- 1Center for Microbial Diseases and Immunity Research, University of British Columbia, Vancouver, BC, Canada
- 2Department of Molecular Biology and Biochemistry, Simon Fraser University, Burnaby, BC, Canada
- 3Keenan Research Center for Biomedical Science and the Department of Critical Care, St. Michael’s Hospital, University of Toronto, Toronto, ON, Canada
- 4Department of Critical Care, Galilee Medical Center, Nahariya, Israel
Introduction: Severe COVID-19 and non-COVID-19 pulmonary sepsis share pathophysiological, immunological, and clinical features, suggesting that severe COVID-19 is a form of viral sepsis. Our objective was to identify shared gene expression trajectories strongly associated with eventual mortality between severe COVID-19 patients and contemporaneous non-COVID-19 sepsis patients in the intensive care unit (ICU) for potential therapeutic implications.
Methods: Whole blood was drawn from 20 COVID-19 patients and 22 non-COVID-19 adult sepsis patients at two timepoints: ICU admission and approximately a week later. RNA-Seq was performed on whole blood to identify differentially expressed genes and significantly enriched pathways. Using systems biology methods, drug candidates targeting key genes in the pathophysiology of COVID-19 and sepsis were identified.
Results: When compared to survivors, non-survivors (irrespective of COVID-19 status) had 3.6-fold more “persistent” genes (genes that stayed up/downregulated at both timepoints) (4,289 vs. 1,186 genes); these included persistently downregulated genes in T-cell signaling and persistently upregulated genes in select innate immune and metabolic pathways, indicating unresolved immune dysfunction in non-survivors, while resolution of these processes occurred in survivors. These findings of persistence were further confirmed using two publicly available datasets of COVID-19 and sepsis patients. Systems biology methods identified multiple immunomodulatory drug candidates that could target this persistent immune dysfunction, which could be repurposed for possible therapeutic use in both COVID-19 and sepsis.
Discussion: Transcriptional evidence of persistent immune dysfunction was associated with 28-day mortality in both COVID-19 and non-COVID-19 septic patients. These findings highlight the opportunity for mitigating common mechanisms of immune dysfunction with immunomodulatory therapies for both diseases.
1 Introduction
As of July 2023, the COVID-19 pandemic has infected >650 million and killed 6-18 million people globally (1, 2). COVID-19 patients who are admitted to the intensive care unit (ICU) have high mortality rates of up to 32%, with multiple organ failure causing the majority of these deaths (3). This is strikingly similar to severe sepsis, which is life-threatening organ failure caused by a dysregulated host response to infection (4), which is often a bacterial infection but can also be viral and fungal etiologies. Sepsis is estimated to kill 11 million people each year and be involved in 1 in 5 deaths globally (5), having average 30-day mortality rates of 24.4% for sepsis and 34.7% for septic shock in North America, Europe, and Australia (6), and even higher in lower and middle income countries (7). Due to similarities in immune dysfunction, endothelial disruption, cytokine levels, gene expression, and long-term consequences, there is a growing consensus that severe COVID-19 should be classified and treated as a form of viral-associated sepsis (8). We recently have shown that our sepsis endotypes can accurately classify COVID-19 patients based on severity (9, 10) and that contemporaneous severe COVID-19 and non-COVID-19 sepsis patients converge into transcriptionally indistinguishable mechanisms after a week in the ICU (11).
Despite high mortality rates, no specific treatment for sepsis is available other than antibiotics and supportive management (e.g., fluid resuscitation) (12). The lack of treatments is not due to a lack of effort, as exemplified by over 30 years of failed sepsis clinical trials (13). However, these clinical trials focused only on the inflammatory aspect of sepsis, while it has become evident that a concurrent immunosuppressive arm of sepsis is also occurring, potentially as a means to limit life-threatening inflammation (14). Thus, simply using immunosuppressive/anti-inflammatory therapies for sepsis patients can exacerbate this immune dysregulation and exposes the patient to lethal opportunistic pathogens. These concurrent immunosuppression and inflammation processes are part of a syndrome termed “Persistent Inflammation, Immunosuppression, and Catabolism Syndrome” (PICS), that is proposed to occur in septic ICU patients with disastrous consequences including recurrent nosocomial infections, poor wound healing, inability of self-care, and eventual death (15), but has not been defined mechanistically. Further research into the specific mechanisms underlying why patients progress to death is critically needed for development of targeted therapies for sepsis. Previous work in the Hancock Lab found five endotypes at early disease presentation, two of which were correlated with higher mortality rates (Neutrophilic-Suppressive and Inflammatory), and a cross-cutting mortality signature was identified (9). Collectively these suggest both mechanistically-variable and conserved pathways are involved in mortality. In addition to these genetic biomarkers, many blood biomarkers have been shown to predict disease severity and mortality in COVID-19, such as C-reactive protein, procalcitonin, D-dimer, interleukin-6, lactate dehydrogenase, ferritin, plasma Gas6, lymphopenia, and thrombocytopenia (16–19), with many previously investigated in sepsis (20).
There has been an unprecedented level of scientific interest and funding, as well as success, for the treatment of COVID-19, resulting in clinical trials discovering effective therapies. The use of immunomodulators such as corticosteroids (21), tocilizumab (22), and baricitinib (23) has shown promising effects on reducing mortality and other severity metrics. If mortality mechanisms are shared between COVID-19 and sepsis patients, these promising results will likely have applications to sepsis therapeutics. Nevertheless, both sepsis and COVID-19 are highly dynamic diseases (11, 14, 24), requiring analysis of multiple timepoints to fully understand disease trajectories and uncover additional pathophysiology that cannot be detected from a single timepoint.
In this study, we aimed to identify shared mechanistic trajectories related to mortality in severe COVID-19 patients and contemporaneous non-COVID-19 sepsis patients. Shared gene expression changes over time could underscore common mechanisms of injury and/or repair, with broad therapeutic implications. We showed that persistent immune dysfunction was highly associated with patients who died regardless of SARS-CoV-2 positivity, a finding that was replicated in other public datasets of COVID-19 and sepsis patients. Based on this finding of persistence, we also identified potential treatments targeting these persistent genes in both COVID-19 and non-COVID-19 sepsis.
2 Materials and methods
2.1 Study design and sample collection
Between March 2020 and February 2021, the prospective observational “COVID-19 Longitudinal Biomarkers of Lung Injury” (COLOBILI) study consented and enrolled 42 ICU adult (>18 years) patients with respiratory deterioration from suspected COVID-19 at St. Michael’s Hospital (Toronto, Canada) (Table 1). Whole blood (2.5 mL) was drawn into PaxGene Blood RNA tubes (BD Biosciences) at admission (Day 1, D1) and Day 7 (D7) in the ICU. After enrollment, 20 patients were identified to be SARS-CoV-2 PCR positive (but blood culture negative at both timepoints), and the remaining 22 SARS-CoV-2 PCR negative patients had ≥2 negative PCR tests. All patients satisfied Sepsis-3 criteria for sepsis (suspected/confirmed infection with a SOFA score ≥2 at ICU admission) (4). After the second blood draw, nine patients (4 SARS-CoV-2 positive, 5 negative) died within 28 days in the ICU. Samples were frozen and transported to Vancouver, Canada, for RNA extraction (PAXgene Blood RNA Kit; Qiagen) followed by RNA-Seq. Whole blood from 5 healthy controls from Vancouver, Canada were processed alongside the patient samples. Further details on study design and RNA-Seq methodology can be found in our previously published protocol (11).
2.2 Bioinformatic and statistical analysis
Differentially expressed (DE) genes were identified between different patient subgroups using the DESeq2 package (25), with DE genes defined as having an adjusted p-value <0.05 (Benjamini-Hochberg correction) and an absolute fold change ≥1.5. The DESeq2 model included sex and batch as confounders to adjust for. Up-/down-regulated DE genes were used to identify significantly enriched pathways/gene sets from the Reactome pathways database (26) using gene-pair overrepresentation analysis with SIGORA (Bonferroni adjusted P-value <0.001), and from the Molecular Signatures Database Hallmark gene sets (27) using overrepresentation analysis from clusterProfiler (28) (Benjamini-Hochberg adjusted P-value <0.05). Significantly enriched pathways/gene sets indicated key dysregulated biological processes. Gene-drug interactions from the Drug Signatures Database (29) were also analyzed in a similar fashion as pathway enrichment using clusterProfiler. Pathway plots, volcano plots, and fold change heatmaps were generated using pathlinkR (https://github.com/hancockinformatics/pathlinkR). Confirmatory studies were done using publicly available datasets GSE196117 (30) and GSE161918 (28).
3 Results
3.1 Non-survivors had substantially more persistent genes compared to survivors
To understand the determinants of gene expression variation, unsupervised principal component analysis (PCA) was used. PCA summarizes gene expression variation into individual principal components (PCs), where the PCs are numbered based on the fraction of variation they describe. PCA of the ICU samples (Figure S1A) demonstrated that eventual mortality, disease severity (SOFA score), and sample collection time were significantly correlated with PC1 (the PC with the largest percentage of variation, 22.5%), while COVID-19 status was only significantly correlated with the smaller PC3 (9.1% of variation) (Figure S1B). This suggested that the pathogen had a smaller impact on overall gene expression variation when compared to eventual mortality in the ICU and disease stage. The importance of mortality and sampling time to gene expression variation prompted a thorough investigation of gene expression trajectories in survivors and non-survivors.
