- Department of Botany and Microbiology, College of Science, King Saud University, Riyadh, Saudi Arabia
Escherichia fergusonii a gram-negative rod-shaped bacterium in the Enterobacteriaceae family, infect humans, causing serious illnesses such as urinary tract infection, cystitis, biliary tract infection, pneumonia, meningitis, hemolytic uremic syndrome, and death. Initially treatable with penicillin, antibiotic misuse led to evolving resistance, including resistance to colistin, a last-resort drug. With no licensed vaccine, the study aimed to design a multi-epitope vaccine against E. fergusonii. The study started with the retrieval of the complete proteome of all known strains and proceeded to filter the surface exposed virulent proteins. Seventeen virulent proteins (4 extracellular, 4 outer membranes, 9 periplasmic) with desirable physicochemical properties were identified from the complete proteome of known strains. Further, these proteins were processed for B-cell and T-cell epitope mapping. Obtained epitopes were evaluated for antigenicity, allergenicity, solubility, MHC-binding, and toxicity and the filtered epitopes were fused by specific linkers and an adjuvant into a vaccine construct. Structure of the vaccine candidate was predicted and refined resulting in 78.1% amino acids in allowed regions and VERIFY3D score of 81%. Vaccine construct was docked with TLR-4, MHC-I, and MHC-II, showing binding energies of -1040.8 kcal/mol, -871.4 kcal/mol, and -1154.6 kcal/mol and maximum interactions. Further, molecular dynamic simulation of the docked complexes was carried out resulting in a significant stable nature of the docked complexes (high B-factor and deformability values, lower Eigen and high variance values) in terms of intermolecular binding conformation and interactions. The vaccine was also reported to stimulate a variety of immunological pathways after administration. In short, the designed vaccine revealed promising predictions about its immune protective potential against E. fergusonii infections however experimental validation is needed to validate the results.
1 Introduction
Escherichia fergusonii, a relatively uncommon opportunistic pathogen in both humans and animals, is a rod-shaped, gram-negative bacterium that belongs to the genus Enterobacteriaceae (1). It is a peritrichous, non-spore-forming, and flagellated bacterium with a diameter between 0.8 and 1.5 mm and lengths between 2 and 5 mm (2). It was first isolated from samples of human blood in 1985 (3). The bacteria of the same genus demonstrated the strongest genetic resemblance to E. coli, with DNA hybridization revealing a 64% similarity (3). E. fergusonii is commonly found worldwide, and is suspected that this bacterium can enter the human body through food and water contaminated with infected feces (2). Furthermore, in a study, various strains of this bacterium were isolated from patients; including 2 strains from blood, 5 strains from urine, 1 strain from abdominal infection, 16 strains from feces, and 1 strain from other sites (4). Some strains of E. fergusonii have been associated with serious conditions in humans such as bloodstream infections, urinary tract infections, wound infections, and biliary tract infections (4).
In the context of antibiotic resistance (AR), E. fergusonii displayed resistance to a wide range of commonly used antibiotics. According to a recent study, ampicillin had proven to be particularly effective against the strains that caused these illnesses, while many showed resistance to gentamicin and chloramphenicol (5). Colistin is considered one of the most potent antibiotics for bacterial infections, and serves as the final line of defense in many cases (6). Even E. fergusonii had demonstrated resistance to colistin (7). A plasmid-borne resistance gene known as mcr-1 was identified as the primary cause of widespread colistin resistance in many strains of E. fergusonii (8). In 2019, in Zhejiang, China, a complete sequence plasmid of E. fergusonii containing both mcr-1 and ESBLs was discovered in chicken feces, suggesting that E. fergusonii may play a crucial role in transmitting mcr-1 (9).
The effectiveness of antibiotics is at risk due to the rapid emergence of AR by bacterial pathogens worldwide (10). Inappropriate antibiotic use and the pharmaceutical industry’s lack of interest in discovering new medications have both been linked to AR (11). Efforts are required to devise new strategies to curb the spread of AR pathogens and effectively manage diseases caused by AR bacteria. To address this issue and combat bacterial infections promptly, vaccine production is one of the most effective approaches. History has shown that vaccines have successfully protected humanity from many deadly infections, such as chickenpox, hepatitis A, arboviruses, and tetanus (12). However, there is currently a challenge in the swift production of vaccines. Traditional vaccine development takes approximately 5-8 years to become available to the public. To overcome this issue and accelerate vaccine production, bioinformatics is playing a crucial role where it proposes a method known as in-silico vaccine designing (13). In-silico vaccine designing is a uniquely innovative approach in which the proteomes of microorganisms are utilized to map epitopes that are complementary to human immune receptors. When introduced into the body, these epitopes can evoke an immune response, directly leading to immune stimulation against the corresponding microorganism (14). This is swift and authentic approach where vaccine candidates can be designed in less time, minimizing the rate of AR pathogens and havoc in the community (15). Keeping the swiftness and accuracy of this approach in consideration, it is crucial for scientific community to use this methodology and design vaccine candidates against deadly bugs. Considering the increasing AR rate of E. fergusonii, there is a gap to use bioinformatics pipelines and design a MEV construct against it that could result in decrease in morbidity rate. Following the mentioned approach, a multi-epitope vaccine (MEV) construct against E. fergusonii was designed in this study by using a variety of bioinformatics, reverse vaccinology, immunoinformatics, and biophysics techniques.
The study was initiated by retrieving the complete proteome of 56 known strains of E. fergusonii from the NCBI database. These proteomes were collectively subjected to bacterial pan-genome analysis (BPGA) to identify the core proteome among the 56 strains. Furthermore, the core proteomes were processed to determine their subcellular localization, focusing on proteins that are more exposed to immune receptors and other immune cells, such as extracellular, outer membrane, and periplasmic proteins. The selected proteins underwent various analyses, including homology checks, assessment of transmembrane helices, and VFDB analysis to identify the most suitable proteins for epitopes prediction. The filtered proteins were then used to predict B-cell and T-cell epitopes. These epitopes were analyzed using various bioinformatics tools, and the selected epitopes were used in MEV candidate designing. Furthermore, the structure of the MEV candidate was predicted, and its binding efficiency to major human immune receptors, such as MHC-I, MHC-II, and TLR4 was evaluated. The docked complexes were simulated to assess their intermolecular binding stability versus time. Finally, the vaccine was expressed through in-silico cloning using the SnapGene tool. This methodology, as demonstrated in many other studies, has consistently yielded promising results, with vaccine candidates showing high efficiency in experimental phases (16, 17).
2 Research methodology
A step-by-step methodology was applied in the study to achieve the objectives as given in Figure 1.
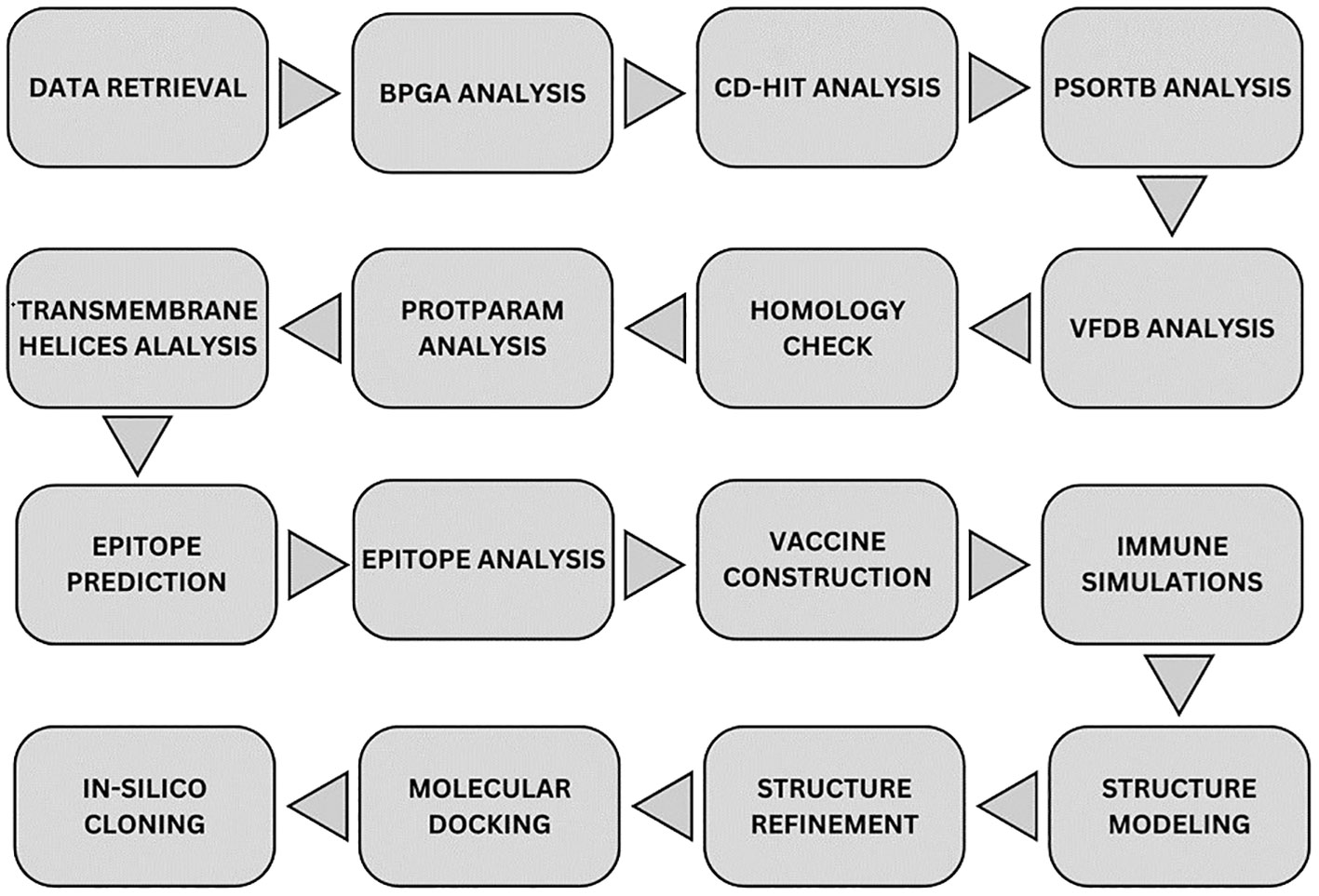
Figure 1 Overall methodology flow. Starting from top-left node, data of strains was downloaded from NCBI where it was processed to obtain core proteins via BPGA analysis. The non-redundant proteins were purified from the data via CD-HIT tool and the resulted data was processed for subcellular localization via PsortB tool. Obtained proteins were processed through four filtration tools in order to get the most virulent, non-homologous, most stable proteins. Epitopes were predicted and all the epitopes were analyzed through multiple filtration tools. MEV candidate was constructed and checked for immune simulation and the final structure was predicted for it. Structure was refined and was docked with the immune receptors in order to map its binding and simulations was carried out for each docked complex. Finally, the MEV candidate was re-expressed via in-silico cloning.
2.1 E. fergusonii proteome retrieval
The study began with the retrieval of proteomic data for all strains of E. fergusonii (https://www.ncbi.nlm.nih.gov/datasets/genome/?taxon=564). This bacterium comprises 56 known strains that have been completely sequenced and are available in the NCBI database (18). All the proteomic data was downloaded and processed for BPGA analysis.
2.2 BPGA analysis
BPGA is a Perl-based pipeline used to organize the proteomes of different strains of bacteria into categories of core proteins (common proteins among strains), unique proteins, and accessory proteins (19). Our main objective was to identify the proteins that are shared across all strains of E. fergusonii.
