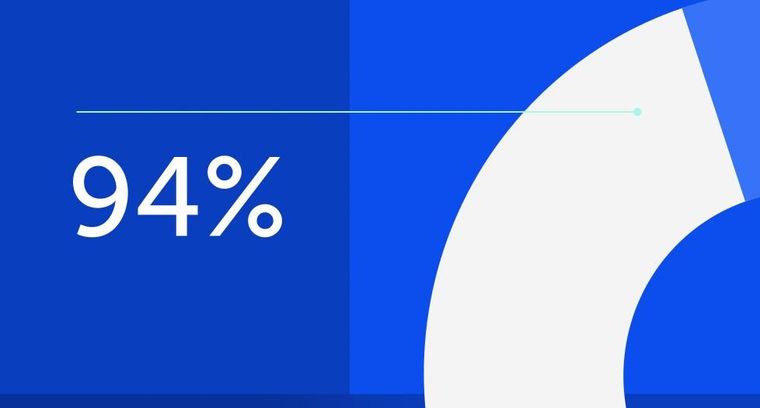
94% of researchers rate our articles as excellent or good
Learn more about the work of our research integrity team to safeguard the quality of each article we publish.
Find out more
REVIEW article
Front. Immunol., 20 September 2023
Sec. Cancer Immunity and Immunotherapy
Volume 14 - 2023 | https://doi.org/10.3389/fimmu.2023.1251645
This article is part of the Research TopicRadiomics and AI for clinical and translational medicineView all 7 articles
Immune checkpoint inhibitors (ICIs) modulate the body’s immune function to treat tumors but may also induce pneumonitis. Immune checkpoint inhibitor-related pneumonitis (ICIP) is a serious immune-related adverse event (irAE). Immunotherapy is currently approved as a first-line treatment for non-small cell lung cancer (NSCLC), and the incidence of ICIP in NSCLC patients can be as high as 5%-19% in clinical practice. ICIP can be severe enough to lead to the death of NSCLC patients, but there is a lack of a gold standard for the diagnosis of ICIP. Radiomics is a method that uses computational techniques to analyze medical images (e.g., CT, MRI, PET) and extract important features from them, which can be used to solve classification and regression problems in the clinic. Radiomics has been applied to predict and identify ICIP in NSCLC patients in the hope of transforming clinical qualitative problems into quantitative ones, thus improving the diagnosis and treatment of ICIP. In this review, we summarize the pathogenesis of ICIP and the process of radiomics feature extraction, review the clinical application of radiomics in ICIP of NSCLC patients, and discuss its future application prospects.
Currently, lung cancer remains the leading cause of cancer-related deaths, with approximately over 20% of cancer patients dying from lung cancer (1, 2). Non-small cell lung cancer (NSCLC) accounts for 85% of all lung cancer cases (3), and it primarily includes adenocarcinoma and squamous cell carcinoma (4). Approximately 70% of NSCLC patients are diagnosed at an advanced stage (5), and for advanced NSCLC, a comprehensive treatment approach is typically employed, including radiation therapy, chemotherapy, targeted therapy, and immunotherapy (6). Immunotherapy has emerged as a novel treatment method and has gained significant attention due to its ability to improve the overall survival and survival rates of patients with NSCLC (7–9). Immunotherapy has made significant progress in advanced NSCLC patients, with an unselected 5-year overall survival rate (OS) of 20%, and a 5-year overall survival rate of up to 40% in patients with high PD-L1 expression (10, 11). However, while immunotherapy enhances patient survival, it can also give rise to a series of immune-related adverse events (irAEs) that involve the skin, respiratory system, digestive system, and cardiovascular system (12–14). Immune checkpoint inhibitor-related pneumonitis (ICIP) is the most common adverse respiratory reaction caused by immunotherapy (15), and it is also the primary cause of death resulting from immune-related adverse events (16).
ICIP refers to the development of newly observed pulmonary inflammatory infiltrates on medical imaging after the initiation of immunotherapy, excluding other causes of pneumonitis during the progression of cancer. A retrospective analysis showed that the overall incidence of pneumonitis in cancer patients receiving treatment with anti-programmed cell death-1/programmed cell death ligand-1 (anti-PD-1/PD-L1) monoclonal antibodies or in combination with anti-cytotoxic T-cell lymphocyte-4 monoclonal antibody was 5% (3%-6%). The incidence of pneumonitis was higher with combination immunotherapy compared to monotherapy (17). Another systematic review and meta-analysis of studies on melanoma, NSCLC, and renal cell carcinoma (RCC) showed that ICIP is common in NSCLC,renal cell carcinoma, and during combination therapy (18). In clinical trials, the incidence of ICIP in NSCLC is generally 3% to 5% (19, 20), but in the real clinical application environment, the incidence of ICIP in NSCLC can range from 5% to 19% (15, 21–23).
Immune-related adverse events (irAEs), including ICIP, can have a certain impact on the treatment plan, prognosis, and treatment cost of patients with NSCLC. During the progression of NSCLC, in addition to pneumonitis caused by immune therapy, infections (24), radiotherapy (25), chemotherapy (26), and targeted therapy (27) can also induce pneumonitis. Currently, there is no established gold standard for diagnosing ICIP, and clinicians usually make subjective inferences based on patient symptoms and imaging findings (28). Diagnostic methods such as imaging characteristics, clinical risk factors, bronchoalveolar lavage, and cell culture lack specificity for diagnosing ICIP. The concept of radiomics was first proposed by Professor Philippe Lambin in 2012 (29), was later elaborated upon by Kumar et al. (30). It refers to the high-throughput extraction and analysis of advanced imaging features from medical images such as CT, PET, or MRI. Radiomics utilizes high-throughput data extraction techniques to identify features in non-invasive imaging data and establish predictive models, solving classification and regression challenges in clinical practice (31). By using radiomics models, relevant features can be screened from medical imaging data, transforming qualitative clinical problems into quantitative ones, thus providing a more objective method for solving clinical problems (32). In recent years, radiomics has been used for the diagnosis, pathological classification, clinical staging, efficacy assessment, and prognosis evaluation of NSCLC (33–36). With the development of precision medicine, radiomics can further be used for predicting, diagnosing, differentiating and prognostic analysis ICIP in NSCLC patients. This can enable close monitoring and guide the diagnosis and treatment of ICIP in NSCLC patient. This paper reviews the pathogenesis of ICIP and the process of radiomics feature extraction. It aims to conduct a comprehensive analysis of the clinical studies applying radiomics to NSCLC patients with ICIP. In addition, this review discusses the limitations of these studies and proposes potential approaches to address them.
Currently, immune checkpoint inhibitors (ICIs) used for NSCLC mainly include Nivolumab, Pembrolizumab, Cemiplimab, Atezolizumab, Avelumab, Durvalumab, and Ipilimumab (37, 38). In addition to monotherapy, ICIs can also be used in combination with chemotherapy drugs, targeted drugs, and anti-angiogenic drugs (39). The overall response rate of immune therapy in NSCLC is approximately 20-30% (40). A phase 1b multicenter trial showed that the combination of Durvalumab and Osimertinib in the treatment of EGFR mutation-positive NSCLC patients can increase the incidence of interstitial lung disease (ILD)-related adverse events (AE) (35%) (41). ICIs have been a hot topic in cancer treatment research in recent years, primarily targeting programmed cell death-1 (PD-1) or programmed cell death ligand-1 (PD-L1) and cytotoxic T-lymphocyte-associated antigen-4 (CTLA-4) in the body’s immune process (42). PD1/PD-L1 primarily suppresses immune responses by inhibiting intracellular signal transduction in effector T cells and regulatory T cells, mainly by reducing the activity of phosphoinositide 3-kinase (PI3K) (43, 44). Cytotoxic T-lymphocyte-associated antigen-4 (CTLA-4) and CD28 have opposing immune regulatory functions, with CTLA4 binding more easily to B7 than CD28, thus preventing CD28 from binding to B7 and inhibiting CD28-mediated T cell proliferation process (45, 46). The mechanism of ICIP is still not fully understood,but ICIs can induce immune dysregulation while targeting tumor cells. Immune dysregulation mainly involves T-cell imbalance, increased production of autoantibodies, and dysregulation of inflammatory cytokines (47). Following anti-PDL1/PD1 immune therapy, infiltration of Th1 cells [most of which express PD-1 (48)] can be observed in corresponding tissues (49, 50). Immune therapy limits the conversion of Th1 cells into Treg cells (51), and a decrease in Treg cells may lead to immune dysfunction. Anti-PD-1 therapy can enhance the anti-tumor function of Th17 cells (52, 53). ICIP may also be associated with autoantibodies, as studies have shown an increase in pre-existing and newly developed autoantibodies following immune checkpoint inhibitor treatment (54, 55). Inflammatory markers such as C-reactive protein (CRP) and IL-6 can induce excessive activation of the immune system (56). The serum levels of IL-17 are increased in patients with NSCLC who develop ICIP after immunotherapy (57). The potential risk factors for ICIP include patient baseline characteristics, disease features, and treatment management (58). These risk factors have a high correlation with ICIP and can be combined with radiomics features to build multimodal models (59, 60).