First, the transcriptional profiles of survivors and non-survivors were compared to healthy controls to determine whether there were “persistent” genes, i.e., DE genes that remained consistently up/downregulated throughout the first week of ICU compared to healthy controls, indicating unresolved dysfunction (Figure 1B). Non-survivors had 3.6-fold the number of persistent genes when compared to survivors (4,289 vs 1,186) (Figure 1A), and this trend of more persistent genes in non-survivors was present even when separating into COVID-19 and non-COVID-19 sepsis patients (Figure S2C). Notable persistent genes found only in non-survivors included immune genes such as IL1R1/2, IL4R, IRAK3, ZAP70, and the sepsis mortality gene PCSK9 (29).
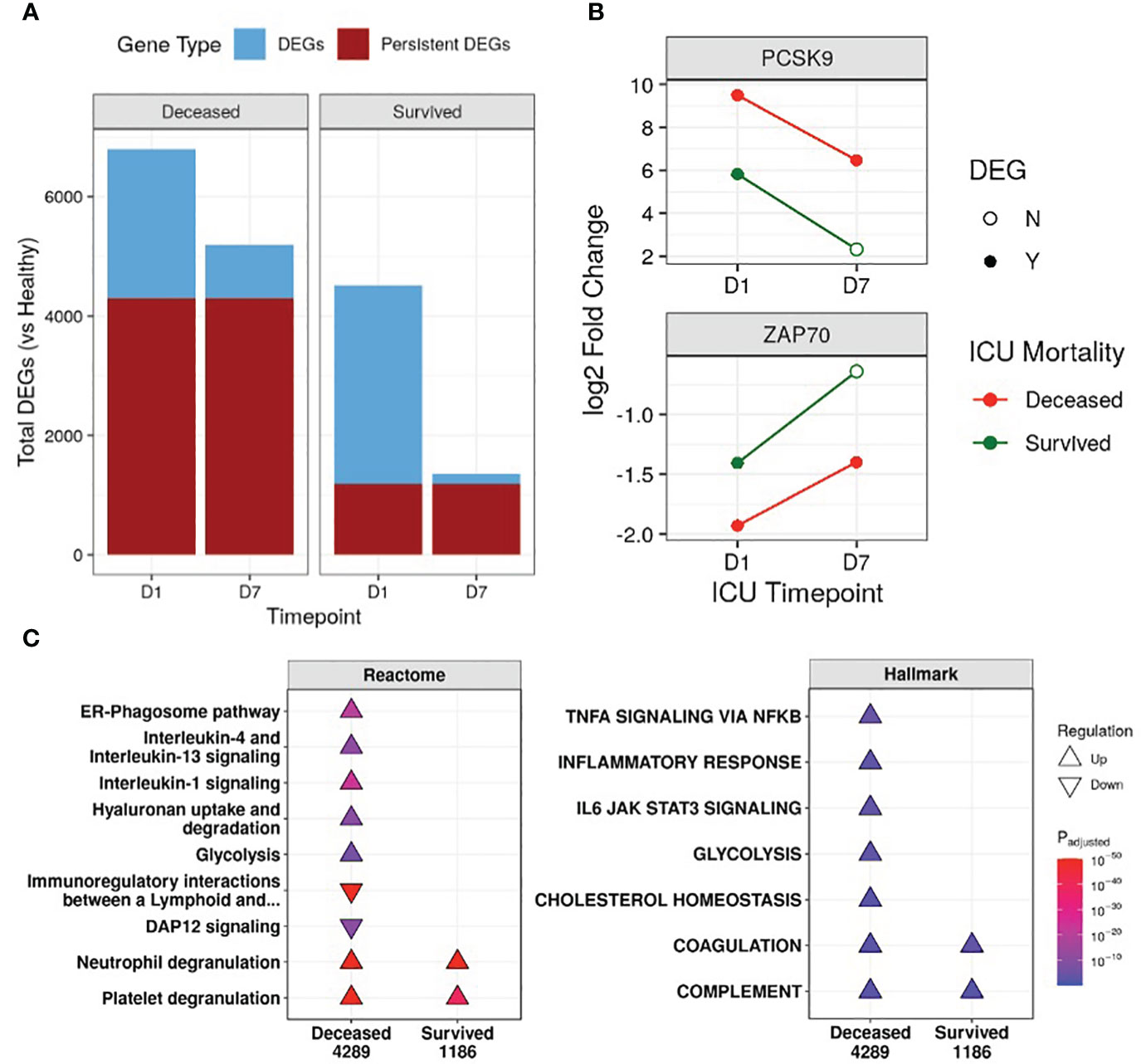
Figure 1 Non-survivors had substantially more persistent genes than survivors. (A) Number of differentially expressed genes (DEGs) at either Day 1 (D1) or Day 7 (D7) in the ICU for non-survivors and survivors, compared to healthy controls. The fraction of persistent DEGs (DEGs that were up/down-regulated at both timepoints) among all DEGs is highlighted. (B) Examples of persistently upregulated (PCSK9) or persistently downregulated (ZAP70) genes only found in non-survivors are shown. Empty circles indicate that the gene was no longer a DEG at that timepoint. (C) Eventually deceased patients had persistent dysfunction of immune and cellular pathways. A subset of enriched Reactome pathways and Hallmark gene sets from persistently upregulated (Δ) and downregulated (∇) genes in patients who eventually were deceased or survived are shown. The total numbers of persistent genes in each comparison are under each label. All enriched pathways and gene sets can be found in Figures S5 and S6. Pathway plots were generated using pathlinkR (https://github.com/hancockinformatics/pathlinkR).
Since persistence suggested potential long-term defects that might be related to genetic differences, we compared these persistent genes to four genome-wide association studies of sepsis (31, 32) and COVID-19 severity (33, 34) (Table S2). Fifty persistent genes overlapped with GWAS identified genes, with almost all (46/50) found in non-survivors (Table S2). These included sepsis severity genes such as PCSK9, CACNA2D2, and HEMK1 (31, 32), and COVID-19 severity genes such as IL10RB, TYK2, and F8 (33, 34) (Table S2). Interestingly, ICAM1 (intercellular adhesion molecule 1) was found in GWAS studies for both sepsis and COVID-19 and was also a persistently upregulated gene only in non-survivors, suggesting persistent dysregulation of this gene may be involved in worse outcomes for both diseases. Increased surface expression of ICAM-1 on leukocytes occurs during inflammation to promote leukocyte adhesion and extravasation (35). Circulating ICAM-1, a marker of endothelial damage, has been documented to be elevated in sepsis and COVID-19 patients, with higher levels associated with increased severity (36–38).
Persistence also implicated epigenetic regulation; we found 34 genes that overlapped between persistent genes in non-survivors and differentially methylated genes of sepsis patients identified in a previous study (39) and those of COVID-19 patients (40, 41) (Figure S3A), suggesting the potential presence of epigenetic switches that might be responsible for persistent dysregulation of genes. Notably, these overlapping genes were mostly immune-related genes, such as CD177, CD3D, and S100P (Figure S3B, C). Lastly, the concept of substantially more persistent genes in patients with worse outcomes was also replicated/validated in 91 samples from two external longitudinal datasets of critically ill sepsis (30) and COVID-19 patients (42) (Figure S4).
3.2 Unresolved immune dysfunction was associated with eventual ICU mortality
We next investigated the functional consequences of persistent genes in non-survivors. Pathway enrichment identified 46 and 10 unique pathways enriched by persistent genes in non-survivors and survivors, respectively, with 6 shared pathways (Figure S5). In non-survivors, these unique Reactome pathways included pathways from the “Immune System”, “Metabolism”, “Metabolism of RNA”, “Metabolism of proteins”, and “Organelle biosynthesis and management” categories, suggesting persistent dysfunction in multiple aspects of cellular function (Figure S5). Specifically, in non-survivors, interleukin (IL) and inflammatory pathways (“IL-1 signaling”, “IL-4/13 signaling”, “ER-phagosome pathway”) were persistently upregulated, while adaptive immune activation pathways such as “Immunoregulatory interactions between a lymphoid and a non-lymphoid cell” and “DAP12 signaling” were persistently downregulated (Figure 1C). This indicated enduring immune dysfunction in non-survivors, but not in survivors, and was recapitulated by the Hallmark gene sets “Inflammatory Response”, “TNFα signaling via NF-kB”, and “IL-6 JAK STAT3 signaling” in non-survivors (Figure 1C). Interestingly, the gene sets “Cholesterol homeostasis” and “Glycolysis” and the pathway “Hyaluronan uptake and degradation” were also persistently upregulated only in non-survivors, highlighting known metabolic dysfunctions associated with worse outcomes for sepsis (43–45). The “Coagulation” and “Complement” gene sets and the “Neutrophil degranulation” and “Platelet degranulation” pathways were persistently upregulated in all patients (Figure 1C), suggesting shared immune and coagulation dysfunction among all patients in the ICU.
Many of these immune pathways (“IL-1 signaling”, “IL-4/13 signaling”, “Neutrophil degranulation”, and “Immunoregulatory interactions”) were also enriched by persistent genes in the two validation datasets of COVID-19 and sepsis patients (Figure S4), as well as in persistent genes of non-survivors even after splitting into COVID-19 and non-COVID-19 sepsis patients (Figure S2D), suggesting shared mechanisms of mortality between these two diseases. This idea of shared mortality mechanisms was further supported by the comparison of COVID-19 to non-COVID-19 sepsis non-survivors at D1 and D7. There were 275 DE genes at D1 between COVID-19 and non-COVID-19 sepsis non-survivors (mostly enriching for antiviral pathways, reflective of the pathogen-specific response), which dropped to just one DE gene at D7, suggesting a convergence to shared mortality mechanisms over time (Figures S2A, B).
