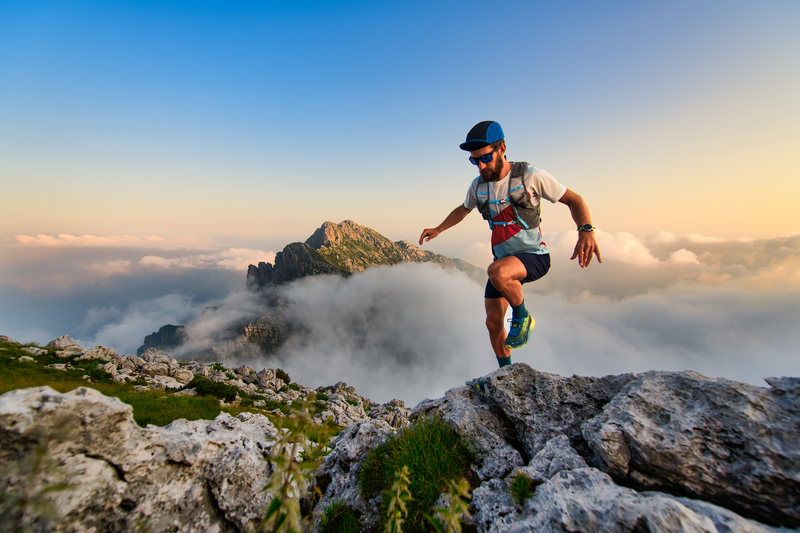
95% of researchers rate our articles as excellent or good
Learn more about the work of our research integrity team to safeguard the quality of each article we publish.
Find out more
ORIGINAL RESEARCH article
Front. Immunol. , 14 November 2023
Sec. Inflammation
Volume 14 - 2023 | https://doi.org/10.3389/fimmu.2023.1295377
Objective: Coronary heart disease (CHD) is one of the major cardiovascular diseases, a common chronic disease in the elderly and a major cause of disability and death in the world. Currently, intensive care unit (ICU) patients have a high probability of concomitant coronary artery disease, and the mortality of this category of patients in the ICU is receiving increasing attention. Therefore, the aim of this study was to verify whether the composite inflammatory indicators are significantly associated with ICU mortality in ICU patients with CHD and to develop a simple personalized prediction model.
Method: 7115 patients from the Multi-Parameter Intelligent Monitoring in Intensive Care Database IV were randomly assigned to the training cohort (n = 5692) and internal validation cohort (n = 1423), and 701 patients from the eICU Collaborative Research Database served as the external validation cohort. The association between various inflammatory indicators and ICU mortality was determined by multivariate Logistic regression analysis and Cox proportional hazards model. Subsequently, a novel predictive model for mortality in ICU patients with CHD was developed in the training cohort and performance was evaluated in the internal and external validation cohorts.
Results: Various inflammatory indicators were demonstrated to be significantly associated with ICU mortality, 30-day ICU mortality, and 90-day ICU mortality in ICU patients with CHD by Logistic regression analysis and Cox proportional hazards model. The area under the curve of the novel predictive model for ICU mortality in ICU patients with CHD was 0.885 for the internal validation cohort and 0.726 for the external validation cohort. The calibration curve showed that the predicted probabilities of the model matched the actual observed probabilities. Furthermore, the decision curve analysis showed that the novel prediction model had a high net clinical benefit.
Conclusion: In ICU patients with CHD, various inflammatory indicators were independent risk factors for ICU mortality. We constructed a novel predictive model of ICU mortality risk in ICU patients with CHD that had great potential to guide clinical decision-making.
Coronary heart disease (CHD), one of the major cardiovascular diseases, is a common chronic disease in the elderly and is the leading cause of disability and death in the world (1). Moreover, due to population growth and ageing, the economic consequences of this chronic disease affect many levels of society and will be an increasing burden, especially for low- and middle-income countries (2). The Prospective Urban and Rural Epidemiology study shows that secondary prevention of cardiovascular disease remains inaccessible and unaffordable for most communities and households in upper middle-income, lower middle-income and low-income countries (3). Patients with CHD are recently receiving increased attention in the ICU, and a dedicated arrhythmia monitoring and treatment unit, the coronary ICU, has emerged to care for ICU patients with CHD. ICU patients with CHD require more life support and have more complications (e.g. central venous catheter infections, ventilator-associated pneumonia, etc.), which have led to a significant increase in ICU mortality, length of ICU stay, and healthcare costs for ICU patients with CHD (4). Therefore, the prognostic management of ICU patients with CHD is of great importance.
