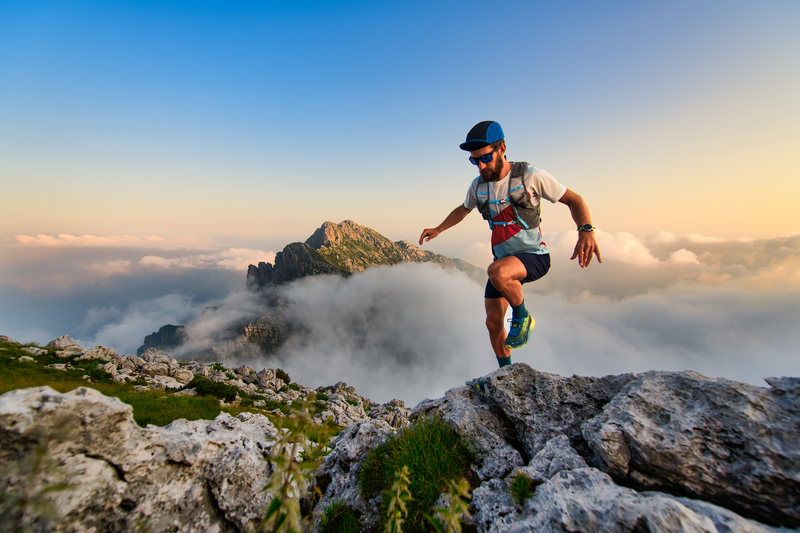
94% of researchers rate our articles as excellent or good
Learn more about the work of our research integrity team to safeguard the quality of each article we publish.
Find out more
ORIGINAL RESEARCH article
Front. Immunol. , 08 January 2024
Sec. Viral Immunology
Volume 14 - 2023 | https://doi.org/10.3389/fimmu.2023.1243450
Background: The extensive spread of coronavirus disease 2019 (COVID-19) has led to a rapid increase in global mortality. Preeclampsia is a commonly observed pregnancy ailment characterized by high maternal morbidity and mortality rates, in addition to the restriction of fetal growth within the uterine environment. Pregnant individuals afflicted with vascular disorders, including preeclampsia, exhibit an increased susceptibility to severe acute respiratory syndrome coronavirus 2 (SARS-CoV-2) infection via mechanisms that have not been fully delineated. Additionally, the intricate molecular mechanisms underlying preeclampsia and COVID-19 have not been fully elucidated. This study aimed to discern commonalities in gene expression, regulators, and pathways shared between COVID-19 and preeclampsia. The objective was to uncover potential insights that could contribute to novel treatment strategies for both COVID-19 and preeclampsia.
Method: Transcriptomic datasets for COVID-19 peripheral blood (GSE152418) and preeclampsia blood (GSE48424) were initially sourced from the Gene Expression Omnibus (GEO) database. Subsequent to that, we conducted a subanalysis by selecting females from the GSE152418 dataset and employed the “Deseq2” package to identify genes that exhibited differential expression. Simultaneously, the “limma” package was applied to identify differentially expressed genes (DEGs) in the preeclampsia dataset (GSE48424). Following that, an intersection analysis was conducted to identify the common DEGs obtained from both the COVID-19 and preeclampsia datasets. The identified shared DEGs were subsequently utilized for functional enrichment analysis, transcription factor (TF) and microRNAs (miRNA) prediction, pathway analysis, and identification of potential candidate drugs. Finally, to validate the bioinformatics findings, we collected peripheral blood mononuclear cell (PBMC) samples from healthy individuals, COVID-19 patients, and Preeclampsia patients. The abundance of the top 10 Hub genes in both diseases was assessed using real-time quantitative polymerase chain reaction (RT-qPCR).
Result: A total of 355 overlapping DEGs were identified in both preeclampsia and COVID-19 datasets. Subsequent ontological analysis, encompassing Gene Ontology (GO) functional assessment and Kyoto Encyclopedia of Genes and Genomes (KEGG) pathway analysis, revealed a significant association between the two conditions. Protein-protein interactions (PPIs) were constructed using the STRING database. Additionally, the top 10 hub genes (MRPL11, MRPS12, UQCRH, ATP5I, UQCRQ, ATP5D, COX6B1, ATP5O, ATP5H, NDUFA6) were selected based on their ranking scores using the degree algorithm, which considered the shared DEGs. Moreover, transcription factor-gene interactions, protein-drug interactions, co-regulatory networks of DEGs and miRNAs, and protein-drug interactions involving the shared DEGs were also identified in the datasets. Finally, RT-PCR results confirmed that 10 hub genes do exhibit distinct expression profiles in the two diseases.
Conclusion: This study successfully identified overlapping DEGs, functional pathways, and regulatory elements between COVID-19 and preeclampsia. The findings provide valuable insights into the shared molecular mechanisms and potential therapeutic targets for both diseases. The validation through RT-qPCR further supports the distinct expression profiles of the identified hub genes in COVID-19 and preeclampsia, emphasizing their potential roles as biomarkers or therapeutic targets in these conditions.
The global outbreak of COVID-19 can be attributed to the emergence of the SARS-CoV-2 virus, and has caused unprecedented health consequences across the world (1). SARS-CoV-2 infection occurs through exposure to respiratory aerosols and droplets (2–4). The virus is capable of inducing direct endothelial injury, thrombo-inflammation, immune system dysregulation, and changes in ACE2-associated pathways (5–8). Severe COVID-19 is typically characterized by pulmonary infection accompanied with cough, fever and dyspnoea (9). The most severe pathophysiologic symptoms of COVID-19 include the damage of pulmonary epithelia, hypercoagulation, thrombosis and excessive vascular permeability resulting into sepsis (10).
Preeclampsia, an ailment associated with pregnancy that commonly presents around the 20-week gestation mark, and is symptomized by placental oxidative stress, endothelial damage and antiangiogenesis that induce proteinuria, hypertension, and similar multiorgan responses as observed in severe COVID-19 cases (11–14). It has been reported in recent studies that there is a strong connection of COVID-19 with preeclampsia in pregnant patients (15, 16). The infection caused by SARS-CoV-2 during pregnancies can induce risks for both the mother and the developing fetus, contributing to pregnancy-related complications like preeclampsia and impaired intrauterine growth (17–26). Several studies have indicated an elevated likelihood of perinatal outcomes in pregnant individuals with COVID-19, including more proneness to preeclampsia and premature parturition (27). It is thus important to assess the impact of COVID-19 on the preeclampsia patients, and identify potential therapeutic interventions to lower the likelihood of hospitalization or mortality.
Through the analysis of blood RNA sequencing data from individuals with COVID-19 and preeclampsia, this study reveals shared DEGs. Moreover, to validate our bioinformatics findings, we performed experimental validation using PBMC samples obtained from healthy individuals, COVID-19 patients, and preeclampsia patients. Our findings further reinforce the potential of the identified molecules in serving as therapeutic targets for both COVID-19 and preeclampsia, providing valuable insights for the development of effective treatments.
Within the GEO database (https://www.ncbi.nlm.nih.gov/geo/), we accessed the expression profiles for this study. The COVID-19 datasets (GSE152418) involved RNA-Seq profiling of peripheral blood samples from 17 COVID-19 patients and 17 healthy subjects (28). Furthermore, the preeclampsia dataset (GSE48424) consisted of whole-blood RNA-seq data from 18 preeclampsia patients and 18 healthy individuals (29).
First, female patients were selected from the GSE48424, including 9 COVID-19 patients and 9 healthy subjects. To identify DEGs in the GSE152418 dataset, we utilized the R package “Deseq2” and applied a significance threshold of padj = 0.05 and |log2 Fold-Change| = 0.5 (30). Additionally, for the GSE48424 dataset, we employed the R package “limma” and identified DEGs using criteria of |log2 Fold-Change| = 0.3 and P-value = 0.05 (31).
Through GO enrichment and KEGG pathway analyses conducted with the “clusterProfiler” R package, we determined the noteworthy functions and pathways linked to the DEGs, considering a threshold of P-value = 0.05 (32).