ICIP essentially refers to an interstitial inflammation. The radiological manifestations of ICIP are similar to those of conventional pneumonia, including ground-glass opacities, consolidations, fibrous bands, thickening of interlobular septa, traction bronchiectasis, small nodular opacities, and reticular opacities (61–63). ICIP can be classified based on its radiological manifestations, including cryptogenic organizing pneumonia (COP), non-specific interstitial pneumonia (NSIP), acute interstitial pneumonia/acute respiratory distress syndrome (AIP/ARDS), and hypersensitivity pneumonitis (HP) (64). ICIP does not exhibit specific features in chest medical imaging pictures (61), and even radiologists with decades of experience cannot visually differentiate ICIP with the naked eye. The heterogeneity of tumor cells at the cellular level is closely related to the radiological features present in medical imaging data (65). Radiomics employs computer algorithms to extract high-throughput information from medical imaging data and transform qualitative data into quantitative data (32). Radiomics is divided into conventional radiomics and deep learning-based radiomics.
Conventional radiomics requires manual extraction and selection of radiomics features. Conventional radiomics features include size, shape, and texture features, with texture features further divided into first-order, second-order, and higher-order texture features (66, 67). Extracting too many radiomics features can lead to model overfitting, hence the need to screen hundreds or thousands of features (68). Common conventional radiomics feature selection methods include the Least Absolute Shrinkage and Selection Operator (LASSO) (69), Principal Component Analysis (PCA) (70), and Minimum Redundancy Maximum Relevance (MRMR) (71). Conventional radiomics methods may overlook higher-level features when extracting radiomics features.
Research has shown that deep learning features are typically more representative and robust than manually designed traditional radiomics features (72). Deep learning radiomics, using architectures like CNNs and Transformers, employ forward propagation for computations and data transformations, yielding predictions. The network’s training hinges on backpropagation, which calculates gradients based on prediction error. These gradients iteratively refine the network’s parameters. This dynamic between forward and backpropagation enables automatic extraction of radiomics features, revealing advanced radiomics traits.
CNNs are the most classical deep neural network architecture. GoogLeNet/Inception, ResNet, VGGNet, and DenseNet are commonly used CNN models in lung cancer radiomics (73). VGGNet (74) are known for their simplicity, using only 3x3 convolutions to build deep networks, which improved accuracy. GoogLeNet/Inception (75) deepens network architectures and uniquely extracts and merges multi-scale image features using its Inception modules, optimizing computational efficiency without compromising performance. However, both VGGNet and GoogLeNet/Inception encounter a common challenge: the potential for vanishing or exploding gradients as the CNN architecture deepens. This can lead to suboptimal weight updates in earlier layers, consequently diminishing the model’s performance. ResNet (76) employs Batch Normalization and skip connections to address the vanishing or exploding gradient problem. This approach effectively mitigates the performance degradation associated with internal covariate shift, which arises from scale variations in input data and layer computations. DenseNet (77), building upon ResNet’s advantages, introduces dense connectivity for enhanced feature reuse and gradient flow. This achieves parameter efficiency and superior performance. It employs a ‘growth rate’ for layer size and transition layers for feature-map management. However, dense connections elevate computational demands and memory usage. Issues like feature redundancy and increased complexity can arise. Kundu et al. developed an integrated model for the early diagnosis of pneumonia using GoogLeNet, ResNet-18, and DenseNet-121 (78). The model was evaluated using chest X-ray images from the Kermany dataset and the RSNA dataset, achieving accuracies of 98.81% and 86.86%, respectively.
The Transformer, originally designed for Natural Language Processing (NLP) tasks, has been adapted for computer vision, capturing intricate contextual relationships within images. In some applications, especially medical image representation, Transformer-based models have demonstrated competitive, if not superior, performance compared to traditional CNNs (79–82). Swin Transformer is a novel Transformer model proposed by Ze Liu et al. in 2021. This model is a hierarchical vision transformer using shifted windows, which enables the application of Transformers from the language domain to the visual domain (83). Ma, X et al. (84) retrospectively collected CT data from 612 patients with lung adenocarcinoma, which were divided into a training set (n=189), an internal validation set (n=123), and an external test set (n=108). In this study, deep learning model based on Swin Transformer, conventional radiomics model, and clinical semantic model were employed to predict lymph node metastasis. The results showed that the deep learning model based on Swin Transformer outperformed both clinical semantic model and conventional radiomics model in all three datasets.
Radiomics models can predict whether NSCLC will develop ICIP before immunotherapy, diagnose and differentiate patients with pneumonitis caused by immunotherapy, and predict the survival prognosis of NSCLC patients with ICIP.
ICIP affects the survival rate and outcomes of patients with NSCLC. Early prediction of whether immunotherapy can cause pneumonitis in NSCLC patients and the selection of the most suitable candidates for immunotherapy can improve the survival outcomes of NSCLC patients. In 2016, Rivka R. Colen et al. (85) first used a radiomics model to predict ICIP (immunotherapy-induced pneumonitis). This study predicted the risk of ICIP in 32 cancer patients. Traditional radiomic methods were used to construct the predictive model, and CT images were segmented using 3Dslicer. A total of 1860 radiomic texture features based on histogram and Gray Level Co-occurrence Matrix (GLCM) were extracted. The MRMR method was applied to select the most relevant features for predicting ICIP. The method achieved a perfect accuracy of 100% (p=0.0033). However, due to the small number of cases in this study, the results may not be representative. Subsequently, Tan Peixin et al. (59) used a multimodal deep learning model to predict the risk of ICIP in 48 lung cancer patients (Including 47 cases of NSCLC), with an equal ratio of ICIP to non-ICIP patients. This study, for the first time, constructed a multimodal deep learning model for ICIP prediction based on nine clinical features and radiomic features. The deep learning model (3D ResNet18) employed in this study consists of 18 convolutional layers and corresponding fully connected layers. In contrast to conventional ResNet models, this model is specifically designed for processing three-dimensional data. The research employed two-stage transfer learning and contrastive learning strategies to enhance the performance of the prediction model. Ultimately, the model achieved an area under the curve (AUC) of 0.918 through five-fold cross-validation. M. Cheng et al. (86) also developed a multi-modal nomogram model, based on clinical and deep radiomics features, to predict the risk of ICIP in 141 lung cancer patients (Including 128 cases of NSCLC). The model’s predictions for ICIP were presented using a nomogram. To enhance the model’s performance and accuracy, they optimized the ResNet-50-V2 model using the Feature Pyramid Network (FPN). The study employed a random allocation method to divide patients into a training group (n=113) and a testing group (n=28). The results demonstrated that, both in the training group (0.910 vs. 0.871 vs. 0.778) and the testing group (0.900 vs. 0.856 vs. 0.869), the performance of the multi-modal nomogram model was superior to that of both radiomics and clinical models.
