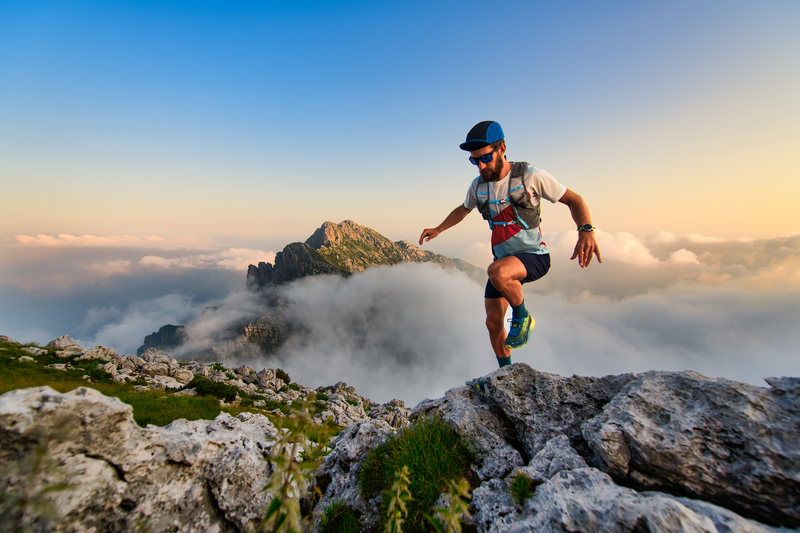
94% of researchers rate our articles as excellent or good
Learn more about the work of our research integrity team to safeguard the quality of each article we publish.
Find out more
SYSTEMATIC REVIEW article
Front. Immunol. , 26 September 2023
Sec. Cancer Immunity and Immunotherapy
Volume 14 - 2023 | https://doi.org/10.3389/fimmu.2023.1265202
This article is part of the Research Topic Novel Biomarkers in Tumor Immunity and Immunotherapy View all 49 articles
Background: Accurate prediction of efficacy of programmed cell death 1 (PD-1)/programmed cell death ligand 1 (PD-L1) checkpoint inhibitors is of critical importance. To address this issue, a network meta-analysis (NMA) comparing existing common measurements for curative effect of PD-1/PD-L1 monotherapy was conducted.
Methods: We searched PubMed, Embase, the Cochrane Library database, and relevant clinical trials to find out studies published before Feb 22, 2023 that use PD-L1 immunohistochemistry (IHC), tumor mutational burden (TMB), gene expression profiling (GEP), microsatellite instability (MSI), multiplex IHC/immunofluorescence (mIHC/IF), other immunohistochemistry and hematoxylin-eosin staining (other IHC&HE) and combined assays to determine objective response rates to anti–PD-1/PD-L1 monotherapy. Study-level data were extracted from the published studies. The primary goal of this study was to evaluate the predictive efficacy and rank these assays mainly by NMA, and the second objective was to compare them in subgroup analyses. Heterogeneity, quality assessment, and result validation were also conducted by meta-analysis.
Findings: 144 diagnostic index tests in 49 studies covering 5322 patients were eligible for inclusion. mIHC/IF exhibited highest sensitivity (0.76, 95% CI: 0.57-0.89), the second diagnostic odds ratio (DOR) (5.09, 95% CI: 1.35-13.90), and the second superiority index (2.86). MSI had highest specificity (0.90, 95% CI: 0.85-0.94), and DOR (6.79, 95% CI: 3.48-11.91), especially in gastrointestinal tumors. Subgroup analyses by tumor types found that mIHC/IF, and other IHC&HE demonstrated high predictive efficacy for non-small cell lung cancer (NSCLC), while PD-L1 IHC and MSI were highly efficacious in predicting the effectiveness in gastrointestinal tumors. When PD-L1 IHC was combined with TMB, the sensitivity (0.89, 95% CI: 0.82-0.94) was noticeably improved revealed by meta-analysis in all studies.
Interpretation: Considering statistical results of NMA and clinical applicability, mIHC/IF appeared to have superior performance in predicting response to anti PD-1/PD-L1 therapy. Combined assays could further improve the predictive efficacy. Prospective clinical trials involving a wider range of tumor types are needed to establish a definitive gold standard in future.
Since the approval of anti-PD-1/PD-L1 inhibitors in the treatment of melanoma in 2014, the overall survival of patients has improved significantly. However, anti-PD-1/PD-L1 immunotherapy still has many shortcomings, such as PD-1/L1-induced immune-related adverse events (irAEs) and hyperprogression (1). It is important to predict patients’ response to PD-1/PD-L1 immunotherapy based on the consideration of medical economics.
Various testing assays have been approved to predict the efficacy of anti-PD-1/PD-L1 immunotherapy response. Food and Drug Administration (FDA) has approved PD-1/PD-L1 IHC, TMB, proficient mismatch repair (pMMR) proteins, deficient mismatch repair (dMMR), and MSI-high (MSI-H) for specific tumor types and drugs as companion or complementary diagnostics (2). Similarly, European Communities (CE) and National Medical Products Administration (NMPA) have carried out their own standards on companion diagnostics and prediction assay applications.
PD-L1 IHC, the first approved companion diagnostic biomarker, aims to detect PD-1/PD-L1 expression on tumor cells or inflammatory cells. However, the efficacy of IHC may be influenced by the experience of pathologists, tumor types examined, and the used scoring methods. Researchers are now exploring the optimal detecting assay and scoring methods for specific tumors (3).
TMB has been found to increase neoantigens of major histocompatibility complexes (MHC) in various cancers, which leading to better immunotherapy response in patients. Increasing evidence indicates that different tumor types own various expression levels of TMB. TMB is usually assessed by next-generation sequencing (NGS) platforms, though standards of threshold and application methods need to be defined exactly to enhance accuracy across different tumor types. This would entail considerations such as genome coverage, workflow, and appropriate cutoff values (4). MSI and GEP display the difference in gene expression as well. MSI-H phenotype arises from numerous frameshift mutations due to deficits of the MMR system (5). Patients with MSI-H are more likely to suffer from various cancers, including colorectal cancer. MMR proteins, which could be detected by IHC, polymerase chain reaction (PCR), and gene sequencing, are now being used to identify MSI-H patients in various cancer types.
Detection and evaluation of tumor microenvironment (TME) have also been explored in recent years (6). For example, researchers have found that the epithelial-mesenchymal transition (EMT)- and stroma-related gene expression status is related to patients’ tumorigenesis and drug resistance (7, 8). mIHC/IF and gene sequencing technique could offer more chances to verify (9). GEP could also allow the integrations of different gene signatures and training models to predict prognosis and drug response based on the results of DNA-microarray and RNA sequencing (RNA-Seq) (10–12). Some researchers have also explored the combined approaches, such as TMB+GEP or TMB+IHC, since such predictors could work through different mechanisms or may be positively correlated with each other. All biomarker assays mentioned above present novel opportunities to predict the response rate of PD-1/PD-L1 inhibitors.
