- 1Burn and Shock Trauma Research Institute, Stritch School of Medicine, Loyola University Chicago Health Sciences Division, Maywood, IL, United States
- 2Department of Surgery, Stritch School of Medicine, Loyola University Chicago Health Sciences Division, Maywood, IL, United States
- 3Department of Mathematics and Statistics at Loyola University Chicago, Chicago, IL, United States
- 4Biomedical Engineering & Medicine, University of Houston, Houston, TX, United States
- 5Department of Medicine, Division of Pulmonary, Critical Care, and Sleep Medicine Yale New Haven Hospital and Yale School of Medicine, New Haven, CT, United States
Sarcoidosis is a chronic granulomatous disorder characterized by unknown etiology, undetermined mechanisms, and non-specific therapies except TNF blockade. To improve our understanding of the pathogenicity and to predict the outcomes of the disease, the identification of new biomarkers and molecular endotypes is sorely needed. In this study, we systematically evaluate the biomarkers identified through Omics and non-Omics approaches in sarcoidosis. Most of the currently documented biomarkers for sarcoidosis are mainly identified through conventional “one-for-all” non-Omics targeted studies. Although the application of machine learning algorithms to identify biomarkers and endotypes from unbiased comprehensive Omics studies is still in its infancy, a series of biomarkers, overwhelmingly for diagnosis to differentiate sarcoidosis from healthy controls have been reported. In view of the fact that current biomarker profiles in sarcoidosis are scarce, fragmented and mostly not validated, there is an urgent need to identify novel sarcoidosis biomarkers and molecular endotypes using more advanced Omics approaches to facilitate disease diagnosis and prognosis, resolve disease heterogeneity, and facilitate personalized medicine.
1 Background
Sarcoidosis is an inflammatory disorder characterized by granuloma formation in affected organs, most often in the lung (~90%) (1, 2). Scadding stage of sarcoidosis is therefore based on intrathoracic involvement. The most common organs involved are the lung, skin, eyes, liver, lymph nodes, salivary glands, bone/joints/muscle, spleen, nervous system, kidneys, sinuses, and heart. For example, lung sarcoidosis is featured by the presence of coalescing, tightly clustered, non-necrotizing granulomas, and is complicated by lung fibrosis in up to 20% progressive patients who account for 75% sarcoidosis-related deaths of respiratory causes (2–5). The etiology of sarcoidosis is still unknown since it was first described 1.5 centuries ago (1). The prevalence, presentation, prognosis, and triggering antigen are extremely variable (6). The outcomes of lung sarcoidosis are affected by age, gender, race, incomes, environment, lung morbidity, lung leucocyte infiltration, requirement of treatment, and genetic variants of associated genes (e.g., human leucocyte antigen class II (HLA class II), tumor necrosis factor α (TNF-α) and annexin XI (ANXA11), among others) (1, 2). However, there are no reliable biomarkers for predicting the propensity of sarcoidosis to progress to lung fibrosis (7).
The combination of advanced Omics techniques and machine learning algorithms holds the potential to facilitate mechanistic investigations and discovery of novel biomarkers for this intriguing illness. Molecular endotypes and biomarkers provide critical information for the development of new therapeutics. Several studies on the phenotypes of sarcoidosis have been published (8–10), but they are outside the scope of this review. In sharp contrast, only one study on the molecular endotypes of the disease has recently been reported to date (11). Biomarkers are widely applied to diagnosis, differential diagnosis, prognosis, treatment response, disease activity, severity assessment, chronicity evaluation, and the implementation of interventions. In this paper, we systematically review the identified biomarkers based on their clinical relevance. The clinical data were analyzed with differentiation analysis, correlation analysis, biomarker-specific tests, and machine learning algorithms. Recently, several publications have comprehensively reviewed sarcoidosis biomarkers identified using genomics (12–14). We will not reiterate this topic here. Instead, we will focus on the transcriptomics (and other Omics) studies published since 2018, as earlier studies have already been covered in a prior review (15).
2 Workflow of omics analysis for biomarkers and endotypes
