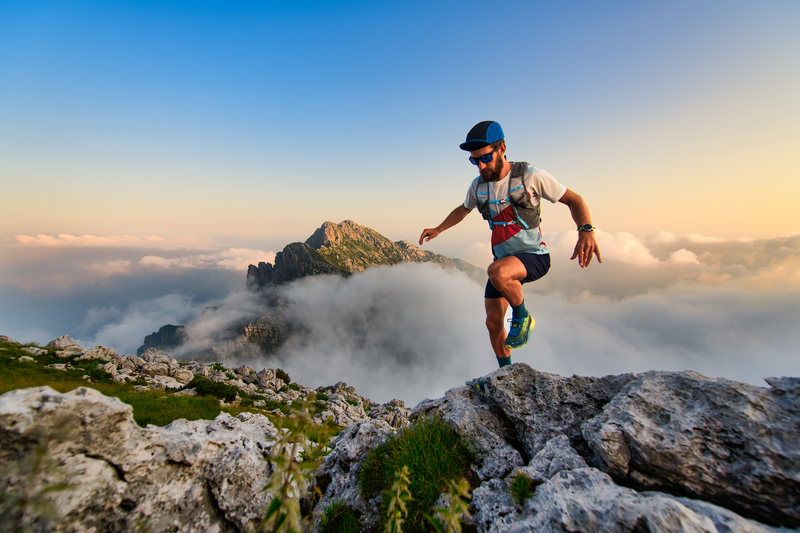
95% of researchers rate our articles as excellent or good
Learn more about the work of our research integrity team to safeguard the quality of each article we publish.
Find out more
ORIGINAL RESEARCH article
Front. Immunol. , 14 December 2023
Sec. Systems Immunology
Volume 14 - 2023 | https://doi.org/10.3389/fimmu.2023.1264599
This article is part of the Research Topic Omics approaches for in-depth analysis of the immune response against infectious diseases in aquaculture View all 4 articles
Piscirickettsia salmonis is the most important health problem facing Chilean Aquaculture. Previous reports suggest that P. salmonis can survive in salmonid macrophages by interfering with the host immune response. However, the relevant aspects of the molecular pathogenesis of P. salmonis have been poorly characterized. In this work, we evaluated the transcriptomic changes in macrophage-like cell line SHK-1 infected with P. salmonis at 24- and 48-hours post-infection (hpi) and generated network models of the macrophage response to the infection using co-expression analysis and regulatory transcription factor-target gene information. Transcriptomic analysis showed that 635 genes were differentially expressed after 24- and/or 48-hpi. The pattern of expression of these genes was analyzed by weighted co-expression network analysis (WGCNA), which classified genes into 4 modules of expression, comprising early responses to the bacterium. Induced genes included genes involved in metabolism and cell differentiation, intracellular transportation, and cytoskeleton reorganization, while repressed genes included genes involved in extracellular matrix organization and RNA metabolism. To understand how these expression changes are orchestrated and to pinpoint relevant transcription factors (TFs) controlling the response, we established a curated database of TF-target gene regulatory interactions in Salmo salar, SalSaDB. Using this resource, together with co-expression module data, we generated infection context-specific networks that were analyzed to determine highly connected TF nodes. We found that the most connected TF of the 24- and 48-hpi response networks is KLF17, an ortholog of the KLF4 TF involved in the polarization of macrophages to an M2-phenotype in mammals. Interestingly, while KLF17 is induced by P. salmonis infection, other TFs, such as NOTCH3 and NFATC1, whose orthologs in mammals are related to M1-like macrophages, are repressed. In sum, our results suggest the induction of early regulatory events associated with an M2-like phenotype of macrophages that drives effectors related to the lysosome, RNA metabolism, cytoskeleton organization, and extracellular matrix remodeling. Moreover, the M1-like response seems delayed in generating an effective response, suggesting a polarization towards M2-like macrophages that allows the survival of P. salmonis. This work also contributes to SalSaDB, a curated database of TF-target gene interactions that is freely available for the Atlantic salmon community.
Piscirickettsia salmonis is the etiological agent of Salmonid Rickettsial Septicaemia (SRS), a contagious systemic disease mainly affecting saltwater salmon (1). P. salmonis is a Gram-negative bacterium described as a facultative intracellular pathogen that resides in vacuoles of macrophages and hepatocytes (1–3). In Chile, the appearance of recurrent and aggressive outbreaks of SRS is the most severe health threat to the salmon industry. In the first half of 2022, mortalities associated with P. salmonis represented 54.2% of the total mortalities attributed to infectious causes in Atlantic salmon (Salmo salar) (4). The prophylaxis and control against P. salmonis have mainly depended on vaccines and antibiotics. However, neither strategy has been effective (5, 6) because vaccines offer short-term protection, and antibiotics are inefficient against intracellular pathogens (5–11).
Despite the severe impact of P. salmonis on Chilean aquaculture, its life cycle and pathogenic mechanisms are poorly characterized (12). Upon entry into macrophages through phagocytosis, P. salmonis induces a significant cytoskeletal reorganization through actin disorganization (13, 14), where they survive and replicate within vacuoles (14–16). P. salmonis evades the lysosomal degradation and inhibits the cell proteolytic activity (12, 14, 15, 17), persists in macrophages (14, 18), modulates apoptosis (14, 16), and inhibits oxidative stress (14, 19–23). Moreover, P. salmonis induces the expression of an anti-inflammatory milieu, probably to ensure survival by downregulating the host antimicrobial response (14, 21, 24, 25). Despite the extensive evidence suggesting that macrophages infected by P. salmonis see their effector functions affected, the regulatory mechanisms of the immune response modulated during the infection, allowing the pathogen to survive, remain unclear.
Macrophages are essential in initiating the pro-inflammatory response against a pathogen. However, they show adapted effector functions for an immune response, and repair previously generated damage. In mammals, this opposite role is known as macrophage polarization, where macrophages can specialize in two opposite phenotypes, M1 and M2 (26, 27). The M1-like profile is associated with a pro-inflammatory macrophage, associated with the production of T helper-1 (Th1) cells and cytokines, such as IL-12, IL-1β, and TNFα, and antimicrobial molecules, such as nitric oxide (NO) and reactive oxygen species (ROS). Conversely, the M2-like profile is associated with Th2 cells and a milieu enriched in anti-inflammatory cytokines, high expression of arginase, IL-1decoyR, IL-10, and IL-1RA, and high phagocytic activity (26, 28–36).
Macrophage polarization is a process tightly regulated by transcription factors (TFs) and associated with extensive transcriptional reprogramming changes. In mammals, the TFs that regulate polarization are widely studied, while in fish, this area is poorly understood (37). In mammals, several TFs, such as peroxisome proliferator-activated receptors (PPARs), signal transducers and activators of transcription (STATs), CCAAT-enhancer-binding proteins (C/EBPs), interferon regulatory factors (IRFs), Krüppel-like factors (KLFs), GATA binding protein (GATA) 3, nuclear transcription factor-kappa β (NF-kβ), c-MYC, and MafB promote the expression of specific genes, which drive the polarization of the macrophages (30, 38). In teleost fish, macrophage polarization has been described as similar to polarization described in mammals (26, 39–41). For example, M1-like macrophages of carp show increased expression of IL-1β and NO production after stimulation with LPS and IFNγ (26, 39–41). In carp and goldfish macrophages, an M2-like profile with a high arginase activity is induced by cAMP and IL-4 (26, 39, 42). In Atlantic salmon, M2-like macrophages seem to be induced for Piscine orthoreovirus 1 (PRV-1) infection due to increased detection of arginase-2 after 4- and 6-week post-challenge, which is associated with a fast recovery following viral clearance (43). Smith et al. characterized the mRNA profile of adherent head kidney leukocytes (HKLs) from Atlantic salmon, which differentiate from monocyte-like cells to macrophage-like cells. The authors observed changes in mRNA expression, including changes in immune-related transcripts (csfr1, arg1, tnfα, mx2) and TFs involved in mammal’s macrophage polarization (klf2, klf9, irf7, irf8, stat1). Many of the TFs and molecules related to immune response identified are markers of macrophages involved in M1/M2 polarization in other species, suggesting a conserved function for some transcripts. Smith et al. observed that the KLF family was downregulated, and members of the IRF family (irf3, irf7, and irf8) and stat1 were upregulated, suggesting that HKLs differentiate to M1-like macrophages (37).
Although pathogens that interfere with M1-like polarization or induce an M2-like phenotype in infected macrophages have not yet been described in fish, manipulating mammals’ macrophage polarization states is emerging as an important pathogenesis mechanism of intracellular bacteria (44). M2-like macrophages appear as a favorable niche for the long-term persistence of numerous intracellular pathogens, such as Mycobacteria, Salmonella, Brucella, Leishmania, Francisella, and Listeria, which can modulate the STAT6-PPARγ/δ pathways to avoid M1-like macrophages polarization (45, 46). Other pathogens, such as Mycobacterium and Coxiella, seem to differentially regulate polarization to M1-like or M2-like phenotypes depending on their infection stage (44); both bacteria modulate the polarization to take advantage of pro-inflammatory response to promote a chronic infection associated to M1-like phenotype. Then, induce an M2-like phenotype associated with an anti-inflammatory milieu to promote tissue repair but contribute to the evasion of the immune response (44, 47–49). Although P. salmonis infection in Atlantic salmon macrophages has not been associated with an M1/M2 profile, the response developed by the infected macrophages could suggest an M2-like polarization, which could explain the bacterial survival and evasion of the effector response.
The immune response of Atlantic salmon infected by P. salmonis has been characterized mainly through the use of transcriptomic analysis in tissue from infected fish (21, 22, 50, 51), highlighting an imbalance response that promotes cell survival and proliferation, decrease the adaptive immune response (22), but activate endocytosis and phagocytosis (52), and modulate the iron and amino acid metabolism, which is convenient for intracellular bacteria that uptake nutrients from its host (21, 50, 51, 53, 54). However, these advances in the understanding are only a global description of the cellular processes modulated in infected fish (21, 22, 50, 51); it is still unknown which modulated master regulators are involved in the macrophage immune response when infected by P. salmonis.
Gene regulatory network (GRN) analysis is a powerful tool to study complex processes containing the regulatory interactions between TFs and target genes. GRNs are widely used to understand regulatory cascades in different organisms as the infective mechanisms of bacterial pathogens like Salmonella, Pseudomonas aeruginosa, and Legionella pneumophila (55–59). GRNs have also been applied to understand the immune response, identifying key regulatory cascades in the analysis of gene expression in septic infections (60), identification of novel TFs linked to the control of stress−related immune response (61), and even include data from single-cell RNA-seq to understand the regulatory cascades for different cellular populations (62). In mammalian macrophages, dissecting GRNs has allowed for modeling the polarization process identifying the main phenotypes and several hybrid phenotypes associated with pathological conditions. In this modeling, hybrid phenotypes mimic a hypothetical continuum of macrophage polarization, with M1 and M2 as the extremes of an uninterrupted sequence of states (63). The GRN has also allowed the identification of two metabolic switches during macrophage polarization, where catabolic processes associated with obtaining energy, such as catabolism for nucleotides, cellular macromolecules, and carbohydrates, were upregulated in M1-like macrophages. Conversely, in M2-like macrophages, the anabolic processes, such as the biosynthesis of amino acids and nucleic acids, fatty acid metabolism, and oxidative phosphorylation were upregulated (64). In M1-like macrophages, this transcriptional reprogramming observed enables a fast energy supply needed for cytokine production and the effective eradication of invading pathogens. In turn, the M2-like macrophages show an upregulation of oxidative phosphorylation and anabolic processes, possibly associated with promoting wound healing processes or supporting the survival of M2-like macrophages during longer and persisting parasitic infections (64).
Our work evaluates how Atlantic salmon macrophages respond to early-stage infection by P. salmonis at a global transcriptomic level, focusing on deciphering the GRNs orchestrating this response. To improve our understanding, we manually curated poorly described genes by bibliographic revision of genes related to the immune response and endocytic pathway. As a result, we generated a GRN that shows the interactions between TFs and target genes using publicly available databases. We aimed to identify which regulatory processes of the macrophage polarization are modulated by the infection, which consequently allows the bacteria to take control of macrophages and even reside in intracellular vacuoles. Our goal was to uncover how P. salmonis induces an immunosuppressive phenotype in infected macrophages, possibly linked to an anti-inflammatory M2-like profile.
