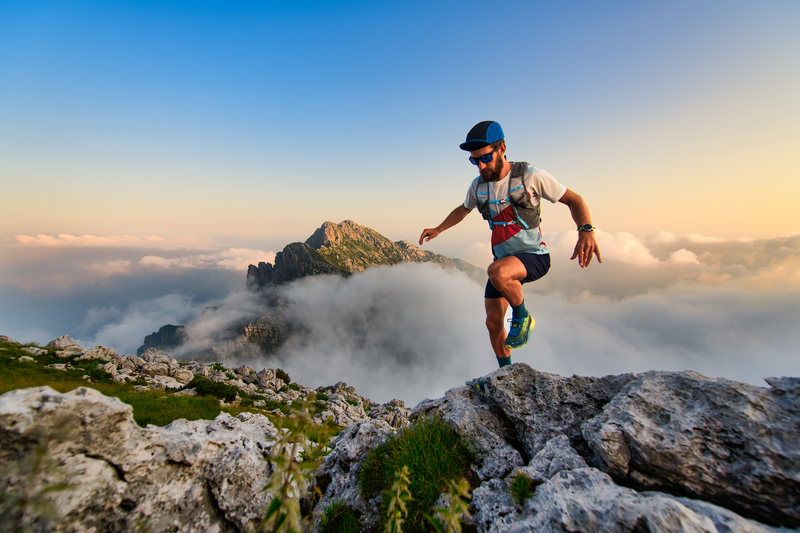
94% of researchers rate our articles as excellent or good
Learn more about the work of our research integrity team to safeguard the quality of each article we publish.
Find out more
ORIGINAL RESEARCH article
Front. Immunol. , 07 December 2023
Sec. Autoimmune and Autoinflammatory Disorders : Autoimmune Disorders
Volume 14 - 2023 | https://doi.org/10.3389/fimmu.2023.1249017
Objectives: Epidemiological studies suggested a potential connection between education and autoimmune disorders. This study investigated the possible cause-and-effect relationship using a Mendelian randomization approach.
Methods: We explored the causality between four education traits (n = 257,841~1,131,881) and 22 autoimmune diseases. The mediating role of smoking (632,802 individuals), BMI (681,275 individuals), alcohol (335,394 individuals), and income (397,751 individuals) was also investigated. Transcriptome-wide association study (TWAS) and enriched signaling pathways analysis were used to investigate the underlying biological mechanisms.
Results: Especially, higher cognitive performance was protective for psoriasis (odds ratio (OR) = 0.69, 95% confidence interval (CI) = 0.60-0.79, p = 6.12×10-8), rheumatoid arthritis (RA) (OR = 0.75, 95% CI = 0.67-0.83, p = 4.62×10-6), and hypothyroidism (OR = 0.83, 95% CI = 0.77-0.90, p = 9.82×10-6). Higher levels of educational attainment decreased risks of psoriasis (OR = 0.61, 95% CI = 0.52-0.72, p = 1.12×10-9), RA (OR = 0.68, 95% CI = 0.59-0.79, p = 1.56×10-7), and hypothyroidism (OR = 0.80, 95% CI = 0.72-0.88, p = 5.00×10-6). The completion of highest-level math class genetically downregulates the incidence of psoriasis (OR = 0.66, 95% CI = 0.58-0.76, p = 2.47×10-9), RA (OR = 0.71, 95% CI = 0.63-0.81, p = 5.28×10-8), and hypothyroidism (OR = 0.85, 95% CI = 0.79-0.92, p = 8.88×10-5). Higher self-reported math ability showed protective effects on Crohn’s disease (CD) (OR = 0.67, 95% CI = 0.55-0.81, p = 4.96×10-5), RA (OR = 0.76, 95% CI = 0.67-0.87, p = 5.21×10-5), and psoriasis (OR = 0.76, 95% CI = 0.65-0.88, p = 4.08×10-4). Protein modification and localization, response to arsenic-containing substances may participate in the genetic association of cognitive performance on UC, RA, psoriasis, and hypothyroidism. According to mediation analyses, BMI, smoking, and income served as significant mediators in the causal connection between educational traits and autoimmune diseases.
Conclusion: Higher levels of education-related factors have a protective effect on the risk of several autoimmune disorders. Reducing smoking and BMI and promoting income equality can mitigate health risks associated with low education levels.
Autoimmune diseases are a breach of immune tolerance that cannot differentiate self from non-self, affect 3-5% of the population, and have more than 100 different types (1). Some have lesions that are localized to specific organs, such as primary sclerosing cholangitis (PSC), while others involve multiple organs and systems, such as systemic lupus erythematosus (SLE) (2). The exact cause of autoimmune diseases remains uncertain and may be influenced by a combination of genetic factors and epigenetic changes caused by environmental factors (3), lifestyle (4), and intestinal microbiota (5). However, it’s worth noting that observational studies don’t always prove causality because of confounding factors and reverse causal effects. More advanced tools are required to investigate the potential etiologies of autoimmune disorders.
Mendelian Randomization (MR) is a research method that enables the investigation of the causal relationship between the factors of interest and outcomes using instrumental variables (IVs) (6). When performing an MR study, genetic variants highly linked to exposure and fulfill specific criteria are utilized as instrumental variables (IVs) to probe the cause-and-effect relationship with an outcome. Proper execution of MR can help mitigate bias caused by confounding environmental factors, as these variants are randomly allocated during conception. Thus, the design of MR closely resembles that of a randomized controlled trial (RCT). When selecting exposures and outcomes, the sample size is crucial as it can lead to weak instrumental variable bias (7).
One of the main focus areas in the summary level of GWAS data is education, which includes cognitive ability, educational achievement, highest-level math class taken, and self-reported math proficiency. The risk factors associated with education, including biological (such as BMI), behavioral (such as smoking and alcohol use), and psychosocial factors (such as income), have been extensively studied in relation to cardiovascular disease (8, 9) psychiatric disorders (10), and neurodegenerative diseases (11, 12). Some observational studies also suggested that higher education has a protective effect on psoriasis (13), RA (14), and SLE (15).
To obtain the causal relationship between education on autoimmune diseases and potential mediator factors, we applied a two-sample and a two-step MR using cognitive ability, educational achievement, highest-level math class taken, and self-reported math proficiency as exposures, 22 traits of autoimmune disorders [asthma and allergy (AA), systemic lupus erythematosus (SLE), rheumatoid arthritis (RA), psoriasis (PsO), hypothyroidism, hyperthyroidism, interstitial lung disease (ILD) related to systemic autoimmune disease, other autoimmune hemolytic anemias (AIHA), scleroderma, sicca syndrome (SS), ankylosing spondylitis (AS), amyotrophic lateral sclerosis (ALS), asthma, Crohn’s disease (CD), ulcerative colitis (UC), celiac disease (CeD), irritable bowel syndrome (IBS), multiple sclerosis (MS), primary biliary cholangitis (PBC), primary sclerosing cholangitis (PSC), and type 1 diabetes (T1D)] as outcomes, and BMI, alcohol use, and income as potential mediators. We also performed TWAS and GO: BP enrichment analyses to explore potential transcriptomic and biological process basis for the genetic associations.