2.3 CD-hit analysis
Redundant sequences are duplicates in the proteome and arise during the evolutionary process. As such, these sequences are not necessary for computational vaccine design strategies and therefore should be removed (20). The core proteome of all strains of E. fergusonii was submitted to the CD-HIT server, with a sequence identity cut-off value of 50% (21). CD-HIT is a fast and widely used tool for comparing and clustering peptide sequences, designed to remove all sequences that exhibit similarity greater than the cut-off value.
2.4 Subcellular localization
The filtered proteins underwent subcellular localization analysis. The entire set of proteins obtained from the CD-HIT tool was processed using the PsortB tool (22). This analysis was conducted to filter out the essential outer membrane, extracellular, and periplasmic proteins, as these protein types are most exposed to the immune system and can be the most effective vaccine candidates to induce immune responses (23). Proteins located elsewhere (cytoplasmic) were excluded from the study.
2.5 Virulence factor database analysis
The obtained proteins were further subjected to virulence factor analysis via the VFDB server (24). Each protein was individually assessed to determine if it qualifies as a virulence factor. The threshold criteria for a protein to be categorized as a virulence factor is a sequence identity of >30% and bit scores greater than 100 (25). This analysis was conducted because the virulence factors of a microorganism are essential for the pathogenesis (23). Therefore, selecting sequences that match the criteria is crucial in designing an effective vaccine (26). Proteins meeting the threshold criteria were chosen for further analyses, while the others were excluded.
2.6 Homology check
The filtered protein sequences underwent further processing for homology check. Each protein sequence was aligned with the proteomes of Homo sapiens and major human flora bacteria, namely Lactobacillus johnsonni, Lactobacillus rhamnosus, and Lactobacillus casei, using NCBI BLASTp tool (27). Proteins showing similarity ≥ 30% and a bit score of ≥ 100 to the mentioned organisms were excluded from the study. The remaining proteins were subjected to further analyses. This step was conducted to ensure that the selected protein sequences do not trigger autoimmune responses (28).
2.7 ProtParam analysis
The physicochemical properties of the filtered proteins were assessed using the ProtParam tool (29). Analyzing the physicochemical properties of proteins is crucial, as it aids experimentalists in their in-vitro and in-vivo examination of the designed vaccine construct (30). This analysis provides information on the molecular weight, number of amino acids, instability index, and aliphatic index of the obtained proteins. Additionally, it provides insight into the hydrophilic and hydrophobic properties of the proteins. Proteins were selected for further analyses based on their instability index. Proteins with an instability index greater than 40 were excluded from the study, as unstable proteins are not suitable for vaccine design (31).
2.8 Transmembrane helices analysis
Next, transmembrane helix analysis was conducted for the filtered proteins. This prediction indicates the likely cellular locations and is achieved through an algorithm known as N-best (or 1-best in this case). The algorithm aggregates all paths across the model that have the same placement and helical orientation. Only proteins containing 0 or 1 transmembrane helix were selected, as they are more amenable to protein purification in experimental analysis. This analysis was carried out using the online tool TMHMM 2.0 (32).
2.9 B-cell and T-cell epitope prediction
The immune prediction database (IEDB) was used to predict the possible B-cell epitopes in the selected proteins of E. fergusonii (33). To assess the effectiveness of these epitopes in binding to MHC-I and MHC-II, the obtained epitopes were further analyzed for T-cell epitopes (34). The peptides were selected based on low percentile scores because the lower the percentile score, the stronger the binding (35).
2.10 Epitopes analysis
The obtained epitopes underwent a series of analyses to filter and use them as part of the vaccine model. Antigenicity and toxicity were assessed for each epitope using Vexigen 2.0 (36) and the ToxinPred tool (37). Epitopes with an antigenicity value greater than or equal to 0.4 and no toxicity were further analyzed for solubility. Solubility for each epitope was determined using the Innovagen Peptide Calculator tool, and epitopes with good water solubility were processed further (38). The filtered epitopes were evaluated using the Allertop 2.0 tool to check for allergic responses, and only those epitopes that showed no allergenicity were considered for further analysis (39). Finally, the filtered epitopes were analyzed with the MHC-Pred tool to assess their binding efficiency to MHC receptors (40).
2.11 Epitopes designing and processing for vaccine candidate
The filtered epitopes were assembled to form a vaccine candidate in such a way that first, an adjuvant was attached via an EAAAK linker to the first epitope, followed by GPGPG linkers, each connecting two consecutive epitopes (41). Cholera toxin-B was added as an adjuvant to ensure that the vaccine candidate could induce a robust immune response both in good magnitude and intensity (42) The linkers were added to maintain the structural integrity of the vaccine and prevent self-complementary binding of the sequences (41). The physicochemical properties of the final construct were also predicted to evaluate its stability, theoretical isoelectric point (PI), half-life, and water solubility.
2.12 Immune simulation
The vaccine construct underwent immune simulation studies to assess the response of host immune system to the vaccine candidate. To accomplish this, the c-IMMSIMM tool was utilized (43). The server employs a position-specific score matrix (PSSM) and various other machine-learning techniques to predict and investigate epitope and immunological interactions (43).
2.13 Structure modeling and refinement
The iTESSOR (Iterative Threading ASSEmbly Refinement modeling tool) was employed to predict the three-dimensional structure of the final vaccine construct (44). iTESSOR employs a hierarchical method combining template-based fragment assembly, structural refinement, and threading (45). To predict a structure, it breaks the query sequence into fragments and utilizes all the structured sequences present in the Protein Data Bank (PDB) as templates against it (46). Upon prediction, it further refines the structure using specific algorithms. Because of this combined approach, iTESSOR is now at the forefront of protein structure prediction, allowing for precise structure prediction for a wide range of protein folds and sequences (47). The obtained structure was further refined using the Galaxy Refine tool to enhance stability (48). The stability of the vaccine structure was examined using Verify3D and PDBsum tools (49, 50).
2.14 Molecular docking and simulations
To assess the vaccine candidate’s ability to bind to the immune cell receptors, molecular docking was performed. The vaccine construct was docked with MHC-I (PDB ID: 1I1Y), MHC-II (PDB ID: 1KG0), and TLR4 (PDB ID: 4G8A) receptors using the ClusPro server (51). The complexes were then visualized using the UCSF Chimera software (52), and the types and number of interactions between the docked complexes were analyzed using the PDBsum tool (50). Understanding the atomic-level molecular behavior and characteristics of the docked complexes is possible to predict through molecular dynamics simulation (MDS). MDS provides data that complement experimental data regarding the dynamics, including structure, interaction energies, and atomic mobility (53). An online tool called iMOD was used to perform MDS, where dynamic parameters such as deformability factor, B-factor, Eigen-values, and variance of the docked complexes were calculated (54).
2.15 In-silico cloning
Finally, to confirm the cloning effectiveness of the designed vaccine candidate, it was re-expressed via in-silico cloning using SnapGene tool. Using E. coli as the expression system, the MEV’s codons were optimized according to the codon usage of the expression system. For this purpose, the Jcat tool was employed (55). The optimized and improved sequence was cloned into a specific vector known as pet28+(a) (56). This vector is mostly used in cloning approach because it consists of T7 promoter, His-tag fusion (polyhistadine which facilitates easy purification of the expressed protein), multiple-cloning-site and a selectable marker (57). The resulting data from this step will provide precise data for experimentalists to enable industrial-level production.
3 Results
3.1 Complete proteome retrieval of E. fergusonii
In this research work, a total 56 of completely sequenced proteomes of E. fergusonii were obtained from NCBI.
3.2 BPGA and CH-Hit analyses
As mentioned, BPGA analysis filtered out the core proteome of all 56 strains of E. fergusonii. A total of 18,621 core proteins were filtered, which were further processed for CD-Hit analysis, resulting in the identification of 2,911 non-redundant proteins, while the remaining redundant proteins were excluded (Figure 2A). Redundant sequences are duplicates that arise due to duplication events during the evolutionary process. Therefore, for the computational vaccine design process, these sequences are not required. All the non-redundant proteins were subjected to subcellular localization and virulence analysis.
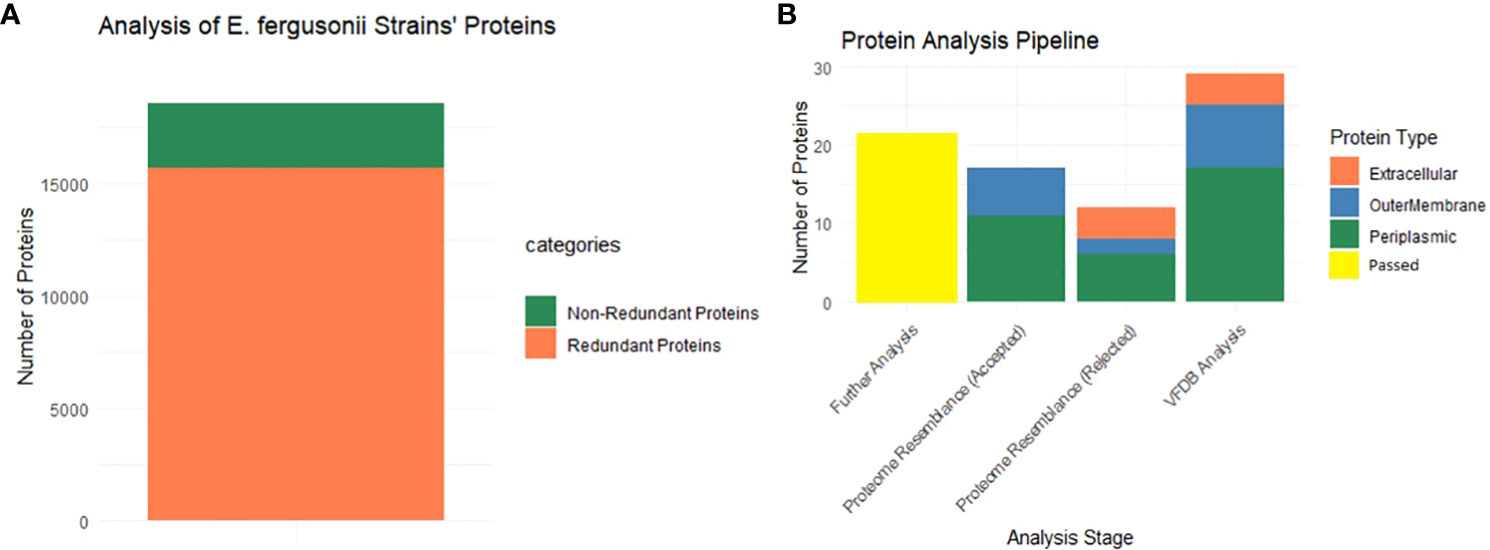
Figure 2 Graphical presentation. (A) This part shows that among the entire core proteins (entire multi-colored bar) 2911 proteins are non-redundant (dark green), and 15710 are redundant proteins. (B) Starting from the extreme right bar, the multi-colored bar shows the localized proteins that passed VFDB analysis (virulence proteins). Second bar shows the localized proteins that were passed in the homology check analysis (rejected word means that no similarity was shown with the proteome of the mentioned organisms) while the next plot is completely opposite of it. The last bar (yellow) shows the filtered proteins that are allowed for further analysis.
