout on overall survival of patients with melanoma treated with immunotherapy using a novel imaging analysis. JAMA Oncol. (2022) 8:385–92. doi: 10.1001/jamaoncol.2021.6818
49. Kelly D, Rose AAN, Muniz TP, Hogg D, Butler MO, Saibil SD, et al. Development of a metastatic uveal melanoma prognostic score (Mumps) for use in patients receiving immune checkpoint inhibitors. Cancers. (2021) 13:3640. doi: 10.3390/cancers13143640
50. Karlsson MJ, Svedman FC, Tebani A, Kotol D, Hoiom V, Fagerberg L, et al. Inflammation and apolipoproteins are potential biomarkers for stratification of cutaneous melanoma patients for immunotherapy and targeted therapy. Cancer Res. (2021) 81:2545–55. doi: 10.1158/0008–5472.CAN-20–2000
51. Guerrisi A, Falcone I, Valenti F, Rao M, Gallo E, Ungania S, et al. Artificial intelligence and advanced melanoma: treatment management implications. Cells. (2022) 11:3965. doi: 10.3390/cells11243965
52. Valenti F, Falcone I, Ungania S, Desiderio F, Giacomini P, Bazzichetto C, et al. Precision medicine and melanoma: multi-omics approaches to monitoring the immunotherapy response. Int J Mol Sci. (2021) 22:3837. doi: 10.3390/ijms22083837
53. Guerrisi A, Loi E, Ungania S, Russillo M, Bruzzaniti V, Elia F, et al. Novel cancer therapies for advanced cutaneous melanoma: the added value of radiomics in the decision making process-a systematic review. Cancer Med. (2020) 9:1603–12. doi: 10.1002/cam4.2709
54. Lugowska I, Teterycz P, Rutkowski P. Immunotherapy of melanoma. Contemp Oncol (Poznan Poland). (2018) 22:61–7. doi: 10.5114/wo.2018.73889
Keywords: machine learning, prediction, melanoma, immune checkpoint inhibitor, meta-analysis
Citation: Li J, Dan K and Ai J (2024) Machine learning in the prediction of immunotherapy response and prognosis of melanoma: a systematic review and meta-analysis. Front. Immunol. 15:1281940. doi: 10.3389/fimmu.2024.1281940
Received: 23 August 2023; Accepted: 08 May 2024;
Published: 21 May 2024.
Edited by:
Jianbin Bi, The First Hospital of China Medical University, ChinaReviewed by:
Peng Ren, Southwest University of Science and Technology, ChinaXiaoyu Hu, Hospital of Chengdu University of Traditional Chinese Medicine, China
Liyuan Hao, Chengdu University of Traditional Chinese Medicine, China, in collaboration with reviewer XH
Copyright © 2024 Li, Dan and Ai. This is an open-access article distributed under the terms of the Creative Commons Attribution License (CC BY). The use, distribution or reproduction in other forums is permitted, provided the original author(s) and the copyright owner(s) are credited and that the original publication in this journal is cited, in accordance with accepted academic practice. No use, distribution or reproduction is permitted which does not comply with these terms.
*Correspondence: Jun Ai, YWlqdW4xOTg3OTI1QDE2My5jb20=
†These authors have contributed equally to this work