- Department of Critical Care Medicine, Ruijin Hospital, Shanghai Jiao Tong University School of Medicine, Shanghai, China
Background: It is unclear what the causal relationship is between the gut microbiota and sepsis. Therefore, we employed Mendelian randomization (MR) to determine whether a causal link exists between the two.
Methods: This study used publicly available genome-wide association studies (GWAS) summary data of gut microbiota, sepsis, sepsis (critical care), and sepsis (28-day death in critical care) to perform a two-sample MR analysis. To ensure the robustness of the results, we also conducted a sensitivity analysis.
Results: For sepsis susceptibility, inverse variance weighted (IVW) estimates revealed that Victivallales (OR = 0.86, 95% CI, 0.78–0.94, p = 0.0017) was protective against sepsis, while Lentisphaerae (OR = 0.89, 95% CI, 0.80–0.99), Gammaproteobacteria (OR = 1.37, 95% CI, 1.08–1.73), Clostridiaceae1 (OR = 1.21, 95% CI, 1.04–1.40), RuminococcaceaeUCG011 (OR = 1.10, 95% CI, 1.01–1.20), Dialister (OR = 0.85, 95% CI, 0.74–0.97), and Coprococcus2 (OR = 0.81, 95% CI, 0.69–0.94) presented a suggestive association with the development of sepsis (all p < 0.05). For sepsis (critical care), IVW estimates indicated that Lentisphaerae (OR = 0.70, 95% CI, 0.53–0.93), Victivallales (OR = 0.67, 95% CI, 0.50–0.91), Anaerostipes (OR = 0.49, 95% CI, 0.31–0.76), LachnospiraceaeUCG004 (OR = 0.51, 95% CI, 0.34–0.77), and Coprococcus1 (OR = 0.66, 95% CI, 0.44–0.99) showed a suggestive negative correlation with sepsis (critical care) (all p < 0.05). For sepsis (28-day death in critical care), IVW estimates suggested that four bacterial taxa had a normally significant negative correlation with the risk of sepsis-related death, including Victivallales (OR = 0.54, 95% CI, 0.30–0.95), Coprococcus2 (OR = 0.34, 95% CI, 0.14–0.83), Ruminiclostridium6 (OR = 0.43, 95% CI, 0.22–0.83), and Coprococcus1 (OR = 0.45, 95% CI, 0.21–0.97), while two bacterial taxa were normally significantly positively linked to the risk of sepsis-related death, namely, Mollicutes (OR = 2.03, 95% CI, 1.01–4.08) and Bacteroidales (OR = 2.65, 95% CI, 1.18–5.96) (all p < 0.05). The robustness of the above correlations was verified by additional sensitivity analyses.
Conclusion: This MR research found that several gut microbiota taxa were causally linked to the risk of sepsis, sepsis in critical care, and sepsis-related 28-day mortality in critical care.
Introduction
Sepsis is an organ dysfunction that is described as a life-threatening disorder deriving from a dysfunctional host response to infection. According to pertinent data, 30 million cases of sepsis occur annually worldwide, with a high mortality rate of 16% to 33% (1, 2). A key step in improving the prognosis of sepsis is early identification and treatment. Blood culture is frequently employed as the diagnostic standard for sepsis. However, it is time-consuming and has a poor positive rate (3). At present, no specific therapeutic drug exists for sepsis, which is managed through a combination of antibiotic therapy, organ protection, and fluid resuscitation. Hence, it is imperative to identify reliable early detection indicators as well as exact therapy targets for sepsis.
Gut microbiota is the collection of all microorganisms that colonize the gastrointestinal system and influence basic body functions such as digestion, energetic metabolism, and immune response (4). A growing number of studies have shown a link between gut microbiota dysbiosis and sepsis, which were mainly manifested as a decrease in beneficial bacteria and an increase in pathogenic bacteria (5–11). For example, Sun et al. discovered that the relative abundance of the Proteobacteria phylum and Enterococcaceae family was significantly elevated in sepsis patients compared to healthy controls, whereas the Firmicutes phylum and Lachnospiraceae family exhibited a relatively lower abundance (11). Furthermore, a retrospective cohort study of 10,996 participants by Prescott et al. suggested that intestinal microbial disturbances increased the risk of sepsis-related hospitalization and were associated with the severity of sepsis (7). Similar effects of gut microbiota exhaustion and loss of diversity to death rates have also been displayed in several sepsis experimental models (12, 13). However, the current associations were largely on the basis of observational studies with confounding factors, and the causality between gut microbiota and sepsis is not clear and requires more direct evidence.
Mendelian randomization (MR) is a type of instrumental variable (IV) analysis that employs genetic variations to determine the causality between exposure and outcome (14). The most common genetic variants are single-nucleotide polymorphisms (SNPs), which are randomly assigned to offspring along with gametes and occur before disease onset, and thus, MR is less susceptible to confounding factors and reverse causation. To date, despite several MR analyses investigating the causal relationship between gut microbiota and sepsis, the selection of genome-wide association studies (GWAS) data for sepsis outcomes is not entirely consistent (15, 16). The GWAS outcome data for sepsis selected by Chen et al. were derived from the 2020 UK Biobank (10,154 cases; 452,764 controls), and these are not the most up-to-date GWAS data available (15), while the study conducted by Zhang et al. incorporated various sepsis outcome GWAS data, which included patients below 75 years, 28-day mortality, critical care units (ICU), and 28-day mortality in ICU. However, they did not opt for the most recent sepsis outcome data available (16). Therefore, we chose to re-perform the MR analysis of gut flora and sepsis using the most comprehensive and up-to-date sepsis GWAS outcome data to investigate the causal relationship between gut microbiota and the risk of sepsis and sepsis-related death, elucidating the etiology of sepsis and suggesting innovative ideas for earlier diagnosis and treatment of this disease.
Materials and methods
Study design
Employing summary statistics derived from GWAS, we conducted two-sample MR analyses to evaluate the causality of the gut microbiota on the risk of sepsis, sepsis requiring critical care, and sepsis (28-day death in critical care). IVs are chosen to meet three assumptions: IVs are required to be strongly associated with the exposure of interest; IVs must be independent of unmeasured confounders; and IVs influence outcomes only through the exposure of interest (17). In this MR study, gut microbiota, and sepsis, sepsis (critical care), and sepsis (28-day death in critical care) were used as exposure and outcome, respectively. A flowchart presenting the whole procedure is shown in Figure 1. The present study used openly de-identified data from participant studies that were approved by the ethical standards committee for human experimentation. This study did not require separate ethical approval.