3.3 Subcellular localization, virulence and homology check
All 2,911 proteins were subjected to subcellular localization analysis resulting in the identification of 4 extracellular proteins, 8 outer membrane proteins, and 17 periplasmic proteins, while the remaining proteins were found to be cytoplasmic and were filtered for further analyses. The entire set of 29 proteins was then subjected to VFDB analysis, and all the proteins were included in the virulence factors list. Additionally, these proteins were aligned with the proteome of H. sapiens and major human flora bacteria, namely L. johnsonni, L. rhamnosus, and L. casei. None of the extracellular proteins showed any resemblance to the proteome of the mentioned organisms, while 2 outer membrane proteins and 6 periplasmic proteins displayed some resemblance to the proteome of the mentioned organisms and were thus rejected for further analyses. The graphical representation of these results is shown in Figure 2B. The remaining 21 proteins were processed further for the assessment of physiochemical properties
3.4 Predicting physicochemical properties and transmembrane helices
The physicochemical properties of the filtered proteins were checked using the ProtParam tool. The proteins with an instability index greater than 40 were classified as unstable and were removed from the list. Four proteins (two outer membrane proteins and two periplasmic proteins) were excluded, while the remaining proteins were stable. The remaining 17 proteins were processed for transmembrane helices analysis, and all of the proteins were within the threshold value (0-1). The final set of proteins is shown in Supplementary Table 1, and their properties are shown in Table 1. Furthermore, identity and functional enrichment analyses were carried out for all of the filtered proteins to determine their exact functions. The functional enrichment analysis of each protein revealed its molecular functions, roles in biological processes, and cellular components. All of the related information is shown in Table 2.
3.5 Immune epitopes prediction
The selected proteins were prioritized for immune epitope prediction sequentially. This was achieved by B-cell epitope prediction followed by T-cell epitope prediction. B-cell epitopes were processed further to identify T-cell epitopes. Helper T lymphocytes stimulate B cells, macrophages, and cytotoxic T lymphocytes. Cytotoxic T lymphocytes, on the other hand, can directly recognize antigens (58). Conversely, B cells can develop into plasma cells, which produce antibodies (59). The lowest percentile score was used to select MHC-I and MHC-II epitopes in T cells, because the lower the score, the greater the binding potential. B-cell epitopes were predicted for each protein, and are shown in Figure 3. The number of amino acids and their sequence location in the proteins are listed in Table 3. The epitopes were processed further for T-cell epitope prediction (MHC-II and MHC-I). Numerous epitopes were predicted and processed for different filtration analyses to obtain the most appropriate epitopes for the final vaccine construct design.
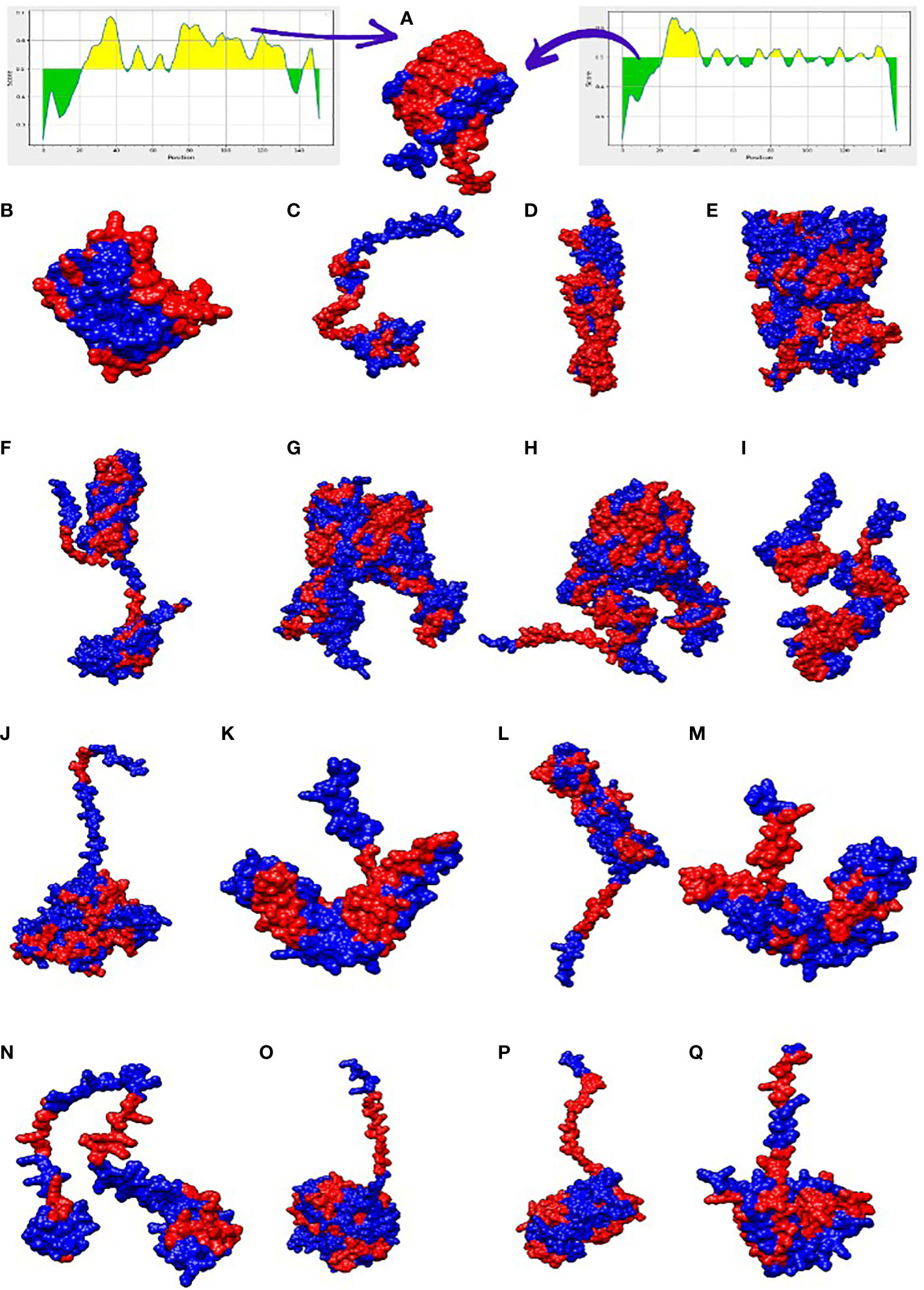
Figure 3 3D representation of the 17 filtered proteins. The graphs shown at top shows range of epitopes (residues) selected in the proteins (yellow region shows residues involved in B-cell epitopes while the green shows the unselected residues). The red regions in the structure represent B-cell epitopes while the blue portion represent the uninvolved residues. Three dimensional structures represent proteins in alphabetical order along with its NCBI accession IDs i-e WP_000765273.1 (A), WP_024256466.1 (B), WP_104920238.1 (C), WP_182211891.1 (D), WP_223665991.1 (E), WP_024256413.1 (F), WP_148048017.1 (G), WP_181588385.1 (H), WP_002431495.1 (I), WP_000753932.1 (J), WP_182210933.1 (K), WP_240828776.1 (L), WP_000465914.1 (M), WP_223666952.1 (N), WP_105283498.1 (O), WP_125400643.1 (P), WP_181198759.1 (Q).
3.6 Epitopes prioritization phase
The obtained epitopes were finally processed for multiple analyses to obtain the most acceptable epitopes for our vaccine construct. Antigenicity, allergenicity, solubility, MHCpred, and toxicity analyses were performed, and the filtered epitopes are shown in Table 4. The non-antigenic epitopes were eliminated from the selected epitopes, as the vaccine should contain epitopes that have the potential to induce antibody production. Epitopes that are less soluble in water, exhibit a toxic character and produce allergic reactions, were further eliminated.
3.7 Vaccine candidate design
Following the completion of the above investigation, 37 distinct epitopes were selected from the list of combined epitopes before filtration. One of the key issues was solved by combining various types of specified epitopes using specific GPGPG linkers to form a multi-epitope-based vaccine construct. Additionally, the cholera toxin B subunit adjuvant and the epitopes peptide were linked using the EAAAK linker. GPGPG linkers were inserted between epitopes because they can prevent junctional folding and effectively trigger an immune response involving T-helper cells (60). EAAAK is a stiff, stable α-helical peptide linker with an intramolecular hydrogen bond and a closed-packed backbone. Thus, in a fusion protein, the EAAAK linker serves as a domain spacer (61). Furthermore, linkers help epitopes to unite and produce a considerable structure having polytope conformation (62). Because cholera toxin B significantly increases the synthesis of mucosal IgA and other immunological responses, it was used as an adjuvant (63). This is because it is non-toxic and can bind to the monosialotetrahexosylganglioside (GM1) receptor, which is present on cell membranes and in the cytosols of a range of cells, including B cells, macrophages, dendritic cells, gut epithelial cells, and antigen-presenting cells (64). Similarly, the adjuvant used is safe and generates potent immune responses that are specific to the antigen it is associated with (65). The MEV construct and its important properties are shown in Figure 4A and Table 5, respectively.
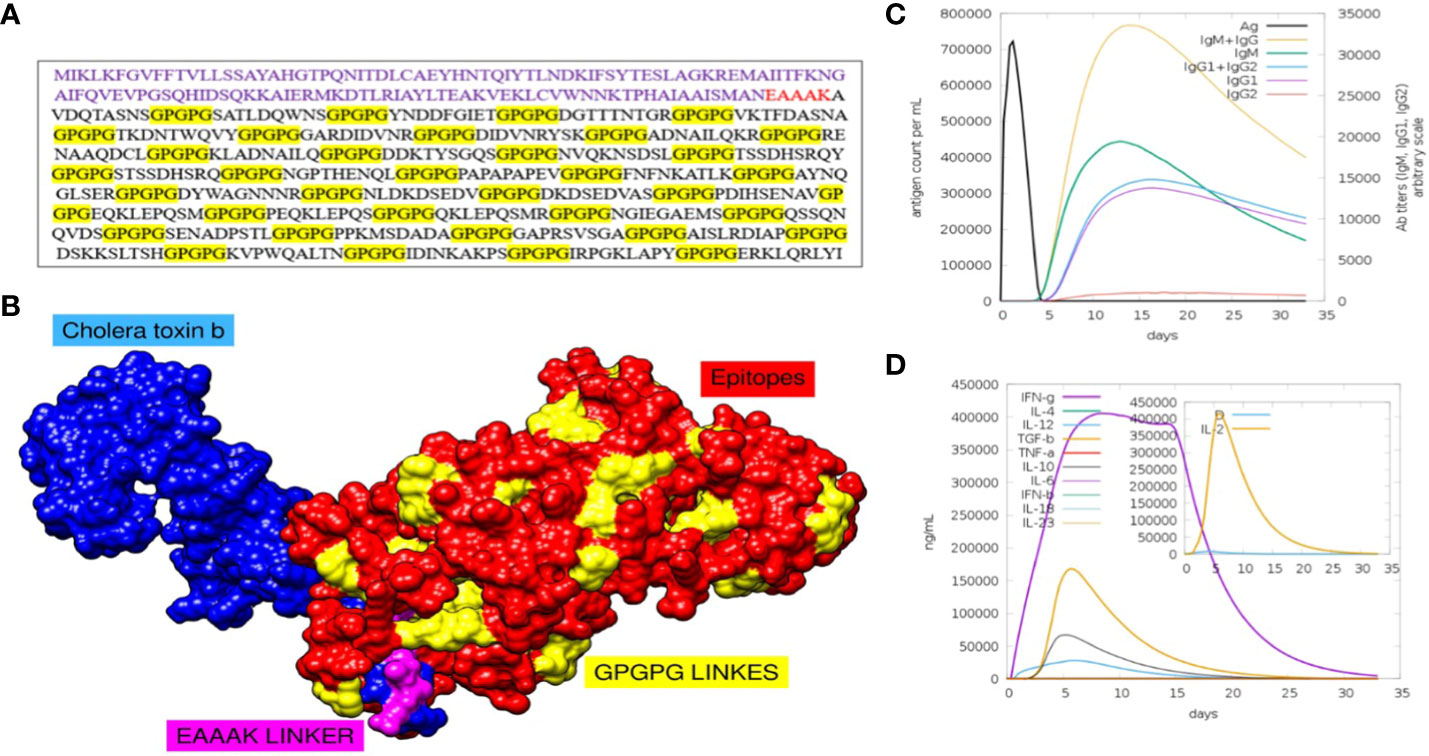
Figure 4 (A) Sequence of final MEV construct. (B) Predicted structure of MEV construct along with its adjuvants and different linkers. (C) Number of different antibodies produced against the vaccine in specific number of days are plotted. (D) Number and types of cytokines with respect to number of days is plotted.