The workflow of clinical omics studies is generally consistent, yet it adapts based on the specific objectives, materials, platforms, and data analysis strategies involved (Figure 1). Omics studies could be applied to the identification of candidate biomarkers, molecular endotypes, gene expression signatures, mechanisms, and druggable targets. The workflow is initiated by collecting relevant biologic specimens, including cells, tissues, and body fluid samples from sarcoidosis patients and healthy controls. The specimens are then pre-processed (e.g., obtaining single-cell suspension, extracellular vesicle isolation, or DNA/RNA/protein extraction) ready for the analysis by Omics platforms to generate Omics datasets. Once the datasets become available, data cleaning is required before downstream analysis, including normalization, missing data imputation, batch effect correction, and application of cut-off criteria. Next, the datasets will be profiled to identify differentially expressed genes/transcripts/proteins/metabolites. Various statistical and machine learning techniques are employed based on the specific tasks at hand. For instance, unsupervised clustering can be utilized to uncover novel molecular endotypes, while supervised logistic regression is appropriate for finding potential biomarkers. Omics studies can shed light on disease signatures, candidate biomarkers, molecular endotypes, and clinical disease-omics correlations. Finally, these observations will be cross-referenced to functional annotations, correlations with clinical variables, and literature reports. Validation of candidate biomarkers and molecular endotypes is also required in a separate independent cohort. An alternative strategy is to split one cohort into training and validation group. Besides, preclinical animal models of the disease and organ-on-chip could be used to validate the identified markers and mechanisms of action (16, 17).
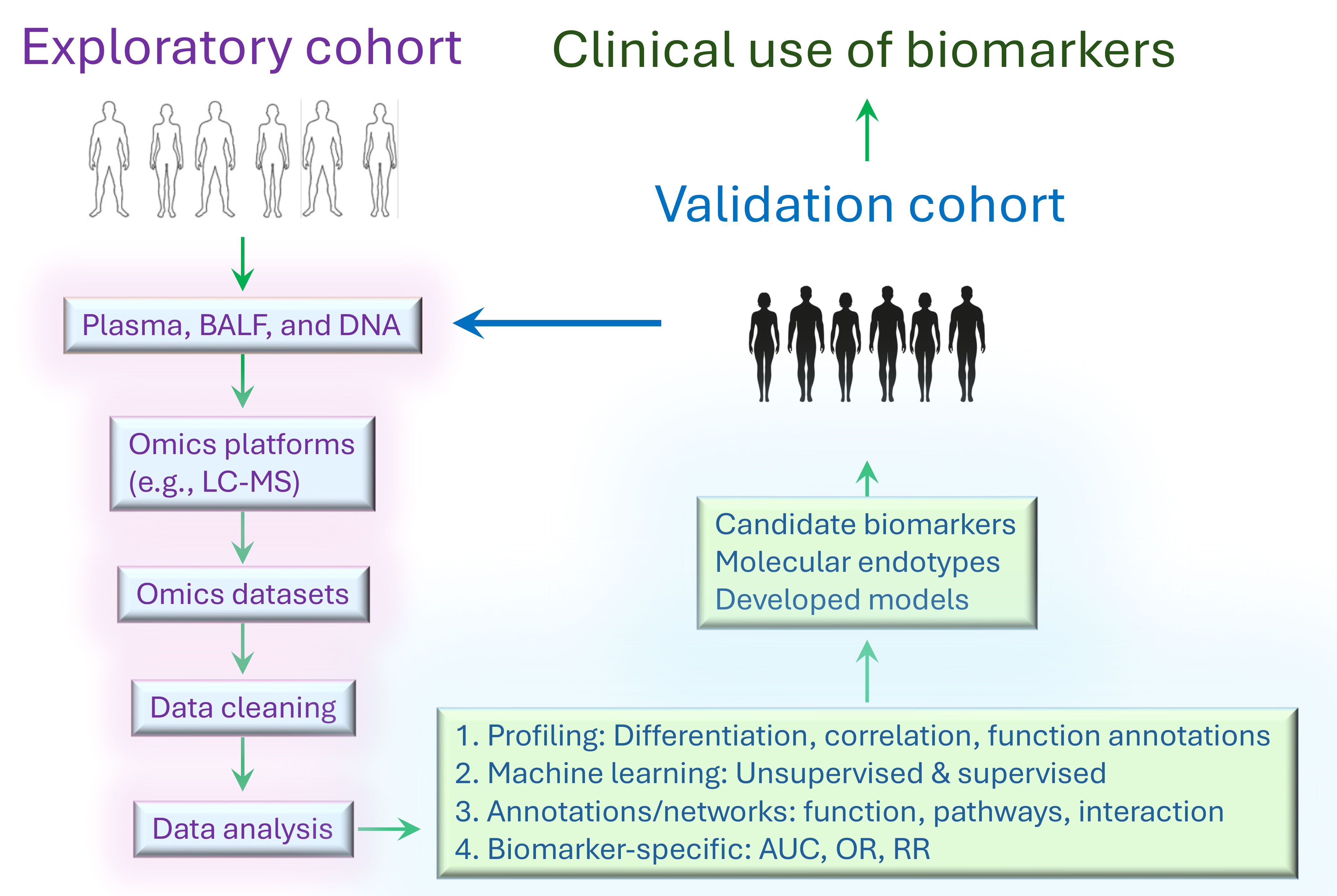
Figure 1 General workflow of Omics study to identify biomarkers and endotypes. First, liquid specimens and tissue samples collected from exploratory cohort are prepared ready for the analysis on the omics platforms. Second, omics datasets, including genomics, transcriptomics, proteomics, metabolomics, and microbiomics, are generated by experiments. Next, the datasets are pre-processed before statistical and machine learning analyses. Statistical analyses include differentiation expression tests, correlation analysis, logistic regression, Bayesian model, etc. Machine learning algorithms encompass supervised methods (e.g., gradient boosting, deep neural network, and laten classification and prediction) and unsupervised methods (e.g., PCA, k-means clustering, hierarchical clustering). Furthermore, network analysis can be performed with weighted gene co-expression network analysis (WGCNA), weighted protein correlation network analysis (WPCNA), genome-wide association study (GWAS) and meta-analysis. In general, function annotations are essential to rank differential gene-, transcript-, protein-, or metabolite-related signaling pathways, functions, and interactions. These analyses help identify candidate biomarkers, molecular endotypes, and unique phenotypes, along with developed predictive models. Specific metrics such as ROC AUC c statistic, odds ratio, risk ratio, and others are applied for biomarker comparisons. Finally, it is critical to validate the results in independent cohorts of multi-ethnic origins and under-represented populations, particularly since sarcoidosis is common in minorities.
3 Characteristics of clinical studies
3.1 Selection criteria of clinical studies to review
We searched the PubMed using sarcoidosis, biomarker, and endotype as input keywords. Only original clinical studies were included. The hits were subsequently categorized by the employed data analysis strategies, including classical statistics, AUC (area under curve), machine learning algorithms, and the utilization of omics platforms. We documented and compared the types of biomarkers, sources of specimens, sample sizes, statistical and machine learning methods used, performance metrics of identified biomarkers and endotypes, and validation procedures.
3.2 Types of biomarkers by purposes
Biomarker refers to a broad spectrum of biomedical features, including the specific analytes, anatomic features, pathophysiological characteristics, and pharmacologic responses to therapeutic interventions that can be measured accurately and reproducibly. The biomarkers of sarcoidosis can be categorized based on their clinical applications. “Diagnostic biomarkers” serve to differentiate sarcoidosis patients from healthy individuals. “Differential diagnosis biomarkers” aid in differentiating sarcoidosis from other similar conditions. Some biomarkers exhibit specificity for disease severity, inflammation, disease activity, and/or chronicity. “Prognostic biomarkers” are predictive for the outcomes of the disease, including mortality, lung fibrosis, and organ failure. Additionally, “predictive” biomarkers are indicative of treatment response and intervention efficacy (12–14, 18–25). As shown in Tables 1, 2, the most common biomarkers reported for sarcoidosis are for diagnosis, followed by prognosis, organ involvement, and treatment response.
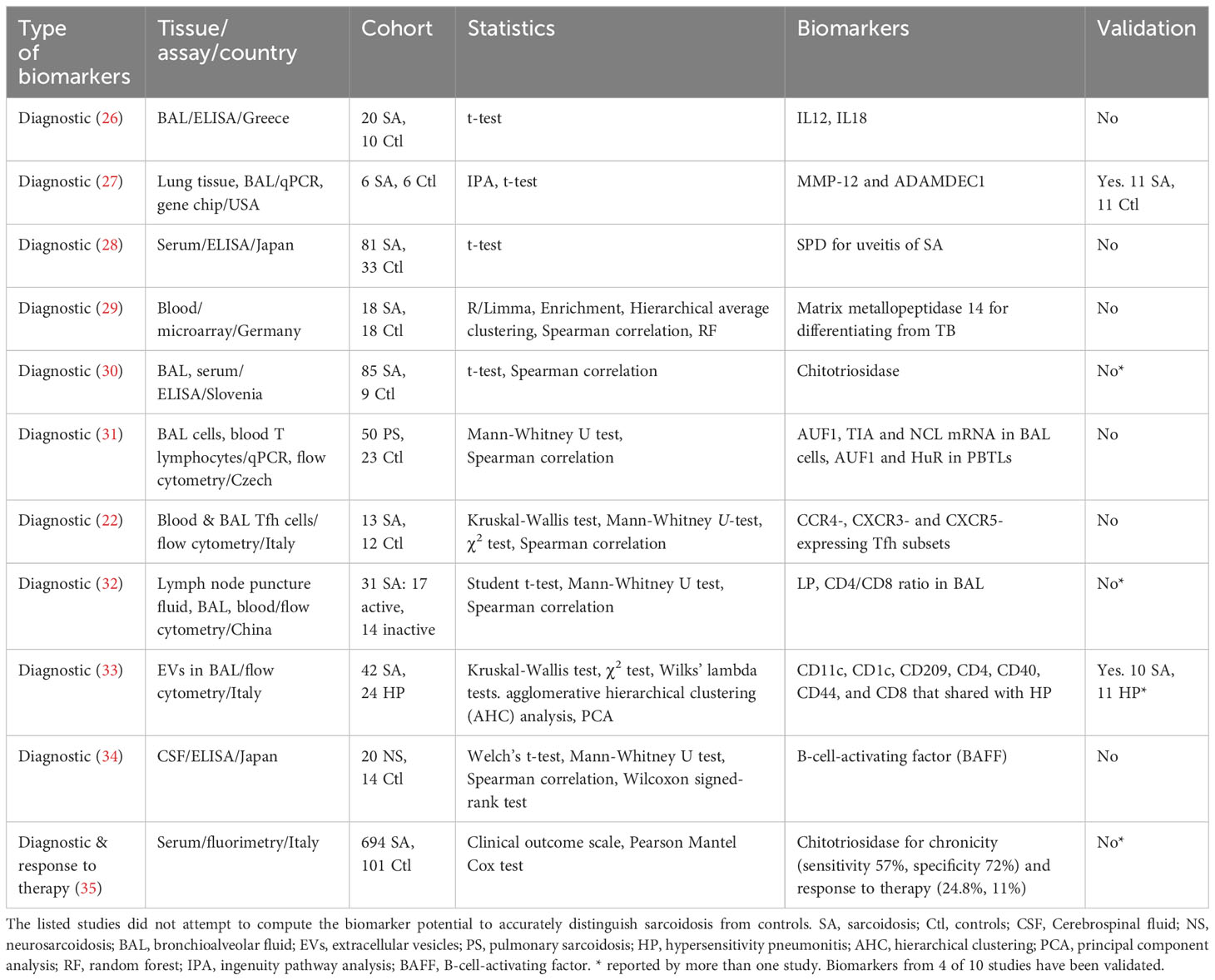
Table 1 Biomarkers associated or correlated with sarcoidosis, identified using non-Omics approaches.