Our experiments used macrophage-like cells SHK-1 (ECACC 97111106) as macrophage models (65), which have been widely used to evaluate salmonid macrophage host interactions with P. salmonis (13, 66–68). These cells were maintained at 16°C in Leibovitz medium (L-15; Corning, New York, USA), supplemented with 36 µM 2−mercaptoethanol (2−ME; Gibco, Thermo Scientific, Massachusetts, USA), 10% fetal bovine serum (FBS) (v/v), and 2.5 mg/mL amphotericin B (Corning). The cells were seeded at 6,000 cells/cm2 before further experiments, obtaining 10,000 cells/cm2 to start the analysis.
Culture and propagation of P. salmonis (LF−89−like) was performed in salmonid cell line CHSE 214 (ATCC N°CRL-1682) as previously described by Fryer et al., 1992 (69) and also used in our previous works (15, 17). The CHSE-214 cell line was maintained at 16°C in minimal essential medium (MEM; Corning) supplemented with 10% (v/v) FBS (Hyclone), 10 mM HEPES buffer (Corning), and 1% (v/v) non-essential amino acids (Corning). The infection was observed through conventional inverted light microscopy (Motic AE31E, Leica Microsystems, Wetzlar, Germany) after 4 to 6 days post-infection (dpi) to determine the cytopathic effect on cells (70). Bacteria were quantified using a Petroff−Hausser chamber (Hausser Scientific, Pennsylvania, USA) according to the instructions provided by the manufacturer.
To obtain the transcriptomic profiles of SHK-1 macrophages-like cells infected by P. salmonis at the early stages of infection, we evaluated the response of SHK-1 cells infected by P. salmonis at two different time points, 24- and 48-hours post-infection (hpi). We defined an early stage of infection as the time before the infected cells show the appearance of cytopathic effect (CPE), a characteristic event in the progression of the infection by P. salmonis in Atlantic salmon macrophage-like cell lines that appears from 72-hpi in advance (19, 71), and therefore, within this time, the key events for infection establishment occur.
The infection was performed using a multiplicity of infection (MOI) of 50 bacteria per cell. SHK-1 cells were washed thrice and then incubated with P. salmonis in a minimal medium volume, centrifuged at 100 g for 3 minutes, and then incubated for 1 hour. After the incubation, we added medium to complete the volume recommended for cell cultivation maintenance; in our case, as we used T-75 cultivation bottles, we completed a final volume of 16 mL. As a control, we analyzed non-infected macrophages. We performed all the experiments in 4 replicates.
The RNA was isolated using the mirVana kit following the manufacturer’s instructions. Briefly, the cell cultures were washed thrice with PBS to eliminate cellular debris and medium culture traces. The cells were treated with the disruption solution from the kit and then transferred to the affinity columns provided by the manufacturer to eliminate contaminants and finally elute the purified RNA in a clean tube. The purified RNA was quantified using a fluorometric assay in a Qubit 2.0 following the manufacturer’s instructions with the RNA BR assay kit (Thermofisher).
For sequencing, we used a Custom AnyDeplete kit (Tecan), which we designed to carry out the depletion of Atlantic salmon rRNA (Supplementary File 1). Library preparation was performed with Universal plus RNA-seq library preparation kit with NuQuant (Tecan) following the instructions provided by the manufacturer. Library quantity and quality were assessed by Qubit 2.0 (Thermofisher) and TapeStation D1000 ScreenTape (Agilent Technologies Inc.), respectively. The libraries obtained have 350 bp with an insert of 200 bp, including the Illumina 8−nt unique dual indices. Sequencing was performed in an Illumina NovaSeq S4 device, with a configuration of 150 pair-end for 50 million pair-end reads per sample.
RNA-seq read quality was analyzed using FastQC v.0.12.1 (72). Possible adaptor sequences were removed using BBDuk from the BBTools toolkit (https://jgi.doe.gov/data-and-tools/software-tools/bbtools/), using a list of Illumina adaptors specified in Supplementary File 2. To visualize the quality of the sequencing files, we used MultiQC v.1.13 (73).
To perform a pseudo-alignment of our reads against the transcriptome of Atlantic salmon, we used Kallisto v.0.46.1 (74). The transcriptome was obtained from ENSEMBL, and we used the most recent version to date (3.1). The transcript-to-gene table needed to collapse the quantifications of the different transcripts into one gene was obtained using the R package BioMart (75, 76). To quantify the aligned reads and perform the statistical analysis to obtain the differentially expressed genes (DEGs), we used DESeq2 (77). The statistical analysis was performed by comparing both times analyzed individually against the non-infected control (24-hpi vs non-infected; 48-hpi vs non-infected). We filtered out the genes that presented less than 10 assigned reads and filtered our DEGs by an adjusted p-value of < 0.1. We did not filter by any value of Fold-change, as we aim to identify novel TFs, and in that context, the slightest change in the gene expression of a TF could mean a complete change in the global gene expression.
The comparison between the obtained in this work and DEGs obtained in different previous studies was performed using GeneSectR (https://github.com/NateyJay/genesectR), a tool based on a one-tailed Fisher Exact Test measuring the probability that two gene lists overlap by more than would be expected by chance (based on the GeneSect tool available in the VirtualPlant 1.3 platform) (78, 79). We used gene lists of DEGs of previous studies from Atlantic salmon infected by P. salmonis and other pathogens to compare our data obtained by RNA-seq to determine the association grade with those datasets and the probability of the interception of the given gene lists. We took into consideration gene lists from transcriptomic studies that analyzed Atlantic salmon infected by P. salmonis, obtaining three datasets that analyzed different tissues from infected fish, such as liver, spleen, muscle, and head-kidney (27, 50, 80); we also selected studies performed in SHK-1 macrophage-like cell challenged with virus to determine if the response that we observed was similar to any of those conditions (81, 82). As an unrelated dataset, we selected a study that performed RNA-seq analysis on intestinal tissue from Atlantic salmon fed with different diets (83).
In order to identify expression patterns of the DEGs, we performed a weighted co-expression analysis using the WGCNA package (84, 85). Normalized expression of DEGs was used as input for WGCNA. A soft threshold power of 27 was chosen to construct the adjacency matrix, considering a scale-free topology model fit R2 of 0.7 and an average connectivity of 10. Network modules were identified using dynamic tree cut, using a minimum cluster size of 10 and a merging threshold of 0.3. Correlation networks were constructed using the corresponding topology overlap matrix, and a threshold of >=0.15 was considered for edge weights. Functional enrichment analysis was performed using the Cytoscape application ClueGo and CluePedia (86, 87). We used the latest annotation version for Atlantic salmon (2017), using all the Gene Ontology (GO) databases. We used mostly the default settings, modifying the following: p-value < 0.05, Min level = 1, Max level = 20, % Genes = 10%, and GO term fusion selected. Networks were visualized using Cytoscape v.3.9.1 software (https://cytoscape.org) (88).
To analyze the regulatory cascades involved in polarization, differentiation, and function of macrophages of Atlantic salmon, we generated the database SalSaDB by gathering information on S. salar gene annotation (gene IDs, gene names, gene descriptions) from NCBI and ENSEMBL and regulatory TF-target gene data from SalMotifDB (89). The database also includes information from literature related to the participation of genes and/or gene products in the immune response of teleost fish. This information describes the impact of the infection on different organs of the fish, such as liver, HK, skeletal muscle, and spleen, as the upregulation of genes related to cellular, humoral, innate, and adaptive immune response, cytoskeleton rearrangement, metabolism, and apoptosis, among others. A detailed description of the generation of SalSaDB is available in (Supplementary Materials and Methods). The SalSaDB database is available on GitHub for public use (https://github.com/SebastianReyesCerpa/SalSaDB).
To understand the regulatory cascades underlying the gene expression response to P. salmonis infection, we extracted regulatory interactions and gene attributes from SalSaDB for all DEGs and generated infection context-dependent GRNs. Three different GRNs were generated, including only genes regulated at 24-hpi, genes regulated at 48-hpi, and a core GRN including genes consistently regulated at 24- and 48-hpi. Edges in the GRNs were complemented with co-expression information obtained by WGCNA analysis. We determined node degrees in each GRN to identify central regulators (highest outdegree values) using Gephi v0.10 (88, 90). We included in the GRNs as node attributes literature information on gene function in macrophage polarization, differentiation, and function.
To understand the regulatory mechanism underlying the response of S. salar macrophages to P. salmonis infection, we infected the macrophage-like cell line SHK-1 for 24- and 48-hpi. We chose these time points because they represent the early stages of infection, before the appearance of characteristic cytopathic effects (CPE), which have been reported since 72-hpi (19, 71). Transcriptomics analysis was performed by RNA-Seq, and differential gene expression was determined using DESeq2 (Materials and Methods) (Figure 1A). We obtained 635 differentially expressed genes (DEGs), of which 113 belong exclusively to 24-hpi, 445 to 48-hpi, and 67 were shared between both conditions (Figure 1B and Supplementary File 3).
Figure 1 Experimental design and comparative analysis of transcriptomic response in Atlantic salmon challenged with P. salmonis. (A) Experimental strategy. (B) Quantification of DEGs at 24- and 48-hpi, shared and exclusively expressed in each experimental condition. (C) Comparison of our DEGs with other studies using GeneSectR (78, 79) where Atlantic salmon immune response was used (Ssa-org: Atlantic salmon tissue analysis; Psa: infection with P. salmonis; POMV: infection with Pilchard orthomyxovirus; ISAV: infection with Infectious Salmon Anaemia virus), obtaining significance of the overlap of genes shared between the different studies. A study about intestine Atlantic salmon without infection feed with different diets was used as an outlier. The upper value is the log2 fisher odds ratio, the middle value is the log10 p-adj, and the lower is the overlap size between datasets. *padj < 0.05, **padj < 0.01. Results >1 log2 transformed Fischer odds ratio along with a log10 adjusted p-value of -1.3 indicates an adjusted p-value of 0.05.
Most analyses of the Atlantic salmon response against P. salmonis have been performed at the tissue level, probably masking critical processes occurring specifically inside macrophages. To address the relationship between our transcriptomic profile and the transcriptomic profile obtained in other studies, we compared our list of DEGs obtained after RNA sequencing with the results from six previous articles. These include three datasets from Atlantic salmon infected with P. salmonis in which the authors analyzed the response in the head kidney, liver, and spleen (27, 50, 80). To gain insights into how specific the response was to P. salmonis, we also compared the response of Atlantic salmon to two common viral pathogens, Infectious salmon anemia virus (ISAv) and another from SHK-1 cells infected with Pilchard orthomyxovirus (POMV) (81, 82) (Figure 1C, Supplementary Table 1). We also included a non−infection dataset as an unrelated control, consisting of DEGs obtained from Atlantic salmon intestine feed with different diets (83).
To determine how similar our list of DEGs was compared to these datasets, we used a one-tailed Fisher Exact test implemented in the GeneSectR tool. This tool calculates a log2 transformed Fisher-odds ratio between two gene lists, indicating how strong the association between two datasets is, and a log10 adjusted p-value, indicating the significance of that association. Our results suggest that the DEGs observed are similar to those obtained in four of five studies analyzed, regardless of whether they were obtained from Atlantic salmon tissue or SHK-1 cell line or if the infection was carried out with P. salmonis or with virus (Figure 1C) (80–82, 91). Altogether, the studies that were similar to our DEGs found KEGG pathways or GO terms enrichment related to inflammatory response, programmed cellular death, phagocytosis, and metabolism, and some of the datasets also show RNA metabolism, extracellular matrix and cytoskeletal organization (80–82, 91).
Intriguingly, we found a less-than-expected overlap between our DEGs and those obtained by Valenzuela-Miranda et al. from the spleen and head kidney of Atlantic salmon infected by P. salmonis, which may be related to the specific set of genes that were found in this study, which is highly related to amino acids metabolism and little representation of other processes as immune response and cellular differentiation (50). Finally, all the overlaps with the unrelated list of DEGs (83) were less than expected by chance (Figure 1C).
