- Department of Obstetrics and Gynecology, The Second Affiliated Hospital of Harbin Medical University, Harbin, China
Background: Hypertensive disorders of pregnancy (HDP) pose a significant risk to maternal and fetal well-being; however, the etiology and pathogenesis of HDP remain ambiguous. It is now widely acknowledged that inflammatory response and the immune system are closely related to HDP. Previous research has identified several inflammatory cytokines are associated with HDP. This study applied Mendelian randomization (MR) analysis to further assess causality.
Methods: Patients with HDP who participated in the MR analysis presented with four types of HDP: pre-eclampsia or eclampsia (PE); gestational hypertension (GH); pre-existing hypertension complicating pregnancy, childbirth and the puerperium (EH); and pre-eclampsia or poor fetal growth (PF). A two-sample MR analysis was used to analyze the data in the study. The causal relationship between exposure and outcome was analyzed with inverse variance weighting (IVW), MR Egger, weighted median, weighted mode, and simple mode methods, where IVW was the primary method employed.
Results: Our MR analysis demonstrated a reliable causative effect of Interleukin-9 (IL-9) and macrophage migration inhibitory factor (MIF) on reducing HDP risk, while macrophage inflammatory protein 1-beta (MIP1b), Interleukin-13 (IL-13), and Interleukin-16 (IL-16) were associated with promoting HDP risk.
Conclusions: This study demonstrated that IL-9, MIF, MIP1b, IL-13, and IL-16 may be cytokines associated with the etiology of HDP, and that a number of inflammatory cytokines are probably involved in the progression of HDP. Additionally, our study revealed that these inflammatory cytokines have causal associations with HDP and may likely be potential therapeutic targets for HDP.
1 Introduction
Hypertensive disorders of pregnancy (HDP)—including pre-eclampsia/eclampsia, gestational hypertension, chronic hypertension, and pre-eclampsia superimposed on chronic hypertension (1)—is ranked as the second leading cause of maternal death globally, after maternal hemorrhage (2). Apart from causing harm to the mother, HDP is also associated with adverse fetal outcomes, such as preterm labor and fetal growth restriction (3). The exact pathogenesis of HDP is unclear, and current studies have found that endothelial dysfunction, insufficient trophoblastic invasion, abnormal angiogenesis, and aberrant uterine artery remodeling are considered to be key elements in its development (4–6). Among women in the gestational state, inappropriate immune activation and subsequent inflammation may result in maternal vasculopathy or placental dysfunction, thereby contributing to HDP (7).. Previous studies have revealed that certain inflammatory cytokines are strongly correlated with HDP, such as macrophage inflammatory protein 1-beta (MIP1b) and interleukin-18 (IL-18) (8, 9). However, the causal link of various cytokines and HDP is difficult to establish through observational studies because of interference from confounding variables and reverse causations (10).
Mendelian randomization (MR) is an instrumental variable (IV) analysis which utilizes genetic variation as the IV to assess the causality for exposure and outcome (11). The advantages of MR analysis are as follows: first, genetic variations are distributed at random during meiosis and, thus, the likelihood of correlation with environmental confounders is rare (12); second, genotype distribution precedes acquired exposure in time and the two are not impacted by reverse causality; third, exposure-related genetic variation generally accompanies individuals from birth to adulthood, thereby avoiding attenuation due to error in causal inference (regression dilution bias) (13). Additionally, Mendel’s laws of inheritance are universal in the population, thereby avoiding the problem of representativeness in randomized controlled experiments. A two-sample MR analysis enables assessment of the relation between exposure and outcome in two independent populations, thereby expanding the scope of application and improving validity. MR analysis was performed on the basis of the following three hypotheses related to IVs. The first hypothesis is that the selected IVs are genetic variants highly correlated to the exposure. The second hypothesis is that IVs have no association with confounding factors between exposure and outcome. The third hypotheses is that IVs make no difference to outcome directly and merely affect outcome through exposure (14).
The aim of this study is to investigate the causal association between HDP and 41 inflammatory cytokines by using MR analysis and to identify inflammatory cytokines that can serve as potential biomarkers to facilitate early detection and treatment strategies for HDP.
2 Materials and methods
2.1 Study design
We used published summarized genome-wide association studies (GWAS) data for 41 circulating inflammatory cytokines and 4 types of HDP. In our study, a two-sample MR analysis was carried out in order to investigate bidirectional causality between inflammatory cytokines and HDP. This MR study was in accordance with the guidelines of Strengthening the Reporting of Observational Studies in Epidemiology using Mendelian Randomization (STROBE-MR) (15). The study design overview is presented in Figure 1.
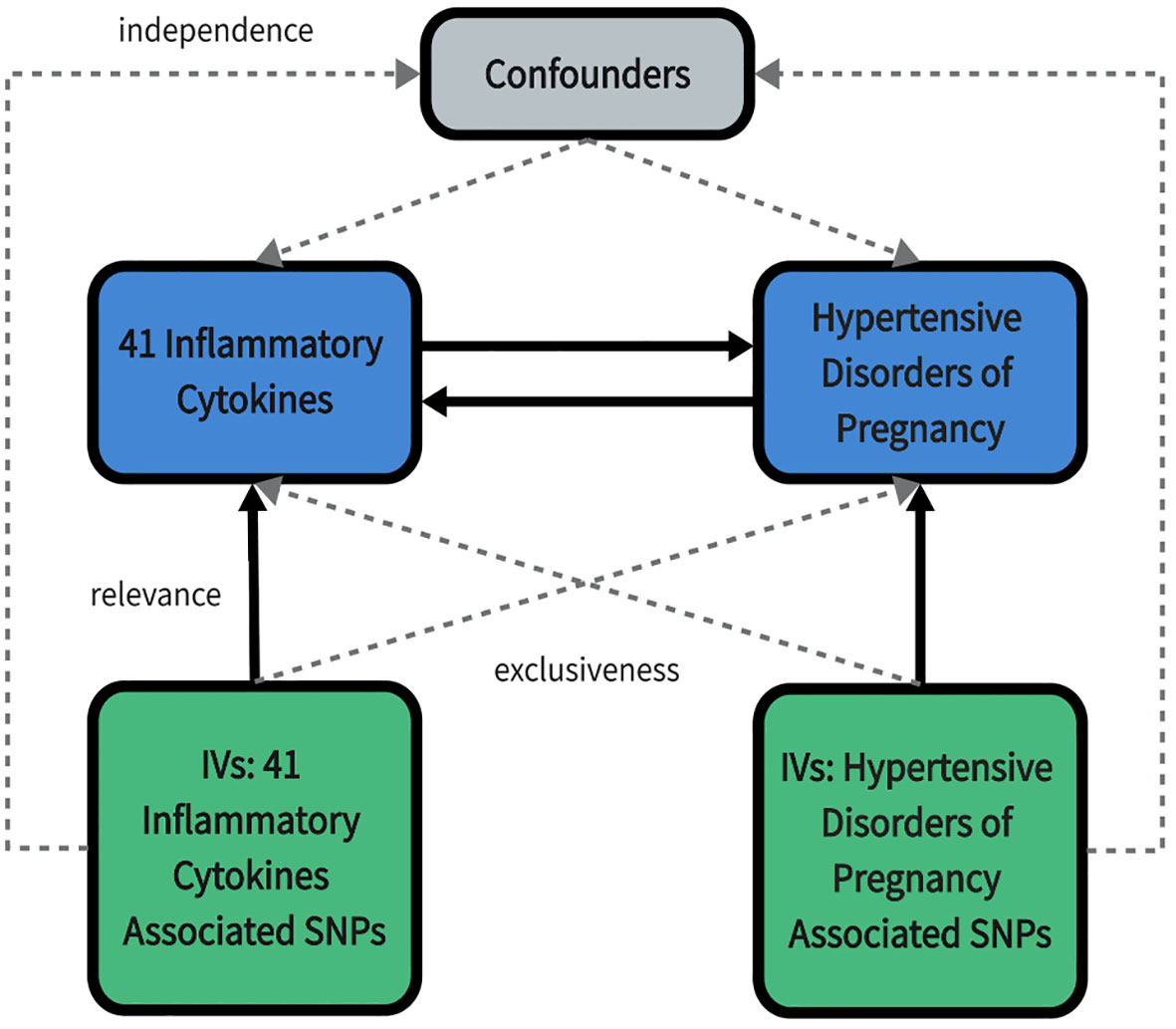
Figure 1 An overall design of the present study. IV, Instrumental variable; SNP, Single nucleotide polymorphism.
2.2 Data sources
Data on inflammatory cytokines was taken from a GWAS meta-analysis that provided genomic variants in 8293 Finnish individuals (16). This study was conducted in three cohorts including The Cardiovascular Risk in Young Finns Study (YFS) and FINRISK surveys of the 1997 and 2002, which have already been completed. The average age of the participants in the YFS study was 37 years and that in the FINRISK survey was 60 years. The GWAS data of inflammatory cytokines used in this MR analysis were after adjusting for age, sex, and body-mass index (BMI). HDP-summarized GWAS data were retrieved from FinnGen database (https://www.finngen.fi/en) R9 version. According to the definition of HDP we included two cohorts of gestational hypertension; and pre-eclampsia/eclampsia. However, since the GWAS data for chronic hypertension were not available and the pre-eclampsia superimposed on chronic hypertension cohort had only 154 case samples, these two categories under the HDP definition were unable to be included in this MR study. In addition, we found two cohorts with larger sample sizes, pre-existing hypertension complicating pregnancy, childbirth and the puerperium and pre-eclampsia or poor fetal growth, which were thus included in the study. Finally, we selected four cohorts for inclusion in this MR analysis, including pre-eclampsia or eclampsia (PE); gestational hypertension (GH); pre-existing hypertension complicating pregnancy, childbirth and the puerperium (EH); and pre-eclampsia or poor fetal growth (PF). No overlap existed between the exposure and outcome groups in the population. Table S1 lists details of the four types of HDP.