To further investigate persistent immune dysfunction, enrichment using all DE genes of survivors and non-survivors vs. healthy controls, not just persistent genes, was performed (Figure 2). This analysis demonstrated that while many of these pathways were enriched in both survivors and non-survivors at D1, they were only enriched in non-survivors at D7, suggesting that initially, all patients had immune dysfunction, but only survivors appeared to resolve their immune dysfunction and accompanying metabolic dysfunction (e.g., glycolysis, hyaluronan metabolism, cholesterol metabolism) by D7 (Figure 2). Inflammatory resolution in survivors was also supported by enrichment results from DE genes that changed over time (Figure 3A). For example, the “Inflammatory response” gene set was upregulated in all patients at D1 compared to healthy controls, but only in non-survivors at D7 (Figure 2). This gene set was downregulated over time only in survivors (Figure 3A). Overall, this enrichment pattern highlighted that the general inflammatory response was resolved by D7 in survivors. A similar pattern underscoring persistent upregulation of the “IL-1 signaling” and “IL-4/13 signaling” pathways and the inflammatory gene sets “TNFα signaling via NF-kB” and “IL6 JAK STAT3 signaling,” was also observed only in non-survivors (Figures 2, 3A), again supporting an unresolved inflammatory state in non-survivors but resolution in survivors.
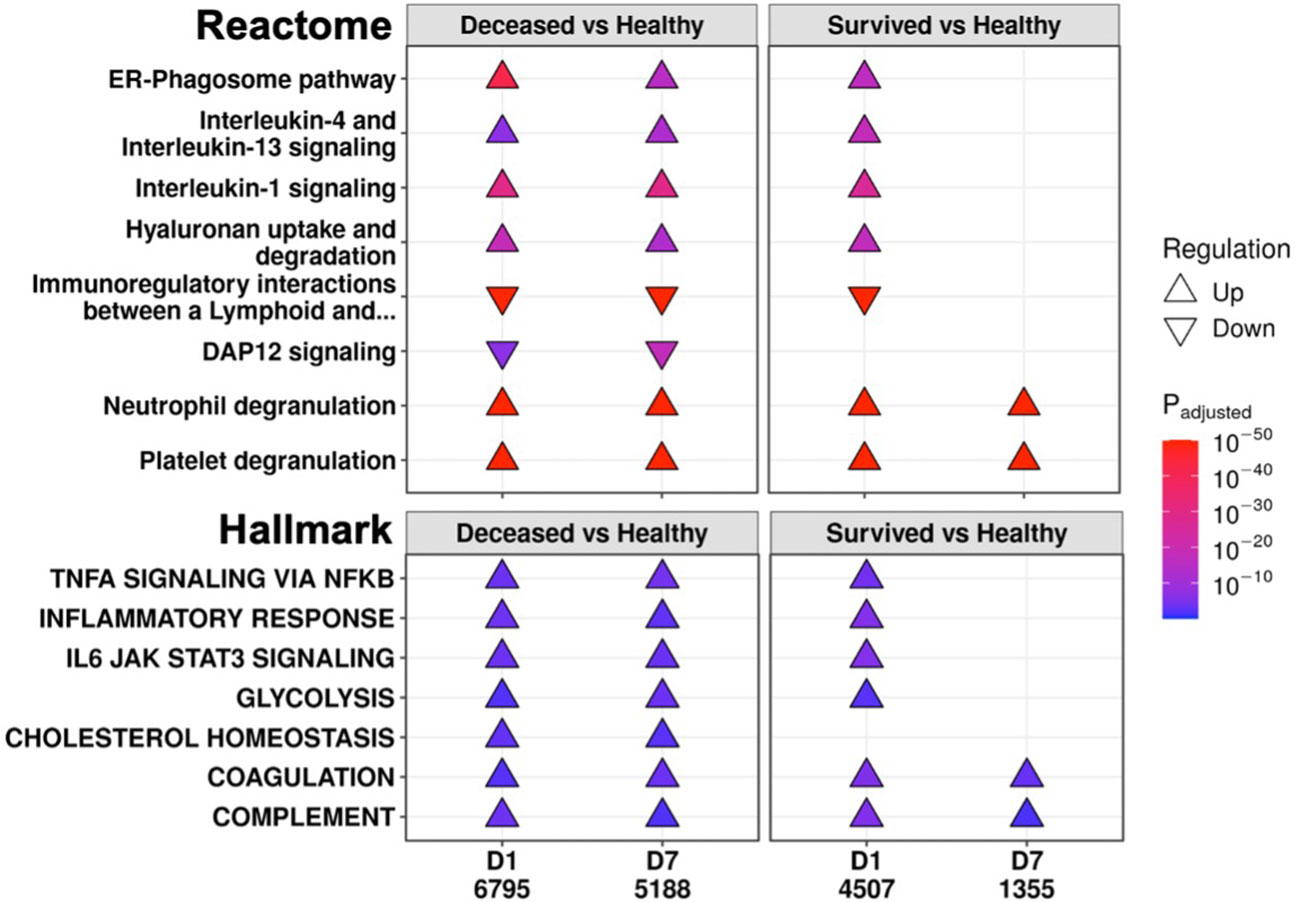
Figure 2 Eventually deceased patients had unresolving immune dysfunction. A subset of significantly enriched Reactome pathways (top) and Hallmark gene sets (bottom) using differentially expressed (DE) genes from comparing eventually deceased or surviving patients to healthy controls at Day 1 (D1) and Day 7 (D7). The total numbers of DE genes in each comparison are under each label. All enriched pathways and gene sets shown in Figures S6 and S7. Pathway plots were generated using pathlinkR.
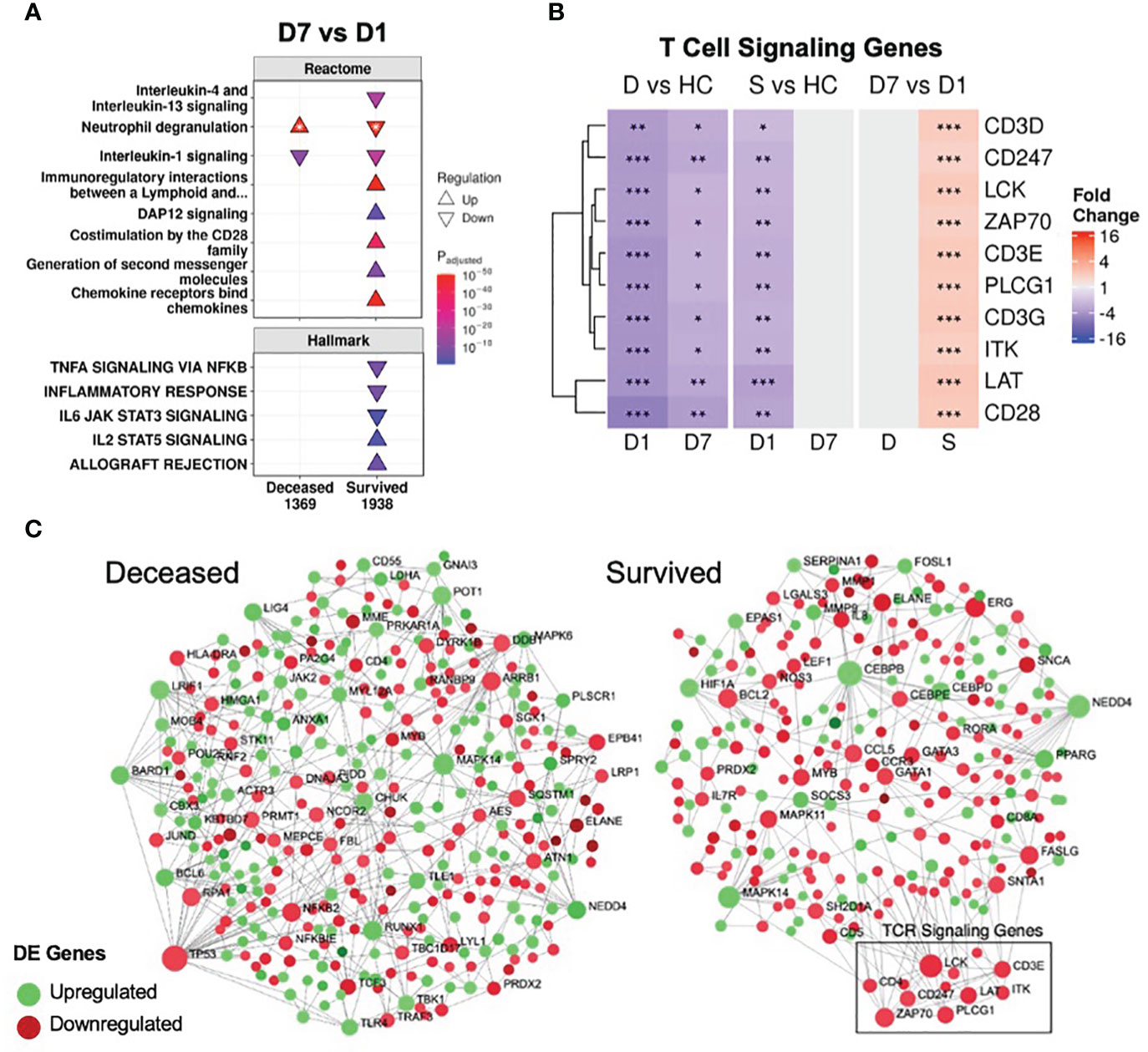
Figure 3 Resolution of inflammation and adaptive suppression occurred only in survivors. (A) A subset of significantly enriched Reactome pathways and Hallmark gene sets using differentially expressed (DE) genes over time. The total numbers of DE genes in each comparison are under each label. All enriched pathways and gene sets shown in Figures S6 and S7. For one pathway, both directions were enriched (indicated by *); the direction with the lower adjusted p-value (more significantly enriched) is shown. (B) Persistent suppression of T cell signaling genes was observed in non-survivors. D = deceased, S = survived, HC = healthy controls. Shading in the heatmap represents fold change. Only DE genes are shown. Significance values are derived from DESeq2 results: *** = p<0.001, ** = p<0.01, and * = p<0.05. Pathway plots and heatmaps were generated using pathlinkR. (C) Network analysis of DE genes over time in survivors and non-survivors. Zero-order (i.e., only dysregulated nodes) functional protein-protein interactions (PPI) networks were drawn using NetworkAnalyst. Dots represent nodes (genes and their protein products) and are colored for directionality. Lines joining the dots represent known PPI from InnateDB. Differential expression of TCR signaling genes was not seen in non-survivors but these genes were upregulated over time in survivors (boxed area).