The main known risk factors for the diagnosis or prognosis of CHD include dyslipidemia, high blood pressure, diabetes, and smoking (5). Also, obesity and the metabolic syndrome would lead to an increased risk of CHD (6), possibly because insulin resistance is a major feature of the metabolic syndrome. Inflammation plays a key role in the development and progression of atherosclerosis, as indicated by a unified view of the pathophysiology of atherosclerosis (7), and inflammation contributes to an increased risk of cardiovascular events (8). Inflammatory signaling alters the behavior of endothelial cells and smooth muscle and recruits more interacting inflammatory cells to promote lesion formation and complications (9). Li et al. reported that cytoleukin-6, C-reactive protein (CRP), complement, CD40 and myeloperoxidase could be used to assess the severity of CHD (10). Overall, inflammatory indicators have great potential as prognostic predictors in ICU patients with CHD.
Blood test is widely used as a simple and inexpensive test for various diseases. Previous studies have shown the predictive value of inflammatory indicators for all-cause mortality in cardiovascular disease. For example, in patients with non-ST-segment elevation myocardial infarction and ST-segment elevation myocardial infarction, the platelet-lymphocyte ratio (PLR) ratio is an independent predictor for mortality (11). Similarly, Osadnik et al. found that higher mortality in patients with stable coronary artery disease who underwent stenting in patients with the highest PLR values (12). Other study indicated that neutrophil-lymphocyte ratio (NLR) had potential to be an independent prognostic factor in CHD patients (13). Moreover, Xiao et al. reported that a U-shaped association between the systemic inflammatory index (SII) and all-cause mortality in patients with cardiovascular disease in the general individuals, which could be used as a clinical predictor (14). Xia et al. revealed that the systemic inflammatory response index (SIRI) was significantly associated with myocardial infarction in patients < 60 years, but not SII (15). In addition, red blood cell distribution width (RDW) is a useful tool for differentiating between inflammatory and non-inflammatory joint disease in clinical practice (16). Hou et al. found RDW to be an independent risk factor for frailty in elderly patients with coronary artery disease (17). However, there is a lack of studies on the correlation between inflammatory markers and mortality in ICU patients with CHD.
The aims of our study were: (1) determining the association of several inflammatory indicators with all-cause ICU mortality in ICU patients with CHD, including SII, SIRI, NLR, PLR, neutrophil to lymphocyte platelet ratio (NLPR), aggregate index of systemic inflammation (AISI), and RDW; (2) constructing a novel model based on these indicators and severity score to predict ICU mortality in ICU patients with CHD.
Our study data were obtained from a publicly accessible Multi-Parameter Intelligent Monitoring in Intensive Care Database IV (MIMIC IV, version 2.0, recruitment during 2012 to 2019) as detailed in previous publications (18). In addition, we extracted external validation cohort from the eICU Collaborative Research Database (EICU, version 2.0, recruitment during 2014 to 2015) (19). As all data were anonymous, the patient consent was irrelevant. All data in this study were extracted by the author Yang Chen, who obtained access to the database and relevant credentials (NO. 36328122).
Our study included all adult patients with CHD admitted to the ICU according to International Classification of Diseases (ICD) version 9 or 10 (We included all patients who contained any ICD codes related to coronary artery). The exclusion criteria were as follows (1) records of multiple ICU admissions other than the first ICU admission; (2) records of ICU stays of less than 24 hours; (3) records of repeated multiple hospitalizations; (4) exclusion of records of missing neutrophils, lymphocytes, monocytes, platelets, and RDW. Finally, a total of 7115 patients were extracted from MIMIC IV for initial analysis and model construction, and 701 patients were extracted from EICU for external validation (details shown in Figure 1).
Figure 1 Flow chart of patient screening. CHD, coronary heart disease; EICU, eICU Collaborative Research Database; ICU, intensive care unit; MIMIC IV, Multi-Parameter Intelligent Monitoring in Intensive Care Database IV; SOFA, sequential organ failure assessment.
We extracted the following data: demographic characteristics, vital signs, comorbidities, severity scores on admission to the ICU, laboratory results (within the first day of admission to the ICU), interventions, and medications. For variables measured multiple times, we used the first value. Our primary outcomes: all-cause mortality during ICU admission (ICU mortality); Secondary outcome: all-cause 30-day mortality after ICU admission (30-day ICU mortality) and all-cause 90-day mortality after ICU admission (90-day ICU mortality).
SII was defined as platelet × neutrophil/lymphocyte. SIRI was defined as neutrophil × monocyte/lymphocyte. NLP was defined as neutrophil/lymphocyte. PLR was defined as platelet/lymphocyte. NLPR was defined as neutrophil/(lymphocyte × platelet). AISI was defined as neutrophils × monocytes × platelets/lymphocytes.
For continuous variables, normality was tested using the Shapiro-Wilk test. And depending on the type and distribution of the variable, the normal distribution was expressed as mean and standard deviation (SD) and differences between groups were assessed using the t-test, and the non-normal distribution was expressed as median and interquartile range (IQR) and differences between groups were assessed using the Kruskal-Wallis test. For categorical variables, expressed as counts and percentages, differences between groups were assessed using the Chi2 or Fisher’s exact test.
