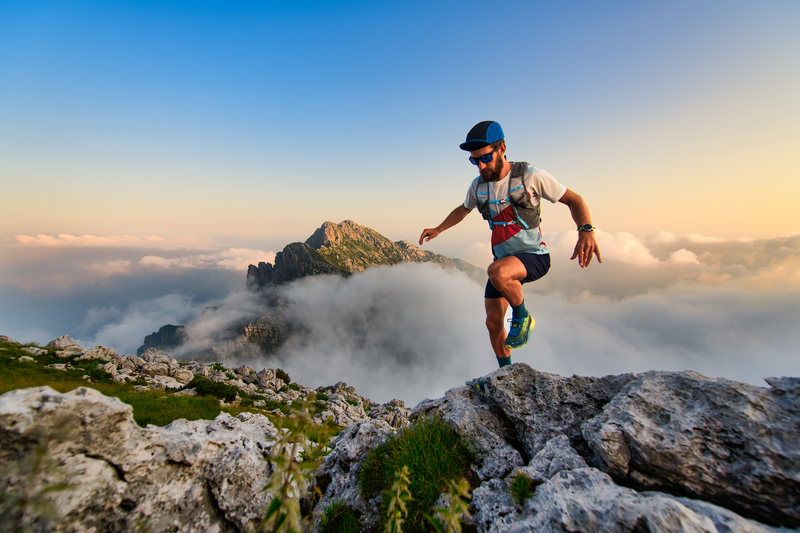
94% of researchers rate our articles as excellent or good
Learn more about the work of our research integrity team to safeguard the quality of each article we publish.
Find out more
ORIGINAL RESEARCH article
Front. Immunol. , 11 January 2024
Sec. Inflammation
Volume 14 - 2023 | https://doi.org/10.3389/fimmu.2023.1334361
Objective: Osteoarthritis (OA) is the most prevalent joint disease globally, serving as a primary cause of pain and disability. However, the pathological processes underlying OA remain incompletely understood. Several studies have noted an association between cytokines and OA, yet the causal link between them remains ambiguous. This study aims to identify cytokines potentially causally related to OA using Mendelian randomization (MR) analysis, informing early clinical diagnosis and treatment decisions.
Methods: We conducted a genome-wide association study (GWAS) on 12 OA traits involving 177,517 cases and 649,173 controls from 9 international cohorts. For discovery MR analysis, we used 103 cytokines from two European populations as instrumental variables (IVs). Concurrently, another European population OA GWAS database (36,185 cases and 135,185 controls) was used to replicate MR analysis, employing the inverse variance weighted (IVW) method as the primary analytic approach. Additional methods tested included MR Egger, Weighted median, and Weighted mode. We merged the MR findings through meta-analysis. Heterogeneity testing, level pleiotropy testing (MR Egger intercept test and MRPRESSO), and sensitivity analysis via Leave One Out (LOO) were conducted to verify result robustness. Lastly, reverse MR analysis was performed.
Results: The meta-analysis merger revealed a correlation between CX3CL1 cycle levels and increased OA risk (OR=1.070, 95% CI: 1.040-1.110; P<0.010). We also observed associations between MCP4 (OR=0.930, 95% CI: 0.890-0.970; P<0.010) and CCL25 (OR=0.930, 95% CI: 0.890-0.970; P<0.010) with reduced OA risk. The sensitivity analysis results corroborate the robustness of these findings.
Conclusion: Our MR analysis indicates a potential causal relationship between CX3CL1, MCP4, CCL25, and OA risk changes. Further research is warranted to explore the influence of cytokines on OA development.
Osteoarthritis (OA) is a chronic degenerative condition marked by osteochondral degradation, manifesting as joint pain, stiffness, swelling, and reduced joint mobility. It predominantly impacts weight-bearing joints like the knee, hip, and spine (1, 2). Statistically, OA affects over 520 million individuals globally (3). with its prevalence escalating alongside population aging, significantly impairing patient quality of life (4). The etiology of OA remains elusive, with studies suggesting its association with factors like genetic variation, mechanical damage, immune inflammatory response, and metabolic irregularities (5, 6). Given the uncertain etiology, there is no definitive cure for OA. Currently, treatments involve general physical therapy to slow disease progression, non-steroidal anti-inflammatory drugs for symptom relief, and agents to promote joint cartilage repair. However, these long-term treatment strategies often yield suboptimal results. Post-joint replacement surgery, many patients continue to experience joint symptoms and are at risk of complications like cardiovascular events and infections (7). Thus, understanding OA’s pathogenesis and its early prevention are crucial. Presently, research primarily focuses on cartilage degeneration as a central aspect of OA’s pathogenesis, yet the underlying causes remain obscure. Clinical and basic research on cartilage remains pivotal in OA prevention and treatment (8, 9). However, the acquisition of research specimens and the associated costs are considerable challenges. Therefore, identifying biochemical biomarkers for OA prevention and treatment could represent a significant advancement.
Cytokines are small molecule proteins secreted and synthesized by immune cells like macrophages, monocytes, and T cells, as well as by non-immune cells such as fibroblasts and epidermal cells. They play a critical role in regulating immunity, stimulating cell activation and proliferation, and promoting hematopoiesis, thus being vital to human cellular function (10). Cytokines also contribute to diseases like inflammation, autoimmune disorders, and tumors (11, 12).