In conjunction with resolving inflammation, survivors also resolved adaptive immune suppression, which was likely key to their survival, since a weakened adaptive immune response is strongly implicated in sepsis and COVID-19 severity/mortality (46, 47). The “Immunoregulatory interactions” pathway (which contains B-cell and T-cell activation genes) was persistently downregulated in non-survivors (Figures 1C, 2); however, this pathway was downregulated in survivors only at D1. Time analysis showed that only in survivors was there upregulation over time of this pathway, as well as T-cell signaling pathways (“Co-stimulation by the CD28 family”, “Generation of second messenger molecules”) and adaptive immunity gene sets (“IL2 STAT5 signaling”, “Allograft rejection”) (Figure 3A), consistent with resolution of adaptive immune suppression in survivors. A closer examination of canonical T-cell signaling genes supported this observation: genes such as CD3D/E/G, CD247, ZAP70, LCK, LAT and ITK were all persistently downregulated in non-survivors, but were initially downregulated and increased back to normal levels in survivors by D7 (Figure 3B). CD3D was also a shared differentially methylated gene observed in sepsis (39) and COVID-19 (40, 41), suggesting potential epigenetic regulation related to T-cell dysfunction (Figure S3C). Lastly, using NetworkAnalyst (48), a functional protein-protein interaction network was created using DE genes over time, revealing that only survivors had a cluster of upregulated T-cell signaling genes (Figure 3C). Combined, these findings highlighted sustained adaptive immune dysfunction as a key aspect in patients who eventually died, and restoration of such adaptive deficits was only observed in survivors.
Various confounders that could affect these adaptive immunity findings were investigated. Corticosteroid use, which can affect leukocyte function, was unlikely to affect this result, since survivors and non-survivors did not have significant differences in the rate of corticosteroid use (Table 1). Differences in leukocyte populations were observed between survivors and non-survivors (Table 1), so differential expression analysis was performed after correcting for cellular composition estimated by CIBERSORTx, a cell deconvolution method based on gene expression data (49). Fold changes for genes before and after correction were highly significantly correlated (Figure S8A), suggesting that gene expression variation was not substantially driven by differences in cell proportions. Indeed, key T cell signaling genes were still persistently suppressed in non-survivors and resolved over time only in survivors after correction (Figure S8B).
When individually analyzing COVID-19 and non-COVID-19 sepsis patients, various adaptive immune pathways, including “Generation of second messenger molecules”, “Costimulation by the CD28 family”, and “Immunoregulatory interactions”, were still upregulated over time in survivors, but not non-survivors, of both groups, while “IL-1 signaling” was downregulated over time in survivors of both groups (Figure S9). This emphasized a common adaptive immune recovery and inflammation resolution mechanism associated with ICU survival. Interestingly, only COVID-19 survivors had downregulation of antiviral pathways over time (Figure S9), suggesting that resolution of elevated antiviral responses was a unique part of survival trajectories in viral sepsis from COVID-19.
While the numbers of survivors and non-survivors were somewhat unbalanced (reflecting expected mortality rates of sepsis in the ICU), performing a subset analysis matching equal numbers of survivors and non-survivors (by age, sex, COVID-19 status, and sequencing batch) resulted in similar results (Figure S10), with substantially more persistent genes in non-survivors (4,366 vs. 831). Again, persistent upregulation of “IL-1 signaling” and “IL-4/13 signaling” and persistent downregulation of “Immunoregulatory interactions” was seen in non-survivors only, suggesting that imbalance was not substantially affecting the results.
3.3 Non-survivors had persistent enrichment of the mortality signature and were “locked-in” to more severe sepsis endotypes
A recently published a 38-gene mortality signature derived from early (emergency room) sepsis patients (9); was then assessed in these ICU patients. Gene set variation analysis (GSVA), an unsupervised gene set enrichment calculation methodology (50), showed that enrichment scores of this signature were significantly and persistently higher in non-survivors at both D1 and D7 compared to survivors (Figure 4A). Moreover, there was greater overlap of the mortality signature with persistent genes of non-survivors (15/38) than those of survivors (3/38) (Figure 4B). This again indicated that persistent cellular dysfunction in non-survivors was associated with mortality in both sepsis and COVID-19.
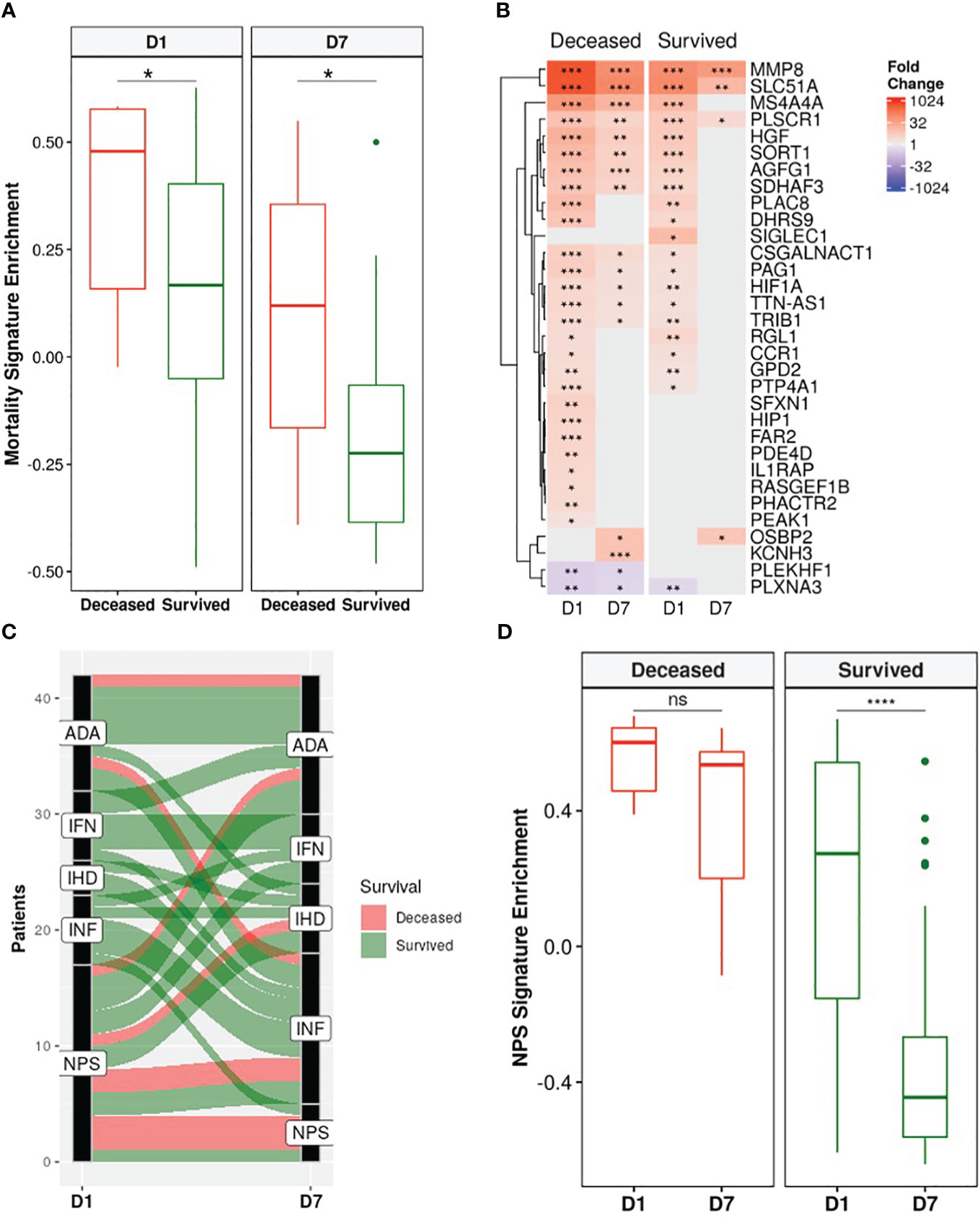
Figure 4 Non-survivors had persistent enrichment of the mortality signature and were “locked-in” to more severe sepsis endotypes. (A) The gene set variation analysis (GSVA) enrichment score of our published 38-gene mortality signature (9) was significantly higher in eventually deceased patients compared to survivors at both D1 and D7 (Wilcoxon rank-sum test, * = p<0.05). (B) Fold changes of mortality signature genes in eventually deceased patients and survivors relative to healthy controls. Shading in the heatmap represents fold change. Only DE genes are shown. Non-survivors had 15 persistently dysregulated (differentially expressed at D1 and D7) mortality signature genes compared to only 3 in survivors. (C) Eventually deceased patients were “locked in” to severe endotypes. Five endotypes were derived from differentially expressed genes in a cohort of emergency room sepsis patients: Neutrophilic-Suppressive (NPS), Inflammatory (INF), Interferon (IFN), Adaptive (ADA), and Innate Host Defense (IHD) (9). Each patient was classified into an endotype based on which of the five endotypes had the highest GSVA enrichment score. An alluvial graph demonstrating transition of each patient’s endotype between D1 and D7 is shown. (D) NPS GSVA enrichment scores were persistently high in eventually deceased patients but significantly decreased in survivors (pair-wise Wilcoxon rank-sum test, * = p<0.05; ** = p<0.01, *** = p<0.001, **** = p<0.0001, ns = not significant).