The toxic side effects of immunotherapy and radiotherapy overlap. The combination of immunotherapy and radiotherapy increases the incidence of pneumonitis (87). A KEYNOTE-001 study demonstrated that the risk of developing pneumonitis was higher with the combined use of immunotherapy and radiotherapy (63%) compared to using immunotherapy alone (40%) (88). Benjamin Spieler et al. (60) conducted an animal experiment in which Lewis lung carcinoma (LLC) cells were injected subcutaneously into 19 genetically identical (C57BL/6) mice. Among them, 15 mice received a combined treatment of radiation and immunotherapy, while the remaining 4 mice served as a control group. The mice in the treatment group were classified into two categories based on the median value of CD45 infiltration in the lungs. The experiment integrated pre-treatment radiomics features, NLR, and GM-CSF to construct a multimodal prediction model. CT and MRI imaging techniques were used, and 92 CT features and 92 MRI features were extracted. These features included geometric features, first-order histogram features, second-order joint probability features (such as co-occurrence matrix), and third-order joint probability features. The third-order joint probability features were developed by the researchers themselves. The experiment employed double cross-validation and demonstrated that the predictive performance of the model constructed based on MRI (AUC=0.834) was superior to that of the model constructed based on CT imaging (AUC=0.787). Linlin yang et al. (72) conducted a retrospective study and developed a Deep Graph Integrative Model (DG) to predict the risk of pneumonitis in NSCLC patients undergoing combined immunotherapy and radiotherapy. They employed two pre-trained CNN models (3D UNet) to extract features separately from tumor and lung volumes in the CT images. The extracted features were then selected and fused using the Graph Attention Layer (GAT) to construct the DG model. Using a five-fold cross-validation approach, the training set consisted of 195 cases (50 symptomatic pneumonitis), and the validation set consisted of 48 cases (12 symptomatic pneumonitis). The study results demonstrated that the DG model (AUC=0.823) outperformed conventional CT radiomics model (AUC=0.743), 3DUNet-based deep learning model (AUC=0.761), and DG models without GAT (AUC=0.796) (Table 1 reports the cases mentioned in this paragraph).
In patients with NSCLC receiving immunotherapy, pneumonitis can be caused by factors other than immunotherapy. To determine whether Summary of some radiomic studies for predicting cancer treatment-related pneumonitis. is attributable to immunotherapy, some studies have used radiomics for the diagnosis of ICIP. The D. De Ruysscher team presented two studies on distinguishing ICIP and other causes of pneumonitis (OP) at the European Society for Medical Oncology (ESMO) in 2021 (90, 91). One study enrolled a total of 450 stage IV non-small cell lung cancer patients for immunotherapy treatment. CT imaging was performed six weeks after immunotherapy, and a total of 837 radiomics features were extracted from the images. Recursive Feature Elimination (RFE) was applied for feature selection, followed by a coherence analysis. Ultimately, 42 radiomics features with the highest correlation were selected. A logistic regression model was constructed using these features, achieving an AUC of 0.91 (95% confidence interval: 0.75 to 0.98). In another study, 556 stage IV NSCLC patients were recruited from six centers, with one center used for external validation. Clinical and radiomic features were used to construct clinical (AUC=0.99), radiomic (AUC=0.65), and combined models (AUC=0.99). The important predictive factors in the combined model are number of comorbidities, type of anti-PD(L)1 medication, dyspnea, and Gray Level Co-occurrence Matrix Inverse Difference Moment Normalized (GLCM IDMN) . In 2023, D. De Ruysscher et al. (92) published a retrospective study aimed at differentiating between ICIP and OP using clinical and radiomics features. They delineated seven different Regions of Interest (ROI) using either deep learning-based automatic segmentation or manual segmentation methods, and found that the radiomics model performed best when based on a 75mm spherical ROI. The first-order, Gray Level Co-occurrence Matrix (GLCM), Gray Level Run Length Matrix (GLRLM), Gray Level Size Zone Matrix (GLSZM), and Gray Level Dependence Matrix (GLDM) features were extracted from the CT images. An unsupervised approach, LASSO regression with 5-fold cross-validation, and stepwise backward logistic regression were employed to select radiomic features and construct the radiomics model, with model parameters being fitted using the original data. Through bootstrap validation, the performance of the radiomics model (AUC=0.83) was significantly superior to that of the clinical model (AUC=0.66).
Among treatment-related pneumonitis of NSCLC, the incidence of radiation therapy pneumonitis and ICIP is higher than that of pneumonitis caused by other treatments. Clinicians often face challenges in distinguishing between pneumonitis caused by radiation therapy and immunotherapy, making it difficult for them to determine the next course of treatment for NSCLC patients. There have been reports of using radiomics models to directly differentiate between radiation-induced pneumonitis (RIP) and ICIP in NSCLC patients (93, 94). Chen, X. G. et al. (93) retrospectively collected CT data from 82 NSCLC patients with pneumonitis (n = 23 after immunotherapy, n = 29 after radiotherapy, and n = 30 after combined immunotherapy and radiotherapy). The data from the immunotherapy and radiotherapy groups were used to train a random forest model, while the data from the combined immunotherapy and radiotherapy group were used for model testing. Seven radiomics features were selected using LASSO to construct the model, and hyperparameters were optimized using grid search. It was ultimately found that a machine learning model built solely on radiomics features could differentiate pneumonitis caused by ICI and RT in the training set (AUC = 0.76). The study also found that ICIP was more likely to occur bilaterally compared to RIP. Jun Cheng et al. (94) extracted three types of texture features from CT images, namely intensity histogram, gray level co-occurrence matrix (GLCM) based, and bag-of-words features. They constructed discrimination models using each of these three features. The radiomics model was built using datasets from patients receiving either immunotherapy or radiotherapy, and the dataset of combined immunotherapy and radiotherapy was employed for model testing. The discrimination model based on bag-of-words features achieved an AUC of 0.937 through 10-fold cross-validation. Qingtao Qiu et al. (95) conducted a retrospective study on 126 stage IV NSCLC patients with pneumonitis. They extracted 837 features from CT images and performed feature selection using LASSO. Eleven selected features were used to construct a nomogram model, which achieved an AUC of 0.901 in the validation set (Table 2 reports the cases mentioned in this paragraph).
With the development of precision medicine, clinicians are increasingly focusing on personalized treatment for cancer patients.Currently, there is a large body of research on using radiomics models to predict the prognosis of immunotherapy in patients with NSCLC. Some studies incorporate clinical features on top of radiomics characteristics to enhance the accuracy of the prediction models (36, 96–102). Michael R. Sayer et al. (103) studied the impact of immune-related adverse events, relative occurrence time, and previous tyrosine kinase inhibitor (TKI) treatment on the performance of prognostic models for NSCLC patients treated with ICIs. The study found that Immune-related adverse events (irAEs) are important predictive factor for the survival prognosis of NSCLC patients. Therefore, in addition to predicting the prognosis of immunotherapy in patients with NSCLC, radiomics models can also be further utilized to predict the prognosis of NSCLC patients with ICIP.
In order to achieve the optimal clinical benefits, patients with NSCLC are typically treated with a comprehensive treatment approach. However, radiotherapy (25), chemotherapy (26), targeted therapy (27, 104–106), and immunotherapy can all potentially lead to pneumonitis, which is known as treatment-related pneumonitis (107, 108). Patients who have received thoracic radiotherapy may experience radiation recall pneumonitis (RRP) after receiving anticancer drugs such as chemotherapy medications (109). Pneumonitis caused by taxanes (110) and platinum drugs is relatively rare. The overall incidence of targeted therapy-related pneumonitis is also low (1.2%) (106). The incidence of RRP induced by immunotherapy is 18.8% (with a median time of 368.5 days from the end of radiotherapy to the start of immunotherapy) (111). Radiomics models can predict and identify the treatment approaches that lead to pneumonitis in NSCLC patients, enabling early modification of the treatment plan to avoid or mitigate pneumonitis symptoms. Models constructed based on radiomics features and dose measurement variables can be used for predicting RIP in NSCLC patients. Studies have shown that a predictive model combining radiomics and dose measurement variables outperforms the use of radiomics alone (89, 112) (Table 1). Currently, dose measurement variables are considered important predictive factors for radiation-induced pneumonitis in NSCLC (113).