Increasing evidence indicates that the inflammatory response, mediated by inflammatory cytokines produced by synovial cells and chondrocytes in OA, is a key factor in OA pathogenesis. This response leads to cartilage matrix destruction and chondrogenesis inhibition (13). Immune cells activation, such as macrophages and lymphocytes, results in the production of tumor necrosis factor-α (TNF-α), interleukin-1β (IL-1β), and other inflammatory cytokines like interleukin-6 (IL-6). These cytokines exacerbate synovial inflammation, stimulate matrix metalloproteinases and oxidative stress, leading to chondrocyte apoptosis and cartilage degradation (14). Additionally, osteolytic cytokines like osteoclast activating factor (RANKL) play a role in OA development (15). However, the precise relationship between OA and the elevation of various cytokines remains unclear. Determining cytokine level alterations can further clarify their association with OA, providing a foundation for disease diagnosis and treatment.
Mendelian Randomization (MR) utilizes genome-wide association studies (GWASs), comprising single nucleotide polymorphisms (SNPs) from the population as genetic instrumental variables (IVs) to assess the causal links between exposure factors and outcomes (16, 17). These genetic variations, randomly assigned at conception, allow MR to circumvent confounding and reverse causal effects. Adhering to the genetic principle of “single gene single phenotype,” MR yields more reliable results and is extensively applied in analyzing relationships between various exposures and diseases (18). Current GWASs data includes IVs for over 100 cytokines. To date, only 2 MR studies have focused on OA, with a limited range of cytokines examined (18, 19). The incidence rate of OA varies across different body parts, and previous MR studies have not only examined a narrow scope of cytokines but also lacked detailed OA classifications. Thus, comprehensive research is necessary to elucidate the causal relationship between circulating cytokine levels and more specific OA categories, advancing our understanding of OA pathogenesis.
Therefore, this study conducts a bidirectional MR analysis based on publicly available GWAS data, employing the most comprehensive set of cytokine and OA data available. This approach aims to provide new insights into the causal relationship between cytokines and OA.
This research executed a broad and in-depth bidirectional MR analysis to explore a possible causal connection between cytokines and OA. The schematic of our MR analysis and design is depicted in Figure 1. MR studies must satisfy three criteria: first, the SNPs of the selected IVs must be significantly associated with cytokines. Second, these IVs should be independent and free from other confounding factors. Third, the IVs must influence outcomes exclusively through the exposure factors and not via alternate biological pathways. As this study utilizes publicly available GWAS data, no additional ethical approval was required.
Cytokine data were sourced from two GWAS datasets (20, 21). Currently, 41 cytokines are commonly utilized in research investigating the correlation between cytokines and diseases. However, the latest GWAS data related to inflammation-related cytokines provide up to 91 kinds of cytokines. This extensive dataset offers more opportunities for investigating the association between inflammatory factors and osteoarthritis. To acquire a broader range of cytokines, this study initially selected GWAS data of 91 inflammation-related cytokines from 11 cohorts comprising 14,824 European individuals and supplemented this with GWAS data from 13 out of 41 cytokines from 8,293 European individuals, as these cytokines did not overlap with the aforementioned 91 cytokines. Additional detailed information about the aggregated GWAS data can be found in the original paper. In the principal analysis, cytokines with independent SNPs were isolated using a P-value threshold of <5×10^-8. Due to the limited number of cytokines reaching genome-wide significance, the P-value threshold was subsequently adjusted to <5×10^-6. Linkage disequilibrium analysis (LDA) was carried out to assure SNP independence, with criteria set at r^2<0.001 and kb=10,000.
Data for OA were extracted from the most comprehensive GWAS database currently available, featuring 12 OA-related traits pooled from 9 international cohorts, totaling 826,690 participants (177,517 OA patients) across Asia, Europe, and European Americans (Supplementary Table 1) (22). Further information is detailed in the original publications. MR analysis was conducted on these OA-related traits, and the P-value threshold for OA-related SNPs was set at <5×10^-6, with concurrent LDA to ensure SNP independence (r^2<0.001 and kb=10,000).
To bolster the validity of the study outcomes and mitigate potential false positives, a supplementary dataset of 181,370 individuals (36,185 OA patients) was procured from the GWAS database, encompassing five OA traits for replication analyses (23). Similar to the primary study, a P-value threshold of <5×10^-6 was used to isolate cytokine SNPs, and LDA was performed to ascertain SNP independence, with criteria set at r^2<0.001 and kb=10,000.
Upon extracting SNP data for cytokines, we initially calculated the F-statistic for cytokines to assess the strength of the IVs (F>10 denotes sufficient strength) (24). All bidirectional MR analyses were conducted using the “TwoSampleMR” software package, with inverse variance weighting (IVW) as the primary, more precise, and unbiased method. Additionally, MR Egger, weighted median, and weighted mode analyses were employed to rule out potential confounders (25). For MR analysis outcomes, the false discovery rate (FDR) corrected p-values were computed using the Benjamin Hochberg method. A p-value below 0.05 post-FDR adjustment indicated a significant correlation. The findings from Discovery and replication MR analyses were then combined for a meta-analysis, integrating at least two reliably identified cytokine types. A merged P-value under 0.05 was deemed significant, with interpretations based on odds ratios (ORs).
To determine result robustness, we conducted heterogeneity testing, level pleiotropy testing, and Leave One Out (LOO) sensitivity analysis. Initially, MR-PRESSO was used to detect horizontal pleiotropy. If detected, outliers were removed for a subsequent MR reanalysis. If absent, the Cochran Q test was applied, with a Q value over 0.05 indicating no heterogeneity. LOO analysis was used to evaluate each SNP’s impact and identify any outliers. All MR analyses were performed using the “TwoSampleMR” and “MRPRESSO” software packages in R software (version 4.2.0) (26, 27).