3.8 Immune simulation analysis and structure modelling
To assess whether the epitopes would be capable of generating sufficient immunity, the immunological responses to the multi-epitope vaccination were evaluated using the C-ImmSim server (66). This method can also be used to determine the development of immunological interactions between the epitopes and their specific targets. The ability of the MEV construct to elicit potent immune responses was evident via this approach. After 35 days of exposure of the human immune system to the highest dose of vaccine antigen, a C-immune simulation analysis revealed an increase in the generation of adaptive responses in the form of IgG and IgM-antibodies (Figure 4C). Similarly, it was noted that interferon-gamma and IL-2 production was greater than 400000 counts per ml for nearly 35 days (Figure 4D). At last, the MEV construct was modelled in Figure 4A. For this, the amino acid of the final MEV construct was uploaded to iTESSOR tool in FASTA format, and the structure was predicted using an ab initio modelling strategy (67). A total of five structures were predicted and the structure having the highest confidence score was selected.
3.9 Structure refinement and stability analysis
The predicted model was further refined using the GALAXY refinement tool to minimize, relax, and stabilize the structure by removing high-energy interactions (68, 69). The stability of the predicted model was examined using the PDBsum and Verify3D tools (49, 50). These tools predicted the stability using plots and graphs. The plot generated by the PDBsum tool is known as the Ramachandran plot, where the amino acids of the MEV construct are divided and placed in different regions of the plot, each of which represents a specific level of stability (70). This plot consists of four regions: most favoured regions (red), additional allowed regions (brown), generously allowed regions (yellow), and disallowed regions (pale). The residues of the MEV construct were placed in these regions and combined stability was calculated. The placement of residues in these regions is based on the Phi and Psi angles of each residue, which are plotted on X-axis and Y-axis of the plot. Upon analysis, most of the residues of the MEV construct were placed in the allowed region (78.1%), followed by the additional allowed regions (16.7%), the generously allowed region (1.7%), and the disallowed region (3.6%) Table 6. The occurrence of more residues in the allowed regions and fewer in the disallowed region indicated that our MEV construct is stable (Figure 5A). Furthermore, plots based on dihedral angles for each residue were shown separately, clearly indicating the occurrence of each residue across the plot. It shows that most of the residue’s dihedral angles are within the supported range, while only a few residues (indicated by yellow and red spots) have controversial angles, collectively clarifying the stability of the MEV construct (Figure 5B). Secondly, Verify3D also clarifies the stability of the MEV construct, showing that the majority of residues (81.66%) lie close to the average score line (Figure 5C).
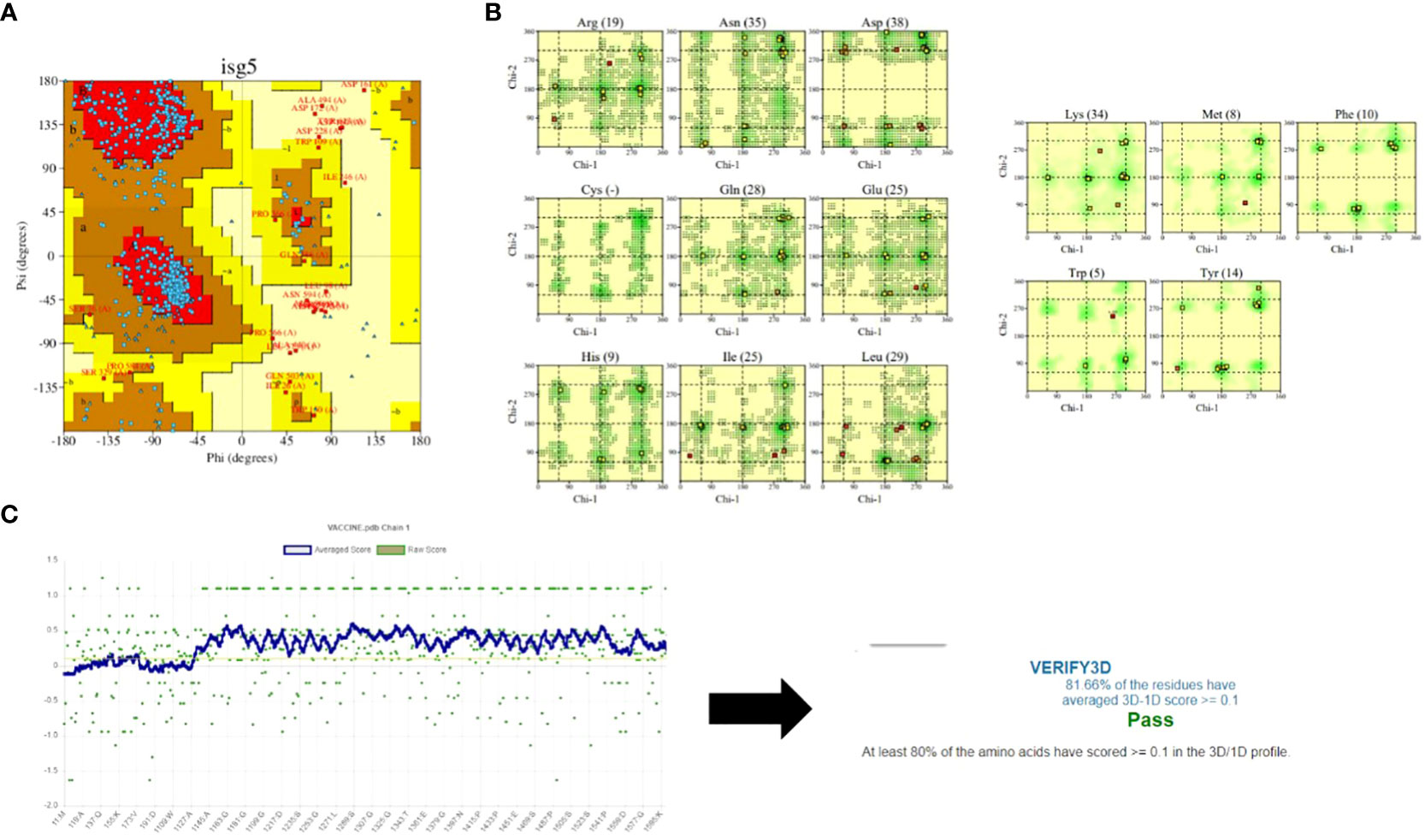
Figure 5 Stability analysis: (A) Ramachandran plot shows maximum of residues (blue dots) in the allowed regions while very few residues (red dots) in the disallowed regions. (B) shows the dihedral angles of each residues in MEV construct. (C) shows that maximum of residues of MEV construct lies among the averaged line that clarify that the structure is almost stable.
3.10 Protein-protein molecular docking
Docking is an important strategy for evaluating the binding efficiency of two molecules. This approach was used to check the binding affinity of the MEV construct with the immune receptors i-e MHC-I, MHC-II, and TLR-4. Three-dimensional structures of immune receptors were retrieved from the Protein Data Bank, and each was separately docked with the MEV construct (51). Binding energies were then calculated (51). Furthermore, the types and number of interactions were also checked (50). The binding of the vaccine with TLR4, MHC-I, and MHC-II was efficient Table 7 and the number of interactions was also maximum Figure 6. Further, hydrogen bonds were also present between the complexes, which further illustrate maximum bindings. The structure of docked complexes and the number and types of interactions between the complexes are shown in Figure 6.
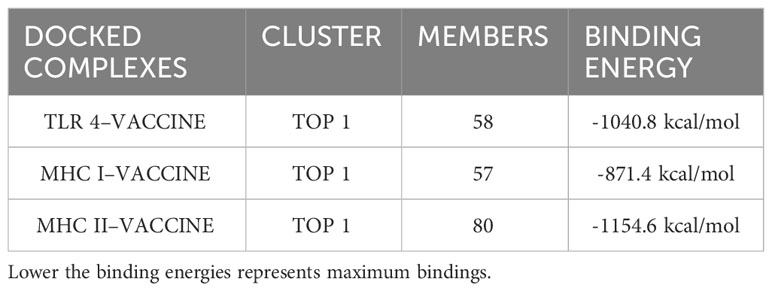
Table 7 Top 1 clusters of the docked complexes along with their number of members (residues) involved in the interaction and its binding energies are shown.
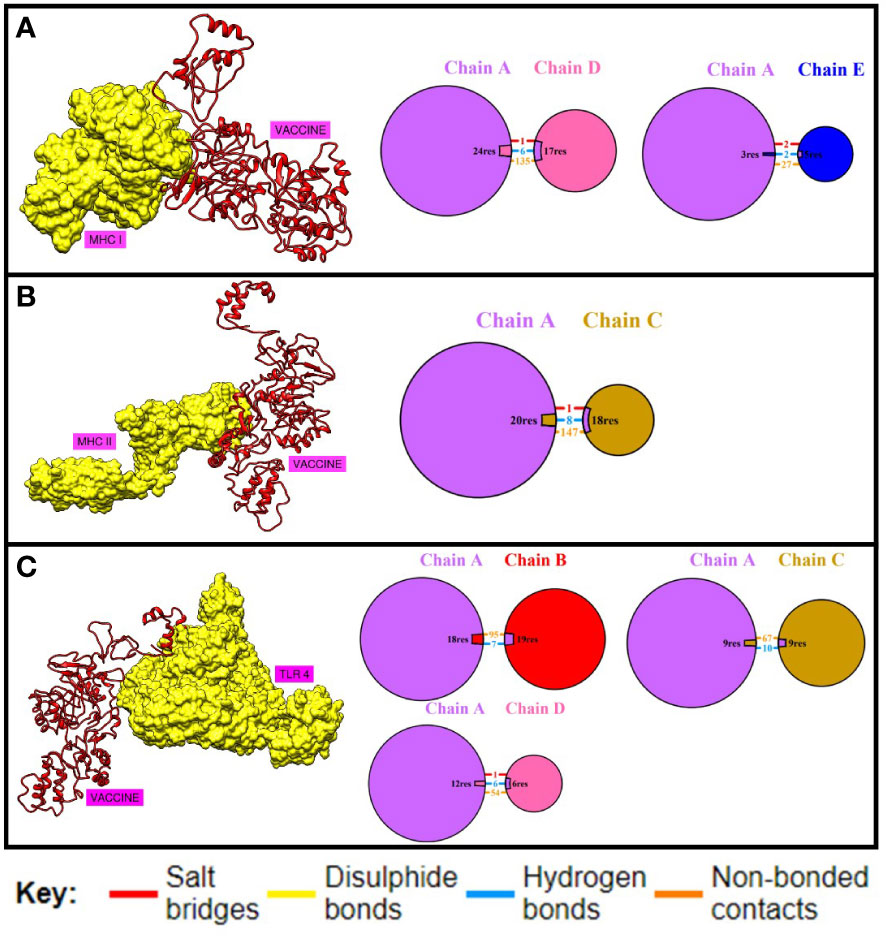
Figure 6 Binding analysis. (A) shows the number and type of interactions between the chains of MHC-I and MEV construct. (B) this shows the number and type of interactions between MHC-II and MEV construct while (C) shows the binding and interactions between TLR04 and MEV construct respectively.