Currently, the radiomics models for NSCLC patients with ICIP primarily focus on diagnostic and differential diagnostic models. The predictive and prognostic analysis models for ICIP lack strong generalizability due to the complexity of cancer types used during model training. To improve model performance, some studies have incorporated highly relevant clinical features in addition to radiomics data. The accuracy of radiomics prediction remains insufficient, as immunotherapy is an emerging treatment approach with a limited number of NSCLC patients receiving immunotherapy, which is inadequate to support the development of a stable and high-performance radiomics model by computer algorithms. There is no gold standard for the diagnosis of ICIP, and clinical doctors primarily rely on patients’ clinical symptoms, pathogen examinations, and imaging manifestations for diagnosis. In the process of acquiring radiomics features, there is a lack of standardized protocols for slice thickness, imaging standards, and delineation of ROI in radiographic images, resulting in low reproducibility. To enhance the reproducibility of radiomics models, it is possible to construct an open-source radiomics data platform to compensate for the insufficient amount of data. The Cancer Imaging Archive (TCIA) is a large-scale cancer medical imaging archive hosting platform that allows for the download of open-source imaging data. The software tools ITK-SNAP (114), 3D Slicer (115), etc., can be used to delineate the ROI in radiological images. In order to establish standardized delineation criteria, software packages can be utilized for semi-automatic or fully automatic segmentation of the target area (116). Efficient and unified standards should be developed for each step of radiomics analysis. By doing so, the diagnostic and treatment process for NSCLC patients with ICIP will become more targeted and aligned with the principles of precision medicine.
YS, WX and RS wrote the main manuscript content and revised it. PR prepared the tables for the manuscript. ZZ, LL and JZ assisted in editing the manuscript. ZC and GF designed the study, revised it, and gave final approval of the manuscript. All authors contributed to the article and approved the submitted version.
Taishan Scholar Foundation of Shandong Province, Grant/Award Number: tsqn202103179; National Natural Science Foundation of China, Grant/Award Number: 81802284; Science and Technology Development Plans of Shandong Province, Grant/Award Number: 2014GSF118157; Scientific Research Foundation of Shandong Province of Outstanding Young Scientists, Grant/Award Number: BS2013YY058; 2021 Shandong Medical Association Clinical Research Fund, Grant/Award Number: YXH2022ZX02176; Natural Science Foundation of Shandong Province, Grant/Award Number: ZR2022MH088.
The authors declare that the research was conducted in the absence of any commercial or financial relationships that could be construed as a potential conflict of interest.
All claims expressed in this article are solely those of the authors and do not necessarily represent those of their affiliated organizations, or those of the publisher, the editors and the reviewers. Any product that may be evaluated in this article, or claim that may be made by its manufacturer, is not guaranteed or endorsed by the publisher.
1. Siddiqui F, Vaqar S, Siddiqui AH. Lung Cancer. Treasure Island (FL): StatPearls Publishing (2022) 2022.
2. Siegel RL, Miller KD, Wagle NS, Jemal A. Cancer statistics, 2023. CA-Cancer J Clin (2023) 73(1):17–48. doi: 10.3322/caac.21763
3. Azzouqa AG, Chen RQ, Lou YY, Ailawadhi S, Manochakian R. Impact of time to treatment initiation (Tti) on survival of patients with newly diagnosed non-small cell lung cancer (Nsclc). J Clin Oncol (2019) 37(15):9058. doi: 10.1200/JCO.2019.37.15_suppl.9058
4. Chen JW, Dhahbi J. Lung adenocarcinoma and lung squamous cell carcinoma cancer classification, biomarker identification, and gene expression analysis using overlapping feature selection methods. Sci Rep (2021) 11(1):13323. doi: 10.1038/s41598-021-92725-8
5. Zhou Y, Ma Y, Shi H, Du Y, Huang Y. Epidermal growth factor receptor T790m mutations in non-small cell lung cancer (Nsclc) of Yunnan in Southwestern China. Sci Rep (2018) 8(1):15426. doi: 10.1038/s41598-018-33816-x
6. Ettinger DS, Wood DE, Aisner DL, Akerley W, Bauman JR, Bharat A, et al. Non-small cell lung cancer, version 3.2022, nccn clinical practice guidelines in oncology. J Natl Compr Cancer Network: JNCCN (2022) 20(5):497–530. doi: 10.6004/jnccn.2022.0025
7. Doroshow DB, Sanmamed MF, Hastings K, Politi K, Rimm DL, Chen L, et al. Immunotherapy in non-small cell lung cancer: facts and hopes. Clin Cancer Res (2019) 25(15):4592–602. doi: 10.1158/1078-0432.Ccr-18-1538
8. Herbst RS, Giaccone G, de Marinis F, Reinmuth N, Vergnenegre A, Barrios CH, et al. Atezolizumab for first-line treatment of pd-L1-selected patients with nsclc. New Engl J Med (2020) 383(14):1328–39. doi: 10.1056/NEJMoa1917346
9. Brahmer JR, Lee JS, Ciuleanu TE, Bernabe Caro R, Nishio M, Urban L, et al. Five-year survival outcomes with nivolumab plus ipilimumab versus chemotherapy as first-line treatment for metastatic non-small-cell lung cancer in checkmate 227. J Clin Oncol (2023) 41(6):1200–12. doi: 10.1200/jco.22.01503
10. Horvath L, Thienpont B, Zhao L, Wolf D, Pircher A. Overcoming immunotherapy resistance in non-small cell lung cancer (Nsclc) - novel approaches and future outlook. Mol Cancer (2020) 19(1):141. doi: 10.1186/s12943-020-01260-z
11. Garon EB, Hellmann MD, Rizvi NA, Carcereny E, Leighl NB, Ahn MJ, et al. Five-year overall survival for patients with advanced non−Small-cell lung cancer treated with pembrolizumab: results from the phase I keynote-001 study. J Clin Oncol (2019) 37(28):2518–27. doi: 10.1200/jco.19.00934
12. Conroy M, Naidoo J. Immune-related adverse events and the balancing act of immunotherapy. Nat Commun (2022) 13(1):392. doi: 10.1038/s41467-022-27960-2
13. Kumar V, Chaudhary N, Garg M, Floudas CS, Soni P, Chandra AB. Current diagnosis and management of immune related adverse events (Iraes) induced by immune checkpoint inhibitor therapy. Front Pharmacol (2017) 8:49. doi: 10.3389/fphar.2017.00049
14. Shankar B, Zhang J, Naqash AR, Forde PM, Feliciano JL, Marrone KA, et al. Multisystem immune-related adverse events associated with immune checkpoint inhibitors for treatment of non-small cell lung cancer. JAMA Oncol (2020) 6(12):1952–6. doi: 10.1001/jamaoncol.2020.5012
15. Gomatou G, Tzilas V, Kotteas E, Syrigos K, Bouros D. Immune checkpoint inhibitor-related pneumonitis. Respiration; Int Rev Thorac Dis (2020) 99(11):932–42. doi: 10.1159/000509941