The STRING database (www.string-db.org) was employed to analyze and integrate the DEGs, resulting in the construction of a PPI network with a median confidence score of 0.7 (33). Subsequently, Cytoscape software was utilized for visualization and subsequent analysis of the network (34).
CytoHubba, an essential plug-in embedded within Cytoscape, provides a comprehensive platform for assessing and identifying the influential modulators of biological networks, harnessing the potential of network metrics (35). CytoHubba was instrumental in examining and scrutinizing the significant nodes present in the modules of the PPI network, thereby uncovering the hub genes (36). By employing the Degree algorithm, the ten genes demonstrating the greatest significance were identified, and their rankings were visually illustrated in plots featuring a gradual change in colors from purple to pink. The hub genes were systematically ranked according to the shortest accessible paths connecting them, facilitating a more accessible and comprehensible visualization of their interrelationships.
Transcription factors (TFs) exert control over transcription and chromatin structure by binding to specific DNA sequences. They form a complex expression-control system within the genome, and is thus crucial for the understanding of involved molecular mechanisms (37). Enrichr operates as a robust gene set search platform that consolidates a broad range of biological information for advanced analysis (38). Common DEGs were imported into Enrichr to infer complete chart of TFs. The top 10 TFs were then selected according to their composite scores. MiRTarBase was used for identifying miRNAs that could interact with the common DEGs via the network analysis tool (39). In addition, the miRTarBase component within Enrichr was performed to explore gene-miRNA connections. Finally, Cytoscape was implemented to exhibit TF-gene and miRNA-gene interactions.
DisGeNET (http://www.disgenet.org/) is a comprehensive database that connects genes to human disorders (40). DisGeNET was used to perform gene-disease correlation analysis and visualization using Cytoscape.
The analysis encompassed an assessment of promising pharmaceutical agents for the management of COVID-19 and preeclampsia. DSigDB is a novel gene-set repository that connect drugs/medicines to target genes for further enrichment analysis (41). A meticulous evaluation resulted in the identification of a set of 10 highly promising candidates, paving the way for subsequent analytical investigations. PubChem, accessible at (https://pubchem.ncbi.nlm.nih.gov), serves as a comprehensive repository housing extensive data regarding chemical compounds and their associated biological functions, enabling the collaborative exchange, examination, and integration of information from diverse databases. Molecular compositions and two-dimensional arrangements of prospective medications were obtained from PubChem to facilitate pharmaceutical research.
We collected peripheral blood cell samples obtained from four distinct groups, including 30 individuals in each group: healthy individuals, COVID-19 patients, Preeclampsia patients, and pregnant women without any complications. Total RNA was extracted from the peripheral blood cell samples using TRIzol reagent (Vazyme, Cat: R401-01). Subsequently, reverse transcription was performed to synthesize complementary DNA (cDNA) using a reverse transcription kit (Vazyme, Cat: R211-01). RT-PCR was then employed to quantitatively measure the expression levels of the top 10 hub genes identified from the bioinformatics analysis. To ensure data normalization, the expression levels of target genes were normalized against GAPDH as an internal reference. The sequence of primers are shown in Supplementary Table 1.
In this study, transcriptomic datasets for COVID-19 and preeclampsia were acquired from the Gene Expression Omnibus database. Utilizing the “Deseq2” and “limma” packages, differential gene expression analysis identified significant genes with adjusted p-values < 0.05. An intersection analysis revealed common differentially expressed genes through hypergeometric testing. Functional enrichment analysis, including Gene Ontology and Kyoto Encyclopedia of Genes and Genomes pathways, was performed on identified genes. Protein-protein interaction networks were constructed using the STRING database, and the top 10 hub genes were determined based on degree algorithm ranking scores. Experimental validation through RT-qPCR on peripheral blood mononuclear cell samples from healthy individuals, COVID-19 patients, and preeclampsia patients confirmed distinct gene expression profiles. The expression levels of the specified genes were evaluated through RT–qPCR, and the data are reported as the mean ± standard error of the mean (s.e.m.). Statistical significance was determined using a one-way analysis of variance (ANOVA), with * denoting p < 0.05, ** indicating p < 0.01, and *** representing p < 0.001.
The critical procedures executed in this research were shown in the flowchart (Figure 1). The peripheral blood expression profile was downloaded from GEO, and the genetic elements that could initiate COVID-19 and preeclampsia were identified to examine the interconnectedness between preeclampsia and COVID-19 as well as their effects. First, an amalgamation of 3724 DEGs associated with COVID-19 was identified, consisting of 2682 upregulated genes and 1042 downregulated genes (Supplementary Table 2). Within the scope of COVID-19, a combined total of 3724 DEGs was identified, consisting of 2682 genes showing upregulated expression and 1042 genes displaying downregulated expression (Supplementary Table 3). The integration of DEGs from both datasets led to the identification of 355 DEGs that were concordantly present (Figure 2; Supplementary Table 4). The results demonstrated a plausible convergence between the fundamental processes of preeclampsia and COVID-19.
Figure 2 DEGs and common DEGs of the two datasets were visualized between COVID-19 (GSE152418) and preeclampsia (GSE48424). (A) Volcano plot of DEGs in the preeclampsia database (GSE48424). Blue represents the downregulated genes, red represents the upregulated genes, and gray represents the rest of DEGs. (B) Volcano plot of DEGs in COVID-19 database (GSE152418). Blue represents the downregulated genes, red represents the upregulated genes, and gray represents the rest of DEGs. (C) Comparison of the number of DEGs between COVID-19 and preeclampsia. (D) Venn diagram visualizing the number of common DEGs between COVID-19 and Preeclampsia.
To elucidate the underlying biological functions and enriched pathways linked to these intersecting DEGs, assessments were executed utilizing the “clusterProfiler” algorithm (Figure 3A). The GO analysis demonstrated that “aerobic electron transport chain”, “ATP synthesis coupled electron transport”, “mitochondrial ATP synthesis coupled electron transport”, “oxidative phosphorylation”, and “aerobic respiration” in biological process, “mitochondrial protein-containing complex”, “mitochondrial inner membrane”, “inner mitochondrial membrane protein complex”, “respiratory chain complex”, and “respirasome” in cellular component, “oxidoreduction-driven active transmembrane transporter activity”, “electron transfer activity” in molecular function were most significantly involved in the effects of DEGs approach between preeclampsia and COVID-19. The circular representation of enrichment results featured the outer layer denoting the notably enriched pathways, and the inner layer provided details regarding the statistical significance and abundance of genes demonstrating enrichment (Figure 3B). The KEGG pathway analysis can be used to analyze the mutual interactions between different diseases via key biological or molecular processes. It was revealed in this study that “ATP metabolic process”, “energy derivation by oxidation of organic compounds”, “aerobic respiration”, “cellular respiration”, and “positive regulation of ion transport” in shaping the pathogenic mechanisms shared by preeclampsia and COVID-19 (Figure 3C). Within the circular visualization of KEGG enrichment analysis, the outer layer portrayed the notably enriched pathway, while the innermost layer denoted the P value and quantity of enriched genes (Figure 3D). The circular visualizations underscored the significance of enriched pathways and detailed the statistical significance and gene abundance, offering a comprehensive understanding of the molecular underpinnings connecting these two conditions.
Figure 3 GO and KEGG functional enrichment analysis of the common DEGs between COVID-19 and preeclampsia. (A) GO enrichment analysis histogram. BP, biological process; CC, cellular components; MF, molecular function. (B) Circle diagram of common DEGs from GO enrichment analysis. The ID of the pathway is indicated on the outermost layer, and P values and gene enrichment numbers are indicated on the innermost layer. (C) Bubble graph for KEGG enrichment in differential genes. The larger bubble represents enrichment of more genes, and the increasing depth of red color represents more significant differences. (D) Circle diagram of common DEGs from KEGG enrichment analysis.