Interestingly, we were able to obtain 320 DEGs that were not previously found in the literature (Supplementary Figure 1). We performed a functional GO term enrichment analysis on those genes, finding that the associated functions are related to myeloid cell differentiation (GO:0030099) and serine-type carboxypeptidase activity (GO:0004185). In summary, the transcriptomic analysis carried out in our research shows a common set of genes with previous analysis performed in the tissue of infected fish and also uncovers novel DEGs that are exclusively found in SHK-1 macrophage-like cell line infected by P. salmonis and that are related to myeloid cell differentiation, a characteristic process of macrophages during its maturation stages to confront a stimulus, as in this case is the infection.
To determine gene expression patterns of a group of genes in the SHK-1 macrophage-like cell line infected by P. salmonis, we performed a co-expression analysis using the R package WGCNA (85). We obtained four different modules of genes that shared a similar expression pattern (Figure 2A), showing genes that are upregulated at 24- and 48-hpi (module 1), genes that are predominantly upregulated at 48-hpi (module 2), genes that are downregulated mainly at 48-hpi (module 3), and genes that are only downregulated at 24-hpi (module 4) (Figure 2A).
Figure 2 Co-expression analysis of all DEGs and GO terms enrichment analysis. (A) WGCNA analysis of all obtained DEGs, rows represent the different co-expression modules, and columns indicate the different infection times analyzed. (B) GO term enrichment analysis for each co-expression module, the size of each point is proportional to the gene ratio calculated between the obtained genes from a determinate GO term and the total genes that have that GO term in its annotation; Enrichment represents the -log10(adjusted p-value).
To identify the function of the DEGs of each module, we performed a gene ontology (GO) pathway enrichment analysis using the ClueGO platform in Cytoscape (Figure 2B and Supplementary File 4). We observed that the genes present in module 1 mainly were involved in metabolism (GO:0006596 [ornithine decarboxylase 1 (ODC1)], GO:0006777 [gephyrin A (GPHNA)]), cell differentiation (GO:0030218 [solute carrier family 25 member 38b(SLC2538B)]), and transmembrane transportation (GO:0015882 [solute carrier family 23 member 2-like (SLC23A2)], GO:0070890 [SLC23A2], GO:0005369 [solute carrier family 6 member 6 (SLC6A6)]), platelet aggregation (GO:0005018 [PDGFRA]), regulation of chondrocyte development (GO:0061181 [RFLNA]), aromatase activity (GO:0070330 [CYP1B1, CYP1A]), and insulin secretion involved in cellular response to glucose (GO:0035773 [ANO1]). On the other side, the most significant biological term associated with DEGs in module 2 were associated with processes related to endocytic pathway, such as endocytosis (GO:0030130 [clathrin heavy chain 1 (CLTCL1), clathrin light chain A (CLTA)], GO:0030132 [CLTCL1, CLTA]), proton transportation (GO:0016471 [ATPase H+ transporting V1 subunit G1 (ATP6V1G1), V-type proton ATPase subunit H (VMA13)], GO:0033180 [V-type proton ATPase subunit S1-like (VAS1), V-type proton ATPase catalytic subunit A (ATP6V1A), VMA13], GO:0046961 [VAS1, ATP6V1A, VMA13]), and lysosome (GO:0005764 [cathepsin D (CTSD), prosaposin (PSAP), lysosome-associated membrane glycoprotein 1-like (LAMP1), cathepsin B (CTSB)]). Similarly, in module 3, the GO terms are associated with metabolic process (GO:0016706 [prolyl 4-hydroxylase subunit alpha-1 (P4HA1), procollagen-lysine,2-oxoglutarate 5-dioxygenase 1a (PLOD1) procollagen-lysine,2-oxoglutarate 5-dioxygenase 2-like (PLOD2), prolyl 4-hydroxylase subunit alpha-2 (P4HA2)], GO:0004353 [glutamate dehydrogenase, mitochondrial (GDH)], GO:0004671 [protein-S-isoprenylcysteine O-methyltransferase-like (ICMT)]), and processes related to collagen formation (GO:0004656 [P4HA1B, P4HA2, P4HA1], GO:0008475 [PLOD2, PLOD1]). Finally, module 4 is involved with RNA metabolism (GO:0000463 [BOP1 ribosomal biogenesis factor (BOP1)], GO:0000154 [FtsJ RNA 2’-O-methyltransferase 3 (FTSJ3), N-acetyltransferase 10 (NAT10)], GO:0016428 [RNA cytosine-C(5)-methyltransferase NSUN2-like (NSUN2)]) (Figure 2B and Supplementary File 4).
In summary, we found two different sets of genes that are differentially expressed in the early stages of macrophages infected by P. salmonis, as genes differentially expressed mainly at 24-hpi (modules 1 and 4) and genes differentially expressed predominantly at 48-hpi (modules 2 and 3). The set of genes differentially expressed mainly at 24-hpi are related to an upregulation in genes related to metabolism, cell differentiation, cell projection, and transmembrane transportation, processes in which the participant genes mainly belong to the solute carrier families; at 24-hpi, we also observed a downregulation in genes related to RNA metabolism. On the other hand, at 48-hpi, we observed an upregulation of genes classified in module 2 related to endocytosis and lysosome, characterized by clathrin-associated genes and several V-type ATPases and cathepsin genes, which are involved in different steps of the endocytic pathway, from the internalization of the extracellular material to the acidification of the vacuole by the action of the V-ATPases; as for the module 3, the downregulated genes are related to metabolism, characterized by some genes related to carbon and collagen metabolism, associated to the extracellular matrix metabolism.
Our results indicate that P. salmonis infection of macrophages elicits significant transcriptomic reprogramming. We next sought to identify the regulatory mechanisms underlying these changes by constructing a context-specific GRN model of TF-target gene interaction between our DEGs. In order to construct this context-specific GRN, we first assembled a reference GRN including all available information on TFs and target genes for Atlantic salmon obtained from SalMotifDB (89). This network represents all the possible regulatory interactions between TFs and target genes, irrespective of developmental stage, tissue, or experimental condition, so we call it a “reference” network (92). This reference GRN is available in the GitHub of SalSaDB (https://github.com/SebastianReyesCerpa/SalSaDB).
SalSaDB contains gene name equivalence from NCBI to ENSEMBL for ~76% of the genes from Atlantic salmon and automatically obtained gene symbol information for ~24% of the genes. We manually curated annotations for our list of DEGs, achieving ~99% of gene information data for our DEGs (Table 1). The reference GRN contains ~66% of the annotated genes in version 3.1 of the genome of Atlantic salmon, with 39,048 nodes corresponding to genes and 10.8 million edges corresponding to regulatory interactions between TFs and target genes.
To identify the regulatory cascades that control gene expression response of the SHK-1 cell line infected by P. salmonis at 24- and 48-hpi, which could lead to an ineffective macrophage response explaining bacterial survival, we used the information in our reference GRN to generate context-specific GRNs containing our DEGs as nodes. The GRN obtained using the 24-hpi DEGs is composed of 175 nodes and 321 edges, with the participation of 15 TFs, from which KLF17 possesses the highest outdegree connectivity (73 regulated nodes) (Table 2 and Supplementary File 5). As for the GRN generated using the 48-hpi DEGs, it is composed of 486 nodes and 1286 edges, regulated by 20 TFs, from which, again, KLF17 is the TF with the highest outdegree connectivity (189 regulated nodes) (Table 2 and Supplementary File 5). To identify the maintained regulatory interactions between TFs and target genes at 24- and 48-hpi, we analyzed the shared response between 24- and 48-hpi; we obtained a core GRN, which is integrated by 65 nodes and 85 edges, with 7 TFs in total, highlighting KLF17 as the TF with the highest outdegree connectivity (31 regulated nodes) (Table 2 and Supplementary File 5).
To classify the TFs in groups related to the M1− or M2−like phenotype of macrophages, we manually assigned the relation of each TF in our DEGs with one phenotype according to bibliography (human, mouse, and zebrafish) (Table 3), and based on the expression pattern, we assigned an expected effect in the macrophage polarization according to our results (e.g., if a TF is related to M1−like phenotype and is downregulated, then the expected effect is to promote M2−like phenotype; Table 3). As for the TFs found in the core GRN, we found that KLF17 (also annotated as KLF4), MAFBA, WT1B, AHR2B, and DLX3B were related to an M2−like phenotype and were upregulated, suggesting an effect towards M2−like phenotype (93–98). On the other hand, NOTCH3 and NFATC1 were related to the M1−like phenotype, but as those genes were downregulated, we expect an effect toward the M2−like phenotype (99, 100). At 24−hpi, we found that ZFHX3, LEF1, and TBX2B were associated with an M2−like phenotype, which matched the expected effect over macrophage polarization, in the same way as the TFs related to M1−like phenotype, such as ARID3A, IRF1, and BNC1 (101–108). Finally, the TFs found at 48−hpi as CBFB, CREB1B, CREB3L2, and GLIS1B were related to an M2-like phenotype (109–113), but as those TFs were downregulated, their expected relation was assigned toward M1−like phenotype. HIVEP2A, associated with an M1−like phenotype, was assigned to an M2−like phenotype (114) as it is downregulated. Finally, the TFs associated with an M2−like phenotype that were upregulated, thus conserving its role, were SREBF1 (two copies), ZNF410, and SREBF2 (115–117).
We performed a GO term enrichment analysis to examine the functional context of the target genes regulated by the TFs observed in our GRN. Then, to obtain a better context of those results, we classified the results by the upstream hierarchy of the obtained GO terms. Moreover, to provide a better context for the regulatory cascade from the different GRNs, we hierarchically organized the TFs, kept the classification from the previous co-expression analysis of the DEGs (Figure 2), and grouped the regulated genes by those modules. We also quantified the percentage of genes present in each module from the DEGs observed at 24- and 48-hpi to determine how represented each module is at both infection times analyzed (Figures 3A, 4A).
Figure 3 Gene regulatory network at 24-hpi. (A) Co-expression network along with regulatory interactions obtained from our reference GRN. Each module is color-coded, module 1: genes upregulated since 24-hpi; module 2: genes upregulated at 48-hpi; module 3: genes downregulated at 48-hpi; module 4: genes downregulated at 24-hpi. Purple, undirected purple dashed edges represent co-expression and directed green edges represent TF-target regulatory interactions. The transcription factors are represented as octagons. (B) GRN of all the DEGs at 24-hpi, the nodes are color-coded to match upregulation (red) and downregulation (blue). The target genes are represented as modules for ease of view of TF hierarchy and regulation. Purple, undirected purple dashed edges represent co-expression, while directed grey edges represent TF-target regulatory interactions. (C) Abundance of DEGs in percentage between 48-hpi DEGs and the DEGs found in our global analysis. (D) Functional analysis by GO term enrichment performed for each co-expression module (horizontal axis). The size of each point is proportional to the gene ratio calculated between the obtained genes from a determinate GO term and the total genes that have that GO term in its annotation; Enrichment represents the -log10(adjusted p-value).
Figure 4 Gene regulatory network at 48-hpi. (A) Co-expression network along with regulatory interactions obtained from our reference GRN. Each module is color-coded, module 1: genes upregulated since 24-hpi; module 2: genes upregulated at 48-hpi; module 3: genes downregulated at 48-hpi. Purple, undirected purple dashed edges represent co-expression and directed green edges represent TF-target regulatory interactions. The transcription factors are represented as octagons. (B) GRN of all the DEGs at 48-hpi, the nodes are color-coded to match upregulation (red) and downregulation (blue). The target genes are represented as modules for ease of view of TF hierarchy and regulation. Purple, undirected purple dashed edges represent co-expression, while directed grey edges represent TF-target regulatory interactions. (C) Abundance of DEGs in percentage between 48-hpi DEGs and all the DEGs found in our global analysis. (D) Functional analysis by GO term enrichment performed for each co-expression module (horizontal axis). The size of each point is proportional to the gene ratio calculated between the obtained genes from a determinate GO term and the total genes that have that GO term in its annotation; Enrichment represents the -log10(adjusted p-value).