16. Sun X, Roudi R, Dai T, Chen S, Fan B, Li H, et al. Immune-related adverse events associated with programmed cell death protein-1 and programmed cell death ligand 1 inhibitors for non-small cell lung cancer: A prisma systematic review and meta-analysis. BMC Cancer (2019) 19(1):558. doi: 10.1186/s12885-019-5701-6
17. Naidoo J, Wang X, Woo KM, Iyriboz T, Halpenny D, Cunningham J, et al. Pneumonitis in patients treated with anti-programmed death-1/programmed death ligand 1 therapy. J Clin Oncol (2017) 35(7):709–17. doi: 10.1200/jco.2016.68.2005
18. Nishino M, Giobbie-Hurder A, Hatabu H, Ramaiya NH, Hodi FS. Incidence of programmed cell death 1 inhibitor-related pneumonitis in patients with advanced cancer: A systematic review and meta-analysis. JAMA Oncol (2016) 2(12):1607–16. doi: 10.1001/jamaoncol.2016.2453
19. Rizvi NA, Mazières J, Planchard D, Stinchcombe TE, Dy GK, Antonia SJ, et al. Activity and safety of nivolumab, an anti-pd-1 immune checkpoint inhibitor, for patients with advanced, refractory squamous non-small-cell lung cancer (Checkmate 063): A phase 2, single-arm trial. Lancet Oncol (2015) 16(3):257–65. doi: 10.1016/s1470-2045(15)70054-9
20. Suresh K, Naidoo J, Lin CT, Danoff S. Immune checkpoint immunotherapy for non-small cell lung cancer: benefits and pulmonary toxicities. Chest (2018) 154(6):1416–23. doi: 10.1016/j.chest.2018.08.1048
21. Atchley WT, Alvarez C, Saxena-Beem S, Schwartz TA, Ishizawar RC, Patel KP, et al. Immune checkpoint inhibitor-related pneumonitis in lung cancer: real-world incidence, risk factors, and management practices across six health care centers in North Carolina. Chest (2021) 160(2):731–42. doi: 10.1016/j.chest.2021.02.032
22. Cho JY, Kim J, Lee JS, Kim YJ, Kim SH, Lee YJ, et al. Characteristics, incidence, and risk factors of immune checkpoint inhibitor-related pneumonitis in patients with non-small cell lung cancer. Lung Cancer (Amsterdam Netherlands) (2018) 125:150–6. doi: 10.1016/j.lungcan.2018.09.015
23. Suresh K, Voong KR, Shankar B, Forde PM, Ettinger DS, Marrone KA, et al. Pneumonitis in non-small cell lung cancer patients receiving immune checkpoint immunotherapy: incidence and risk factors. J Thorac Oncol (2018) 13(12):1930–9. doi: 10.1016/j.jtho.2018.08.2035
24. Stoclin A, Rotolo F, Hicheri Y, Mons M, Chachaty E, Gachot B, et al. Ventilator-associated pneumonia and bloodstream infections in intensive care unit cancer patients: A retrospective 12-year study on 3388 prospectively monitored patients. Support Care Cancer (2020) 28(1):193–200. doi: 10.1007/s00520-019-04800-6
25. Menoux I, Antoni D, Mazzara C, Labani A, Charloux A, Quoix E, et al. Radiation-induced lung toxicity predictors: retrospective analysis of 90 patients treated with stereotactic body radiation therapy for stage I non-small-cell lung carcinoma. Cancer radiotherapie: J la Societe francaise radiotherapie oncologique (2020) 24(2):120–7. doi: 10.1016/j.canrad.2019.11.003
26. Neuville M, Borie R, Rodier JM, Debray MP, Danel C, Dombret MC, et al. [Pneumocystis pneumonia in a patient treated with pemetrexed for non small cell lung cancer]. Rev Des Maladies Respiratoires (2011) 28(1):97–100. doi: 10.1016/j.rmr.2010.06.029
27. Lim SM, An HJ, Park HS, Kwon HJ, YK E, Hur J, et al. Organizing pneumonia resembling disease progression in a non-small-cell lung cancer patient receiving ceritinib: A case report. Medicine (2018) 97(31):e11646. doi: 10.1097/md.0000000000011646
28. Haanen J, Obeid M, Spain L, Carbonnel F, Wang Y, Robert C, et al. Management of toxicities from immunotherapy: esmo clinical practice guideline for diagnosis, treatment and follow-up. Ann Oncol (2022) 33(12):1217–38. doi: 10.1016/j.annonc.2022.10.001
29. Lambin P, Rios-Velazquez E, Leijenaar R, Carvalho S, van Stiphout RG, Granton P, et al. Radiomics: extracting more information from medical images using advanced feature analysis. Eur J Cancer (Oxford England: 1990) (2012) 48(4):441–6. doi: 10.1016/j.ejca.2011.11.036
30. Kumar V, Gu Y, Basu S, Berglund A, Eschrich SA, Schabath MB, et al. Radiomics: the process and the challenges. Magnetic Resonance Imaging (2012) 30(9):1234–48. doi: 10.1016/j.mri.2012.06.010
31. Shur JD, Doran SJ, Kumar S, Ap Dafydd D, Downey K, O’Connor JPB, et al. Radiomics in oncology: A practical guide. Radiographics: Rev Publ Radiological Soc North America Inc (2021) 41(6):1717–32. doi: 10.1148/rg.2021210037
32. Rogers W, Thulasi Seetha S, Refaee TAG, Lieverse RIY, Granzier RWY, Ibrahim A, et al. Radiomics: from qualitative to quantitative imaging. Br J Radiol (2020) 93(1108):20190948. doi: 10.1259/bjr.20190948
33. Qin K, Fu X. [Research progress in imaging-based diagnosis of benign and Malignant Enlarged lymph nodes in non-small cell lung cancer]. Zhongguo fei ai za zhi = Chin J Lung Cancer (2023) 26(1):31–7. doi: 10.3779/j.issn.1009-3419.2023.101.01
34. Han Y, Ma Y, Wu Z, Zhang F, Zheng D, Liu X, et al. Histologic subtype classification of non-small cell lung cancer using pet/ct images. Eur J Nucl Med Mol Imaging (2021) 48(2):350–60. doi: 10.1007/s00259-020-04771-5
35. Xie Y, Zhao H, Guo Y, Meng F, Liu X, Zhang Y, et al. A pet/ct nomogram incorporating suvmax and ct radiomics for preoperative nodal staging in non-small cell lung cancer. Eur Radiol (2021) 31(8):6030–8. doi: 10.1007/s00330-020-07624-9
36. He BX, Zhong YF, Zhu YB, Deng JJ, Fang MJ, She YL, et al. Deep learning for predicting immunotherapeutic efficacy in advanced non-small cell lung cancer patients: A retrospective study combining progression-free survival risk and overall survival risk. Trans Lung Cancer Res (2022) 11(4):670–85. doi: 10.21037/tlcr-22-244
37. Tang SJ, Qin C, Hu HY, Liu T, He YW, Guo HY, et al. Immune checkpoint inhibitors in non-small cell lung cancer: progress, challenges, and prospects. CELLS (2022) 11(3):320. doi: 10.3390/cells11030320
38. Hellmann MD, Paz-Ares L, Bernabe Caro R, Zurawski B, Kim SW, Carcereny Costa E, et al. Nivolumab plus ipilimumab in advanced non-small-cell lung cancer. New Engl J Med (2019) 381(21):2020–31. doi: 10.1056/NEJMoa1910231
39. Chen Y, Chen Z, Chen R, Fang C, Zhang C, Ji M, et al. Immunotherapy-based combination strategies for treatment of egfr-tki-resistant non-small-cell lung cancer. Future Oncol (London England) (2022) 18(14):1757–75. doi: 10.2217/fon-2021-0862
40. Punekar SR, Shum E, Grello CM, Lau SC, Velcheti V. Immunotherapy in non-small cell lung cancer: past, present, and future directions. Front Oncol (2022) 12:877594. doi: 10.3389/fonc.2022.877594
41. Ahn MJ, Cho BC, Ou X, Walding A, Dymond AW, Ren S, et al. Osimertinib plus durvalumab in patients with egfr-mutated, advanced nsclc: A phase 1b, open-label, multicenter trial. J Thorac Oncol (2022) 17(5):718–23. doi: 10.1016/j.jtho.2022.01.012