Assessment and evaluation of diagnostic tests could also benefit from the increasing diagnostic test accuracy (DTA) studies and the continuous development of statistical methods. In the era of evidence-based medicine, meta-analysis plays an important role in integrating of different studies with pairs of intervention using various methodological methods. To enable the comparison of different assays with limited data and generate a whole scale ranking results, NMA turned out to be a better tool to indirectly compare and jointly analyze three or more DTA studies simultaneously.
In this study, we compared the diagnostic accuracy of seven biomarker testing assays, including PD-L1 IHC, TMB, GEP, MSI, mIHC/IF, other IHC&HE, as well as combined assays for predicting anti-PD-1/PD-L1 immunotherapeutic response. Diagnostic accuracy measures used in this study included sensitivity, specificity, relative sensitivity, relative specificity, PPV, NPV, relative predictive values, DOR, and superiority index (13). It is believed that the NMA performed here could provide stronger clinical evidence for current medical practice.
This NMA was performed according to the Preferred Reporting Items for Systematic Reviews and Meta-analyses (PRISMA) NMA checklist.
The included research articles in this study were based on real-world data, and English translations were available. The studies were required to conduct PD-1/PD-L1 monotherapies and utilize at least two predictive biomarker testing assays on pre-treatment tissue samples. These assays could include PD-L1 IHC, TMB, GEP, MSI, mIHC/IF, HE for tumor-infiltrating lymphocytes (TIL), or other IHC methods. Each biomarker testing assay should provide sufficient information to determine the objective response rate (ORR) or non-progression rate (NPR) and allow for the calculation of sensitivity and specificity. If any testing assay had fewer than 15 tissue samples, it would not be considered. Hematologic cancers and flow cytometry studies on tumor lysates were excluded.
We systematically searched PubMed, Embase, and the Cochrane Library database for relevant studies and their errata (till February 2023). Additionally, we manually searched articles related to relevant clinical trials. For example, the search formula of Embase included: (“Immunohistochemistry “ OR “ Tumor mutational burden “ OR “ gene expression profiling “ OR “ multiplex immunofluorescence “ OR “ neoantigen load “ OR “ Immunofluorescence “)[Find articles with these terms] AND (“Pembrolizumab “ OR “ Nivolumab “ OR “ Durvalumab “ OR “ Toripalimab “ OR “ Camrelizumab “ OR “ Atezolizumab “ OR “ Avelumab “ OR “ Avelumab “ OR “ Budigalimab “)[Title, abstract or author-specified keywords] AND (Research articles)[Filter]. The intact search formula and results were in the Supplementary material.
Necessary information from eligible studies was extracted by three researchers independently and all inconsistencies were settled by discussion. The trial name, first author, year of publication, sample size, trial phase, tumor type, PD-1/PD-L1 antibody, and index test assay was recorded. To calculate sensitivity and specificity for each index test, we organized ORR-related information into a 2x2 table. We used Youden’s index, which combines values for sensitivity and specificity to indicate test accuracy, to select the best-performing threshold among multiple thresholds. If a clinical trial has multiply publications, the one with most complete information was adopted.
The main outcomes were calculated by NMA. As for Bayesian NMA, the ANOVA model made it possible to use the original data and arm-based (AB) model (14). The latter shows superiority to contrast-based (CB) models by accommodating more complex variance-covariance structures. NMA was mainly performed with the R package “Rstan” (R version 4.2.2). In order to improve accuracy and compare diagnostic assays one by one, calculations were repeated 7 times (model_code = model, chains = 2, iterations = 10000, warmup = 5000, thin = 5), and then, we draw league tables for relative comparations. Given numerical variance, we chose the median of sensitivity, specificity, PPV, NPV, SROC, and superiority index.
The Midas module for DTA meta-analysis facilitated validation of results and assessment of heterogeneity by forest plot and I2 analysis for every 7 biomarker modalities. Sensitivity, specificity, DOR, and summary receiver operating characteristic (SROC) curves and their associated area under the curve (AUC) were analyzed by Midas, which employs a bivariate mixed-effects logistic regression modeling framework and empirical Bayesian predictions. Publication bias of studies was also evaluated by Deeks’ funnel plot asymmetry test (p<0.05 indicating significant asymmetry). The network graphs package on Stata were used to draw the network graphs. Meta-analysis and drawing figures were fulfilled in Stata (17.0 MP—Parallel Edition).
The QUADAS-C (Quality Assessment of Diagnostic Accuracy Study) tool was used to assess the risk of bias and applicability in each selected study. There were 4 sections for risk of bias: patient selection, index test, reference standard, and flow and timing; meanwhile, concerns regarding applicability were presented in 3 sections: patient selection, index test, and reference standard.
3652 articles from databases and an additional 304 articles related to clinical trials were retrieved in total. After removing duplicates and glancing at the abstracts and titles, 294 articles were identified for full-text scrutiny. The literature search and study selection flow were recorded in Figure 1. Ultimately, a total of 49 studies involving 5322 patients were included in our analysis. 144 diagnostic index tests were extracted across all 49 studies, comprising PD-L1 IHC (n=46) (15–58), TMB (n=27) (15–33, 58–62), combined assays (n=22) (7, 16, 18, 20, 23, 31, 34–38, 61, 62), other IHC&HE (n=19) (7, 16–18, 21, 30, 33–35, 37–45), MSI (n=13) (21, 39, 46-53, 58, 61), GEP (n=13) (7, 16, 20, 23, 51, 53–56, 60, 62) and mIHC/IF (n=4)(36, 37, 43, 57). HE staining was used to score TIL. The situation where testing assays had been directly compared was represented by a network plot (Figure 2). 15 types of tumors accounted for the majority of the studies, while 7 studies (18, 20, 27, 31, 42, 60, 61) involved several solid tumors. 8 of 13 MSI tests (39, 46, 47, 50–53, 58) detected gastrointestinal cancer. The summary of included articles and details of studies can be found in Supplementary Tables 1, 2.
Figure 1 Flowchart Showing Literature Search and Study Selection. The study process followed the PRISMA guidelines. NMA, network meta-analysis.
Figure 2 Network Plot. Both nodes and lines are weighted according to the number of studies involved in each treatment and direct comparison, respectively. PD-L1 IHC, Programmed cell death ligand 1 immunohistochemistry; TMB, Tumor mutational burden; GEP, Gene expression profiling; MSI, Microsatellite instability; mIHC/IF, Multiplex immunohistochemistry/immunofluorescence; other IHC&HE, Other Immunohistochemistry and hematoxylin-eosin staining.