2.3 Instrumental variable selection
First, we applied a genome-wide significance threshold of p < 5 × 10-8 so as to filter out single nucleotide polymorphisms (SNP) that were closely associated with HDP and inflammatory cytokines. A few inflammatory cytokines were found under this criterion that did not have a sufficient number of SNPs to perform MR analysis; thus, a higher cutoff value (p < 5 × 10-6) was selected. Second, when inflammatory cytokines were used as exposure, we clumped SNPs (kb = 10000, r2 = 0.01), which implies that SNPs with r2 > 0.01 in the 10000 kb range with the most significant SNPs were eliminated, thereby avoiding linkage disequilibrium (LD). Whereas for HDP as exposure, the number of SNPs was sufficient and, hence, a higher criterion for eliminating LD was set—kb = 10000, r2 = 0.001. As we could not determine whether the palindromic SNPs were oriented in a consistent direction between exposure and outcome, these SNPs were not involved in MR analysis. Third, the R2 value was calculated for each SNP, which indicates the extent of exposure explained by IVs; moreover, to avoid weak instrumental bias, we evaluated the strength of the IV correlations by calculating the F-statistic and selected SNPs with F-statistic >10 for inclusion in this MR analysis. Finally, whenever unavailable SNPs are found, we use the LDlink Website (https://ldlink.nci.nih.gov/) to search for proxy SNPs (r2 > 0.8) to replace them.
2.4 Statistical analysis
MR analysis is conducted utilizing the “TwoSampleMR” R package (Version 0.5.6) of the RStudio (version 4.2.3), which includes five MR analysis methods. Of these five methods, inverse variance weighting (IVW) was employed as the main method, with MR Egger regression, weighted median, simple mode, and weighted mode as complementary methods. The IVW method, which ignores the presence of the intercept term in the regression and takes the inverse of the result variance (quadratic of se) as the weight for the fit, assumes that all of the SNPs turned out to be valid instrumental variables and are completely independent of each other (17). MR Eggar regression accounts for the existence of an intercept term. It assumes that the instrument-exposure and instrument-outcome associations are independent—that is, the instrument strength independent of the assumption of direct effect (18). The weighted median method requires a minimum of 50% of the IVs to be valid to obtain a robust estimate; this method tolerates more invalid IVs (19). Further, inflammatory cytokines that were ultimately identified as statistically significant were subject to the following conditions: The IVW results revealed that the 95% confidence intervals (CI) did not cross over with 1 (or 0), p < 0.05, and met the consistent trend of inflammatory cytokine effects derived from the five MR analyses.
We employed three sensitivity analysis methods to assess the sensitivity of MR results, including the heterogeneity test, pleiotropy test, and leave-one-out sensitivity test. Cochran’s Q test and Rucker’s Q test were used to detect the heterogeneity, and p > 0.05 was regarded as no heterogeneity. The intercept of the MR Eggar analysis results were used for testing the horizontal pleiotropy, with p > 0.05 considered as no pleiotropy (20). Additionally, the MR PRESSO method is capable of outlier identification and horizontal pleiotropy detection simultaneously (21). When applying MR PRESSO analysis, the NbDistribution parameter refers to the number of simulations calculated, and we set this parameter to 1000. Leave-one-out sensitivity was used to investigate whether the causal association was influenced by an SNP between exposure and outcome (22). Further, this study was not pre-registered on any platform.
3 Results
In this two-sample MR study, we investigated the correlation of each of the 4 types of HDP with 41 biomarkers—including interleukins, growth factors, and chemokines— thereby demonstrating that the inflammatory cytokines variably impact each disease type. Interleukin-9 (IL-9), macrophage migration inhibitory factor (MIF), MIP1b, interleukin-13 (IL-13), interleukin-16 (IL-16), and tumor necrosis factor-related apoptosis-inducing ligand (TRAIL) in PE; MIF and vascular endothelial growth factor (VEGF) in GH; interleukin-18 (IL-18), TRAIL, and MIP1b in EH; and IL-9, MIP1b, IL-13 and IL-16 in PF may serve as potential upstream factors for disease when inflammatory cytokines are used as exposures and disease is used as an outcome. In addition, VEGF, interleukin-12p70 (IL-12p70), interleukin-1 beta (IL-1b), interleukin-1 receptor antagonist (IL-1ra), interleukin-4 (IL4), interleukin-7 (IL-7), interleukin-8 (IL-8), macrophage colony stimulating factor (MCSF) in PE and macrophage inflammatory protein 1-alpha (MIP1a), hepatocyte growth factor (HGF), IL-18, IL-8 in GH might cause alterations in the expression levels of these cytokines through the pathogenic pathway if the disease type is used as exposure. Further, there is no reciprocal causal association between individual biomarkers and diseases under each disease type. The results of bidirectional MR analysis among the four types of HDP and inflammatory cytokines are presented in Figures 2–5.
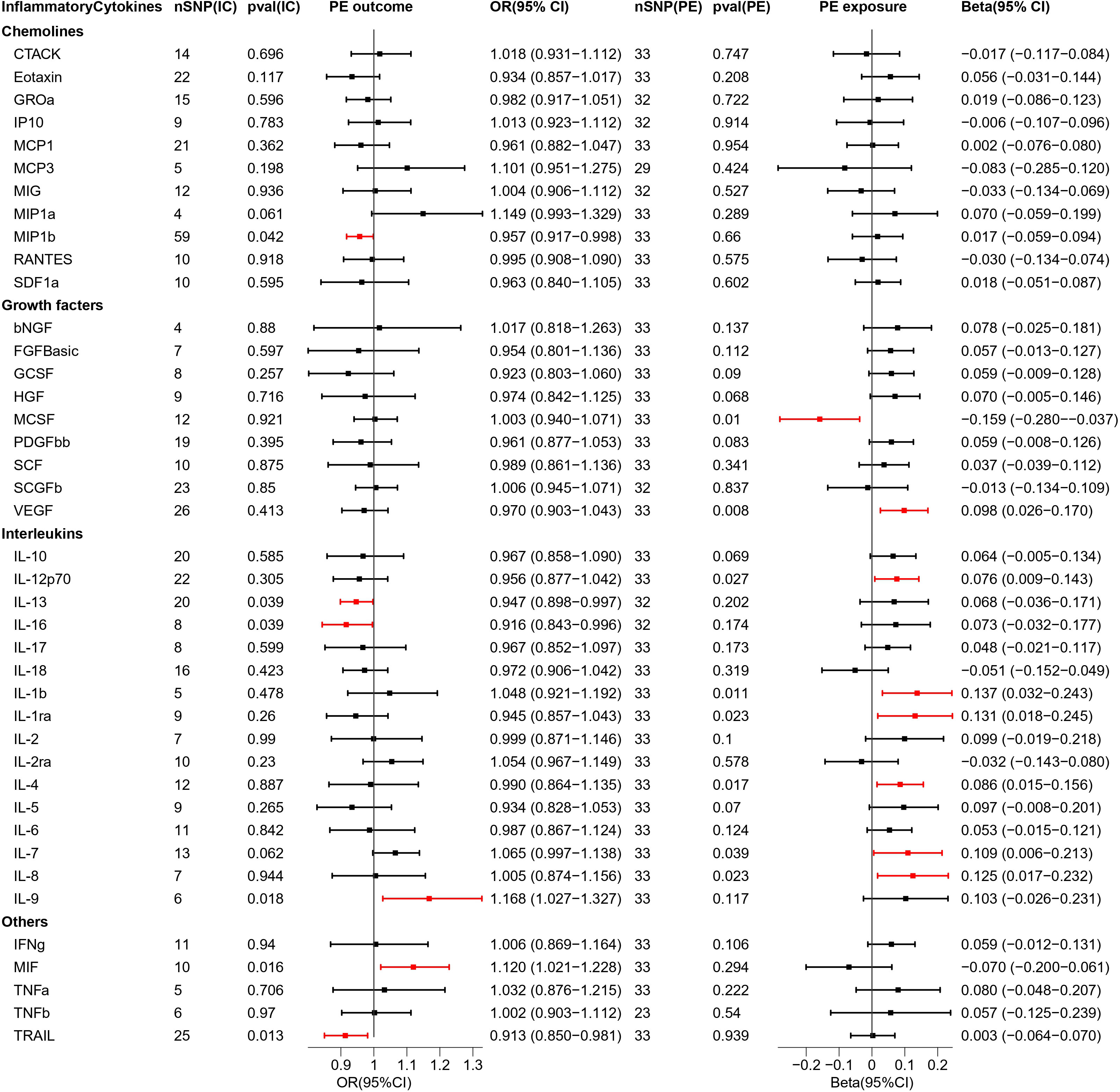
Figure 2 Causal estimations between 41 inflammatory cytokines and PE. The odds ratio (OR) was estimated using the random effect IVW method. The horizontal bars represent 95% confidence intervals (CI). SNP, Single nucleotide polymorphism; IC, Inflammatory Cytokine; pval, P-value; PE, Pre-eclampsia or eclampsia; OR, Odds ratio; CI, Confidence interval; CTACK, Cutaneous T cell-attracting chemokine; bNGF, Basic-nerve growth factor; FGFBasic, Basic fibroblast growth factor; GCSF, Granulocyte colony-stimulating factor; GROa, Gastroesophageal resuscitative occlusion of the aorta; HGF, Hepatocyte growth factor; IFNg, Interferon-gamma; IL-10, Interleukin-10; IL-12p70, Interleukin-12p70; IL-13, Interleukin-13; IL-16, Interleukin-16; IL-17, Interleukin-17; IL-18, Interleukin-18; IL-1b, Interleukin-1 beta; IL-1ra, Interleukin-1 receptor antagonist ; IL-2, Interleukin-2; IL-2ra, Interleukin-2 receptor antagonist; IL-4, Interleukin-4; IL-5, Interleukin-5; IL-6, Interleukin-6; IL-7, Interleukin-7; IL-8, Interleukin-8; IL-9, Interleukin-9; IP10, Interferon gamma-induced protein 10; MCP1, Monocyte chemotactic protein 1; MCP3, Monocyte chemotactic protein 3; MCSF, Macrophage colony stimulating factor; MIF, Macrophage migration inhibitory factor; MIG, Monokine induced by interferon (IFN)-gamma; MIP1a, Macrophage inflammatory protein 1-alpha; MIP1b, Macrophage inflammatory protein 1-beta; PDGFbb, Platelet derived growth factor bb; RANTES, Regulated upon activation normal T cell expressed and presumably secreted; SCF, Stem cell factor; SCGFb, Stem cell growth factor-beta; SDF1a, Stromal cell-derived factor-1alpha; TNFa, Tumor necrosis factor-alpha; TNFb, Tumor necrosis factor-beta; TRAIL, Tumor necrosis factor-related apoptosis-inducing ligand; VEGF, Vascular endothelial growth factor.