The overlapping DEGs from COVID-19 and preeclampsia datasets were subjected to STRING analysis to reveal protein-protein interactions (PPIs) and determine hub genes (Figure 4). By utilizing the Degree algorithm, the top ten genes of remarkable significance were unveiled, assuming pivotal roles as central nodes with exceptional prominence and regulatory functions. These genes include MRPL11, MRPS12, UQCRH, ATP5I, UQCRQ, ATP5D, COX6B1, ATP5O, ATP5H, and NDUFA6 (Figure 5). Notably, among these genes, MRPL11 stands out with the highest node count, hinting at its potential to play a pivotal role, given its extensive connectivity within the network. The diagnostic utility of the identified hub genes was examined by conducting ROC analysis for preeclampsia and COVID-19. The AUC values of almost all hub genes were above 0.7 in both pre-eclampsia and COVID-19, highlighting their exceptional prognostic potential in both disease cohorts (Supplementary Figures 1, 2). The findings shed light on the shared genetic mechanisms and offer insights for potential therapeutic strategies in these two diseases.
Figure 4 The PPI network of common DEGs between COVID-19 and preeclampsia. The circular node represents DEGs and the edge represents the interaction between nodes.
Figure 5 Top 10 hub genes in the PPI network identified based on their scores. In the circle, red stands for the identified top 10 hub genes.
To identify major transcriptional variations and better understand critical regulatory molecules related to shared DEGs, a web-dependent methodology was utilized to elucidate the governing TFs and miRNAs. The interaction of regulatory TFs and regulatory miRNA factors with common DEGs was respectively displayed in Figures 6 and 7. Our results indicated the existence of potential connections between common DEGs and TFs or miRNA (Supplementary Tables 5, 6). These results facilitate further investigations into the specific roles and functions of TFs and miRNAs in the context of the shared DEGs, ultimately advancing our understanding of the biological mechanisms and potential therapeutic targets in the studied diseases.
Figure 6 Top 10 transcription factors (TFs) ranked according to P values and their interactions with common DEGs. In this network, the red diamonds represent the top 10TFs with lowest P values. Other colored circles represent common DEGs correlated with TFs.
Figure 7 Interconnected regulatory interaction network of the top 10 microRNAs with lowest P values. In the middle circle, the orange circles represent common DEGs correlated with miRNAs.
In the pursuit of identifying potential drugs targeting shared DEGs in preeclampsia and COVID-19, a screening process utilizing Enrichr was employed. Transcriptional profiles sourced from DSigDB were utilized to discern ten promising molecules. The selection of the top ten compounds was based on their P-values, indicative of their potential therapeutic significance. Supplementary Figure 3 showcases the names, chemical formulas, and 2D structures of these drugs. Comprehensive results of all Drug Candidates can be found in Supplementary Table 7. These results provided valuable insights and resources for further exploration and potential drug development in the context of these diseases.
Overlapping genetic factors contribute to the interconnectedness of different diseases (42). Upon uploading the shared DEGs of COVID-19 and preeclampsia to DisGeNET for analysis, potential connections emerged between these diseases and various conditions, such as Renal Fibrosis, Ureteral Obstruction, Colonic Neoplasms, Idiopathic Nephrotic Syndrome, IgA Glomerulonephritis, Burkitt Lymphoma, Medullary Neoplasms, Neuroblastoma, Congenital Aneurysm of Ascending Aorta, and Mitochondrial Diseases (Supplementary Figure 4, Supplementary Table 8). These findings highlight the interplay and shared genetic factors between preeclampsia, COVID-19, and other related diseases, providing valuable insights for further research and a deeper understanding of their pathogenesis and potential therapeutic strategies.
To establish the credibility of hub genes as potential biomarkers in COVID-19 and Preeclampsia, PBMC were gathered from four distinct populations, including female patients with COVID-19, healthy females, patients with Preeclampsia, and healthy pregnant women. The RT-PCR analysis of the PBMC samples revealed significant differences in mRNA abundance of the hub genes (MRPL11, MRPS12, UQCRH, ATP5I, UQCRQ, ATP5D, COX6B1, ATP5O, ATP5H, and NDUFA6) between COVID-19 and Preeclampsia cohorts, respectively (Figure 8). Consistent with the bioinformatics analysis, these hub genes exhibited distinct expression patterns. Notably, PBMC samples from healthy individuals displayed differential expression profiles compared to patients diagnosed with COVID-19 and Preeclampsia, suggesting the prospective applicability of the genes as biomarkers for assessing the presence and progression of both conditions. The distinct expression patterns of these hub genes align with the bioinformatics analysis, suggesting their prospective applicability as reliable biomarkers for assessing the presence and progression of both conditions, providing valuable insights for diagnostic and monitoring purposes.
Figure 8 Comparison of HUB gene expression levels in PBMCs from healthy individuals, patients with COVID-19, and patients with Preeclampsia. Expressions of MRPL1 (A), DNUFA6 (B), ATP5H (C), ATP5O (D), COX6B1 (E), ATP5D (F), UQCRQ (G), ATP5I (H), UQCRH (I) and MRPS12 (J) in healthy individuals, patients with COVID-19, and patients with Preeclampsia. The bar graph depicts the expression levels of HUB genes, with black bars representing healthy individuals, red bars representing COVID-19 patients, and green bars representing Preeclampsia patients. Error bars indicate the standard error of the mean. One-way ANOVA was employed for statistical analysis, and each group comprised 30 individuals. The asterisks on the bar graph indicate the significance of the differences between each group and the healthy group. Significance levels are denoted as follows: p < 0.05, *; p < 0.01, **; p < 0.001, ***.
It has been demonstrated by more and more recent studies that plausible associations could exist between distinct medical conditions. Identification of these associations can be a promising step for future disease studies (43–45). Public health systems are facing unprecedented challenges due to the COVID-19 pandemic. In spite of being primarily deemed as a respiratory complication, infection with SARS-CoV-2 has the potential to influence on endothelial cells, resulting into microvascular dysfunction, microthrombosis and endotheliitis (46). It has been shown recently that preeclampsia patients are more prone to SARS-CoV-2 infection and high COVID-19 mortality than healthy populations (18, 47–49). Instead of immunosuppression observed during normal pregnancies, preeclampsia patients shows aberrant and excessive activation of immune system, characterized by an upregulation of antiangiogenic factors and proinflammatory cytokines in both the maternal endothelia and intrauterine environment, inducing placental insufficiency and systemic complication (50, 51). However, it is still unclear what molecular mechanism contributes to a worse COVID-19 prognosis among preeclampsia patients. Inspired by the finding of possible correlation of Coronavirus infection and an increased risk of preeclampsia during gestation, we investigated the possible connections between preeclampsia and COVID-19 at the transcriptomic level. A network-centric strategy was utilized for analyzing the RNA-seq profiling datasets for both preeclampsia and COVID-19, and for identifying the potential diagnostic biomarkers for preeclampsia among COVID-19 pregnancies.
MRPL11 is a coding gene, and has connection with diseases such as Dyskinetic Cerebral Palsy (52). MRPS12 is located cytogenetically on 19q13.2, and responsible for encoding a 28S ribosomal subunit of S12P family. It has been reported in previous studies that MRPS12 is a critical component of small ribosomal subunits, and regulates the fidelity of decoding and the vulnerability to aminoglycosidic antibiotics (53, 54). An upregulation of MRPS12 is observed in different types of cancer as opposed to non-tumor controls (55). UQCRH is a vital element of the multisubunit transmembrane complex within the mitochondrial electron transport chain, facilitating the movement of electrons from cytochrome c to c1 (56). UQCRH can serve as an indicative marker for assessing the prognosis of liver cancer. Alteration in mitochondrial respiration could be conducive to tumorigenic processes through aberrant biological stress, such as reactive oxygen species (57). ATP5I assists in forming the structure of ATP-generating enzyme, correlating with oxidative phosphorylation in the mitochondria (58). UQCRQ mutation can lead to deficiency of mitochondrial complex III, inducing neurodegeneration characterized by psychomotor retardation or encephalopathy (59, 60). ATP5D is a subunit of ATP synthase, and its knockdown can lead to reduction in the ATP synthase level (61–63). COX6B1 is a subunit of COX complex, and is present in various types of cells, such as HeLa cells and yeast (64). In addition, recent research has reported that COX6B1 dysregulation could have significant effect on the COX functions, possibly resulting into the development of hydrocephalus, cerebromyopathy and other disorders (65, 66). The ATP5O expression has been reported to play critical roles in diagnosing and prognosing gastric cancer, and the analysis based on NextBio database reveals a downregulation of ATP5O expression in ccRCC (67, 68). ATP5H serves as an essential part of mitochondria responsible for energy production in eukaryotes; therefore, it is reasonable to expect dysregulation of ATP synthase expression in cancer cells would affect tumoral metabolism (69). The NDUFA6 protein is a subunit of NADH dehydrogenase (ubiquinone), the largest of five electron transport chain complexes localized within the internal membrane of mitochondria.