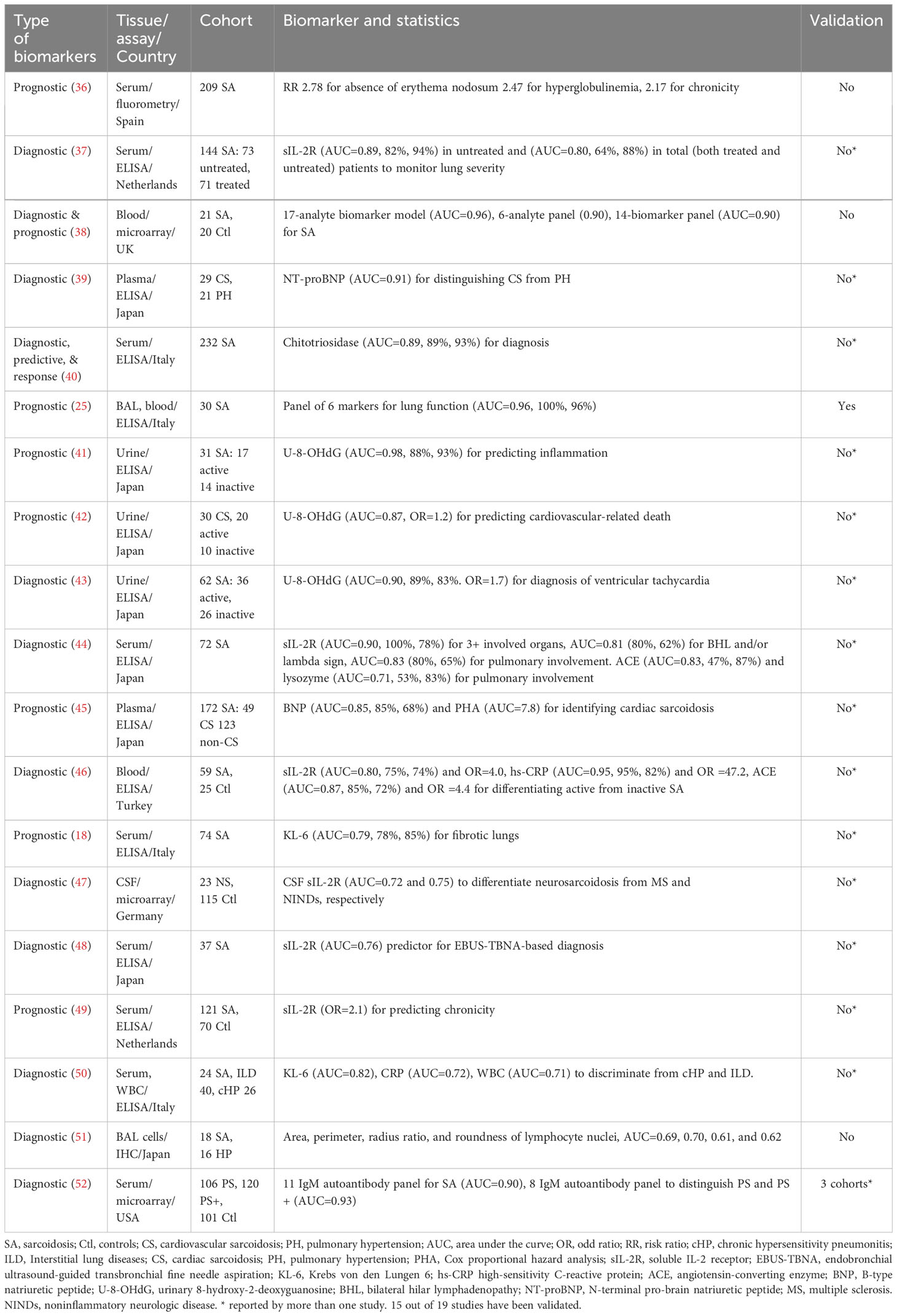
Table 2 Sarcoidosis biomarkers identified in non-omics studies that have been assessed for their potential to accurately distinguish disease groups.
Biomarker is generally identified combining biomedical tests and biostatistic/bioinformatic analyses. Biomedical measurements include ELISA, MRI, IHC, echocardiogram, flow cytometry, etc., as summarized in Tables 1–4 for sarcoidosis. These data will then be analyzed using t-test, odd ratio, AUC, logistic regression, and machine learning algorithms based on the nature of data. Validation in a separate cohort is essential for any potential biomarker candidate. AUC is an acceptable approach to compute the sensitivity, specificity, and accuracy of individual biomarker or a biomarker panel. This is a common strategy to identify and evaluate the biomarkers of sarcoidosis in both Omics and non-Omics studies.
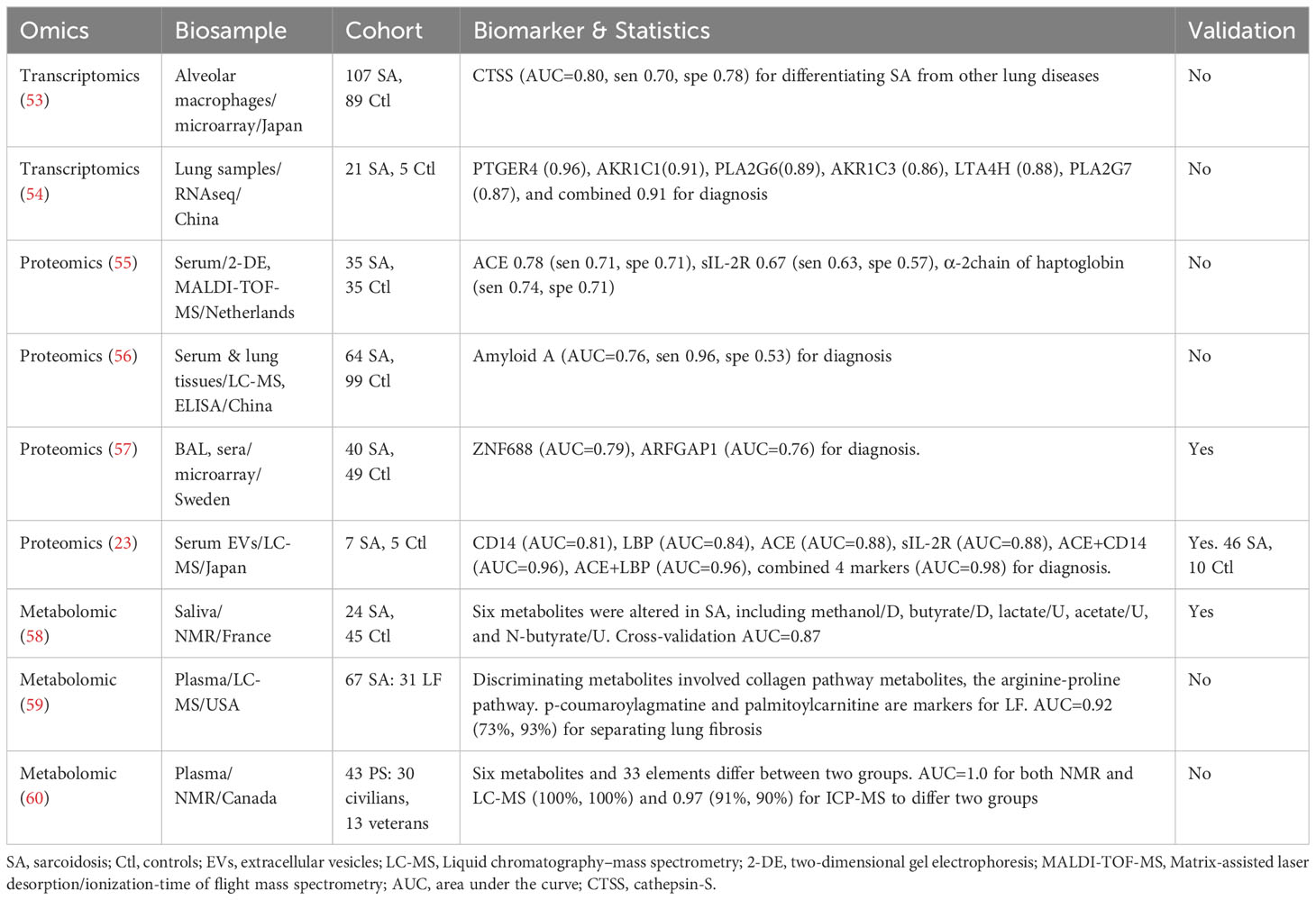
Table 3 Sarcoidosis Biomarkers identified in Omics studies that have been assessed for their potential to accurately distinguish disease groups.