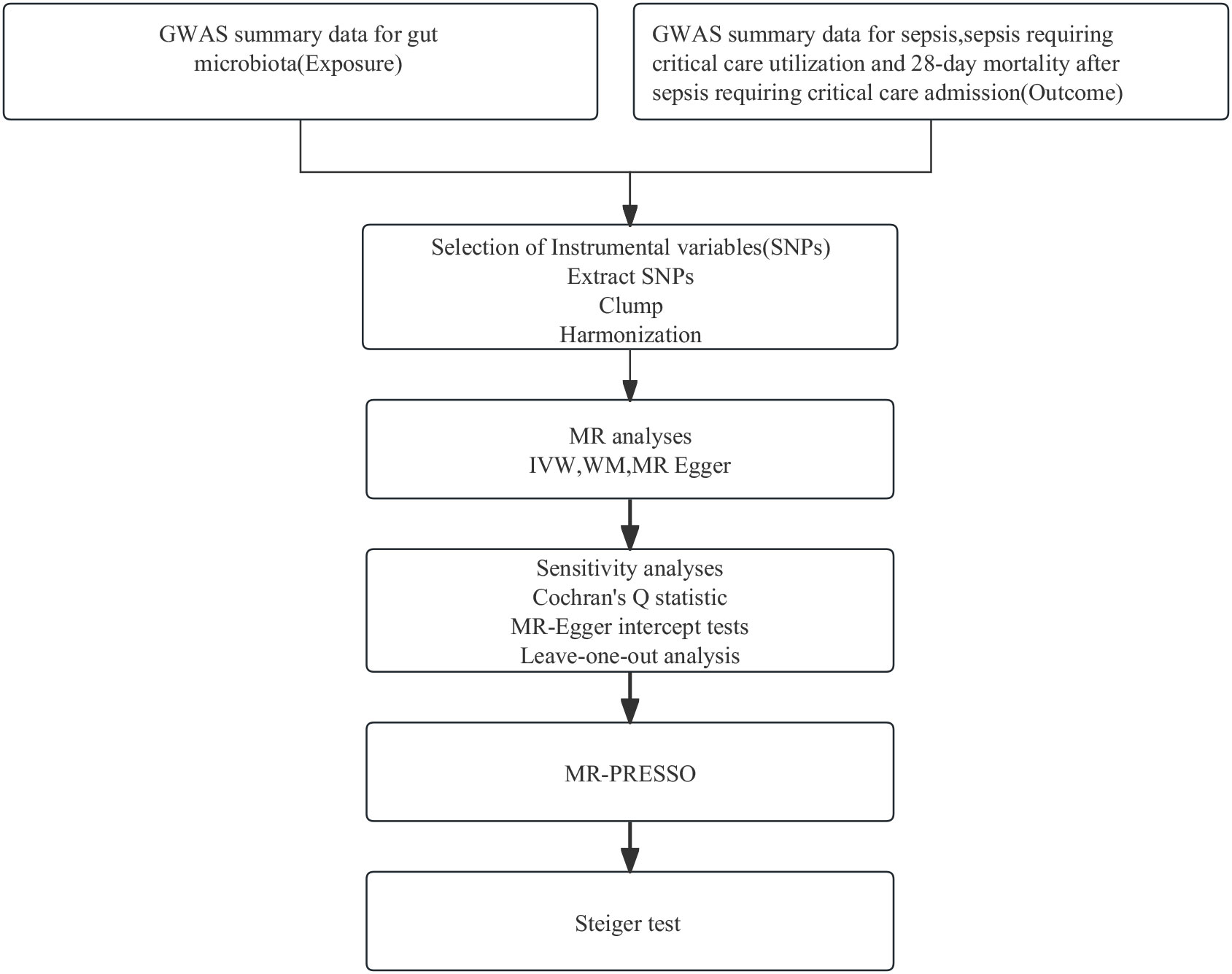
Figure 1 The analysis process of our research. GWAS, genome-wide association study; MR, Mendelian randomization; SNPs, single-nucleotide polymorphisms; IVW, inverse variance weighted; WM, weighted median; MR-PRESSO, Mendelian Randomization Pleiotropy RESidual Sum and Outlier.
Gut microbiota sample
Summary statistics pertaining to the human gut microbiota composition were derived from a comprehensive GWAS meta-analysis encompassing 24 cohorts derived from ethnically diverse backgrounds, including the United States, Canada, Israel, Korea, Germany, Denmark, the Netherlands, Belgium, Sweden, Finland, and the United Kingdom (N = 18,340). Among these, 20 cohorts included a sole representative sample, with the majority of participants being of European descent (16 cohorts, N = 13,226). A total of 122,110 variant sites for 211 taxa (9 phyla, 16 classes, 20 orders, 35 families, and 131 genera) were identified. Adjustments were made for sex, age, first 10 principal components and genotyping batch during the analysis (18). Summary-level statistics for the associated studies can be found on the website (https://mibiogen.gcc.rug.nl).
Sepsis samples
Summary statistics for sepsis phenotypes were extracted from the IEU OpenGWAS, while summary-level data from the UK Biobank were also utilized (https://gwas.mrcieu.ac.uk/). The UK Biobank is an extensive cohort of UK adult participants; details were found in other sections (19). Sepsis phenotypes included sepsis (total cases: 11,643; total controls: 474,841), sepsis requiring critical care (total cases: 1,380; total controls: 429,985), and sepsis-related 28-day mortality in critical care (total cases: 347; total controls: 431,018). In the hospital episode statistics provided by the UK Biobank, cases were incorporated when the code was in the primary or secondary diagnostic category, adjusted for age, gender, microchip, and the first 10 principal components using regenie v2.2.4 (20). Sepsis admissions were identified by ICD codes from the UK Biobank linking secondary care data. In line with existing literature, ICD-10 codes A02, A39, A40, and A41 were used to identify sepsis (21). All study participants were of European ancestry.
Selection of IVs
Five levels of assessment of bacterial taxa (phylum, order, order, family, and genus) were performed. As the eligible number of IVs was extremely small (p < 5×10−8), a relatively high threshold (p < 1×10−5) was used, which was in line with Ni et al. (22). In parallel, we performed quality control according to the following steps: First, the linkage disequilibrium (LD) threshold was set as 0.001, and the clumping window was 10 Mb. Second, we computed the F-statistic to quantify the genetic variation strength and then abandoned SNPs with an F-statistic of less than 10, indicating insufficient strength (23). Third, data were harmonized between gut microbiota and sepsis datasets, and SNPs with minor allele frequency (MAF)≤0.01, ambiguities, and palindromes were excluded. Ultimately, the gut microbiota linked to the outcome (p < 1.0×10−5) and gut microbiota with fewer than three SNPs were eliminated from the analysis. Following the above steps, the remaining SNPs were eventually used as IVs.
Primary analysis
For the primary analysis, we employed random-effects inverse variance weighted (IVW) estimation, which combines the Wald ratio of each SNP to the outcome, assuming all genetic variations are valid. This approach offers the highest power for MR estimation, yet it is susceptible to multidirectional bias (24). Therefore, IVW was used as the primary method to estimate the cause and effect of gut microbiota on the risk of sepsis, sepsis requiring critical care, and sepsis-related 28-day mortality in critical care.
Sensitivity analysis
Sensitivity analyses were subsequently performed to assess the bias of the MR assumptions for the identified significant estimates (pIVW < 0.05). Some other MR analyses, such as weighted median and MR-Egger regression, were also used as complementary methods. MR-Egger regressions can test for multiplicity and considerable heterogeneity of imbalances, whereas for the same change in underexposure, larger sample sizes are required (25). In situations where at least half of the weighted variance provided by the horizontal pleated product effect is valid, the weighted median method offers consistent estimates of the effect (26). In addition, sensitivity analyses are also critical in MR studies to evaluate any bias of the MR assumptions. Consequently, Cochran’s Q statistic, MR-Egger intercept tests, and leave-one-out (LOO) analyses are employed to identify the presence of heterogeneity and pleiotropy, as well as evaluate the robustness of the obtained results. In particular, Mendelian Randomization Pleiotropy RESidual Sum and Outlier (MR-PRESSO) tests were conducted to test for outliers with potential horizontal pleiotropy. MR-PRESSO enables the estimation of SNP levels and overall heterogeneity, facilitating the detection of horizontal pleiotropy. In contrast, the outlier test comparing expected and observed variant distributions identifies outlier variants. Should any outliers be discovered, they are eliminated to produce unbiased causal estimates from the outlier-corrected MR analysis (27).
Hence, potential eligible candidate gut microbiome for participation in sepsis development was identified as follows: (1) pIVW < 0.05; (2) the direction and amplitude of the three MR methods were consistent; (3) no heterogeneity or multiplicity was observed; and (4) no high-impact points were noted in the LOO analyses. The significant thresholds for each level were adjusted for multiple testing as follows: phylum p = 5.56 × 10−3 (0.05/9), class p = 3.13×10−3 (0.05/16), order p = 2.50 ×10−3 (0.05/20), family p = 1.43 × 10−3 (0.05/35), and genus p = 3.82 × 10−4 (0.05/131).
A nominal significant association was considered for pIVW < 0.05 but exceeding the Bonferroni-corrected threshold. All analyses were run by using the R package TwoSampleMR (version 0.5.6) in R (version 4.1.1).
Confounding analysis and Steiger test
Despite employing various statistical approaches in sensitivity analyses to investigate potential violations of MR assumptions, we also utilized the Phenoscanner V2 website (http://www.phenoscanner.medchsl.cam.ac.uk/) to examine whether gut microbial-related SNPs were concurrently associated with multiple common risk factors that might influence MR estimates, including sex (28), obesity (29), and diabetes (30). If the correlation between SNPs and these potential confounders reached a threshold value of p < 1×10−5, IVW was reiterated following the removal of these SNPs to validate the robustness of the findings. In addition, the MR Steiger test was performed on bacteria found to be causally related to sepsis, sepsis requiring critical care, and sepsis-related 28-day mortality in critical care, to verify the directionality of the results due to exposure, and p < 0.05 was considered statistically significant (31).
Reporting guidelines
This study followed the guidelines of the Strengthening the Reporting of Observational Studies in Epidemiology Using MR (STROBE-MR), a checklist of which can be found in the Supporting Information (Supplementary Table S1) (32).