We analyzed the cumulative rate of all-cause mortality in CHD patients within 90 days of ICU admission using Kaplan-Meier survival analysis to compare the cumulative distribution of deaths among patients in four-score subgroups for each indicator at admission. We also used the restricted cubic spline function (RCS) to explore the nonlinear relationship between these metrics and the study outcomes when used as continuous variables.
To further assess the independent associations between the indicators and the primary endpoints, we used Logistic regression model and Cox proportional hazards model, and we used different models to adjust for potential confounders. Model 1: crude analysis without adjusting for any confounders; Model 2: adjustments including age, male, and race; Model 3: additional adjusting for confounders (heart rate, respiratory rate, saturation of peripheral oxygen, sequential organ failure assessment (SOFA), acute exacerbation of chronic obstructive pulmonary disease, congestive heart failure, malignant cancer, dyslipidemia, acute respiratory failure, acute kidney failure, potassium, aniongap, blood urea nitrogen, glucose, serum creatinine, hematocrit, hemoglobin, mean corpuscular volume, red blood cell, dialysis, vasopressor, invasive mechanical ventilation, coronary artery bypass graft, angiotensin-converting enzyme inhibitors or angiotensin receptor blocker, antiplatelet, statin, non-vitamin K antagonist oral anticoagulant, and Vitamin K antagonists) based on Model 2. Once the indicators were grouped based on quartiles, we chose G1 as the reference group and calculated the adjusted odds ratio (OR) or hazard ratio (HR) of the primary endpoints for the other groups in comparison to the reference group.
Given the importance of mortality risk management in ICU patients with CHD, then we used these inflammatory indictors in conjunction with the widely utilized SOFA to construct a novel predictive model of ICU mortality in ICU patients with CHD. We first divided these inflammatory indicators into elevated value and non-elevated value groups based on their respective third quartiles. Subsequently, we divided the entire cohort into a training cohort and an internal validation cohort on a 8:2 basis. In the training corhort, we used univariate Logistic regression analyses followed by stepwise forward multivariate Logistic regression analyses to select the variables used to construct the novel predictive model, computed correlation coefficient and variance inflation factor (VIF) to detect covariance of the variables in the model, and used the Hosmer-Lemeshow test to assess the fit of the logistic regression models. Then, the area under the curve (AUC) of receiver operating characteristic (ROC) curves, plotting of calibration curves, decision curve analysis (DCA) (compared with SOFA) were used in both the internal and external validation cohorts, in addition, we computed the integrated discrimination improvement (IDI) to validate the variability of the predictive performance of the new model between the novel model and SOFA. Furthermore, we transformed the novel model obtained from the training cohort to nomogram and interactive network dynamic nomogram.
We performed all statistical processing using SPSS (version 29), Stata (version 17), and R (version 4.2.3). In all analyses, a two-tailed P < 0.05 was considered statistically significant.
In our study, 7115 ICU patients with CHD were included and the median (IQR) age of all patients was 71.43 (63.08 - 79.60), 68.9% patients were male and 69.7% patients were White. We divided all patients into two groups based on ICU survival and death (details shown in Table 1), and we found that the ICU death group was significantly older than the survival group (median 75.25, IQR [65.65 - 82.34] vs. median 71.19, IQR [71.19 - 79.14], P < 0.001), and that the ICU death group had fewer males, lower White individuals, and more comorbidities. In addition, the ICU death group had higher value of indicators than the survivor group.
Based on Table 2, the results of multivariate logistic regression models after adjusting for baseline characteristics, vital signs, comorbidities, laboratory results, interventions, and medications showed the elevated inflammatory indicators, except for the NLPR, were independent risk factors for ICU mortality in ICU patients with CHD, among which the highest risk was for RDW (first quartile as reference group; fourth quartile: OR = 2.05, 95% CI: 1.35 - 3.10, P < 0.001) and AISI (first quartile as reference group; fourth quartile: OR = 1.68, 95% CI: 1.19 - 2.36, P = 0.003). Furthermore, the association of each inflammation indicator with ICU mortality when used as a continuous variable was shown in Figure 2A, which implied that the greater the values of SII, NLR, PLR, AISI, and RDW, the higher ICU mortality of ICU patients with CHD.
Figure 2 Restricted cubic spline function between inflammation indicators (SII, SIRI, NLR, PLR, NLPR, AISI, RDW) and ICU mortality (A), ICU 30-day mortality (B), and ICU 90-day mortality (C). AISI, aggregate index of systemic inflammation; NLPR, neutrophil to lymphocyte platelet ratio; NLR, neutrophil-lymphocyte ratio; PLR, platelet-lymphocyte ratio; RDW, red blood cell volume distribution width; SII, systemic inflammatory index; SIRI, systemic inflammatory response index.