It has been reported that binding between the SARS-CoV-2 and ACE2 receptor could reduce the angiotensin 1-7 level, and induce vasoconstrictive, pro-inflammatory, and pro-coagulant effects, potentially resulting into vascular lesions in the placenta and preeclampsia (46, 70–72). Based on these data, it is of urgent need to perform mechanistic studies to better understand the infection of COVID-19 associated with preeclampsia. GO and KEGG analysis was performed in this study for identifying the connection between preeclampsia and COVID-19. clusterProfiler was used for GO analysis of BPs, CCs, and MFs. The MFs for these common DEGs primarily showed enrichment in the mitochondrial protein-containing complexes, inner membranes of mitochondria, and mitochondrial inner membrane protein complexes. The pathological and biochemical effects induced by COVID-19 could result into an acute inflammatory state (73). Such inflammatory conditions might have associations with hypermetabolic status, e.g., hyperglycemia, and with cellular dysregulation, e.g., mitochondrial dysfunction, considering their involvement in cellular functions and metabolic pathways (74, 75). Therefore, it is essential to analyze the roles played by cellular apparatus and molecules that have direct links with oxidative-stress regulations (73, 76). Identification of significant GO and molecular pathways could help us better understand the mechanism by which preeclampsia increases the COVID-19 mortality rate.
The links between TFs, miRNA, and common DEGs were then investigated. TFs are critical cellular expression-controlling factors. The activities of TFs dictate the cellular functions and responses to environments (77). TFs are also critical cancer-cell stemness enablers, providing support for maintenance and functions of cancer stem cells which are deemed as sources for tumoral development and metastasis, as well as drug resistance (78). In this study, ILF3, HTT, AR, MYC, RARA, IRF4, RAD21, JUN, SREBF1, and JUND were ranked and identified as the top 10 TFs based on the P-values. miRNAs could serve as both tumor suppressors and oncogenes depending on cellular conditions. miRNA could function as a down-regulator of mRNAs by competitively forming base-pairs with them (79). miRNA dysregulation has been reported to affect cancer phenotypes, including proliferative signaling activation, growth suppression evasion, apoptosis suppression, enhanced cellular invasion and metastasis, and increase of angiogenic activities (80). Hsa-miR-126-3p, hsa-miR-32-3p, hsa-miR-192-5p, hsa-miR-215-5p, hsa-miR-380-3p, hsa-miR-9-3p, hsa-miR-4666a-3p, hsa-miR-381-3p, hsa-miR-34a-5p, and hsa-miR-5692b were among the ten most significant miRNAs.
Furthermore, gene-disease relationship analysis was performed for the identification of common DEGs linked to diseases. It was revealed by the analysis that common DEGs are associated with various types of diseases including HIV and COVID-19, including Renal fibrosis, Ureteral obstruction, Colonic Neoplasms, Idiopathic Nephrotic Syndrome, IGA Glomerulonephritis, Burkitt Lymphoma, Medullary Neoplasms, Neuroblastoma, Congenital aneurysm of ascending aorta, and Mitochondrial Diseases. It has been suggested by recent studies that COVID-19 patients with cancer are more likely to develop severe symptoms and even suffer high mortality than non-cancer patients (81–86). COVID-19 is also shown to have a significant connection with neoplasms, such as gastrointestinal, colonic and prostatic neoplasm (83, 87, 88). These results are in consistency with our finding. More and more evidences suggest worse clinical outcomes in cancer patients with COVID-19 as opposed to non-cancer patients (89–93).
It is a high priority to develop effective and safe drugs for preeclampsia-affected COVID-19 patients. Multiple molecules and drugs have been identified in this study that might have therapeutic potential for COVID-19 patients affected with preeclampsia, including valproic acid, verteporfin, 7646-79-9, astemizole, vinblastine, colchicine, doxorubicin, ambroxol, phenobarbital, and copper sulfate. Valproic acid as well as its amidic derivatives (valnoctamide and valpromide) have long been demonstrated as an effective antiviral by inhibiting in vitro infection of a wide range of viruses (94, 95). Valproic acid may decrease the SARS-CoV-2 viral load, thus reducing infection risk by targeting the ACE2 receptor and transmembrane serine protease 2 (96). Verteporfin and protoporphyrin IX (PpIX), both approved by FDA, could inhibit the SARS-CoV-2-induced cytopathic effects, both at a low EC50 level (nanomolar) (97). Astemizole could also suppress the invasion of SARS-COV-2 Spike pseudovirus by binding with its ACE2 receptor (98). Colchicine can induce anti-inflammatory effects, and thus contribute to the mitigation of cytokine storm by acting on NLRP3 and inhibiting activities of IL-1β, IL-6, and IL-18 (99). Doxorubicin has reported efficacy against viral proteases in SARS-CoV-2 (100), while Ambroxol, known for its mucolytic effects in treating respiratory disorders, may show promise against SARS-CoV-2 by inducing autophagy (101).
Our study presents certain limitations. Firstly, while we identified potential drugs, the absence of clear targets necessitates further in-depth exploration into their specific mechanisms in future research. Secondly, our data did not include patients simultaneously affected by both COVID-19 and preeclampsia. Analyzing data with such dual conditions holds substantial implications. This analysis facilitates the identification of shared genetic features and molecular mechanisms between preeclampsia and COVID-19, shedding light on their underlying connections. Moreover, the discovery of common gene expression changes in both diseases opens avenues for potential novel biomarkers, contributing to the development of diagnostic or prognostic indicators. As pandemic restrictions ease, the increasing opportunities to collect specimens with these coexisting conditions will fuel continued in-depth research in the future.
In summary, our study may provide a new line of research in identifying the crosstalk between COVID-19 and Preeclampsia. First, we used GEO databases to identify hub genes which could contribute to the occurrence and development of COVID-19 and preeclampsia. Secondly, the interactions between CVOID-19 and preeclampsia were identified, thus shedding novel light on the molecular mechanisms that underlie the COVID-19 infection and preeclampsia. Finally, ten candidate drugs, which could potentially act as therapeutic biomarkers for COVID-19 and preeclampsia, were identified.
In summary, this study uncovered shared molecular pathways and hub genes between COVID-19 and preeclampsia. The identified hub genes (MRPL11, MRPS12, UQCRH, ATP5I, UQCRQ, ATP5D, COX6B1, ATP5O, ATP5H, NDUFA6) exhibited distinct expression patterns validated through RT-qPCR. The findings provide crucial insights for potential therapeutic targets and further understanding of the molecular interplay between these conditions.
The original codes used for the analyses presented in the study are publicly available. This data can be found here: https://github.com/Liujunxiu97/Covid19-and-Preeclampsia.
The studies involving humans were approved by the affiliated hospital of Qingdao university. The studies were conducted in accordance with the local legislation and institutional requirements. The participants provided their written informed consent to participate in this study. The participants gave their consent to publish the study.
YC, LX, and ML designed the project. YC, JW, MS and WX developed the experiments. YC, and LX analyzed the data. YC and LX wrote the paper. All authors contributed to the article and approved the submitted version.
The author(s) declare that no financial support was received for the research, authorship, and/or publication of this article.
The authors thank participants and staff of Qingdao University for their contributions. Schematic figure was drawn by Figdraw (www.figdraw.com).