Results
Sepsis risk
Following several quality control procedures, a total of 2,220 IVs were found to be related to sepsis susceptibility, and 2,219 IVs associated with sepsis (critical care) and sepsis-related death. All F-values for inclusion of SNPs >10. For sepsis susceptibility, as shown in Figure 2, IVW analysis indicated that 11 bacterial taxa were associated with sepsis susceptibility. Through sensitivity analysis, eight of them fulfilled the criteria for gut microbiota related to sepsis development, including the phylum Lentisphaerae [odds ratio (OR) = 0.89, 95% confidence interval (CI), 0.80–0.99, p = 0.0354], class Lentisphaeria (OR = 0.86, 95% CI, 0.78–0.94, p = 0.0017), order Victivallales (OR = 0.86, 95% CI, 0.78–0.94, p = 0.0017), genus Dialister (OR = 0.85, 95% CI, 0.74–0.97, p = 0.0158), class Gammaproteobacteria (OR = 1.37, 95% CI, 1.08–1.73, p = 0.0097), family Clostridiaceae1 (OR = 1.21, 95% CI, 1.04–1.40, p = 0.0111), and genera RuminococcaceaeUCG01 1 (OR = 1.10, 95% CI, 1.01–1.20, p = 0.0237) and Coprococcus2 (OR = 0.81, 95% CI, 0.69–0.94, p = 0.0066).
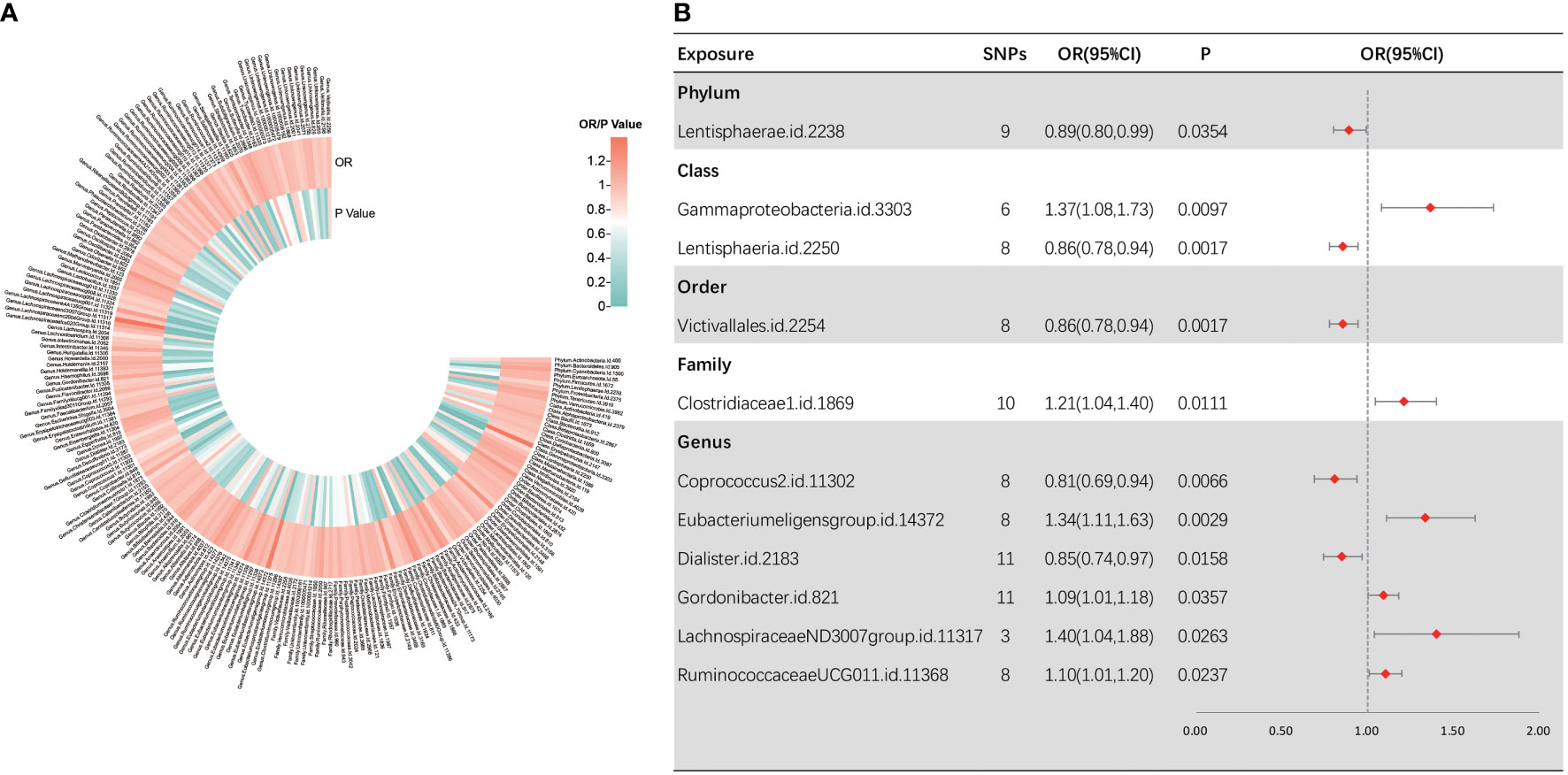
Figure 2 Causal analysis of gut microbiota and sepsis. (A) All results of IVW between gut microbiota and sepsis. (B) IVW results of gut microbiota taxa with causality to sepsis.
Among them, the class Lentisphaeria and the order Victivallales were significantly correlated with a reduced sepsis susceptibility risk. Notably, as the two types of gut microbial taxa are identical, we retained only the result for the order Victivallales. Three MR analysis methods showed inconsistency in the direction of the effects of the three bacterial taxa, namely, the genera Eubacteriumeligensgroup, Gordonibacter, and LachnospiraceaeND3007group. In Supplementary Figure S1, scatter plots from various tests were presented. Cochran Q-derived p-values suggested the absence of heterogeneity. Additionally, both the MR-Egger regression and MR-PRESSO tests failed to demonstrate horizontal pleiotropy (all p > 0.05) (Supplementary Table S2). LOO analyses revealed that individual SNPs do not bias the estimates (Supplementary Figure S2). To be clear, MR-PRESSO values and global test could not be measured because there were not enough instrumental variables for the genus LachnospiraceaeND3007group.
Sepsis (critical care) risk
As depicted in Figure 3, the results of IVW analyses revealed that the phylum Lentisphaerae (OR = 0.70, 95% CI, 0.53–0.93, p = 0.0143), class Lentisphaeria (OR = 0.67, 95% CI, 0.50–0.91, p = 0.0114), order Victivallales (OR = 0.67, 95% CI, 0.50–0.91, p = 0.0114), and genera Anaerostipes (OR = 0.49, 95% CI, 0.31–0.76, p = 0.0016), LachnospiraceaeUCG004 (OR = 0.51, 95% CI, 0.34–0.77, p = 0.0014), and Coprococcus1 (OR = 0.66, 95% CI, 0.44–0.99, p = 0.0425) showed a suggestive negative correlation with sepsis requiring critical care. Supplementary Figure S3 shows the scatter plots for various tests. A range of sensitivity analyses were conducted, consisting of MR-Egger regression, weighted median, Cochran’s Q test, MR-Egger intercept test, MR-PRESSO global test, and LOO analyses to confirm the rigidity of the results presented above (Supplementary Table S3, Supplementary Figure S4). As the order Victivallales and the class Lentisphaeria are exactly the same, we only kept the results of the order Victivallales.