Upon eliminating redundant cytokines, the analysis incorporated a total of 103 unique cytokines from diversified sources (Supplementary Table 2). Utilizing a significance criterion of P<5×10^-6, the study identified 1677 SNPs associated with the 103 cytokines. These SNPs exhibited an F-statistic range spanning from 18 to 1510, thereby confirming the statistical potency and reliability of the selected cytokines as instrumental variables (IVs) in the MR analysis (Supplementary Table 3). In an expansive MR analysis that focused on the 103 cytokines and 12 distinct OA traits, the Inverse Variance Weighted (IVW) method elucidated potential causal associations between 35 cytokines and the 12 specific OA characteristics (Supplementary Table 4; Figure 2). Post-FDR adjustment, negative correlations with varying OA traits were observed for higher levels of CCL19 [KneeHipOA, 0.953 (0.921-0.987), P=0.047], CD6 [TJR, 0.955 (0.925-0.985), P=0.039; TKR, 0.938 (0.893-0.984), P=0.048], CXCL9 [ThumbOA, 0.848 (0.760-0.946) were found.), P=0.047] DNER [ThumbOA, 0.877 (0.793 0.970), P=0.047], LTA [KneeHipOA, 0.927 (0.889 0.966), P=0.024; KneeOA, 0.918 (0.872 0.966), P=0.017], MIP1A [HandOA, 0.928 (0.876 0.983), P=0.040; ThumbOA, 0.896 (0.831 0.966), P=0.045], TNFB [AllOA.0.976 (0.959 0.993), P=0.047; HandOA, 0.921 (0.821 78-0.966), P=0.026; SpineOA, 0.948 (0.909-0.988), P=0.048], TRAIL [AllOA, 0.975 (0.957-0.994), P=0.046], and UPA [TKR, 0.908 (0.848 0.971), P=0.048]. Conversely, significant positive correlations with OA risk were found for elevated levels of FLT3LG [HandOA, 1.091 (1.029 1.156), P=0.043], IL10RB [THR, 1.060 (1.014 1.109), P=0.049], RANTES [ThumbOA, 1.164 (1.072 1.264), P=0.030], and TRANSCE [TJR, 1.059 (1.016 1.104), P=0.047; TKR, 1.084 (1.024 1.148), A significant correlation was observed between OA risk with diverse characteristics and a P-value of 0.044 (Figures 2, 3). The consistency of MR Egger, Weighted Median, and Weighted Mode methods with the IVW trend in MR analysis underscores the reliability of these results.
In the sensitivity analysis, no SNP pleiotropy was detected in the level pleiotropy and MR-PRESSO analysis (Supplementary Table 5), indicating the robustness of our instrumental variables. Additionally, the heterogeneity test revealed no heterogeneity in the MR results (Supplementary Table 6), further supporting the reliability of our findings. The LOO method also did not identify any significant bias points (Supplementary Figure 1).
In the MR analysis assessing potential causal relationships between 12 OA traits and cytokines, no such relationships were established post-FDR correction (Supplementary Table 7). This outcome underscores the complexity of the relationship between OA and cytokines. Overall, our results are reliable and suggest that multiple cytokines may be causally linked to OA.
As with discovery analysis, we extracted a GWAS data of 5 OA traits from the database, including 1649 sufficiently robust SPNs (F>10) related to 103 cytokines (Supplementary Tables 1, 8). MR analysis found that CD5 [SR, 0.909 (0.829 0.995), P=0.039], CCL25 [SR, 0.931 (0.881 0.984), P=0.012], IL0RA [HD_ken, 0.842 (0.725 0.979), P=0.025], GROa [HD_hipkeen, 0.931 (0.876 0.990), P=0.023], MCP1 [HD, 0.915 (0.893 0.999), P=0.047], MCP4 [HD, 0.924 (0.857 0.997), P=0.041] were associated with different features of OAA. There is a causal relationship, while CASP8 [SR, 1.137 (1.015-1.273), P=0.027], CATCK [HD_ken, 1.133 (1.016-1.263), P=0.025] CXCL9 [HD_hipkeen, 1.249 (1.046 1.492), P=0.014], GDNF [SR, 1.111 (1.027 1.202), P=0.009], TNFB [HD, 1.081 (1.021 1.144), P=0.007; HD_hipkeen, 1.103 (1.027 1.184), P=0.007; HD_ken, 1.117 (1.025 1.217), P=0.012], CX3CL1 [SR, 1.107 (1.003 1.224), P=0.044], and CXCL9 [HD_hipkeen, 1.213 (1.072 1.373), P=0.002; HD, 1.164 (1.054 1.285), P=0.003] indicated increased OA risk. However, post-correction, no significant causal relationships were established among all cytokines (Figure 4). Horizontal pleiotropy testing and MR-PRESSO did not show heterogeneity or pleiotropy (Supplementary Tables 9, 10), and the homogeneity test of MR results also indicated no heterogeneity (Supplementary Table 11). LOO analysis further confirmed the robustness of these findings (Supplementary Figure 2). Reverse MR analysis did not demonstrate causal relationships between different OA traits and the aforementioned cytokines (Supplementary Table 12).
Through meta-analysis, we ultimately found a positive causal relationship between CX3CL1 and OA (OR=1.070,95% CI: 1.040-1.110; P<0.010), while MCP4 (OR=0.930,95% CI: 0.890-0.970; P<0.010) and CCL25 (OR=0.930,95% CI: 0.890-0.970; P<0.010) demonstrated negative causal relationships with OA (Figure 5).
OA is a highly complex degenerative disease, with its pathogenesis being multifaceted and intricate. Increasing evidence underscores the significance of cytokines in the onset and progression of OA. However, the causal link and mechanism of action between cytokines and OA remain largely undefined. Our study utilized the most comprehensive set of cytokine type SNPs with identified sites and the largest OA GWAS database for discovery MR, supplemented by ample OA GWAS data for replication MR, to assess the causal impact of cytokines on OA. Collectively, the integration of MR analysis and meta-analysis highlighted a causal relationship between three distinct cytokines and OA.