The connectivity analysis of this GRN shows that KLF17 is an important regulatory component of this context-specific GRN (Figures 3A, B). As for the functional analysis of the DEGs from each module at 24-hpi, we observed that DEGs involved with module 1, which were upregulated and co-expressed with most upregulated TFs, represent ~98 of this module (Figure 3C). These DEGs are involved with functions related to cell differentiation (GO:0030218), signal transduction (GO:0070527, GO:0035790, GO:0005018), basal plasma membrane (GO:0009925), microvillus membrane (GO:0031528), and transmembrane transportation (GO:0005369, GO:0015882) (Figure 3D); module 2 (upregulated, ~11%; Figure 3C) was related to collagen catabolic process (GO:0030574) (Figure 3D); module 3 (downregulated, ~9%; Figure 3C) was co-expressed with downregulated TFs and showed processes related to carbon metabolism (GO:0004612, GO:0004613); module 4, which is downregulated and it is an exclusive module for 24-hpi, were related mainly to RNA metabolism (GO:0051391, GO:0000154, GO:0008649, GO:0000453, GO:0032790, GO:0000463, GO:0000466) and carbon metabolism (GO:0004641, GO:0004644) (Figure 3 and Supplementary File 6).
At 48-hpi, the connectivity analysis shows that KLF17 is also an important regulator (Figures 4A, B). As for the functional analysis of the DEGs from each module at 48-hpi, we observed that the genes involved in module 1 (upregulated, ~33%; Figure 4C) were mainly related to metabolism (GO:0006777, GO:0032324, GO:0015882), basal plasma membrane (GO:0009925), microvillus membrane (GO:0031528), and transmembrane transportation (GO:0015882, GO:0070890). The DEGs grouped in module 2 (upregulated, ~99%; Figure 4C) were related to cell differentiation (GO:0030099, GO:0010092), proton transportation (GO:0033180, GO:0016471), lysosome and trans-Golgi network (GO:0005764, GO:0030130), endocytosis (GO:0030132, GO:0030130), and transmembrane transportation (GO:0046961). DEGs from module 3 (upregulated, ~94%; Figure 4C) are related to transmembrane transportation (GO:0005369), metabolism in general (GO:0006481, GO:0019511, GO:0004353, GO:0004671, GO:0016706), and collagen metabolism (GO:0008475, GO:0004656) (Figure 4D and Supplementary File 7).
Finally, our core GRN was generated by selecting the DEGs shared between 24- and 48-hpi, we delimited our search of relevant genes to less than 100, which allowed us to put more effort into contextualizing the role of those genes in the macrophage response while also getting the TFs that control the regulatory cascade through both times analyzed. This information allowed us to determine the process involved in the establishment of infection of P. salmonis in SHK-1 cells that even show the same expression pattern, up- or down-regulated at both times analyzed, showing a consistent response through early infection times. In this GRN, we also observed that KLF17 is an important regulator with the highest outdegree connectivity (Figures 5A, B). As for the functional analysis of each module from core DEGs (overlap between 24- and 48-hpi), we observed that genes from module 1 (upregulated, ~32%) were related to metabolism (GO:0006777), plasma membrane projection (GO:0031528, GO:0009925), and transmembrane transportation (GO:0015882, GO:0070890). The genes in module 2 (upregulated, ~9%) were related to the collagen catabolic process (GO:0030574), and genes co-expressed in module 3 did not show significant results from the functional analysis (Figure 5 and Supplementary File 8).
Figure 5 Gene regulatory network for core genes. (A) Co-expression network along with regulatory interactions obtained from our reference GRN. Each module is color-coded, module 1: genes upregulated since 24-hpi; module 2: genes upregulated at 48-hpi. Purple, undirected purple dashed edges represent co-expression and directed green edges represent TF-target regulatory interactions. The transcription factors are represented as octagons. (B) GRN of all the core DEGs, the nodes are color-coded to match upregulation (red) and downregulation (blue). The target genes are represented as modules for ease of view of TF hierarchy and regulation. Purple, undirected purple dashed edges represent co-expression, while directed grey edges represent TF-target regulatory interactions. (C) Abundance of DEGs in percentage between core DEGs and all the DEGs found in our global analysis. (D) Functional analysis by GO term enrichment performed for each co-expression module (horizontal axis). The size of each point is proportional to the gene ratio calculated between the obtained genes from a determinate GO term and the total genes that have that GO term in its annotation; Enrichment represents the -log10(adjusted p-value).
Altogether, our results show that the SHK-1 macrophage-like cell line infected by P. salmonis shows a regulatory cascade with KLF17 as an essential regulator at early times of infection. This cascade is also integrated by NOCH3, FOXF1, DLX3B, AHR2B, WT1B, NFATC1, and MAFBA, whose we could classify as M1-like related TFs and M2-related TFs. The M1-related TFs are NOTCH3 and NFATC1, which are downregulated, thus not promoting an M1-like phenotype. The TFs related to an M2-like phenotype are KLF17, DLX3B, AHR2B, WT1B, and MAFBA, which are upregulated and promote differentiation toward an M2-like phenotype (Figure 6).
Figure 6 Atlantic salmon macrophage response against P. salmonis. Summary of the regulatory interactions between TFs and the biological processes they regulate in Atlantic salmon macrophage-like cells SHK-1 infected by P. salmonis.
The understanding of the specific transcriptomic response of Atlantic salmon macrophages against P. salmonis has yet to be well explored because most analyses have been performed at the tissue level, probably masking critical processes occurring specifically inside macrophages. First, we determined how much we contributed to the state of the art of Atlantic salmon macrophage immune response with our transcriptomic analyses in macrophage-like cells (SHK-1) infected with P. salmonis. Although we found 320 DEGs that were not previously found in the literature and are related to the myeloid cell differentiation process, which has been observed in macrophages stimulated by pathogen-associated molecular patterns (PAMPs) from P. salmonis (118, 119) (Supplementary Figure 1), the biological process in which they are involved were also found in other studies of Atlantic salmon infected by P. salmonis, as is downstream the terms of cell differentiation and cell development (21, 22, 91).
Interestingly, in Gervais et al. (81), the authors analyze the transcriptomic response of SHK-1 challenged by ISAV by RNA-seq, obtaining 2279 DEGs, of which only 58 are shared genes with our results. However, in Moraleda et al. (80), the authors also analyze by RNA-seq the transcriptomic response of the head kidney and spleen from Atlantic salmon infected by P. salmonis, obtaining 9827 DEGs, of which 214 are shared with our DEGs, which corresponds to 34,5% of our DEGs are shared with those reported by Moraleda et al. (80). Conversely, only 9,35% of our DEGs are shared with those reported by Gervais et al. (Figure 1C), suggesting that our study seems to show a more shared response with the studies in which the transcriptomic response was analyzed by RNA-seq in Atlantic salmon challenged with P. salmonis, rather than a response against viruses infecting the same macrophage-like cell line (81). Moreover, the DEGs shared between our research and the previously reported DEGs are statistically similar, suggesting that in our results, we also found genes commonly expressed in Atlantic salmon tissues when infected by P. salmonis. Altogether, the processes observed in the other studies performed in Atlantic salmon infected by P. salmonis have shown that the DEGs were related to amino acid metabolism, adaptive immune response, phagocytosis, apoptosis, cytoskeleton, and intracellular trafficking, biological processes that were also observed in our results (Figures 3–5 and Supplementary Files 6-8), supporting this significant overlap between the different datasets (50, 80, 91).
The co-expression analysis of our DEGs allowed us to categorize our genes into four modules, which helped us to analyze the functionality of those genes by groups; in this way, we achieved a detailed assignation of functions by modules (Figure 2), gaining depth in our analysis, as it can be compared with our previous result, when we analyzed our unique 320 DEGs, in which we could not obtain detailed information performing the same analysis. As we observed the expression patterns of our DEGs in the WGCNA analysis, we noticed that some modules were almost exclusive for each condition, such as modules 2 and 3 at 48−hpi and modules 1 and 4 at 24−hpi; that was confirmed when we calculated the percentage of genes present in each module for each time of infection analyzed (Figures 2–4). We also observed the conservation of the assigned functions by the GO term enrichment analysis between all the genes present in each module and the further analysis for the gene modules specific for 24− and 48−hpi (Figures 2–4 and Supplementary Files 3-5). Interestingly, we found several processes that are related to M2 phenotype polarization, such as platelet aggregation, reportedly related as a product of M2 differentiation (120); development of chondrocytes, a process associated with collagen secretion, that also is associated with M2 macrophage differentiation (121); and insulin secretion response to glucose which has been linked to M2 activation by an increase of sensitivity to glucose (122). Nevertheless, we also observed several processes linked to M1 macrophage polarization, such as aromatase activity, a process linked to an inflammatory response by the induction of aromatase in pair with the development of an inflammatory response (123); procollagen activity, a process involved in the synthesis of collagen, and as the genes related to this are downregulated, we might assume a decrease in the collagen synthesis (124). Altogether, these results suggest that macrophages infected by P. salmonis after 24-hpi present a phenotype towards M2-like macrophage spectrum by the proportion of processes associated with each phenotype.
In the functional analysis of the DEGs at 24-hpi, we found that the genes belonging to module 1 were related to processes, such as cell differentiation, membrane process, and transmembrane transportation, while the genes grouped in module 2 were mainly related to collagen metabolism and genes co-expressed in module 3 were related to carbon metabolism. All the biological processes grouped in both module 1 and module 2 may be associated with the induction of endocytosis of P. salmonis through the modulation of the clathrin and actin cytoskeleton (Figure 3) (13, 125, 126). On the other hand, the genes grouped in module 4 (downregulated) were related mainly to RNA metabolism, suggesting that the bacteria promotes RNA metabolism (Figure 3), which could be a signal of post-transcriptional gene regulation or even transcriptional regulation mediated by miRNAs, mechanisms previously reported in mammals hosts infected by other intracellular pathogens, as mice infected by Pseudomonas aeruginosa, and human HeLa cells infected by Salmonella enterica. Moreover, this has also been suggested in Atlantic salmon infected by P. salmonis, raising the possibility of further investigating the non-coding RNAs in the macrophage immune response of Atlantic salmon (51, 127, 128).
In macrophages, after 48-hpi, we found several enriched processes that reinforce the idea of the imbalanced membrane transportation in infected macrophages, such as podosome, cell projection membrane, clathrin-related processes, and lamellipodium (Figure 4), along with endocytic/phagocytic vesicle transportation, as a lysosome-related process, vacuole, small GTPases (such as Rab proteins), and v-ATPases (characteristic of lysosomal acidification) (Figure 4). Those results correlate with previous findings, where P. salmonis was found to imbalance the endocytic pathway of infected macrophages, partially inactivating the lysosomal acidification and its degradation capacity (15, 17).
To contribute to the Atlantic salmon transcriptomics community, we created SalSaDB as a new resource to analyze the Atlantic salmon gene expression results. This contribution was one of our most significant achievements due to the lack of easy-to-access information that crosslinks different databases. This work would be trivial using R studio packages that allow users to access this information quickly. However, those resources are currently available for model organisms, such as mice, zebrafish, and cows, but not Atlantic salmon. SalSaDB proved its value in analyzing our data, helping us translate our results obtained using ENSEMBL genomic information to the NCBI genomic information and take advantage of the GRN created using the publicly available information from SalMotifDB (89). Generating the reference GRN for Atlantic salmon resulted in a non-condition-biased result that will be useful to several researchers who need to perform a regulatory analysis in a different context of the Atlantic salmon research field. We quickly observed which genes were relevant in our experimental conditions using the GRN and our dictionary for the gene names and gene product, making efficient analysis of the DEGs found in SHK-1 cells infected by P. salmonis. As SalSaDB has the potential to become a helpful resource for the scientific community that studies Atlantic salmon, and with the spirit of making it accessible to researchers, we make it publicly available in GitHub, including a detailed description in the code used to obtain the information and how to use the GRN.
Our transcriptional analysis observed several TFs controlling the gene expression in 24− and 48−hpi and exclusively to each time point analyzed (Table 3). First, we found essential TFs in our core GRN, which previously were reported as regulators of macrophage polarization, such as KLF17 and MAFBA (both upregulated) and NFATC1 and NOTCH3 (both downregulated) (Figure 5). In mammals (mice and humans), KLF-17 and MAFBA have been associated with an M2-like macrophage profile. Conversely, NFATC1 and NOTCH3 have been associated with M1-like macrophages (94, 96, 99, 100), suggesting that our results in macrophage-like cells SHK-1 infected by P. salmonis possibly develop a regulation towards M2-like phenotype.