The authors declare that the research was conducted in the absence of any commercial or financial relationships that could be construed as a potential conflict of interest.
All claims expressed in this article are solely those of the authors and do not necessarily represent those of their affiliated organizations, or those of the publisher, the editors and the reviewers. Any product that may be evaluated in this article, or claim that may be made by its manufacturer, is not guaranteed or endorsed by the publisher.
The Supplementary Material for this article can be found online at: https://www.frontiersin.org/articles/10.3389/fimmu.2023.1243450/full#supplementary-material
Supplementary Figure 1 | Identification of top 10 potential drugs. Drug names, P-values, molecular formulas, and two-dimensional structures are shown.
Supplementary Figure 2 | ROC analysis of the hub genes in the COVID-19 dataset.
Supplementary Figure 3 | ROC analysis of the hub genes in the preeclampsia dataset.
Supplementary Figure 4 | The gene-disease association network represents diseases associated with common DEGs. The red squares represent the top 10 diseases with lowest P values. Other color circles represent common DEGs correlated with diseases.
Supplementary Table 1 | qPCR primer sequences.
Supplementary Table 2 | COVID-19 differentially expressed genes.
Supplementary Table 3 | Preeclampsia differentially expressed genes.
Supplementary Table 4 | Common DEGs between COVID-19 and preeclampsia.
Supplementary Table 5 | The TFs and their interactions with DEGs.
Supplementary Table 6 | The microRNAs and their interactions with DEGs.
Supplementary Table 7 | The potential drugs for the treatment of COVID-19 and preeclampsia.
Supplementary Table 8 | Regulatory interaction between common DEGs and diseases.
1. Ren SY, Gao RD, Chen YL. Fear can be more harmful than the severe acute respiratory syndrome coronavirus 2 in controlling the corona virus disease 2019 epidemic. World J Clin cases (2020) 8(4):652–7. doi: 10.12998/wjcc.v8.i4.652
2. Shereen MA, Khan S, Kazmi A, Bashir N, Siddique R. COVID-19 infection: Origin, transmission, and characteristics of human coronaviruses. J Adv Res (2020) 24:91–8. doi: 10.1016/j.jare.2020.03.005
3. Solomon M, Liang C. Human coronaviruses: The emergence of SARS-CoV-2 and management of COVID-19. Virus Res (2022) 319:198882. doi: 10.1016/j.virusres.2022.198882
4. Jayaweera M, Perera H, Gunawardana B, Manatunge J. Transmission of COVID-19 virus by droplets and aerosols: A critical review on the unresolved dichotomy. Environ Res (2020) 188:109819. doi: 10.1016/j.envres.2020.109819
5. Nelwan EJ, Tunjungputri RN, Tetrasiwi EN, Lauditta RK, Nainggolan L. Extrapulmonary manifestations COVID-19. Acta Med Indones (2022) 54(2):314–5.
6. Al-Kuraishy HM, Al-Gareeb AI, Almulaiky YQ, Cruz-Martins N, El-Saber Batiha G. Role of leukotriene pathway and montelukast in pulmonary and extrapulmonary manifestations of Covid-19: The enigmatic entity. Eur J Pharmacol (2021) 904:174196. doi: 10.1016/j.ejphar.2021.174196
7. Laya BF, Cledera THC, Lim TRU, Baluyut JMP, Medina JMP, Pasia NV. Cross-sectional imaging manifestations of extrapulmonary involvement in COVID-19 disease. J Comput Assist Tomogr (2021) 45(2):253–62. doi: 10.1097/RCT.0000000000001120
8. Vazquez JB, Menchen DA, Llorens MMM, Moreno JS. [Systemic and extrapulmonary manifestations of COVID-19]. Med (Madr) (2022) 13(55):3235–45. doi: 10.1016/j.med.2022.05.004
9. Attaway AH, Scheraga RG, Bhimraj A, Biehl M, Hatipoglu U. Severe covid-19 pneumonia: pathogenesis and clinical management. BMJ (2021) 372:n436. doi: 10.1136/bmj.n436
10. Pollard CA, Morran MP, Nestor-Kalinoski AL. The COVID-19 pandemic: a global health crisis. Physiol Genomics (2020) 52(11):549–57. doi: 10.1152/physiolgenomics.00089.2020
11. Ghulmiyyah L, Sibai B. Maternal mortality from preeclampsia/eclampsia. Semin Perinatol (2012) 36(1):56–9. doi: 10.1053/j.semperi.2011.09.011
12. Moodley J. Maternal deaths associated with hypertensive disorders of pregnancy: a population-based study. Hypertens Pregnancy (2004) 23(3):247–56. doi: 10.1081/PRG-200030301
13. Rana S, Burke SD, Karumanchi SA. Imbalances in circulating angiogenic factors in the pathophysiology of preeclampsia and related disorders. Am J Obstet Gynecol (2022) 226(2S):S1019–34. doi: 10.1016/j.ajog.2020.10.022
14. Turpin CA, Sakyi SA, Owiredu WK, Ephraim RK, Anto EO. Association between adverse pregnancy outcome and imbalance in angiogenic regulators and oxidative stress biomarkers in gestational hypertension and preeclampsia. BMC Pregnancy Childbirth (2015) 15:189. doi: 10.1186/s12884-015-0624-y
15. Papageorghiou AT, Deruelle P, Gunier RB, Rauch S, García-May PK, Mhatre M, et al. Preeclampsia and COVID-19: results from the INTERCOVID prospective longitudinal study. Am J obstetrics gynecology (2021) 225(3):289.e1–289.e17. doi: 10.1016/j.ajog.2021.05.014
16. Narang K, Enninga EAL, Gunaratne MD, Ibirogba ER, Trad ATA, Elrefaei A, et al. SARS-CoV-2 infection and COVID-19 during pregnancy: a multidisciplinary review. In: Proceedings. Elsevier (2020). p. 1750–65.
17. Morhart P, Mardin C, Rauh M, Jungert J, Hammersen J, Kehl S, et al. Maternal SARS-CoV-2 infection during pregnancy: possible impact on the infant. Eur J Pediatr (2022) 181(1):413–8. doi: 10.1007/s00431-021-04221-w
18. Di Mascio D, Khalil A, Saccone G, Rizzo G, Buca D, Liberati M, et al. Outcome of coronavirus spectrum infections (SARS, MERS, COVID-19) during pregnancy: a systematic review and meta-analysis. Am J Obstet Gynecol MFM (2020) 2(2):100107. doi: 10.1016/j.ajogmf.2020.100107
19. Zaigham M, Andersson O. Maternal and perinatal outcomes with COVID-19: A systematic review of 108 pregnancies. Acta Obstet Gynecol Scand (2020) 99(7):823–9. doi: 10.1111/aogs.13867
20. Woodworth KR, Olsen EO, Neelam V, Lewis EL, Galang RR, Oduyebo T, et al. Birth and infant outcomes following laboratory-confirmed SARS-CoV-2 infection in pregnancy - SET-NET, 16 jurisdictions, March 29-October 14, 2020. MMWR Morb Mortal Wkly Rep (2020) 69(44):1635–40. doi: 10.15585/mmwr.mm6944e2
21. Verma S, Carter EB, Mysorekar IU. SARS-CoV2 and pregnancy: An invisible enemy? Am J Reprod Immunol (2020) 84(5):e13308. doi: 10.1111/aji.13308
22. Mahajan NN, Ansari M, Gaikwad C, Jadhav P, Tirkey D, Pophalkar MP, et al. Impact of SARS-CoV-2 on multiple gestation pregnancy. Int J Gynaecol Obstet (2021) 152(2):220–5. doi: 10.1002/ijgo.13508
23. Capobianco G, Saderi L, Aliberti S, Mondoni M, Piana A, Dessole F, et al. COVID-19 in pregnant women: A systematic review and meta-analysis. Eur J Obstet Gynecol Reprod Biol (2020) 252:543–58. doi: 10.1016/j.ejogrb.2020.07.006
24. Zamaniyan M, Ebadi A, Aghajanpoor S, Rahmani Z, Haghshenas M, Azizi S. Preterm delivery, maternal death, and vertical transmission in a pregnant woman with COVID-19 infection. Prenat Diagn (2020) 40(13):1759–61. doi: 10.1002/pd.5713
25. Rosenbloom JI, Raghuraman N, Carter EB, Kelly JC. Coronavirus disease 2019 infection and hypertensive disorders of pregnancy. Am J Obstet Gynecol (2021) 224(6):623–4. doi: 10.1016/j.ajog.2021.03.001
26. Pulinx B, Kieffer D, Michiels I, Petermans S, Strybol D, Delvaux S, et al. Vertical transmission of SARS-CoV-2 infection and preterm birth. Eur J Clin Microbiol Infect Dis (2020) 39(12):2441–5. doi: 10.1007/s10096-020-03964-y
27. Perritt J, Grossman D. The health consequences of restrictive abortion laws. JAMA Intern Med (2021) 181(5):713–4. doi: 10.1001/jamainternmed.2020.9279
28. Arunachalam PS, Wimmers F, Mok CKP, Perera RA, Scott M, Hagan T, et al. Systems biological assessment of immunity to mild versus severe COVID-19 infection in humans. Science (2020) 369(6508):1210–20. doi: 10.1126/science.abc6261
29. Textoris J, Ivorra D, Ben Amara A, Sabatier F, Ménard J-P, Heckenroth H, et al. Evaluation of current and new biomarkers in severe preeclampsia: a microarray approach reveals the VSIG4 gene as a potential blood biomarker. PloS One (2013) 8(12):e82638. doi: 10.1371/journal.pone.0082638
30. Love MI, Huber W, Anders S. Moderated estimation of fold change and dispersion for RNA-seq data with DESeq2. Genome Biol (2014) 15(12):1–21. doi: 10.1186/s13059-014-0550-8
31. Smyth GK. Limma: linear models for microarray data. In: Bioinformatics and computational biology solutions using R and Bioconductor (2005) Springer. p. 397–420.