The sensitivity and specificity of NMA were summarized in Table 1. Among the diagnostic index tests, mIHC/IF (0.76, 95% CI: 0.57-0.89) exhibited the highest sensitivity, whereas GEP (0.52, 95% CI: 0.42-0.63), multi-assay (0.46, 95% CI: 0.39-0.52) and MSI (0.42, 95% CI: 0.30-0.53) have low efficacy. Other IHC&HE (0.66, 95% CI: 0.57-0.73), PD-L1 IHC (0.63, 95% CI: 0.59-0.67), and TMB (0.62, 95% CI: 0.56-0.68) presented similar sensitivities to rule out stable disease and progressive disease. As for specificity, MSI (0.90, 95% CI: 0.85-0.94) and combined assays (0.84, 95% CI: 0.79-0.87) performed better than the others. The specificities of the remaining testing assays were quite close, with TMB, other IHC&HE, PD-L1 IHC, GEP, and mIHC/IF having specificities of 0.65 (95% CI: 0.60-0.70), 0.63 (95% CI: 0.55-0.69), 0.61 (95% CI: 0.58-0.64), 0.61 (95% CI: 0.52-0.69) and 0.57 (95% CI: 0.39-0.73), respectively.
Table 1 Sensitivity, specificity, PPV, NPV, and diagnostic odds ratio (DOR) and superiority index by network meta-analysis.
Table 1 also revealed that the PPV for each assay was below 0.60, indicating that positive results may not correctly predict the response to PD-1/PD-L1 checkpoint inhibitors. MSI (0.56, 95% CI: 0.45-0.67) had the highest PPV, while GEP (0.33, 95% CI: 0.28-0.38) was the lowest. However, all assays provided relatively good performance in NPV, with even the lowest being near 0.80 (GEP: 0.8, 95% CI: 0.77-0.83). This suggested that these assays were useful in providing evidence to refuse immunologic therapy due to the accuracy of figuring out non-responsive patients.
Relative sensitivity, relative specificity, relative PPV, and relative NPV were shown in the league table (Table 2). From the league table for relative sensitivity (lower triangle of Table 2 (A), we can see that mIHC/IF, other IHC&HE, and PD-L1 IHC had similar efficacy and performed better than TMB, GEP, combined assays, and MSI according to the relative risk (RR) values. The upper triangle of Table 2(A) represented the relative specificity, MSI and multi-assay showed superiority to the other, meanwhile, the remaining tests exhibited comparable efficacy. Similarly, MSI and combined assays demonstrated higher relative PPVs among assays, as shown in the lower triangle of Table 2(B). There was no difference among relative NPVs (upper triangle of Table 2(B).
Table 2 Relative sensitivity, relative specificity, relative PPV, and relative NPV by network meta-analysis.
Table 1 presented the odds of responsive patients in test positives versus the odds of responsive patients in test negatives as measured by the DOR. MSI (6.79, 95% CI: 3.48-11.91) has the highest DOR as its high specificity, followed by mIHC/IF (4.44, 95% CI: 3.19-5.93), largely driven by its high sensitivity. In contrast, the DOR for gene expression profiling (GEP) was noticeably lower at 1.81 (95% CI: 1.31-2.40). The high superiority index indicated biomarkers modality performs comparatively well in both sensitivity and specificity. In contrast, the low superiority index represents biomarkers that had a poor performance of at least one assessment measure. As Table 1 summarized, the ranks of superiority index from highest to lowest were TMB, mIHC/IF, other IHC&HE, MSI, PD-L1 IHC, combined assays, and GEP.
To further validate these present results, a meta-analysis was conducted and revealed the same ranks of sensitivity, specificity, and DOR as NMA (Table 3). The value of sensitivity and specificity were very similar, indicating reliable results from the ANOVA model used in the NMA. SROC generated through meta-analysis displayed the AUC for each biomarker testing assay. mIHC/IF had the largest AUC (0.80), while GEP exhibited the smallest (0.61) and AUC of all others were close to 0.70 (Figure 3). Ranking trends for AUC and DOR were similar, indicating the reliability of our ranking results for NMA.
Figure 3 SROC Plot of “mIHC/IF” “combined assays” “MSI “ “TMB” “other IHC&HE” “PDL1 IHC” and “GEP” by Meta-analysis. SROC, Summary receiver operating characteristic curves; AUC, Area under the curve; PD-L1 IHC, Programmed cell death ligand 1 immunohistochemistry; TMB, Tumor mutational burden; GEP, Gene expression profiling; MSI, Microsatellite instability; mIHC/IF, Multiplex immunohistochemistry/immunofluorescence; other IHC&HE, Other Immunohistochemistry and hematoxylin-eosin staining.
However, the heterogeneity for each biomarker was high due to the absence of testing standards and various tumor types and thresholds. Although we chose the best performance threshold, I2 was higher than 50% (Supplementary Figure 1). Nonetheless, publication bias wasn’t obvious (p>0.1), according to Supplementary Figure 2. QUADAS-C tools allowed us to evaluate the quality (Supplementary Table 3).
We conducted NMA for two subgroups of studies: 10 studies focused on non-small cell lung cancer (NSCLC) (7, 23, 32–34, 45, 54, 58) and 12 studies centered around gastrointestinal tumors (19, 33, 39, 46, 47, 50–53, 58, 59) as reported in Table 4 and Table 5. For NSCLC, mIHC/IF and multi-assay had high sensitivity (0.90, 95% CI: 0.44-1.00) and specificity (0.90, 95% CI: 0.84-0.95) separately. mIHC/IF, with only one study available, exhibited both high sensitivity and specificity (0.89, 95% CI: 0.69-0.98), suggesting its potential as a reliable biomarker modality. Further analysis based on the ranks of DOR and superiority index suggested mIHC/IF, multi-assay and other IHC&HE were better among the 6 testing assays investigated.
In the case of gastrointestinal cancers, MSI had high specificity (0.89, 95% CI: 0.82-0.92) and low sensitivity (0.40, 95% CI: 0.27-0.54). PD-L1 IHC along with other IHC&HE demonstrated relatively high DOR and superiority index, besides MSI.
Concerning that the majority of combined assays contained 3 models, namely, TMB+GEP (n=6) (16, 20, 23), TMB+PD-L1 IHC (n=6) (18, 20, 30), and PD-L1 IHC+other IHC&HE (n=5) (34–38). A meta-analysis was performed to explore sensitivity, specificity, DOR, and AUC (Supplementary Figure 3) in these models. TMB+PD-L1 IHC showed the best balance between sensitivity (0.89, 95% CI: 0.82-0.94) and specificity (0.68, 95% CI: 0.53-0.81) with high DOR (18, 95% CI: 9-37) and AUC (0.87, 95% CI: 0.84-0.90). Conversely, the other models yielded higher sensitivity but lower specificity compared to a single assay in the meta-analysis (Supplementary Figure 3).
In this article, we compared 7 common biomarker testing assays to assess their efficacy in predicting response to PD-1/PD-L1 checkpoint inhibitors. mIHC/IF had the highest sensitivity (0.76, 95% CI: 0.57-0.89) and AUC (0.80), the second highest DOR (5.09, 95% CI: 1.35-13.90) and superiority index (2.86), but relative lower specificity (0.57, 95% CI: 0.39-0.73). Although MSI exhibited the highest DOR (6.79, 95% CI: 3.48-11.91), its application is mainly limited to gastrointestinal tumors. Despite being the most commonly used method in clinical practice, PD-L1 IHC had not demonstrated obvious advantages in terms of sensitivity, specificity, DOR, as well as superiority index. Yet, when PD-L1 IHC is combined with TMB, a notable increase in sensitivity (0.89, 95% CI: 0.82-0.94) was observed.