3.11 Molecular dynamic simulations of the docked complexes
Online simulation studies were performed for all the docked complexes separately using the iMOD tool to check the behavior of the docked complexes (54). Simulation results shown in Figure 7 reveal that all the docked complexes showed considerable deformability values, which illustrates that most of the residues of the complex are deforming and showing movement from their actual position. This indicates that greater deformability results in active binding. Second, the eigenvalues of all the complexes show the lowest values, which indicates that less energy is required to deform the structures, which correlates with effective binding. Variance is the opposite of eigenvalues, with high values indicating low difficulty in deforming the structure. The B-factor of all the complexes represents high values, which also correlates with the deformability of complexes. Red, white, and blue colours are used in the covariance matrix to indicate the graph’s correlated, uncorrelated, and anti-correlated motions, respectively. In short, the molecular dynamics investigation clearly showed that our complexes had a fair deal of deformability and displayed an acceptable eigenvalue, indicating effective and uniform binding to its immune receptors.
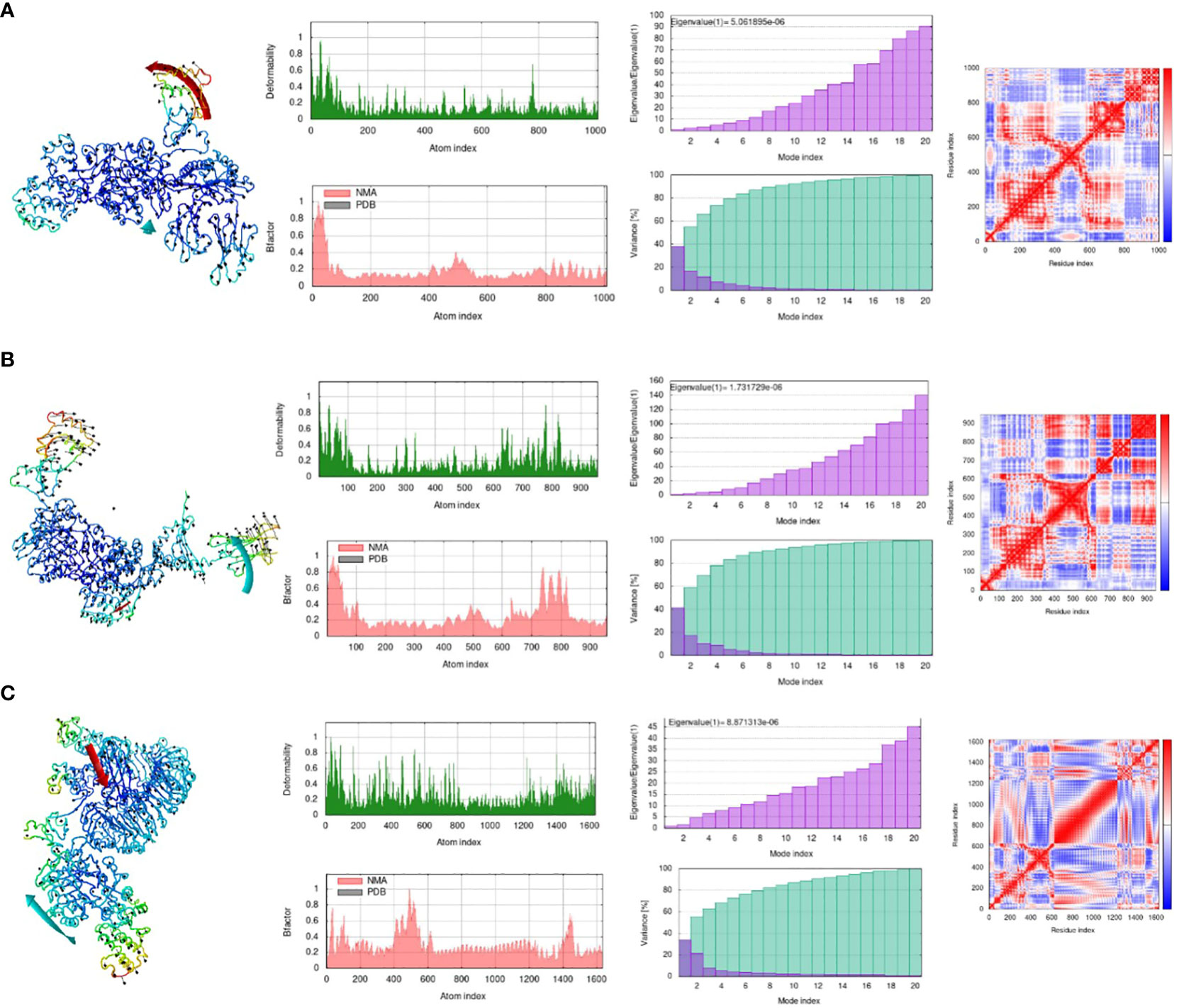
Figure 7 Molecular Dynamic Simulation. (A) MHC-I-MEV complex, (B) MHC-II-MEV complex, (C) TLR4-MEV complex. Deformability plot, eigenvalue, B-factor, variance plot, covariance matrix analysis and elastic network model. (Analysis in sequential manner).
3.12 In-silico cloning and codon optimization
The predicted vaccine was finally subjected to in-silico cloning because it assured us that it was the finest vaccine candidate to evoke immune responses and prevent E. fergusonii infection in individuals. For cloning, the MEV construct was optimized using the codon adaptation tool (55) according to the expression system E. coli K-12 (71). Codon optimization was performed to match the expression system used because the efficiency of codons varies among different organisms due to specific codon usage patterns. The MEV construct was successfully cloned into a special vector named pet28a(+) with modified restriction sites using the SnapGene tool, and the results are shown in Figure 8 (72).
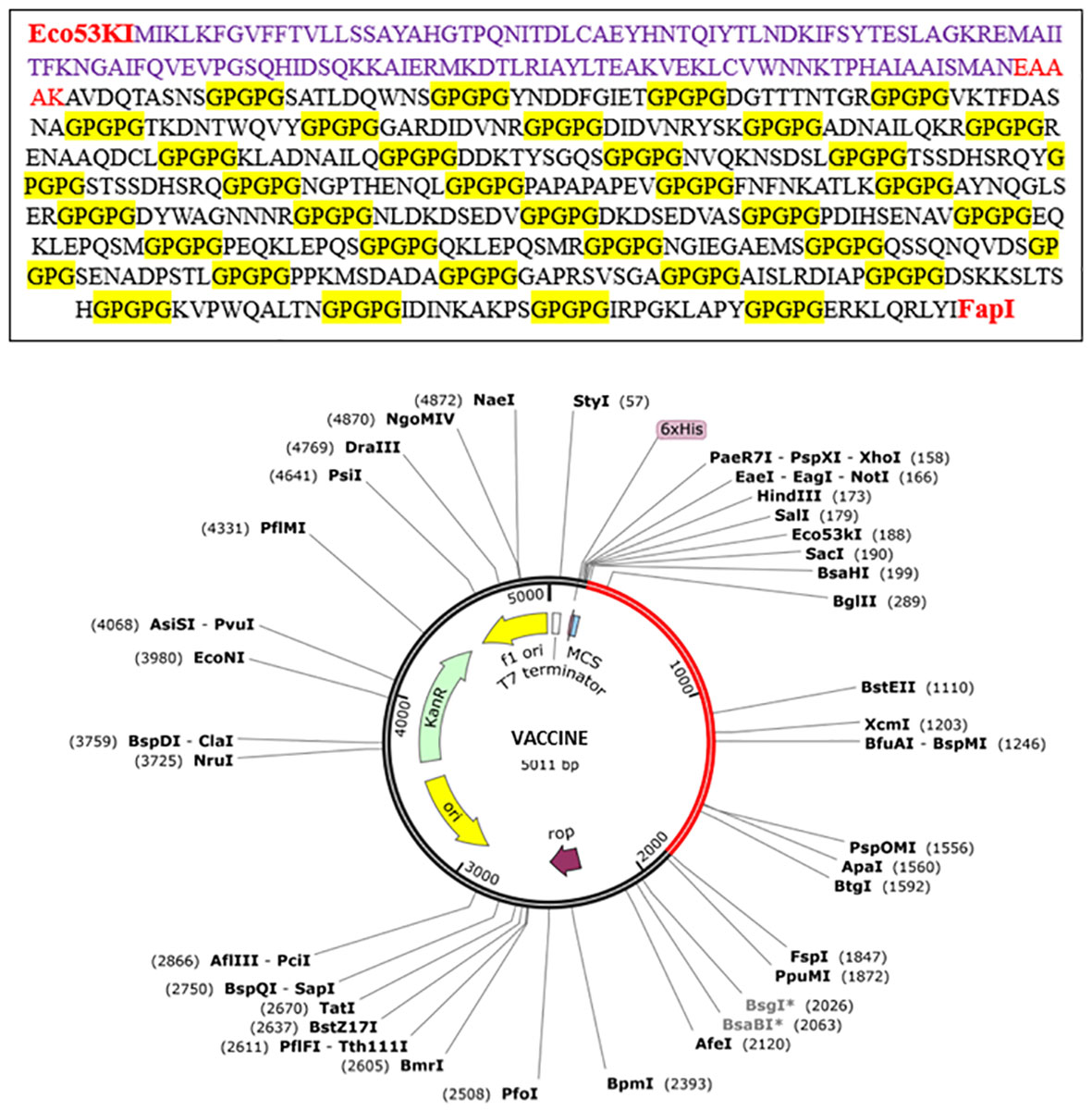
Figure 8 In-silico cloning of the vaccine construct. Red part shows the vaccine construct cloned into the vector pet28a(+) with restriction sites Eco53kI and FspI used. Translated sequence of the red area is also shown.
4 Discussion
AR among pathogenic microorganisms is increasing at a rapid pace (10). As a result, most of the known antibiotics available are becoming less effective, leading to an increase in morbidity and mortality rates (73). This situation is primarily a consequence of antibiotic misuse by the population (74). E. fergusonii is a minor pathogenic bacterium, that is responsible for causing UTIs, biliary infections, and hemolytic uremic syndrome (HUS). It was once easily treated with common antibiotics, but over time, this bacterium has developed AR properties. Recently, it had shown resistance to one of the most potent antibiotics, colistin, leading to a public health crisis (8). The main objective of this research was to design an MEV based on the core proteome of all strains of E. fergusonii. The goal is to confer immunity against this bacterium, preventing its pathogenesis within the host’s body (75). MEV constructs have been designed by many researchers against many pathogens considering their entire proteomes. For example, a recent study used superantigens of Staphylococcus aureus to construct a MEV construct (76). Another study focused on designing an MEV against Helicobacter pylori (77). Furthermore, MEV constructs have been designed against the spike proteins of coronaviruses, yielding promising results (78)
The field of vaccine design is seeing significant advancements due to reverse vaccinology, and the availability of proteomics data and development in artificial and machine learning techniques (79). Additionally, the successful application of bioinformatics technologies is advantageous in comparison to conventional vaccine design (80). The designing of the MEV construct has also shown promising experimental outcomes that include a study by (17) where the MEV construct against influenza-A showed robust production of neutralizing antibodies in mice models. Further, (16) proposed a study in which an MEV construct designed against Leishmania infantum proteins showed abundant production of interferons and interleukins in BALB mice when administered.