3.3 Sources of specimens for biomarker study
Albeit sarcoidosis being a systemic disorder, both local and circulating biomarkers have been explored. Common biopsy specimens include whole blood, plasma, serum, blood cells, tissues from different organs, and liquid biosamples (bronchioalveolar lavage fluid (BAL), joint fluid, spinal fluid, urine, and lymph node puncture fluid), along with their derivatives (cells, extracellular vesicles (EVs), DNA, microRNA (miRNA), etc.). Both Omics and non-Omics approaches have been reported. Targeted non-Omics methods include colorimetry, fluorimetry, enzyme linked immunosorbent assay (ELISA), Western blot (WB), qPCR (quantitative polymerase chain reaction), microarray, flow cytometry, and immunohistochemistry (IHC) analyses of targeted molecules. The non-targeted Omics studies, on the other hand, utilized gene chips, advanced arrays, RNA sequencing (RNA-seq), mass spectrometry, nuclear magnetic resonance (NMR), and 16S rRNA-seq (see Section 4), permitting a more comprehensive screen, in an unbiased manner. Omics approaches have the advantage of being comprehensive and unbiassed despite being more expensive and computation intensive.
4 Sarcoidosis biomarkers identified by targeted non-omics studies
Studies designed to identify diagnostic markers typically compare patients with healthy controls. For the identification of differential diagnosis biomarkers, other diseases often serve as control groups. In contrast, individuals with only sarcoidosis are required for the studies aimed to identify predictive biomarkers, biomarkers for the response to therapy, activity, chronicity, organ involvement, and severity of the disease, with longitudinal follow up in some cases. The average sample size across 30 non-omics studies is 88, ranging from 6 to 694. Only two studies included a separate validation cohort (27, 33).
We first review cross-sectional studies (Tables 1–3). Among the diagnostic biomarkers for distinguishing sarcoidosis from healthy control, the following have been independently validated: serum and CSF soluble interleukin-2 receptor (sIL-2R) (AUCs: 0.67 - 0.90) (23, 37, 44, 46–49, 55), urinary U-8-OHdG (AUCs: 0.87 - 0.98) (41–43), serum angiotensin converting enzyme (ACE) (AUC: 0.78-0.88) (23, 44, 46, 55), serum chitotriosidase (AUC: 0.89) (30, 35, 40), serum KL-6 (AUC: 0.79-0.83) (18, 50), serum CRP (AUC: 0.72-0.95) (46, 50), and serum BNP (0.85-0.91) (39, 45). In addition, several isolated reports of diagnostic biomarkers have also been reported, without independent validation: BAL IL12, IL18, MMP14, CTSS, serum amyloid A, ZNF688, ARFGAP1, CD14, LBP, a-2chain of haptoglobin, and PHA, with AUCs ranging from 0.76 to 0.84. The best performers among these include serum LBP (AUCs: 0.84) and CD14 (AUC: 0.81) (23).
Serum sIL-2R have been reported to be reflective of lung dysfunction, inflammation, multiple organ involvement including thoracic phenotype, and diagnosis of neurosarcoidosis based on cerebrospinal fluid (CSF) level (37, 44, 46–49). Urinary 8-hydroxy-2′-deoxyguanosine (U-8-OHdG) has been reported to predict inflammation in active patients, cardiac death, and ventricular tachycardia (41–43). Serum chitotriosidase has been validated for the diagnosis, chronicity, and response to therapy (30, 35, 40), Serum ACE has been proposed as a biomarker for identifying active parenchymal infiltration and pulmonary involvement (44, 46). Similarly, C-reactive protein (CRP) can serve as a biomarker to discriminate between inactive and active cases, and also distinguishing sarcoidosis from interstitial lung disease (ILD) and chronic hypersensitivity pneumonitis (46, 50). Krebs von den Lungen-6 (KL-6) is a biomarker of fibrotic lungs in sarcoidosis (18, 50), as reported by Bergantini and coworkers. B-type natriuretic peptide (BNP) is a diagnostic marker for cardiac sarcoidosis and a predictor for heart failure (39, 45). Besides protein biomarkers, CD4 and CD8 T-cells, and their respective ratios in BAL extracellular vesicles (BAL EVs) and lymph node puncture fluid can potentially function as diagnostic biomarkers (32, 33). The sensitivity and specificity of these biomarkers vary. Other biomarkers reported in one study but not yet cross-validated include neopterin, interleukin-12 (IL12), interleukin 18 (IL18), surfactant protein D (SPD), metallic metalloproteinase-14 (MMP-14), a disintegrin and metalloproteinase domain-like protein decysin-1 (ADAMDEC1), B-cell-activating factor (BAFF), C-C motif chemokine receptor 4 (CCR4), chemokine receptor 3 (CXCR3), chemokine receptor 5 (CXCR5), lymphocyte profile (LP), and those composite biomarker panels (22, 25–29, 31–34, 36, 38, 51, 52).
5 Omics-based identification of biomarkers for sarcoidosis
In recent years, Omics studies for the identification of biomarkers and molecular endotypes have been emerging, using a wide array of platforms. They apply both machine learning algorithms and traditional biostatistics for data analysis. The biomarkers identified by genome-wide association and transcriptomics studies have been comprehensively reviewed recently (15, 20, 69, 70). Thus, this review will specifically delve into transcriptomics studies since 2018. Finally, we review other Omics studies, including proteomics, metabolomics, and microbiomics.
5.1 Transcriptomics
RNA-seq has been employed to identify transcriptomic biomarkers in biopsied lung tissue (54). Notably, prostaglandin E receptor 4 (PTGER4), aldo-keto reductase family 1 member C1 (AKR1C1), phospholipase A2 group VI (PLA2G6), aldo-keto reductase family 1 member C3 (AKR1C3), leukotriene A4 hydrolase (LTA4H), and phospholipase A2 group VI (PLA2G7) mRNA levels exhibited potential significance for distinguishing sarcoidosis from healthy controls, each yielding an AUC value exceeding 0.85 (Table 3). A gene microarray analysis unveiled Cathepsin-S (with an AUC of 0.80) in alveolar macrophages as a potential differentiator between sarcoidosis and other lung diseases (53).
Other transcriptomic studies have attempted to differentiate sarcoidosis patients by clinical phenotypes. Unsupervised clustering analyses have been used to identify biomarkers associated with pulmonary sarcoidosis, cardiac sarcoidosis, and other phenotypes (Table 4). miR-155 and miR-223 were associated with pulmonary sarcoidosis. In addition, upregulated TLR/NOD (toll-like receptor/nucleotide-binding oligomerization domain) signaling, intrinsic apoptosis, and inflammatory pathways were uncovered in sarcoidosis patients, compared to healthy controls (61). A clustering of 12 patients suggested an increase in mRNA for ribosome biogenesis and lymphocytes in BAL cells in all patients (62). Moreover, GPNMB (transmembrane glycoprotein NMB) emerged as a potential biomarker for multinucleated giant cells associated with cardiac sarcoidosis (63). It is worth noting that these studies are limited by their small sample sizes, which pose challenges in identifying highly sensitive and specific biomarkers. Another concern is the lack of cross-validation. Consequently, further investigations using independent cohorts, along with the integration of machine learning algorithms and classical statistics (AUC), warrant consideration.