To conduct an MR study, the genetic variants (single nucleotide polymorphisms, SNPs) must meet several essential requirements to be used as IVs. Firstly, they should be strongly connected with the exposure of being studied (relevance assumption). Secondly, they are not associated with confounders of the risk factor-outcome association (independence assumption). Finally, it is crucial that the SNPs only impact the outcomes through the exposures (restriction assumption) (16).
The available GWAS summary statistics correlated with cognitive performance (n= 257,841), educational attainment (n= 1,131,881), highest-level math class completion (n= 430,445), and self-reported math ability (n= 564,698) was derived from the GWAS meta-analysis of European ancestry of the Social Science Genetic Association Consortium (SSGAC) (17). This MR study used a meta-analysis of cognitive performance based on published data from the Cognitive Genomics Consortium (COGENT) and new analyses of the United Kingdom Biobank (UKB) of European ancestry (18). The study collected data on educational attainment from 766,345 participants of European ancestry who were over 30 years old, and one standard deviation represents an increase of 4.2 years of education completion (17). Detailed information of data sources for exposures is in Supplementary Table 1.
The GWAS data of 22 traits of autoimmune diseases including asthma and allergy (AA) (n= 197,963), systemic lupus erythematosus (SLE) (n= 257,998), rheumatoid arthritis (RA) (n= 232,501), psoriasis (n= 339,050), hypothyroidism (n= 287,247), hyperthyroidism (n= 257,552), interstitial lung disease (ILD) related to systemic autoimmune disease (n= 341,986), other autoimmune hemolytic anemias (AIHA) (n= 341,986), scleroderma (n= 322,208), sicca syndrome (SS) (n= 334,362), ankylosing spondylitis (AS) (n= 251,394), and autoimmune diseases (n= 342,499) are from the FINNGEN dataset (19), amyotrophic lateral sclerosis (ALS) (n= 138,086) (20), asthma (n= 408,422) (21), Crohn’s disease (CD) (n= 40,266) (22), celiac disease (CeD) (n= 456,348) (23), irritable bowel syndrome (IBS) (n= 486,601), multiple sclerosis (MS) (n= 115,803) (24), primary biliary cholangitis (PBC) (n= 24,510) (25), primary sclerosing cholangitis (PSC) (n= 14,890) (26), type 1 diabetes (T1D) (n= 24,840) (27), ulcerative colitis (UC) (n= 45,975) (26). Detailed information of data sources for outcomes is in Supplementary Table 1.
The summary-level GWAS data correlated with smoking (n= 632,802) and alcohol use (n= 335,394) were derived from a meta-analysis of European participants conducted by Mengzhen Liu et al. (28). The summary-level GWAS data correlated with BMI (n= 681,275, each SD =4.8 kg/m2) was gathered through a meta-analysis of individuals from Europe conducted by Loic Yengo et al. (29) The GWAS data related to average total household income before tax (n= 397,751; GWAS ID: ukb-b-7408) was derived from the UK Biobank (http://www.nealelab.is/uk-biobank/) (30) of European ancestry, conducted through an open-ended questionnaire. Detailed information of data sources for mediators is in Supplementary Table 1.
To choose the appropriate genetic tools for each of the four exposures (including cognitive performance, educational attainment, highest-level math class completed, and self-reported math ability), we used the default settings in the R package TwoSampleMR (31, 32) to identify significant genetic variations, we extracted SNPs with a P-value less than 5.0×10-8. We used standard clumping criteria to identify distinct SNPs. Specifically, we set the clumping window to 10,000 kb and applied an LD r2 threshold of 0.001. We then calculated the R2 and F-statistics to determine the strength of the identified genetic variations in explaining the proportion of exposure variance (33). , K= n SNPs and N= n sample, the F-number should be greater than 10). Detailed information on instrumental SNPs for exposures and mediators is in Supplementary Tables 2–9.
To account for variant heterogeneity and the pleiotropy effect, we employed three distinct techniques in MR analysis: Random-effect inverse-variance weighted (IVW), MR Egger, and weighted median (32). We also removed any SNPs related to 22 traits of autoimmune diseases mentioned above and outliers identified with MRPRESSO. We then utilized MR-Egger and weighted median in conjunction with the primary outcome of IVW. These approaches are recognized for producing more reliable results in various situations, albeit with slightly broader confidence intervals. MR-Egger considers the possibility of pleiotropic effects in all genetic variants, but it is essential that these effects do not influence the variant-exposure association (34). To conduct a test on a global scale, a significant two-sided P-value of 0.05 was established. For regional-level analyses utilizing 88 MR estimates, a Bonferroni-corrected P-value of 0.05/88 (5.68×10-4) was used, while any p<0.05 was set as nominally significant.
To investigate the extent to which education affects autoimmune diseases via mediators (including BMI, income, smoking, and alcohol use), a 2-step MR by the product of the coefficient method was performed using public GWAS summary statistics (35, 36). The first step involved estimating the impact between education and BMI, alcohol consumption, smoking habits, and income. Following this, we investigated the genetic predisposition of these mediators on autoimmune disorders. The overall effect of education was divided into a direct effect (the genetic predisposition between education and autoimmune disorders independent of the mediator) and an indirect effect (the genetic predisposition between education and autoimmune disorders by the mediator). The mediating effect from the exposure to the outcome was calculated using the product of coefficients method, which involved converting ORs for binary outcomes to log ORs. We then analyzed the data and calculated the proportion of the effect. Standard errors were calculated by the Delta method.
We used the FUSION (37) method to transform GWAS into TWAS. This was done by utilizing a linear model of expression quantitative trait loci (eQTL) to estimate gene expression from European Blood RNA-seq Genotype-Tissue Expression version 8 (GTEx v8) (38). We then used the TWAS method to identify homozygous gene clusters linked to education measures and autoimmune disease traits. After analyzing the genes involved, we proceeded to conduct GO enrichment analyses (39) to better comprehend the biological processes that link education and autoimmune diseases. We used R packages such as clusterProfiler (40), enrichplot, and DOSE (41) to perform the analyses based on the Gene Ontology database (42, 43). The P-value was calculated using Fisher’s Combined P-value (FCP) method. As we only obtained cognitive performance data from the exposure, we performed TWAS and GO:BP enrichment analyses solely on positive cognitive performance results. The experimental design flow chart of MR and TWAS is shown in Figure 1.
Figure 1 (A) Four steps of two-sample and two-step MR analysis of cause-effect of education-related factors on autoimmune diseases. The first step is to obtain the summary-level GWAS statistics of exposures, outcomes, and mediators. The second step is to select the qualified instrumental variables (SNPs), the third step is to perform the two-sample and two-step MR, and the last step is to conduct sensitivity analysis. (B) Design of the two-sample Mendelian randomization study. Three core assumptions were as follows: 1) the SNPs should be strongly associated with education-related factors; 2) the SNPs should not be related to confounders; 3) the SNPs should not be directly associated with autoimmune diseases. (C) The total effect (β0) was decomposed into the cause-effect of education-related factors on mediators (β1) and the cause-effect of mediators on autoimmune diseases (β2). The indirect effect equals β0-β1×β2. (D) The process of TWAS and GO: BP enrichment analyses. The first step is to identify the common genes between education and autoimmune diseases. The second step was to perform an enrichment analysis of biological processes targeting the transcriptome corresponding to the genes. The figure was built by BioRender.