Research indicates significant correlations between CCL19, CD6, CXCL9, DNER, LTA, MIP1A, TNFB, TRAIL, UPA, FLT3LG, IL10RB, RANTES, TRANSCE, and OA or bone metabolism (28–40). Our genetic MR analysis corroborated the causal association between these cytokines and OA. The meta-analysis integrated evidence suggesting a causal link between elevated levels of CCL25 and MCP4 and reduced OA risk. Conversely, an increase in CX3CL1 levels correlates with heightened OA risk.
CCL25 (TECK) is a key small molecular weight chemokine and a vital component of the inflammatory system. Typically, CCL25 interacts with its receptor CCR9, contributing to cell migration and metastasis, and it plays a role in recruiting inflammatory factors, thereby enhancing the inflammatory response. Moreover, CCL25 is involved in the chemotaxis of T lymphocytes, participating in a variety of physiological and pathological processes, including immune cell development, differentiation, and allergic diseases. It is recognized as a surface marker of homing lymphocytes (41). However, the relationship between CCL25 and OA has not been extensively reported. In research on porcine mesenchymal stem cells (MSCs) and their migration and chondrogenesis induced by CCL25, it was observed that CCL25 notably influenced MSC chemotaxis without causing significant cell death, even at higher concentrations. Studies suggest that certain concentrations of CCL25 may promote cartilage repair (42). In experiments involving intra-articular CCL25 injections in guinea pig knee OA, CCL25 not only effectively delayed OA progression and reduced cartilage damage (as indicated by lower Mankin scores) but also significantly increased MSC migration, suggesting that high CCL25 levels might be beneficial in preventing or treating OA (43). Additionally, a study on the chemotactic potential of CCL25 on human mesenchymal progenitor cells from subchondral cortical sponge bone in normal, rheumatoid arthritis (RA), and OA synovial fluid (SF) showed that CCL25 could enhance subchondral progenitor cell migration and induce cartilage tissue repair (44). Our genetic MR analysis investigated the causal relationship between CCL25 and OA, aligning with these findings. The results indicate that CCL25 may exert a protective effect against OA. However, reverse MR analysis did not establish a direct causal link between OA and CCL25.
MCP-4 is a chemokine known for its affinity to CCR3, and it has been implicated in autoimmune diseases like RA as well as allergic conditions (45, 46). It mediates the migration of eosinophils, basophils, macrophages, and lymphocytes in allergic diseases and possesses marked chemotactic activity for monocytes and T lymphocytes in inflammatory contexts (47). To date, no studies explicitly delineate the role of MCP-4 in OA. However, a study on MCP-4 expression in cartilage tissue, using OA and normal human articular cartilage as controls, revealed that MCP-4 expression was significantly elevated in OA cartilage compared to the normative cohort (48). Additionally, body mass index (BMI), known to be positively correlated with OA risk, has been linked to MCP-4. A Japanese study involving 39 overweight individuals showed that serum MCP-4 levels positively correlated with BMI, waist circumference, waist-to-hip ratio, and hypersensitive C-reactive protein (49). Another study focusing on severely obese patients confirmed that MCP-4 serum levels were elevated compared to those in normal-weight individuals and positively correlated with BMI (50). Our MR analysis indicates a causal relationship between elevated MCP-4 expression and reduced OA risk. However, the connection between MCP4 and OA pathogenesis remains unexplored. Our MR results suggest a causal link between high MCP4 expression and a reduced risk of OA. Yet, reverse MR did not identify a causal relationship between OA and MCP4. Given these conflicting findings, further research, particularly focusing on underlying mechanisms, is essential to clarify MCP4’s potential role in OA development.
CX3CL1, the sole member of the CX3C class of chemokines, exerts chemotactic effects on T cells and monocytes and is involved in various signaling pathways, such as p38MAPK and Akt. These pathways play a crucial role in mediating inflammatory diseases (51–53). Studies focusing on OA and related pain behaviors in mice have demonstrated that CX3CL1 is intricately linked to OA and varying pain intensities (54). In research on degenerative joint disease-related pain in cats, CX3CL1 levels were found to be upregulated in both the dorsal root ganglia affected by the disease and the spinal cord in cats displaying clinical signs of OA pain, compared to those without (55). A study examining the methylation genes in articular cartilage from OA patients identified CX3CL1 as a differentially elevated gene. Notably, its expression level increased exclusively in the OA group, distinguishing it from the control and RA groups (56), suggesting a potential genetic association with OA. Furthermore, an investigation into the expression levels of CX3CL1 in the serum and synovial fluid of 193 knee OA patients revealed not only elevated levels in OA patients but also a positive correlation with the degree of physical pain and disability (57). Our MR analysis, backed by a sufficient number of SNPs, indicates a positive causal relationship between CX3CL1 and OA. Additionally, the study design effectively excluded the influence of horizontal pleiotropy, reverse causal relationships, and confounding factors.
Previously, MR studies investigating the causal relationship between cytokines and OA identified links with MIP-1B, TNFB, and RANTS (58), aligning with our MR analysis results. Another study found a causal relationship between MCSF and VEGF and OA (19),yet these associations were not replicated in our MR and replication MR analyses. Our study, with its extensive OA sample size and the broadest range of cytokines to date in GWAS, also included discovery MR analysis, replication MR analysis, and meta-analysis, ensuring robust results. Notably, our analysis revealed a causal relationship between MCP4 and OA, a finding unprecedented in existing literature. This novel association warrants further investigation to validate MCP4 as a potential biomarker for OA prevention and treatment.