5.2 Proteomics
Proteins are the main vehicles of cellular function, and their abnormal alterations can result in organ disorders. A couple of proteomic studies of sarcoidosis have been reported. Serum zinc finger protein 687 (ZNF688), ADP-ribosylation factor GTPase-activating protein 1 (ARFGAP1), CD14, and LBP (lipopolysaccharide-binding protein) have been identified as validated biomarkers for differentiating sarcoidosis from healthy control (23, 57). By comparison, serum α-2 chain of haptoglobin and amyloid A present potential as diagnostic biomarkers but await validation. Clustering analyses, including principal component analysis (PCA) and oracle product lifecycle analytics (OPLA), have been applied to select proteomics-based serum biomarkers for identifying sarcoidosis (Table 4). Typically, these analyses cluster protein panels to differentiate controls and disease cases. For example, a 25-protein panel related to Fcγ-mediated phagocytosis and clathrin-mediated endocytosis exhibited diagnostic potential, as did panels involving regulation-associated factors (64). Furthermore, Fc-regulation-associated factors and IgG-related factors have been reported to be biomarkers for the presence of Lofgren’s syndrome, a distinct phenotype of sarcoidosis (65, 66). The sample sizes of the studies using LC-MS (liquid chromatography mass spectroscopy) were generally small, with fewer than 10 cases. The identified proteins and protein panels should be analyzed individually for the AUC value of each panel member. Moreover, targeted proteomics using antibodies or aptamers as ligands, which exhibit increased technical sensitivity, have barely been applied to the study of sarcoidosis. Given that global proteomics is time-consuming and resource-intensive, there is a long way to go before the identification of individual proteins for clinical use and the translation of proteomics biomarkers to the bedside.
5.3 Metabolomics
To date, four studies have analyzed blood and saliva metabolites in sarcoidosis (58–60, 67). Either LC-MS or NMR was used to detect metabolites. Notably, a saliva-based panel of six metabolites demonstrated the ability to differentiate sarcoidosis patients from healthy controls, yielding an AUC of 0.87 (58). Moreover, plasma p-coumaroylagmatine and palmitoylcarnitine were identified as differential diagnosis biomarkers for lung fibrosis, with their involvement extending to collagen and arginine-proline pathways (59). To compare veteran (military or other occupation) from civilian sarcoidosis patients, one study identified six differentially expressed metabolites and 33 trace elements (60). Metabolomics has also been applied to characterize lipid profile responses to exercise in sarcoidosis patients (67). Fatty acids, triglycerides, and total cholesterol were significantly reduced in patients on exercise regimen, suggesting the potential of using blood lipid profile as a prognosticator for recovery of lung function. Clearly, metabolomics is a powerful approach to identify potential biomarkers for sarcoidosis. More studies are warranted to validate the identified metabolic biomarkers and to identify additional biomarkers for diagnosis, differential diagnosis, activity, severity, chronicity of the disease, outcomes, and response to treatment.
5.4 Microbiome
Accumulating evidence suggests that the crosstalk between the gut microbiota and the lung, known as the gut-lung axis, is critical. The lung microbiota of sarcoidosis has been reviewed recently (71). The identification of microbial biomarkers for sarcoidosis is an emerging direction. To date, only one study has performed 16S rRNA-seq of BAL on 8 sarcoidosis cases aiming to identify microbial markers for diagnosis. This study identified three taxa as potential biomarkers in BAL specific to sarcoidosis: Corynebacteriales, Corynebacterium, and Neisseria.OTUID_476, each with a linear discriminant analysis (LDA) score greater than 3.0 (68). Nonetheless, these potential microbial biomarkers await confirmation and rigorous statistical analyses. In addition to lung microbiota, microbial markers should be identified from the gut or other involved organs in future studies.
6 Molecular endotypes
The identification of molecular endotypes for a given disease will shed light on disease pathogenicity, diagnosis, stratification, prognosis, and development of new personalized therapies. Currently, there is only one report on the “transcriptomic” endotypes of sarcoidosis (11). Four potential endotypes were identified by unsupervised analysis of RNA-seq data in BAL cells, including hilar lymphadenopathy with increased acute T-cell immune response, extraocular organ involvement with phosphatidylinositol-3-kinase (PI3K) activation pathways, chronic and multiorgan disease with increased immune response pathways, and multiorgan disease with increased IL-1 and IL-18 immune and inflammatory responses. These mRNA-based endotypes based on signatures from BAL cells await independent validation (72). In addition, a clinical trial has recently been registered to define the endotypes of CD4 T helper and T regulator cell in sarcoidosis (73). Clearly, molecular endotype studies of sarcoidosis is just in its infancy.
7 Conclusion
Despite decades of both basic and clinician research, our understanding of sarcoidosis remains limited. No specific interventions exist for systemic or single organ sarcoidosis due to the incomplete understanding of its pathogenicity. Consequently, there is an urgent need to find the molecular basis of various phenotypes and biomarkers. This pursuit is crucial for predicting long-term outcomes and responses to the therapy targeting the different manifestations of sarcoidosis. So far, a substantial portion (70%) of biomarkers identified through targeted non-Omics studies have been cross validated by different groups, various phenotypes, or distinct organ involvements (Table 5). These non-Omics-derived biomarkers may serve multiple purpose, including roles in diagnosis, differential diagnosis, prognosis determination, and assessment of disease activity, chronicity, and severity, and evaluation of therapeutic response. However, these focused studies need to be expanded to or fortified with larger, unbiased Omics based studies, in order to uncover improved biomarkers for this disease.
Very few individual biomarkers are identified by both Omics and non-Omics studies. It is most likely due to divergent approaches and tissues. Non-Omics studies measured one or few biomarkers at the protein level. In contrast, Omics studies profile the landscape of genes, transcripts, proteins or metabolites and identify a panel of top-ranked biomarkers. These Omics biomarkers shall be validated by other clinical studies independently. One challenge of implementing these biomarkers clinically is the difficulty of identifying these phenotypes at the bedside using the same approaches. It remains a question whether genomics, transcriptomics, and metabolomics biomarkers could be validated by proteomics. In addition, the organ specificity of identified biomarkers may lead to the inconsistency between identified Omics and non-Omics biomarkers. Of note, the organ specific biomarker could be applied to differentiate involved organs. All validated high-quality biomarkers are highlighted in italic in Table 5. These validated biomarkers are recommended for sarcoidosis. Without doubt, the combination of non-Omics and Omics assays will improve the identification of biomarkers in sarcoidosis.
One advantage of Omics studies is their high throughput capacity. This feature enables the identification of molecular endotypes in sarcoidosis and multiple biomarkers ranked by their importance. The development of new bioinformatics and machine learning algorithms holds significant potential for extracting more accurate or predictive information from Omics datasets, to prioritize critical biomarkers, meet clinical needs, and to identify molecular endotypes associated with different phenotypes. With respect to sarcoidosis, Omics studies are still in its infancy. These reported Omics studies allude to several potential biomarkers/panels, signaling pathways, and integrated networks, but the general paucity of sensitivity, specificity and accuracy comparisons, insufficient statistical power, and few cross-validations prevent rigorous conclusions from being drawn. Most of Omics-derived biomarker panels are not ready to be translated to bedside. More Omics studies and multi-Omic integrative investigations are needed to validate published biomarkers, and to identify more accurate biomarkers for sarcoidosis, using well annotated reference cohorts. Clinical trials are necessary to evaluate the clinical application of top-ranked biomarkers, each on an individual basis. To sum, there is an urgent need to identify novel sarcoidosis biomarkers and molecular endotypes to facilitate disease diagnosis and prognosis, resolve disease heterogeneity and facilitate personalized medicine.
Author contributions
H-LJ: Conceptualization, Funding acquisition, Project administration, Resources, Supervision, Validation, Visualization, Writing – original draft, Writing – review & editing. NX: Writing – original draft, Writing – review & editing. CM: Writing – review & editing. XY: Writing – review & editing. KJ: Writing – review & editing. RZ: Writing – original draft, Writing – review & editing. QZ: Writing – review & editing. VG: Writing – review & editing.