Additionally, we also categorized the other TFs that regulate the core GRN, which were all upregulated, WT1B, FOXF1, AHR2B, and DLX3B, which have been described to be related to M2-like phenotype in zebrafish (WT1B), mice (AHR2B), and mouse (DLX3B). Conversely, FOXF1 is not described in the context of macrophage polarization (93, 95, 97, 98). Altogether, those results suggest that the core regulation of macrophages infected by P. salmonis at early times is tightly controlled by TFs related to an M2-like phenotype (Figure 6).
As we analyze the TFs that belongs to each time of infection evaluated, we found that at 24-hpi, more than half of TFs are related to an M1 phenotype in humans and mouse, and also were upregulated in our results, corresponding to ARID3A, IRF1, MXD4, and BNC1. On the other hand, we also observed TFs that in mammals have been associated with an M2-like polarization, which corresponds to ZFHX3, LEF1, and TBX2B (101–108). Similarly, the classification of the TFs at 48-hpi shows that TFs with a known role in macrophage polarization are related to M1-like and M2-like macrophages. The TFs related to an M1-like phenotype in mammals were CBFB (indirectly, by regulating EIF4B), CREB1B, GLIS1B, and CREB3L2 (109–113), while the TFs related to an M2-like phenotype in mammals were two copies of SREBF1, SREBF2, ZNF410, and HIVEP2A (114–117). Although at 48-hpi the macrophage polarization phenotype seems to be shifting to a less M2-like phenotype but maintaining a predominant regulatory cascade related to M2-like macrophages.
To understand the root of the imbalance that P. salmonis generates in infected macrophages and its impact on macrophage polarization, differentiation, and function, we used our GRN to unravel the regulatory interaction of the DEGs found in our RNA sequencing. In our work, we select the most relevant genes found in our GRN by connectivity and magnitude of the log2(FC). Additionally, we include a bibliographic review of the previously reported relevance in this process. Our GRNs are relatively large, with 321 and 1,286 edges at 24- and 48-hpi, so we constructed a core GRN of the DEGs shared at both times. This core GRN allows us to find the genes maintained as differentially expressed, which present a similar expression pattern. Altogether, the core genes should be key to modulating the macrophage response in P. salmonis infection.
The involvement of TFs belonging to the KLF family has been described previously in the polarization of Atlantic salmon macrophages. A study conducted in primary culture of head kidney leukocytes (HKLs) from Atlantic salmon enriched in macrophage−like populations reported two TFs from the Krüppel−like factors family, KLF2 and KLF9, which were downregulated during monocyte to macrophage differentiation (37). Our results showed an upregulation of KLF17, a TF whose upregulation is negatively associated with cell motility in different human cancer cell lines (129–131). Moreover, the Atlantic salmon KLF17 gene is also annotated as KLF4-like, a characteristic TF of M2 polarized macrophages (96). Together, our results suggest that the upregulation of KLF17 in SHK-1 cells infected by P. salmonis could be suppressing the cell motility of the infected macrophages at early infection times and probably promoting an M2-like phenotype in infected macrophages.
Among TFs observed upregulated in our experimental conditions, we observed MAFBA. Some studies link the expression of this TF to an increased proteasome activity and activation of the innate immune response in human cancer cell lines (132, 133). Moreover, this TF has been described as an exclusive macrophage TF, expressed in macrophages polarized to an M2−like phenotype (94). Our results show that MAFBA is upregulated in SHK-1 cells infected by P. salmonis. In this context, we can suggest that MAFBA regulates polarization towards an M2-like phenotype in macrophages infected by P. salmonis, promoting an innate immune response instead of a specific immune response against infection. On the other hand, NOTCH3 is a macrophage-exclusive TF that positively regulates the NF-κB pathway, which is characteristic of M1-like macrophages (100). Our study showed downregulation of NOTCH3 expression, suggesting an impaired response mediated by NF-κB and an M2-like macrophage profile in SHK-1 cells infected by P. salmonis.
Understanding the regulatory mechanisms of macrophage polarization opens the possibility of modulating the response mediated by macrophages. Our work presents a comprehensive description of the transcriptomic response of macrophages infected by P. salmonis with an emphasis on the regulatory cascades involved in macrophage polarization and function. Although we observed changes in the expression of genes associated with an M1-like profile in infected SHK-1 cells, our results suggest that this response is probably not robust and early enough to generate an effective response; on the contrary, we uncovered a regulatory cascade that shifts gene expression towards an M2-like phenotype, affecting diverse effectors related to the lysosome, RNA metabolism, cytoskeleton organization, remodeling of extracellular matrix, and cell migration, among others. These concerted changes in gene expression could explain the survival of P. salmonis when it infects Atlantic salmon macrophages (Figure 6). Moreover, this work highlights the use of a curated database of TF-target gene interactions for Atlantic salmon, SalSaDB, that is freely available for the scientific community.
The datasets presented in this study can be found in online repositories. The names of the repository/repositories and accession number(s) can be found below: PRJNA996662 (SRA).
Ethical approval was not required for the studies on animals in accordance with the local legislation and institutional requirements because only commercially available established cell lines were used.
DP-S: Data curation, Formal analysis, Investigation, Methodology, Software, Validation, Writing – original draft, Writing – review & editing. MF: Investigation, Methodology, Writing – review & editing. VI: Investigation, Methodology, Writing – review & editing. BB: Investigation, Methodology, Writing – review & editing. ES: Data curation, Formal analysis, Methodology, Writing – review & editing. JR-P: Formal analysis, Investigation, Methodology, Supervision, Writing – review & editing. EV-V: Formal analysis, Investigation, Methodology, Writing – review & editing. FR-L: Conceptualization, Formal analysis, Investigation, Methodology, Writing – review & editing. DT-A: Formal analysis, Investigation, Methodology, Writing – review & editing. EAV: Conceptualization, Formal analysis, Funding acquisition, Investigation, Methodology, Supervision, Writing – review & editing. SR-C: Conceptualization, Formal analysis, Funding acquisition, Investigation, Methodology, Project administration, Supervision, Writing – original draft, Writing – review & editing.
The author(s) declare financial support was received for the research, authorship, and/or publication of this article. This research was funded by Agencia Nacional de Investigación y Desarrollo (ANID) Subdirección de Investigación Aplicada FONDEF ID22I10211 (SR-C), ANID FONDECYT 1230809 (DT-A), ANID FONDECYT 1221064 (JR-P), ANID PCI REDES180097 (EAV and SR-C), ANID Millennium Science Initiative Program - ICN17_022 (EAV), and national doctoral scholarship ANID 21191135 (DP-S).
We thank Dr. Leandro Murgas and Dr. Eduardo Martínez, who helped through all this work by giving space to talk about coding. Special mention to Negrita, one of the cats of DP-S, who passed away during this work.
The authors declare that the research was conducted in the absence of any commercial or financial relationships that could be construed as a potential conflict of interest.
All claims expressed in this article are solely those of the authors and do not necessarily represent those of their affiliated organizations, or those of the publisher, the editors and the reviewers. Any product that may be evaluated in this article, or claim that may be made by its manufacturer, is not guaranteed or endorsed by the publisher.
The Supplementary Material for this article can be found online at: https://www.frontiersin.org/articles/10.3389/fimmu.2023.1264599/full#supplementary-material
Supplementary Figure 1 | Venn diagram of overlap between DEGs from different studies and ours. Venn diagram shows the overlap between our DEGs and the gene lists obtained from online data from studies about Atlantic salmon infected by P. salmonis and the virus. We grouped the DEGs by Ssa_Psa for the gene list from papers about Atlantic salmon infected by P. salmonis and Ssa_virus for the gene lists from papers about Atlantic salmon infected by virus (ISAv and POMV).
SUPPLEMENTARY FIGURE 2 | Manual gene homologation from NCBI to ENSEMBL databases. Gene ID homologation was carried out by comparison of genomic coordinates in NCBI Genome Data Viewer. The top panel represents a complete overlap between the exons of a gene annotation from both databases. The mid panel shows an example of a partial overlap of exons, in which the observed difference of exons coordinates between both databases and the absence of exons from one database from another. The bottom panel shows the decision flow diagram that was used to assign the homologation of a partial overlap between the gene annotation from NCBI and ENSEMBL, with the priority of databases to assign the gene ID when ENSEMBL external references matched NCBI annotation.
SUPPLEMENTARY FIGURE 3 | SalSaDB recompilation diagram. SalSaDB was constructed using information from several databases and research papers to improve Atlantic salmon genomic information for this work. This diagram shows the quantity of genes successfully gathered and linked with NCBI information, with the steps we followed to improve the automatically obtained data for our DEGs.
Supplementary File 1 | rRNA sequences of Atlantic salmon.txt.
Supplementary File 2 | Adaptors.txt.
Supplementary File 3 | Differentially expressed genes (DEGs).
Supplementary File 4 | All DEGs GO annotation.
Supplementary File 5 | GRN tables from cytoscape.
Supplementary File 6 | 24-hpi GO annotation.
Supplementary File 7 | 48-hpi GO annotation.
Supplementary File 8 | Core GO annotation.
Supplementary Materials and Methods | Description of generation of SalsaDB.
1. Fryer JL, Hedrick RP. Piscirickettsia salmonis: a Gram-negative intracellular bacterial pathogen of fish. J Fish Dis (2003) 26(5):251–62. doi: 10.1046/j.1365-2761.2003.00460.x
2. Branson EJ, Nieto-Diaz Muñoz D. Description of a new disease condition occurring in farmed coho salmon, Oncorhynchus kisutch (Walbaum), in South America. J Fish Dis (1991) 14:147–56. doi: 10.1111/j.1365-2761.1991.tb00585.x
3. Cvitanich JD, Garate N O, Smith CE. The isolation of a rickettsial-like organism causing disease and mortality in Chilean salmonids and its confirmation by Koch’s postulate. J Fish Dis (1991) 14:121–45. doi: 10.1111/j.1365-2761.1991.tb00584.x
4. Sernapesca. Informe Sanitario de la salmonicultura Primer Semestre año 2022, Departamento de salud animal, Subdirección de acuicultura. Departamento de Salud Animal, Subdirección de Acuicultura, Servicio Nacional de Pesca: Valparaíso, Chile (2022).
5. Flores-Kossack C, Montero R, Kollner B, Maisey K. Chilean aquaculture and the new challenges: Pathogens, immune response, vaccination and fish diversification. Fish Shellfish Immunol (2020) 98:52–67. doi: 10.1016/j.fsi.2019.12.093
6. Maisey K, Montero R, Christodoulides M. Vaccines for piscirickettsiosis (salmonid rickettsial septicaemia, SRS): the Chile perspective. Expert Rev Vaccines (2017) 16(3):215–28. doi: 10.1080/14760584.2017.1244483
7. Evensen O. Immunization Strategies against Piscirickettsia salmonis Infections: Review of Vaccination Approaches and Modalities and Their Associated Immune Response Profiles. Front Immunol (2016) 7:482. doi: 10.3389/fimmu.2016.00482
8. Rozas M, Enríquez R. Piscirickettsiosis and Piscirickettsia salmonis in fish: a review. J Fish Dis (2014) 37(3):163–88. doi: 10.1111/jfd.12211
9. Shoemaker CA, Klesius PH, Evans JJ, Arias CR. Use of modified live vaccines in aquaculture. J World Aquaculture Soc (2009) 40(5):573–85. doi: 10.1111/j.1749-7345.2009.00279.x
10. Miranda CD, Godoy FA, Lee MR. Current status of the use of antibiotics and the antimicrobial resistance in the Chilean salmon farms. Front Microbiol (2018) 9:1284. doi: 10.3389/fmicb.2018.01284
11. Oceana. Uso de antibióticos en la salmonicultura Chilena: Causas, efectos y riesgos asociados, in Oceana, Santiago, Chile. (2018).