Despite these advancements, our research has limitations. First, the replication analysis for OA with 12 traits did not yield sufficient OA GWAS features. Additionally, akin to other studies (59–61), the paucity of SNPs led us to set a P-value threshold of <5×10^-6, potentially introducing false positives. However, the F-statistic for SNPs IV in our MR analysis exceeded 10, attesting to the robustness of our results. Finally, while we identified three cytokines potentially causally related to OA, their roles in affecting OA survival or disease progression merit further investigation.
Our MR analysis, with ample samples and a comprehensive range of cytokines, uncovered a potential causal relationship between CX3CL1, MCP4, and CCL25 and OA risk alterations. These findings, particularly regarding the novel cytokine markers, necessitate additional research to elucidate their mechanisms. The insights gained may significantly contribute to OA prevention and treatment strategies.
The original contributions presented in the study are included in the article/Supplementary Material. Further inquiries can be directed to the corresponding author/s.
ZoJ: Conceptualization, Funding acquisition, Methodology, Writing – original draft. XC: Data curation, Methodology, Software, Writing – review & editing. XiY: Formal analysis, Resources, Visualization, Writing – review & editing. SZ: Formal analysis, Supervision, Writing – review & editing. WL: Data curation, Validation, Visualization, Writing – review & editing. ZeJ: Formal analysis, Methodology, Software, Visualization, Writing – review & editing. FT: Project administration, Resources, Supervision, Writing – review & editing. WM: Funding acquisition, Resources, Supervision, Validation, Writing – review & editing. XuY: Funding acquisition, Supervision, Visualization, Writing – review & editing. CC: Investigation, Supervision, Writing – review & editing. TL: Investigation, Supervision, Writing – review & editing.
The author(s) declare financial support was received for the research, authorship, and/or publication of this article. This research was funded by Guizhou Provincial Clinical Research Center for Rheumatism and Immunology (Qian Kehe Platform Talent No. 2202); Guizhou Provincial Basic Research Program(Natural Science)(Fundamentals of Qian Kehe-ZK General 436); Guizhou Provincial Department of Education Youth Science and Technology Talent Growth Project (Qian Jiaohe-KY Word No. 262); Guizhou University of Traditional Chinese Medicine Graduate Education Innovation Program Project (YCXZRB202201); Guizhou Province College Student Innovation and Entrepreneurship Training Program Project (S202310662064).
The author expresses gratitude to every participant in the OA cohort study, as well as to every member involved in cytokine research.
The authors declare that the research was conducted in the absence of any commercial or financial relationships that could be construed as a potential conflict of interest.
All claims expressed in this article are solely those of the authors and do not necessarily represent those of their affiliated organizations, or those of the publisher, the editors and the reviewers. Any product that may be evaluated in this article, or claim that may be made by its manufacturer, is not guaranteed or endorsed by the publisher.
The Supplementary Material for this article can be found online at: https://www.frontiersin.org/articles/10.3389/fimmu.2023.1334361/full#supplementary-material
Supplementary Figure 1 | Discovery of Leave One Out.
Supplementary Figure 2 | Replication of Leave One Out.
1. Yin B, Ni J, Witherel CE, Yang M, Burdick JA, Wen C, et al. Harnessing tissue-derived extracellular vesicles for osteoarthritis theranostics. Theranostics (2022) 12(1):207–31. doi: 10.7150/thno.62708
2. Cho Y, Jeong S, Kim H, Kang D, Lee J, Kang SB, et al. Disease-modifying therapeutic strategies in osteoarthritis: current status and future directions. Exp Mol Med (2021) 53(11):1689–96. doi: 10.1038/s12276-021-00710-y
3. Arruda AL, Hartley A, Katsoula G, Smith GD, Morris AP, Zeggini E. Genetic underpinning of the comorbidity between type 2 diabetes and osteoarthritis. Am J Hum Genet (2023) 110(8):1304–18. doi: 10.1016/j.ajhg.2023.06.010
4. Beckmann NM, Villamaria EE. Interventional therapies for osteoarthritis: an update. Am J Roentgenol (2022) 219(6):929–39. doi: 10.2214/AJR.22.27548
5. Nedunchezhiyan U, Varughese I, Sun AR, Wu X, Crawford R, Prasadam I. Obesity, inflammation, and immune system in osteoarthritis. Front Immunol (2022) 13:907750. doi: 10.3389/fimmu.2022.907750
6. Watt FE. Posttraumatic osteoarthritis: what have we learned to advance osteoarthritis? Curr Opin Rheumatol (2021) 33(1):74–83. doi: 10.1097/BOR.0000000000000760
7. Geng R, Li J, Yu C, Zhang C, Chen F, Chen J, et al. Knee osteoarthritis: Current status and research progress in treatment (Review). Exp Ther Med (2023) 26(4):481. doi: 10.3892/etm.2023.12180