Funding
The author(s) declare financial support was received for the research, authorship, and/or publication of this article. This study was supported by NIH R01HL134828 (to H-LJ), R01GM111295 (to QZ),R21AI166913 (to QZ), and R21AI178434 (to QZ).
Conflict of interest
The authors declare that the research was conducted in the absence of any commercial or financial relationships that could be construed as a potential conflict of interest.
Publisher’s note
All claims expressed in this article are solely those of the authors and do not necessarily represent those of their affiliated organizations, or those of the publisher, the editors and the reviewers. Any product that may be evaluated in this article, or claim that may be made by its manufacturer, is not guaranteed or endorsed by the publisher.
Glossary
References
1. Grunewald J, Grutters JC, Arkema EV, Saketkoo LA, Moller DR, Müller-Quernheim J. Sarcoidosis. Nat Rev Dis Primers. (2019) 5(1):45. doi: 10.1038/s41572-019-0096-x
2. Spagnolo P, Rossi G, Trisolini R, Sverzellati N, Baughman RP, Wells AU. Pulmonary sarcoidosis. Lancet Respir Med (2018) 6(5):389–402. doi: 10.1016/S2213-2600(18)30064-X
3. Nardi A, Brillet PY, Letoumelin P, Girard F, Brauner M, Uzunhan Y, et al. Stage IV sarcoidosis: comparison of survival with the general population and causes of death. Eur Respir J (2011) 38(6):1368–73. doi: 10.1183/09031936.00187410
4. Gupta R, Kim JS, Baughman RP. An expert overview of pulmonary fibrosis in sarcoidosis. Expert Rev Respir Med (2023) 17(2):119–30. doi: 10.1080/17476348.2023.2183193
5. Patterson KC, Strek ME. Pulmonary fibrosis in sarcoidosis. Clinical features and outcomes. Ann Am Thorac Soc (2013) 10(4):362–70. doi: 10.1513/AnnalsATS.201303-069FR
6. Drent M, Crouser ED, Grunewald J. Challenges of sarcoidosis and its management. N Engl J Med (2021) 385(11):1018–32. doi: 10.1056/NEJMra2101555
7. Belperio JA, Shaikh F, Abtin FG, Fishbein MC, Weigt SS, Saggar R, et al. Diagnosis and treatment of pulmonary sarcoidosis: A review. Jama (2022) 327(9):856–67. doi: 10.1001/jama.2022.1570
8. Lin NW, Arbet J, Mroz MM, Liao SY, Restrepo CI, Mayer AS, et al. Clinical phenotyping in sarcoidosis using cluster analysis. Respir Res (2022) 23(1):88. doi: 10.1186/s12931-022-01993-z
9. Fermon C, El-Jammal T, Kodjikian L, Burillon C, Hot A, Pérard L, et al. Identification of multidimensional phenotypes using cluster analysis in sarcoid uveitis patients. Am J Ophthalmol (2022) 242:107–15. doi: 10.1016/j.ajo.2022.06.001
10. d’Alessandro M, Carleo A, Cameli P, Bergantini L, Perrone A, Vietri L, et al. BAL biomarkers’ panel for differential diagnosis of interstitial lung diseases. Clin Exp Med (2020) 20(2):207–16. doi: 10.1007/s10238-020-00608-5
11. Vukmirovic M, Yan X, Gibson KF, Gulati M, Schupp JC, DeIuliis G, et al. Transcriptomics of bronchoalveolar lavage cells identifies new molecular endotypes of sarcoidosis. Eur Respir J (2021) 58(6):2002950. doi: 10.1183/13993003.02950-2020
12. Kraaijvanger R, Janssen Bonás M, Vorselaars ADM, Veltkamp M. Biomarkers in the diagnosis and prognosis of sarcoidosis: current use and future prospects. Front Immunol (2020) 11:1443. doi: 10.3389/fimmu.2020.01443
13. Ramos-Casals M, Retamozo S, Sisó-Almirall A, Pérez-Alvarez R, Pallarés L, Brito-Zerón P. Clinically-useful serum biomarkers for diagnosis and prognosis of sarcoidosis. Expert Rev Clin Immunol (2019) 15(4):391–405. doi: 10.1080/1744666X.2019.1568240
14. Chopra A, Kalkanis A, Judson MA. Biomarkers in sarcoidosis. Expert Rev Clin Immunol (2016) 12(11):1191–208. doi: 10.1080/1744666X.2016.1196135
15. Schupp JC, Vukmirovic M, Kaminski N, Prasse A. Transcriptome profiles in sarcoidosis and their potential role in disease prediction. Curr Opin Pulm Med (2017) 23(5):487–92. doi: 10.1097/MCP.0000000000000403
16. Komorowski M, Green A, Tatham KC, Seymour C, Antcliffe D. Sepsis biomarkers and diagnostic tools with a focus on machine learning. EBioMedicine (2022) 86:104394. doi: 10.1016/j.ebiom.2022.104394
17. Tan MS, Cheah PL, Chin AV, Looi LM, Chang SW. A review on omics-based biomarkers discovery for Alzheimer’s disease from the bioinformatics perspectives: Statistical approach vs machine learning approach. Comput Biol Med (2021) 139:104947. doi: 10.1016/j.compbiomed.2021.104947
18. Bergantini L, Bianchi F, Cameli P, Mazzei MA, Fui A, Sestini P, et al. Prognostic biomarkers of sarcoidosis: A comparative study of serum chitotriosidase, ACE, lysozyme, and KL-6. Dis Markers. (2019) 2019:8565423. doi: 10.1155/2019/8565423
19. Carleo A, Bennett D, Rottoli P. Biomarkers in sarcoidosis: the contribution of system biology. Curr Opin Pulm Med (2016) 22(5):509–14. doi: 10.1097/MCP.0000000000000306
20. Casanova N, Zhou T, Knox KS, Garcia JGN. Identifying novel biomarkers in sarcoidosis using genome-based approaches. Clin Chest Med (2015) 36(4):621–30. doi: 10.1016/j.ccm.2015.08.005
21. Casanova NG, Reyes-Hernon V, Gregory T, Sun B, Bermudez T, Hufford MK, et al. Biochemical and genomic identification of novel biomarkers in progressive sarcoidosis: HBEGF, eNAMPT, and ANG-2. Front Med (Lausanne). (2022) 9:1012827. doi: 10.3389/fmed.2022.1012827
22. d’Alessandro M, Bergantini L, Cameli P, Mezzasalma F, Refini RM, Pieroni M, et al. Adaptive immune system in pulmonary sarcoidosis-Comparison of peripheral and alveolar biomarkers. Clin Exp Immunol (2021) 205(3):406–16. doi: 10.1111/cei.13635
23. Futami Y, Takeda Y, Koba T, Narumi R, Nojima Y, Ito M, et al. Identification of CD14 and lipopolysaccharide-binding protein as novel biomarkers for sarcoidosis using proteomics of serum extracellular vesicles. Int Immunol (2022) 34(6):327–40. doi: 10.1093/intimm/dxac009
24. Lin NW, Maier LA, Mroz MM, Jacobson S, MacPhail K, Liu S, et al. Genomic biomarkers in chronic beryllium disease and sarcoidosis. Respir Med (2021) 187:106390. doi: 10.1016/j.rmed.2021.106390
25. Paone G, Leone A, Batzella S, Conti V, Belli F, De Marchis L, et al. Use of discriminant analysis in assessing pulmonary function worsening in patients with sarcoidosis by a panel of inflammatory biomarkers. Inflammation Res (2013) 62(3):325–32. doi: 10.1007/s00011-012-0585-9
26. Antoniou KM, Tzouvelekis A, Alexandrakis MG, Tsiligianni I, Tzanakis N, Sfiridaki K, et al. Upregulation of Th1 cytokine profile (IL-12, IL-18) in bronchoalveolar lavage fluid in patients with pulmonary sarcoidosis. J Interferon Cytokine Res (2006) 26(6):400–5. doi: 10.1089/jir.2006.26.400
27. Crouser ED, Culver DA, Knox KS, Julian MW, Shao G, Abraham S, et al. Gene expression profiling identifies MMP-12 and ADAMDEC1 as potential pathogenic mediators of pulmonary sarcoidosis. Am J Respir Crit Care Med (2009) 179(10):929–38. doi: 10.1164/rccm.200803-490OC
28. Kitaichi N, Kitamura M, Namba K, Ishida S, Ohno S. Elevation of surfactant protein D, a pulmonary disease biomarker, in the sera of uveitis patients with sarcoidosis. Jpn J Ophthalmol (2010) 54(1):81–4. doi: 10.1007/s10384-009-0756-6
29. Maertzdorf J, Weiner J 3rd, Mollenkopf HJ, Bauer T, Prasse A, Müller-Quernheim J, et al. Common patterns and disease-related signatures in tuberculosis and sarcoidosis. Proc Natl Acad Sci U S A. (2012) 109(20):7853–8. doi: 10.1073/pnas.1121072109
30. Harlander M, Salobir B, Zupančič M, Terčelj M. Bronchoalveolar lavage chitotriosidase activity as a biomarker of sarcoidosis. Sarcoidosis Vasc Diffuse Lung Dis (2016) 32(4):313–7.