Following the methodology of the study, core proteomes from the known strains of completely sequenced E. fergusonii were obtained, and CD-Hit analysis resulted in the generation of non-redundant sequences from the core proteomes. Vaccines can only be effective if they contain portions of the bacteria that are more readily exposed to the body’s immune cells and are highly virulent (contributing to pathogenesis), resulting in rapid immune responses (81, 82). Following this concept, exposed proteins were filtered out from the non-redundant proteins and were further prioritized for a virulence check. Same concepts were used by different researchers like, (83) designed a MEV against Mycobacterium ulcerans strain Agy99 by considering its chromosome-encoded virulence proteins and (84) produced an epitope-based vaccine against Mycobacterium spp. by utilizing its extracellular 85B protein, respectively. (85) designed an effective MEV candidate based on major virulence factors of Clostridium perfringens, i.e., alpha-toxin, NetB, and metallopeptidase protein (NAM). Thereafter, the highly virulent and exposed proteins’ sequences were processed through homology checks by aligning the sequences with the proteomes of Homo sapiens and important human normal flora bacteria. The aligned sequences were removed from the study, and the remaining sequences were allowed to be processed further. (86) also used the same homology check protocol for Chlamydia psittaci proteins when designing MEV.
Physiochemical properties analysis of proteins is one of the important steps in MEV designing (87). Upon examining, 21 selected proteins exhibited high stability, with instability index values ranging from 14 to 36. Four proteins were excluded due to instability index values exceeding 40. The remaining proteins had molecular weights between 149 and 879 kDa and high aliphatic indices, indicating their ability to withstand high temperatures and suitability for vaccine design.
The active acquired immune responses are extremely specialized and focused on eliminating pathogens or preventing their development (88). Adaptive immunity produces memory B-cells that identify the organism after the initial recognition of subsequent encounters (89). Vaccination is based on such immunological recall of adaptive immunity (90). The primary function of the B and T lymphocyte cells in the adaptive immune system is to produce cellular immunity against invader organisms that are reliant on antibodies. Multiple B-cell epitopes were predicted for the proteins that were further processed for T-cell epitopes prediction in the form of MHC-I and MHC-II receptors. Because epitopes with the lowest percentile scores demonstrate the greatest affinity to receptors, they were given priority and chosen based on their low percentile scores (75).
Approximately 324 different epitopes were predicted, which were then processed for various analyses. The epitopes were filtered based on the following criteria: non-toxicity in living organisms, maximum solubility in water, maximum antigenicity, no allergic responses, and maximum binding with HLA-DRB1*0101 alleles. An essential immune system protein is made according to directions from the HLA-DRB1 gene. The human leukocyte antigen (HLA) complex is a cluster of genes that includes the HLA-DRB1 gene. The HLA complex helps the immune system distinguish between proteins produced by the host’s cells and those produced by foreign invaders, such as viruses and bacteria. The major histocompatibility complex (MHC), a gene family found in many species, is represented in humans by the HLA complex (91). The MHC class II subset of MHC genes includes the HLA-DRB1 gene (92). Certain immune system cells have proteins on their surface that are produced according to the instructions of MHC class II genes (93). These proteins bind to peptides, or short fragments of proteins, outside the cell. These peptides are presented to the immune system by MHC class II proteins. When the immune system detects peptides that are foreign to it, such as viral or bacterial peptides, it launches an attack against the invaders (94).
The final vaccine candidate was prepared by linking the epitopes together with the help of linkers that make the vaccine more stable and avoid self-binding between it. These linkers make the vaccine intact and expose the epitopes to bind the immune receptors sufficiently. The vaccine was also linked with an adjuvant known as cholera b toxin so that immune activation could be enhanced. The physicochemical properties of the final vaccine construct were checked which showed that it has 637 amino acids and has a molecular weight of 63.930 kDa which is far more in the acceptable range. The instability index showed a value of 28.82 which clearly illustrates that the vaccine is quite stable. The vaccine candidate was slightly acidic having a smaller number of positive and more negative residues and the theoretical PI was 5.51. It showed a hydrophilic nature due to a negative GRAVY value i-e -0.825 which is good for a normal protein to act efficiently inside the body (87). Antigenic analysis showed that the vaccine is highly antigenic and its half-life inside the body is greater than 20 hours which is normal for maximum proteins. The aliphatic index also had a maximum value of 49.09 which shows that the vaccine can withstand high temperatures.
The vaccine’s three-dimensional structure was obtained and refined for enhanced stability. Ramachandran plot and Verify3D analyses were performed to assess stability. The plot features four quadrants (I, II, III, and IV) where Phi angles (angles between alpha carbon and nitrogen) and Psi angles (angles between alpha carbon and carboxyl carbon) were plotted on the Y and X axes, respectively plus red and blue dots represent amino acid residues. The plot shown below Figure 5A) exhibits a common feature: it comprises four regions distinguished by color. The red region, known as the most favored region, represents amino acids with angle values (Phi and Psi) that lack steric hindrance, indicating that their molecules do not obstruct each other (95). In simpler terms, a greater abundance of amino acids in favored regions suggests enhanced flexibility for docking. The brown region, an additional permitted area, also signifies protein flexibility. The yellow region, or generously allowed region, poses high hindrance to Phi and Psi angles; an increased presence of amino acids here indicates processing challenges. The pale yellow, or disallowed region, strictly restricts Phi and Psi angle rotation due to steric hindrance (96). A higher proportion of amino acids in this region suggests that the protein cannot be processed. For the vaccine, the majority of amino acids were present in the red region, yielding a value of 78.1%, which is far more favorable and indicative of maximum stability.
Maximum binding of the vaccine with important receptors of the immune cells is important to evoke a robust immune response. The vaccine candidate was docked with the immune receptors i-e TLR4, MHC-I, and MHC-II respectively. The binding energy was quite high and a maximum number of amino acids were involved in the binding of both proteins. Examining the docked complex of MEV-TRL4, the binding energy was -1040.8 kcal/mol, the number of non-bonded interactions was 216, hydrogen bonds were 23, and the disulfide bond was 1. Number of residues involved in these interactions were 58. The docked complex of MEV-MHC I illustrates a binding energy of -871.4 kcal/mol and several non-bonded and bonded interactions and were i-e 162 non-bonded, 8 hydrogen bonds, and 3 disulfide bonds, and many residues involved in hydrophobic interactions were 57. The docking complex of Vaccine-MHC II resulted a binding energy of -1154.5 kcal/mol showed 147 non-bonded interactions, 8 hydrogen bonds, 1 disulfide bond, and 80 residues involved. Simulation studies for the complexes also show promising results which revealed acceptable deformability and B-factor values which means that residues of both proteins show deformability from their actual position at the time of interactions. Eigenvalues and variances show that less energy is required to displace the residues from their origins showing easy deformability of the complexes.
Being a promising vaccine candidate fulfilling all the requirements, in-silico cloning was assured to produce this vaccine and make it available to humanity. To achieve this, the principles of recombinant DNA technology were followed, involving finding a suitable vector to insert the desired vaccine sequence and using an expression system to produce it. For this, the pet28a(+) vector and E. coli were used as the expression system because it has the highest multiplication rate.
5 Conclusions
In this work, we have developed a multi-epitope vaccine construct against the pathogenic bacteria E. fergusonii, which causes a range of diseases in people, utilizing several bioinformatics techniques. This bacterium has acquired antibiotic resistance as a result of improper use of antibiotics and the absence of a recognized immunization. The sole means of preventing illnesses brought on by E. fergusonii was vaccination. For the components of the immune system, the MEV sequences utilized in the produced vaccine have the best adhesion efficacy and are antigenic, non-allergic, highly soluble, and non-toxic. To elicit a strong immune response against MEV, an adjuvant was added and these obtained epitopes were connected by linkers. The immunological responses to the planned vaccine were highly concerning, and the vaccine itself was fairly stable. The results and predictions of the current study should expedite the process of developing a vaccination against E. fergusonii and provide insights that may help in this regard. The study’s findings will also help save time and money, and experimental vaccine scientists will be helped by the in-silico vaccine design to produce a vaccine against E. fergusonii infections for both therapeutic and preventive uses.
Data availability statement
The original contributions presented in the study are included in the article/Supplementary Material. Further inquiries can be directed to the corresponding author.
Author contributions
TA: Conceptualization, Data curation, Formal analysis, Funding acquisition, Investigation, Methodology, Project administration, Resources, Software, Supervision, Validation, Visualization, Writing – original draft, Writing – review & editing.
Funding
The author(s) declare financial support was received for the research, authorship, and/or publication of this article. The author would like to acknowledge the Researchers Supporting Project number (RSPD2023R632), King Saud University, Riyadh, Saudi Arabia.
Acknowledgments
The author extend their appreciation to the Researchers Supporting Project number (RSPD2023R632), King Saud University, Riyadh, Saudi Arabia.
Conflict of interest
The author declares that the research was conducted in the absence of any commercial or financial relationships that could be construed as a potential conflict of interest.
Publisher’s note
All claims expressed in this article are solely those of the authors and do not necessarily represent those of their affiliated organizations, or those of the publisher, the editors and the reviewers. Any product that may be evaluated in this article, or claim that may be made by its manufacturer, is not guaranteed or endorsed by the publisher.