To obtain accurate estimates, we thoroughly evaluated horizontal pleiotropy through MR-Egger intercept testing and leave-one-out analyses. We also utilized Cochran’s Q tests to detect heterogeneity (44) and funnel plots to assess the possible presence of pleiotropy.
The study solely relied upon publicly available information, resulting in the waiver of ethical approval. Each of the studies that contributed to the GWAS has information on ethical approval and participant consent in their original publications. No specific ethical approval is required in this study.
Four different measures of education (cognitive performance, educational attainment, highest-level math class completed, and self-reported math ability) affect different autoimmune diseases to varying degrees. The complete results of the inverse variance-weighted (IVW) method are shown in Figure 2.
Figure 2 IVW estimates from cognitive performance, educational attainment, highest-level math class completed, and self-reported math ability on 22 traits of autoimmune diseases. The color of each block represents the IVW-derived P-values of every MR analysis. P-values of< 0.05 were shown in orange and set as nominal significant, and P-values of > 0.05 were shown in yellow and set as non-significant. P value< 5.68×10-4 is set as significant and shown in red.
Higher cognitive performance can downregulate risks of autoimmune disorders, including autoimmune diseases, psoriasis, RA, hypothyroidism, and UC, with the OR of 95% CI of 0.84 (0.80, 0.90), 0.69 (0.60, 0.79), 0.75 (0.67, 0.83), 0.83 (0.77, 0.90), and 0.76 (0.64, 0.88), respectively. The P-values of each trait were 1.06×10-9, 6.12×10-8, 4.62×10-6, 9.82×10-6, and 4.72×10-4, respectively; an increase of completion of 4.2 years of education was protective for autoimmune diseases, psoriasis, RA, hypothyroidism, asthma, CD, and IBS, and the ORs with 95% CI were 0.82 (0.76, 0.87), 0.61 (0.52, 0.72), 0.68 (0.59, 0.79), 0.80 (0.72, 0.88), 0.84 (0.77, 0.90), 0.62 (0.50, 0.77), and 0.73 (0.62, 0.85) with the P-values for each disease of 1.44×10-9, 1.12×10-9, 1.56×10-7, 5.00×10-6, 6.63×10-6, 1.28×10-5, and 9.95×10-5, respectively; highest-level math class completed is related to decreased incidence of autoimmune diseases, psoriasis, RA, IBS, hypothyroidism, PBC, and CD. The ORs with 95% CI were 0.86 (0.81, 0.91), 0.66 (0.58, 0.76), 0.71 (0.63, 0.81), 0.73 (0.63, 0.83), 0.85 (0.79, 0.92), 0.53 (0.38, 0.74), and 0.71 (0.59, 0.85), with P-values of 1.05×10-7, 2.47×10-9, 5.28×10-8, 5.25×10-6, 8.88×10-5, 1.54×10-4, and 2.31×10-4, respectively. Higher self-reported math ability shows protective effects against autoimmune diseases, CD, RA, IBS, and psoriasis. The OR with 95% CI for each trait was 0.87 (0.82, 0.92), 0.67 (0.55, 0.81), 0.76 (0.67, 0.87), 0.75 (0.65, 0.88), 0.76 (0.65, 0.88) with P-values of 1.25×10-5, 4.96×10-5, 5.21×10-5, 2.44×10-4, 4.08×10-4. Scatter plots displaying the significant outcomes of MR effect regarding the impact of cognitive performance (Figure 3), self-reported ability (Figure 4), educational attainment (Figure 5), and completion of highest-level math class (Figure 6) on autoimmune disorders, separately. Detailed results are shown in Table 1 and Supplementary Table 10.
Figure 3 Scatter plots displaying the significant outcomes of MR effect regarding the impact of cognitive performance on autoimmune disorders. The x-axis represents the genetic association with cognitive performance risk; the y-axis represents the genetic association with the risk of autoimmune diseases, UC, hypothyroidism, psoriasis, and RA.
Figure 4 Scatter plots displaying the significant outcomes of MR effect regarding the impact of self-reported ability on autoimmune disorders. The x-axis represents the genetic association with self-reported ability risk; the y-axis represents the genetic association with the risk of autoimmune diseases, CD, RA, psoriasis, and IBS.
Figure 5 Scatter plots displaying the significant outcomes of the MR effect regarding the impact of educational attainment on autoimmune disorders. The x-axis represents the genetic association with educational attainment risk; the y-axis represents the genetic association with the risk of hypothyroidism, IBS, psoriasis, RA, asthma, autoimmune diseases, and CD.
Figure 6 Scatter plots displaying the significant outcomes of MR effect regarding the impact of the completion of highest-level math class on autoimmune disorders. The x-axis represents the genetic association with the completion of the highest-level math class risk; the y-axis represents the genetic association with the risk of autoimmune diseases, CD, hypothyroidism, IBS, PBC, psoriasis, and RA.
Higher cognitive performance is associated with higher personal pretax income and reduced smoking behavior; the ORs with 95% CI were 1.51 (1.47, 1.55), 0.81 (0.76, 0.85), and the P-values were 2.34×10-185, and 5.82×10-15, respectively. Every increase of one standard deviation in educational attainment, equivalent to 4.2 years of schooling, has a positive effect on the average total household income before tax and has a protective effect against smoking and BMI. The ORs with 95% CI for each trait were 1.90 (1.85, 1.96), 0.63 (0.60, 0.67), and 0.86 (0.82, 0.91); the values were less than 2.34×10-185, 3.18×10-58, and 8.57×10-8, respectively. Highest-level math class completed can also increase average total household income before tax and reduce exposure of smoking as well as BM; the ORs with 95% CI for each mediator were 1.64 (1.59, 1.68), 0.70 (0.66, 0.73), and 0.91 (0.87, 0.96). P-values were 6.65×10-266, 1.14×10-49, and 1.75×10-4, respectively. Higher self-reported math ability is related to higher average total household income before tax and decreased smoking behavior. The ORs with 95% CI for these two mediators were 1.43 (1.38, 1.48) and 0.80 (0.75, 0.85), and the P-values were 2.90×10-95 and 1.13×10-13. There is no significant genetic predisposition of these four education-related exposures to alcohol use (drinks per week).
Higher average total household income before tax has a protective effect against three traits of autoimmune disorders, including psoriasis, autoimmune diseases, and hypothyroidism; the 95% confidence interval dominance ratios were 0.55 (0.41, 0.74), 0.78 (0.68, 0.89), and 0.77 (0.64, 0.92) with the P-values of 5.73×10-5, 2.92×10-4, and 5.71×10-3, respectively. Smoking can raise the incidence of asthma and RA while reducing PSC, the 95% CI dominance ORs were 1.13 (1.03, 1.24), 1.22 (1.05, 1.43), and 0.63 (0.39, 0.99), and P-values were 7.45×10-3, 1.13×10-2, and 4.82×10-2, respectively; higher BMI increases the risk of autoimmune diseases, psoriasis, asthma, hypothyroidism, multiple sclerosis, RA, asthma and allergy and PBC. The ORs with 95% CI for the above traits were 1.18 (1.13, 1.24), 1.49 (1.33, 1.67), 1.20 (1.14, 1.27), 1.24 (1.15, 1.33), 1.36 (1.20, 1.55), 1.27 (1.15, 1.41), 1.29 (1.09, 1.52), and 1.30 (1.09, 1.54), respectively. The P-values of these autoimmune disorders were 5.89×10-13, 2.75×10-12, 2.74×10-11, 2.43×10-9, 2.91×10-6, 4.09×10-6, 2.60×10-3, and 3.40×10-3, respectively.