Based on Table 3, the results of multivariate Cox proportional hazards models after adjusting for baseline characteristics, vital signs, comorbidities, laboratory results, interventions, and medications showed all the elevated inflammatory indicators were independent risk factors for ICU mortality in ICU patients with CHD, among which the highest risk was for RDW (first quartile as reference group; second quartile: HR = 1.62, 95% CI: 1.13 - 2.32, P = 0.009; third quartile: HR = 1.80, 95% CI: 1.30 - 2.52, P < 0.001; fourth quartile: HR = 2.96, 95% CI: 2.10 - 4.17, P < 0.001) and AISI (first quartile as reference group; fourth quartile: HR = 1.80, 95% CI: 1.37 - 2.37, P < 0.001). Moreover, the association of each inflammation indicator with 30-day ICU mortality when used as a continuous variable was shown in Figure 2B, which implied that the greater the values of SII, NLR, PLR, NLPR, and RDW, the higher 30-day ICU mortality of ICU patients with CHD.
Table 3 The association of each inflammatory indicator with 30-day ICU mortality in ICU patients with CHD.
Based on Table 4, the results of multivariate Cox proportional hazards models after adjusting for baseline characteristics, vital signs, comorbidities, laboratory results, interventions, and medications showed the elevated inflammatory indicators, except for the NLPR, were independent risk factors for ICU mortality in ICU patients with CHD, among which the highest risk was for RDW (first quartile as reference group; second quartile: HR = 1.42, 95% CI: 1.04 - 1.93, P = 0.028; third quartile: HR = 1.73, 95% CI: 1.30 - 2.30, P < 0.001; fourth quartile: HR = 3.04, 95% CI: 2.27 - 4.08, P < 0.001) and AISI (first quartile as reference group; fourth quartile: OR = 1.63, 95% CI: 1.28 - 2.08, P < 0.001). Furthermore, the association of each inflammation indicator with 90-day ICU mortality when used as a continuous variable was shown in Figure 2C, which implied that the greater the values of SII, NLR, PLR, NLPR, and RDW, the higher 90-day ICU mortality of ICU patients with CHD.
Table 4 The association of each inflammatory indicator with 90-day ICU mortality in ICU patients with CHD.
To further explore the association of inflammation indicators with ICU deaths, we plotted Kaplan-Meier cumulative curves for the study outcomes. According to Figure 3, among all the inflammation indicators, the higher subgroups had the most cumulative ICU deaths and were statistically different (all P < 0.001).
Figure 3 Kaplan-Mill survival analysis of cumulative all-cause mortality in CHD patients within 90 Days of ICU admission. CHD, coronary heart disease; ICU, intensive care unit.
Given that these inflammatory indicators were independent risk factors for ICU mortality in ICU patients with CHD, our aim was to construct a novel model to predict ICU mortality in ICU patients with CHD using these inflammatory indicators combined with the SOFA score. We divided patients from MIMIC into a training cohort (n = 5692) and an internal validation cohort (n = 1423) in an 8:2 ratio. Table 5 showed the results of univariate logistic regression analyses within the training cohort.
Table 5 Univariate and multifactorial analyses about the association between variables and ICU mortality.
Then, based on the results of univariate logistic regression, these variables were subjected to multivariate stepwise forward logistic regression (Table 5), and four variables were included in the final predictive model. Regarding the covariance of the variables, correlation coefficient and VIF were calculated and visualized in Figure 4, with the results of all variables’ correlation coefficient < 0.5 and VIF < 2, indicating that there was no covariance in the model. The P-value of the Hosmer-Lemeshow test was 0.425, indicating that the model was well fitted, and a nomogram was also plotted based on our model (Figure 5).
Figure 4 Correlation coefficients and variance inflation factors of the variables in the model. AISI, aggregate index of systemic inflammation; PLR, platelet-lymphocyte ratio; RDW, red blood cell volume distribution width; SOFA, sequential organ failure assessment.
Figure 5 Nomogram for the novel model. AISI, aggregate index of systemic inflammation; PLR, platelet-lymphocyte ratio; RDW, red blood cell volume distribution width; SOFA, sequential organ failure assessment.
Our external validation cohort was derived from the EICU database, based on the same inclusion and exclusion criteria, the SOFA value was calculated, and excluded individuals with missing data, finally 701 patients were obtained. According to Figure 6, the ROC indicated that our model had excellent discriminative performance in both the internal cohort (AUC = 0.885, 95% CI: 0.884 - 0.886) and external validation cohort (AUC = 0.726, 95% CI: 0.725 - 0.728). Furthermore, we used bootstrapping to describe the calibration curves (Figure 6), and in both the internal and external validation cohorts, although both the apparent curves and the bias-correction curves deviated slightly from the reference line, the good consistency between observations and predictions were presented.
Figure 6 ROC, calibration curves, and DCA in the internal validation cohort (A) and external validation cohort (B). DCA, decision curve analysis; ROC, receiver operating characteristic; SOFA, sequential organ failure assessment.