32. Yu G, Wang L-G, Han Y, He Q-Y. clusterProfiler: an R package for comparing biological themes among gene clusters. Omics: J Integr Biol (2012) 16(5):284–7. doi: 10.1089/omi.2011.0118
33. Szklarczyk D, Franceschini A, Wyder S, Forslund K, Heller D, Huerta-Cepas J, et al. STRING v10: protein–protein interaction networks, integrated over the tree of life. Nucleic Acids Res (2015) 43(D1):D447–52. doi: 10.1093/nar/gku1003
34. Shannon P, Markiel A, Ozier O, Baliga NS, Wang JT, Ramage D, et al. Cytoscape: a software environment for integrated models of biomolecular interaction networks. Genome Res (2003) 13(11):2498–504. doi: 10.1101/gr.1239303
35. Chin CH, Chen SH, Wu HH, Ho CW, Ko MT, Lin CY. cytoHubba: identifying hub objects and sub-networks from complex interactome. BMC Syst Biol (2014) 8 Suppl 4:S11. doi: 10.1186/1752-0509-8-S4-S11
36. Chin C-H, Chen S-H, Wu H-H, Ho C-W, Ko M-T, Lin C-Y. cytoHubba: identifying hub objects and sub-networks from complex interactome. BMC Syst Biol (2014) 8(4):1–7. doi: 10.1186/1752-0509-8-S4-S11
37. Lambert SA, Jolma A, Campitelli LF, Das PK, Yin Y, Albu M, et al. The human transcription factors. Cell (2018) 172(4):650–65. doi: 10.1016/j.cell.2018.01.029
38. Kuleshov MV, Jones MR, Rouillard AD, Fernandez NF, Duan Q, Wang Z, et al. Enrichr: a comprehensive gene set enrichment analysis web server 2016 update. Nucleic Acids Res (2016) 44(W1):W90–97. doi: 10.1093/nar/gkw377
39. Huang HY, Lin YC, Li J, Huang KY, Shrestha S, Hong HC, et al. miRTarBase 2020: updates to the experimentally validated microRNA-target interaction database. Nucleic Acids Res (2020) 48(D1):D148–54. doi: 10.1093/nar/gkz896
40. Piñero J, Bravo À, Queralt-Rosinach N, Gutiérrez-Sacristán A, Deu-Pons J, Centeno E, et al. DisGeNET: a comprehensive platform integrating information on human disease-associated genes and variants. Nucleic Acids Res (2016) 45(D1):D833-D839. doi: 10.1093/nar/gkw943
41. Yoo M, Shin J, Kim J, Ryall KA, Lee K, Lee S, et al. DSigDB: drug signatures database for gene set analysis. Bioinformatics (2015) 31(18):3069–71. doi: 10.1093/bioinformatics/btv313
42. Mahmud SMH, Al-Mustanjid M, Akter F, Rahman MS, Ahmed K, Rahman MH, et al. Bioinformatics and system biology approach to identify the influences of SARS-CoV-2 infections to idiopathic pulmonary fibrosis and chronic obstructive pulmonary disease patients. Brief Bioinform (2021) 22(5):bbab115. doi: 10.1093/bib/bbab115
43. Rahman MR, Islam T, Shahjaman M, Islam MR, Lombardo SD, Bramanti P, et al. Discovering common pathogenetic processes between COVID-19 and diabetes mellitus by differential gene expression pattern analysis. Brief Bioinform (2021) 22(6):bbab262. doi: 10.1093/bib/bbab262
44. Peng WF, Bai F, Shao K, Shen LS, Li HH, Huang S. The key genes underlying pathophysiology association between the type 2-diabetic and colorectal cancer. J Cell Physiol (2018) 233(11):8551–7. doi: 10.1002/jcp.26440
45. Ahmed F, Ansari JA, Ansari ZE, Alam Q, Gan SH, Kamal MA, et al. A molecular bridge: connecting type 2 diabetes and Alzheimer’s disease. CNS Neurol Disord Drug Targets (2014) 13(2):312–21. doi: 10.2174/18715273113126660133
46. Narang K, Enninga EAL, Gunaratne M, Ibirogba ER, Trad ATA, Elrefaei A, et al. SARS-CoV-2 infection and COVID-19 during pregnancy: A multidisciplinary review. Mayo Clin Proc (2020) 95(8):1750–65. doi: 10.1016/j.mayocp.2020.05.011
47. Wei SQ, Bilodeau-Bertrand M, Liu S, Auger N. The impact of COVID-19 on pregnancy outcomes: a systematic review and meta-analysis. CMAJ (2021) 193(16):E540–8. doi: 10.1503/cmaj.202604
48. Azarkish F, Sheikhi F, Mirkazehi Z, Kalkali S, Bameni Moghadam P, Zahirniya M. Preeclampsia and the crucial postpartum period for Covid-19 infected mothers: A case report. Pregnancy Hypertens (2021) 23:136–9. doi: 10.1016/j.preghy.2020.10.012
49. Garcia Rodriguez A, Marcos Contreras S, Fernandez Manovel SM, Marcos Vidal JM, Diez Buron F, Fernandez Fernandez C, et al. SARS-COV-2 infection during pregnancy, a risk factor for eclampsia or neurological manifestations of COVID-19? Case report. BMC Pregnancy Childbirth (2020) 20(1):587. doi: 10.21203/rs.3.rs-40372/v1
50. Bennett WA, Lagoo-Deenadayalan S, Stopple JA, Barber WH, Hale E, Brackin MN, et al. Cytokine expression by first-trimester human chorionic villi. Am J Reprod Immunol (1998) 40(5):309–18. doi: 10.1111/j.1600-0897.1998.tb00059.x
51. Baumann M, Becker S, Kruger HJ, Vogler H, Maurer T, Beck-Bornholdt HP. Flow cytometric determination of the time of metastasis during fractionated radiation therapy of the rat rhabdomyosarcoma R1H. Int J Radiat Biol (1990) 58(2):361–9. doi: 10.1080/09553009014551691
52. Besse A, Brezavar D, Hanson J, Larson A, Bonnen PE. LONP1 de novo dominant mutation causes mitochondrial encephalopathy with loss of LONP1 chaperone activity and excessive LONP1 proteolytic activity. Mitochondrion (2020) 51:68–78. doi: 10.1016/j.mito.2020.01.004
53. Pacheu-Grau D, Perez-Delgado L, Gomez-Diaz C, Fraile-Rodrigo J, Montoya J, Ruiz-Pesini E. Mitochondrial ribosome and Meniere’s disease: a pilot study. Eur Arch Otorhinolaryngol (2012) 269(8):2003–8. doi: 10.1007/s00405-012-2066-8