8. Herman K, Gobbi A. Evidence-based approach to orthobiologics for osteoarthritis and other joint disorders. Phys Med Reh Clin N (2023) 34(1):71–81. doi: 10.1016/j.pmr.2022.08.019
9. Shen P, Lohning M. Insights into osteoarthritis development from single-cell RNA sequencing of subchondral bone. Rmd Open (2022) 8(2):e002617. doi: 10.1136/rmdopen-2022-002617
10. Liu C, Chu D, Kalantar-Zadeh K, George J, Young HA, Liu G. Cytokines: from clinical significance to quantification. Adv Sci (2021) 8(15):e2004433. doi: 10.1002/advs.202004433
11. Propper DJ, Balkwill FR. Harnessing cytokines and chemokines for cancer therapy. Nat Rev Clin Oncol (2022) 19(4):237–53. doi: 10.1038/s41571-021-00588-9
12. Jang S, Kwon EJ, Lee JJ. Rheumatoid arthritis: pathogenic roles of diverse immune cells. Int J Mol Sci (2022) 23(2):905. doi: 10.3390/ijms23020905
13. Liu S, Deng Z, Chen K, Jian S, Zhou F, Yang Y, et al. Cartilage tissue engineering: From proinflammatory and anti−inflammatory cytokines to osteoarthritis treatments (Review). Mol Med Rep (2022) 25(3):99. doi: 10.3892/mmr.2022.12615
14. Chou CH, Jain V, Gibson J, Attarian DE, Haraden CA, Yohn CB, et al. Synovial cell cross-talk with cartilage plays a major role in the pathogenesis of osteoarthritis. Sci Rep-Uk (2020) 10(1):10868. doi: 10.1038/s41598-020-67730-y
15. Zhu S, Zhu J, Zhen G, Hu Y, An S, Li Y, et al. Subchondral bone osteoclasts induce sensory innervation and osteoarthritis pain. J Clin Invest (2019) 129(3):1076–93. doi: 10.1172/JCI121561
16. Davies NM, Holmes MV, Davey SG. Reading Mendelian randomisation studies: a guide, glossary, and checklist for clinicians. Bmj-Brit Med J (2018) 362:k601. doi: 10.1136/bmj.k601
17. Skrivankova VW, Richmond RC, Woolf B, Davies NM, Swanson SA, VanderWeele TJ, et al. Strengthening the reporting of observational studies in epidemiology using mendelian randomisation (STROBE-MR): explanation and elaboration. Bmj-Brit Med J (2021) 375:n2233. doi: 10.1136/bmj.n2233
18. Smith GD, Ebrahim S. ‘Mendelian randomization’: can genetic epidemiology contribute to understanding environmental determinants of disease? Int J Epidemiol (2003) 32(1):1–22. doi: 10.1093/ije/dyg070
19. Huang G, Li W, Kan H, Lu X, Liao W, Zhao X. Genetic influences of the effect of circulating inflammatory cytokines on osteoarthritis in humans. Osteoarthr Cartilage (2023) 31(8):1047–55. doi: 10.1016/j.joca.2022.12.007
20. Ahola-Olli AV, Wurtz P, Havulinna AS, Aalto K, Pitkanen N, Lehtimaki T, et al. Genome-wide association study identifies 27 loci influencing concentrations of circulating cytokines and growth factors. Am J Hum Genet (2017) 100(1):40–50. doi: 10.1016/j.ajhg.2016.11.007
21. Zhao JH, Stacey D, Eriksson N, Macdonald-Dunlop E, Hedman AK, Kalnapenkis A, et al. Genetics of circulating inflammatory proteins identifies drivers of immune-mediated disease risk and therapeutic targets. Nat Immunol (2023) 24(9):1540–51. doi: 10.1038/s41590-023-01588-w
22. Boer CG, Hatzikotoulas K, Southam L, Stefansdottir L, Zhang Y, Coutinho DAR, et al. Deciphering osteoarthritis genetics across 826,690 individuals from 9 populations. Cell (2021) 184(18):4784–818. doi: 10.1016/j.cell.2021.07.038
23. Zengini E, Hatzikotoulas K, Tachmazidou I, Steinberg J, Hartwig FP, Southam L, et al. Genome-wide analyses using UK Biobank data provide insights into the genetic architecture of osteoarthritis. Nat Genet (2018) 50(4):549–58. doi: 10.1038/s41588-018-0079-y
24. Bowden J, Del GMF, Minelli C, Davey SG, Sheehan NA, Thompson JR. Assessing the suitability of summary data for two-sample Mendelian randomization analyses using MR-Egger regression: the role of the I2 statistic. Int J Epidemiol (2016) 45(6):1961–74. doi: 10.1093/ije/dyw220
25. Burgess S, Bowden J, Fall T, Ingelsson E, Thompson SG. Sensitivity analyses for robust causal inference from Mendelian randomization analyses with multiple genetic variants. Epidemiology (2017) 28(1):30–42. doi: 10.1097/EDE.0000000000000559
26. Verbanck M, Chen CY, Neale B, Do R. Publisher Correction: Detection of widespread horizontal pleiotropy in causal relationships inferred from Mendelian randomization between complex traits and diseases. Nat Genet (2018) 50(8):1196. doi: 10.1038/s41588-018-0164-2
27. Yavorska OO, Burgess S. MendelianRandomization: an R package for performing Mendelian randomization analyses using summarized data. Int J Epidemiol (2017) 46(6):1734–9. doi: 10.1093/ije/dyx034
28. Mochizuki A, Takami M, Kawawa T, Suzumoto R, Sasaki T, Shiba A, et al. Identification and characterization of the precursors committed to osteoclasts induced by TNF-related activation-induced cytokine/receptor activator of NF-kappa B ligand. J Immunol (2006) 177(7):4360–8. doi: 10.4049/jimmunol.177.7.4360