Our conclusion is in alignment with those from a previous meta-analyses that had addressed similar topics (63, 64), which indicated that mIHC/IF was superior to PD-L1 IHC, TMB and GEP in predicting response to PD-1/PD-L1 checkpoint inhibitors and that combinatorial assays could improve predictive efficacy. Yet, to our best of knowledge, our study was the first to use NMA to demonstrate the objective benefits of mIHC/IF in predicting patients’ response to PD-1/PD-L1 checkpoint inhibitors. Upon stratifying by tumor types, we also observed that mIHC/IF had both remarkable sensitivity and specificity in NSCLC. PD-L1, mIHC/IF and IHC also manifested relatively high DOR and superiority index in gastrointestinal cancers, which further substantiated the strengths of mIHC/IF.
To address the challenge of ranking multiple diagnostic tests simultaneously, statistical scientists have developed several new models based on the Bayesian setting for NMA of DTA studies (65), since traditional meta-analysis and NMA of intervention were not efficient enough to handle this issue. Multivariate extensions of meta-analysis models of DTA had been applied to NMA. In addition, the ANOVA model used in this NMA could facilitate ORR to be compared indirectly and rank testing assays directly (14). Researchers could also compare multiple thresholds per testing assay using certain models (66).
High sensitivity, DOR, and AUC of mIHC/IF collectively indicated its superiority in identification of potential patients who may benefit most from immunotherapy. mIHC/IF facilitates the acquisition of quantitative multiplexed data, which plays a pivotal role in deciphering the intricate relationship between tumor cells, their microenvironment, and antigen expressions at the single-cell level. This capability assumes paramount importance in understanding tumorigenesis, cancer progression, and immunotherapy responses. In all instances of mIHC/IF index testing, CD8 was included, and T cell antigen expression was examined. Various studies have established a link between T cells’ cytotoxicity and pro-inflammatory activity with patients prognosis through its regulation of inherent immunological function by tumor antigens like CD8 or PD-1 (67–70), which further supports the potency of antigens on tumor-infiltrating lymphocytes (TILs). However, false negative results obtained from mIHC/IF screening may exclude some patients who may could benefit from immunotherapy, suggesting the need to explore additional proteins and combined assays to improve specificity. To enhance the precision in scoring staining, many researchers have incorporated artificial intelligence with mIHC/IF, rendering it a relatively convenient and cost-effective method when compared to combined assays (71). Thus, our study has concluded that mIHC/IF had the best performance and a broad range of applications.
PD-L1 IHC, the most widely used assay, exhibited suboptimal performance in sensitivity, specificity, and DOR. As previously mentioned, TME is excessively intricate and heterogeneous to be comprehensively elucidated by a singular mechanism. Furthermore, expressions of PD-1 and PD-L1 exhibit considerable interpatient variability. These two factors collectively contribute to the suboptimal performance of PD-L1 IHC as a predictive marker. The possible reasons for such unsatisfactory results varied, including the lack of experience for pathologists, sample type examined, and IHC assays used (72). A meta-analysis that scrutinized and compared different IHC assays using tumor proportion score (TPS) revealed that the sensitivity and specificity values were similar except SP142 with lower sensitivity (73). The quantification and assessment of PD-1 protein expression through scoring methods varied among different assays, such as TPS, combined positivity score (CPS), and immune cell (IC) score (3). Gastrointestinal tumors were characterized by their most extensive proportions of MSI-H/dMMR, therefore, MSI status detection could be a reasonable approach to predict the response to immunotherapy. Subgroup analysis of gastrointestinal tumors indicated that MSI detection offered a valuable method for ruling out non-responsive patients due to its high specificity performance. MSI detection was also conducted in other solid tumors, including endometrial cancer, adrenocortical carcinomas, and multiple endocrine neoplasias (MENs). High specificity, DOR, and AUC of MSI suggested its potential applications in some other tumor types. Regrettably, generalization of MSI detections to a wider range of tumors may be prevented by the fact that most tumors in fact exhibit microsatellite stability (MSS) status.
Our efficacy rankings placed TMB and other IHC&HE in the middle, while GEP was ranked last, although they are closely related to crucial aspects of tumor immunology such as neoantigen, TME, and inflammatory gene signature. Nevertheless, it is important to note that the MSI status, TMB, and GEP serve as indicators of the gene phenotype, which is not directly associated with the primary mechanism of PD-1/PD-L1 immunotherapy compared to protein expression. The measurements obtained through MSI, TMB, and GEP reflect events upstream of gene expression, which may potentially diminish their predictive efficacy. Uncovering specific and precise gene pathways solely through these indicators can prove to be challenging. Whereas thresholds for TMB and GEP were mainly determined by proportions, other IHC&HE methods typically detected CD8 and TILs with different methods. This highlights the potential impossibility that some immature tests could have covered all types of tumors.
Combined assays provided more chances to improve the prediction accuracy in current challenging scenario. When TMB was combined with PD-L1 IHC, the performance of sensitivity was improved noticeably without sacrificing specificity. Ricciuti, B. et al. have explored the association of high TMB with other biomarkers and found that high TMB was related to higher proportions of tumor-infiltrating CD8+, PD1+ T cells, and high PD-L1 expression in cancer cells (74). Fumet, J.-D. et al. reported that tumors displaying high PD-L1/low CD8 TILs developed microenvironments conducive to tumor proliferation and exhibited poor outcomes (75). This may explain the enhanced efficacy of combined assays. Yet, the shortcomings of combined assays were high cost and technical complexity.
Despite nearly a decade of research on companion or complementary diagnostics for prediction purposes, the most effective indicators for PD-1/PD-L1 inhibitors have not yet been established for most tumors. While some testing assays such as mIHC/IF and combined tests hold potential values, there was still no perfect test with satisfactory sensitivity and specificity simultaneously in our analysis. Consequently, clinicians should exert appropriate caution when detecting predictive biomarkers and interpreting associated results. Additionally, it is believed that our NMA could provide supporting evidence to researchers and clinicians for amelioration of predictive tests in future.
It is crucial to note that a high ORR doesn’t necessarily translate into a high OS. It is essential to take care when interpreting results based on studies that relied solely on ORR which may not take into account of OS or progressive rate. To mitigate bias, it is worth noting that the threshold we chose with Youden’s index may favor higher sensitivity and specificity. An article with two or more biomarker tests was selected, which may cause bias by giving up some robust data in each test. Moreover, there was a significant disparity between the number of studies conducted in PD-L1 IHC versus mIHC/IF. Last but not least, although our study mainly covered 15 types of tumors, the generalization of the conclusion still requires deliberation.