As for the genetic predisposition of self-reported math ability on autoimmune disorders, average total household income before tax mediated 78% (95% CI: 20% to 135%) on psoriasis, 63% (95% CI: 18% to 107%) on autoimmune diseases, and 67% (95% CI: 2% to 131%) on hypothyroidism; smoking mediated 164% (95% CI: -516% to 844%) on asthma, 17% (95% CI: 1% to 33%) on RA, and 53% (95% CI: -53% to 160%) on primary sclerosing cholangitis.
Regarding the cause-effect of cognitive performance and autoimmune disorders, it was found that the average total household income before tax acted as a mediator, accounting for 66% (95% CI: 26% to 106%) of the effect, 58% (95% CI: 21% to 96%) on autoimmune diseases, and 60% (95% CI: 10% to 110%) on hypothyroidism; smoking mediated 38% (95% CI: -6% to 81%) on asthma, and 15% (95% CI: 1% to 29%) on RA, and 84% (95% CI: -94% to 235%) on primary sclerosing cholangitis.
In regards to autoimmune disorders and educational attainment, income was found to have a mediating effect of 78% (95% CI: 32% to 124%), specifically on psoriasis, 78% (95% CI: 29% to 128%) on autoimmune diseases, and 75% (95% CI: 13% to 138%) on hypothyroidism while smoking mediated 33% (95% CI: 4% to 61%) on asthma, 24% (95% CI: 3% to 46%) on RA, 47% (95% CI: -13% to 107%) on primary sclerosing cholangitis. BMI mediated 12% (95% CI: 5% to 19%) on autoimmune diseases, 12% (95% CI: 5% to 18%) on psoriasis, 15% (95% CI: 5% to 24%) on asthma, 14% (95% CI: 5% to 22%) on hypothyroidism, 46% (95% CI: -53% to 145%) on multiple sclerosis, 9% (95% CI: 3% to 15%) on RA, 27% (95% CI: -24% to 78%) on asthma and allergy, and 12% (95% CI: -3% to 27%) on primary biliary cholangitis.
Completing the highest-level math class also genetically decreases the risks of autoimmune disorders. Specifically, there is a 71% mediation effect (with a 95% confidence interval of 29% to 113%) of the average total household income before the tax on psoriasis, 78% (95% CI: 27% to 130%) on autoimmune diseases, 82% (95% CI: 11% to 153%) on hypothyroidism. In comparison, smoking mediated 44% (95% CI: 1% to 87%) on asthma, 22% (95% CI: 3% to 40%) on RA, and 38% (95% CI: -9% to 85%) on PSC. The proportion of the indirect effect of BMI on autoimmune disorders was 9% (95% CI: 3% to 15%) on psoriasis, 10% (95% CI: 3% to 17%) on autoimmune diseases, 16% (95% CI: 2% to 31%) on asthma, 12% (95% CI: 2% to 22%) on hypothyroidism, 25% (95% CI: -17% to 67%) on multiple sclerosis, 7% (95% CI: 2% to 12%) on RA, 17% (95% CI: -10% to 44%) on asthma and allergy, 4% (95% CI: 0% to 8%) on PBC. However, it is important to note that not all exposures and outcomes have a significant causal relationship. Therefore, the results of some mediation analyses may not be credible, including the genetic links of self-reported math ability on asthma and PSC; cognitive performance on PSC; and educational attainment on asthma and allergy and PBC. Table 2 and Supplementary Table 13 provide comprehensive results.
Table 2 Causal effect of education on mediators and of mediators on autoimmune diseases in two-step Mendelian randomization analyses.
Using the TWAS method, we identified the commonly differentially expressed genes in education and autoimmune diseases. Afterward, we investigated the biological processes enrichment of these differentially expressed genes on the Gene Ontology database. A variety of biological processes were found to be enriched in the genetic predisposition of autoimmune diseases and education, providing MR studies with a potential biological and functional basis for transcriptomics. For the connection of cognitive performance on UC, biological processes including response to arsenic-containing substance (p = 7.39×10-4), cellular response to arsenic-containing substance (p = 2.96×10-4) were highly enriched; as for the association cognitive performance of RA, biological processes including positive regulation of protein targeting to membrane (p = 1.24×10-3) and regulation of protein targeting to membrane (p = 1.82×10-3) were significantly enriched; positive regulation of cold-induced thermogenesis (p = 3.67×10-4) fatty acid oxidation (p = 4.62×10-4), and lipid oxidation (p = 5.42×10-4) may participate in the development of psoriasis; protein polymerization (p = 6.27×10-5) and actin polymerization or depolymerization (p = 1.35×10-4) were highly enriched in the genetic association of cognitive performance on hypothyrodism; meiosis-related cell cycle signaling pathways including meiosis I (p = 4.57×10-4), meiosis I cell cycle process (p = 5.42×10-4), and chromosome organization involved in meiotic cell cycle (p = 1.35×10-3)may participate in the occurrence of autoimmune disorders related to the cognitive performance. The detailed results of GO: BP enrichment analyses are in Supplementary Tables 14–18. Visualization of enrichment analysis results is shown in Supplementary Figures 9–13.
MR sensitivity methods include MR-Egger intercept testing, leave-one-out analyses, and Cochran’s Q test, which can ensure the results’ stability. The MR-Egger intercept (Supplementary Table 11) testing showed no horizontal pleiotropy in the significant results of education-related factors on the autoimmune disorders. The funnel plot (Supplementary Figures 1–4) also proved the absence of pleiotropy. Cochran’s Q test (Supplementary Table 12) showed heterogeneity. However, it does not affect the results of IVW, and our conclusion is still reliable. Leave-one-out analyses (Supplementary Figures 5–8) showed that when a single SNP was gradually removed for analyses, error lines were all on the left side of zero, indicating that a single SNP does not significantly affect the overall outcome.
As far as we know, this is the first extensive analysis using MR that thoroughly investigates the genetic predisposition of cognitive performance, educational attainment, highest-level math class completion, and self-reported math ability on 22 traits of autoimmune disorders (AA, SLE RA, psoriasis, hypothyroidism, hyperthyroidism, ILD, AIHA, SS, scleroderma, AS, autoimmune diseases, asthma, ALS, CD, CeD, IBS, PBC, PSC, MS, T1D, and UC), and the potential mediating effect of BMI, income, smoking and alcohol use. The GWAS dataset used in this study is sizeable and can potentially mitigate the weak instrument bias in the MR study. In the present MR analysis, we determined that the genetic predisposition of higher levels of education-related factors led to lower relative odds (OR) of several autoimmune diseases, including psoriasis, hypothyroidism, asthma, RA, CD, UC, and IBS to different degrees. The influence of education-related factors on autoimmune diseases seems partially explained by smoking, income, and BMI, accounting for 10% to 80% of the effects.