42. Wei SC, Duffy CR, Allison JP. Fundamental mechanisms of immune checkpoint blockade therapy. Cancer Discov (2018) 8(9):1069–86. doi: 10.1158/2159-8290.Cd-18-0367
43. Oh SA, Wu DC, Cheung J, Navarro A, Xiong H, Cubas R, et al. Pd-L1 expression by dendritic cells is a key regulator of T-cell immunity in cancer. Nat Cancer (2020) 1(7):681–91. doi: 10.1038/s43018-020-0075-x
44. Francisco LM, Salinas VH, Brown KE, Vanguri VK, Freeman GJ, Kuchroo VK, et al. Pd-L1 regulates the development, maintenance, and function of induced regulatory T cells. J Exp Med (2009) 206(13):3015–29. doi: 10.1084/jem.20090847
45. Chikuma S. Ctla-4, an essential immune-checkpoint for T-cell activation. Curr Topics Microbiol Immunol (2017) 410:99–126. doi: 10.1007/82_2017_61
46. Lin H, Rathmell JC, Gray GS, Thompson CB, Leiden JM, Alegre ML. Cytotoxic T lymphocyte antigen 4 (Ctla4) blockade accelerates the acute rejection of cardiac allografts in cd28-deficient mice: ctla4 can function independently of cd28. J Exp Med (1998) 188(1):199–204. doi: 10.1084/jem.188.1.199
47. Yin J, Wu Y, Yang X, Gan L, Xue J. Checkpoint inhibitor pneumonitis induced by anti-pd-1/pd-L1 therapy in non-small-cell lung cancer: occurrence and mechanism. Front Immunol (2022) 13:830631. doi: 10.3389/fimmu.2022.830631
48. Kim ST, Sheshadri A, Shannon V, Kontoyiannis DP, Kantarjian H, Garcia-Manero G, et al. Distinct immunophenotypes of T cells in bronchoalveolar lavage fluid from leukemia patients with immune checkpoint inhibitors-related pulmonary complications. Front Immunol (2020) 11:590494. doi: 10.3389/fimmu.2020.590494
49. Dulos J, Carven GJ, van Boxtel SJ, Evers S, Driessen-Engels LJ, Hobo W, et al. Pd-1 blockade augments th1 and th17 and suppresses th2 responses in peripheral blood from patients with prostate and advanced melanoma cancer. J immunother (Hagerstown Md: 1997) (2012) 35(2):169–78. doi: 10.1097/CJI.0b013e318247a4e7
50. Yoshino K, Nakayama T, Ito A, Sato E, Kitano S. Severe colitis after pd-1 blockade with nivolumab in advanced melanoma patients: potential role of th1-dominant immune response in immune-related adverse events: two case reports. BMC Cancer (2019) 19(1):1019. doi: 10.1186/s12885-019-6138-7
51. Zhang L, Zhang M, Xu J, Li S, Chen Y, Wang W, et al. The role of the programmed cell death protein-1/programmed death-ligand 1 pathway, regulatory T cells and T helper 17 cells in tumor immunity: A narrative review. Ann Trans Med (2020) 8(22):1526. doi: 10.21037/atm-20-6719
52. Martin-Orozco N, Muranski P, Chung Y, Yang XO, Yamazaki T, Lu S, et al. T helper 17 cells promote cytotoxic T cell activation in tumor immunity. Immunity (2009) 31(5):787–98. doi: 10.1016/j.immuni.2009.09.014
53. Ankathatti Munegowda M, Deng Y, Mulligan SJ, Xiang J. Th17 and th17-stimulated cd8+ T cells play a distinct role in th17-induced preventive and therapeutic antitumor immunity. Cancer Immunol Immunother: CII (2011) 60(10):1473–84. doi: 10.1007/s00262-011-1054-y
54. Toi Y, Sugawara S, Sugisaka J, Ono H, Kawashima Y, Aiba T, et al. Profiling preexisting antibodies in patients treated with anti-pd-1 therapy for advanced non-small cell lung cancer. JAMA Oncol (2019) 5(3):376–83. doi: 10.1001/jamaoncol.2018.5860
55. de Souza Costa VH Jr., Baurakiades E, Viola Azevedo ML, Traiano G, Kowal Rosales J, Kunze Larsen KS, et al. Immunohistochemistry analysis of pulmonary infiltrates in necropsy samples of children with non-pandemic lethal respiratory infections (Rsv; adv; piv1; piv2; piv3; flu a; flu B). J Clin Virol (2014) 61(2):211–5. doi: 10.1016/j.jcv.2014.06.026
56. Pepys MB, Hirschfield GM. C-reactive protein: A critical update. J Clin Invest (2003) 111(12):1805–12. doi: 10.1172/jci18921
57. Luo W, Wang Z, Tian P, Li W. Safety and tolerability of pd-1/pd-L1 inhibitors in the treatment of non-small cell lung cancer: A meta-analysis of randomized controlled trials. J Cancer Res Clin Oncol (2018) 144(10):1851–9. doi: 10.1007/s00432-018-2707-4
58. Zhang Q, Tang L, Zhou Y, He W, Li W. Immune checkpoint inhibitor-associated pneumonitis in non-small cell lung cancer: current understanding in characteristics, diagnosis, and management. Front Immunol (2021) 12:663986. doi: 10.3389/fimmu.2021.663986
59. Tan P, Huang W, Wang L, Deng G, Yuan Y, Qiu S, et al. Deep learning predicts immune checkpoint inhibitor-related pneumonitis from pretreatment computed tomography images. Front Physiol (2022) 13:978222. doi: 10.3389/fphys.2022.978222
60. Spieler B, Giret TM, Welford S, Totiger TM, Mihaylov IB. Lung inflammation predictors in combined immune checkpoint-inhibitor and radiation therapy-proof-of-concept animal study. Biomedicines (2022) 10(5):1173. doi: 10.3390/biomedicines10051173
61. Kalisz KR, Ramaiya NH, Laukamp KR, Gupta A. Immune checkpoint inhibitor therapy-related pneumonitis: patterns and management. Radiographics: Rev Publ Radiological Soc North America Inc (2019) 39(7):1923–37. doi: 10.1148/rg.2019190036
62. Pang L, Xie M, Ma X, Huang A, Song J, Yao J, et al. Clinical characteristics and therapeutic effects of checkpoint inhibitor-related pneumonitis in patients with non-small cell lung cancer. BMC Cancer (2023) 23(1):203. doi: 10.1186/s12885-023-10649-0
63. Zhang Q, Tao X, Zhao S, Li N, Wang S, Wu N. Association of clinical and radiological features with disease severity of symptomatic immune checkpoint inhibitor-related pneumonitis. Diagn (Basel Switzerland) (2023) 13(4):691. doi: 10.3390/diagnostics13040691
64. Travis WD, Costabel U, Hansell DM, King TE Jr., Lynch DA, Nicholson AG, et al. An official american thoracic society/european respiratory society statement: update of the international multidisciplinary classification of the idiopathic interstitial pneumonias. Am J Respir Crit Care Med (2013) 188(6):733–48. doi: 10.1164/rccm.201308-1483ST
65. Choi ER, Lee HY, Jeong JY, Choi YL, Kim J, Bae J, et al. Quantitative image variables reflect the intratumoral pathologic heterogeneity of lung adenocarcinoma. Oncotarget (2016) 7(41):67302–13. doi: 10.18632/oncotarget.11693
66. Koçak B, Durmaz E, Ateş E, Kılıçkesmez Ö. Radiomics with artificial intelligence: A practical guide for beginners. Diagn Interventional Radiol (Ankara Turkey) (2019) 25(6):485–95. doi: 10.5152/dir.2019.19321
67. Chang R, Qi S, Zuo Y, Yue Y, Zhang X, Guan Y, et al. Predicting chemotherapy response in non-small-cell lung cancer via computed tomography radiomic features: peritumoral, intratumoral, or combined? Front Oncol (2022) 12:915835. doi: 10.3389/fonc.2022.915835
68. Hawkins DM. The problem of overfitting. J Chem Inf Comput Sci (2004) 44(1):1–12. doi: 10.1021/ci0342472
69. Huang Y, Liu Z, He L, Chen X, Pan D, Ma Z, et al. Radiomics signature: A potential biomarker for the prediction of disease-free survival in early-stage (I or ii) non-small cell lung cancer. Radiology (2016) 281(3):947–57. doi: 10.1148/radiol.2016152234