To validate the performance of our model for clinical applications, we plotted DCA curves and compared our model to SOFA scores. In both the internal validation set and the external validation set, our model-guided medical interventions provide excellent net benefits and outperform the SOFA score, the details of which are depicted in Figure 6. We also compared the IDI difference between our model and the SOFA score, with our model’s predictive performance improving in both the internal and external validation cohorts (internal validation cohort: 6.35% [3.62% - 9.09%]; external validation cohort: 2.12% [0.87% - 3.36%]; both P < 0.001). In addition, to facilitate clinical use, we transformed the model into an online dynamic prediction tool with a simple, easy-to-use user interface (Figure 7), which is available at https://yangprediction.shinyapps.io/Prediction/.
Figure 7 User interface for a novel model-based online prediction tool. AISI, aggregate index of systemic inflammation; PLR, platelet-lymphocyte ratio; RDW, red blood cell volume distribution width; SOFA, sequential organ failure assessment.
This was the first study to assess the relationship between the composite inflammation indicators and mortality in ICU patients with CHD. Most inflammation indicators in our study were significantly associated with ICU mortality in the retrospective MIMIC IV database of ICU patients with CHD. Demonstrating the significant promise of these inflammation indicators for mortality risk management in ICU patients with CHD.
The basic mechanism of CHD is abnormal lipid metabolism leading to coronary atherosclerosis (20). Atherosclerosis is commonly characterized by complex interactions between apoptosis and autophagy in endothelial cells, smooth muscle cells, or macrophages, leading to the formation of atherosclerotic plaques (21). Inflammation, which often serves as a risk stratification marker and predicts adverse events, plays an equally important role in the development of atherosclerosis formation (22). Inflammatory-endothelial dysfunction promotes atherosclerosis (23). Of the traditional indicators of inflammation, such as elevated IL-6 may promote elevated CRP levels in those at risk for CHD (24). In circulatory disorders, elevated CRP levels in patients with coronary syndromes, which may progress to heart failure, are strongly correlated with dense plaque composition (25).
Neutrophils, the most abundant subtype of leukocytes in the blood, are critical in mediating inflammation. Neutrophils cause smooth muscle cell lysis and death, which has been shown to cause tissue damage and inflammation in advanced stages of atherosclerosis (26). Platelets actively promote the process of atherosclerosis in response to the interaction of endothelial cells, leukocytes, and unactivated platelets (27). Neutrophil-platelet interaction is an important biological process associated with atherosclerosis, thrombosis, and ischemic stroke (28). Lymphocyte apoptosis tends to increase progressively with increasing atherosclerosis, and lymphocytes are involved in anti-inflammatory and endothelial protection (29). Li et al. evaluated the prognostic values of five lymphocyte-based inflammation indices, including PLR, NLR, SII, and SIRI, in patients with acute coronary syndrome who underwent first-time percutaneous coronary intervention, and found that the addition of NLR, SII, or SIRI, and especially SIRI, to the Global Registry of Acute Coronary Events (GRACE) score, resulted in a better risk-predicting performance than the use of the GRACE score alone (30). It has also been confirmed that NLR is associated with the incidence and susceptibility to carotid plaque detected by carotid ultrasound in patients with acute ischemic stroke (31). And SII was initially defined as the prognosis for cancer, cerebral hemorrhage, and coronary artery stenosis (32). In a previous study, Qu et al. indicated that a lower peripheral hemoglobin to erythrocyte distribution width ratio (HRR) was associated with an increased risk of CHD (33). Decreased HRR is not only associated with decreased hemoglobin, but also with increased RDW.
In our study, RDW was the prognostic independent factor with the most pronounced correlation among the inflammatory indicators studied above. It has been shown that RDW affects the poor prognosis of CHD patients, but the underlying causes remain unclear. Although anaemia is a known risk factor for mortality, we adjusted for haemoglobin in Model 3 and, therefore, still consider RDW to be a risk factor for mortality separate from anaemia. We hypothesized two main causes for the higher risk mortality of CHD patients with high RDW: (a) RDW is associated with a variety of inflammatory markers, e.g., interleukin-6 (34), and erythrocyte sedimentation rate (35). (b) Chronic inflammation may induce disturbances in iron metabolism (36), and the latter is significantly associated with all-cause mortality in CHD patients (37). In addition, RDW may affect dysglycaemia, vitamin D3 deficiency, oxidative stress, and renal failure, all of which are common risk factors for mortality (38).
Our novel predictive model provides an accurate risk assessment and helps ICU physicians identify which ICU patients with CHD are at high mortality risk. And allows ICU healthcare teams to improve the prognosis of high mortality risk patients through more frequent monitoring, more urgent interventions, and stricter medication management at the early stage. In addition, earlier identification of high mortality risk patients can facilitate more informed discussions about the patient’s condition and prognosis between ICU physicians and the patient’s families. Overall, our model has a potentially important role in mortality risk management of ICU patients with CHD, helping to improve the accuracy of clinical decision-making and the quality of healthcare. However, the validity and feasibility of the model have only been confirmed in the datasets. Despite the strong clinical utility of the nomogram, further validation and adjustment is needed in the actual ICU clinical setting.