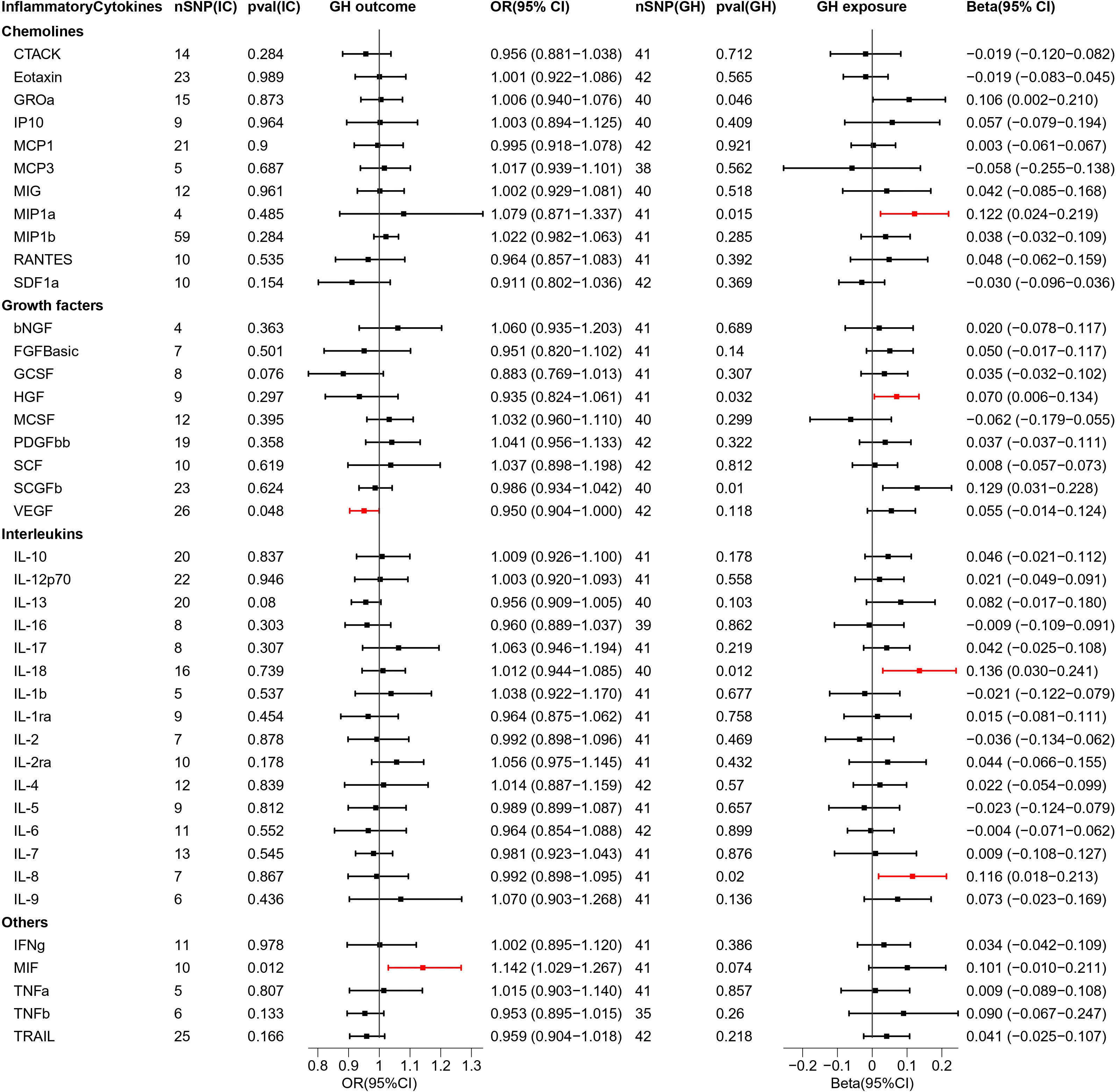
Figure 3 Causal estimations between 41 inflammatory cytokines and GH. The odds ratio (OR) was estimated using the random effect IVW method. The horizontal bars represent 95% confidence intervals (CI). SNP, Single nucleotide polymorphism; IC, Inflammatory Cytokine; pval, P-value; GH, Gestational hypertension; OR, Odds ratio; CI, Confidence interval; CTACK, Cutaneous T cell-attracting chemokine; bNGF, Basic-nerve growth factor; FGFBasic, Basic fibroblast growth factor; GCSF, Granulocyte colony-stimulating factor; GROa, Gastroesophageal resuscitative occlusion of the aorta; HGF, Hepatocyte growth factor; IFNg, Interferon-gamma; IL-10, Interleukin-10; IL-12p70, Interleukin-12p70; IL-13, Interleukin-13; IL-16, Interleukin-16; IL-17, Interleukin-17; IL-18, Interleukin-18; IL-1b, Interleukin-1 beta; IL-1ra, Interleukin-1 receptor antagonist ; IL-2, Interleukin-2; IL-2ra, Interleukin-2 receptor antagonist; IL-4, Interleukin-4; IL-5, Interleukin-5; IL-6, Interleukin-6; IL-7, Interleukin-7; IL-8, Interleukin-8; IL-9, Interleukin-9; IP10, Interferon gamma-induced protein 10; MCP1, Monocyte chemotactic protein 1; MCP3, Monocyte chemotactic protein 3; MCSF, Macrophage colony stimulating factor; MIF, Macrophage migration inhibitory factor; MIG, Monokine induced by interferon (IFN)-gamma; MIP1a, Macrophage inflammatory protein 1-alpha; MIP1b, Macrophage inflammatory protein 1-beta; PDGFbb, Platelet derived growth factor bb; RANTES, Regulated upon activation normal T cell expressed and presumably secreted; SCF, Stem cell factor; SCGFb, Stem cell growth factor-beta; SDF1a, Stromal cell-derived factor-1alpha; TNFa, Tumor necrosis factor-alpha; TNFb, Tumor necrosis factor-beta; TRAIL, Tumor necrosis factor-related apoptosis-inducing ligand; VEGF, Vascular endothelial growth factor.
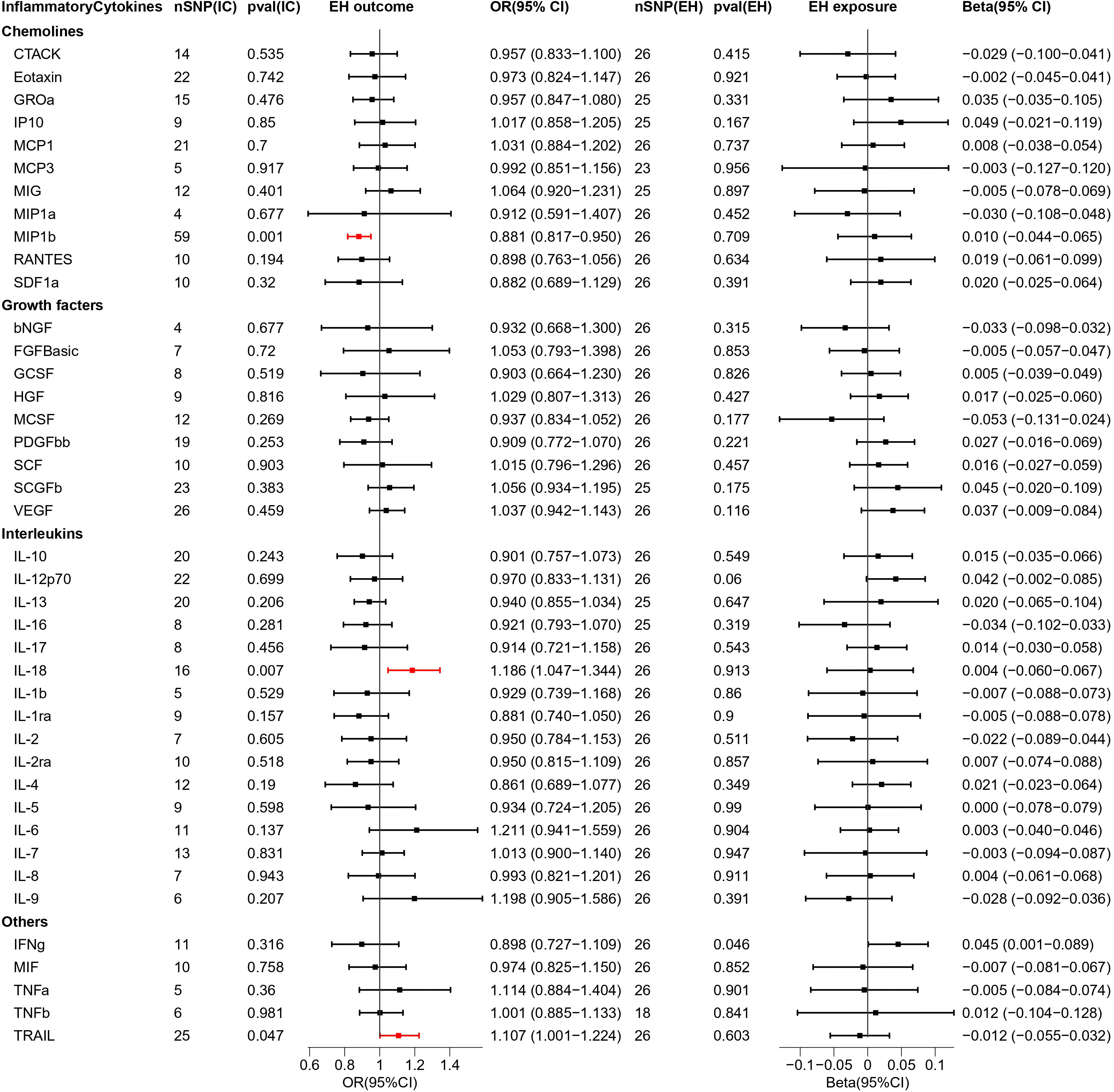
Figure 4 Causal estimations between 41 inflammatory cytokines and EH. The odds ratio (OR) was estimated using the random effect IVW method. The horizontal bars represent 95% confidence intervals (CI). SNP, Single nucleotide polymorphism; IC, Inflammatory Cytokine; pval, P-value; EH, Pre-existing hypertension complicating pregnancy, childbirth and the puerperium; OR, Odds ratio; CI, Confidence interval; CTACK, Cutaneous T cell-attracting chemokine; bNGF, Basic-nerve growth factor; FGFBasic, Basic fibroblast growth factor; GCSF, Granulocyte colony-stimulating factor; GROa, Gastroesophageal resuscitative occlusion of the aorta; HGF, Hepatocyte growth factor; IFNg, Interferon-gamma; IL-10, Interleukin-10; IL-12p70, Interleukin-12p70; IL-13, Interleukin-13; IL-16, Interleukin-16; IL-17, Interleukin-17; IL-18, Interleukin-18; IL-1b, Interleukin-1 beta; IL-1ra, Interleukin-1 receptor antagonist ; IL-2, Interleukin-2; IL-2ra, Interleukin-2 receptor antagonist; IL-4, Interleukin-4; IL-5, Interleukin-5; IL-6, Interleukin-6; IL-7, Interleukin-7; IL-8, Interleukin-8; IL-9, Interleukin-9; IP10, Interferon gamma-induced protein 10; MCP1, Monocyte chemotactic protein 1; MCP3, Monocyte chemotactic protein 3; MCSF, Macrophage colony stimulating factor; MIF, Macrophage migration inhibitory factor; MIG, Monokine induced by interferon (IFN)-gamma; MIP1a, Macrophage inflammatory protein 1-alpha; MIP1b, Macrophage inflammatory protein 1-beta; PDGFbb, Platelet derived growth factor bb; RANTES, Regulated upon activation normal T cell expressed and presumably secreted; SCF, Stem cell factor; SCGFb, Stem cell growth factor-beta; SDF1a, Stromal cell-derived factor-1alpha; TNFa, Tumor necrosis factor-alpha; TNFb, Tumor necrosis factor-beta; TRAIL, Tumor necrosis factor-related apoptosis-inducing ligand; VEGF, Vascular endothelial growth factor.