70. Zhang Y, Oikonomou A, Wong A, Haider MA, Khalvati F. Radiomics-based prognosis analysis for non-small cell lung cancer. Sci Rep (2017) 7:46349. doi: 10.1038/srep46349
71. Yang F, Zhang J, Zhou L, Xia W, Zhang R, Wei H, et al. Ct-based radiomics signatures can predict the tumor response of non-small cell lung cancer patients treated with first-line chemotherapy and targeted therapy. Eur Radiol (2022) 32(3):1538–47. doi: 10.1007/s00330-021-08277-y
72. Yang L, Cui H, Duan Y, Yao Y, Zou B, Wang L. Radiotherapy-immunotherapy related pneumonitis prediction from pre-treatment ct using a deep graph-based integrative model. J Clin Oncol (2022) 40(16):1. doi: 10.1200/JCO.2022.40.16_suppl.2580
73. Zhou Y, Xu X, Song L, Wang C, Guo J, Yi Z, et al. The application of artificial intelligence and radiomics in lung cancer. Precis Clin Med (2020) 3(3):214–27. doi: 10.1093/pcmedi/pbaa028
74. Simonyan K, Zisserman A. Very deep convolutional networks for large-scale image recognition. [arXiv preprint] arXiv:1409.1556. (2014). doi: 10.48550/arXiv.1409.1556
75. Szegedy C, Liu W, Jia Y, Sermanet P, Reed S, Anguelov D, et al. editors. Going deeper with convolutions. 2015 IEEE Conference on Computer Vision and Pattern Recognition (CVPR); 2015 June 7-12; Boston, Massachusetts, USA. (2015). pp. 1–10. doi: 10.1109/CVPR.2015.7298594
76. He K, Zhang X, Ren S, Sun J. editors. Deep residual learning for image recognition. 2016 IEEE Conference on Computer Vision and Pattern Recognition (CVPR); 2016 June 27-30; Las Vegas, Nevada, USA. (2016). pp. 770–8. doi: 10.1109/CVPR.2016.90
77. Huang G, Liu Z, van der Maaten L, Weinberger KQ. editors. Densely connected convolutional networks. 2017 IEEE Conference on Computer Vision and Pattern Recognition (CVPR); 2017 July 21-26; Honolulu, Hawaii, USA. (2017), pp. 2261–9. doi: 10.1109/CVPR.2017.243
78. Kundu R, Das R, Geem ZW, Han GT, Sarkar R. Pneumonia detection in chest X-ray images using an ensemble of deep learning models. PloS One (2021) 16(9):e0256630. doi: 10.1371/journal.pone.0256630
79. Dosovitskiy A, Beyer L, Kolesnikov A, Weissenborn D, Zhai X, Unterthiner T, et al. An image is worth 16x16 words: transformers for image recognition at scale. [arXiv preprint] arXiv:2010.11929. (2020). doi: 10.48550/arXiv.2010.11929
80. Wu J, Hu R, Xiao Z, Chen J, Liu J. Vision transformer-based recognition of diabetic retinopathy grade. Med Phys (2021) 48(12):7850–63. doi: 10.1002/mp.15312
81. He S, Grant PE, Ou Y. Global-local transformer for brain age estimation. IEEE Trans Med Imaging (2022) 41(1):213–24. doi: 10.1109/tmi.2021.3108910
82. Cheng J, Liu J, Kuang H, Wang J. A fully automated multimodal mri-based multi-task learning for glioma segmentation and idh genotyping. IEEE Trans Med Imaging (2022) 41(6):1520–32. doi: 10.1109/tmi.2022.3142321
83. Liu Z, Lin Y, Cao Y, Hu H, Wei Y, Zhang Z, et al. editors. (2021). Swin transformer: hierarchical vision transformer using shifted windows. 2021 IEEE/CVF International Conference on Computer Vision (ICCV); 2021 Oct. 10-17; Montreal, Quebec, Canada. (2021). pp. 9992–10002. doi: 10.1109/ICCV48922.2021.00986
84. Ma X, Xia L, Chen J, Wan W, Zhou W. Development and validation of a deep learning signature for predicting lymph node metastasis in lung adenocarcinoma: comparison with radiomics signature and clinical-semantic model. Eur Radiol (2023) 33(3):1949–62. doi: 10.1007/s00330-022-09153-z
85. Colen RR, Fujii T, Bilen MA, Kotrotsou A, Abrol S, Hess KR, et al. Radiomics to predict immunotherapy-induced pneumonitis: proof of concept. Investigational New Drugs (2018) 36(4):601–7. doi: 10.1007/s10637-017-0524-2
86. Cheng M, Lin R, Bai N, Zhang Y, Wang H, Guo M, et al. Deep learning for predicting the risk of immune checkpoint inhibitor-related pneumonitis in lung cancer. Clin Radiol (2023) 78(5):e377–e85. doi: 10.1016/j.crad.2022.12.013
87. Chen F, Niu J, Wang M, Zhu H, Guo Z. Re-evaluating the risk factors for radiation pneumonitis in the era of immunotherapy. J Trans Med (2023) 21(1):368. doi: 10.1186/s12967-023-04212-5
88. Shaverdian N, Lisberg AE, Bornazyan K, Veruttipong D, Goldman JW, Formenti SC, et al. Previous radiotherapy and the clinical activity and toxicity of pembrolizumab in the treatment of non-small-cell lung cancer: A secondary analysis of the keynote-001 phase 1 trial. Lancet Oncol (2017) 18(7):895–903. doi: 10.1016/s1470-2045(17)30380-7
89. Kawahara D, Imano N, Nishioka R, Ogawa K, Kimura T, Nakashima T, et al. Prediction of radiation pneumonitis after definitive radiotherapy for locally advanced non-small cell lung cancer using multi-region radiomics analysis. Sci Rep (2021) 11(1):16232. doi: 10.1038/s41598-021-95643-x
90. Romita A, Tohidinezhad F, Traverso A, Dekker A, De Ruysscher D. A radiomic approach to differentiate the immunotherapy-induced pneumonitis in patients with stage iv nsclc. Ann Oncol (2021) 32:S1381–S. doi: 10.1016/j.annonc.2021.10.034
91. Tohidinezhad F, Traverso A, Zhovannik I, Romita A, Hendriks LEL, Dingemans AMC, et al. Predicting the differential diagnosis of pneumonitis in stage iv non-small cell lung cancer (Nsclc) patients treated with immune checkpoint inhibitors (Icis) by combining clinical and radiomic features. Ann Oncol (2021) 32:S997–S8. doi: 10.1016/j.annonc.2021.08.1885
92. Tohidinezhad F, Bontempi D, Zhang Z, Dingemans AM, Aerts J, Bootsma G, et al. Computed tomography-based radiomics for the differential diagnosis of pneumonitis in stage iv non-small cell lung cancer patients treated with immune checkpoint inhibitors. Eur J Cancer (2023) 183:142–51. doi: 10.1016/j.ejca.2023.01.027
93. Chen X, Sheikh K, Nakajima E, Lin CT, Lee J, Hu C, et al. Radiation versus immune checkpoint inhibitor associated pneumonitis: distinct radiologic morphologies. Oncologist (2021) 26(10):e1822–e32. doi: 10.1002/onco.13900
94. Cheng J, Pan Y, Huang W, Huang K, Cui Y, Hong W, et al. Differentiation between immune checkpoint inhibitor-related and radiation pneumonitis in lung cancer by ct radiomics and machine learning. Med Phys (2022) 49(3):1547–58. doi: 10.1002/mp.15451
95. Qiu Q, Xing L, Wang Y, Feng A, Wen Q. Development and validation of a radiomics nomogram using computed tomography for differentiating immune checkpoint inhibitor-related pneumonitis from radiation pneumonitis for patients with non-small cell lung cancer. Front Immunol (2022) 13:870842. doi: 10.3389/fimmu.2022.870842
96. Cousin F, Louis T, Occhipinti M, Vos W, Guiot J, Hustinx R. Radiomics and delta-radiomics signatures to predict response and survival in patient with non-small cell lung cancer treated with immune checkpoint inhibitors. J Immunother Cancer (2022) 10:A1335–A. doi: 10.1136/jitc-2022-SITC2022.1287