29. Kisand K, Tamm AE, Lintrop M, Tamm AO. New insights into the natural course of knee osteoarthritis: early regulation of cytokines and growth factors, with emphasis on sex-dependent angiogenesis and tissue remodeling. A pilot study Osteoarthr Cartilage (2018) 26(8):1045–54. doi: 10.1016/j.joca.2018.05.009
30. Saxton RA, Tsutsumi N, Su LL, Abhiraman GC, Mohan K, Henneberg LT, et al. Structure-based decoupling of the pro- and anti-inflammatory functions of interleukin-10. Science (2021) 371(6535):eabc8433. doi: 10.1126/science.abc8433
31. Cedeno-Veloz BA, Lozano-Vicario L, Zambom-Ferraresi F, Fernandez-Irigoyen J, Santamaria E, Rodriguez-Garcia A, et al. Effect of immunology biomarkers associated with hip fracture and fracture risk in older adults. Immun Ageing (2023) 20(1):55. doi: 10.1186/s12979-023-00379-z
32. Perez-Garcia S, Carrion M, Gutierrez-Canas I, Villanueva-Romero R, Castro D, Martinez C, et al. Profile of matrix-remodeling proteinases in osteoarthritis: impact of fibronectin. Cells-Basel (2019) 9(1):40. doi: 10.3390/cells9010040
33. Park DR, Kim J, Kim GM, Lee H, Kim M, Hwang D, et al. Osteoclast-associated receptor blockade prevents articular cartilage destruction via chondrocyte apoptosis regulation. Nat Commun (2020) 11(1):4343. doi: 10.1038/s41467-020-18208-y
34. Zhao J, Duan L, Wang R, Liu Y, Jiang J. Roflumilast prevents lymphotoxin alpha (TNF-beta)-induced inflammation activation and degradation of type 2 collagen in chondrocytes. Inflammation Res (2020) 69(12):1191–9. doi: 10.1007/s00011-020-01404-3
35. Rubenhagen R, Schuttrumpf JP, Sturmer KM, Frosch KH. Interleukin-7 levels in synovial fluid increase with age and MMP-1 levels decrease with progression of osteoarthritis. Acta Orthop (2012) 83(1):59–64. doi: 10.3109/17453674.2011.645195
36. Tachmazidou I, Hatzikotoulas K, Southam L, Esparza-Gordillo J, Haberland V, Zheng J, et al. Identification of new therapeutic targets for osteoarthritis through genome-wide analyses of UK Biobank data. Nat Genet (2019) 51(2):230–6. doi: 10.1038/s41588-018-0327-1
37. Geyer M, Grassel S, Straub RH, Schett G, Dinser R, Grifka J, et al. Differential transcriptome analysis of intraarticular lesional vs intact cartilage reveals new candidate genes in osteoarthritis pathophysiology. Osteoarthr Cartilage (2009) 17(3):328–35. doi: 10.1016/j.joca.2008.07.010
38. Mahon OR, Kelly DJ, McCarthy GM, Dunne A. Osteoarthritis-associated basic calcium phosphate crystals alter immune cell metabolism and promote M1 macrophage polarization. Osteoarthr Cartilage (2020) 28(5):603–12. doi: 10.1016/j.joca.2019.10.010
39. Remacha AR, BarraChina L, Alvarez-Arguedas S, Ranera B, Romero A, Vazquez FJ, et al. Expression of genes involved in immune response and in vitro immunosuppressive effect of equine MSCs. Vet Immunol Immunop (2015) 165(3-4):107–18. doi: 10.1016/j.vetimm.2015.04.004
40. Nair A, Gan J, Bush-Joseph C, Verma N, Tetreault MW, Saha K, et al. Synovial chemokine expression and relationship with knee symptoms in patients with meniscal tears. Osteoarthr Cartilage (2015) 23(7):1158–64. doi: 10.1016/j.joca.2015.02.016
41. Wu X, Sun M, Yang Z, Lu C, Wang Q, Wang H, et al. The roles of CCR9/CCL25 in inflammation and inflammation-associated diseases. Front Cell Dev Biol (2021) 9:686548. doi: 10.3389/fcell.2021.686548
42. Luderitz L, Dehne T, Sittinger M, Ringe J. Dose-dependent effect of mesenchymal stromal cell recruiting chemokine CCL25 on porcine tissue-engineered healthy and osteoarthritic cartilage. Int J Mol Sci (2018) 20(1):52. doi: 10.3390/ijms20010052
43. Ringe J, Hemmati-Sadeghi S, Frohlich K, Engels A, Reiter K, Dehne T, et al. CCL25-supplemented hyaluronic acid attenuates cartilage degeneration in a Guinea pig model of knee osteoarthritis. J Orthop Res (2019) 37(8):1723–9. doi: 10.1002/jor.24312
44. Endres M, Andreas K, Kalwitz G, Freymann U, Neumann K, Ringe J, et al. Chemokine profile of synovial fluid from normal, osteoarthritis and rheumatoid arthritis patients: CCL25, CXCL10 and XCL1 recruit human subchondral mesenchymal progenitor cells. Osteoarthr Cartilage (2010) 18(11):1458–66. doi: 10.1016/j.joca.2010.08.003
45. Baumann R, Rabaszowski M, Stenin I, Tilgner L, Scheckenbach K, Wiltfang J, et al. Comparison of the nasal release of IL-4, IL-10, IL-17, CCL13/MCP-4, and CCL26/eotaxin-3 in allergic rhinitis during season and after allergen challenge. Am J Rhinol Allergy (2013) 27(4):266–72. doi: 10.2500/ajra.2013.27.3913
46. Iwamoto T, Okamoto H, Kobayashi S, Ikari K, Toyama Y, Tomatsu T, et al. A role of monocyte chemoattractant protein-4 (MCP-4)/CCL13 from chondrocytes in rheumatoid arthritis. FEBS J (2007) 274(18):4904–12. doi: 10.1111/j.1742-4658.2007.06013.x
47. Kalayci O, Birben E, Wu L, Oguma T, Storm VGK, Subramaniam V, et al. Monocyte chemoattractant protein-4 core promoter genetic variants: influence on YY-1 affinity and plasma levels. Am J Resp Cell Mol (2003) 29(6):750–6. doi: 10.1165/rcmb.2003-0024OC