12. Gómez FA, Tobar JA, Henríquez V, Sola M, Altamirano C, Marshall SH. Evidence of the presence of a functional Dot/Icm type IV-B secretion system in the fish bacterial pathogen Piscirickettsia salmonis. PloS One (2013) 8(1):e54934. doi: 10.1371/journal.pone.0054934
13. Ramírez R, Gómez FA, Marshall SH. The infection process of Piscirickettsia salmonis in fish macrophages is dependent upon interaction with host-cell clathrin and actin. FEMS Microbiol Lett (2015) 362(1):1–8. doi: 10.1093/femsle/fnu012
14. Rozas-Serri M. Why does piscirickettsia salmonis break the immunological paradigm in farmed salmon? Biological context to understand the relative control of piscirickettsiosis. Front Immunol (2022) 13:856896. doi: 10.3389/fimmu.2022.856896
15. Pérez-Stuardo D, Morales-Reyes J, Tapia S, Ahumada DE, Espinoza A, Soto-Herrera V, et al. Non-lysosomal Activation in Macrophages of Atlantic Salmon (Salmo salar) After Infection With Piscirickettsia salmonis. Front Immunol (2019) 10:434. doi: 10.3389/fimmu.2019.00434
16. Rojas V, Galanti N, Bols NC, Marshall SH. Productive infection of Piscirickettsia salmonis in macrophages and monocyte-like cells from rainbow trout, a possible survival strategy. J Cell Biochem (2009) 108(3):631–7. doi: 10.1002/jcb.22295
17. Pérez-Stuardo D, Espinoza A, Tapia S, Morales-Reyes J, Barrientos C, Vallejos-Vidal E, et al. Non-specific antibodies induce lysosomal activation in atlantic salmon macrophages infected by piscirickettsia salmonis. Front Immunol (2020) 11:544718. doi: 10.3389/fimmu.2020.544718
18. Isla A, Haussmann D, Vera T, Kausel G, Figueroa J. Identification of the clpB and bipA genes and an evaluation of their expression as related to intracellular survival for the bacterial pathogen Piscirickettsia salmonis. Veterinary Microbiol (2014) 173(3-4):390–4. doi: 10.1016/j.vetmic.2014.08.014
19. Ortiz-Severín J, Travisany D, Maass A, Cambiazo V, Chávez FP. Global Proteomic Profiling of Piscirickettsia salmonis and Salmon Macrophage-Like Cells during Intracellular Infection. Microorganisms (2020) 8(12):1845. doi: 10.3390/microorganisms8121845
20. Rise ML, Jones SRM, Brown GD, von Schalburg KR, Davidson WS, Koop BF. Microarray analyses identify molecular biomarkers of Atlantic salmon macrophage and hematopoietic kidney response to Piscirickettsia salmonis infection. Physiol Genomics (2004) 20(1):21–35. doi: 10.1152/physiolgenomics.00036.2004
21. Rozas-Serri M, Peña A, Maldonado L. Transcriptomic profiles of post-smolt Atlantic salmon challenged with Piscirickettsia salmonis reveal a strategy to evade the adaptive immune response and modify cell-autonomous immunity. Dev Comp Immunol (2018) 81:348–62. doi: 10.1016/j.dci.2017.12.023
22. Tacchi L, Bron JE, Taggart JB, Secombes CJ, Bickerdike R, Adler MA, et al. Multiple tissue transcriptomic responses to Piscirickettsia salmonis in Atlantic salmon (Salmo salar). Physiol Genomics (2011) 43(21):1241–54. doi: 10.1152/physiolgenomics.00086.2011
23. Zúñiga A, Aravena P, Pulgar R, Travisany D, Ortiz-Severín J, Chávez FP, et al. Transcriptomic changes of piscirickettsia salmonis during intracellular growth in a salmon macrophage-like cell line. Front Cell Infection Microbiol (2019) 9:426. doi: 10.3389/fcimb.2019.00426
24. Álvarez CA, Gomez FA, Mercado L, Ramírez R, Marshall SH. Piscirickettsia salmonis imbalances the innate immune response to succeed in a productive infection in a salmonid cell line model. PloS One (2016) 11(10):e0163943. doi: 10.1371/journal.pone.0163943
25. Rozas-Serri M, Peña A, Arriagada G, Enríquez R, Maldonado L. Comparison of gene expression in post-smolt Atlantic salmon challenged by LF-89-like and EM-90-like Piscirickettsia salmonis isolates reveals differences in the immune response associated with pathogenicity. J Fish Dis (2018) 41(3):539–52. doi: 10.1111/jfd.12756
26. Wentzel AS, Janssen JJE, de Boer VCJ, van Veen WG, Forlenza M, Wiegertjes GF. Fish macrophages show distinct metabolic signatures upon polarization. Front Immunol (2020) 11:152. doi: 10.3389/fimmu.2020.00152
27. Xue J, Schmidt SV, Sander J, Draffehn A, Krebs W, Quester I, et al. Transcriptome-based network analysis reveals a spectrum model of human macrophage activation. Immunity (2014) 40(2):274–88. doi: 10.1016/j.immuni.2014.01.006
28. Biswas SK, Chittezhath M, Shalova IN, Lim J-Y. Macrophage polarization and plasticity in health and disease. Immunologic Res (2012) 53(1-3):11–24. doi: 10.1007/s12026-012-8291-9
29. Italiani P, Boraschi D. From monocytes to M1/M2 macrophages: phenotypical vs. Functional differentiation. Front Immunol (2014) 5:514. doi: 10.3389/fimmu.2014.00514
30. Li H, Jiang T, Li M-Q, Zheng X-L, Zhao G-J. Transcriptional regulation of macrophages polarization by microRNAs. Front Immunol (2018) 9:1175. doi: 10.3389/fimmu.2018.01175
31. Mills CD, Kincaid K, Alt JM, Heilman MJ, Hill AM. M-1/M-2 macrophages and the Th1/Th2 paradigm. J Immunol (Baltimore Md.: 1950) (2000) 164(12):6166–73. doi: 10.4049/jimmunol.164.12.6166
32. Nathan CF, Hibbs JB. Role of nitric oxide synthesis in macrophage antimicrobial activity. Curr Opin Immunol (1991) 3(1):65–70. doi: 10.1016/0952-7915(91)90079-G
33. O’Neill LAJ, Kishton RJ, Rathmell J. A guide to immunometabolism for immunologists. Nat Rev Immunol (2016) 16(9):553–65. doi: 10.1038/nri.2016.70
34. Sica A, Erreni M, Allavena P, Porta C. Macrophage polarization in pathology. Cell Mol Life sciences: CMLS (2015) 72(21):4111–26. doi: 10.1007/s00018-015-1995-y
35. Tannahill GM, Curtis AM, Adamik J, Palsson-McDermott EM, McGettrick AF, Goel G, et al. Succinate is an inflammatory signal that induces IL-1β through HIF-1α. Nature (2013) 496(7444):238–42. doi: 10.1038/nature11986
36. Van den Bossche J, O’Neill LA, Menon D. Macrophage immunometabolism: where are we (Going)? Trends Immunol (2017) 38(6):395–406. doi: 10.1016/j.it.2017.03.001
37. Smith NC, Umasuthan N, Kumar S, Woldemariam NT, Andreassen R, Christian SL, et al. Transcriptome profiling of Atlantic salmon adherent head kidney leukocytes reveals that macrophages are selectively enriched during culture. Front Immunol (2021) 12. doi: 10.3389/fimmu.2021.709910
38. Kim H. The transcription factor MafB promotes anti-inflammatory M2 polarization and cholesterol efflux in macrophages. Sci Rep (2017) 7(1):7591. doi: 10.1038/s41598-017-07381-8
39. Joerink M, Ribeiro CMS, Stet RJM, Hermsen T, Savelkoul HFJ, Wiegertjes GF. Head kidney-derived macrophages of common carp (Cyprinus carpio L.) show plasticity and functional polarization upon differential stimulation. J Immunol (Baltimore Md.: 1950) (2006) 177(1):61–9. doi: 10.4049/jimmunol.177.1.61
40. Piazzon MC, Savelkoul HSJ, Pietretti D, Wiegertjes GF, Forlenza M. Carp il10 has anti-inflammatory activities on phagocytes, promotes proliferation of memory T cells, and regulates B cell differentiation and antibody secretion. J Immunol (Baltimore Md.: 1950) (2015) 194(1):187–99. doi: 10.4049/jimmunol.1402093
41. Saeij JP, Stet RJ, Groeneveld A, Verburg-van Kemenade LB, van Muiswinkel WB, Wiegertjes GF. Molecular and functional characterization of a fish inducible-type nitric oxide synthase. Immunogenetics (2000) 51(4-5):339–46. doi: 10.1007/s002510050628
42. Hodgkinson JW, Fibke C, Belosevic M. Recombinant IL-4/13A and IL-4/13B induce arginase activity and down-regulate nitric oxide response of primary goldfish (Carassius auratus L.) macrophages. Dev Comp Immunol (2017) 67:377–84. doi: 10.1016/j.dci.2016.08.014
43. Malik MS, Nyman IB, Wessel Ø, Dahle MK, Rimstad E. Dynamics of polarized macrophages and activated CD8+ Cells in heart tissue of atlantic salmon infected with piscine orthoreovirus-1. Front Immunol (2021) 12:729017. doi: 10.3389/fimmu.2021.729017
44. Thiriot JD, Martinez-Martinez YB, Endsley JJ, Torres AG. Hacking the host: exploitation of macrophage polarization by intracellular bacterial pathogens. Pathog Dis (2020) 78(1):ftaa009. doi: 10.1093/femspd/ftaa009
45. Muraille E, Leo O, Moser M. TH1/TH2 paradigm extended: macrophage polarization as an unappreciated pathogen-driven escape mechanism? Front Immunol (2014) 5:603. doi: 10.3389/fimmu.2014.00603
46. Price JV, Vance RE. The macrophage paradox. Immunity (2014) 41(5):685–93. doi: 10.1016/j.immuni.2014.10.015
47. Marino S, Cilfone NA, Mattila JT, Linderman JJ, Flynn JL, Kirschner DE. Macrophage polarization drives granuloma outcome during Mycobacterium tuberculosis infection. Infection Immun (2015) 83(1):324–38. doi: 10.1128/IAI.02494-14
48. Amara AB, Bechah Y, Mege J-L. Immune response and Coxiella burnetii invasion. Adv Exp Med Biol (2012) 984:287–98. doi: 10.1007/978-94-007-4315-1_15
49. Ghigo E, Imbert G, Capo C, Raoult D, Mege J-L. Interleukin-4 induces Coxiella burnetii replication in human monocytes but not in macrophages. Ann New York Acad Sci (2003) 990:450–9. doi: 10.1111/j.1749-6632.2003.tb07410.x
50. Valenzuela-Miranda D, Gallardo-Escárate C. Dual RNA-seq uncovers metabolic amino acids dependency of the intracellular bacterium piscirickettsia salmonis infecting atlantic salmon. Front Microbiol (2018) 9:2877. doi: 10.3389/fmicb.2018.02877
51. Valenzuela-Miranda D, Valenzuela-Muñoz V, Farlora R, Gallardo-Escárate C. MicroRNA-based transcriptomic responses of Atlantic salmon during infection by the intracellular bacterium Piscirickettsia salmonis. Dev Comp Immunol (2017) 77:287–96. doi: 10.1016/j.dci.2017.08.016
52. Mukiibi R, Peñaloza C, Gutierrez A, Yáñez JM, Houston RD, Robledo D. The impact of Piscirickettsia salmonis infection on genome-wide DNA methylation profile in Atlantic Salmon. Genomics (2022) 114(6):110503. doi: 10.1016/j.ygeno.2022.110503
53. Calquín P, Ruiz P, Oliver C, Sánchez P, Haro R, Oliva H, et al. Physiological evidence that Piscirickettsia salmonis produces siderophores and uses iron from different sources. J Fish Dis (2018) 41(3):553–8. doi: 10.1111/jfd.12745
54. Pulgar R, Hödar C, Travisany D, Zuñiga A, Domínguez C, Maass A, et al. Transcriptional response of Atlantic salmon families to Piscirickettsia salmonis infection highlights the relevance of the iron-deprivation defence system. BMC Genomics (2015) 16(1):495. doi: 10.1186/s12864-015-1716-9