A growing body of research has determined that social determinants of health (SDH), especially education-related factors, have an increasingly close relationship to a variety of diseases, including neoplastic diseases (45), cardiovascular diseases (46, 47), autoimmune diseases, etc. Several cross-section and cohort studies have demonstrated that higher education-related factors can protect the occurrence and progression of autoimmune diseases. For instance, a study conducted in Denmark that analyzed 61,153 children discovered that those who had atopic dermatitis (AD) had a higher likelihood of not achieving lower secondary education and upper secondary education (48). E Portaccio et al. (49) investigated 115 adult-onset MS and 111 pediatric-onset MS patients and found that cognitive performance and educational attainment have a protective effect on socio-professional outcomes, and lower education levels also increase the inflammatory activity in MS (50).
However, autoimmune diseases are an extremely large spectrum of diseases, and research on the genetic predisposition of educational factors on many autoimmune diseases is still lacking. A significant advantage of this study is that MR can evaluate both causal and mediating effects. This not only minimizes the confounding errors that arise in observational experiments but also eliminates the ethical and economic issues associated with randomized controlled trials. In our MR study, we found that a higher level of education can genetically decrease the incidence of various autoimmune disorders; we then investigated the potential mediators of the genetic predisposition between education and autoimmune disorders.
To obtain possible mediating effects, we utilized a two-step MR analysis for potential mediators and their proportion mediating effect of the total genetic cause-effect of the education-related factors on autoimmune diseases. According to a multivariable two-sample MR analysis conducted by Davis NM et al. (51), obtaining a higher level of education has been found to have a positive impact on income and alcohol consumption while showing negative effects on smoking, BMI, and sedentary behavior. Therefore, we chose BMI, average total household income before tax, smoking, and alcohol use as mediators. The results of the two-step MR analysis suggested that income, smoking, and BMI mediated different cause-effect of exposures and outcomes to different degrees, which was similar to the former MR study conducted by Ferguson LD (52), which demonstrated that the central adiposity is related to psoriasis and RA. The result of the mediating effect of BMI and smoking on educational attainment on RA also agreed with Zhao SS et al. (53). In contrast, the mediating effect of alcohol use was insignificant, which corresponded with what Bae SC et al. (54) and Wei J et al. (55) did before. Smoking has been demonstrated to increase the risks of MS (56), RA (57), and other autoimmune disorders (58). Higher BMI is also related to higher incidence and poorer treatment response of RA, SLE, IBD, and psoriasis (59). The biological mechanism of mediating the effect of BMI may relate to the pro-inflammatory processes of adipose tissue, which can decrease Bregs and Tregs, promote the activation of Th17 and Th1 cells (60), and generate dysregulated intestinal flora metabolites (61). Smoking can cause oxidative stress and produce ROS and other free radicals, generating autoreactive pro-inflammatory T cells, autoantibodies, and pro-inflammatory cytokines (58).
We then conducted TWAS and GO: BP enrichment analyses to explore the biological mechanisms that may explain the genetic link between education and autoimmune diseases. Because of the integrity of the GWAS database, we only studied the relationship of cognitive performance on UC, RA, psoriasis, hypothyroidism, and autoimmune disease. According to our enrichment analyses, biological processes, including response to arsenic-containing substance and protein transcription, may participate in the occurrence of UC. Trivalent arsenic [As(III)] has recently been found to be an immunomodulatory agent (62), and the organic arsenic derivative acetarsol has been studied for mesalazine-refractory UC and showed some curative effects (63). The exposure to As can influence the induction and modulation of regulatory T cells, thereby decreasing immune surveillance and increasing autoimmune disease risk (64). Biological processes, such as protein localization, play a role in the genetic association of cognitive performance on RA, including regulating protein targeting to the membrane. The distinct expressions of the regulators of protein trafficking and the membrane adapter protein are essential indicators of metabolically reprogrammed T cells. Naive CD4 T cells can turn into pro-inflammatory helper T cells through protein targeting process, which contributes to the development of RA (65). Fatty acid oxidation and lipid oxidation participate in the development of psoriasis. The oxidative stress in psoriasis increases the production of lipid mediators, particularly eicosanoids. These are vital for the differentiation of Th1 and Th17 cells and the modulation of cellular immunity in psoriasis patients (66). Abnormal lipid metabolism can lead to ferroptosis, and inhibiting keratinocyte ferroptosis can suppress psoriatic inflammation by reducing cytokine production (67). Actin polymerization or depolymerization may partially explain the relationship between cognitive performance and hypothyroidism. An animal study found that defects in AFAP1L2 can affect cellular polarity and cytoskeletal structure, resulting in epithelial function disorders like congenital hypothyroidism (68). Targeting this process in the future could be an effective method for regulating immune cells and controlling autoimmune diseases.
This study has several limitations. First, although some cohort studies suggest that autoimmune diseases may impede academic development in patients (69–72), we were unable to conduct a reverse MR study due to the incomplete summary-level GWAS statistics of the exposure. Second, compared to other observational designs, MR is less prone to confounding as genetic variants are predetermined; the population stratification can still be a potential confounder at the sample level. This occurs when there is a correlation resulting from sub-populations that have varying distributions of genetic variants and exposure/outcome (73, 74). For example, the summary level of GWAS data for this MR analysis was limited to those of Europeans, and the incidence of autoimmune diseases varies among different races, ethnicities, and regions (75). Additionally, we identified smoking, alcohol consumption, BMI, and income as potential factors that could help explain the link between education and autoimmune diseases based on previous research. However, it’s important to note that other factors, such as external environment, self-induced stress, and dietary habits, may also play a role but were not taken into consideration in this study. Last, MR may not accurately capture changes in exposures over time, and a single BMI measurement may not provide a complete picture of BMI throughout one’s life (76).
In conclusion, our two-sample and two-step MR showed a protective effect of higher levels of education-related factors (including cognitive performance, educational attainment, highest-level math class completed, and self-reported math ability) on particular autoimmune diseases (including psoriasis, hypothyroidism, asthma, RA, CD, UC, and IBS); encouraging population-level interventions to decrease smoking, manage excess weight, and promote income equality could effectively mitigate the increased risks. However, the impact of education on autoimmune diseases is still largely uncertain, but it’s certain that enhancing access to education and controlling modifiable factors, including BMI and smoking, are vital measures for lower risk of autoimmune diseases and better health outcomes. Moreover, it’s imperative to conduct more studies on environmental risk factors that can be altered to reduce the elevated morbidity of autoimmune diseases caused by a lower level of education.
The original contributions presented in the study are included in the article/Supplementary Material. Further inquiries can be directed to the corresponding author.
Writing -Original Draft, Methodology, Validation, Visualization: YJL and JZ. Data Collection and Validation: JW, ML, and WL. Conceptualization, Methodology, Supervision, Project Administration, and Funding Acquisition: YZL. All authors contributed to the article and approved the submitted version.
The author(s) declare financial support was received for the research, authorship, and/or publication of this article. This work was supported by the Hunan Provincial Health Committee Foundation of China (no. B202306017169) and Changsha Natural Science Foundation (kq2208314).
The authors express gratitude to the public databases, websites, and software used in the paper. We want to acknowledge the participants and investigators of the FinnGen study. This research has been conducted using data from UK Biobank, a major biomedical database. The authors are grateful to the High-Performance Computing Center of Central South University for partial support of this work.
The authors declare that the research was conducted in the absence of any commercial or financial relationships that could be construed as a potential conflict of interest.