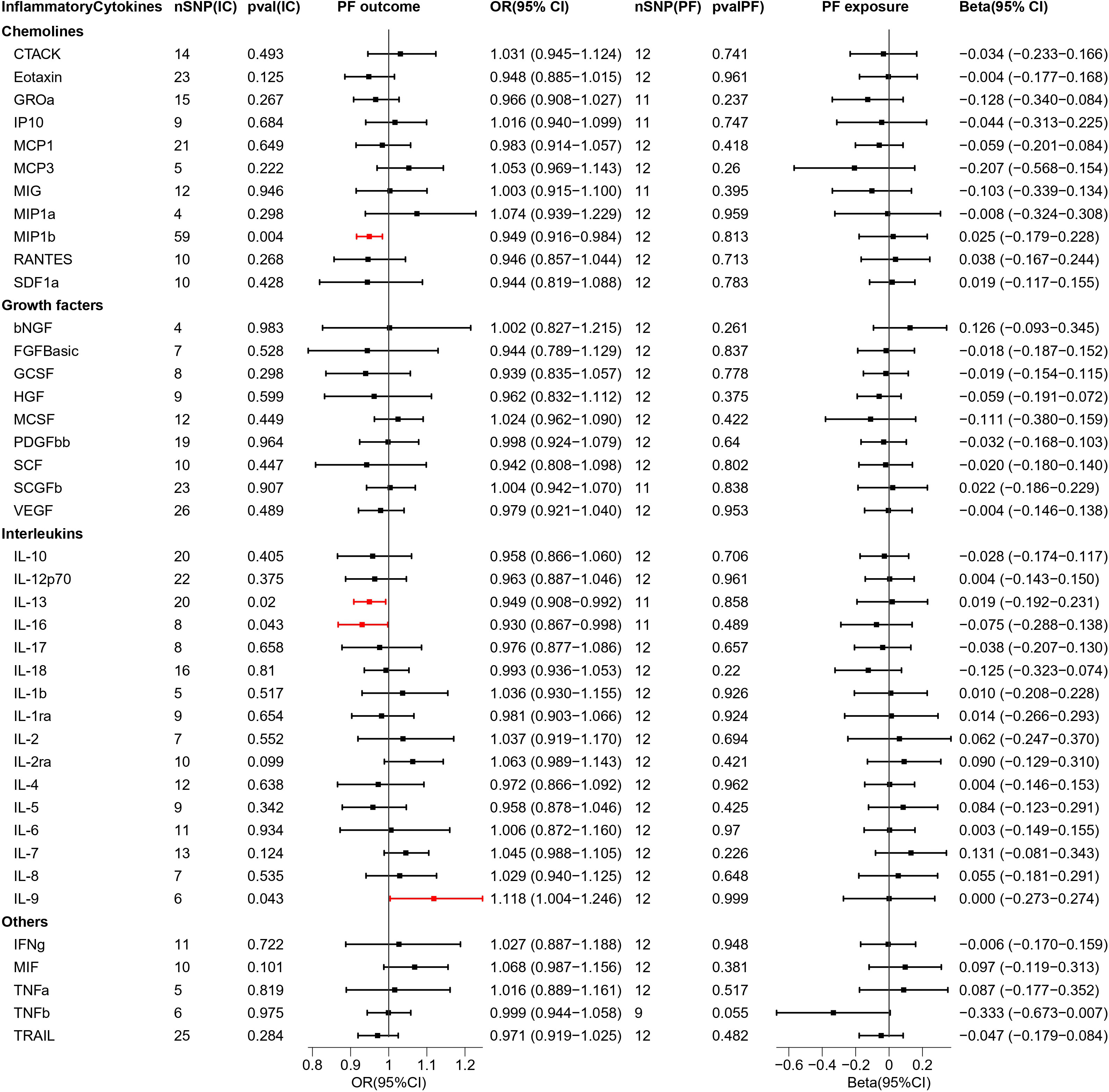
Figure 5 Causal estimations between 41 inflammatory cytokines and PF. The odds ratio (OR) was estimated using the random effect IVW method. The horizontal bars represent 95% confidence intervals (CI). SNP, Single nucleotide polymorphism; IC, Inflammatory Cytokine; pval, P-value; PF, Pre-eclampsia or poor fetal growth; OR, Odds ratio; CI, Confidence interval; CTACK, Cutaneous T cell-attracting chemokine; bNGF, Basic-nerve growth factor; FGFBasic, Basic fibroblast growth factor; GCSF, Granulocyte colony-stimulating factor; GROa, Gastroesophageal resuscitative occlusion of the aorta; HGF, Hepatocyte growth factor; IFNg, Interferon-gamma; IL-10, Interleukin-10; IL-12p70, Interleukin-12p70; IL-13, Interleukin-13; IL-16, Interleukin-16; IL-17, Interleukin-17; IL-18, Interleukin-18; IL-1b, Interleukin-1 beta; IL-1ra, Interleukin-1 receptor antagonist ; IL-2, Interleukin-2; IL-2ra, Interleukin-2 receptor antagonist; IL-4, Interleukin-4; IL-5, Interleukin-5; IL-6, Interleukin-6; IL-7, Interleukin-7; IL-8, Interleukin-8; IL-9, Interleukin-9; IP10, Interferon gamma-induced protein 10; MCP1, Monocyte chemotactic protein 1; MCP3, Monocyte chemotactic protein 3; MCSF, Macrophage colony stimulating factor; MIF, Macrophage migration inhibitory factor; MIG, Monokine induced by interferon (IFN)-gamma; MIP1a, Macrophage inflammatory protein 1-alpha; MIP1b, Macrophage inflammatory protein 1-beta; PDGFbb, Platelet derived growth factor bb; RANTES, Regulated upon activation normal T cell expressed and presumably secreted; SCF, Stem cell factor; SCGFb, Stem cell growth factor-beta; SDF1a, Stromal cell-derived factor-1alpha; TNFa, Tumor necrosis factor-alpha; TNFb, Tumor necrosis factor-beta; TRAIL, Tumor necrosis factor-related apoptosis-inducing ligand; VEGF, Vascular endothelial growth factor.
3.1 Bidirectional interactions between 41 inflammatory cytokines and PE
The results of bidirectional MR analysis of inflammatory cytokines correlated with PE are depicted in Figure 2. When 41 inflammatory cytokines were used as exposures, 6 inflammatory factors were causally associated with the pathogenesis of PE disease, including IL9 (odds ratio (OR) = 1.168, 95%CI = 1.027–1.327, p = 0.018) and MIF (OR = 1.120, 95% CI = 1.021–1.228, p = 0.016) as facilitators, MIP1b (OR = 0.957, 95% CI = 0.917–0.998, p = 0.042), IL13 (OR = 0.947, 95% CI = 0.898–0.997, p = 0.039), IL16 (OR = 0.916, 95% CI = 0.843–0.996, p = 0.039), and TRAIL (OR = 0.913, 95% CI = 0.850–0.981, p = 0.013) playing inhibitory roles. Scatter plots were used to demonstrate the trends of inflammatory cytokines under the five MR methods (Figure S1).
When PE was used as exposure, the remaining 33 SNPs were involved in the MR analysis. The results are depicted in Figure 2. Eight inflammatory cytokines were affected by PE, among which the growth factor MCSF (Beta = -0.159, 95% CI= -0.280–0.037, p = 0.01) was negatively regulated, while the others were positively regulated. The remaining seven inflammatory factors were VEGF (Beta = 0.098, 95% CI = 0.026–0.170, p = 0.008), IL12p70 (Beta = 0.076, 95% CI = 0.009–0.143, p = 0.027), IL1b (Beta = 0.137, 95% CI = 0.032–0.243, p = 0.011), IL1ra (Beta = 0.131, 95% CI = 0.018–0.245, p = 0.023), IL4 (Beta = 0.086, 95% CI = 0.015–0.156, p = 0.017), IL7 (Beta = 0.109, 95% CI = 0.006–0.213, p = 0.039), IL8 (Beta = 0.125, 95% CI = 0.017–0.232, p = 0.023). The scatter plot of the above inflammatory cytokines is presented in Figure S2.