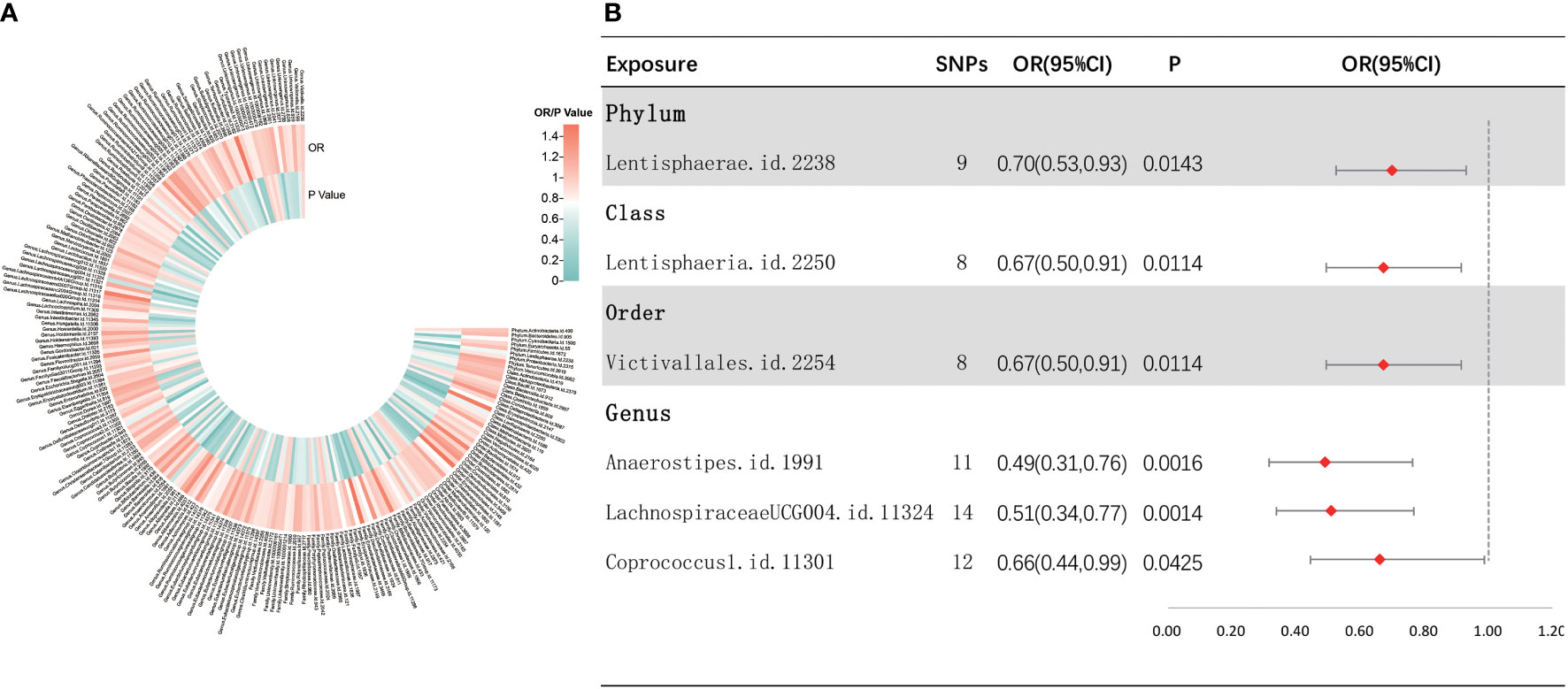
Figure 3 Investigating the causal relationship between gut microbiota and sepsis requiring critical care. (A) All results of IVW between gut microbiota and sepsis requiring critical care. (B) IVW results of gut microbiota taxa with causality to sepsis requiring critical care.
Sepsis (28-day death in critical care) risk
As shown in Figure 4, IVW analysis indicated that 11 bacterial taxa were associated with sepsis-related 28-day mortality in critical care. The three MR analysis methods were found to affect two bacterial taxa (i.e., Sellimonas and Tyzzerella3) in different directions by performing sensitivity analyses (Supplementary Figure S5). Finally, nine met the eligibility criteria for gut microbiota in relation to the occurrence of sepsis-related deaths in critical care. Five bacterial taxa were normally significantly negatively related to the risk of sepsis-related death in critical care, including the class Lentisphaeria (OR = 0.54, 95% CI, 0.30–0.95, p = 0.0335), order Victivallales (OR = 0.54, 95% CI, 0.30–0.95, p = 0.0335), and genera Coprococcus2 (OR = 0.34, 95% CI, 0.14–0.83, p = 0.0178), Ruminiclostridium6 (OR = 0.43, 95% CI, 0.22–0.83, p = 0.0122), and Coprococcus1 (OR = 0.45, 95% CI, 0.21–0.97, p = 0.0412), while four bacterial taxa showed a normally significant positive association with the risk of death from sepsis in critically ill patients, including the phylum Tenericutes (OR = 2.03, 95% CI, 1.01–4.08, p = 0.0456), classes Mollicutes (OR = 2.03, 95% CI, 1.01–4.08, p = 0.0456) and Bacteroidia (OR = 2.65, 95% CI, 1.18–5.96, p = 0.0289), and order Bacteroidales (OR = 2.65, 95% CI, 1.18–5.96, p = 0.0184). Furthermore, nine bacterial taxa were subjected to a sequential sensitivity analyses involving MR-Egger regression, weighted median, Cochran’s Q test, MR-Egger intercept test, MR-PRESSO global test, and LOO analyses, which confirmed the robustness of the above results (Supplementary Table S4, Supplementary Figure S6). Interestingly, the order Victivallales is identical to the class Lentisphaeria, the order Bacteroidales is identical to the class Bacteroidia, and the class Mollicutes is identical to the phylum Mycoplasmatota, which is equivalent to Tenericutes. Thus, we have retained only the order Victivallales, the order Bacteroidales, and the class Mollicutes.
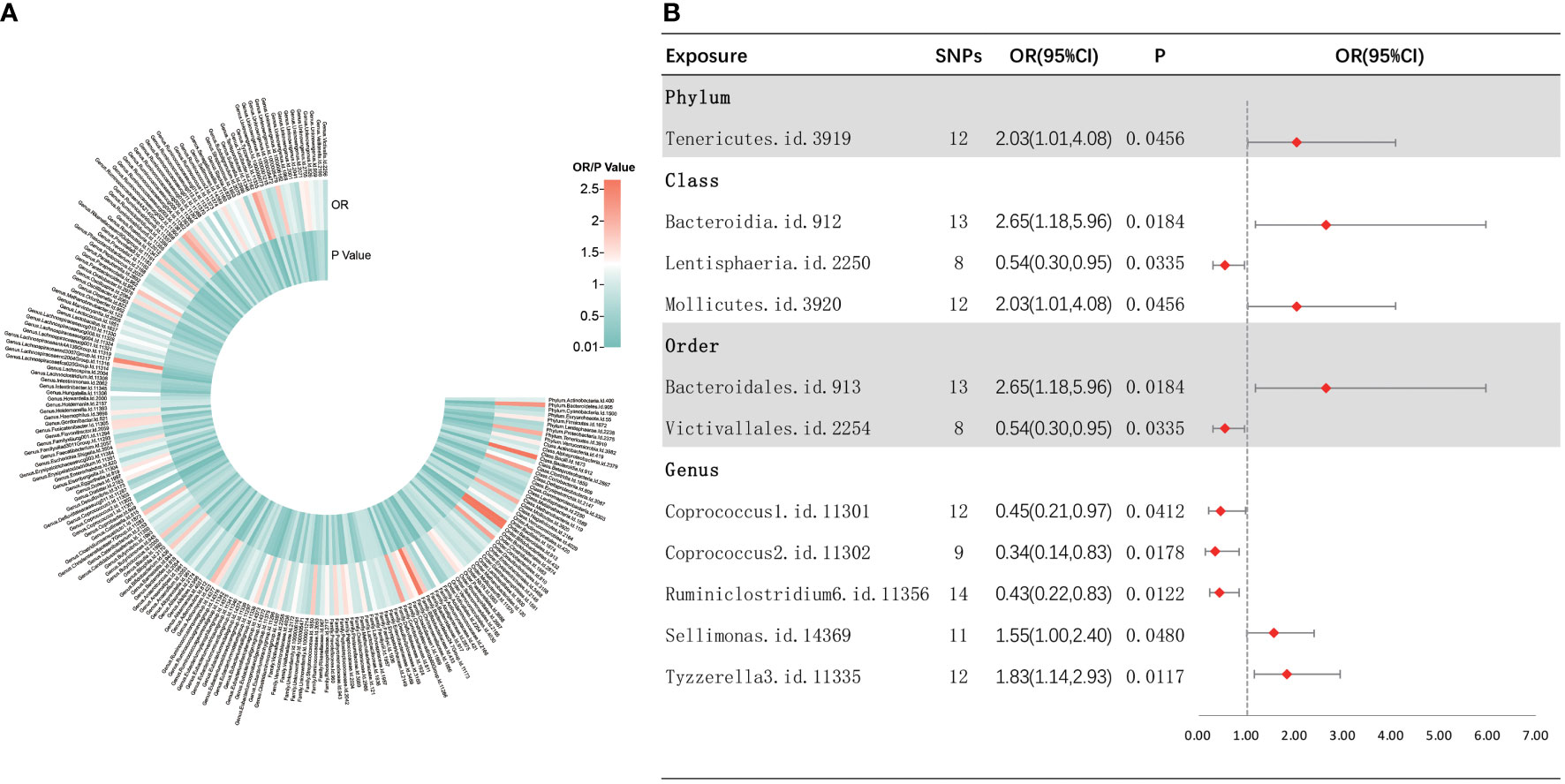
Figure 4 Causal analysis of gut microbiota and sepsis-related 28-day mortality in critical care. (A) All results of IVW between gut microbiota and sepsis-related 28-day mortality in critical care. (B) IVW results of gut microbiota taxa with causality to sepsis-related 28-day mortality in critical care.