All claims expressed in this article are solely those of the authors and do not necessarily represent those of their affiliated organizations, or those of the publisher, the editors and the reviewers. Any product that may be evaluated in this article, or claim that may be made by its manufacturer, is not guaranteed or endorsed by the publisher.
The Supplementary Material for this article can be found online at: https://www.frontiersin.org/articles/10.3389/fimmu.2023.1249017/full#supplementary-material
1. Wang L, Wang FS, Gershwin ME. Human autoimmune diseases: a comprehensive update. J Intern Med (2015) 278(4):369–95. doi: 10.1111/joim.12395
2. Yu C, Gershwin ME, Chang C. Diagnostic criteria for systemic lupus erythematosus: a critical review. J Autoimmun (2014) 48-49:10–3. doi: 10.1016/j.jaut.2014.01.004
3. Hewagama A, Richardson BA. The genetics and epigenetics of autoimmune diseases. J Autoimmun (2009) 33(1):3–11. doi: 10.1016/j.jaut.2009.03.007
4. Sharif K, Watad A, Bragazzi NL, Lichtbroun M, Amital H, Shoenfeld Y. Physical activity and autoimmune diseases: Get moving and manage the disease. Autoimmun Rev (2018) 17(1):53–72. doi: 10.1016/j.autrev.2017.11.010
5. Miyauchi E, Shimokawa C, Steimle A, Desai MS, Ohno H. The impact of the gut microbiome on extra-intestinal autoimmune diseases. Nat Rev Immunol (2023) 23(1):9–23. doi: 10.1038/s41577-022-00727-y
6. Burgess S, Small DS, Thompson SG. A review of instrumental variable estimators for Mendelian randomization. Stat Methods Med Res (2017) 26(5):2333–55. doi: 10.1177/0962280215597579
7. Carter AR, Sanderson E, Hammerton G, Richmond RC, Davey Smith G, Heron J, et al. Mendelian randomisation for mediation analysis: current methods and challenges for implementation. Eur J Epidemiol (2021) 36(5):465–78. doi: 10.1007/s10654-021-00757-1
8. Schultz WM, Kelli HM, Lisko JC, Varghese T, Shen J, Sandesara P, et al. Socioeconomic status and cardiovascular outcomes: challenges and interventions. Circulation (2018) 137(20):2166–78. doi: 10.1161/CIRCULATIONAHA.117.029652
9. Zeng L, Ntalla I, Kessler T, Kastrati A, Erdmann J, UK Biobank CardioMetabolic Consortium CHD Working Group, et al. Genetically modulated educational attainment and coronary disease risk. Eur Heart J (2019) 40(29):2413–20. doi: 10.1093/eurheartj/ehz328
10. Tesli M, Degerud E, Plana-Ripoll O, Gustavson K, Torvik FA, Ystrom E, et al. Educational attainment and mortality in schizophrenia. Acta Psychiatr Scand (2022) 145(5):481–93. doi: 10.1111/acps.13407
11. Hu Y, Zhang Y, Zhang H, Gao S, Wang L, Wang T, et al. Cognitive performance protects against Alzheimer's disease independently of educational attainment and intelligence. Mol Psychiatry (2022) 27(10):4297–306. doi: 10.1038/s41380-022-01695-4
12. Takasugi T, Tsuji T, Hanazato M, Miyaguni Y, Ojima T, Kondo K. Community-level educational attainment and dementia: a 6-year longitudinal multilevel study in Japan. BMC Geriatr (2021) 21(1):661. doi: 10.1186/s12877-021-02615-x
13. Brown SG 3rd, Cobb CBC, Harvey VM. Racial and ethnic health disparities in dermatology. Dermatol Clin (2023) 41(2):325–33. doi: 10.1016/j.det.2022.10.003
14. Calixto OJ, Anaya JM. Socioeconomic status. The relationship with health and autoimmune diseases. Autoimmun Rev (2014) 13(6):641–54. doi: 10.1016/j.autrev.2013.12.002
15. Williams JN, Drenkard C, Lim SS. The impact of social determinants of health on the presentation, management and outcomes of systemic lupus erythematosus. Rheumatol (Oxford) (2023) 62(Suppl 1):i10–i4. doi: 10.1093/rheumatology/keac613
16. Davies NM, Holmes MV, Davey Smith G. Reading Mendelian randomisation studies: a guide, glossary, and checklist for clinicians. BMJ (2018) 362:k601. doi: 10.1136/bmj.k601
17. Lee JJ, Wedow R, Okbay A, Kong E, Maghzian O, Zacher M, et al. Gene discovery and polygenic prediction from a genome-wide association study of educational attainment in 1.1 million individuals. Nat Genet (2018) 50(8):1112–21. doi: 10.1038/s41588-018-0147-3
18. Trampush JW, Yang ML, Yu J, Knowles E, Davies G, Liewald DC, et al. GWAS meta-analysis reveals novel loci and genetic correlates for general cognitive function: a report from the COGENT consortium. Mol Psychiatry (2017) 22(11):1651–2. doi: 10.1038/mp.2017.197
19. Kurki MI, Karjalainen J, Palta P, Sipilä TP, Kristiansson K, Donner KM, et al. FinnGen provides genetic insights from a well-phenotyped isolated population. Nature (2023) 613(7944):508–18. doi: 10.1038/s41586-022-05473-8
20. van Rheenen W, van der Spek RAA, Bakker MK, van Vugt JJFA, Hop PJ, Zwamborn RAJ, et al. Common and rare variant association analyses in amyotrophic lateral sclerosis identify 15 risk loci with distinct genetic architectures and neuron-specific biology. Nat Genet (2021) 53(12):1636–48. doi: 10.1038/s41588-021-00973-1
21. Valette K, Li Z, Bon-Baret V, Chignon A, Bérubé JC, Eslami A, et al. Prioritization of candidate causal genes for asthma in susceptibility loci derived from UK Biobank. Commun Biol (2021) 4(1):700. doi: 10.1038/s42003-021-02227-6
22. de Lange KM, Moutsianas L, Lee JC, Lamb CA, Luo Y, Kennedy NA, et al. Genome-wide association study implicates immune activation of multiple integrin genes in inflammatory bowel disease. Nat Genet (2017) 49(2):256–61. doi: 10.1038/ng.3760
23. Jiang L, Zheng Z, Fang H, Yang J. A generalized linear mixed model association tool for biobank-scale data. Nat Genet (2021) 53(11):1616–21. doi: 10.1038/s41588-021-00954-4
24. CONSORTIUM I M S G. Multiple sclerosis genomic map implicates peripheral immune cells and microglia in susceptibility. Sci (New York NY) (2019) 365(6460):eaav7188. doi: 10.1126/science.aav7188
25. Cordell HJ, Fryett JJ, Ueno K, Darlay R, Aiba Y, Hitomi Y, et al. An international genome-wide meta-analysis of primary biliary cholangitis: Novel risk loci and candidate drugs. J Hepatol (2021) 75(3):572–81. doi: 10.1016/j.jhep.2021.04.055
26. Ji SG, Juran BD, Mucha S, Folseraas T, Jostins L, Melum E, et al. Genome-wide association study of primary sclerosing cholangitis identifies new risk loci and quantifies the genetic relationship with inflammatory bowel disease. Nat Genet (2017) 49(2):269–73. doi: 10.1038/ng.3745