3.2 Bidirectional interactions between 41 inflammatory cytokines and GH
As 41 inflammatory cytokines were used for MR analysis as exposure, MIF (OR = 1.142, 95% CI = 1.029–1.267, p = 0.012), which acted as a promoter, and VEGF (OR = 0.950, 95% CI = 0.904–0.9995, p = 0.048), which acted as an inhibitor, were identified. The scatter plot is depicted in Figure S3.
Next, we reversed the exposure and outcome. MR analysis revealed that four inflammatory cytokines were screened out and all of them had increased expression under the influence of GH. The four cytokines are MIP1a (Beta = 0.122,95% CI = 0.024–0.219, p = 0.015) HGF (Beta = 0.070, 95% CI = 0.006–0.134, p = 0.032), IL-18 (Beta = 0.136, 95% CI = 0.030–0.241, p = 0.012), and IL-8 (Beta = 0.116, 95% CI = 0.018–0.213, p = 0.02). The scatter plot is depicted in Figure S4. In addition, the 95% CIs for GROa and SCGFb did not pass through 0 (Figure 3). The beta values for MR Eggar were in the opposite direction as that of IVW; thus, GROa (IVW beta = 0.106, MR Eggar beta = -0.143) and SCGFb (IVW beta = 0.129, MR Eggar Beta = -0.263) were not considered as statistically significant cytokines.
3.3 Bidirectional interactions between 41 inflammatory cytokines and EH
We explored the causal association of 41 inflammatory cytokines with EH as outcome and obtained three inflammatory cytokines with causal potential—IL-18 (OR = 1.186, 95% CI = 1.047–1.344, p = 0.007) and TRAIL (OR = 1.107, 95% CI = 1.001–1.224, p = 0.047) factors produced positive regulatory effects, while MIP1b (OR = 0.881, 95% CI = 0.817–0.950, p = 0.001) factor exerted negative effects. The scatter plot is depicted in Figure S5.
When exploring the MR analysis of the role of EH as exposure, only the IFNg was revealed as a statistically significant responder by the IVW method. However, the MR Eggar method obtained results with the opposite trend to that of the former; thus, the IFNg (IVW beta = 0.045, MR Eggar beta = -0.004) was eliminated.
3.4 Bidirectional interactions between 41 inflammatory cytokines and PF
When MR analysis was performed with 41 inflammatory cytokines as exposure and PF as outcome, one facilitator, IL-9 (OR = 1.118, 95% CI = 1.004–1.246, p = 0.043), and three inhibitory factors, MIP1b (OR = 0.949, 95% CI = 0.916–0.984, p = 0.004), IL-13 (OR = 0.949, 95% CI = 0.908–0.992, p = 0.02), and IL-16 (OR = 0.930, 95% CI = 0.867–0.998, p = 0.043) were found. The scatterplot is presented in Figure S6.
Reversing exposure and outcome, no significant inflammatory cytokines were found.
3.5 Sensitivity analysis
The results of the tests for heterogeneity and pleiotropy when inflammatory cytokines were used as exposures are presented in Table S2. The results of the tests for heterogeneity and pleiotropy when inflammatory cytokines were used as outcomes are presented in Table S4. In addition, the leave-one-out analysis results are presented in Figure S7-12. Details of SNPs when inflammatory cytokines were used as exposures are presented in Table S3. Details of SNPs when inflammatory cytokines were used as outcomes are presented in Table S5.
4 Discussion
Recently, numerous studies that explore the association of HDP with the immune system and the inflammatory cytokines produced by immune cells have been published. For example, TH17 cells and their secreted interleukin-17 (IL-17) levels are found to be increased in PE patients; TH17 cells induce pre-eclampsia by participating in placental ischemia, oxidative stress, and other pathways (23). It has also been suggested that VEGF may be an important biomarker for pre-eclampsia and has significant value in predicting its severity (24, 25). Despite the rich results of previous observational studies, the causal association among numerous important inflammatory cytokines with HDP has not been established due to the limitations of traditional epidemiology (26). Observational studies often provide biased associations due to the presence of confounding factors or reverse causality, thereby making it difficult to obtain credible conclusions using this method (27). In terms of pregnancy disorders, it is difficult to identify the causes of changes in inflammatory cytokine levels in patients with HDP through general observational studies. The presence of the disease itself, side effects caused by therapeutic drugs, underlying pathological immune response, the state of hidden infection, and harmful lifestyle habits (such as alcohol abuse and smoking) can cause changes in the levels of inflammatory cytokines (28–30). Recently, it has been reported that cytokine storms are highlighted as a common feature in pre-eclampsia and severe forms of coronavirus disease 2019 disease (31).
Our study found that more inflammatory cytokines exhibited significant changes in the initiation and progression of PE compared to GH. Among the four types of HDP, the inflammatory cytokines that recurrently appear upstream of the disease are IL-9, MIF, MIP1b, IL-13, IL-16, and TRAIL. The IL-9/IL-9R pathway is involved in regulating trophectoderm function, and increased IL-9 levels were found to result in increased tissue proliferation and invasiveness (32). IL-9 and IL-9R increase fibroblast proliferation as well as induce pro-inflammatory cytokines and metalloproteinases, which are key pathological processes that promote the formation of vascular opacities (33). Prior studies have suggested that Th9 cells may be involved in immune-mediated diseases such as allergy and autoimmune inflammatory diseases (34). Th1 or Th17 cells can induce CD4+IL-9+ T cell differentiation by promoting factors such as IL-1b and IL-12 (35). Under condition of TGF-β presence, Th-17 cells can produce IL-9 to participate in pro-inflammatory response (36). At present, research on Th9 cells in human pregnancy is limited, and the possible role of Th9 cells in preeclampsia needs to be further investigated. In addition, MIF is involved in multiple biophysiological processes that contribute to cell proliferation and differentiation, innate immune responses, and angiogenic biological activities (37). Previous studies have confirmed that large amounts of pro-inflammatory mediators are produced by MIF stimulation, including cytokines tumor necrosis factor-alpha (TNFa), IL-1b, interleukin-6 (IL-6), interferon-gamma (IFNg), matrix metalloproteinases, and nitric oxide (38, 39). Circulating concentrations of MIF are elevated in infected patients, inflammatory conditions, and autoimmune diseases (40). Therefore, MIF is recognized as a biomarker or pharmacological target for different diseases (41, 42), and MIF inhibitors might have considerable therapeutic benefit for numerous inflammatory and autoimmune diseases (43–46). Similar conditions have been found in mothers with pathological pregnancies (preterm birth (47) and pre-eclampsia (48). MIP1b was revealed to have the potential to recruit macrophages and dendritic cells (49, 50), which have the ability to secrete a range of cytokines/chemokines and enzymes implicated in angiogenesis and tissue remodeling (51). Furthermore, it has been demonstrated that macrophages promote trophoblastic invasion in pregnant mice (52). IL-13 exerts its anti-inflammatory effects by binding to IL-4 receptor alpha (IL-4ra) (53). In addition, IL-13 can downregulate the expression of IL-1b, IL-8, TNFa, and MIP1a (54–56). The anti-inflammatory potential of IL-13 has been therapeutically investigated in a variety of pathologies, including psoriasis, arthritis, and Alzheimer’s disease (57–59). Therefore, IL13 may be a key therapeutic target in pre-eclampsia. Currently, there are very few studies that investigate the correlation between IL-16 and HDP. It has been shown that IL-16 can initiate and amplify inflammatory responses through the release of cell signaling molecules that bind to CD4+ T cells (60). It is worth noting that Andrea L et al. found a noticeable decrease of IL-16 expression in hemolysis, elevated liver enzymes, and low platelet count (HELLP) syndrome (61). Due to the lack of clarity regarding how IL-16 is implicated in the etiopathogenesis of pre-eclampsia suggests, it is noteworthy that future research on IL-16 in such disorders is necessary. Tumor necrosis factor-related apoptosis-inducing ligand (TRAIL) is part of the TNF ligand family, and TRAIL and its receptor play essential roles in trophoblastic immunity and invasion during early pregnancy (62, 63). TRAIL induces apoptosis in vascular endothelial cells and vascular smooth muscle cells during the process of remodeling the uterine spiral arteries (64). It has recently been demonstrated that pregnant women who subsequently develop HDP have reduced plasma levels of TRAIL prior to 20 weeks gestation (65), thereby suggesting that TRAIL could serve as a new predictive, noninvasive biomarker for pregnant women with HDP.
Further, previous studies have revealed that IL-12p70, IL-1ra, IL-1b, IL-8, and IL-18 are considerably higher in PE group than in normal pregnant women (66, 67), which supports our findings. IL-1ra is elevated in PE, and elevated circulating IL-1ra reflect increased activity of the pro-inflammatory cytokines IL-1a and IL-1b, both of which are difficult to identify in serum levels because of their short circulating half-lives (68, 69). Moreover, it has been revealed that IL-1b and TNFa can significantly increase MCSF expression in early pregnancy decidual cells (70). IL-7 cytokine is necessary for the proliferation and survival of pathogenic Th17 cells, and has a promotional role in autoimmune diseases such as experimental autoimmune encephalomyelitis (71, 72).. In addition, previous evidence suggests that Th17 cells are observed to be increased in the decidual cells of patients with pre-eclampsia (73). IL-17 is a major effector molecule of Th17 cells, which plays an important role in inflammatory response, and IL-17 also induces the production of factors such as IL-6 and IL-8. However, IL-17 was not a statistically significant factor in the results of this MR analysis. In a meta-analysis published in 2020, the authors found no evidence that circulating IL-17 levels differed between pre-eclampsia and controls (74). Furthermore, Brewster et al. found that IL-17 levels were lower in patients with early-onset pre-eclampsia and higher in patients with late-onset compared to controls (75). Consequently, we consider that the role of IL-17 in pre-eclampsia needs to be approached with caution and further studies are still needed. Karol Charkiewicz et al. demonstrated that HGF was expressed in the plasma of patients with PE at a higher level than that in healthy pregnant women (76).