31. Navratilova Z, Novosadova E, Hagemann-Jensen M, Kullberg S, Kolek V, Grunewald J, et al. Expression profile of six RNA-binding proteins in pulmonary sarcoidosis. PloS One (2016) 11(8):e0161669. doi: 10.1371/journal.pone.0161669
32. Mi S, Cui N, Feng X, Jiang C, Huang K, Zhang L. Analysis of lymphocyte profile in lymph nodes, bronchoalveolar lavage fluid and peripheral blood in patients with stage II sarcoidosis. Respir Med (2022) 205:107039. doi: 10.1016/j.rmed.2022.107039
33. d’Alessandro M, Gangi S, Soccio P, Cantó E, Osuna-Gómez R, Bergantini L, et al. The effects of interstitial lung diseases on alveolar extracellular vesicles profile: A multicenter study. Int J Mol Sci (2023) 24(4):4071. doi: 10.3390/ijms24044071
34. Sumi K, Masuda T, Kimura N, Akiyoshi Y, Obayashi K, Matsubara E. Cerebrospinal fluid B-cell activating factor levels as a novel biomarker in patients with neurosarcoidosis. J Neurol Sci (2023) 449:120668. doi: 10.1016/j.jns.2023.120668
35. Bennett D, Cameli P, Lanzarone N, Carobene L, Bianchi N, Fui A, et al. Chitotriosidase: a biomarker of activity and severity in patients with sarcoidosis. Respir Res (2020) 21(1):6. doi: 10.1186/s12931-019-1263-z
36. Mañá J, Salazar A, Pujol R, Manresa F. Are the pulmonary function tests and the markers of activity helpful to establish the prognosis of sarcoidosis? Respiration (1996) 63(5):298–303. doi: 10.1159/000196564
37. Rothkrantz-Kos S, van Dieijen-Visser MP, Mulder PG, Drent M. Potential usefulness of inflammatory markers to monitor respiratory functional impairment in sarcoidosis. Clin Chem (2003) 49(9):1510–7. doi: 10.1373/49.9.1510
38. Beirne P, Pantelidis P, Charles P, Wells AU, Abraham DJ, Denton CP, et al. Multiplex immune serum biomarker profiling in sarcoidosis and systemic sclerosis. Eur Respir J (2009) 34(6):1376–82. doi: 10.1183/09031936.00028209
39. Handa T, Nagai S, Ueda S, Chin K, Ito Y, Watanabe K, et al. Significance of plasma NT-proBNP levels as a biomarker in the assessment of cardiac involvement and pulmonary hypertension in patients with sarcoidosis. Sarcoidosis Vasc Diffuse Lung Dis (2010) 27(1):27–35.
40. Bargagli E, Bennett D, Maggiorelli C, Di Sipio P, Margollicci M, Bianchi N, et al. Human chitotriosidase: a sensitive biomarker of sarcoidosis. J Clin Immunol (2013) 33(1):264–70. doi: 10.1007/s10875-012-9754-4
41. Kobayashi S, Myoren T, Oda S, Inari M, Ishiguchi H, Murakami W, et al. Urinary 8-hydroxy-2’-deoxyguanosine as a novel biomarker of inflammatory activity in patients with cardiac sarcoidosis. Int J Cardiol (2015) 190:319–28. doi: 10.1016/j.ijcard.2015.04.144
42. Myoren T, Kobayashi S, Oda S, Nanno T, Ishiguchi H, Murakami W, et al. An oxidative stress biomarker, urinary 8-hydroxy-2’-deoxyguanosine, predicts cardiovascular-related death after steroid therapy for patients with active cardiac sarcoidosis. Int J Cardiol (2016) 212:206–13. doi: 10.1016/j.ijcard.2016.03.003
43. Ishiguchi H, Kobayashi S, Myoren T, Kohno M, Nanno T, Murakami W, et al. Urinary 8-hydroxy-2’-deoxyguanosine as a myocardial oxidative stress marker is associated with ventricular tachycardia in patients with active cardiac sarcoidosis. Circ Cardiovasc Imaging (2017) 10(12):e006764. doi: 10.1161/CIRCIMAGING.117.006764
44. Thi Hong Nguyen C, Kambe N, Kishimoto I, Ueda-Hayakawa I, Okamoto H. Serum soluble interleukin-2 receptor level is more sensitive than angiotensin-converting enzyme or lysozyme for diagnosis of sarcoidosis and may be a marker of multiple organ involvement. J Dermatol (2017) 44(7):789–97. doi: 10.1111/1346-8138.13792
45. Kiko T, Yoshihisa A, Kanno Y, Yokokawa T, Abe S, Miyata-Tatsumi M, et al. A multiple biomarker approach in patients with cardiac sarcoidosis. Int Heart J (2018) 59(5):996–1001. doi: 10.1536/ihj.17-695
46. Uysal P, Durmus S, Sozer V, Gelisgen R, Seyhan EC, Erdenen F, et al. YKL-40, soluble IL-2 receptor, angiotensin converting enzyme and C-reactive protein: comparison of markers of sarcoidosis activity. Biomolecules (2018) 8(3):84. doi: 10.3390/biom8030084
47. Otto C, Wengert O, Unterwalder N, Meisel C, Ruprecht K. Analysis of soluble interleukin-2 receptor as CSF biomarker for neurosarcoidosis. Neurol Neuroimmunol Neuroinflamm. (2020) 7(4):e725. doi: 10.1212/NXI.0000000000000725
48. Miyata J, Ogawa T, Tagami Y, Sato T, Nagayama M, Hirano T, et al. Serum soluble interleukin-2 receptor level is a predictive marker for EBUS-TBNA-based diagnosis of sarcoidosis. Sarcoidosis Vasc Diffuse Lung Dis (2020) 37(1):8–16. doi: 10.36141/svdld.v37i1.8313
49. Schimmelpennink MC, Quanjel M, Vorselaars A, Wiertz I, Veltkamp M, Van Moorsel C, et al. Value of serum soluble interleukin-2 receptor as a diagnostic and predictive biomarker in sarcoidosis. Expert Rev Respir Med (2020) 14(7):749–56. doi: 10.1080/17476348.2020.1751614
50. Bergantini L, d’Alessandro M, Vietri L, Rana GD, Cameli P, Acerra S, et al. Utility of serological biomarker’ panels for diagnostic accuracy of interstitial lung diseases. Immunol Res (2020) 68(6):414–21. doi: 10.1007/s12026-020-09158-0
51. Horimasu Y, Yamaguchi K, Sakamoto S, Masuda T, Miyamoto S, Nakashima T, et al. Quantitative parameters of lymphocyte nuclear morphology in bronchoalveolar lavage fluid as novel biomarkers for sarcoidosis. Orphanet J Rare Dis (2021) 16(1):298. doi: 10.1186/s13023-021-01926-x
52. Khassawneh B, Zhu C, Barkes B, Vestal B, Shrock S, Gillespie M, et al. Autoantibody profile in sarcoidosis, analysis from the GRADS sarcoidosis cohort. PloS One (2022) 17(10):e0274381. doi: 10.1371/journal.pone.0274381
53. Tanaka H, Yamaguchi E, Asai N, Yokoi T, Nishimura M, Nakao H, et al. Cathepsin S, a new serum biomarker of sarcoidosis discovered by transcriptome analysis of alveolar macrophages. Sarcoidosis Vasc Diffuse Lung Dis (2019) 36(2):141–7. doi: 10.36141/svdld.v36i2.7620
54. Chai Q, Lu Z, Liu Z, Zhong Y, Zhang F, Qiu C, et al. Lung gene expression signatures suggest pathogenic links and molecular markers for pulmonary tuberculosis, adenocarcinoma and sarcoidosis. Commun Biol (2020) 3(1):604. doi: 10.1038/s42003-020-01318-0
55. Bons JA, Drent M, Bouwman FG, Mariman EC, van Dieijen-Visser MP, Wodzig WK. Potential biomarkers for diagnosis of sarcoidosis using proteomics in serum. Respir Med (2007) 101(8):1687–95. doi: 10.1016/j.rmed.2007.03.002
56. Zhang Y, Chen X, Hu Y, Du S, Shen L, He Y, et al. Preliminary characterizations of a serum biomarker for sarcoidosis by comparative proteomic approach with tandem-mass spectrometry in ethnic Han Chinese patients. Respir Res (2013) 14(1):18. doi: 10.1186/1465-9921-14-18
57. Häggmark A, Hamsten C, Wiklundh E, Lindskog C, Mattsson C, Andersson E, et al. Proteomic profiling reveals autoimmune targets in sarcoidosis. Am J Respir Crit Care Med (2015) 191(5):574–83. doi: 10.1164/rccm.201407-1341OC
58. Duchemann B, Triba MN, Guez D, Rzeznik M, Savarin P, Nunes H, et al. Nuclear magnetic resonance spectroscopic analysis of salivary metabolome in sarcoidosis. Sarcoidosis Vasc Diffuse Lung Dis (2016) 33(1):10–6.