We next investigated the utility of five endotypes previously identified in emergency room sepsis patients (9) and validated in COVID-19 patients (10). Two of the five endotypes were associated with worse outcomes: Neutrophilic-Suppressive (NPS) and Inflammatory (INF). Consistent with this, the majority (7/9) of eventually deceased patients started as NPS, and most continued as NPS or transitioned into the other severe outcome endotype INF, with 6/9 deceased patients fitting into either the NPS or INF endotypes at D7 (Figure 4C). Furthermore, NPS signature enrichment scores remained persistently elevated over time in non-survivors, but in survivors significantly decreased over time (Figure 4D), suggesting that patients who eventually died might be “locked-in” to the more severe endotypes, and that these emergency room sepsis endotypes are still useful for stratifying patients at later timepoints in the ICU. Ultimately, the utility of these sepsis mortality signatures and endotypes in this group of ICU patients further confirmed shared mechanisms of mortality between sepsis and COVID-19.
3.4 Repurposed drugs were identified to target persistent genes and hub genes
We have shown that persistent gene expression changes, reflective of immune dysfunction, were highly associated with eventual mortality; however, survivors were able to resolve this dysfunction. Therefore, currently approved medications that can target the persistent genes in non-survivors to resolve this dysfunction might lead to successful and rapid repurposing of drugs for sepsis and COVID-19 therapy. To identify drugs that resolve persistent dysfunction, two systems-biology approaches were utilized, rather than focusing on targeting single genes.
The first approach was to use the Drug Signatures Database (DSigDB) (29), which is a repository of FDA-approved medications and their interactions (e.g., antagonism) with genes/proteins. Using this approach considers the overall systems-level cellular effect of a drug rather than its effect on just one target. These drug-gene sets were enriched using upregulated persistent genes only in non-survivors to identify possible inhibitors for these processes (Table 2). There was significant enrichment of numerous anti-inflammatory drugs including aspirin, sulfasalazine, and numerous corticosteroids including dexamethasone, likely targeting persistently upregulated inflammatory pathways. Surprisingly, other enriched drugs included antipsychotics (thioridazine and pimozide), the anti-arrhythmic agent flecainide, and various anti-infectives (mefloquine, ribavirin).
The second systems-biology approach was based on network analysis of persistent genes only found in non-survivors (Figure 5). Hub genes/proteins, which are genes in a network with multiple connections to other genes/proteins, are attractive druggable targets since they are expected to drive biology by regulating and/or interacting with multiple dysregulated genes/proteins. The top 15 most interconnected upregulated hub genes were SIRT7, GRB2, ARRB2, IKBKG, NEDD8, PPP1CA, HLA-B, NEDD4, STAT3, NFKB1, PXN, JUN, ATXN1, ACTB, and NOTCH1 (Figure 5). Approved pharmaceutical drugs that target these hub genes were obtained from genecards.org, which sources information from a variety of databases including DrugBank, PharmGKB, DGIdb, and Novoseek (Table 3). The drug that covered the most hub genes was dexamethasone (6/15 hub genes), followed by the immunomodulator cyclosporine and proteasome inhibitor bortezomib (4/15 hub genes), and a variety of drugs with immunomodulatory function were also identified (e.g., tacrolimus, thalidomide, sulfasalazine, and infliximab). Interestingly, ICAM1, which was the only persistent gene seen in both GWAS of sepsis and COVID-19 (Table S2), has known protein-protein interactions with the two hub genes NFKB1 and STAT3 and was also part of drug-gene sets of aspirin, thioridazine, pimozide, mefloquine, dexamethasone, and sulfasalazine (Table 2), suggesting these repurposed drugs may also modulate ICAM1. Several of the top persistently downregulated hub genes were ribosomal proteins (RPS6, RPS8, RPL5) indicating that ribosomal dysfunction might be another area to target therapeutically (Figure 5). Other persistently downregulated hub genes included CAND1, ITGA4, PAN2, ILF3, EEF1A1, SIRT1, FYN, IL7R, SMAD3, and NCL; finding drugs that can activate these hub genes/proteins may also be useful therapeutically.
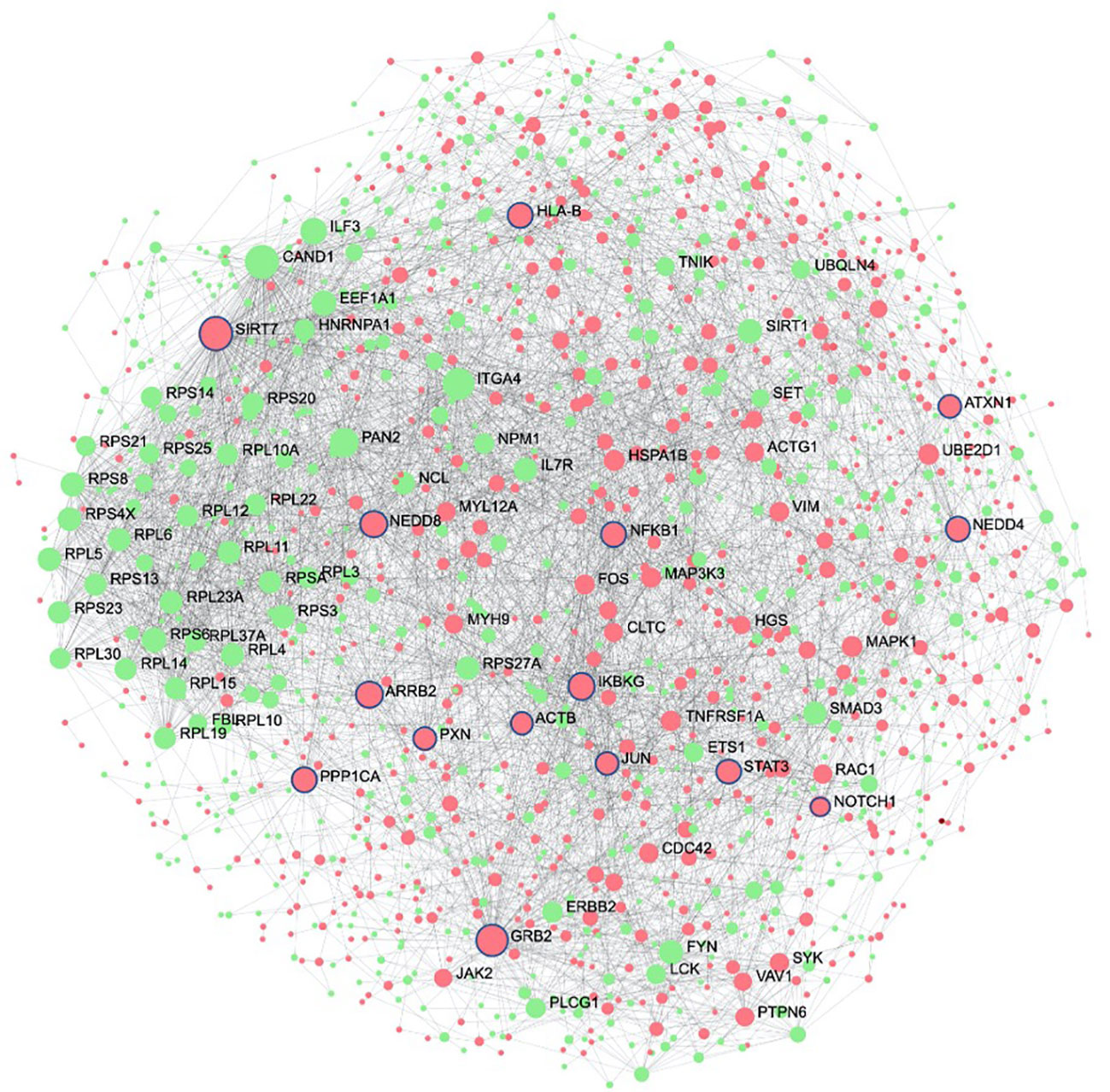
Figure 5 Protein interaction network of persistent genes in deceased patients highlight potential hub genes for drug targeting. Zero-order (i.e., only dysregulated nodes) functional protein-protein interactions (PPI) networks were drawn using NetworkAnalyst. Dots represent nodes (genes and their protein products) and are coloured red for upregulated and green for downregulated. Lines joining the dots represent known PPI from InnateDB. Hub genes, which are genes with multiple interactions, are displayed as the largest nodes (size related to hub degree) and are labelled. Hub genes are attractive targets for drugs as they are expected to regulate or interact with multiple other dysregulated genes and proteins during severe disease. Drugs with known interactions with the top 15 upregulated hubs (circled in blue) are listed in Table 3.