Supplementary material
The Supplementary Material for this article can be found online at: https://www.frontiersin.org/articles/10.3389/fimmu.2023.1332378/full#supplementary-material
References
1. Glover B, Wentzel J, Jenkins A, Van Vuuren M. The first report of Escherichia fergusonii isolated from non-human primates, in Africa. One Heal (2017) 3:70–5. doi: 10.1016/j.onehlt.2017.05.001
2. Gaastra W, Kusters JG, Van Duijkeren E, Lipman LJA. Escherichia fergusonii. Vet Microbiol (2014) 172:7–12. doi: 10.1016/j.vetmic.2014.04.016
3. Farmer 3JJ, Fanning GR, Davis BR, O’hara CM, Riddle C, Hickman-Brenner FW, et al. Escherichia fergusonii and Enterobacter taylorae, two new species of Enterobacteriaceae isolated from clinical specimens. J Clin Microbiol (1985) 21:77–81. doi: 10.1128/jcm.21.1.77-81.1985
4. Lai C-C, Cheng A, Huang Y-T, Chung K-P, Lee M-R, Liao C-H, et al. Escherichia fergusonii bacteremia in a diabetic patient with pancreatic cancer. J Clin Microbiol (2011) 49:4001–2. doi: 10.1128/JCM.05355-11
5. Dahal RH, Choi Y-J, Kim S, Kim J. Differentiation of Escherichia fergusonii and Escherichia coli Isolated from Patients with Inflammatory Bowel Disease/Ischemic Colitis and Their Antimicrobial Susceptibility Patterns. Antibiotics (2023) 12:154. doi: 10.3390/antibiotics12010154
6. Paul M, Bishara J, Levcovich A, Chowers M, Goldberg E, Singer P, et al. Effectiveness and safety of colistin: prospective comparative cohort study. J Antimicrob Chemother (2010) 65:1019–27. doi: 10.1093/jac/dkq069
7. Lin J, Tang B, Zheng X, Chang J, Ma J, He Y, et al. Emergence of Incl2 plasmid-mediated colistin resistance in avian Escherichia fergusonii. FEMS Microbiol Lett (2022) 369:fnac016. doi: 10.1093/femsle/fnac016
8. Tang B, Chen Y, Zhang L, Chang J, Xia X, Yang H. Complete genome sequence of colistin-resistant Escherichia fergusonii strain EFCF056. Microbiol Resour Announc. (2020) 9:e01571–19. doi: 10.1128/MRA.01571-19
9. Tang B, Chang J, Chen Y, Lin J, Xiao X, Xia X, et al. Escherichia fergusonii, an underrated repository for antimicrobial resistance in food animals. Microbiol Spectr. (2022) 10:e01617–21. doi: 10.1128/spectrum.01617-21
10. Serwecińska L. Antimicrobials and antibiotic-resistant bacteria: a risk to the environment and to public health. Water (2020) 12:3313. doi: 10.3390/w12123313
11. MacLean RC, San Millan A. The evolution of antibiotic resistance. Sci (80-.). (2019) 365:1082–3. doi: 10.1126/science.aax3879
12. Volz A, Sutter G. Modified vaccinia virus Ankara: history, value in basic research, and current perspectives for vaccine development. Adv Virus Res (2017) 97:187–243. doi: 10.1016/bs.aivir.2016.07.001
13. Ribas-Aparicio RM, Castelán-Vega JA, Jiménez-Alberto A, Monterrubio-López GP, Aparicio-Ozores G. The impact of bioinformatics on vaccine design and development. Vaccines (2017) 2:3–6. doi: 10.5772/intechopen.69273
14. Chukwudozie OS, Duru VC, Ndiribe CC, Aborode AT, Oyebanji VO, Emikpe BO. The relevance of bioinformatics applications in the discovery of vaccine candidates and potential drugs for COVID-19 treatment. Bioinform Biol Insights (2021) 15:11779322211002168. doi: 10.1177/11779322211002168
15. Dhanda SK, Usmani SS, Agrawal P, Nagpal G, Gautam A, Raghava GPS. Novel in silico tools for designing peptide-based subunit vaccines and immunotherapeutics. Brief. Bioinform (2017) 18:467–78. doi: 10.1093/bib/bbw025
16. Agallou M, Athanasiou E, Koutsoni O, Dotsika E, Karagouni E. Experimental validation of multi-epitope peptides including promising MHC class I-and II-restricted epitopes of four known Leishmania infantum proteins. Front Immunol (2014) 5:268. doi: 10.3389/fimmu.2014.00268
17. Ramirez-Salinas GL, Garcia-Machorro J, Rojas-Hernández S, Campos-Rodriguez R, de Oca AC-M, Gomez MM, et al. Bioinformatics design and experimental validation of influenza A virus multi-epitopes that induce neutralizing antibodies. Arch Virol (2020) 165:891–911. doi: 10.1007/s00705-020-04537-2
18. Agarwala R, Barrett T, Beck J, Benson DA, Bollin C, Bolton E, et al. Database resources of the national center for biotechnology information. Nucleic Acids Res (2018) 46(D1):D8–D13. doi: 10.1093/nar/gkx1095
19. Chaudhari NM, Gupta VK, Dutta C. BPGA-an ultra-fast pan-genome analysis pipeline. Sci Rep (2016) 6:1–10. doi: 10.1038/srep24373
20. Sanober G, Ahmad S, Azam SS. Identification of plausible drug targets by investigating the druggable genome of MDR Staphylococcus epidermidis. Gene Rep (2017) 7:147–53. doi: 10.1016/j.genrep.2017.04.008
21. Huang Y, Niu B, Gao Y, Fu L, Li W. CD-HIT Suite: a web server for clustering and comparing biological sequences. Bioinformatics (2010) 26:680–2. doi: 10.1093/bioinformatics/btq003
22. Gardy JL, Laird MR, Chen F, Rey S, Walsh CJ, Ester M, et al. PSORTb v. 2.0: expanded prediction of bacterial protein subcellular localization and insights gained from comparative proteome analysis. Bioinformatics (2005) 21:617–23. doi: 10.1093/bioinformatics/bti057
23. Naz A, Awan FM, Obaid A, Muhammad SA, Paracha RZ, Ahmad J, et al. Identification of putative vaccine candidates against Helicobacter pylori exploiting exoproteome and secretome: a reverse vaccinology based approach. Infect Genet Evol (2015) 32:280–91. doi: 10.1016/j.meegid.2015.03.027
24. Liu B, Zheng D, Zhou S, Chen L, Yang J. VFDB 2022: a general classification scheme for bacterial virulence factors. Nucleic Acids Res (2022) 50:D912–7. doi: 10.1093/nar/gkab1107
25. Chen L, Yang J, Yu J, Yao Z, Sun L, Shen Y, et al. VFDB: a reference database for bacterial virulence factors. Nucleic Acids Res (2005) 33:D325–8. doi: 10.1093/nar/gki008
26. Flower DR, Macdonald IK, Ramakrishnan K, Davies MN, Doytchinova IA. Computer aided selection of candidate vaccine antigens. Immunome Res (2010) 6:1–16. doi: 10.1186/1745-7580-6-S2-S1
27. Mahram A, Herbordt MC. NCBI BLASTP on high-performance reconfigurable computing systems. ACM Transactions on Reconfigurable Technology and Systems (TRETS) (2015) 7(4), 1–20.
28. Hassan A, Naz A, Obaid A, Paracha RZ, Naz K, Awan FM, et al. Pangenome and immuno-proteomics analysis of Acinetobacter baumannii strains revealed the core peptide vaccine targets. BMC Genomics (2016) 17:1–25. doi: 10.1186/s12864-016-2951-4
29. Gasteiger E, Hoogland C, Gattiker A, Duvaud S, Wilkins MR, Appel RD, et al. Protein identification and analysis tools on the ExPASy server. Switzerland: Springer (2005). doi: 10.1385/1-59259-584-7:531
30. Rashid MI, Naz A, Ali A, Andleeb S. Prediction of vaccine candidates against Pseudomonas aeruginosa: An integrated genomics and proteomics approach. Genomics (2017) 109:274–83. doi: 10.1016/j.ygeno.2017.05.001
31. Gamage DG, Gunaratne A, Periyannan GR, Russell TG. Applicability of instability index for in vitro protein stability prediction. Protein Pept Lett (2019) 26:339–47. doi: 10.2174/0929866526666190228144219
32. Möller S, Croning MDR, Apweiler R. Evaluation of methods for the prediction of membrane spanning regions. Bioinformatics (2001) 17:646–53. doi: 10.1093/bioinformatics/17.7.646
33. Jespersen MC, Peters B, Nielsen M, Marcatili P. BepiPred-2.0: improving sequence-based B-cell epitope prediction using conformational epitopes. Nucleic Acids Res (2017) 45:W24–9. doi: 10.1093/nar/gkx346
34. Larsen MV, Lundegaard C, Lamberth K, Buus S, Lund O, Nielsen M. Large-scale validation of methods for cytotoxic T-lymphocyte epitope prediction. BMC Bioinf (2007) 8:1–12. doi: 10.1186/1471-2105-8-424
35. Dhanda SK, Mahajan S, Paul S, Yan Z, Kim H, Jespersen MC, et al. IEDB-AR: immune epitope database—analysis resource in 2019. Nucleic Acids Res (2019) 47:W502–6. doi: 10.1093/nar/gkz452
36. Doytchinova IA, Flower DR. VaxiJen: a server for prediction of protective antigens, tumour antigens and subunit vaccines. BMC Bioinf (2007) 8:1–7. doi: 10.1186/1471-2105-8-4
37. Gupta S, Kapoor P, Chaudhary K, Gautam A, Kumar R, Raghava GPS. Peptide toxicity prediction. In: Computational peptidology. India: Springer (2015). p. 143–57.
38. Masadeh MM, Laila SA, Haddad R, Alzoubi K, Alhaijaa AA, Alrabadi N. The antimicrobial effect against multi-drug resistant bacteria of the SK4 peptide: A novel hybrid peptide of cecropin-A and BMAP-27. Curr Pharm Biotechnol (2023) 24:1070–8. doi: 10.2174/1566524023666221031144028
39. Dimitrov I, Bangov I, Flower DR, Doytchinova I. AllerTOP v. 2—a server for in silico prediction of allergens. J Mol Model (2014) 20:1–6. doi: 10.1007/s00894-014-2278-5
40. Khan F, Srivastava V, Kumar A. Computational identification and characterization of potential T-cell epitope for the utility of vaccine design against enterotoxigenic Escherichia coli. Int J Pept Res Ther (2019) 25:289–302. doi: 10.1007/s10989-018-9671-3
41. Arai R, Ueda H, Kitayama A, Kamiya N, Nagamune T. Design of the linkers which effectively separate domains of a bifunctional fusion protein. Protein Eng. (2001) 14:529–32. doi: 10.1093/protein/14.8.529
42. Coler RN, Baldwin SL, Shaverdian N, Bertholet S, Reed SJ, Raman VS, et al. A synthetic adjuvant to enhance and expand immune responses to influenza vaccines. PloS One (2010) 5:e13677. doi: 10.1371/journal.pone.0013677
43. Castiglione F, Bernaschi M. (2004). C-immsim: playing with the immune response, in: Proceedings of the Sixteenth International Symposium on Mathematical Theory of Networks and Systems (MTNS2004). Available at: https://150.146.2.1/C-IMMSIM/index.php.