Various large prospective and retrospective studies have investigated biomarkers for the prediction of PD-1/PD-L1 checkpoint inhibitors response. According to our network meta-analysis, mIHC/IF had the best performance and a large range of applications. Given the diverse employment of mIHC/IF with different biomarkers across various studies, further investigations involving precise combinations are warranted to enhance prognostic prediction. When considering the selection of specific markers, it is crucial to take into account not only their efficiency and cost-effectiveness but also rely on substantiation from evidence derived from molecular mechanisms. Further exploration was required in combined assays of the high efficacy of TMB+PD-L1 IHC. Currently, there is a lack of studies or consensus regarding the workflow of companion or complementary diagnostics in this context. The existing approach is primarily based on clinicians’ acknowledgment, and we anticipate that future research will provide more foundational evidence to support these practices. What’ more, more evidence based medicine are needed to determine detailed testing modalities and thresholds for all types of tumors, e.g. advanced ovarian cancer. Clinicians should be cautious that the prognostic accuracy of each index test should be interpreted in a particular situation.
The original contributions presented in the study are included in the article/Supplementary Material. Further inquiries can be directed to the corresponding author.
HS: Conceptualization, Data curation, Formal Analysis, Investigation, Writing – original draft. WZ: Investigation, Validation, Visualization, Writing – review & editing. LZ: Investigation, Validation, Visualization, Writing – review & editing. YZ: Conceptualization, Data curation, Formal Analysis, Supervision, Writing – review & editing. TD: Conceptualization, Funding acquisition, Software, Supervision, Writing – review & editing.
The author(s) declare financial support was received for the research, authorship, and/or publication of this article. This work was supported by Innovation and Development Joint Funds of Natural Science Foundation of Shandong Province [ZR2021LZL009] and National Natural Science Foundation of China [82303956].
We acknowledge all the authors whose papers have been included in this network meta-analysis for their work, which made this analysis possible.
The authors declare that the research was conducted in the absence of any commercial or financial relationships that could be construed as a potential conflict of interest.
All claims expressed in this article are solely those of the authors and do not necessarily represent those of their affiliated organizations, or those of the publisher, the editors and the reviewers. Any product that may be evaluated in this article, or claim that may be made by its manufacturer, is not guaranteed or endorsed by the publisher.
The Supplementary Material for this article can be found online at: https://www.frontiersin.org/articles/10.3389/fimmu.2023.1265202/full#supplementary-material
1. Makuku R, Khalili N, Razi S, Keshavarz-Fathi M, Rezaei N. Current and future perspectives of PD-1/PDL-1 blockade in cancer immunotherapy. J Immunol Res (2021) 2021:6661406. doi: 10.1155/2021/6661406
2. Health C for D and R. List of cleared or approved companion diagnostic devices (In vitro and imaging tools) . FDA. Available at: https://www.fda.gov/medical-devices/in-vitro-diagnostics/list-cleared-or-approved-companion-diagnostic-devices-in-vitro-and-imaging-tools (Accessed February 22, 2023).
3. Paver EC, Cooper WA, Colebatch AJ, Ferguson PM, Hill SK, Lum T, et al. Programmed death ligand-1 (PD-L1) as a predictive marker for immunotherapy in solid tumours: a guide to immunohistochemistry implementation and interpretation. Pathology (2021) 53(2):141–56. doi: 10.1016/j.pathol.2020.10.007
4. Sha D, Jin Z, Budzcies J, Kluck K, Stenzinger A, Sinicrope FA. Tumor mutational burden as a predictive biomarker in solid tumors. Cancer Discovery (2020) 10(12):1808–25. doi: 10.1158/2159-8290.CD-20-0522
5. Baretti M, Le DT. DNA mismatch repair in cancer. Pharmacol Ther (2018) 189:45–62. doi: 10.1016/j.pharmthera.2018.04.004
6. Erin N, Grahovac J, Brozovic A, Efferth T. Tumor microenvironment and epithelial mesenchymal transition as targets to overcome tumor multidrug resistance. Drug Resistance Updates (2020) 53:100715. doi: 10.1016/j.drup.2020.100715
7. Wang L, Saci A, Szabo PM, Chasalow SD, Castillo-Martin M, Domingo-Domenech J, et al. EMT- and stroma-related gene expression and resistance to PD-1 blockade in urothelial cancer. Nat Commun (2018) 9(1):3503. doi: 10.1038/s41467-018-05992-x
8. Hugo W, Zaretsky JM, Sun L, Song C, Moreno BH, Hu-Lieskovan S, et al. Genomic and transcriptomic features of response to anti-PD-1 therapy in metastatic melanoma. Cell (2017) 168(3):542. doi: 10.1016/j.cell.2017.01.010
9. Gide TN, Quek C, Menzies AM, Tasker AT, Shang P, Holst J, et al. Distinct immune cell populations define response to anti-PD-1 monotherapy and anti-PD-1/Anti-CTLA-4 combined therapy. Cancer Cell (2019) 35(2):238–255.e6. doi: 10.1016/j.ccell.2019.01.003
10. Fan C, Oh DS, Wessels L, Weigelt B, Nuyten DSA, Nobel AB, et al. Concordance among Gene-Expression–based predictors for breast cancer. New Engl J Med (2006) 355:560–9.