4 Discussion
By analyzing COVID-19 and non-COVID-19 sepsis patients longitudinally, we showed that, based on gene expression data, persistent immune dysfunction occurred in patients who eventually died, while partial immune resolution occurred in survivors, regardless of COVID-19 status. This persistent immune dysfunction involved both inflammatory and immunosuppressive components (Figures 1C, 2, 3A), as observed in both COVID-19 and non-COVID-19 sepsis non-survivors (Figure S2C, D) and in external datasets of COVID-19 and sepsis patients (Figure S4). While differences in the early antiviral response existed between these two groups (Figures S2B, S9), consistent with our previous work (11), overall, the underlying persistent immune dysfunction involved in mortality was highly conserved in both COVID-19 and non-COVID-19 sepsis (Figure S2D). The connection between persistence and mortality was further supported by persistent enrichment of our published mortality signature (Figure 4A) and the results from the endotype analysis, where most non-survivors remained associated with the high severity endotypes NPS and INF throughout disease (Figure 4C).
Persistently dysregulated inflammatory processes included inflammatory processes involving IL-1, IL-6, TNFα, and complement that failed to resolve in patients who died (Figures 1, 2). Interestingly, IL-4 and IL-13 signaling was also persistently upregulated in non-survivors (Figures 1, 2); this could be reflective of a transition towards Type-2 immunity, which could occur during increased pathogen burden (51) and is associated with poor outcomes in sepsis (52). In addition, this shift could be reflective of cellular reprogramming (CR): the process by which innate immune cells such as monocytes or macrophages lose their ability to respond appropriately to pathogens (53, 54), which could be highly detrimental during infection. CR macrophages have certain properties aligned with the Type-2 immunity associated M2 macrophage phenotype, and we previously showed that a CR gene signature predicted severe sepsis and organ failure (55). Adaptive immune deficits centered around persistent T-cell dysfunction in non-survivors, but reversal/correction of this dysfunction occurred in survivors (Figure 3). Overall, this persistence was consistent with the general concept of PICS, but this syndrome has only been described to date by using blood cytokine/protein markers and changes in specific cell populations (56). This is the first gene expression study showing that persistent gene expression analogous to PICS occurred similarly in both sepsis and severe COVID-19 ICU patients with worse outcomes.
Currently, it is unclear what enables some patients to correct their immune dysfunction, although this could involve individual factors such as natural immunity, predisposing conditions, or underlying patient genetics. Additionally, the idea of persistence is consistent with epigenetic mechanisms, and multiple immune genes were found both in persistent genes and differentially methylated genes in sepsis and COVID-19 (Figure S3). However, since non-survivors had persistently dysregulated pathways that resolved in survivors, this indicated that there may be treatable traits and pharmacological methods that could reverse persistence and decrease mortality. Currently-approved and in-trial immunomodulatory therapies for COVID-19 include corticosteroids such as dexamethasone (21), IL-6 signaling inhibitors such as tocilizumab (57) and baricitinib (23), the recombinant IL-1 receptor antagonist anakinra (58), and the complement inhibitor vilobelimab (59); these all target enriched pathways/gene sets that were observed to be persistently dysregulated in non-survivors (“Inflammatory response”, “IL-6 JAK STAT3 signaling”, “IL-1 signaling”, and “Complement”) (Figures 1, 2). Thus, targeting persistent genes and mechanisms appears to be a valid approach to find additional therapies. The identification of dexamethasone, a known effective treatment for COVID-19 (and for subsets of septic shock (60)), using both drug-gene set enrichment (Table 2) and network hub genes (Table 3), supported the validity of these methods for identifying potential repurposed drugs for sepsis and COVID-19. Other potential immunomodulators that could address the persistent inflammation included aspirin, sulfasalazine, and cyclosporine. There were also other drugs identified without evident immunomodulator functions, including the anti-psychotic thioridazine and the anti-arrhythmic flecainide, which have demonstrated survival benefits in mouse and rat sepsis models, respectively, likely through off-target inhibition of the NF-κB pathway (61, 62). These identified drugs should be further assessed in vitro, in vivo, and in clinical trials for potential efficacy.
While these identified drugs primarily dampen inflammatory mechanisms, it is also important to address the adaptive immune deficits observed in this cohort, since only focusing on anti-inflammatory therapies has not proven to be successful in sepsis (13). Thus, treatments that aim to restore adaptive suppression, focusing on T-cell functions/numbers should also be considered. They could be potentially concurrently administered with anti-inflammatories, or selectively applied to patients that require more immune stimulation rather than anti-inflammation based on underlying gene expression profiles or clinical variables. Therapies that addresses adaptive immune suppression could include checkpoint inhibitors (63) and IL-7 (64), which are currently being evaluated for sepsis and perhaps should also be considered for severe COVID-19. For example, IL7R was one of the top downregulated hub genes (Figure 5), further supporting potential use of IL-7. Monitoring immune function longitudinally throughout hospitalization (e.g., using gene-expression panels, cytokine measurements) could identify patients that fail to resolve immune dysfunction within the first week of ICU hospitalization, which could inform healthcare providers to consider additional care or enrollment into immunomodulatory clinical trials. As shown in this cohort, the time at which a patient is on their disease timeline (in this case, D1 or D7 in the ICU) needs to be considered when evaluating therapies.
There are some limitations to this study. First, these results are from a single cohort of mostly male patients and, while the major finding of persistence has been confirmed by re-analysis of other studies (30, 42) (Figure S4), they should be validated in larger, sex-balanced studies. Despite the modest sample size, thousands of DE genes were still identified, suggesting that the study was adequately powered for finding gene expression differences. Critically, these samples were paired with two timepoints, enabling patient indexing, which can help to eliminate various sources of patient heterogeneity and potential confounders that could potentially overshadow true DE changes. It is also possible that bulk RNA-Seq results could be altered by different cell proportions. However, even after adjusting with cell proportion data, similar patterns of differential expression were observed (Figure S8). Multiple other potential confounders were also addressed, including corticosteroid use, COVID-19 status (Figures S2, S9), and sample imbalance (Figure S10), all of which showed no substantial effects on the gene expression patterns observed.
In summary, mortality in both sepsis and COVID-19 was highly associated with persistent immune dysfunction during the first week in the ICU, with both inflammatory and immunosuppressive components. To improve outcomes, these patients require novel immunomodulatory treatments to treat immune dysfunction throughout the ICU stay, with multiple immunomodulatory drug candidates identified for further in vitro and in vivo testing.
Data availability statement
The datasets presented in this study can be found on the Gene Expression Omnibus (https://www.ncbi.nlm.nih.gov/geo/). The accession numbers are: GSE185263 and GSE222393.
Ethics statement
The studies involving humans were approved by Research Ethics Boards of St. Michael’s Hospital (“COLOBILI”, REB#20-078) and the University of British Columbia (“COVID-19 Sepsis Study”, REB#H20-02441). The studies were conducted in accordance with the local legislation and institutional requirements. The participants provided their written informed consent to participate in this study.
Author contributions
AA: Formal Analysis, Investigation, Validation, Visualization, Writing – original draft, Writing – review & editing. AB: Formal Analysis, Validation, Writing – review & editing. PZ: Formal Analysis, Writing – review & editing. RF: Methodology, Writing – review & editing. AL: Formal Analysis, Funding acquisition, Validation, Writing – review & editing. UT: Conceptualization, Writing – review & editing. AJB: Conceptualization, Funding acquisition, Resources, Writing – review & editing. CS: Conceptualization, Funding acquisition, Resources, Writing – review & editing, Project administration, Supervision. RH: Conceptualization, Formal Analysis, Funding acquisition, Investigation, Project administration, Resources, Supervision, Writing – original draft, Writing – review & editing.
Funding
Funding from Canadian Institutes for Health Research (CIHR) FDN-154287 to RH and CIHR COVID-19 Rapid Research Funding to RH and AL is gratefully acknowledged. RH holds a UBC Killam Professorship and held a Canada Research Chair. AA is funded by a Canada Graduate Scholarships Doctoral (CGS-D) program. The COVID-19 Longitudinal Biomarkers of Lung Injury study (COLOBILI) study under which these samples were collected was funded by the St. Michael’s Foundation, an Immune Task Force Grant, and a CIHR grant (GA4-177735) to CS and AJB. CS is supported by the CIHR (MOP-130331, MOP-106545, CIHR/NSERC MOP-510282 2020) and the University of Toronto Robert and Dorothy Pitts Research Chair in Acute Care and Emergency Medicine.
Acknowledgments
We thank the research coordinators (Marlene Santos, Gyan Sadhu, Alizay Javed, and Imrana Khalid) who collected all the patient samples. We thank the patients and care givers who generously donated samples for the purpose of research.