Currently, the most effective way to deal with pre-eclampsia is the prompt delivery of the fetus and placenta (77). Symptoms of pre-eclampsia and eclampsia are usually greatly relieved when the fetus and placental tissue are delivered from the mother. In our study, circulating inflammatory cytokines were also no longer active in patients after delivery. Moreover, we found that IL-9, MIP1b, IL-13, and IL-16 might be associated with fetal dysplasia. Previous researches have suggested that IL-9 induces immune tolerance during pregnancy; if abnormalities occur, the fetus is no longer tolerated by the mother and has adverse outcomes for both the mother and fetus (78). MIP1b affects cytotoxicity and causes tissue damage in the developing fetal brain and is a potential mechanism for intrauterine fetal dysplasia (79). Fetal growth restriction (FGR) maternal IL-13 levels are lower than those in normal pregnancies (80). IL-13 is a Th2 cytokine with anti-inflammatory characteristics. IL-13 inhibits the production of IL-6, IL-8, TNFa, and IL-12 as well as suppresses cytotoxicity and blocks pathologic inflammation (81). Lower levels of IL13 in FGR may indicate a more pronounced Th1 bias or pro-inflammatory cytokine bias. Denihan et al. investigated that IL-16 participated in the process of fetal brain injury and that its level associated with the long-term prognosis of neonatal hypoxic-ischemic encephalopathy (82).
For the first time, we adopted bidirectional two-sample MR analysis to explore the causal association between circulating inflammatory cytokines and the four types of HDP. Additionally, attention has been focused on inflammatory cytokines in pre-eclampsia that may contribute to poor fetal growth and development. Prior literature reviewed upstream and downstream inflammatory cytokines in HDP, thereby providing strong evidence for our results. Our study obtained different inflammatory cytokine profiles for both the incidence and progression of the disease, which offers new directions in the prevention, surveillance, and treatment of HDP. The pathogenesis and pathophysiology of HDP, represented by pre-eclampsia, are very complex and multifactorial. This includes endothelial dysfunction, oxidative stress, angiogenesis, neutrophil activation, anti-angiogenic factors, and syncytiotrophoblast microparticles, which are all involved in the pathophysiologic process of pre-eclampsia. Inflammatory cytokines play an important role in disease progression, but not all. This study only included 41 inflammatory cytokines, which is obviously a limitation. With in-depth study of HDP, more cytokines will be explored by researchers in the future and bring new perspectives and insights for early detection and therapeutic strategies of HDP.
There are several strengths of this study. First, genetic variation is randomly distributed during gamete formation and conception; thus, MR analysis avoids the limitations of observational studies (confounding, regression dilution, and reverse causation bias) (11). Second, we used separate samples for the circulating inflammatory cytokines and HDP data, and two-sample data avoided bias from weak instrumental variables. Third, we included two large-scale cohorts were included for MR analysis, thereby applying a sufficiently large sample size to ensure generalizability of causality. Moreover, two sets of data were treated as exposure and outcome to perform bidirectional MR analysis, respectively. Finally, so as to test the validity of the IV hypotheses, we performed sensitivity analyses, which included heterogeneity, pleiotropy, and leave-one-out analyses.
Nevertheless, there are also a few limitations of this study that must be discussed. First, the number of IVs as exposure varied for each inflammatory cytokine. During our analysis, a few IVs were discarded after screening; thus, the final MR analysis results could be influenced by the limited number of IVs. Despite this, we calculated statistical efficacy for each IV and excluded IVs with low efficacies in order to ensure that the results presented in this study remain reliable. Second, HDP occurs only in the female population, whereas circulating inflammatory cytokine GWASs are found in both male and female populations; thus, the presence of gender differences could potentially impact MR results. Third, this research data pertains to European populations; therefore, it needs to be treated with caution when generalizing to other populations. Finally, the immune system in pregnancy is complex and variable, with multiple changes in inflammatory cytokines influenced by a variety of factors, whereas it is difficult to dynamically monitor such a complex process in our study. Early-onset preeclampsia (onset < 34 weeks of gestation) and late-onset preeclampsia (onset ≥ 34 weeks of gestation), have different pathophysiological and immunopathological origins. However, limited by the availability of GWAS data, this study did not conduct subgroup analyses. With regard to the causal association of circulating inflammatory cytokines with HDP, deeper and more extensive studies are required in the future.
Data availability statement
The datasets presented in this study can be found in online repositories. The names of the repository/repositories and accession number(s) can be found in the article/Supplementary Material.
Author contributions
SG: Data curation, Formal Analysis, Investigation, Methodology, Project administration, Resources, Visualization, Writing – original draft, Writing – review & editing. XB: Conceptualization, Investigation, Methodology, Project administration, Resources, Supervision, Visualization, Writing – original draft, Writing – review & editing. JD: Formal Analysis, Investigation, Validation, Visualization, Writing – original draft, Writing – review & editing. RZ: Conceptualization, Investigation, Project administration, Resources, Supervision, Writing – original draft, Writing – review & editing.
Funding
The author(s) declare financial support was received for the research, authorship, and/or publication of this article. This study was funded by Wu Jieping Medical Foundation (Grant No. 320.6750.2020-06-83).
Acknowledgments
We sincerely thank the FinnGen database and Ari V. Aholai et al. and other related researchers for providing GWASs summary data.
Conflict of interest
The authors declare that the research was conducted in the absence of any commercial or financial relationships that could be construed as a potential conflict of interest.
Publisher’s note
All claims expressed in this article are solely those of the authors and do not necessarily represent those of their affiliated organizations, or those of the publisher, the editors and the reviewers. Any product that may be evaluated in this article, or claim that may be made by its manufacturer, is not guaranteed or endorsed by the publisher.
Supplementary material
The Supplementary Material for this article can be found online at: https://www.frontiersin.org/articles/10.3389/fimmu.2023.1297929/full#supplementary-material
References
1. Garovic VD, Dechend R, Easterling T, Karumanchi SA, McMurtry Baird S, Magee LA, et al. Hypertension in pregnancy: diagnosis, blood pressure goals, and pharmacotherapy: A scientific statement from the American heart association. Hypertension (Dallas Tex: 1979) (2022) 79(2):e21–41. doi: 10.1161/HYP.0000000000000208
2. GBD 2015 Maternal Mortality Collaborators. Global, regional, and national levels of maternal mortality, 1990-2015: a systematic analysis for the Global Burden of Disease Study 2015 Vol. 388. London, England: Lancet (2016) p. 1775–812.
3. Bakker R, Steegers EA, Hofman A, Jaddoe VW. Blood pressure in different gestational trimesters, fetal growth, and the risk of adverse birth outcomes: the generation R study. Am J Epidemiol (2011) 174(7):797–806. doi: 10.1093/aje/kwr151
4. Staff AC, Benton SJ, von Dadelszen P, Roberts JM, Taylor RN, Powers RW, et al. Redefining preeclampsia using placenta-derived biomarkers. Hypertension (Dallas Tex: 1979) (2013) 61(5):932–42. doi: 10.1161/HYPERTENSIONAHA.111.00250
5. Garovic VD. The role of the podocyte in preeclampsia. Clin J Am Soc Nephrol: CJASN (2014) 9(8):1337–40. doi: 10.2215/CJN.05940614
6. Lyall F, Robson SC, Bulmer JN. Spiral artery remodeling and trophoblast invasion in preeclampsia and fetal growth restriction: relationship to clinical outcome. Hypertension (Dallas Tex: 1979) (2013) 62(6):1046–54. doi: 10.1161/HYPERTENSIONAHA.113.01892
7. Pierik E, Prins JR, van Goor H, Dekker GA, Daha MR, Seelen MAJ, et al. Dysregulation of complement activation and placental dysfunction: A potential target to treat preeclampsia? Front Immunol (2019) 10:3098. doi: 10.3389/fimmu.2019.03098
8. Hart PMB, Stephenson NL, Scime NV, Tough SC, Slater DM, Chaput KH. Second trimester cytokine profiles associated with gestational diabetes and hypertensive disorders of pregnancy. PloS One (2022) 17(12):e0279072. doi: 10.1371/journal.pone.0279072