55. Feldheim YS, Zusman T, Speiser Y, Segal G. The Legionella pneumophila CpxRA two-component regulatory system: new insights into CpxR’s function as a dual regulator and its connection to the effectors regulatory network. Mol Microbiol (2016) 99(6):1059–79. doi: 10.1111/mmi.13290
56. Galán-Vásquez E, Luna B, Martínez-Antonio A. The regulatory network of pseudomonas aeruginosa. Microbial Inf Experimentation (2011) 1(1):3. doi: 10.1186/2042-5783-1-3
57. Medeiros Filho F, do Nascimento APB, Dos Santos MT, Carvalho-Assef APDA, da Silva FAB. Gene regulatory network inference and analysis of multidrug-resistant Pseudomonas aeruginosa. Memorias Do Instituto Oswaldo Cruz (2019) 114:e190105. doi: 10.1101/610493
58. Smith C, Stringer AM, Mao C, Palumbo MJ, Wade JT. Mapping the regulatory network for salmonella enterica serovar typhimurium invasion. mBio (2016) 7(5):e01024–16. doi: 10.1128/mBio.01024-16
59. Steinert M, Heuner K, Buchrieser C, Albert-Weissenberger C, Glöckner G. Legionella pathogenicity: genome structure, regulatory networks and the host cell response. Int J Med microbiol: IJMM (2007) 297(7-8):577–87. doi: 10.1016/j.ijmm.2007.03.009
60. Kim KS, Jekarl DW, Yoo J, Lee S, Kim M, Kim Y. Immune gene expression networks in sepsis: A network biology approach. PloS One (2021) 16(3):e0247669. doi: 10.1371/journal.pone.0247669
61. Behdani E, Bakhtiarizadeh MR. Construction of an integrated gene regulatory network link to stress-related immune system in cattle. Genetica (2017) 145(4-5):441–54. doi: 10.1007/s10709-017-9980-z
62. Hoch M, Rauthe J, Cesnulevicius K, Schultz M, Lescheid D, Wolkenhauer O, et al. Cell-type-specific gene regulatory networks of pro-inflammatory and pro-resolving lipid mediator biosynthesis in the immune system. Int J Mol Sci (2023) 24(5):4342. doi: 10.3390/ijms24054342
63. Palma A, Jarrah AS, Tieri P, Cesareni G, Castiglione F. Gene regulatory network modeling of macrophage differentiation corroborates the continuum hypothesis of polarization states. Front Physiol (2018) 9:1659. doi: 10.3389/fphys.2018.01659
64. Horhold F, Eisel D, Oswald M, Kolte A, Roll D, Osen W, et al. Reprogramming of macrophages employing gene regulatory and metabolic network models. PloS Comput Biol (2020) 16(2):e1007657. doi: 10.1371/journal.pcbi.1007657
65. Dannevig BH, Brudeseth BE, Gjøen T, Rode M, Wergeland HI, Evensen Ø, et al. Characterisation of a long-term cell line (SHK-1) developed from the head kidney of Atlantic salmon (Salmo salarL.). Fish shellfish Immunol (1997) 7(4):213–26. doi: 10.1006/fsim.1996.0076
66. Salazar C, Haussmann D, Kausel G, Figueroa J. Molecular cloning of Salmo salar Toll-like receptors (TLR1, TLR22, TLR5M and TLR5S) and expression analysis in SHK-1 cells during Piscirickettsia salmonis infection. J Fish Dis (2016) 39(2):239–48. doi: 10.1111/jfd.12354
67. Cortés M, Sánchez P, Ruiz P, Haro R, Sáez J, Sánchez F, et al. In vitro expression of Sec-dependent pathway and type 4B secretion system in Piscirickettsia salmonis. Microbial Pathogenesis (2017) 110:586–93. doi: 10.1016/j.micpath.2017.08.003
68. Carril GP, Gómez FA, Marshall SH. Expression of flagellin and key regulatory flagellar genes in the non-motile bacterium Piscirickettsia salmonis. Dis Aquat Organisms (2017) 123(1):29–43. doi: 10.3354/dao03079
69. Fryer JL, Lannan CN, Giovannoni SJ, Wood ND. Piscirickettsia salmonis gen. nov., sp. nov., the causative agent of an epizootic disease in salmonid fishes. Int J Systematic Bacteriology (1992) 42(1):120–6. doi: 10.1099/00207713-42-1-120
70. Henríquez M, González E, Marshall SH, Henríquez V, Gómez FA, Martínez I, et al. A novel liquid medium for the efficient growth of the salmonid pathogen Piscirickettsia salmonis and optimization of culture conditions. PloS One (2013) 8(9):e71830. doi: 10.1371/journal.pone.0071830
71. Smith PA, Diaz FE, Rojas ME, Diaz S, Galleguillos M, Carbonero A. Effect of Piscirickettsia salmonis inoculation on the ASK continuous cell line. J Fish Dis (2015) 38(3):321–4. doi: 10.1111/jfd.12248
72. Andrews S. FastQC: a quality control tool for high throughput sequence data. Cambridge, United Kingdom: Babraham Bioinformatics, Babraham Institute (2010).
73. Ewels P, Magnusson M, Lundin S, Käller M. MultiQC: summarize analysis results for multiple tools and samples in a single report. Bioinformatics (2016) 32(19):3047–8. doi: 10.1093/bioinformatics/btw354
74. Bray NL, Pimentel H, Melsted P, Pachter L. Near-optimal probabilistic RNA-seq quantification. Nat Biotechnol (2016) 34:525–7. doi: 10.1038/nbt.3519
75. Durinck S, Moreau Y, Kasprzyk A, Davis S, De Moor B, Brazma A, et al. BioMart and Bioconductor: a powerful link between biological databases and microarray data analysis. Bioinf (Oxford England) (2005) 21(16):3439–40. doi: 10.1093/bioinformatics/bti525
76. Durinck S, Spellman PT, Birney E, Huber W. Mapping identifiers for the integration of genomic datasets with the R/Bioconductor package biomaRt. Nat Protoc (2009) 4(8):1184–91. doi: 10.1038/nprot.2009.97
77. Love M, Anders S, Huber W. Moderated estimation of fold change and dispersion for RNA-seq data with DESeq2. Genome Biol (2014) 15(550):10–1186. doi: 10.1186/s13059-014-0550-8
78. Brooks MD, Juang C-L, Katari MS, Alvarez JM, Pasquino A, Shih H-J, et al. ConnecTF: A platform to integrate transcription factor–gene interactions and validate regulatory networks. Plant Physiol (2021) 185(1):49–66. doi: 10.1093/plphys/kiaa012
79. Katari MS, Nowicki SD, Aceituno FF, Nero D, Kelfer J, Thompson LP, et al. VirtualPlant: a software platform to support systems biology research. Plant Physiol (2010) 152(2):500–15. doi: 10.1104/pp.109.147025
80. Moraleda CP, Robledo D, Gutiérrez AP, del-Pozo J, Yáñez JM, Houston RD. Investigating mechanisms underlying genetic resistance to Salmon Rickettsial Syndrome in Atlantic salmon using RNA sequencing. BMC Genomics (2021) 22(1):156. doi: 10.1186/s12864-021-07443-2
81. Gervais O, Peñaloza C, Gratacap R, Papadopoulou A, Beltrán M, Henderson NC, et al. Understanding host response to infectious salmon anaemia virus in an Atlantic salmon cell line using single-cell RNA sequencing. BMC Genomics (2023) 24(1):161. doi: 10.1186/s12864-023-09254-z
82. Samsing F, Alexandre P, Rigby M, Taylor RS, Chong R, Wynne JW. Transcriptome response of atlantic salmon (Salmo salar) to a new piscine orthomyxovirus. Pathog (Basel Switzerland) (2020) 9(10):807. doi: 10.3390/pathogens9100807
83. Kiron V, Park Y, Siriyappagouder P, Dahle D, Vasanth GK, Dias J, et al. Intestinal transcriptome analysis reveals soy derivative-linked changes in atlantic salmon. Front Immunol (2020) 11:596514. doi: 10.3389/fimmu.2020.596514
84. Zhang B, Horvath S. A general framework for weighted gene co-expression network analysis. Stat Appl Genet Mol Biol (2005) 4(1):17. doi: 10.2202/1544-6115.1128
85. Langfelder P, Horvath S. WGCNA: an R package for weighted correlation network analysis. BMC Bioinf (2008) 9(1):559. doi: 10.1186/1471-2105-9-559
86. Bindea G, Mlecnik B, Hackl H, Charoentong P, Tosolini M, Kirilovsky A, et al. ClueGO: a Cytoscape plug-in to decipher functionally grouped gene ontology and pathway annotation networks. Bioinformatics (2009) 25(8):1091–3. doi: 10.1093/bioinformatics/btp101
87. Bindea G, Galon J, Mlecnik B. CluePedia Cytoscape plugin: pathway insights using integrated experimental and in silico data. Bioinformatics (2013) 29(5):661–3. doi: 10.1093/bioinformatics/btt019
88. Shannon P, Markiel A, Ozier O, Baliga NS, Wang JT, Ramage D, et al. Cytoscape: a software environment for integrated models of biomolecular interaction networks. Genome Res (2003) 13(11):2498–504. doi: 10.1101/gr.1239303
89. Mulugeta TD, Nome T, To T-H, Gundappa MK, Macqueen DJ, Våge DI, et al. SalMotifDB: a tool for analyzing putative transcription factor binding sites in salmonid genomes. BMC Genomics (2019) 20(1):1–8. doi: 10.1186/s12864-019-6051-0
90. Bastian M, Heymann S, Jacomy M. Gephi: An open source software for exploring and manipulating networks. Proceedings of the international aaai conference on web and social media. PKP publishing services network, 8888 University Drive, Burnaby, B.C., Canada (2009) 3(1):361–2. doi: 10.1609/icwsm.v3i1.13937
91. Xue X, Caballero-Solares A, Hall JR, Umasuthan N, Kumar S, Jakob E, et al. Transcriptome Profiling of Atlantic Salmon (Salmo salar) Parr With Higher and Lower Pathogen Loads Following Piscirickettsia salmonis Infection. Front Immunol (2021) 12:789465. doi: 10.3389/fimmu.2021.789465
92. Murgas L, Contreras-Riquelme S, Martínez-Hernandez JE, Villaman C, Santibáñez R, Martin AJM. Automated generation of context-specific gene regulatory networks with a weighted approach in Drosophila melanogaster. Interface Focus (2021) 11(4):20200076. doi: 10.1098/rsfs.2020.0076
93. Climaco-Arvizu S, Domínguez-Acosta O, Cabañas-Cortés MA, Rodríguez-Sosa M, Gonzalez FJ, Vega L, et al. Aryl hydrocarbon receptor influences nitric oxide and arginine production and alters M1/M2 macrophage polarization. Life Sci (2016) 155:76–84. doi: 10.1016/j.lfs.2016.05.001
94. Hamada M, Tsunakawa Y, Jeon H, Yadav MK, Takahashi S. Role of mafB in macrophages. Exp Anim (2020) 69(1):1–10. doi: 10.1538/expanim.19-0076
95. Hwang J, Kita R, Kwon H-S, Choi EH, Lee SH, Udey MC, et al. Epidermal ablation of Dlx3 is linked to IL-17-associated skin inflammation. Proc Natl Acad Sci United States America (2011) 108(28):11566–71. doi: 10.1073/pnas.1019658108
96. Liao X, Sharma N, Kapadia F, Zhou G, Lu Y, Hong H, et al. Krüppel-like factor 4 regulates macrophage polarization. J Clin Invest (2011) 121(7):2736–49. doi: 10.1172/JCI45444
97. Sanz-Morejón A, García-Redondo AB, Reuter H, Marques IJ, Bates T, Galardi-Castilla M, et al. Wilms tumor 1b expression defines a pro-regenerative macrophage subtype and is required for organ regeneration in the zebrafish. Cell Rep (2019) 28(5):1296–1306.e6. doi: 10.1016/j.celrep.2019.06.091