For a better understanding of the causal relationship between gut microbiota and sepsis, a summary network is shown in Figure 5.
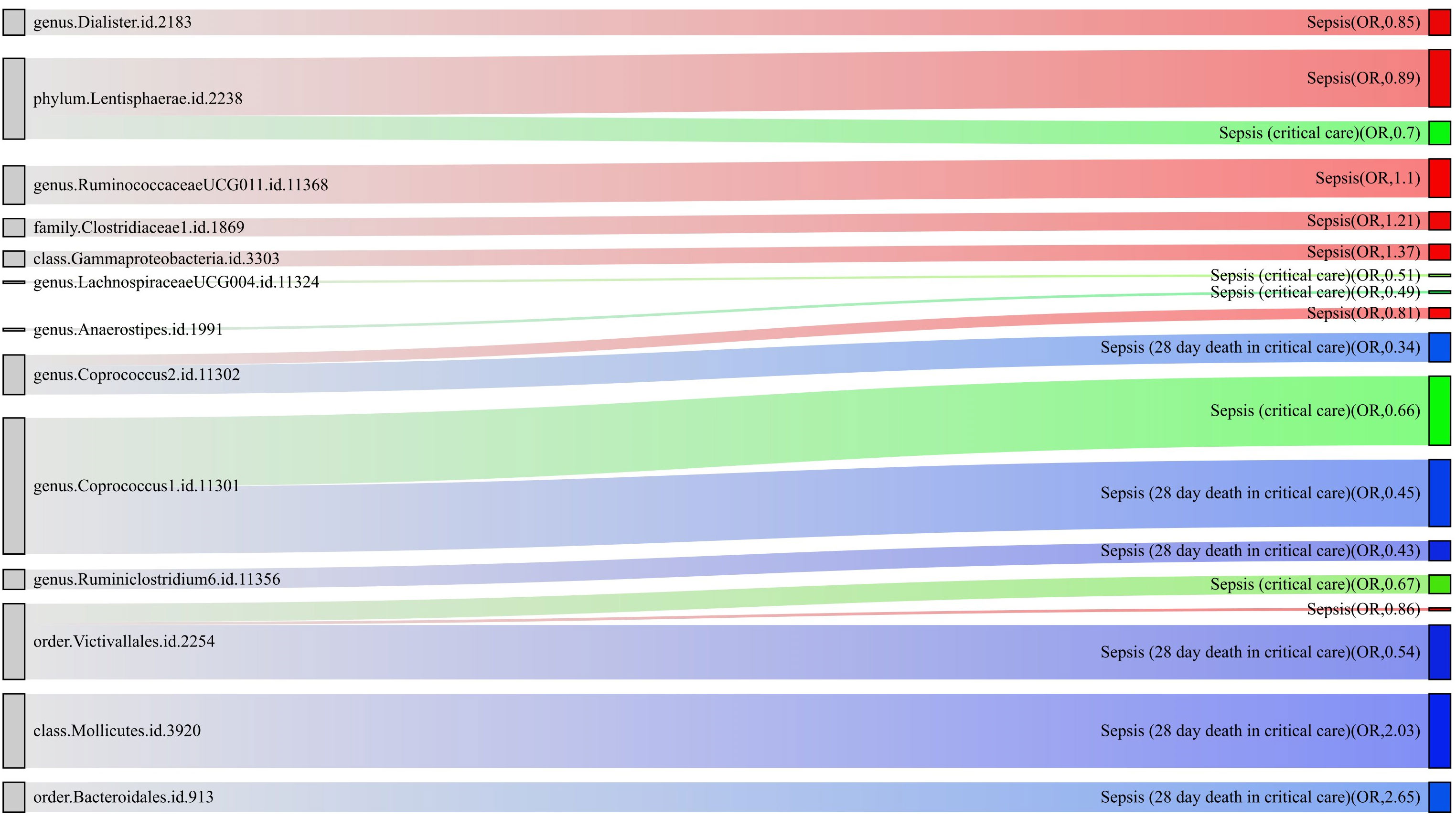
Figure 5 The causality between gut microbiota and sepsis, sepsis requiring critical care, and sepsis-related 28-day mortality in critical care by Mendelian randomization analysis. The thickness of the line represents the p-value.
Confounding analysis and Steiger test
To assess the impact of confounders, we searched the Phenoscanner V2 website for several of the confounders we identified, including sex, obesity, and diabetes-related instrumental variables.
Regarding sepsis susceptibility, one SNP (rs12636310) of RuminococcaceaeUCG011 was linked to diabetes-related phenotypes. Causality remained significant after removal of this SNP (IVW OR = 1.11, 95% CI, 1.02–1.22, p = 0.0224). Regarding sepsis (critical care) risk, one SNP (rs128942721) for LachnospiraceaeUCG004 was correlated with obesity-related phenotypes, and the causal relationship remained significant after the removal of one SNP (IVW OR = 0.52, 95% CI, 0.33–0.82, p = 0.0045). Regarding sepsis-related 28-day mortality in critical care, SNPs of the bacteria were discovered to be causally related to sepsis-related death, independent of any confounding factors. Further Steiger tests were performed to verify the direction of the gut microbiota influence on sepsis, sepsis requiring critical care, and sepsis (28-day death in critical care). The Steiger p-values suggested that established causality is not affected by reverse causality (Table 1).
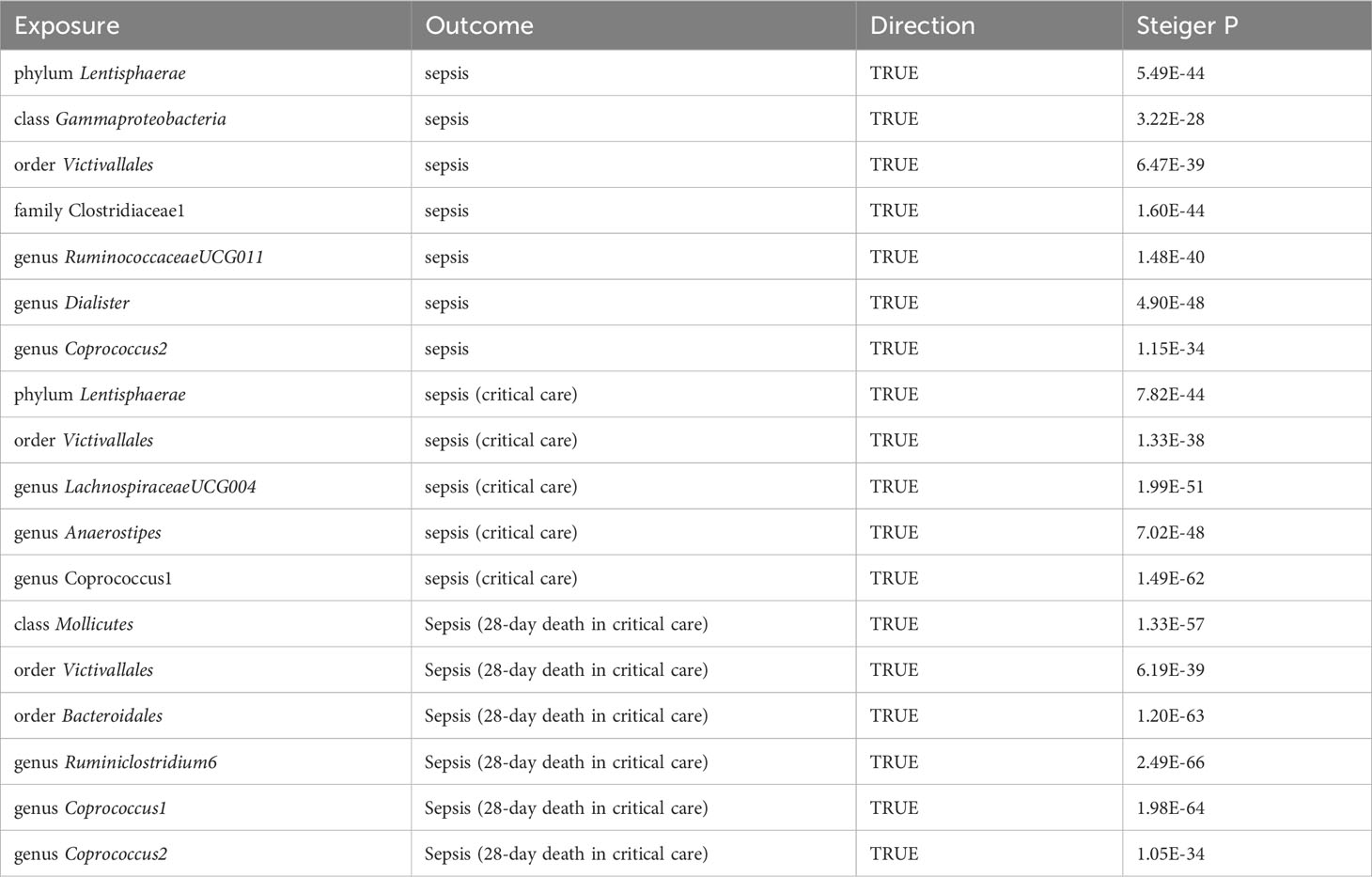
Table 1 Steiger direction test from the gut microbiota to sepsis, sepsis (critical care), and sepsis-related 28-day mortality in critical care.