9. Wang Y, Shi D, Chen L. Lipid profile and cytokines in hypertension of pregnancy: A comparison of preeclampsia therapies. J Clin Hypertens (Greenwich Conn) (2018) 20(2):394–9. doi: 10.1111/jch.13161
10. Burgess S, Butterworth A, Thompson SG. Mendelian randomization analysis with multiple genetic variants using summarized data. Genet Epidemiol (2013) 37(7):658–65. doi: 10.1002/gepi.21758
11. Verduijn M, Siegerink B, Jager KJ, Zoccali C, Dekker FW. Mendelian randomization: use of genetics to enable causal inference in observational studies. Nephrol Dial Transplant (2010) 25(5):1394–8. doi: 10.1093/ndt/gfq098
12. Smith GD, Lawlor DA, Harbord R, Timpson N, Day I, Ebrahim S. Clustered environments and randomized genes: a fundamental distinction between conventional and genetic epidemiology. PloS Med (2007) 4(12):e352. doi: 10.1371/journal.pmed.0040352
13. Smith GD, Ebrahim S. Mendelian randomization: prospects, potentials, and limitations. Int J Epidemiol (2004) 33(1):30–42. doi: 10.1093/ije/dyh132
14. Emdin CA, Khera AV, Kathiresan S. Mendelian randomization. Jama (2017) 318(19):1925–6. doi: 10.1001/jama.2017.17219
15. Skrivankova VW, Richmond RC, Woolf BAR, Yarmolinsky J, Davies NM, Swanson SA, et al. Strengthening the reporting of observational studies in epidemiology using Mendelian randomization: the STROBE-MR statement. Jama (2021) 326(16):1614–21. doi: 10.1001/jama.2021.18236
16. Ahola-Olli AV, Würtz P, Havulinna AS, Aalto K, Pitkänen N, Lehtimäki T, et al. Genome-wide association study identifies 27 loci influencing concentrations of circulating cytokines and growth factors. Am J Hum Genet (2017) 100(1):40–50. doi: 10.1016/j.ajhg.2016.11.007
17. Lee YH. Causal association between smoking behavior and the decreased risk of osteoarthritis: a Mendelian randomization. Z fur Rheumatol (2019) 78(5):461–6. doi: 10.1007/s00393-018-0505-7
18. Bowden J. Misconceptions on the use of MR-Egger regression and the evaluation of the InSIDE assumption. Int J Epidemiol (2017) 46(6):2097–9. doi: 10.1093/ije/dyx192
19. Li C, Niu M, Guo Z, Liu P, Zheng Y, Liu D, et al. A mild causal relationship between tea consumption and obesity in general population: A two-sample Mendelian randomization study. Front Genet (2022) 13:795049. doi: 10.3389/fgene.2022.795049
20. Burgess S, Thompson SG. Interpreting findings from Mendelian randomization using the MR-Egger method. Eur J Epidemiol (2017) 32(5):377–89. doi: 10.1007/s10654-017-0255-x
21. Verbanck M, Chen CY, Neale B, Do R. Detection of widespread horizontal pleiotropy in causal relationships inferred from Mendelian randomization between complex traits and diseases. Nat Genet (2018) 50(5):693–8. doi: 10.1038/s41588-018-0099-7
22. Hemani G, Zheng J, Elsworth B, Wade KH, Haberland V, Baird D, et al. The MR-Base platform supports systematic causal inference across the human phenome. eLife (2018) 7. doi: 10.7554/eLife.34408
23. Cornelius DC, Hogg JP, Scott J, Wallace K, Herse F, Moseley J, et al. Administration of interleukin-17 soluble receptor C suppresses TH17 cells, oxidative stress, and hypertension in response to placental ischemia during pregnancy. Hypertension (Dallas Tex: 1979) (2013) 62(6):1068–73. doi: 10.1161/HYPERTENSIONAHA.113.01514
24. Kurtoglu E, Avci B, Kokcu A, Celik H, Cengiz Dura M, Malatyalioglu E, et al. Serum VEGF and PGF may be significant markers in prediction of severity of preeclampsia. J Maternal fetal Neonatal Med (2016) 29(12):1987–92. doi: 10.3109/14767058.2015.1072157
25. Deshpande JS, Sundrani DP, Sahay AS, Gupte SA, Joshi SR. Unravelling the potential of angiogenic factors for the early prediction of preeclampsia. Hypertens Res (2021) 44(7):756–69. doi: 10.1038/s41440-021-00647-9
26. Davey Smith G, Hemani G. Mendelian randomization: genetic anchors for causal inference in epidemiological studies. Hum Mol Genet (2014) 23(R1):R89–98. doi: 10.1093/hmg/ddu328
27. Sekula P, Del Greco MF, Pattaro C, Köttgen A. Mendelian randomization as an approach to assess causality using observational data. J Am Soc Nephrol: JASN. (2016) 27(11):3253–65. doi: 10.1681/ASN.2016010098
28. Sayad B, Mohseni Afshar Z, Mansouri F, Salimi M, Miladi R, Rahimi S, et al. Pregnancy, preeclampsia, and COVID-19: susceptibility and mechanisms: A review study. Int J Fertil Steril (2022) 16(2):64–9. doi: 10.22074/IJFS.2022.539768.1194
29. Gaydos J, McNally A, Guo R, Vandivier RW, Simonian PL, Burnham EL. Alcohol abuse and smoking alter inflammatory mediator production by pulmonary and systemic immune cells. Am J Physiol Lung Cell Mol Physiol (2016) 310(6):L507–18. doi: 10.1152/ajplung.00242.2015
30. Deer E, Herrock O, Campbell N, Cornelius D, Fitzgerald S, Amaral LM, et al. The role of immune cells and mediators in preeclampsia. Nat Rev Nephrol (2023) 19(4):257–70. doi: 10.1038/s41581-022-00670-0
31. Todros T, Masturzo B, De Francia S. COVID-19 infection: ACE2, pregnancy and preeclampsia. Eur J Obstet Gynecol Reprod Biol (2020) 253:330. doi: 10.1016/j.ejogrb.2020.08.007
32. Sun Y, Liu S, Hu R, Zhou Q, Li X. Decreased placental IL9 and IL9R in preeclampsia impair trophoblast cell proliferation, invasion, and angiogenesis. Hypertens Pregnancy (2020) 39(3):228–35. doi: 10.1080/10641955.2020.1754852
33. Raychaudhuri SK, Abria C, Maverakis EM, Raychaudhuri SP. IL-9 receptor: Regulatory role on FLS and pannus formation. Cytokine (2018) 111:58–62. doi: 10.1016/j.cyto.2018.08.001
34. Kaplan MH. Th9 cells: differentiation and disease. Immunol Rev (2013) 252(1):104–15. doi: 10.1111/imr.12028
35. Wang W, Sung N, Gilman-Sachs A, Kwak-Kim J. T helper (Th) cell profiles in pregnancy and recurrent pregnancy losses: Th1/Th2/Th9/Th17/Th22/Tfh cells. Front Immunol (2020) 11:2025. doi: 10.3389/fimmu.2020.02025
36. Nowak EC, Weaver CT, Turner H, Begum-Haque S, Becher B, Schreiner B, et al. IL-9 as a mediator of Th17-driven inflammatory disease. J Exp Med (2009) 206(8):1653–60. doi: 10.1084/jem.20090246
37. Harris J, VanPatten S, Deen NS, Al-Abed Y, Morand EF. Rediscovering MIF: new tricks for an old cytokine. Trends Immunol (2019) 40(5):447–62. doi: 10.1016/j.it.2019.03.002
38. Calandra T, Roger T. Macrophage migration inhibitory factor: a regulator of innate immunity. Nat Rev Immunol (2003) 3(10):791–800. doi: 10.1038/nri1200
39. Bucala R, Donnelly SC. Macrophage migration inhibitory factor: a probable link between inflammation and cancer. Immunity (2007) 26(3):281–5. doi: 10.1016/j.immuni.2007.03.005
40. Bilsborrow JB, Doherty E, Tilstam PV, Bucala R. Macrophage migration inhibitory factor (MIF) as a therapeutic target for rheumatoid arthritis and systemic lupus erythematosus. Expert Opin Ther Targets (2019) 23(9):733–44. doi: 10.1080/14728222.2019.1656718
41. Grieb G, Merk M, Bernhagen J, Bucala R. Macrophage migration inhibitory factor (MIF): a promising biomarker. Drug News Perspect (2010) 23(4):257–64. doi: 10.1358/dnp.2010.23.4.1453629
42. Hertelendy J, Reumuth G, Simons D, Stoppe C, Kim BS, Stromps JP, et al. Macrophage migration inhibitory factor - A favorable marker in inflammatory diseases? Curr Medicinal Chem (2018) 25(5):601–5. doi: 10.2174/0929867324666170714114200
43. Mikulowska A, Metz CN, Bucala R, Holmdahl R. Macrophage migration inhibitory factor is involved in the pathogenesis of collagen type II-induced arthritis in mice. J Immunol (Baltimore Md: 1950) (1997) 158(11):5514–7. doi: 10.4049/jimmunol.158.11.5514
44. Calandra T, Echtenacher B, Roy DL, Pugin J, Metz CN, Hültner L, et al. Protection from septic shock by neutralization of macrophage migration inhibitory factor. Nat Med (2000) 6(2):164–70. doi: 10.1038/72262
45. Amano T, Nishihira J, Miki I. Blockade of macrophage migration inhibitory factor (MIF) prevents the antigen-induced response in a murine model of allergic airway inflammation. Inflammation Res (2007) 56(1):24–31. doi: 10.1007/s00011-007-5184-9
46. Cavalli E, Ciurleo R, Petralia MC, Fagone P, Bella R, Mangano K, et al. Emerging role of the macrophage migration inhibitory factor family of cytokines in neuroblastoma. Pathogenic effectors and novel therapeutic targets? Mol (Basel Switzerland) (2020) 25(5). doi: 10.3390/molecules25051194
47. Pearce BD, Garvin SE, Grove J, Bonney EA, Dudley DJ, Schendel DE, et al. Serum macrophage migration inhibitory factor in the prediction of preterm delivery. Am J Obstet Gynecol (2008) 199(1):46.e1–6. doi: 10.1016/j.ajog.2007.11.066
48. Todros T, Bontempo S, Piccoli E, Ietta F, Romagnoli R, Biolcati M, et al. Increased levels of macrophage migration inhibitory factor (MIF) in preeclampsia. Eur J Obstet Gynecol Reprod Biol (2005) 123(2):162–6. doi: 10.1016/j.ejogrb.2005.03.014
49. Chiba K, Zhao W, Chen J, Wang J, Cui HY, Kawakami H, et al. Neutrophils secrete MIP-1 beta after adhesion to laminin contained in basement membrane of blood vessels. Br J Haematol (2004) 127(5):592–7. doi: 10.1111/j.1365-2141.2004.05242.x