54. Emperador S, Pacheu-Grau D, Bayona-Bafaluy MP, Garrido-Perez N, Martin-Navarro A, Lopez-Perez MJ, et al. An MRPS12 mutation modifies aminoglycoside sensitivity caused by 12S rRNA mutations. Front Genet (2014) 5:469. doi: 10.3389/fgene.2014.00469
55. Qiu X, Guo D, Du J, Bai Y, Wang F. A novel biomarker, MRPS12 functions as a potential oncogene in ovarian cancer and is a promising prognostic candidate. Med (Baltimore) (2021) 100(8):e24898. doi: 10.1097/MD.0000000000024898
56. Luo Y, Medina Bengtsson L, Wang X, Huang T, Liu G, Murphy S, et al. UQCRH downregulation promotes Warburg effect in renal cell carcinoma cells. Sci Rep (2020) 10(1):15021. doi: 10.1038/s41598-020-72107-2
57. Park ER, Kim SB, Lee JS, Kim YH, Lee DH, Cho EH, et al. The mitochondrial hinge protein, UQCRH, is a novel prognostic factor for hepatocellular carcinoma. Cancer Med (2017) 6(4):749–60. doi: 10.1002/cam4.1042
58. Arselin G, Giraud MF, Dautant A, Vaillier J, Brethes D, Coulary-Salin B, et al. The GxxxG motif of the transmembrane domain of subunit e is involved in the dimerization/oligomerization of the yeast ATP synthase complex in the mitochondrial membrane. Eur J Biochem (2003) 270(8):1875–84. doi: 10.1046/j.1432-1033.2003.03557.x
59. Mordaunt DA, Jolley A, Balasubramaniam S, Thorburn DR, Mountford HS, Compton AG, et al. Phenotypic variation of TTC19-deficient mitochondrial complex III deficiency: a case report and literature review. Am J Med Genet A (2015) 167(6):1330–6. doi: 10.1002/ajmg.a.36968
60. Barrera G, Gentile F, Pizzimenti S, Canuto RA, Daga M, Arcaro A, et al. Mitochondrial dysfunction in cancer and neurodegenerative diseases: spotlight on fatty acid oxidation and lipoperoxidation products. Antioxidants (Basel) (2016) 5(1):7. doi: 10.3390/antiox5010007
61. Duvezin-Caubet S, Rak M, Lefebvre-Legendre L, Tetaud E, Bonnefoy N, di Rago JP. A “petite obligate” mutant of Saccharomyces cerevisiae: functional mtDNA is lethal in cells lacking the delta subunit of mitochondrial F1-ATPase. J Biol Chem (2006) 281(24):16305–13. doi: 10.1074/jbc.M513805200
62. Geisler DA, Papke C, Obata T, Nunes-Nesi A, Matthes A, Schneitz K, et al. Downregulation of the delta-subunit reduces mitochondrial ATP synthase levels, alters respiration, and restricts growth and gametophyte development in Arabidopsis. Plant Cell (2012) 24(7):2792–811. doi: 10.1105/tpc.112.099424
63. Hilbers F, Eggers R, Pradela K, Friedrich K, Herkenhoff-Hesselmann B, Becker E, et al. Subunit delta is the key player for assembly of the H(+)-translocating unit of Escherichia coli F(O)F1 ATP synthase. J Biol Chem (2013) 288(36):25880–94. doi: 10.1074/jbc.M113.484675
64. Lenka N, Vijayasarathy C, Mullick J, Avadhani NG. Structural organization and transcription regulation of nuclear genes encoding the mammalian cytochrome c oxidase complex. Prog Nucleic Acid Res Mol Biol (1998) 61:309–44. doi: 10.1016/S0079-6603(08)60830-2
65. Massa V, Fernandez-Vizarra E, Alshahwan S, Bakhsh E, Goffrini P, Ferrero I, et al. Severe infantile encephalomyopathy caused by a mutation in COX6B1, a nucleus-encoded subunit of cytochrome c oxidase. Am J Hum Genet (2008) 82(6):1281–9. doi: 10.1016/j.ajhg.2008.05.002
66. Abdulhag UN, Soiferman D, Schueler-Furman O, Miller C, Shaag A, Elpeleg O, et al. Mitochondrial complex IV deficiency, caused by mutated COX6B1, is associated with encephalomyopathy, hydrocephalus and cardiomyopathy. Eur J Hum Genet (2015) 23(2):159–64. doi: 10.1038/ejhg.2014.85
67. Song Y, Wang J, Sun J, Chen X, Shi J, Wu Z, et al. Screening of potential biomarkers for gastric cancer with diagnostic value using label-free global proteome analysis. Genomics Proteomics Bioinf (2020) 18(6):679–95. doi: 10.1016/j.gpb.2020.06.012
68. Bruggemann M, Gromes A, Poss M, Schmidt D, Klumper N, Tolkach Y, et al. Systematic analysis of the expression of the mitochondrial ATP synthase (Complex V) subunits in clear cell renal cell carcinoma. Transl Oncol (2017) 10(4):661–8. doi: 10.1016/j.tranon.2017.06.002
69. Song KH, Kim JH, Lee YH, Bae HC, Lee HJ, Woo SR, et al. Mitochondrial reprogramming via ATP5H loss promotes multimodal cancer therapy resistance. J Clin Invest (2018) 128(9):4098–114. doi: 10.1172/JCI96804
70. Wiersinga WJ, Rhodes A, Cheng AC, Peacock SJ, Prescott HC. Pathophysiology, transmission, diagnosis, and treatment of coronavirus disease 2019 (COVID-19): A review. JAMA (2020) 324(8):782–93. doi: 10.1001/jama.2020.12839
71. Verma S, Joshi CS, Silverstein RB, He M, Carter EB, Mysorekar IU. SARS-CoV-2 colonization of maternal and fetal cells of the human placenta promotes alteration of local renin-angiotensin system. Med (N Y) (2021) 2(5):575–590 e575. doi: 10.1016/j.medj.2021.04.009
72. Resta L, Vimercati A, Cazzato G, Fanelli M, Scarcella SV, Ingravallo G, et al. SARS-CoV-2, placental histopathology, gravity of infection and immunopathology: is there an association? Viruses (2022) 14(6):1330. doi: 10.3390/v14061330
73. Sin R, Kubiska M, Cmorej PC, Vachalova J. Clinical and laboratory characteristics of the COVID-19 disease in adult patients. Neuro Endocrinol Lett (2020) 41(5):223–30.