11. Jamin SP, Hikmet F, Mathieu R, Jégou B, Lindskog C, Chalmel F, et al. Combined RNA/tissue profiling identifies novel cancer/testis genes. Mol Oncol (2021) 15(11):3003–23. doi: 10.1002/1878-0261.12900
12. Ayers M, Lunceford J, Nebozhyn M, Murphy E, Loboda A, Kaufman DR, et al. IFN-γ-related mRNA profile predicts clinical response to PD-1 blockade. J Clin Invest (2017) 127(8):2930–40. doi: 10.1172/JCI91190
13. Deutsch R, Mindt MR. Quantifying relative superiority among many binary-valued diagnostic tests in the presence of a gold standard. J Data Science (2021) 7:161–77. doi: 10.6339/JDS.2009.07(2).450
14. Nyaga VN, Aerts M, Arbyn M. ANOVA model for network meta-analysis of diagnostic test accuracy data. Stat Methods Med Res (2018) 27(6):1766–84. doi: 10.1177/0962280216669182
15. Hellmann MD, Callahan MK, Awad MM, Calvo E, Ascierto PA, Atmaca A, et al. Tumor mutational burden and efficacy of nivolumab monotherapy and in combination with ipilimumab in small-cell lung cancer. Cancer Cell (2018) 33(5):853–861.e4. doi: 10.1016/j.ccell.2018.04.001
16. Hodi FS, Wolchok JD, Schadendorf D, Larkin J, Long GV, Qian X, et al. TMB and inflammatory gene expression associated with clinical outcomes following immunotherapy in advanced melanoma. Cancer Immunol Res (2021) 9(10):1202–13. doi: 10.1158/2326-6066.CIR-20-0983
17. Hamid O, Molinero L, Bolen CR, Sosman JA, Muñoz-Couselo E, Kluger HM, et al. Safety, clinical activity, and biological correlates of response in patients with metastatic melanoma: Results from a phase i trial of atezolizumab. Clin Cancer Res (2019) 25(20):6061–72. doi: 10.1158/1078-0432.CCR-18-3488
18. Tang B, Yan X, Sheng X, Si L, Cui C, Kong Y, et al. Safety and clinical activity with an anti-PD-1 antibody JS001 in advanced melanoma or urologic cancer patients. J Hematol Oncol (2019) 12(1):7. doi: 10.1186/s13045-018-0693-2
19. Huang J, Mo H, Zhang W, Chen X, Qu D, Wang X, et al. Promising efficacy of SHR-1210, a novel anti-programmed cell death 1 antibody, in patients with advanced gastric and gastroesophageal junction cancer in china. Cancer (2019) 125(5):742–9. doi: 10.1002/cncr.31855
20. Cristescu R, Mogg R, Ayers M, Albright A, Murphy E, Yearley J, et al. Pan-tumor genomic biomarkers for PD-1 checkpoint blockade-based immunotherapy. Science (2018) 362(6411):eaar3593. doi: 10.1126/science.aar3593
21. Tanizaki J, Yonemori K, Akiyoshi K, Minami H, Ueda H, Takiguchi Y, et al. Open-label phase II study of the efficacy of nivolumab for cancer of unknown primary. Ann Oncol (2022) 33(2):216–26. doi: 10.1016/j.annonc.2021.11.009
22. Galsky MD, Saci A, Szabo PM, Azrilevich A, Horak C, Lambert A, et al. Impact of zumor mutation burden on nivolumab efficacy in second-line urothelial carcinoma patients: Exploratory analysis of the phase ii checkmate 275 study. Ann Oncol (2017) 28:v296–7. doi: 10.1093/annonc/mdx371.003
23. Shim JH, Kim HS, Cha H, Kim S, Kim TM, Anagnostou V, et al. HLA-corrected tumor mutation burden and homologous recombination deficiency for the prediction of response to PD-(L)1 blockade in advanced non-small-cell lung cancer patients. Ann Oncol (2020) 31(7):902–11. doi: 10.1016/j.annonc.2020.04.004
24. Hanna GJ, Lizotte P, Cavanaugh M, Kuo FC, Shivdasani P, Frieden A, et al. Frameshift events predict anti-PD-1/L1 response in head and neck cancer. JCI Insight (2018) 3(4):e98811. doi: 10.1172/jci.insight.98811
25. Carbone DP, Reck M, Paz-Ares L, Creelan B, Horn L, Steins M, et al. First-line nivolumab in stage IV or recurrent non-Small-Cell lung cancer. N Engl J Med (2017) 376(25):2415–26. doi: 10.1056/NEJMoa1613493
26. Lu M, Zhang P, Zhang Y, Li Z, Gong J, Li J, et al. Efficacy, safety, and biomarkers of toripalimab in patients with recurrent or metastatic neuroendocrine neoplasms: A multiple-center phase ib trial. Clin Cancer Res (2020) 26(10):2337–45. doi: 10.1158/1078-0432.CCR-19-4000
27. Liu D, Ma C, Lu P, Gong J, Ye D, Wang S, et al. Dose escalation and expansion (phase Ia/Ib) study of GLS-010, a recombinant fully human antiprogrammed death-1 monoclonal antibody for advanced solid tumors or lymphoma. Eur J Cancer (2021) 148:1–13. doi: 10.1016/j.ejca.2021.01.020
28. Gogas H, Dréno B, Larkin J, Demidov L, Stroyakovskiy D, Eroglu Z, et al. Cobimetinib plus atezolizumab in BRAFV600 wild-type melanoma: primary results from the randomized phase III IMspire170 study. Ann Oncol (2021) 32(3):384–94. doi: 10.1016/j.annonc.2020.12.004
29. Stratigos AJ, Sekulic A, Peris K, Bechter O, Prey S, Kaatz M, et al. Cemiplimab in locally advanced basal cell carcinoma after hedgehog inhibitor therapy: an open-label, multi-centre, single-arm, phase 2 trial. Lancet Oncol (2021) 22(6):848–57. doi: 10.1016/S1470-2045(21)00126-1
30. D’Angelo SP, Bhatia S, Brohl AS, Hamid O, Mehnert JM, Terheyden P, et al. Avelumab in patients with previously treated metastatic merkel cell carcinoma: long-term data and biomarker analyses from the single-arm phase 2 JAVELIN merkel 200 trial. J Immunother Cancer (2020) 8(1):e000674. doi: 10.1136/jitc-2020-000674
31. Marabelle A, Fakih M, Lopez J, Shah M, Shapira-Frommer R, Nakagawa K, et al. Association of tumour mutational burden with outcomes in patients with advanced solid tumours treated with pembrolizumab: prospective biomarker analysis of the multicohort, open-label, phase 2 KEYNOTE-158 study. Lancet Oncol (2020) 21(10):1353–65. doi: 10.1016/S1470-2045(20)30445-9
32. Hayashi H, Sugawara S, Fukuda Y, Fujimoto D, Miura S, Ota K, et al. A randomized phase II study comparing nivolumab with carboplatin-pemetrexed for EGFR-mutated NSCLC with resistance to EGFR tyrosine kinase inhibitors (WJOG8515L). Clin Cancer Res (2022) 28(5):893–902. doi: 10.1158/1078-0432.CCR-21-3194
33. Rizvi NA, Hellmann MD, Snyder A, Kvistborg P, Makarov V, Havel JJ, et al. Cancer immunology. mutational landscape determines sensitivity to PD-1 blockade in non-small cell lung cancer. Science (2015) 348(6230):124–8. doi: 10.1126/science.aaa1348
34. Shimoda Y, Shibaki R, Yoshida T, Murakami S, Shirasawa M, Torasawa M, et al. Concurrent high PD-L1 expression and CD8+ immune cell infiltration predict PD-1 blockade efficacy in advanced EGFR-mutant NSCLC patients. Clin Lung Cancer (2022) 23(6):477–86. doi: 10.1016/j.cllc.2022.04.001