50. Cheung R, Malik M, Ravyn V, Tomkowicz B, Ptasznik A, Collman RG. An arrestin-dependent multi-kinase signaling complex mediates MIP-1beta/CCL4 signaling and chemotaxis of primary human macrophages. J Leuk Biol (2009) 86(4):833–45. doi: 10.1189/jlb.0908551
51. David Dong ZM, Aplin AC, Nicosia RF. Regulation of angiogenesis by macrophages, dendritic cells, and circulating myelomonocytic cells. Curr Pharm Design (2009) 15(4):365–79. doi: 10.2174/138161209787315783
52. Abrahams VM, Kim YM, Straszewski SL, Romero R, Mor G. Macrophages and apoptotic cell clearance during pregnancy. Am J Reprod Immunol (New York NY: 1989) (2004) 51(4):275–82. doi: 10.1111/j.1600-0897.2004.00156.x
53. Jiang H, Harris MB, Rothman P. IL-4/IL-13 signaling beyond JAK/STAT. J Allergy Clin Immunol (2000) 105(6 Pt 1):1063–70. doi: 10.1067/mai.2000.107604
54. Herbert JM, Savi P, Laplace MC, Lale A. IL-4 inhibits LPS-, IL-1 beta- and TNF alpha-induced expression of tissue factor in endothelial cells and monocytes. FEBS Lett (1992) 310(1):31–3. doi: 10.1016/0014-5793(92)81139-D
55. Berkman N, John M, Roesems G, Jose P, Barnes PJ, Chung KF. Interleukin 13 inhibits macrophage inflammatory protein-1 alpha production from human alveolar macrophages and monocytes. Am J Respir Cell Mol Biol (1996) 15(3):382–9. doi: 10.1165/ajrcmb.15.3.8810643
56. Standiford TJ, Kunkel SL, Liebler JM, Burdick MD, Gilbert AR, Strieter RM. Gene expression of macrophage inflammatory protein-1 alpha from human blood monocytes and alveolar macrophages is inhibited by interleukin-4. Am J Respir Cell Mol Biol (1993) 9(2):192–8. doi: 10.1165/ajrcmb/9.2.192
57. Kiyota T, Okuyama S, Swan RJ, Jacobsen MT, Gendelman HE, Ikezu T. CNS expression of anti-inflammatory cytokine interleukin-4 attenuates Alzheimer’s disease-like pathogenesis in APP+PS1 bigenic mice. FASEB J (2010) 24(8):3093–102. doi: 10.1096/fj.10-155317
58. Horsfall AC, Butler DM, Marinova L, Warden PJ, Williams RO, Maini RN, et al. Suppression of collagen-induced arthritis by continuous administration of IL-4. J Immunol (Baltimore Md: 1950) (1997) 159(11):5687–96. doi: 10.4049/jimmunol.159.11.5687
59. Martin R. Interleukin 4 treatment of psoriasis: are pleiotropic cytokines suitable therapies for autoimmune diseases? Trends Pharmacol Sci (2003) 24(12):613–6. doi: 10.1016/j.tips.2003.10.006
60. Gu Y, Lewis DF, Deere K, Groome LJ, Wang Y. Elevated maternal IL-16 levels, enhanced IL-16 expressions in endothelium and leukocytes, and increased IL-16 production by placental trophoblasts in women with preeclampsia. J Immunol (Baltimore Md: 1950) (2008) 181(6):4418–22. doi: 10.4049/jimmunol.181.6.4418
61. Tranquilli AL, Landi B, Corradetti A, Giannubilo SR, Sartini D, Pozzi V, et al. Inflammatory cytokines patterns in the placenta of pregnancies complicated by HELLP (hemolysis, elevated liver enzyme, and low platelet) syndrome. Cytokine (2007) 40(2):82–8. doi: 10.1016/j.cyto.2007.08.010
62. Whitley GS, Cartwright JE. Trophoblast-mediated spiral artery remodelling: a role for apoptosis. J Anatomy (2009) 215(1):21–6. doi: 10.1111/j.1469-7580.2008.01039.x
63. Chen L, Liu X, Zhu Y, Cao Y, Sun L, Jin B. Localization and variation of TRAIL and its receptors in human placenta during gestation. Life Sci (2004) 74(12):1479–86. doi: 10.1016/j.lfs.2003.07.044
64. Keogh RJ, Harris LK, Freeman A, Baker PN, Aplin JD, Whitley GS, et al. Fetal-derived trophoblast use the apoptotic cytokine tumor necrosis factor-alpha-related apoptosis-inducing ligand to induce smooth muscle cell death. Circ Res (2007) 100(6):834–41. doi: 10.1161/01.RES.0000261352.81736.37
65. Zhou C, Long Y, Yang H, Zhu C, Ma Q, Zhang Y. TRAIL is decreased before 20 weeks gestation in women with hypertensive disorders of pregnancy. PloS One (2015) 10(6):e0128425. doi: 10.1371/journal.pone.0128425
66. Daniel Y, Kupferminc MJ, Baram A, Jaffa AJ, Fait G, Wolman I, et al. Plasma interleukin-12 is elevated in patients with preeclampsia. Am J Reprod Immunol (New York NY: 1989) (1998) 39(6):376–80. doi: 10.1111/j.1600-0897.1998.tb00372.x
67. Szarka A, Rigó J Jr., Lázár L, Beko G, Molvarec A. Circulating cytokines, chemokines and adhesion molecules in normal pregnancy and preeclampsia determined by multiplex suspension array. BMC Immunol (2010) 11:59. doi: 10.1186/1471-2172-11-59
68. Southcombe JH, Redman CW, Sargent IL, Granne I. Interleukin-1 family cytokines and their regulatory proteins in normal pregnancy and pre-eclampsia. Clin Exp Immunol (2015) 181(3):480–90. doi: 10.1111/cei.12608
69. Greer IA, Lyall F, Perera T, Boswell F, Macara LM. Increased concentrations of cytokines interleukin-6 and interleukin-1 receptor antagonist in plasma of women with preeclampsia: a mechanism for endothelial dysfunction? Obstet Gynecol (1994) 84(6):937–40.
70. Wu ZM, Yang H, Li M, Yeh CC, Schatz F, Lockwood CJ, et al. Pro-inflammatory cytokine-stimulated first trimester decidual cells enhance macrophage-induced apoptosis of extravillous trophoblasts. Placenta (2012) 33(3):188–94. doi: 10.1016/j.placenta.2011.12.007
71. Bikker A, Hack CE, Lafeber FP, van Roon JA. Interleukin-7: a key mediator in T cell-driven autoimmunity, inflammation, and tissue destruction. Curr Pharm Design (2012) 18(16):2347–56. doi: 10.2174/138161212800165979
72. Arbelaez CA, Glatigny S, Duhen R, Eberl G, Oukka M, Bettelli E. IL-7/IL-7 receptor signaling differentially affects effector CD4+ T cell subsets involved in experimental autoimmune encephalomyelitis. J Immunol (Baltimore Md: 1950) (2015) 195(5):1974–83. doi: 10.4049/jimmunol.1403135
73. Toldi G, Rigó J Jr., Stenczer B, Vásárhelyi B, Molvarec A. Increased prevalence of IL-17-producing peripheral blood lymphocytes in pre-eclampsia. Am J Reprod Immunol (New York NY: 1989) (2011) 66(3):223–9. doi: 10.1111/j.1600-0897.2011.00987.x
74. Deng Z, Zhang L, Tang Q, Xu Y, Liu S, Li H. Circulating levels of IFN-γ, IL-1, IL-17 and IL-22 in pre-eclampsia: A systematic review and meta-analysis. Eur J Obstet Gynecol Reprod Biol (2020) 248:211–21. doi: 10.1016/j.ejogrb.2020.03.039
75. Brewster JA, Orsi NM, Gopichandran N, McShane P, Ekbote UV, Walker JJ. Gestational effects on host inflammatory response in normal and pre-eclamptic pregnancies. Eur J Obstet Gynecol Reprod Biol (2008) 140(1):21–6. doi: 10.1016/j.ejogrb.2007.12.020
76. Charkiewicz K, Jasinska E, Goscik J, Koc-Zorawska E, Zorawski M, Kuc P, et al. Angiogenic factor screening in women with mild preeclampsia - New and significant proteins in plasma. Cytokine (2018) 106:125–30. doi: 10.1016/j.cyto.2017.10.020
77. Hypertension in pregnancy. Report of the American College of Obstetricians and Gynecologists’ Task Force on hypertension in pregnancy. Obstet Gynecol (2013) 122(5):1122–31. doi: 10.1097/01.AOG.0000437382.03963.88
78. Sun Y, Wu S, Zhou Q, Li X. Trophoblast-derived interleukin 9 mediates immune cell conversion and contributes to maternal-fetal tolerance. J Reprod Immunol (2021) 148:103379. doi: 10.1016/j.jri.2021.103379
79. Akhtar F, Rouse CA, Catano G, Montalvo M, Ullevig SL, Asmis R, et al. Acute maternal oxidant exposure causes susceptibility of the fetal brain to inflammation and oxidative stress. J Neuroinflamm (2017) 14(1):195. doi: 10.1186/s12974-017-0965-8
80. Raghupathy R, Al-Azemi M, Azizieh F. Intrauterine growth restriction: cytokine profiles of trophoblast antigen-stimulated maternal lymphocytes. Clin Dev Immunol (2012) 2012:734865. doi: 10.1155/2012/734865
81. Yano S, Sone S, Nishioka Y, Mukaida N, Matsushima K, Ogura T. Differential effects of anti-inflammatory cytokines (IL-4, IL-10 and IL-13) on tumoricidal and chemotactic properties of human monocytes induced by monocyte chemotactic and activating factor. J Leuk Biol (1995) 57(2):303–9. doi: 10.1002/jlb.57.2.303
Keywords: hypertensive disorders of pregnancy, pre-eclampsia, inflammatory cytokine, Mendelian randomization, genome-wide association study
Citation: Guan S, Bai X, Ding J and Zhuang R (2023) Circulating inflammatory cytokines and hypertensive disorders of pregnancy: a two-sample Mendelian randomization study. Front. Immunol. 14:1297929. doi: 10.3389/fimmu.2023.1297929
Received: 20 September 2023; Accepted: 06 November 2023;
Published: 16 November 2023.
Edited by:
Giselle Penton-Rol, Center for Genetic Engineering and Biotechnology (CIGB), CubaReviewed by:
Estibalitz Laresgoiti-Servitje, Tecnológico de Monterrey, MexicoMaria Laura Manca, University of Pisa, Italy
Copyright © 2023 Guan, Bai, Ding and Zhuang. This is an open-access article distributed under the terms of the Creative Commons Attribution License (CC BY). The use, distribution or reproduction in other forums is permitted, provided the original author(s) and the copyright owner(s) are credited and that the original publication in this journal is cited, in accordance with accepted academic practice. No use, distribution or reproduction is permitted which does not comply with these terms.
*Correspondence: Rujin Zhuang, hmuzrj@163.com