98. Yang X, Liu H, Ye T, Duan C, Lv P, Wu X, et al. AhR activation attenuates calcium oxalate nephrocalcinosis by diminishing M1 macrophage polarization and promoting M2 macrophage polarization. Theranostics (2020) 10(26):12011–25. doi: 10.7150/thno.51144
99. Klein-Hessling S, Muhammad K, Klein M, Pusch T, Rudolf R, Flöter J, et al. NFATc1 controls the cytotoxicity of CD8+ T cells. Nat Commun (2017) 8(1):511. doi: 10.1038/s41467-017-00612-6
100. López-López S, Monsalve EM, Romero de Ávila MJ, González-Gómez J, Hernández de León N, Ruiz-Marcos F, et al. NOTCH3 signaling is essential for NF-κB activation in TLR-activated macrophages. Sci Rep (2020) 10(1):14839. doi: 10.1038/s41598-020-71810-4
101. Ayer DE, Eisenman RN. A switch from Myc : Max to Mad : Max heterocomplexes accompanies monocyte/macrophage differentiation. Genes Dev (1993) 7(11):2110–9. doi: 10.1101/gad.7.11.2110
102. Casalino-Matsuda SM, Chen F, Gonzalez-Gonzalez FJ, Matsuda H, Nair A, Abdala-Valencia H, et al. Myeloid zfhx3 deficiency protects against hypercapnia-induced suppression of host defense against influenza A virus. bioRxiv (2023). doi: 10.1101/2023.02.28.530480
103. Liang Z-Q, Zhong L-Y, Li J, Shen J-H, Tu X-Y, Zhong Z-H, et al. Clinicopathological significance and underlying molecular mechanism of downregulation of basonuclin 1 expression in ovarian carcinoma. Exp Biol Med (2022) 247(2):106–19. doi: 10.1177/15353702211052036
104. Lin L, Hu K. Tissue-type plasminogen activator modulates macrophage M2 to M1 phenotypic change through annexin A2-mediated NF-κB pathway. Oncotarget (2017) 8(50):88094–103. doi: 10.18632/oncotarget.21510
105. Luquero A, Vilahur G, Crespo J, Badimon L, Borrell‐Pages M. Microvesicles carrying LRP5 induce macrophage polarization to an anti-inflammatory phenotype. J Cell Mol Med (2021) 25(16):7935–47. doi: 10.1111/jcmm.16723
106. Ratliff ML, Garton J, Garman L, Barron MD, Georgescu C, White KA, et al. ARID3a gene profiles are strongly associated with human interferon alpha production. J Autoimmun (2019) 96:158–67. doi: 10.1016/j.jaut.2018.09.013
107. Truxova I, Cibula D, Spisek R, Fucikova J. Targeting tumor-associated macrophages for successful immunotherapy of ovarian carcinoma. J ImmunoTherapy Cancer (2023) 11(2):e005968. doi: 10.1136/jitc-2022-005968
108. Xie C, Liu C, Wu B, Lin Y, Ma T, Xiong H, et al. Effects of IRF1 and IFN-β interaction on the M1 polarization of macrophages and its antitumor function. Int J Mol Med (2016) 38(1):148–60. doi: 10.3892/ijmm.2016.2583
109. Chen A-N, Luo Y, Yang Y-H, Fu J-T, Geng X-M, Shi J-P, et al. Lactylation, a novel metabolic reprogramming code: current status and prospects. Front Immunol (2021) 12:688910. doi: 10.3389/fimmu.2021.688910
110. Luan B, Yoon Y-S, Le Lay J, Kaestner KH, Hedrick S, Montminy M. CREB pathway links PGE2 signaling with macrophage polarization. Proc Natl Acad Sci United States America (2015) 112(51):15642–7. doi: 10.1073/pnas.1519644112
111. Malik N, Yan H, Moshkovich N, Palangat M, Yang H, Sanchez V, et al. The transcription factor CBFB suppresses breast cancer through orchestrating translation and transcription. Nat Commun (2019) 10(1):2071. doi: 10.1038/s41467-019-10102-6
112. Nowak W, Grendas LN, Sanmarco LM, Estecho IG, Arena ÁR, Eberhardt N, et al. Pro-inflammatory monocyte profile in patients with major depressive disorder and suicide behaviour and how ketamine induces anti-inflammatory M2 macrophages by NMDAR and mTOR. EBioMedicine (2019) 50:290–305. doi: 10.1016/j.ebiom.2019.10.063
113. Polumuri S, Perkins DJ, Vogel SN. cAMP levels regulate macrophage alternative activation marker expression. Innate Immun (2021) 27(2):133–42. doi: 10.1177/1753425920975082
114. Lu L, McCurdy S, Huang S, Zhu X, Peplowska K, Tiirikainen M, et al. Time Series miRNA-mRNA integrated analysis reveals critical miRNAs and targets in macrophage polarization. Sci Rep (2016) 6:37446. doi: 10.1038/srep37446
115. Benanti JA, Williams DK, Robinson KL, Ozer HL, Galloway DA. Induction of extracellular matrix-remodeling genes by the senescence-associated protein APA-1. Mol Cell Biol (2002) 22(21):7385–97. doi: 10.1128/MCB.22.21.7385-7397.2002
116. Bidault G, Virtue S, Petkevicius K, Jolin HE, Dugourd A, Guénantin A-C, et al. SREBP1-induced fatty acid synthesis depletes macrophages antioxidant defences to promote their alternative activation. Nat Metab (2021) 3(9):1150–62. doi: 10.1038/s42255-021-00440-5
117. Kusnadi A, Park SH, Yuan R, Pannellini T, Giannopoulou E, Oliver D, et al. The cytokine TNF promotes transcription factor SREBP activity and binding to inflammatory genes to activate macrophages and limit tissue repair. Immunity (2019) 51(2):241–257.e9. doi: 10.1016/j.immuni.2019.06.005
118. Martínez DP, Oliver C, Santibañez N, Coronado JL, Oyarzún-Salazar R, Enriquez R, et al. PAMPs of piscirickettsia salmonis trigger the transcription of genes involved in nutritional immunity in a salmon macrophage-like cell line. Front Immunol (2022) 13:849752. doi: 10.3389/fimmu.2022.849752
119. Tarique AA, Logan J, Thomas E, Holt PG, Sly PD, Fantino E. Phenotypic, functional, and plasticity features of classical and alternatively activated human macrophages. Am J Respir Cell Mol Biol (2015) 53(5):676–88. doi: 10.1165/rcmb.2015-0012OC
120. Glim JE, Niessen FB, Everts V, van Egmond M, Beelen RHJ. Platelet derived growth factor-CC secreted by M2 macrophages induces alpha-smooth muscle actin expression by dermal and gingival fibroblasts. Immunobiology (2013) 218(6):924–9. doi: 10.1016/j.imbio.2012.10.004
121. Fernandes TL, Gomoll AH, Lattermann C, Hernandez AJ, Bueno DF, Amano MT. Macrophage: A potential target on cartilage regeneration. Front Immunol (2020) 11:111. doi: 10.3389/fimmu.2020.00111
122. Fujisaka S, Usui I, Nawaz A, Takikawa A, Kado T, Igarashi Y, et al. M2 macrophages in metabolism. Diabetol Int (2016) 7(4):342–51. doi: 10.1007/s13340-016-0290-y
123. Morris PG, Hudis CA, Giri D, Morrow M, Falcone DJ, Zhou XK, et al. Inflammation and increased aromatase expression occur in the breast tissue of obese women with breast cancer. Cancer Prev Res (Philadelphia Pa.) (2011) 4(7):1021–9. doi: 10.1158/1940-6207.CAPR-11-0110
124. Eastell R, Hannon RA. CHAPTER 27 - biochemical markers of bone turnover. In: Lobo RA, editor. Treatment of the Postmenopausal Woman, 3rd ed. St. Louis: Academic Press (2007). p 337–49.
125. Muniz-Bongers LR, McClain CB, Saxena M, Bongers G, Merad M, Bhardwaj N. MMP2 and TLRs modulate immune responses in the tumor microenvironment. JCI Insight (2021) 6(12):e144913. doi: 10.1172/jci.insight.144913
126. Zhang H, Liu L, Jiang C, Pan K, Deng J, Wan C. MMP9 protects against LPS-induced inflammation in osteoblasts. Innate Immun (2020) 26(4):259–69. doi: 10.1177/1753425919887236
127. Koeppen K, Hampton TH, Jarek M, Scharfe M, Gerber SA, Mielcarz DW, et al. A Novel Mechanism of Host-Pathogen Interaction through sRNA in Bacterial Outer Membrane Vesicles. PloS Pathog (2016) 12(6):e1005672. doi: 10.1371/journal.ppat.1005672
128. Westermann AJ, Förstner KU, Amman F, Barquist L, Chao Y, Schulte LN, et al. Dual RNA-seq unveils noncoding RNA functions in host-pathogen interactions. Nature (2016) 529(7587):496–501. doi: 10.1038/nature16547
129. An Z-j, Li Y, Tan B-B, Zhao Q, Fan L-Q, Zhang Z-D, et al. Up-regulation of KLF17 expression increases the sensitivity of gastric cancer to 5-fluorouracil. Int J Immunopathol Pharmacol (2021) 35:20587384211010925. doi: 10.1177/20587384211010925
130. Cai X-D, Che L, Lin J-X, Huang S, Li J, Liu X-Y, et al. Krüppel-like factor 17 inhibits urokinase plasminogen activator gene expression to suppress cell invasion through the Src/p38/MAPK signaling pathway in human lung adenocarcinoma. Oncotarget (2017) 8(24):38743–54. doi: 10.18632/oncotarget.17020
131. Liu F-Y, Deng Y-L, Li Y, Zeng D, Zhou Z-Z, Tian D-A, et al. Down-regulated KLF17 expression is associated with tumor invasion and poor prognosis in hepatocellular carcinoma. Med Oncol (Northwood London England) (2013) 30(1):425. doi: 10.1007/s12032-012-0425-3
132. Qiang Y-W, Ye S, Huang Y, Chen Y, Van Rhee F, Epstein J, et al. MAFb protein confers intrinsic resistance to proteasome inhibitors in multiple myeloma. BMC Cancer (2018) 18:724. doi: 10.1186/s12885-018-4602-4
Keywords: Piscirickettsia salmonis, host-pathogen interaction, macrophage polarization, gene regulatory network, Atlantic salmon
Citation: Pérez-Stuardo D, Frazão M, Ibaceta V, Brianson B, Sánchez E, Rivas-Pardo JA, Vallejos-Vidal E, Reyes-López FE, Toro-Ascuy D, Vidal EA and Reyes-Cerpa S (2023) KLF17 is an important regulatory component of the transcriptomic response of Atlantic salmon macrophages to Piscirickettsia salmonis infection. Front. Immunol. 14:1264599. doi: 10.3389/fimmu.2023.1264599
Received: 21 July 2023; Accepted: 07 November 2023;
Published: 14 December 2023.
Edited by:
Gokhlesh Kumar, University of Veterinary Medicine Vienna, AustriaReviewed by:
Kumar Pavanish, Singhealth/Duke-NUS Acedemic Medical Centre, SingaporeCopyright © 2023 Pérez-Stuardo, Frazão, Ibaceta, Brianson, Sánchez, Rivas-Pardo, Vallejos-Vidal, Reyes-López, Toro-Ascuy, Vidal and Reyes-Cerpa. This is an open-access article distributed under the terms of the Creative Commons Attribution License (CC BY). The use, distribution or reproduction in other forums is permitted, provided the original author(s) and the copyright owner(s) are credited and that the original publication in this journal is cited, in accordance with accepted academic practice. No use, distribution or reproduction is permitted which does not comply with these terms.
*Correspondence: Elena A. Vidal, ZWxlbmEudmlkYWxAdW1heW9yLmNs; Sebastián Reyes-Cerpa, c2ViYXN0aWFuLnJleWVzQHVtYXlvci5jbA==
Disclaimer: All claims expressed in this article are solely those of the authors and do not necessarily represent those of their affiliated organizations, or those of the publisher, the editors and the reviewers. Any product that may be evaluated in this article or claim that may be made by its manufacturer is not guaranteed or endorsed by the publisher.
Research integrity at Frontiers
Learn more about the work of our research integrity team to safeguard the quality of each article we publish.