35. Raghav KP, Stephen B, Karp DD, Piha-Paul SA, Hong DS, Jain D, et al. Efficacy of pembrolizumab in patients with advanced cancer of unknown primary (CUP): a phase 2 non-randomized clinical trial. J Immunother Cancer (2022) 10(5):e004822. doi: 10.1136/jitc-2022-004822
36. Ficial M, Jegede OA, Sant’Angelo M, Hou Y, Flaifel A, Pignon JC, et al. Expression of t-cell exhaustion molecules and human endogenous retroviruses as predictive biomarkers for response to nivolumab in metastatic clear cell renal cell carcinoma. Clin Cancer Res (2021) 27:1371–80. doi: 10.1158/1078-0432.CCR-20-3084
37. Pignon JC, Jegede O, Shukla SA, Braun DA, Horak CE, Wind-Rotolo M, et al. irRECIST for the evaluation of candidate biomarkers of response to nivolumab in metastatic clear cell renal cell carcinoma: Analysis of a phase II prospective clinical trial. Clin Cancer Res (2019) 25(7):2174–84. doi: 10.1158/1078-0432.CCR-18-3206
38. Takada K, Takamori S, Yoneshima Y, Tanaka K, Okamoto I, Shimokawa M, et al. Serum markers associated with treatment response and survival in non-small cell lung cancer patients treated with anti-PD-1 therapy. Lung Cancer (2020) 145:18–26. doi: 10.1016/j.lungcan.2020.04.034
39. Pedersen KS, Foster NR, Overman MJ, Boland PM, Kim SS, Arrambide KA, et al. ZEBRA: A multicenter phase II study of pembrolizumab in patients with advanced small-bowel adenocarcinoma. Clin Cancer Res (2021) 27(13):3641–8. doi: 10.1158/1078-0432.CCR-21-0159
40. Miyama Y, Morikawa T, Miyakawa J, Koyama Y, Kawai T, Kume H, et al. Squamous differentiation is a potential biomarker predicting tumor progression in patients treated with pembrolizumab for urothelial carcinoma. Pathol Res Pract (2021) 219:153364. doi: 10.1016/j.prp.2021.153364
41. Ahmadzada T, Cooper WA, Holmes M, Mahar A, Westman H, Gill AJ, et al. Retrospective evaluation of the use of pembrolizumab in malignant mesothelioma in a real-world australian population. JTO Clin Res Rep (2020) 1(4):100075. doi: 10.1016/j.jtocrr.2020.100075
42. Naing A, Meric-Bernstam F, Stephen B, Karp DD, Hajjar J, Rodon Ahnert J, et al. Phase 2 study of pembrolizumab in patients with advanced rare cancers. J Immunother Cancer (2020) 8(1):e000347. doi: 10.1136/jitc-2019-000347
43. Kato K, Doki Y, Ura T, Hamamoto Y, Kojima T, Tsushima T, et al. Long-term efficacy and predictive correlates of response to nivolumab in japanese patients with esophageal cancer. Cancer Sci (2020) 111(5):1676–84. doi: 10.1111/cas.14380
44. Emens LA, Cruz C, Eder JP, Braiteh F, Chung C, Tolaney SM, et al. Long-term clinical outcomes and biomarker analyses of atezolizumab therapy for patients with metastatic triple-negative breast cancer: A phase 1 study. JAMA Oncol (2019) 5(1):74–82. doi: 10.1001/jamaoncol.2018.4224
45. Lambert SL, Zhang C, Guo C, Turan T, Masica DL, Englert S, et al. Association of baseline and pharmacodynamic biomarkers with outcomes in patients treated with the PD-1 inhibitor budigalimab. J Immunother (2022) 45(3):167–79. doi: 10.1097/CJI.0000000000000408
46. Kelly RJ, Lee J, Bang YJ, Almhanna K, Blum-Murphy M, Catenacci DVT, et al. Safety and efficacy of durvalumab and tremelimumab alone or in combination in patients with advanced gastric and gastroesophageal junction adenocarcinoma. Clin Cancer Res (2020) 26(4):846–54. doi: 10.1158/1078-0432.CCR-19-2443
47. Fuchs CS, Doi T, Jang RW, Muro K, Satoh T, Machado M, et al. Safety and efficacy of pembrolizumab monotherapy in patients with previously treated advanced gastric and gastroesophageal junction cancer: Phase 2 clinical KEYNOTE-059 trial. JAMA Oncol (2018) 4(5):e180013. doi: 10.1001/jamaoncol.2018.0013
48. Konstantinopoulos PA, Luo W, Liu JF, Gulhan DC, Krasner C, Ishizuka JJ, et al. Phase II study of avelumab in patients with mismatch repair deficient and mismatch repair proficient Recurrent/Persistent endometrial cancer. J Clin Oncol (2019) 37(30):2786–94. doi: 10.1200/JCO.19.01021
49. Raj N, Zheng Y, Kelly V, Katz SS, Chou J, Do RKG, et al. PD-1 blockade in advanced adrenocortical carcinoma. J Clin Oncol (2020) 38(1):71–80. doi: 10.1200/JCO.19.01586
50. Overman MJ, McDermott R, Leach JL, Lonardi S, Lenz HJ, Morse MA, et al. Nivolumab in patients with metastatic DNA mismatch repair-deficient or microsatellite instability-high colorectal cancer (CheckMate 142): an open-label, multicentre, phase 2. Lancet Oncol (2017) 18(9):1182–91. doi: 10.1016/S1470-2045(17)30422-9
51. Kim ST, Cristescu R, Bass AJ, Kim KM, Odegaard JI, Kim K, et al. Comprehensive molecular characterization of clinical responses to PD-1 inhibition in metastatic gastric cancer. Nat Med (2018) 24(9):1449–58. doi: 10.1038/s41591-018-0101-z
52. Eng C, Kim TW, Bendell J, Argilés G, Tebbutt NC, Di Bartolomeo M, et al. Atezolizumab with or without cobimetinib versus regorafenib in previously treated metastatic colorectal cancer (IMblaze370): a multicentre, open-label, phase 3, randomised, controlled trial. Lancet Oncol (2019) 20(6):849–61. doi: 10.1016/S1470-2045(19)30027-0
53. Kwon M, Hong JY, Kim ST, Kim KM, Lee J. Association of serine/threonine kinase 11 mutations and response to programmed cell death 1 inhibitors in metastatic gastric cancer. Pathol Res Pract (2020) 216(6):152947. doi: 10.1016/j.prp.2020.152947
54. Higgs BW, Morehouse CA, Streicher K, Brohawn PZ, Pilataxi F, Gupta A, et al. Interferon gamma messenger RNA signature in tumor biopsies predicts outcomes in patients with non-small cell lung carcinoma or urothelial cancer treated with durvalumab. Clin Cancer Res (2018) 24(16):3857–66. doi: 10.1158/1078-0432.CCR-17-3451
55. McDermott DF, Huseni MA, Atkins MB, Motzer RJ, Rini BI, Escudier B, et al. Clinical activity and molecular correlates of response to atezolizumab alone or in combination with bevacizumab versus sunitinib in renal cell carcinoma. Nat Med (2018) 24(6):749–57. doi: 10.1038/s41591-018-0053-3
56. Sharma P, Retz M, Siefker-Radtke A, Baron A, Necchi A, Bedke J, et al. Nivolumab in metastatic urothelial carcinoma after platinum therapy (CheckMate 275): a multicentre, single-arm, phase 2 trial. Lancet Oncol (2017) 18(3):312–22. doi: 10.1016/S1470-2045(17)30065-7
57. Yeong J, Suteja L, Simoni Y, Lau KW, Tan AC, Li HH, et al. Intratumoral CD39+CD8+ t cells predict response to programmed cell death protein-1 or programmed death ligand-1 blockade in patients with NSCLC. J Thorac Oncol (2021) 16(8):1349–58. doi: 10.1016/j.jtho.2021.04.016