97. Farina B, Guerra ADR, Bermejo-Pelaez D, Miras CP, Peral AA, Madueno GG, et al. Integration of longitudinal deep-radiomics and clinical data improves the prediction of durable benefits to anti-pd-1/pd-L1 immunotherapy in advanced nsclc patients. J Trans Med (2023) 21(1):174. doi: 10.1186/s12967-023-04004-x
98. Gong J, Bao X, Wang T, Liu JY, Peng WJ, Shi JY, et al. A short-term follow-up ct based radiomics approach to predict response to immunotherapy in advanced non-small-cell lung cancer. Oncoimmunology (2022) 11(1):2028962. doi: 10.1080/2162402X.2022.2028962
99. Jazieh K, Khorrami M, Saad A, Gad M, Gupta A, Patil P, et al. Novel imaging biomarkers predict outcomes in stage iii unresectable non-small cell lung cancer treated with chemoradiation and durvalumab. J Immunother Cancer (2022) 10(3):e003778. doi: 10.1136/jitc-2021-003778
100. Mu W, Tunali I, Gray JE, Qi J, Schabath MB, Gillies RJ. Radiomics of (18)F-fdg pet/ct images predicts clinical benefit of advanced nsclc patients to checkpoint blockade immunotherapy. Eur J Nucl Med Mol Imaging (2020) 47(5):1168–82. doi: 10.1007/s00259-019-04625-9
101. Tankyevych O, Trousset F, Latappy C, Berraho M, Dutilh J, Tasu JP, et al. Development of radiomic-based model to predict clinical outcomes in non-small cell lung cancer patients treated with immunotherapy. Cancers (2022) 14(23):5931. doi: 10.3390/cancers14235931
102. Xie D, Xu FY, Zhu WC, Pu CL, Huang SY, Lou KH, et al. Delta radiomics model for the prediction of progression-free survival time in advanced non-small-cell lung cancer patients after immunotherapy. Front Oncol (2022) 12:990608. doi: 10.3389/fonc.2022.990608
103. Sayer MR, Mambetsariev I, Lu KH, Wong CW, Duche A, Beuttler R, et al. Predicting survival of nsclc patients treated with immune checkpoint inhibitors: impact and timing of immune-related adverse events and prior tyrosine kinase inhibitor therapy. Front Oncol (2023) 13:1064169. doi: 10.3389/fonc.2023.1064169
104. Endo M, Johkoh T, Kimura K, Yamamoto N. Imaging of gefitinib-related interstitial lung disease: multi-institutional analysis by the west Japan thoracic oncology group. Lung Cancer (Amsterdam Netherlands) (2006) 52(2):135–40. doi: 10.1016/j.lungcan.2006.02.002
105. Inoue A, Saijo Y, Maemondo M, Gomi K, Tokue Y, Kimura Y, et al. Severe acute interstitial pneumonia and gefitinib. Lancet (London England) (2003) 361(9352):137–9. doi: 10.1016/s0140-6736(03)12190-3
106. Yoneda KY, Scranton JR, Cadogan MA, Tassell V, Nadanaciva S, Wilner KD, et al. Interstitial lung disease associated with crizotinib in patients with advanced non-small cell lung cancer: independent review of four profile trials. Clin Lung Cancer (2017) 18(5):472–9. doi: 10.1016/j.cllc.2017.03.004
107. Nishino M. Imaging of oncologic treatment-related pneumonitis: A focused review on emerging issues of immune checkpoint inhibitor pneumonitis, from the ajr special series on inflammation. AJR Am J Roentgenol (2022) 218(1):19–27. doi: 10.2214/ajr.21.25454
108. Nishino M, Hatabu H, Hodi FS, Ramaiya NH. Drug-related pneumonitis in the era of precision cancer therapy. JCO Precis Oncol (2017) 1:1–12. doi: 10.1200/po.17.00026
109. Arroyo-Hernández M, Maldonado F, Lozano-Ruiz F, Muñoz-Montaño W, Nuñez-Baez M, Arrieta O. Radiation-induced lung injury: current evidence. BMC Pulmonary Med (2021) 21(1):9. doi: 10.1186/s12890-020-01376-4
111. Cousin F, Desir C, Ben Mustapha S, Mievis C, Coucke P, Hustinx R. Incidence, risk factors, and ct characteristics of radiation recall pneumonitis induced by immune checkpoint inhibitor in lung cancer. Radiother oncol: J Eur Soc Ther Radiol Oncol (2021) 157:47–55. doi: 10.1016/j.radonc.2021.01.001
112. Bousabarah K, Blanck O, Temming S, Wilhelm ML, Hoevels M, Baus WW, et al. Radiomics for prediction of radiation-induced lung injury and oncologic outcome after robotic stereotactic body radiotherapy of lung cancer: results from two independent institutions. Radiat Oncol (London England) (2021) 16(1):74. doi: 10.1186/s13014-021-01805-6
113. Dang J, Li G, Zang S, Zhang S, Yao L. Risk and predictors for early radiation pneumonitis in patients with stage iii non-small cell lung cancer treated with concurrent or sequential chemoradiotherapy. Radiat Oncol (London England) (2014) 9:172. doi: 10.1186/1748-717x-9-172
114. Yushkevich PA, Yang G, Gerig G. editors. Itk-snap: an interactive tool for semi-automatic segmentation of multi-modality biomedical images. 2016 38th Annual International Conference of the IEEE Engineering in Medicine and Biology Society (EMBC); 2016 Aug. 16-20; Orlando, Florida, USA. (2016). pp. 3342–5. doi: 10.1109/embc.2016.7591443
115. Virzì A, Muller CO, Marret JB, Mille E, Berteloot L, Grévent D, et al. Comprehensive review of 3d segmentation software tools for mri usable for pelvic surgery planning. J Digital Imaging (2020) 33(1):99–110. doi: 10.1007/s10278-019-00239-7
Keywords: radiomics, non-small cell lung cancer, immune checkpoint inhibitor-related pneumonitis, deep learning, immunotherapy
Citation: Shu Y, Xu W, Su R, Ran P, Liu L, Zhang Z, Zhao J, Chao Z and Fu G (2023) Clinical applications of radiomics in non-small cell lung cancer patients with immune checkpoint inhibitor-related pneumonitis. Front. Immunol. 14:1251645. doi: 10.3389/fimmu.2023.1251645
Received: 02 July 2023; Accepted: 24 August 2023;
Published: 20 September 2023.
Edited by:
Eros Montin, New York University, United StatesReviewed by:
Ilias Giannakopoulos, New York University, United StatesCopyright © 2023 Shu, Xu, Su, Ran, Liu, Zhang, Zhao, Chao and Fu. This is an open-access article distributed under the terms of the Creative Commons Attribution License (CC BY). The use, distribution or reproduction in other forums is permitted, provided the original author(s) and the copyright owner(s) are credited and that the original publication in this journal is cited, in accordance with accepted academic practice. No use, distribution or reproduction is permitted which does not comply with these terms.
*Correspondence: Zhen Chao, WmhlbkNoYW9Ac2RmbXUuZWR1LmNu; Guobin Fu, Zmdic0BzaW5hLmNvbQ==
†These authors have contributed equally to this work and share first authorship
Disclaimer: All claims expressed in this article are solely those of the authors and do not necessarily represent those of their affiliated organizations, or those of the publisher, the editors and the reviewers. Any product that may be evaluated in this article or claim that may be made by its manufacturer is not guaranteed or endorsed by the publisher.
Research integrity at Frontiers
Learn more about the work of our research integrity team to safeguard the quality of each article we publish.