Some limitations of our study should be noted. First, this was a retrospective study in which retrospective bias is unavoidable, and thus more rigorous prospective studies would be required in the future. Second, previously mentioned traditional inflammatory indicators of IL-6 and CRP are important in mortality risk of CHD. We tried to extract them but due to the limitations of MIMIC IV, the percentage of missing values for CRP was about 98% and no record of IL-6 was retrieved. Further studies are still needed to prove whether they will have an impact on our results. Third, our study data were from the database of the United States and were overwhelmingly of White race, so applicability to ICU patients from other countries or other races requires further validation. Fourth, despite we adjusted for virous potential confounders, there may still be some important factors that were missed, and these may have a non-negligible impact on our inflammatory indicators. For example, neutrophil counts are usually elevated when there is an active infection or inflammation in the patient’s body, while lymphocyte counts may be decreased, thus affecting our inflammatory indicators. However, due to MIMIC’s limitations, it is hard to definitively determine the patient’s infection condition. Fifth, although our novel model demonstrated promising predictive performance in the internal and external validation cohort form MIMIC IV and EICU, however scalability to other hospitals remains an issue as the model’s performance in the external validation cohort is weaker than the internal validation cohort. Therefore, we also need a larger external validation cohort to validate the performance of our model. Despite these limitations, our study shows that the new model we constructed is remarkably promising and deserves further exploration in future clinical work and research.
Our study revealed that inflammation indicators SII, SIRI, NLR, PLR, NLPR, AISI, and RDW were significantly associated with ICU mortality. Furthermore, we constructed a novel predictive model by combining some of these indicators with SOFA to predict the risk of ICU death in ICU patients with CHD, which has a remarkable potential to guide clinical decision-making and prognostic management.
Publicly available datasets were analyzed in this study. This data can be found here: MIMIC IV, EICU.
Ethical approval was not required for the study involving humans in accordance with the local legislation and institutional requirements. Written informed consent to participate in this study was not required from the participants or the participants’ legal guardians/next of kin in accordance with the national legislation and the institutional requirements.
YCheng: Investigation, Methodology, Writing – original draft, Writing – review & editing. YChen: Data curation, Formal Analysis, Investigation, Methodology, Software, Validation, Visualization, Writing – review & editing. MM: Formal Analysis, Software, Writing – original draft. RW: Formal Analysis, Software, Writing – original draft. JZ: Software, Writing – original draft. QH: Methodology, Project administration, Resources, Supervision, Writing – review & editing.
The author(s) declare financial support was received for the research, authorship, and/or publication of this article.
We appreciate all of the investigators and subjects who took part in this study.
The authors declare that the research was conducted in the absence of any commercial or financial relationships that could be construed as a potential conflict of interest.
All claims expressed in this article are solely those of the authors and do not necessarily represent those of their affiliated organizations, or those of the publisher, the editors and the reviewers. Any product that may be evaluated in this article, or claim that may be made by its manufacturer, is not guaranteed or endorsed by the publisher.
1. Ang CS, Chan KM. A review of coronary artery disease research in Malaysia. Med J Malaysia. (2016) 71(Suppl 1):42–57.
2. Gheorghe A, Griffiths U, Murphy A, Legido-Quigley H, Lamptey P, Perel P. The economic burden of cardiovascular disease and hypertension in low- and middle-income countries: a systematic review. BMC Public Health (2018) 18(1):975. doi: 10.1186/s12889-018-5806-x
3. Khatib R, McKee M, Shannon H, Chow C, Rangarajan S, Teo K, et al. Availability and affordability of cardiovascular disease medicines and their effect on use in high-income, middle-income, and low-income countries: an analysis of the PURE study data. Lancet (London England). (2016) 387(10013):61–9. doi: 10.1016/S0140-6736(15)00469-9
4. van Diepen S, Sligl WI, Washam JB, Gilchrist IC, Arora RC, Katz JN. Prevention of critical care complications in the coronary intensive care unit: protocols, bundles, and insights from intensive care studies. Can J Cardiol (2017) 33(1):101–9. doi: 10.1016/j.cjca.2016.06.011
5. Nauta ST, Deckers JW, van der Boon RM, Akkerhuis KM, van Domburg RT. Risk factors for coronary heart disease and survival after myocardial infarction. Eur J Prev Cardiol (2014) 21(5):576–83. doi: 10.1177/2047487312460514
6. Grundy SM. Obesity, metabolic syndrome, and coronary atherosclerosis. Circulation (2002) 105(23):2696–8. doi: 10.1161/01.CIR.0000020650.86137.84
7. Alwi I. Targeting inflammation and immune system in acute myocardial infarction. Acta Med Indonesiana. (2019) 51(4):287–9.