27. Forgetta V, Manousaki D, Istomine R, Ross S, Tessier MC, Marchand L, et al. Rare genetic variants of large effect influence risk of type 1 diabetes. Diabetes (2020) 69(4):784–95. doi: 10.2337/db19-0831
28. Liu M, Jiang Y, Wedow R, Li Y, Brazel DM, Chen F, et al. Association studies of up to 1.2 million individuals yield new insights into the genetic etiology of tobacco and alcohol use. Nat Genet (2019) 51(2):237–44. doi: 10.1038/s41588-018-0307-5
29. Yengo L, Sidorenko J, Kemper KE, Zheng Z, Wood AR, Weedon MN, et al. Meta-analysis of genome-wide association studies for height and body mass index in ∼700000 individuals of European ancestry. Hum Mol Genet (2018) 27(20):3641–9. doi: 10.1093/hmg/ddy271
30. Sudlow C, Gallacher J, Allen N, Beral V, Burton P, Danesh J, et al. UK biobank: an open access resource for identifying the causes of a wide range of complex diseases of middle and old age. PloS Med (2015) 12(3):e1001779. doi: 10.1371/journal.pmed.1001779
31. Hemani G, Tilling K, Davey Smith G. Orienting the causal relationship between imprecisely measured traits using GWAS summary data. PloS Genet (2017) 13(11):e1007081. doi: 10.1371/journal.pgen.1007081
32. Hemani G, Zheng J, Elsworth B, Wade KH, Haberland V, Baird D, et al. The MR-Base platform supports systematic causal inference across the human phenome. eLife (2018) 7:e34408. doi: 10.7554/eLife.34408
33. Burgess S, Thompson SG, CRP CHD Genetics Collaboration. Avoiding bias from weak instruments in Mendelian randomization studies. Int J Epidemiol (2011) 40(3):755–64. doi: 10.1093/ije/dyr036
34. Bowden J, Davey Smith G, Burgess S. Mendelian randomization with invalid instruments: effect estimation and bias detection through Egger regression. Int J Epidemiol (2015) 44(2):512–25. doi: 10.1093/ije/dyv080
35. Relton CL, Davey Smith G. Two-step epigenetic Mendelian randomization: a strategy for establishing the causal role of epigenetic processes in pathways to disease. Int J Epidemiol (2012) 41(1):161–76. doi: 10.1093/ije/dyr233
36. Burgess S, Butterworth A, Thompson SG. Mendelian randomization analysis with multiple genetic variants using summarized data. Genet Epidemiol (2013) 37(7):658–65. doi: 10.1002/gepi.21758
37. Gusev A, Ko A, Shi H, Bhatia G, Chung W, Penninx BW, et al. Integrative approaches for large-scale transcriptome-wide association studies. Nat Genet (2016) 48(3):245–52. doi: 10.1038/ng.3506
38. CONSORTIUM G. The GTEx Consortium atlas of genetic regulatory effects across human tissues. Sci (New York NY) (2020) 369(6509):1318–30. doi: 10.1126/science.aaz1776
39. Thomas PD, Ebert D, Muruganujan A, Mushayahama T, Albou LP, Mi H. PANTHER: Making genome-scale phylogenetics accessible to all. Protein Sci Publ Protein Soc (2022) 31(1):8–22. doi: 10.1002/pro.4218
40. Clusterprofiler: An R Package for Comparing Biological Themes among Gene Clusters. OMICS (2012) 16(5):284–7. doi: 10.1089/omi.2011.0118
41. Yu G, Wang L-G, Yan G-R, He Y-Q. DOSE: an R/Bioconductor package for disease ontology semantic and enrichment analysis. Bioinformatics (2014) 31(4):608–9. doi: 10.1093/bioinformatics/btu684
42. Ashburner M, Ball CA, Blake JA, Botstein D, Butler H, Cherry JM, et al. Gene ontology: tool for the unification of biology. The Gene Ontology Consortium. Nat Genet (2000) 25(1):25–9. doi: 10.1038/75556
43. Gene Ontology Consortium, Aleksander SA, Balhoff J, Carbon S, Cherry JM, Drabkin HJ, et al. The gene ontology knowledgebase in 2023. Genetics (2023) 224(1):iyad031. doi: 10.1093/genetics/iyad031
44. Bowden J, Del Greco MF, Minelli C, Zhao Q, Lawlor DA, Sheehan NA, et al. Improving the accuracy of two-sample summary-data Mendelian randomization: moving beyond the NOME assumption. Int J Epidemiol (2019) 48(3):728–42. doi: 10.1093/ije/dyy258
45. Zhou HZY, Liu J, Yang Y, Fang W, Hong S, Chen G, et al. Education and lung cancer: a Mendelian randomization study. Int J Epidemiol (2019) 48(3):743–50. doi: 10.1093/ije/dyz121
46. Schunkert HEJ, Samani NJ, Deloukas P, Z Eng L. Genetics of educational attainment and coronary risk in Mendelian randomization studies. Eur Heart J (2020) 41(7):894–5. doi: 10.1093/eurheartj/ehz946
47. Carter Ar GD, Davies NM, Taylor AE, Tillmann T, Vaucher J, Wootton RE, et al. Understanding the consequences of education inequality on cardiovascular disease: mendelian randomisation study. BMJ (Clin Res ed) (2019) 365:l1855. doi: 10.1136/bmj.l1855
48. Groot J, Nybo Andersen AM, Adam A, Tind Nielsen TE, Blegvad C, Skov L. Associations between maternal socioeconomic position and psoriasis: a cohort study among the offspring of the Danish National Birth Cohort. Br J Dermatol (2019) 180(2):321–8. doi: 10.1111/bjd.17091
49. Portaccio E, Simone M, Prestipino E, Bellinvia A, Pastò L, Niccolai M, et al. Cognitive reserve is a determinant of social and occupational attainment in patients with pediatric and adult onset multiple sclerosis. Multiple Sclerosis Related Disord (2020) 42:102145. doi: 10.1016/j.msard.2020.102145
50. Rimkus CM, Avolio IMB, Miotto EC, Pereira SA, Mendes MF, Callegaro D, et al. The protective effects of high-education levels on cognition in different stages of multiple sclerosis. Multiple Sclerosis Related Disord (2018) 22:41–8. doi: 10.1016/j.msard.2018.03.001
51. Davies Nm HW, Anderson EL, Sanderson E, Deary IJ, Davey Smith G. Multivariable two-sample Mendelian randomization estimates of the effects of intelligence and education on health. eLife (2019) 8:e43990. doi: 10.7554/eLife.43990
52. Ferguson LD, Brown R, Celis-Morales C, Welsh P, Lyall DM, Pell JP, et al. Association of central adiposity with psoriasis, psoriatic arthritis and rheumatoid arthritis: a cross-sectional study of the UK Biobank. Rheumatol (Oxford) (2019) 58(12):2137–42. doi: 10.1093/rheumatology/kez192
53. Zhao SSHM, Zheng J, Sanderson E, Carter AR. The impact of education inequality on rheumatoid arthritis risk is mediated by smoking and body mass index: Mendelian randomization study. Rheumatol (Oxford) (2022) 61(5):2167–75. doi: 10.1093/rheumatology/keab654
54. Bae SCLY. Alcohol intake and risk of systemic lupus erythematosus: a Mendelian randomization study. Lupus (2019) 28(2):174–80. doi: 10.1177/0961203318817832
55. Wei J, Zhu J, Xu H, Zhou D, Elder JT, Tsoi LC, et al. Alcohol consumption and smoking in relation to psoriasis: a Mendelian randomization study. Br J Dermatol (2022) 187(5):684–91. doi: 10.1111/bjd.21718