Conflict of interest
RH has a significant ownership position in Sepset Biotherapeutics Inc and RH and AJB have filed patents for sepsis diagnostic gene expression assays an indirect relationship to this work. CS is on the Data and Safety Monitoring Board of SEMPATICO NCT04615871.
The remaining authors declare that the research was conducted in the absence of any commercial or financial relationships that could be construed as a potential conflict of interest.
Publisher’s note
All claims expressed in this article are solely those of the authors and do not necessarily represent those of their affiliated organizations, or those of the publisher, the editors and the reviewers. Any product that may be evaluated in this article, or claim that may be made by its manufacturer, is not guaranteed or endorsed by the publisher.
Supplementary material
The Supplementary Material for this article can be found online at: https://www.frontiersin.org/articles/10.3389/fimmu.2023.1254873/full#supplementary-material
References
1. Coronavirus Statistics. Worldometer. Available at: https://www.worldometers.info/coronavirus/.
2. Wang H, Paulson KR, Pease SA, Watson S, Comfort H, Zheng P, et al. Estimating excess mortality due to the COVID-19 pandemic: a systematic analysis of COVID-19-related mortality, 2020–21. Lancet (2022) 399:1513–36. doi: 10.1016/S0140-6736(21)02796-3
3. de Roquetaillade C, Bredin S, Lascarrou J-B, Soumagne T, Cojocaru M, Chousterman BG, et al. Timing and causes of death in severe COVID-19 patients. Crit Care (2021) 25:224. doi: 10.1186/s13054-021-03639-w
4. Singer M, Deutschman CS, Seymour CW, Shankar-Hari M, Annane D, Bauer M, et al. The third international consensus definitions for sepsis and septic shock (Sepsis-3). JAMA (2016) 315:801–10. doi: 10.1001/jama.2016.0287
5. Rudd KE, Johnson SC, Agesa KM, Shackelford KA, Tsoi D, Kievlan DR, et al. Global, regional, and national sepsis incidence and mortality, 1990-2017: analysis for the Global Burden of Disease Study. Lancet (2020) 395:200–11. doi: 10.1016/S0140-6736(19)32989-7
6. Bauer M, Gerlach H, Vogelmann T, Preissing F, Stiefel J, Adam D. Mortality in sepsis and septic shock in Europe, North America and Australia between 2009 and 2019— results from a systematic review and meta-analysis. Crit Care (2020) 24:239. doi: 10.1186/s13054-020-02950-2
7. Rice B, Calo S, Kamugisha JB, Kamara N, Chamberlain S, Group, on behalf of G. E. C. I. S. Emergency care of sepsis in sub-Saharan Africa: mortality and non-physician clinician management of sepsis in rural Uganda from 2010 to 2019. PloS One (2022) 17:e0264517. doi: 10.1371/journal.pone.0264517
8. Vincent J-L. COVID-19: it is all about sepsis. Future Microbiol (2021) 16:131–3. doi: 10.2217/fmb-2020-0312
9. Baghela A, Pena OM, Lee AH, Baquir B, Falsafi R, An A, et al. Predicting sepsis severity at first clinical presentation: the role of endotypes and mechanistic signatures. eBioMedicine (2022) 75:103776. doi: 10.1016/j.ebiom.2021.103776
10. Baghela A, An A, Zhang P, Acton E, Gauthier J, Brunet-Ratnasingham E, et al. Predicting severity in COVID-19 disease using sepsis blood gene expression signatures. Sci Rep (2023) 13:1247. doi: 10.1038/s41598-023-28259-y
11. An AY, Baghela AS, Falsafi R, Lee AH, Trahtemberg U, Baker AJ, et al. Severe COVID-19 and non-COVID-19 severe sepsis converge transcriptionally after a week in the intensive care unit, indicating common disease mechanisms. Front Immunol (2023) 6:1167917. doi: 10.3389/fimmu.2023.1167917
12. Polat G, Ugan RA, Cadirci E, Halici Z. Sepsis and septic shock: current treatment strategies and new approaches. Eurasian J Med (2017) 49:53–8. doi: 10.5152/eurasianjmed.2017.17062
13. Cavaillon J, Singer M, Skirecki T. Sepsis therapies: learning from 30 years of failure of translational research to propose new leads. EMBO Mol Med (2020) 12:e10128. doi: 10.15252/emmm.201810128
14. Hotchkiss RS, Monneret G, Payen D. Sepsis-induced immunosuppression: from cellular dysfunctions to immunotherapy. Nat Rev Immunol (2013) 13:862–74. doi: 10.1038/nri3552
15. Mira JC, Brakenridge SC, Moldawer LL, Moore FA. Persistent inflammation, immunosuppression and catabolism syndrome (PICS). Crit Care Clin (2017) 33:245–58. doi: 10.1016/j.ccc.2016.12.001
16. Khedar RS, Gupta R, Sharma K, Mittal K, Ambaliya HC, Gupta JB, et al. Biomarkers and outcomes in hospitalised patients with COVID-19: a prospective registry. BMJ Open (2022) 12:e067430. doi: 10.1136/bmjopen-2022-067430
17. Malik P, Patel U, Mehta D, Patel N, Kelkar R, Akrmah M, et al. Biomarkers and outcomes of COVID-19 hospitalisations: systematic review and meta-analysis. BMJ Evidence-Based Med (2021) 26:107–8. doi: 10.1136/bmjebm-2020-111536
18. Semiz S. COVID19 biomarkers: What did we learn from systematic reviews? Front Cell Infect Microbiol (2022) 12:1038908. doi: 10.3389/fcimb.2022.1038908
19. Tonello S, Rizzi M, Matino E, Costanzo M, Casciaro GF, Croce A, et al. Baseline plasma gas6 protein elevation predicts adverse outcomes in hospitalized COVID-19 patients. Dis Markers (2022) 2022:e1568352. doi: 10.1155/2022/1568352
20. Pierrakos C, Vincent J-L. Sepsis biomarkers: a review. Crit Care (2010) 14:R15. doi: 10.1186/cc8872
21. The RECOVERY Collaborative Group. Dexamethasone in hospitalized patients with covid-19. N Engl J Med (2021) 384:693–704. doi: 10.1056/NEJMoa2021436
22. Gupta S, Leaf DE. Tocilizumab in COVID-19: some clarity amid controversy. Lancet (2021) 397:1599–601. doi: 10.1016/S0140-6736(21)00712-1
23. Supady A, Zeiser R. Baricitinib for patients with severe COVID-19—time to change the standard of care? Lancet Respir Med (2022) 10:314–5. doi: 10.1016/S2213-2600(22)00021-2
24. Bernardes JP, Mishra N, Tran F, Bahmer T, Best L, Blase JI, et al. Longitudinal multi-omics analyses identify responses of megakaryocytes, erythroid cells, and plasmablasts as hallmarks of severe COVID-19. Immunity (2020) 53:1296–1314.e9. doi: 10.1016/j.immuni.2020.11.017
25. Love MI, Huber W, Anders S. Moderated estimation of fold change and dispersion for RNA-seq data with DESeq2. Genome Biol (2014) 15:550. doi: 10.1186/s13059-014-0550-8
26. Fabregat A, Sidiropoulos K, Viteri G, Forner O, Marin-Garcia P, Arnau V, et al. Reactome pathway analysis: a high-performance in-memory approach. BMC Bioinform (2017) 18:142. doi: 10.1186/s12859-017-1559-2
27. Liberzon A, Birger C, Thorvaldsdóttir H, Ghandi M, Mesirov JP, Tamayo P. The Molecular Signatures Database (MSigDB) hallmark gene set collection. Cell Syst (2015) 1:417–25. doi: 10.1016/j.cels.2015.12.004
28. Yu G, Wang L-G, He Q-Y. clusterProfiler: an R package for comparing biological themes among gene clusters. OMICS (2012) 16:284–7. doi: 10.1089/omi.2011.0118
29. Yoo M, Shin J, Kim J, Ryall KA, Lee K, Lee S, et al. DSigDB: drug signatures database for gene set analysis. Bioinformatics (2015) 31:3069–71. doi: 10.1093/bioinformatics/btv313
30. Karakike E, Scicluna BP, Roumpoutsou M, Mitrou I, Karampela N, Karageorgos A, et al. Effect of intravenous clarithromycin in patients with sepsis, respiratory and multiple organ dysfunction syndrome: a randomized clinical trial. Crit Care (2022) 26:183. doi: 10.1186/s13054-022-04055-4
31. Rosier F, Brisebarre A, Dupuis C, Baaklini S, Puthier D, Brun C, et al. Genetic predisposition to the mortality in septic shock patients: from GWAS to the identification of a regulatory variant modulating the activity of a CISH enhancer. Int J Mol Sci (2021) 22:5852. doi: 10.3390/ijms22115852
32. Engoren M, Jewell ES, Douville N, Moser S, Maile MD, Bauer ME. Genetic variants associated with sepsis. PloS One (2022) 17:e0265052. doi: 10.1371/journal.pone.0265052
33. Kousathanas A, Pairo-Castineira E, Rawlik K, Stuckey A, Odhams CA, Walker S, et al. Whole genome sequencing reveals host factors underlying critical COVID-19. Nature (2022) 607:1–10. doi: 10.1038/s41586-022-04576-6