8. Ross R. Atherosclerosis–an inflammatory disease. New Engl J Med (1999) 340(2):115–26. doi: 10.1056/NEJM199901143400207
9. Libby P, Ridker PM, Hansson GK. Progress and challenges in translating the biology of atherosclerosis. Nature (2011) 473(7347):317–25. doi: 10.1038/nature10146
10. Li H, Sun K, Zhao R, Hu J, Hao Z, Wang F, et al. Inflammatory biomarkers of coronary heart disease. Front bioscience (Scholar edition). (2018) 10(1):185–96. doi: 10.2741/s508
11. Çiçek G, Açıkgoz SK, Bozbay M, Altay S, Uğur M, Uluganyan M, et al. Neutrophil-lymphocyte ratio and platelet-lymphocyte ratio combination can predict prognosis in patients with ST-segment elevation myocardial infarction undergoing primary percutaneous coronary intervention. Angiology (2015) 66(5):441–7. doi: 10.1177/0003319714535970
12. Osadnik T, Wasilewski J, Lekston A, Strzelczyk J, Kurek A, Gonera M, et al. The platelet-to-lymphocyte ratio as a predictor of all-cause mortality in patients with coronary artery disease undergoing elective percutaneous coronary intervention and stent implantation. J Saudi Heart Assoc (2015) 27(3):144–51. doi: 10.1016/j.jsha.2015.02.004
13. Balta S, Celik T, Mikhailidis DP, Ozturk C, Demirkol S, Aparci M, et al. The relation between atherosclerosis and the neutrophil-lymphocyte ratio. Clin Appl thrombosis/hemostasis (2016) 22(5):405–11. doi: 10.1177/1076029615569568
14. Xiao S, Wang Z, Zuo R, Zhou Y, Yang Y, Chen T, et al. Association of systemic immune inflammation index with all-cause, cardiovascular disease, and cancer-related mortality in patients with cardiovascular disease: A cross-sectional study. J Inflammation Res (2023) 16:941–61. doi: 10.2147/JIR.S402227
15. Jin Z, Wu Q, Chen S, Gao J, Li X, Zhang X, et al. The associations of two novel inflammation indexes, SII and SIRI with the risks for cardiovascular diseases and all-cause mortality: A ten-year follow-up study in 85,154 individuals. J Inflammation Res (2021) 14:131–40. doi: 10.2147/JIR.S283835
16. Horta-Baas G, Romero-Figueroa MDS. Clinical utility of red blood cell distribution width in inflammatory and non-inflammatory joint diseases. Int J rheumatic diseases. (2019) 22(1):47–54. doi: 10.1111/1756-185X.13332
17. Hou P, Xue HP, Mao XE, Li YN, Wu LF, Liu YB. Inflammation markers are associated with frailty in elderly patients with coronary heart disease. Aging (2018) 10(10):2636–45. doi: 10.18632/aging.101575
18. Johnson AEW, Bulgarelli L, Shen L, Gayles A, Shammout A, Horng S, et al. MIMIC-IV, a freely accessible electronic health record dataset. Sci data. (2023) 10(1):1. doi: 10.1038/s41597-023-01945-2
19. Peng S, Huang J, Liu X, Deng J, Sun C, Tang J, et al. Interpretable machine learning for 28-day all-cause in-hospital mortality prediction in critically ill patients with heart failure combined with hypertension: A retrospective cohort study based on medical information mart for intensive care database-IV and eICU databases. Front Cardiovasc Med (2022) 9:994359. doi: 10.3389/fcvm.2022.994359
20. Borén J, Chapman MJ, Krauss RM, Packard CJ, Bentzon JF, Binder CJ, et al. Low-density lipoproteins cause atherosclerotic cardiovascular disease: pathophysiological, genetic, and therapeutic insights: a consensus statement from the European Atherosclerosis Society Consensus Panel. Eur Heart J (2020) 41(24):2313–30. doi: 10.1093/eurheartj/ehz962
21. Dong Y, Chen H, Gao J, Liu Y, Li J, Wang J. Molecular machinery and interplay of apoptosis and autophagy in coronary heart disease. J Mol Cell Cardiol (2019) 136:27–41. doi: 10.1016/j.yjmcc.2019.09.001
22. Crea F, Libby P. Acute coronary syndromes: the way forward from mechanisms to precision treatment. Circulation (2017) 136(12):1155–66. doi: 10.1161/CIRCULATIONAHA.117.029870
23. Wu L, Shi Y, Kong C, Zhang J, Chen S. Dietary inflammatory index and its association with the prevalence of coronary heart disease among 45,306 US adults. Nutrients (2022) 14(21):4553. doi: 10.3390/nu14214553
24. Li H, Sun K, Zhao R, Hu J, Hao Z, Wang F, et al. Inflammatory biomarkers of coronary heart disease. FBL (2017) 22(3):504–15.