56. Rosso M, Chitnis T. Association between cigarette smoking and multiple sclerosis: A review. JAMA Neurol (2020) 77(2):245–53. doi: 10.1001/jamaneurol.2019.4271
57. Deane KD, Holers VM. Rheumatoid arthritis pathogenesis, prediction, and prevention: an emerging paradigm shift. Arthritis Rheumatol (Hoboken NJ) (2021) 73(2):181–93. doi: 10.1002/art.41417
58. Hussain MS, Tripathi V. Smoking under hypoxic conditions: a potent environmental risk factor for inflammatory and autoimmune diseases. Military Med Res (2018) 5(1):11. doi: 10.1186/s40779-018-0158-5
59. Versini M, Jeandel PY, Rosenthal E, Shoenfeld Y. Obesity in autoimmune diseases: not a passive bystander. Autoimmun Rev (2014) 13(9):981–1000. doi: 10.1016/j.autrev.2014.07.001
60. Tsigalou C, Vallianou N, Dalamaga M. Autoantibody production in obesity: is there evidence for a link between obesity and autoimmunity? Curr Obes Rep (2020) 9(3):245–54. doi: 10.1007/s13679-020-00397-8
61. Forno E, Han YY, Libman IM, Muzumdar RH, Celedón JC. Adiposity and asthma in a nationwide study of children and adults in the United States. Ann Am Thorac Soc (2018) 15(3):322–30. doi: 10.1513/AnnalsATS.201709-723OC
62. Ye Y, Gaugler B, Mohty M, Malard F. Old dog, new trick: Trivalent arsenic as an immunomodulatory drug. Br J Pharmacol (2020) 177(10):2199–214. doi: 10.1111/bph.15011
63. Argyriou K, Samuel S, Moran GW. Acetarsol in the management of mesalazine-refractory ulcerative proctitis: a tertiary-level care experience. Eur J Gastroenterol Hepatol (2019) 31(2):183–6. doi: 10.1097/MEG.0000000000001326
64. Haque R, Chaudhary A, Sadaf N. Immunomodulatory role of arsenic in regulatory T cells. Endocr Metab Immune Disord Drug Targets (2017) 17(3):176–81. doi: 10.2174/1871530317666170818114454
65. Weyand CM, Goronzy JJ. Immunometabolism in the development of rheumatoid arthritis. Immunol Rev (2020) 294(1):177–87. doi: 10.1111/imr.12838
66. Wójcik P, Gęgotek A, Žarković N, Skrzydlewska E. Oxidative stress and lipid mediators modulate immune cell functions in autoimmune diseases. Int J Mol Sci (2021) 22(2):723. doi: 10.3390/ijms22020723
67. Shou Y, Yang L, Yang Y, Xu J. Inhibition of keratinocyte ferroptosis suppresses psoriatic inflammation. Cell Death Dis (2021) 12(11):1009. doi: 10.1038/s41419-021-04284-5
68. Wang Y, Xiang YY, Sugihara J, Lu WY, Liao XH, Arvan P, et al. XB130 plays an essential role in folliculogenesis through mediating interactions between microfilament and microtubule systems in thyrocytes. Thyroid Off J Am Thyroid Assoc (2022) 32(2):128–37. doi: 10.1089/thy.2021.0461
69. El-Matary W, Dufault B, Moroz SP, Schellenberg J, Bernstein CN. Education, employment, income, and marital status among adults diagnosed with inflammatory bowel diseases during childhood or adolescence. Clin Gastroenterol Hepatol Off Clin Pract J Am Gastroenterol Assoc (2017) 15(4):518–24. doi: 10.1016/j.cgh.2016.09.146
70. Warren RB, Kleyn CE, Gulliver WP. Cumulative life course impairment in psoriasis: patient perception of disease-related impairment throughout the life course. Br J Dermatol (2011) 164Suppl1:1–14. doi: 10.1111/j.1365-2133.2011.10280.x
71. Lavoie L, Dupéré V, Dion E, Crosnoe R, Lacourse É, Archambault I. Gender differences in adolescents' Exposure to stressful life events and differential links to impaired school functioning. J Abnorm Child Psychol (2019) 47(6):1053–64. doi: 10.1007/s10802-018-00511-4
72. Bukhari M. The influence of low educational attainment on progress in rheumatoid arthritis: nature or nurture? Rheumatol (Oxford) (2023) 62(5):1736–7. doi: 10.1093/rheumatology/keac615
73. Sanderson E, Richardson TG, Hemani G, Davey Smith G. The use of negative control outcomes in Mendelian randomization to detect potential population stratification. Int J Epidemiol (2021) 50(4):1350–61. doi: 10.1093/ije/dyaa288
74. Brumpton B, Sanderson E, Heilbron K, Hartwig FP, Harrison S, Vie GÅ, et al. Avoiding dynastic, assortative mating, and population stratification biases in Mendelian randomization through within-family analyses. Nat Commun (2020) 11(1):3519. doi: 10.1038/s41467-020-17117-4
75. Conrad N, Misra S, Verbakel JY, Verbeke G, Molenberghs G, Taylor PN, et al. Incidence, prevalence, and co-occurrence of autoimmune disorders over time and by age, sex, and socioeconomic status: a population-based cohort study of 22 million individuals in the UK. Lancet (London England) (2023) 401(10391):1878–90. doi: 10.1136/annrheumdis-2023-eular.4269
Keywords: Mendelian randomization, education, autoimmune diseases, causal relationship, Transcriptome-wide association study
Citation: Li Y, Zhang J, Wen J, Liu M, Liu W and Li Y (2023) Large-scale genome-wide association study to identify causal relationships and potential mediators between education and autoimmune diseases. Front. Immunol. 14:1249017. doi: 10.3389/fimmu.2023.1249017
Received: 28 June 2023; Accepted: 08 November 2023;
Published: 07 December 2023.
Edited by:
Kerstin Klein, University Hospital Bern, SwitzerlandReviewed by:
Du Liping, Chongqing Eye Institute, ChinaCopyright © 2023 Li, Zhang, Wen, Liu, Liu and Li. This is an open-access article distributed under the terms of the Creative Commons Attribution License (CC BY). The use, distribution or reproduction in other forums is permitted, provided the original author(s) and the copyright owner(s) are credited and that the original publication in this journal is cited, in accordance with accepted academic practice. No use, distribution or reproduction is permitted which does not comply with these terms.
*Correspondence: Yongzhen Li, NTA3MjcxQGNzdS5lZHUuY24=
†These authors have contributed equally to this work
Disclaimer: All claims expressed in this article are solely those of the authors and do not necessarily represent those of their affiliated organizations, or those of the publisher, the editors and the reviewers. Any product that may be evaluated in this article or claim that may be made by its manufacturer is not guaranteed or endorsed by the publisher.
Research integrity at Frontiers
Learn more about the work of our research integrity team to safeguard the quality of each article we publish.