Discussion
In this research, we adopted a two-sample MR study to explore the causality between gut microbiota and the onset and progression of sepsis. We identified one causal bacterial taxa and six suggestive bacterial taxa associated with the development of sepsis. In addition, five bacterial taxa were suggested to be causally related to sepsis requiring critical care, and six bacterial taxa were suggested to be causally related to sepsis-related 28-day mortality in critical care.
Three bacteria taxa (RuminococcaceaeUCG011, Clostridiaceae1, and Gammaproteobacteria) were positively correlated with susceptibility to sepsis and two bacteria taxa (class Mollicutes and order Bacteroidales) were positively correlated with sepsis-related mortality. Previous research had shown a higher relative abundance of Bacteroidetes and a significantly higher enrichment of Gammaproteobacteria in the sepsis group compared to healthy controls, in accordance with our findings (5). Murine studies had also indicated that post-sepsis mice lung communities were clearly enriched with the order Bacteroidales found in the murine gut (33). High levels of Bacteroides spp. were prevalent in bronchoalveolar lavage fluid from ARDS patients and were significantly correlated with serum TNF-α concentrations, a critical mediator of the septic stress reaction and a predictor of patient mortality (33), while the class Gammaproteobacteria included pathogens such as Escherichia coli and Klebsiella spp., which were normally found in small numbers but have the potential to overgrow and dominate the intestinal tract during dysbiosis (34). The fecal microbiota following injury in a mouse model of sepsis showed that Ruminococcaceae increased in the subacute phase (35). Ruminococcaceae is a genus in the class Clostridia, and Clostridiaceae is also a family in the class Clostridia. Liu et al. found that the class Clostridia was significantly more enriched in the healthy group compared to the sepsis group (5), which contradicts our results. However, their results were limited due to methodological shortcomings (e.g., residual confounding factors). In addition, in the results of our analyses, the genera RuminococcaceaeUCG011 and Ruminiclostridium6 had different effects on sepsis risk. The inconsistency of the results may be attributed to the fact that the genus-level classification of the gut microbiota has not been explored in sufficient depth. Research related to Mollicutes and sepsis is lacking, but there is some indirect evidence of their relevance. For instance, Sompolinsky et al. found puerperal sepsis caused by T-strain Mycoplasma (36), a genus belonging to the class Mollicutes.
The MR study found that one causal bacterial taxon (order Victivallales) and three suggestive bacterial taxa (genera Dialister and Coprococcus2, and phylum Lentisphaerae) were causally related to sepsis, five bacterial taxa (genera LachnospiraceaeUCG004, Anaerostipes, and Coprococcus1, order Victivallales, and phylum Lentisphaerae) were suggested to be causally related to sepsis requiring critical care, and four bacterial taxa (genera Ruminiclostridium6, Coprococcus2, and Coprococcus1, and order Victivallales) were suggested to be causally related to sepsis-related 28-day mortality in critical care. Interestingly, two species of gut flora (Coprococcus and Victivallales) have a negative regulatory role in both the development and progression of sepsis. The genera Coprococcus1, Coprococcus2, LachnospiraceaeUCG004, Anaerostipes, and Ruminiclostridium6 all belong to the class Clostridia. Children with sepsis had more pathogens of opportunistic origin and fewer beneficial bacteria (e.g., Clostridia) detected in their bodies (9). In addition, the adult with sepsis was found to be significantly less enriched in the class Clostridia compared to the healthy group (5). The short-chain fatty acid (SCFA) butyrate, generated by Clostridia, exerts its effects on colonic regulatory T-cell differentiation by upregulating the key regulatory T-cell transcription factor Foxp3 (37) and suppressing histone deacetylation, thereby reducing the expression of NF-κB-regulated pro-inflammatory cytokines, such as TNF-α and IL-6 (38).
For Dialister, Victivallales, and Lentisphaerae, very limited investigations have reported their associations with sepsis. Dialister was previously found to be closely linked to early-onset neonatal sepsis, but the exact action and its mechanism are not clarified (39), while the order Victivallales belongs to the phylum Lentisphaerae, which are all Gram-negative bacteria that produce extracellular mucus substances. We hypothesize that this group of bacteria may be involved in the formation of the intestinal mucus barrier and thus play a protective role in the gut (40). Moreover, a reduction in Lentisphaerae and others was found in individuals over 65 years, which may affect changes in intestinal physiology such as reduced intestinal contractility, reduced mucus production, intestinal barrier dysfunction, and the ensuing dysbiosis (41).
The gut microbiome may influence host vulnerability and response to sepsis by the following mechanisms. The first mechanism is the expansion of gut pathogenic bacteria. Animal studies have shown that inflammation of the colon and exposure to antibiotics in mice lead to amplification and systemic spread of pathogenic clones of multidrug-resistant E. coli, and that the systemic presence of this pathogen is sufficient to induce sepsis-like disease (42). In addition, a single-center cohort study suggested that gut dysbiosis, characterized by an accumulation of bacilli and their fermentation metabolites, might precede the onset of late-onset sepsis (43). Second, the immune system produces a powerful pro-inflammatory effect. For instance, in a mouse model of Streptococcus pneumoniae sepsis, it was observed that administering oral antibiotics prior to the onset of sepsis was associated with decreased levels of TNF-α (a pro-inflammatory cytokine) in the lungs (44). However, other studies have shown that depletion of the gut microbiota has an increased effect on TNF-α (45, 46). Although the expression of specific cytokines varied across studies, it seems that a stronger inflammatory response to sepsis is the overall effect of altering the structure of the normal gut microbiota prior to the onset of sepsis (47). Third, the production of beneficial microbial products (e.g., SCFAs) is reduced. These metabolites may be involved in the initiation of the innate and adaptive immune system to protect distant organs from infection (48). Fourth, once sepsis is established, the gut microbiome deteriorates and leads to increased susceptibility to visceral organ dysfunction (47). In sum, there is a causality between gut flora and sepsis, which provides a basis for the therapy of sepsis through dietary intervention, the addition of prebiotics, fecal microbiota transplantation (FMT), or the addition of the gut flora metabolite SCFA (47, 49).
This study has the following advantages: MR analysis identified a causal relationship between the gut microbiota and the onset and progression of sepsis, excluding the influence of confounding factors and reverse caution. Genetic variation in the intestinal flora was derived from the largest existing GWAS meta-analysis, and the causal influence of various gut microbiota on sepsis was analyzed from the genus to the phylum level. However, the study suffers from several limitations. First, the majority of the incorporated studies focused on European populations; thus, the findings of this research might not be entirely transferable to other ethnic groups. Second, considering the moderate sample size of the gut microbiota, we opted not to conduct reverse MR analyses, as this approach may be susceptible to potential instrumental biases in the obtained results. Third, 16S rRNA gene sequencing can only depict the gut microbiota at the genus to phylum level; metagenomic and multi-omics approaches might present the possibility of targeting the composition of the gut microbiota at a more concrete level, thus discovering whether a more specific species level is linked to sepsis. Finally, the intestinal microbiota is influenced by a variety of environmental factors such as diet, lifestyle, and medication, and the lack of detailed information in the raw data about disease diet or medication status makes further subgroup analyses difficult.