59. Mirsaeidi M, Banoei MM, Nienow CK, Abassi T, Hakim A, Schraufnagel D, et al. Plasma metabolomic profile in fibrosing pulmonary sarcoidosis. Sarcoidosis Vasc Diffuse Lung Dis (2016) 33(1):29–38.
60. Banoei MM, Iupe I, Bazaz RD, Campos M, Vogel HJ, Winston BW, et al. Metabolomic and metallomic profile differences between Veterans and Civilians with Pulmonary Sarcoidosis. Sci Rep (2019) 9(1):19584. doi: 10.1038/s41598-019-56174-8
61. Kachamakova-Trojanowska N, Jazwa-Kusior A, Szade K, Kasper L, Soja J, Andrychiewicz A, et al. Molecular profiling of regulatory T cells in pulmonary sarcoidosis. J Autoimmun (2018) 94:56–69. doi: 10.1016/j.jaut.2018.07.012
62. Paplińska-Goryca M, Goryca K, Misiukiewicz-Stępień P, Nejman-Gryz P, Proboszcz M, Górska K, et al. mRNA expression profile of bronchoalveolar lavage fluid cells from patients with idiopathic pulmonary fibrosis and sarcoidosis. Eur J Clin Invest. (2019) 49(9):e13153. doi: 10.1111/eci.13153
63. Liu J, Ma P, Lai L, Villanueva A, Koenig A, Bean GR, et al. Transcriptional and immune landscape of cardiac sarcoidosis. Circ Res (2022) 131(8):654–69. doi: 10.1161/CIRCRESAHA.121.320449
64. Silva E, Souchelnytskyi S, Kasuga K, Eklund A, Grunewald J, Wheelock ÅM. Quantitative intact proteomics investigations of alveolar macrophages in sarcoidosis. Eur Respir J (2013) 41(6):1331–9. doi: 10.1183/09031936.00178111
65. Kjellin H, Silva E, Branca RM, Eklund A, Jakobsson PJ, Grunewald J, et al. Alterations in the membrane-associated proteome fraction of alveolar macrophages in sarcoidosis. Sarcoidosis Vasc Diffuse Lung Dis (2016) 33(1):17–28.
66. Lundström SL, Heyder T, Wiklundh E, Zhang B, Eklund A, Grunewald J, et al. SpotLight proteomics-A igG-enrichment phenotype profiling approach with clinical implications. Int J Mol Sci (2019) 20(9):2157. doi: 10.3390/ijms20092157
67. Jastrzebski D, Toczylowska B, Zieminska E, Zebrowska A, Kostorz-Nosal S, Swietochowska E, et al. The effects of exercise training on lipid profile in patients with sarcoidosis. Sci Rep (2021) 11(1):5551. doi: 10.1038/s41598-021-84815-4
68. Gupta S, Shariff M, Chaturvedi G, Sharma A, Goel N, Yadav M, et al. Comparative analysis of the alveolar microbiome in COPD, ECOPD, Sarcoidosis, and ILD patients to identify respiratory illnesses specific microbial signatures. Sci Rep (2021) 11(1):3963. doi: 10.1038/s41598-021-83524-2
69. Calender A, Weichhart T, Valeyre D, Pacheco Y. Current insights in genetics of sarcoidosis: functional and clinical impacts. J Clin Med (2020) 9(8):2633. doi: 10.3390/jcm9082633
70. Garman L, Montgomery CG, Rivera NV. Recent advances in sarcoidosis genomics: epigenetics, gene expression, and gene by environment (G × E) interaction studies. Curr Opin Pulm Med (2020) 26(5):544–53. doi: 10.1097/MCP.0000000000000719
71. Arger NK, O’Connor B, Koth LL. Molecular profiling in sarcoidosis. Curr Opin Pulm Med (2020) 26(5):562–7. doi: 10.1097/MCP.0000000000000716
72. Fraser E, Weeratunga P, Ho L-P. Management of sarcoidosis: when to treat, how to treat and for how long? Curr Pulmonology Rep (2022) 11(4):151–9. doi: 10.1007/s13665-022-00298-2
73. Koth LL, Harmacek LD, White EK, Arger NK, Powers L, Werner BR, et al. Defining CD4 T helper and T regulatory cell endotypes of progressive and remitting pulmonary sarcoidosis (BRITE): protocol for a US-based, multicentre, longitudinal observational bronchoscopy study. BMJ Open (2021) 11(11):e056841. doi: 10.1136/bmjopen-2021-056841
Keywords: sarcoidosis, biomarker, endotype, omics, machine learning algorithms
Citation: Ji H-L, Xi NMS, Mohan C, Yan X, Jain KG, Zang QS, Gahtan V and Zhao R (2024) Biomarkers and molecular endotypes of sarcoidosis: lessons from omics and non-omics studies. Front. Immunol. 14:1342429. doi: 10.3389/fimmu.2023.1342429
Received: 21 November 2023; Accepted: 05 December 2023;
Published: 04 January 2024.
Edited by:
Guo-Chang Fan, University of Cincinnati, United StatesReviewed by:
Luhan Yang, Eurofins DiscoverX Products, United StatesWenxin Yu, Genentech Inc., United States
Songyun Deng, Mylike Aesthetic Hospital, China
Copyright © 2024 Ji, Xi, Mohan, Yan, Jain, Zang, Gahtan and Zhao. This is an open-access article distributed under the terms of the Creative Commons Attribution License (CC BY). The use, distribution or reproduction in other forums is permitted, provided the original author(s) and the copyright owner(s) are credited and that the original publication in this journal is cited, in accordance with accepted academic practice. No use, distribution or reproduction is permitted which does not comply with these terms.
*Correspondence: Hong-Long Ji, aGppQGx1Yy5lZHU=