58. Wang F, Wei XL, Wang FH, Xu N, Shen L, Dai GH, et al. Safety, efficacy and tumor mutational burden as a biomarker of overall survival benefit in chemo-refractory gastric cancer treated with toripalimab, a PD-1 antibody in phase Ib/II clinical trial NCT02915432. Ann Oncol (2019) 30(9):1479–86. doi: 10.1093/annonc/mdz197
59. Zeng D, Wu J, Luo H, Li Y, Xiao J, Peng J, et al. Tumor microenvironment evaluation promotes precise checkpoint immunotherapy of advanced gastric cancer. J Immunother Cancer (2021) 9(8):e002467. doi: 10.1136/jitc-2021-002467
60. Cindy Yang SY, Lien SC, Wang BX, Clouthier DL, Hanna Y, Cirlan I, et al. Pan-cancer analysis of longitudinal metastatic tumors reveals genomic alterations and immune landscape dynamics associated with pembrolizumab sensitivity. Nat Commun (2021) 12(1):5137. doi: 10.1038/s41467-021-25432-7
61. Friedman CF, Hainsworth JD, Kurzrock R, Spigel DR, Burris HA, Sweeney CJ, et al. Atezolizumab treatment of tumors with high tumor mutational burden from MyPathway, a multicenter, open-label, phase IIa multiple basket study. Cancer Discovery (2022) 12(3):654–69. doi: 10.1158/2159-8290.CD-21-0450
62. Cuppens K, Baas P, Geerdens E, Cruys B, Froyen G, Decoster L, et al. HLA-i diversity and tumor mutational burden by comprehensive next-generation sequencing as predictive biomarkers for the treatment of non-small cell lung cancer with PD-(L)1 inhibitors. Lung Cancer (2022) 170:1–10. doi: 10.1016/j.lungcan.2022.05.019
63. Lu S, Stein JE, Rimm DL, Wang DW, Bell JM, Johnson DB, et al. Comparison of biomarker modalities for predicting response to PD-1/PD-L1 checkpoint blockade: A systematic review and meta-analysis. JAMA Oncol (2019) 5(8):1195–204. doi: 10.1001/jamaoncol.2019.1549
64. Yarchoan M, Albacker LA, Hopkins AC, Montesion M, Murugesan K, Vithayathil TT, et al. PD-L1 expression and tumor mutational burden are independent biomarkers in most cancers. JCI Insight (2019) 4(6):e126908. doi: 10.1172/jci.insight.126908
65. Veroniki AA, Tsokani S, Rücker G, Mavridis D, Takwoingi Y. Challenges in comparative meta-analysis of the accuracy of multiple diagnostic tests. Methods Mol Biol (2022) 2345:299–316. doi: 10.1007/978-1-0716-1566-9_18
66. Owen RK, Cooper NJ, Quinn TJ, Lees R, Sutton AJ. Network meta-analysis of diagnostic test accuracy studies identifies and ranks the optimal diagnostic tests and thresholds for health care policy and decision-making. J Clin Epidemiol (2018) 99:64–74. doi: 10.1016/j.jclinepi.2018.03.005
67. Banchereau R, Chitre AS, Scherl A, Wu TD, Patil NS, de Almeida P, et al. Intratumoral CD103+ CD8+ t cells predict response to PD-L1 blockade. J Immunother Cancer (2021) 9(4):e002231. doi: 10.1136/jitc-2020-002231
68. Corgnac S, Malenica I, Mezquita L, Auclin E, Voilin E, Kacher J, et al. CD103+CD8+ TRM cells accumulate in tumors of anti-PD-1-Responder lung cancer patients and are tumor-reactive lymphocytes enriched with Tc17. Cell Rep Med (2020) 1(7):100127. doi: 10.1016/j.xcrm.2020.100127
69. Oja AE, Piet B, van der Zwan D, Blaauwgeers H, Mensink M, de Kivit S, et al. Functional heterogeneity of CD4+ tumor-infiltrating lymphocytes with a resident memory phenotype in NSCLC. Front Immunol (2018) 9:2654. doi: 10.3389/fimmu.2018.02654
70. Li Z, Zheng B, Qiu X, Wu R, Wu T, Yang S, et al. The identification and functional analysis of CD8+PD-1+CD161+ t cells in hepatocellular carcinoma. NPJ Precis Oncol (2020) 4:28–8. doi: 10.1038/s41698-020-00133-4
71. Berry S, Giraldo NA, Green BF, Cottrell TR, Stein JE, Engle EL, et al. Analysis of multispectral imaging with the AstroPath platform informs efficacy of PD-1 blockade. Science (2021) 372(6547):eaba2609. doi: 10.1126/science.aba2609
72. Doroshow DB, Bhalla S, Beasley MB, Sholl LM, Kerr KM, Gnjatic S, et al. PD-L1 as a biomarker of response to immune-checkpoint inhibitors. Nat Rev Clin Oncol (2021) 18(6):345–62. doi: 10.1038/s41571-021-00473-5
73. Torlakovic E, Lim HJ, Adam J, Barnes P, Bigras G, Chan AWH, et al. "Interchangeability" of PD-L1 immunohistochemistry assays: a meta-analysis of diagnostic accuracy. Mod Pathol (2020) 33(1):4–17. doi: 10.1038/s41379-019-0327-4
74. Ricciuti B, Wang X, Alessi JV, Rizvi H, Mahadevan NR, Li YY, et al. Association of high tumor mutation burden in non-small cell lung cancers with increased immune infiltration and improved clinical outcomes of PD-L1 blockade across PD-L1 expression. JAMA Oncol (2022) 8(8):1160–8. doi: 10.1001/jamaoncol.2022.1981
Keywords: anti-PD-1/PD-L1 inhibitors immunotherapy, biomarkers, predictive value of tests, solid tumor, meta-analysis
Citation: Shi H, Zhang W, Zhang L, Zheng Y and Dong T (2023) Comparison of different predictive biomarker testing assays for PD-1/PD-L1 checkpoint inhibitors response: a systematic review and network meta-analysis. Front. Immunol. 14:1265202. doi: 10.3389/fimmu.2023.1265202
Received: 22 July 2023; Accepted: 08 September 2023;
Published: 26 September 2023.
Edited by:
Eyad Elkord, University of Salford, United KingdomReviewed by:
Xin Yu, Merck, United StatesCopyright © 2023 Shi, Zhang, Zhang, Zheng and Dong. This is an open-access article distributed under the terms of the Creative Commons Attribution License (CC BY). The use, distribution or reproduction in other forums is permitted, provided the original author(s) and the copyright owner(s) are credited and that the original publication in this journal is cited, in accordance with accepted academic practice. No use, distribution or reproduction is permitted which does not comply with these terms.
*Correspondence: Taotao Dong, ZG9uZ3R0ODJAMTYzLmNvbQ==; Yawen Zheng, emhlbmd5YXdlbjE5OTNAZ21haWwuY29t
Disclaimer: All claims expressed in this article are solely those of the authors and do not necessarily represent those of their affiliated organizations, or those of the publisher, the editors and the reviewers. Any product that may be evaluated in this article or claim that may be made by its manufacturer is not guaranteed or endorsed by the publisher.
Research integrity at Frontiers
Learn more about the work of our research integrity team to safeguard the quality of each article we publish.