74. Shenoy S. Coronavirus (Covid-19) sepsis: revisiting mitochondrial dysfunction in pathogenesis, aging, inflammation, and mortality. Inflammation Res (2020) 69(11):1077–85. doi: 10.1007/s00011-020-01389-z
75. Saleh J, Peyssonnaux C, Singh KK, Edeas M. Mitochondria and microbiota dysfunction in COVID-19 pathogenesis. Mitochondrion (2020) 54:1–7. doi: 10.1016/j.mito.2020.06.008
76. Delgado-Roche L, Mesta F. Oxidative stress as key player in severe acute respiratory syndrome coronavirus (SARS-CoV) infection. Arch Med Res (2020) 51(5):384–7. doi: 10.1016/j.arcmed.2020.04.019
77. Vaquerizas JM, Kummerfeld SK, Teichmann SA, Luscombe NM. A census of human transcription factors: function, expression and evolution. Nat Rev Genet (2009) 10(4):252–63. doi: 10.1038/nrg2538
78. Islam Z, Ali AM, Naik A, Eldaw M, Decock J, Kolatkar PR. Transcription factors: The fulcrum between cell development and carcinogenesis. Front Oncol (2021) 11:681377. doi: 10.3389/fonc.2021.681377
79. Macfarlane LA, Murphy PR. MicroRNA: biogenesis, function and role in cancer. Curr Genomics (2010) 11(7):537–61. doi: 10.2174/138920210793175895
80. Peng Y, Croce CM. The role of MicroRNAs in human cancer. Signal Transduct Target Ther (2016) 1:15004. doi: 10.1038/sigtrans.2015.4
81. Liang W, Guan W, Chen R, Wang W, Li J, Xu K, et al. Cancer patients in SARS-CoV-2 infection: a nationwide analysis in China. Lancet Oncol (2020) 21(3):335–7. doi: 10.1016/S1470-2045(20)30096-6
82. Lee LY, Cazier J-B, Angelis V, Arnold R, Bisht V, Campton NA, et al. COVID-19 mortality in patients with cancer on chemotherapy or other anticancer treatments: a prospective cohort study. Lancet (2020) 395(10241):1919–26. doi: 10.1016/S0140-6736(20)31173-9
83. Mehta V, Goel S, Kabarriti R, Cole D, Goldfinger M, Acuna-Villaorduna A, et al. Case fatality rate of cancer patients with COVID-19 in a New York hospital system. Cancer Discovery (2020) 10(7):935–41. doi: 10.1158/2159-8290.CD-20-0516
84. Yu J, Ouyang W, Chua ML, Xie C. SARS-CoV-2 transmission in patients with cancer at a tertiary care hospital in Wuhan, China. JAMA Oncol (2020) 6(7):1108–10. doi: 10.1001/jamaoncol.2020.0980
85. Mehta V, Goel S, Kabarriti R, Cole D, Goldfinger M, Acuna-Villaorduna A, et al. Case fatality rate of cancer patients with COVID-19 in a New York hospital systemCase fatality rate of cancer patients with COVID-19. Cancer Discovery (2020) 10(7):935–41. doi: 10.1158/2159-8290.CD-20-0516
86. Tian J, Yuan X, Xiao J, Zhong Q, Yang C, Liu B, et al. Clinical characteristics and risk factors associated with COVID-19 disease severity in patients with cancer in Wuhan, China: a multicentre, retrospective, cohort study. Lancet Oncol (2020) 21(7):893–903. doi: 10.1016/S1470-2045(20)30309-0
87. Lin B, Ferguson C, White JT, Wang S, Vessella R, True LD, et al. Prostate-localized and androgen-regulated expression of the membrane-bound serine protease TMPRSS2. Cancer Res (1999) 59(17):4180–4.
88. Hu H, Tang N, Zhang F, Li L, Li L. Bioinformatics and system biology approach to identify the influences of COVID-19 on rheumatoid arthritis. Front Immunol (2022) 13. doi: 10.3389/fimmu.2022.860676
89. Albiges L, Foulon S, Bayle A, Gachot B, Pommeret F, Willekens C, et al. Determinants of the outcomes of patients with cancer infected with SARS-CoV-2: results from the Gustave Roussy cohort. Nat Cancer (2020) 1(10):965–75. doi: 10.1038/s43018-020-00120-5
90. Garassino MC, Whisenant JG, Huang L-C, Trama A, Torri V, Agustoni F, et al. COVID-19 in patients with thoracic Malignancies (TERAVOLT): first results of an international, registry-based, cohort study. Lancet Oncol (2020) 21(7):914–22. doi: 10.1016/S1470-2045(20)30314-4
91. Kuderer NM, Choueiri TK, Shah DP, Shyr Y, Rubinstein SM, Rivera DR, et al. Clinical impact of COVID-19 on patients with cancer (CCC19): a cohort study. Lancet (2020) 395(10241):1907–18. doi: 10.1016/S0140-6736(20)31187-9
92. Robilotti EV, Babady NE, Mead PA, Rolling T, Perez-Johnston R, Bernardes M, et al. Determinants of COVID-19 disease severity in patients with cancer. Nat Med (2020) 26(8):1218–23. doi: 10.1038/s41591-020-0979-0
93. Giannakoulis VG, Papoutsi E, Siempos II. Effect of cancer on clinical outcomes of patients with COVID-19: a meta-analysis of patient data. JCO Global Oncol (2020) 6:799–808. doi: 10.1200/GO.20.00225
94. Vázquez-Calvo A, Saiz J-C, Sobrino F, Martín-Acebes MA. Inhibition of enveloped virus infection of cultured cells by valproic acid. J Virol (2011) 85(3):1267–74. doi: 10.1128/JVI.01717-10
95. Andreu S, Ripa I, Bello-Morales R, López-Guerrero JA. Valproic acid and its amidic derivatives as new antivirals against alphaherpesviruses. Viruses (2020) 12(12):1356. doi: 10.3390/v12121356
96. Hoffmann M, Kleine-Weber H, Schroeder S, Krüger N, Herrler T, Erichsen S, et al. SARS-CoV-2 cell entry depends on ACE2 and TMPRSS2 and is blocked by a clinically proven protease inhibitor. cell (2020) 181(2):271–280.e278. doi: 10.1016/j.cell.2020.02.052
97. Gu C, Wu Y, Guo H, Zhu Y, Xu W, Wang Y, et al. Protoporphyrin IX and verteporfin potently inhibit SARS-CoV-2 infection in vitro and in a mouse model expressing human ACE2. Sci Bull (2021) 66(9):925–36. doi: 10.1016/j.scib.2020.12.005
98. Wang X, Lu J, Ge S, Hou Y, Hu T, Lv Y, et al. Astemizole as a drug to inhibit the effect of SARS-COV-2 in vitro. Microbial Pathogenesis (2021) 156:104929. doi: 10.1016/j.micpath.2021.104929
99. Vitiello A, Ferrara F, Pelliccia C, Granata G, La Porta R. Cytokine storm and colchicine potential role in fighting SARS-CoV-2 pneumonia. Ital J Med (2020) 14(2):88–94. doi: 10.4081/itjm.2020.1284
100. Sajid Jamal QM, Alharbi AH, Ahmad V. Identification of doxorubicin as a potential therapeutic against SARS-CoV-2 (COVID-19) protease: a molecular docking and dynamics simulation studies. J Biomolecular Structure Dynamics (2022) 40(17):7960–74. doi: 10.1080/07391102.2021.1905551
Keywords: COVID-19, preeclampsia, differentially expressed genes, protein-protein interaction, drug molecule
Citation: Chu Y, Li M, Sun M, Wang J, Xin W and Xu L (2024) Gene crosstalk between COVID-19 and preeclampsia revealed by blood transcriptome analysis. Front. Immunol. 14:1243450. doi: 10.3389/fimmu.2023.1243450
Received: 22 June 2023; Accepted: 14 December 2023;
Published: 08 January 2024.
Edited by:
Arif Nur Muhammad Ansori, Airlangga University, IndonesiaReviewed by:
Muhammad Widyananda, University of Brawijaya, IndonesiaCopyright © 2024 Chu, Li, Sun, Wang, Xin and Xu. This is an open-access article distributed under the terms of the Creative Commons Attribution License (CC BY). The use, distribution or reproduction in other forums is permitted, provided the original author(s) and the copyright owner(s) are credited and that the original publication in this journal is cited, in accordance with accepted academic practice. No use, distribution or reproduction is permitted which does not comply with these terms.
*Correspondence: Lin Xu, eHVsaW5xZEBzaW5hLmNvbQ==
Disclaimer: All claims expressed in this article are solely those of the authors and do not necessarily represent those of their affiliated organizations, or those of the publisher, the editors and the reviewers. Any product that may be evaluated in this article or claim that may be made by its manufacturer is not guaranteed or endorsed by the publisher.
Research integrity at Frontiers
Learn more about the work of our research integrity team to safeguard the quality of each article we publish.