In summary, the MR study has identified several bacteria that were causally linked to the onset and progression of sepsis, providing new ideas for early diagnosis, personalized treatment, and outcome prognosis of sepsis. However, large samples of population studies as well as in vivo and ex vivo experimental evidence are still needed to further elucidate the role and mechanisms of specific groups of bacteria in the occurrence and progression of sepsis.
Data availability statement
Publicly available datasets were analyzed in this study.
Ethics statement
This study used de-identified data from an open participant study that had been approved by the Ethical Standards Committee for Human Experimentation, so this study did not require separate ethical approval.
Author contributions
WS: Data curation, Formal analysis, Investigation, Methodology, Software, Validation, Visualization, Writing – original draft. SZ: Formal Analysis, Writing – original draft. HQ: Writing – original draft, Data curation, Software, Visualization. SH: Data curation, Software, Writing – original draft, Investigation. HL: Data curation, Investigation, Software, Writing – original draft. JL: Conceptualization, Supervision, Writing – review & editing. DC: Conceptualization, Supervision, Writing – review & editing.
Funding
The author(s) declare financial support was received for the research, authorship, and/or publication of this article. This study was funded by the National Natural Science Foundation of China (No. 82172152 and 82241044) and the Science and Technology Commission of Shanghai (No. 22692192200).
Acknowledgments
We express our gratitude to all authors and participants of GWAS, the online scientific mapping site ChiPlot (https://www.chiplot.online/).
Conflict of interest
The authors declare that the research was conducted in the absence of any commercial or financial relationships that could be construed as a potential conflict of interest.
Publisher’s note
All claims expressed in this article are solely those of the authors and do not necessarily represent those of their affiliated organizations, or those of the publisher, the editors and the reviewers. Any product that may be evaluated in this article, or claim that may be made by its manufacturer, is not guaranteed or endorsed by the publisher.
Supplementary material
The Supplementary Material for this article can be found online at: https://www.frontiersin.org/articles/10.3389/fimmu.2024.1266230/full#supplementary-material
Supplementary Figure 1 | Scatter plots for the causality between gut microbiota and sepsis susceptibility.
Supplementary Figure 2 | Leave-one-out analyses for the causality between gut microbiota and sepsis susceptibility.
Supplementary Figure 3 | Scatter plots for the causality between gut microbiota and sepsis related to critical care.
Supplementary Figure 4 | Leave-one-out analyses for the causality between gut microbiota and sepsis related to critical care.
Supplementary Figure 5 | Scatter plots for the causality between gut microbiota and sepsis-related 28-day mortality in critical care.
Supplementary Figure 6 | Leave-one-out analyses for the causality between gut microbiota and sepsis-related 28-day mortality in critical care.
References
1. Evans L, Rhodes A, Alhazzani W, Antonelli M, Coopersmith CM, French C, et al. Surviving sepsis campaign: international guidelines for management of sepsis and septic shock 2021. Intensive Care Med (2021) 47:1181–247. doi: 10.1007/s00134-021-06506-y
2. Minasyan H. Sepsis: mechanisms of bacterial injury to the patient. Scand J Trauma Resusc Emerg Med (2019) 27:19. doi: 10.1186/s13049-019-0596-4
3. Costa SP, Carvalho CM. Burden of bacterial bloodstream infections and recent advances for diagnosis. Pathog Dis (2022) 80:ftac027. doi: 10.1093/femspd/ftac027
4. Lange K, Buerger M, Stallmach A, Bruns T. Effects of antibiotics on gut microbiota. Dig Dis (2016) 34:260–8. doi: 10.1159/000443360
5. Liu Z, Li N, Fang H, Chen X, Guo Y, Gong S, et al. Enteric dysbiosis is associated with sepsis in patients. FASEB J (2019) 33:12299–310. doi: 10.1096/fj.201900398RR
6. Zaborin A, Smith D, Garfield K, Quensen J, Shakhsheer B, Kade M, et al. Membership and behavior of ultra-low-diversity pathogen communities present in the gut of humans during prolonged critical illness. mBio (2014) 5:e01361–01314. doi: 10.1128/mBio.01361-14
7. Prescott HC, Dickson RP, Rogers MA, Langa KM, Iwashyna TJ. Hospitalization type and subsequent severe sepsis. Am J Respir Crit Care Med (2015) 192:581–8. doi: 10.1164/rccm.201503-0483OC
8. Liu J, Wang M, Chen W, Ma J, Peng Y, Zhang M, et al. Altered gut microbiota taxonomic compositions of patients with sepsis in a pediatric intensive care unit. Front Pediatr (2021) 9:645060. doi: 10.3389/fped.2021.645060
9. Du B, Shen N, Tao Y, Sun S, Zhang F, Ren H, et al. Analysis of gut microbiota alteration and application as an auxiliary prognostic marker for sepsis in children: a pilot study. Transl Pediatr (2021) 10:1647–57. doi: 10.21037/tp-21-51
10. Wan YD, Zhu RX, Wu ZQ, Lyu SY, Zhao LX, Du ZJ, et al. Gut microbiota disruption in septic shock patients: A pilot study. Med Sci Monit (2018) 24:8639–46. doi: 10.12659/msm.911768
11. Sun S, Wang D, Dong D, Xu L, Xie M, Wang Y, et al. Altered intestinal microbiome and metabolome correspond to the clinical outcome of sepsis. Crit Care (2023) 27:127. doi: 10.1186/s13054-023-04412-x
12. Panpetch W, Somboonna N, Bulan DE, Issara-Amphorn J, Worasilchai N, Finkelman M, et al. Gastrointestinal colonization of candida albicans increases serum (1→3)-β-D-glucan, without candidemia, and worsens cecal ligation and puncture sepsis in murine model. Shock (2018) 49:62–70. doi: 10.1097/shk.0000000000000896
13. Chen G, Huang B, Fu S, Li B, Ran X, He D, et al. G protein-coupled receptor 109A and host microbiota modulate intestinal epithelial integrity during sepsis. Front Immunol (2018) 9:2079. doi: 10.3389/fimmu.2018.02079
14. Smith GD, Ebrahim S. 'Mendelian randomization': can genetic epidemiology contribute to understanding environmental determinants of disease? Int J Epidemiol (2003) 32:1–22. doi: 10.1093/ije/dyg070
15. Chen JH, Zeng LY, Zhao YF, Tang HX, Lei H, Wan YF, et al. Causal effects of gut microbiota on sepsis: a two-sample Mendelian randomization study. Front Microbiol (2023) 14:1167416. doi: 10.3389/fmicb.2023.1167416
16. Zhang Z, Cheng L, Ning D. Gut microbiota and sepsis: bidirectional Mendelian study and mediation analysis. Front Immunol (2023) 14:1234924. doi: 10.3389/fimmu.2023.1234924
17. Boef AG, Dekkers OM, le Cessie S. Mendelian randomization studies: a review of the approaches used and the quality of reporting. Int J Epidemiol (2015) 44:496–511. doi: 10.1093/ije/dyv071
18. Kurilshikov A, Medina-Gomez C, Bacigalupe R, Radjabzadeh D, Wang J, Demirkan A, et al. Large-scale association analyses identify host factors influencing human gut microbiome composition. Nat Genet (2021) 53:156–65. doi: 10.1038/s41588-020-00763-1
19. Bycroft C, Freeman C, Petkova D, Band G, Elliott LT, Sharp K, et al. The UK Biobank resource with deep phenotyping and genomic data. Nature (2018) 562:203–9. doi: 10.1038/s41586-018-0579-z
20. Zekavat SM, Lin SH, Bick AG, Liu A, Paruchuri K, Wang C, et al. Hematopoietic mosaic chromosomal alterations increase the risk for diverse types of infection. Nat Med (2021) 27:1012–24. doi: 10.1038/s41591-021-01371-0