48. Iwamoto T, Okamoto H, Iikuni N, Takeuchi M, Toyama Y, Tomatsu T, et al. Monocyte chemoattractant protein-4 (MCP-4)/CCL13 is highly expressed in cartilage from patients with rheumatoid arthritis. Rheumatology (2006) 45(4):421–4. doi: 10.1093/rheumatology/kei209
49. Hashimoto I, Wada J, Hida A, Baba M, Miyatake N, Eguchi J, et al. Elevated serum monocyte chemoattractant protein-4 and chronic inflammation in overweight subjects. Obesity (2006) 14(5):799–811. doi: 10.1038/oby.2006.93
50. Gentili A, Zaibi MS, Alomar SY, De Vuono S, Ricci MA, Alaeddin A, et al. Circulating levels of the adipokines monocyte chemotactic protein-4 (MCP-4), macrophage inflammatory protein-1beta (MIP-1beta), and eotaxin-3 in severe obesity and following bariatric surgery. Horm Metab Res (2016) 48(12):847–53. doi: 10.1055/s-0042-108731
51. Wu XM, Liu Y, Qian ZM, Luo QQ, Ke Y. CX3CL1/CX3CR1 Axis Plays a Key Role in Ischemia-Induced Oligodendrocyte Injury via p38MAPK Signaling Pathway. Mol Neurobiol (2016) 53(6):4010–8. doi: 10.1007/s12035-015-9339-3
52. Li D, Chen H, Luo XH, Sun Y, Xia W, Xiong YC. CX3CR1-mediated akt1 activation contributes to the paclitaxel-induced painful peripheral neuropathy in rats. Neurochem Res (2016) 41(6):1305–14. doi: 10.1007/s11064-016-1827-y
53. Hou SM, Hou CH, Liu JF. CX3CL1 promotes MMP-3 production via the CX3CR1, c-Raf, MEK, ERK, and NF-kappaB signaling pathway in osteoarthritis synovial fibroblasts. Arthritis Res Ther (2017) 19(1):282. doi: 10.1186/s13075-017-1487-6
54. Miller RE, Tran PB, Ishihara S, Syx D, Ren D, Miller RJ, et al. Microarray analyses of the dorsal root ganglia support a role for innate neuro-immune pathways in persistent pain in experimental osteoarthritis. Osteoarthr Cartilage (2020) 28(5):581–92. doi: 10.1016/j.joca.2020.01.008
55. Ashwell M, Freire M, O’Nan AT, Benito J, Hash J, McCulloch RS, et al. Characterization of gene expression in naturally occurring feline degenerative joint disease-associated pain. Vet J (2019) 243:42–7. doi: 10.1016/j.tvjl.2018.11.008
56. Zhao L, Wang Q, Zhang C, Huang C. Genome-wide DNA methylation analysis of articular chondrocytes identifies TRAF1, CTGF, and CX3CL1 genes as hypomethylated in osteoarthritis. Clin Rheumatol (2017) 36(10):2335–42. doi: 10.1007/s10067-017-3667-9
57. Huo LW, Ye YL, Wang GW, Ye YG. Fractalkine (CX3CL1): a biomarker reflecting symptomatic severity in patients with knee osteoarthritis. J Invest Med (2015) 63(4):626–31. doi: 10.1097/JIM.0000000000000158
58. Su D, Ai Y, Zhu G, Yang Y, Ma P. Genetically predicted circulating levels of cytokines and the risk of osteoarthritis: A mendelian randomization study. Front Genet (2023) 14:1131198. doi: 10.3389/fgene.2023.1131198
59. Liu B, Lyu L, Zhou W, Song J, Ye D, Mao Y, et al. Associations of the circulating levels of cytokines with risk of amyotrophic lateral sclerosis: a Mendelian randomization study. BMC Med (2023) 21(1):39. doi: 10.1186/s12916-023-02736-7
60. Yeung C, Schooling CM. Systemic inflammatory regulators and risk of Alzheimer’s disease: a bidirectional Mendelian-randomization study. Int J Epidemiol (2021) 50(3):829–40. doi: 10.1093/ije/dyaa241
Keywords: cytokines, osteoarthritis, Mendelian randomization, bidirectional, meta analysis
Citation: Jiang Z, Cai X, Yao X, Zhang S, Lan W, Jin Z, Tang F, Ma W, Yao X, Chen C and Lan T (2024) The causal effect of cytokine cycling levels on osteoarthritis: a bidirectional Mendelian randomized study. Front. Immunol. 14:1334361. doi: 10.3389/fimmu.2023.1334361
Received: 07 November 2023; Accepted: 27 December 2023;
Published: 11 January 2024.
Edited by:
Zhong Zheng, University of California, Los Angeles, United StatesReviewed by:
Xue Xu, Capital Medical University, ChinaCopyright © 2024 Jiang, Cai, Yao, Zhang, Lan, Jin, Tang, Ma, Yao, Chen and Lan. This is an open-access article distributed under the terms of the Creative Commons Attribution License (CC BY). The use, distribution or reproduction in other forums is permitted, provided the original author(s) and the copyright owner(s) are credited and that the original publication in this journal is cited, in accordance with accepted academic practice. No use, distribution or reproduction is permitted which does not comply with these terms.
*Correspondence: Fang Tang, NjQ1NTA5MzJAcXEuY29t; Wukai Ma, d2Fsa2VyNTVAMTYzLmNvbQ==
†These authors have contributed equally to this work
Disclaimer: All claims expressed in this article are solely those of the authors and do not necessarily represent those of their affiliated organizations, or those of the publisher, the editors and the reviewers. Any product that may be evaluated in this article or claim that may be made by its manufacturer is not guaranteed or endorsed by the publisher.
Research integrity at Frontiers
Learn more about the work of our research integrity team to safeguard the quality of each article we publish.