44. Zhou X, Zheng W, Li Y, Pearce R, Zhang C, Bell EW, et al. I-TASSER-MTD: a deep-learning-based platform for multi-domain protein structure and function prediction. Nat Protoc (2022) 17:2326–53. doi: 10.1038/s41596-022-00728-0
45. Yang J, Zhang Y. Protein structure and function prediction using I-TASSER. Curr Protoc Bioinforma. (2015) 52:5–8. doi: 10.1002/0471250953.bi0508s52
46. Yang J, Zhang W, He B, Walker SE, Zhang H, Govindarajoo B, et al. Template-based protein structure prediction in CASP11 and retrospect of I-TASSER in the last decade. Proteins Struct Funct Bioinforma. (2016) 84:233–46. doi: 10.1002/prot.24918
47. Xu D, Zhang J, Roy A, Zhang Y. Automated protein structure modeling in CASP9 by I-TASSER pipeline combined with QUARK-based ab initio folding and FG-MD-based structure refinement. Proteins Struct Funct Bioinforma. (2011) 79:147–60. doi: 10.1002/prot.23111
48. Shin W-H, Lee GR, Heo L, Lee H, Seok C. Prediction of protein structure and interaction by GALAXY protein modeling programs. Bio Des (2014) 2:1–11. Available at: https://www.bdjn.org/journal/view.html?uid=6
49. Grotthuss M, Pas J, Wyrwicz L, Ginalski K, Rychlewski L. Application of 3D-Jury, GRDB, and Verify3D in fold recognition. Proteins Struct Funct Bioinforma. (2003) 53:418–23. doi: 10.1002/prot.10547
50. Laskowski RA, Chistyakov VV, Thornton JM. PDBsum more: new summaries and analyses of the known 3D structures of proteins and nucleic acids. Nucleic Acids Res (2005) 33:D266–8. doi: 10.1093/nar/gki001
51. Kozakov D, Hall DR, Xia B, Porter KA, Padhorny D, Yueh C, et al. The ClusPro web server for protein–protein docking. Nat Protoc (2017) 12:255–78. doi: 10.1038/nprot.2016.169
52. Pettersen EF, Goddard TD, Huang CC, Couch GS, Greenblatt DM, Meng EC, et al. UCSF Chimera—a visualization system for exploratory research and analysis. J Comput Chem (2004) 25:1605–12. doi: 10.1002/jcc.20084
53. Van Gunsteren WF, Berendsen HJC. Computer simulation of molecular dynamics: methodology, applications, and perspectives in chemistry. Angew. Chemie Int Ed English (1990) 29:992–1023. doi: 10.1002/anie.199009921
54. Lopéz-Blanco JR, Garzón JI, Chacón P. iMod: multipurpose normal mode analysis in internal coordinates. Bioinformatics (2011) 27:2843–50. doi: 10.1093/bioinformatics/btr497
55. Grote A, Hiller K, Scheer M, Münch R, Nörtemann B, Hempel DC, et al. JCat: a novel tool to adapt codon usage of a target gene to its potential expression host. Nucleic Acids Res (2005) 33:W526–31. doi: 10.1093/nar/gki376
56. Dana H, Mahmoodi Chalbatani G, Gharagouzloo E, Miri SR, Memari F, Rasoolzadeh R, et al. In silico analysis, molecular docking, molecular dynamic, cloning, expression and purification of chimeric protein in colorectal cancer treatment. Drug Des Devel. Ther (2020) 14:309–29. doi: 10.2147/DDDT.S231958
57. Li L, Li H, Tian Q, Ge B, Xu X, Chi Y, et al. Expression and purification of soluble recombinant $β$-lactamases using Escherichia coli as expression host and pET-28a as cloning vector. Microb Cell Fact. (2022) 21:1–9. doi: 10.1186/s12934-022-01972-5
58. Tupin E, Kinjo Y, Kronenberg M. The unique role of natural killer T cells in the response to microorganisms. Nat Rev Microbiol (2007) 5:405–17. doi: 10.1038/nrmicro1657
59. Umlauf BJ, Haralambieva IH, Ovsyannikova IG, Kennedy RB, Pankratz VS, Jacobson RM, et al. Associations between demographic variables and multiple measles-specific innate and cell-mediated immune responses after measles vaccination. Viral Immunol (2012) 25:29–36. doi: 10.1089/vim.2011.0051
60. Kolla HB, Tirumalasetty C, Sreerama K, Ayyagari VS. An immunoinformatics approach for the design of a multi-epitope vaccine targeting super antigen TSST-1 of Staphylococcus aureus. J Genet Eng. Biotechnol (2021) 19:1–14. doi: 10.1186/s43141-021-00160-z
61. Dong R, Chu Z, Yu F, Zha Y. Contriving multi-epitope subunit of vaccine for COVID-19: immunoinformatics approaches. Front Immunol (2020) 11:1784. doi: 10.3389/fimmu.2020.01784
62. Venkateswarulu TC, Shaik AT, Meduri DS, Dhusia K, Peele A. In silico designing of a multitope vaccine against Rhizopus microspores. Arab Gulf J Sci Res (2023). doi: 10.1108/AGJSR-11-2022-0274
63. Holmgren J, Lycke N, Czerkinsky C. Cholera toxin and cholera B subunit as oral—mucosal adjuvant and antigen vector systems. Vaccine (1993) 11:1179–84. doi: 10.1016/0264-410X(93)90039-Z
64. Stratmann T. Cholera toxin subunit B as adjuvant—an accelerator in protective immunity and a break in autoimmunity. Vaccines (2015) 3:579–96. doi: 10.3390/vaccines3030579
65. Ghaem-Maghami M, Simmons CP, Daniell S, Pizza M, Lewis D, Frankel G, et al. Intimin-specific immune responses prevent bacterial colonization by the attaching-effacing pathogen Citrobacter rodentium. Infect Immun (2001) 69:5597–605. doi: 10.1128/IAI.69.9.5597-5605.2001
66. Mahapatra SR, Dey J, Kaur T, Sarangi R, Bajoria AA, Kushwaha GS, et al. Immunoinformatics and molecular docking studies reveal a novel Multi-Epitope peptide vaccine against pneumonia infection. Vaccine (2021) 39:6221–37. doi: 10.1016/j.vaccine.2021.09.025
67. Wu S, Skolnick J, Zhang Y. Ab initio modeling of small proteins by iterative TASSER simulations. BMC Biol (2007) 5:1–10. doi: 10.1186/1741-7007-5-17
68. Kaur R, Arora N, Jamakhani MA, Malik S, Kumar P, Anjum F, et al. Development of multi-epitope chimeric vaccine against Taenia solium by exploring its proteome: an in silico approach. Expert Rev Vaccines (2020) 19:105–14. doi: 10.1080/14760584.2019.1711057
69. Raj VSS, Monisha M, Paramasivam G. In-silico screening of synthetic inhibitors for human poly (Adp-ribose) polymerase 2 enzyme using patch dock software for ovarian cancer therapy. Rev GEINTEC-GESTAO Inov. E Tecnol. (2021) 11:5910–23. Available at: https://www.academia.edu/70120190/In_silico_Screening_of_Synthetic_Inhibitors_for_Human_Poly_Adp_ribose_Polymerase_2_Enzyme_Using_Patch_Dock_Software_for_Ovarian_Cancer_Therapy
70. Hollingsworth SA, Karplus PA. A fresh look at the Ramachandran plot and the occurrence of standard structures in proteins. Biomol Concepts (2010) 1(3-4):271–83. doi: 10.1515/BMC.2010.022
71. Haridhasapavalan KK, Sundaravadivelu PK, Thummer RP. Codon optimization, cloning, expression, purification, and secondary structure determination of human ETS2 transcription factor. Mol Biotechnol (2020) 62:485–94. doi: 10.1007/s12033-020-00266-8
72. Hess EJ, Jinnah HA, Kozak CA, Wilson MC. Spontaneous locomotor hyperactivity in a mouse mutant with a deletion including the Snap gene on chromosome 2. J Neurosci (1992) 12:2865–74. doi: 10.1523/JNEUROSCI.12-07-02865.1992
73. Breijyeh Z, Jubeh B, Karaman R. Resistance of gram-negative bacteria to current antibacterial agents and approaches to resolve it. Molecules (2020) 25:1340. doi: 10.3390/molecules25061340
74. Pechere JC. Patients’ interviews and misuse of antibiotics. Clin Infect Dis (2001) 33:S170–3. doi: 10.1086/321844
75. Khan Z, Sanjai S, Harshitha BV, Patil S. In-silico multi-epitope vaccine candidate against type-1 parainfluenza virus. (2023). doi: 10.21203/rs.3.rs-2455059/v1
76. Rahman S, Sarkar K, Das AK. Exploring staphylococcal superantigens to design a potential multi-epitope vaccine against Staphylococcus aureus: an in-silico reverse vaccinology approach. J Biomol Struct Dyn. (2023) 22:1–15. doi: 10.1080/07391102.2023.2171138
77. Meza B, Ascencio F, Sierra-Beltrán AP, Torres J, Angulo C. A novel design of a multi-antigenic, multistage and multi-epitope vaccine against Helicobacter pylori: an in silico approach. Infect Genet Evol (2017) 49:309–17. doi: 10.1016/j.meegid.2017.02.007
78. Abraham Peele K, Srihansa T, Krupanidhi S, Ayyagari VS, Venkateswarulu TC. Design of multi-epitope vaccine candidate against SARS-CoV-2: A in-silico study. J Biomol Struct Dyn. (2021) 39:3793–801. doi: 10.1080/07391102.2020.1770127
79. Narang PK, Dey J, Mahapatra SR, Roy R, Kushwaha GS, Misra N, et al. Genome-based identification and comparative analysis of enzymes for carotenoid biosynthesis in microalgae. World J Microbiol Biotechnol (2022) 38:1–22. doi: 10.1007/s11274-021-03188-y
80. Reginald K, Chan Y, Plebanski M, Poh CL. Development of peptide vaccines in dengue. Curr Pharm Des (2018) 24:1157–73.
81. Li Y, Zheng K, Tan Y, Wen Y, Wang C, Chen Q, et al. A recombinant multi-epitope peptide vaccine based on MOMP and CPSIT\_p6 protein protects against Chlamydia psittaci lung infection. Appl Microbiol Biotechnol (2019) 103:941–52. doi: 10.1007/s00253-018-9513-4
82. Bhattacharya M, Sharma AR, Sharma G, Patra P, Mondal N, Patra BC, et al. Computer aided novel antigenic epitopes selection from the outer membrane protein sequences of Aeromonas hydrophila and its analyses. Infect Genet Evol (2020) 82:104320. doi: 10.1016/j.meegid.2020.104320
83. Mohinani T, Saxena A, Singh SV. In silico prediction of epitopes in virulence proteins of mycobacterium ulcerans for vaccine designing. Curr Genomics (2021) 22:512. doi: 10.2174/1389202922666211129113917
84. Hossain MS, Azad AK, Chowdhury PA, Wakayama M. Computational identification and characterization of a promiscuous T-cell epitope on the extracellular protein 85B of Mycobacterium spp. for peptide-based subunit vaccine design. BioMed Res Int (2017), 4826030. doi: 10.1155/2017/4826030
85. Katalani C, Nematzadeh G, Ahmadian G, Amani J, Kiani G, Ehsani P. In silico design and in vitro analysis of a recombinant trivalent fusion protein candidate vaccine targeting virulence factor of Clostridium perfringens. Int J Biol Macromol. (2020) 146:1015–23. doi: 10.1016/j.ijbiomac.2019.09.227
86. Alsubaiyel AM, Bukhari SI. Computational exploration and design of a multi-epitopes vaccine construct against Chlamydia psittaci. J Biomol Struct Dyn. (2023) 1–17. doi: 10.1080/07391102.2023.2268173
87. Garg VK, Avashthi H, Tiwari A, Jain PA, Ramkete PW, Kayastha AM, et al. MFPPI–multi FASTA protParam interface. Bioinformation (2016) 12:74. doi: 10.6026/97320630012074
88. Chaplin DD. Overview of the immune response. J Allergy Clin Immunol (2010) 125:S3–S23. doi: 10.1016/j.jaci.2009.12.980
89. Merlo LMF, Mandik-Nayak L. Adaptive immunity: B cells and antibodies. In: Cancer immunotherapy. United Kingdom: Elsevier (2013). p. 25–40.
90. Oberholzer A, Oberholzer C, Moldawer LL. Sepsis syndromes: understanding the role of innate and acquired immunity. Shock (2001) 16:83–96. doi: 10.1097/00024382-200116020-00001
91. Janeway JCA, Travers P, Walport M, Shlomchik MJ. The major histocompatibility complex and its functions. In: Immunobiology: The Immune System in Health and Disease, 5th edition. New York, United States of America: Garland Science (2001).
92. Houtman M, Hesselberg E, Rönnblom L, Klareskog L, Malmström V, Padyukov L. Haplotype-specific expression analysis of MHC class II genes in healthy individuals and rheumatoid arthritis patients. Front Immunol (2021) 12:707217. doi: 10.3389/fimmu.2021.707217
93. Villadangos JA. Presentation of antigens by MHC class II molecules: getting the most out of them. Mol Immunol (2001) 38:329–46. doi: 10.1016/S0161-5890(01)00069-4
94. Strobel S, Ferguson A. Immune responses to fed protein antigens in mice. 3. Systemic tolerance or priming is related to age at which antigen is first encountered. Pediatr Res (1984) 18:588–94. doi: 10.1203/00006450-198407000-00004
95. Zhou AQ, O’Hern CS, Regan L. Revisiting the Ramachandran plot from a new angle. Protein Sci (2011) 20:1166–71. doi: 10.1002/pro.644
Keywords: Escherichia fergusonii, multi-epitopes vaccine, immunoinformatics, molecular dynamics simulation, reverse vaccinology
Citation: Almanaa TN (2023) Design of a novel multi-epitopes vaccine against Escherichia fergusonii: a pan-proteome based in- silico approach. Front. Immunol. 14:1332378. doi: 10.3389/fimmu.2023.1332378
Received: 02 November 2023; Accepted: 23 November 2023;
Published: 08 December 2023.
Edited by:
Sumra Wajid Abbasi, National University of Medical Sciences (NUMS), PakistanReviewed by:
Anam Naz, University of Lahore, PakistanBilal Shaker, Ewha Womans University, Republic of Korea
Copyright © 2023 Almanaa. This is an open-access article distributed under the terms of the Creative Commons Attribution License (CC BY). The use, distribution or reproduction in other forums is permitted, provided the original author(s) and the copyright owner(s) are credited and that the original publication in this journal is cited, in accordance with accepted academic practice. No use, distribution or reproduction is permitted which does not comply with these terms.
*Correspondence: Taghreed N. Almanaa, dGFsbWFuYWFAa3N1LmVkdS5zYQ==