21. Hamilton FW, Thomas M, Arnold D, Palmer T, Moran E, Mentzer AJ, et al. Therapeutic potential of IL6R blockade for the treatment of sepsis and sepsis-related death: A Mendelian randomisation study. PloS Med (2023) 20:e1004174. doi: 10.1371/journal.pmed.1004174
22. Ni JJ, Xu Q, Yan SS, Han BX, Zhang H, Wei XT, et al. Gut microbiota and psychiatric disorders: A two-sample mendelian randomization study. Front Microbiol (2021) 12:737197. doi: 10.3389/fmicb.2021.737197
23. Pierce BL, Ahsan H, Vanderweele TJ. Power and instrument strength requirements for Mendelian randomization studies using multiple genetic variants. Int J Epidemiol (2011) 40:740–52. doi: 10.1093/ije/dyq151
24. Pierce BL, Burgess S. Efficient design for Mendelian randomization studies: subsample and 2-sample instrumental variable estimators. Am J Epidemiol (2013) 178:1177–84. doi: 10.1093/aje/kwt084
25. Bowden J, Davey Smith G, Burgess S. Mendelian randomization with invalid instruments: effect estimation and bias detection through Egger regression. Int J Epidemiol (2015) 44:512–25. doi: 10.1093/ije/dyv080
26. Bowden J, Davey Smith G, Haycock PC, Burgess S. Consistent estimation in mendelian randomization with some invalid instruments using a weighted median estimator. Genet Epidemiol (2016) 40:304–14. doi: 10.1002/gepi.21965
27. Verbanck M, Chen CY, Neale B, Do R. Detection of widespread horizontal pleiotropy in causal relationships inferred from Mendelian randomization between complex traits and diseases. Nat Genet (2018) 50:693–8. doi: 10.1038/s41588-018-0099-7
28. Thompson KJ, Finfer SR, Woodward M, Leong RNF, Liu B. Sex differences in sepsis hospitalisations and outcomes in older women and men: A prospective cohort study. J Infect (2022) 84:770–6. doi: 10.1016/j.jinf.2022.04.035
29. Wang HE, Griffin R, Judd S, Shapiro NI, Safford MM. Obesity and risk of sepsis: a population-based cohort study. Obes (Silver Spring) (2013) 21:E762–769. doi: 10.1002/oby.20468
30. Koh GC, Peacock SJ, van der Poll T, Wiersinga WJ. The impact of diabetes on the pathogenesis of sepsis. Eur J Clin Microbiol Infect Dis (2012) 31:379–88. doi: 10.1007/s10096-011-1337-4
31. Hemani G, Tilling K, Davey Smith G. Orienting the causal relationship between imprecisely measured traits using GWAS summary data. PloS Genet (2017) 13:e1007081. doi: 10.1371/journal.pgen.1007081
32. Skrivankova VW, Richmond RC, Woolf BAR, Davies NM, Swanson SA, VanderWeele TJ, et al. Strengthening the reporting of observational studies in epidemiology using mendelian randomisation (STROBE-MR): explanation and elaboration. Bmj (2021) 375:n2233. doi: 10.1136/bmj.n2233
33. Dickson RP, Singer BH, Newstead MW, Falkowski NR, Erb-Downward JR, Standiford TJ, et al. Enrichment of the lung microbiome with gut bacteria in sepsis and the acute respiratory distress syndrome. Nat Microbiol (2016) 1:16113. doi: 10.1038/nmicrobiol.2016.113
34. Kim S, Covington A, Pamer EG. The intestinal microbiota: Antibiotics, colonization resistance, and enteric pathogens. Immunol Rev (2017) 279:90–105. doi: 10.1111/imr.12563
35. Muratsu A, Ikeda M, Shimizu K, Kameoka S, Motooka D, Nakamura S, et al. Dynamic change of fecal microbiota and metabolomics in a polymicrobial murine sepsis model. Acute Med Surg (2022) 9:e770. doi: 10.1002/ams2.770
36. Sompolinsky D, Solomon F, Leiba H, Caspi E, Lewinsohn G, Almog C. Puerperal sepsis due to T-strain Mycoplasma. Isr J Med Sci (1971) 7:745–8.
37. Furusawa Y, Obata Y, Fukuda S, TA E, Nakato G, Takahashi D, et al. Commensal microbe-derived butyrate induces the differentiation of colonic regulatory T cells. Nature (2013) 504:446–50. doi: 10.1038/nature12721
38. Parada Venegas D, de la Fuente MK, Landskron G, González MJ, Quera R, Dijkstra G, et al. Short chain fatty acids (SCFAs)-mediated gut epithelial and immune regulation and its relevance for inflammatory bowel diseases. Front Immunol (2019) 10:277. doi: 10.3389/fimmu.2019.00277
39. Dornelles LV, Procianoy RS, Roesch LFW, Corso AL, Dobbler PT, Mai V, et al. Meconium microbiota predicts clinical early-onset neonatal sepsis in preterm neonates. J Matern Fetal Neonatal Med (2022) 35:1935–43. doi: 10.1080/14767058.2020.1774870
40. Cho JC, Vergin KL, Morris RM, Giovannoni SJ. Lentisphaera araneosa gen. nov., sp. nov, a transparent exopolymer producing marine bacterium, and the description of a novel bacterial phylum, Lentisphaerae. Environ Microbiol (2004) 6:611–21. doi: 10.1111/j.1462-2920.2004.00614.x
41. Kundu P, Blacher E, Elinav E, Pettersson S. Our gut microbiome: the evolving inner self. Cell (2017) 171:1481–93. doi: 10.1016/j.cell.2017.11.024
42. Ayres JS, Trinidad NJ, Vance RE. Lethal inflammasome activation by a multidrug-resistant pathobiont upon antibiotic disruption of the microbiota. Nat Med (2012) 18:799–806. doi: 10.1038/nm.2729
43. Graspeuntner S, WasChina S, Künzel S, Twisselmann N, Rausch TK, Cloppenborg-Schmidt K, et al. Gut dysbiosis with bacilli dominance and accumulation of fermentation products precedes late-onset sepsis in preterm infants. Clin Infect Dis (2019) 69:268–77. doi: 10.1093/cid/ciy882
44. Schuijt TJ, Lankelma JM, Scicluna BP, de Sousa e Melo F, Roelofs JJ, de Boer JD, et al. The gut microbiota plays a protective role in the host defence against pneumococcal pneumonia. Gut (2016) 65:575–83. doi: 10.1136/gutjnl-2015-309728
45. Ikeda M, Shimizu K, Ogura H, Kurakawa T, Umemoto E, Motooka D, et al. Hydrogen-rich saline regulates intestinal barrier dysfunction, dysbiosis, and bacterial translocation in a murine model of sepsis. Shock (2018) 50:640–7. doi: 10.1097/shk.0000000000001098
46. Morowitz MJ, Di Caro V, Pang D, Cummings J, Firek B, Rogers MB, et al. Dietary supplementation with nonfermentable fiber alters the gut microbiota and confers protection in murine models of sepsis. Crit Care Med (2017) 45:e516–23. doi: 10.1097/ccm.0000000000002291
47. Adelman MW, Woodworth MH, Langelier C, Busch LM, Kempker JA, Kraft CS, et al. The gut microbiome's role in the development, maintenance, and outcomes of sepsis. Crit Care (2020) 24:278. doi: 10.1186/s13054-020-02989-1
48. Biemond JJ, McDonald B, Haak BW. Leveraging the microbiome in the treatment of sepsis: potential pitfalls and new perspectives. Curr Opin Crit Care (2023) 29:123–9. doi: 10.1097/mcc.0000000000001019
Keywords: gut microbiota, sepsis, sepsis-related death, causality, Mendelian randomization
Citation: Shang W, Zhang S, Qian H, Huang S, Li H, Liu J and Chen D (2024) Gut microbiota and sepsis and sepsis-related death: a Mendelian randomization investigation. Front. Immunol. 15:1266230. doi: 10.3389/fimmu.2024.1266230
Received: 24 July 2023; Accepted: 02 January 2024;
Published: 31 January 2024.
Edited by:
Louisy Santos, Rio de Janeiro State University, BrazilReviewed by:
Flavia Silva, Rio de Janeiro State University, BrazilGeorgia Damoraki, National and Kapodistrian University of Athens, Greece
Copyright © 2024 Shang, Zhang, Qian, Huang, Li, Liu and Chen. This is an open-access article distributed under the terms of the Creative Commons Attribution License (CC BY). The use, distribution or reproduction in other forums is permitted, provided the original author(s) and the copyright owner(s) are credited and that the original publication in this journal is cited, in accordance with accepted academic practice. No use, distribution or reproduction is permitted which does not comply with these terms.
*Correspondence: Dechang Chen, MTg5MTg1MjAwMDJAMTg5LmNu; Jiao Liu, Y2F0aGVyaW5lMDE1QDE2My5jb20=
†These authors have contributed equally to this work