34. Pairo-Castineira E, Rawlik K, Bretherick AD, Qi T, Wu Y, Nassiri I, et al. GWAS and meta-analysis identifies 49 genetic variants underlying critical COVID-19. Nature (2023) 617:764–8. doi: 10.1038/s41586-023-06034-3
35. Bui TM, Wiesolek HL, Sumagin R. ICAM-1: A master regulator of cellular responses in inflammation, injury resolution, and tumorigenesis. J Leukoc Biol (2020) 108:787–99. doi: 10.1002/JLB.2MR0220-549R
36. Sessler CN, Windsor AC, Schwartz M, Watson L, Fisher BJ, Sugerman HJ, et al. Circulating ICAM-1 is increased in septic shock. Am J Respir Crit Care Med (1995) 151:1420–7. doi: 10.1164/ajrccm.151.5.7735595
37. Kaur S, Hussain S, Kolhe K, Kumar G, Tripathi DM, Tomar A, et al. Elevated plasma ICAM1 levels predict 28-day mortality in cirrhotic patients with COVID-19 or bacterial sepsis. JHEP Rep (2021) 3:100303. doi: 10.1016/j.jhepr.2021.100303
38. Bruni F, Charitos P, Lampart M, Moser S, Siegemund M, Bingisser R, et al. Complement and endothelial cell activation in COVID-19 patients compared to controls with suspected SARS-CoV-2 infection: A prospective cohort study. Front Immunol (2022) 13:941742. doi: 10.3389/fimmu.2022.941742
39. Binnie A, Walsh CJ, Hu P, Dwivedi DJ, Fox-Robichaud A, Liaw PC, et al. Epigenetic profiling in severe sepsis: a pilot study of DNA methylation profiles in critical illness. Crit Care Med (2020) 48:142–50. doi: 10.1097/CCM.0000000000004097
40. Castro de Moura M, Davalos V, Planas-Serra L, Alvarez-Errico D, Arribas C, Ruiz M, et al. Epigenome-wide association study of COVID-19 severity with respiratory failure. EBioMedicine (2021) 66:103339. doi: 10.1016/j.ebiom.2021.103339
41. Balnis J, Madrid A, Hogan KJ, Drake LA, Chieng HC, Tiwari A, et al. Blood DNA methylation and COVID-19 outcomes. Clin Epigenet (2021) 13:118. doi: 10.1186/s13148-021-01102-9
42. Liu C, Martins AJ, Lau WW, Rachmaninoff N, Chen J, Imberti L, et al. Time-resolved systems immunology reveals a late juncture linked to fatal COVID-19. Cell (2021) 184:1836–1857.e22. doi: 10.1016/j.cell.2021.02.018
43. Hofmaenner DA, Kleyman A, Press A, Bauer M, Singer M. The many roles of cholesterol in sepsis: a review. Am J Respir Crit Care Med (2022) 205:388–96. doi: 10.1164/rccm.202105-1197TR
44. Van Wyngene L, Vandewalle J, Libert C. Reprogramming of basic metabolic pathways in microbial sepsis: therapeutic targets at last? EMBO Mol Med (2018) 10:e8712. doi: 10.15252/emmm.201708712
45. Berg S, Brodin B, Hesselvik F, Laurent TC, Maller R. Elevated levels of plasma hyaluronan in septicaemia. Scand J Clin Lab (1988) 48:727–32. doi: 10.3109/00365518809088752
46. Jensen IJ, Sjaastad FV, Griffith TS, Badovinac VP. Sepsis induced T cell immunoparalysis: the ins and outs of impaired T cell immunity. J Immunol (2018) 200:1543–53. doi: 10.4049/jimmunol.1701618
47. Zhou Y, Liao X, Song X, He M, Xiao F, Jin X, et al. Severe adaptive immune suppression may be why patients with severe COVID-19 cannot be discharged from the ICU even after negative viral tests. Front Immunol (2021) 12:755579. doi: 10.3389/fimmu.2021.755579
48. Xia J, Gill EE, Hancock REW. NetworkAnalyst for statistical, visual and network-based meta-analysis of gene expression data. Nat Protoc (2015) 10:823–44. doi: 10.1038/nprot.2015.052
49. Newman AM, Steen CB, Liu CL, Gentles AJ, Chaudhuri AA, Scherer F, et al. Determining cell type abundance and expression from bulk tissues with digital cytometry. Nat Biotechnol (2019) 37:773–82. doi: 10.1038/s41587-019-0114-2
50. Hänzelmann S, Castelo R, Guinney J. GSVA: gene set variation analysis for microarray and RNA-Seq data. BMC Bioinform (2013) 14:7. doi: 10.1186/1471-2105-14-7
51. Spellberg B, Edwards JE Jr. Type 1/Type 2 immunity in infectious diseases. Clin Infect Dis (2001) 32:76–102. doi: 10.1086/317537
52. Xue M, Xie J, Liu L, Huang Y, Guo F, Xu J, et al. Early and dynamic alterations of Th2/Th1 in previously immunocompetent patients with community-acquired severe sepsis: a prospective observational study. J Transl Med (2019) 17:57. doi: 10.1186/s12967-019-1811-9
53. Cavaillon J-M, Adib-Conquy M. Bench-to-bedside review: endotoxin tolerance as a model of leukocyte reprogramming in sepsis. Crit Care (2006) 10:233. doi: 10.1186/cc5055
54. Vergadi E, Vaporidi K, Tsatsanis C. Regulation of endotoxin tolerance and compensatory anti-inflammatory response syndrome by non-coding RNAs. Front Immunol (2018) 9. doi: 10.3389/fimmu.2018.02705
55. Pena OM, Hancock DG, Lyle NH, Linder A, Russell JA, Xia J, et al. An endotoxin tolerance signature predicts sepsis and organ dysfunction at initial clinical presentation. eBioMedicine (2014) 1:64–71. doi: 10.1016/j.ebiom.2014.10.003
56. Mira JC, Gentile LF, Mathias BJ, Efron PA, Brakenridge SC, Mohr AM, et al. Sepsis pathophysiology, chronic critical illness, and persistent inflammation-immunosuppression and catabolism syndrome. Crit Care Med (2017) 45:253–62. doi: 10.1097/CCM.0000000000002074
57. Mikulska M, Nicolini LA, Signori A, Di Biagio A, Sepulcri C, Russo C, et al. Tocilizumab and steroid treatment in patients with COVID-19 pneumonia. PloS One (2020) 15:e0237831. doi: 10.1371/journal.pone.0237831
58. Kyriazopoulou E, Poulakou G, Milionis H, Metallidis S, Adamis G, Tsiakos K, et al. Early treatment of COVID-19 with anakinra guided by soluble urokinase plasminogen receptor plasma levels: a double-blind, randomized controlled phase 3 trial. Nat Med (2021) 27:1752–60. doi: 10.1038/s41591-021-01499-z
59. Vlaar APJ, Witzenrath M, van Paassen P, Heunks LMA, Mourvillier B, de,Bruin S, et al. Anti-C5a antibody (vilobelimab) therapy for critically ill, invasively mechanically ventilated patients with COVID-19 (PANAMO): a multicentre, double-blind, randomised, placebo-controlled, phase 3 trial. Lancet Respir Med (2022) 10:1137–46. doi: 10.1016/S2213-2600(22)00297-1
60. Annane D, Bellissant E, Bollaert P-E, Briegel J, Confalonieri M, De Gaudio R, et al. Corticosteroids in the treatment of severe sepsis and septic shock in adults: a systematic review. JAMA (2009) 301:2362–75. doi: 10.1001/jama.2009.815
61. Baig MS, Roy A, Saqib U, Rajpoot S, Srivastava M, Naim A, et al. Repurposing Thioridazine (TDZ) as an anti-inflammatory agent. Sci Rep (2018) 8:12471. doi: 10.1038/s41598-018-30763-5
62. Chung SY, Kim J, Bae HB, Tin TD, Ju W, Kwak SH. The effects of flecainide acetate on inflammatory-immune response in lipopolysaccharide-stimulated neutrophils and on mortality in septic rats. Acute Crit Care (2018) 33:34–41. doi: 10.4266/acc.2017.00577
63. Hotchkiss RS, Colston E, Yende S, Angus DC, Moldawer LL, Crouser ED, et al. Immune checkpoint inhibition in sepsis: a phase 1b randomized, placebo-controlled, single ascending dose study of antiprogrammed cell death-ligand 1 antibody (BMS-936559). Crit Care Med (2019) 47:632–42. doi: 10.1097/CCM.0000000000003685
Keywords: COVID-19, sepsis, immune dysfunction, gene expression, drug repurposing
Citation: An AY, Baghela A, Zhang P, Falsafi R, Lee AH, Trahtemberg U, Baker AJ, Santos CC and Hancock REW (2023) Persistence is key: unresolved immune dysfunction is lethal in both COVID-19 and non-COVID-19 sepsis. Front. Immunol. 14:1254873. doi: 10.3389/fimmu.2023.1254873
Received: 07 July 2023; Accepted: 04 September 2023;
Published: 26 September 2023.
Edited by:
Eizo Watanabe, Aichi Medical University, JapanCopyright © 2023 An, Baghela, Zhang, Falsafi, Lee, Trahtemberg, Baker, Santos and Hancock. This is an open-access article distributed under the terms of the Creative Commons Attribution License (CC BY). The use, distribution or reproduction in other forums is permitted, provided the original author(s) and the copyright owner(s) are credited and that the original publication in this journal is cited, in accordance with accepted academic practice. No use, distribution or reproduction is permitted which does not comply with these terms.
*Correspondence: Robert E. W. Hancock, Ym9iQGhhbmNvY2tsYWIuY29t
†These authors share senior authorship