25. Kivimäki M, Kawachi I. Regarding the relationship between the inflammatory marker C-reactive protein and coronary heart disease. Am J Epidemiol (2013) 178(1):154–5. doi: 10.1093/aje/kwt105
26. Silvestre-Roig C, Braster Q, Wichapong K, Lee EY, Teulon JM, Berrebeh N, et al. Externalized histone H4 orchestrates chronic inflammation by inducing lytic cell death. Nature (2019) 569(7755):236–40. doi: 10.1038/s41586-019-1167-6
27. Pasalic L, Wang SS, Chen VM. Platelets as biomarkers of coronary artery disease. Semin Thromb hemostasis. (2016) 42(3):223–33. doi: 10.1055/s-0036-1572328
28. Jickling GC, Liu D, Ander BP, Stamova B, Zhan X, Sharp FR. Targeting neutrophils in ischemic stroke: translational insights from experimental studies. J Cereb Blood Flow Metab (2015) 35(6):888–901. doi: 10.1038/jcbfm.2015.45
29. Núñez J, Sanchis J, Bodí V, Núñez E, Mainar L, Heatta AM, et al. Relationship between low lymphocyte count and major cardiac events in patients with acute chest pain, a non-diagnostic electrocardiogram and normal troponin levels. Atherosclerosis (2009) 206(1):251–7. doi: 10.1016/j.atherosclerosis.2009.01.029
30. Li Q, Ma X, Shao Q, Yang Z, Wang Y, Gao F, et al. Prognostic impact of multiple lymphocyte-based inflammatory indices in acute coronary syndrome patients. Front Cardiovasc Med (2022) 9:811790. doi: 10.3389/fcvm.2022.811790
31. Li X, Li J, Wu G. Relationship of neutrophil-to-lymphocyte ratio with carotid plaque vulnerability and occurrence of vulnerable carotid plaque in patients with acute ischemic stroke. BioMed Res Int (2021) 2021:6894623. doi: 10.1155/2021/6894623
32. Di X, Liu S, Xiang L, Jin X. Association between the systemic immune-inflammation index and kidney stone: A cross-sectional study of NHANES 2007-2018. Front Immunol (2023) 14:1116224. doi: 10.3389/fimmu.2023.1116224
33. Qu J, Zhou T, Xue M, Sun H, Shen Y, Chen Y, et al. Correlation analysis of hemoglobin-to-red blood cell distribution width ratio and frailty in elderly patients with coronary heart disease. Front Cardiovasc Med (2021) 8:728800. doi: 10.3389/fcvm.2021.728800
34. Miyamoto K, Inai K, Takeuchi D, Shinohara T, Nakanishi T. Relationships among red cell distribution width, anemia, and interleukin-6 in adult congenital heart disease. Circ J Off J Japanese Circ Society. (2015) 79(5):1100–6. doi: 10.1253/circj.CJ-14-1296
35. Lippi G, Targher G, Montagnana M, Salvagno GL, Zoppini G, Guidi GC. Relation between red blood cell distribution width and inflammatory biomarkers in a large cohort of unselected outpatients. Arch Pathol Lab Med (2009) 133(4):628–32. doi: 10.5858/133.4.628
36. Konijn AM. Iron metabolism in inflammation. Baillieres Clin Haematol (1994) 7(4):829–49. doi: 10.1016/S0950-3536(05)80127-1
37. Ponikowska B, Suchocki T, Paleczny B, Olesinska M, Powierza S, Borodulin-Nadzieja L, et al. Iron status and survival in diabetic patients with coronary artery disease. Diabetes Care (2013) 36(12):4147–56. doi: 10.2337/dc13-0528
Keywords: inflammatory indicator, coronary heart disease, intensive care unit, MIMIC, eICU, mortality, nomogram
Citation: Cheng Y, Chen Y, Mao M, Wang R, Zhu J and He Q (2023) Association of inflammatory indicators with intensive care unit mortality in critically ill patients with coronary heart disease. Front. Immunol. 14:1295377. doi: 10.3389/fimmu.2023.1295377
Received: 16 September 2023; Accepted: 30 October 2023;
Published: 14 November 2023.
Edited by:
Pål Aukrust, Oslo University Hospital, NorwayReviewed by:
Wenyue Cao, Huazhong University of Science and Technology, ChinaCopyright © 2023 Cheng, Chen, Mao, Wang, Zhu and He. This is an open-access article distributed under the terms of the Creative Commons Attribution License (CC BY). The use, distribution or reproduction in other forums is permitted, provided the original author(s) and the copyright owner(s) are credited and that the original publication in this journal is cited, in accordance with accepted academic practice. No use, distribution or reproduction is permitted which does not comply with these terms.
*Correspondence: Qing He, MTIzaHFAMTYzLmNvbQ==
†These authors have contributed equally to this work
Disclaimer: All claims expressed in this article are solely those of the authors and do not necessarily represent those of their affiliated organizations, or those of the publisher, the editors and the reviewers. Any product that may be evaluated in this article or claim that may be made by its manufacturer is not guaranteed or endorsed by the publisher.
Research integrity at Frontiers
Learn more about the work of our research integrity team to safeguard the quality of each article we publish.