- 1Department of General Surgery, The Second Affiliated Hospital of Nanjing Medical University, Nanjing, Jiangsu, China
- 2Department of Clinical Laboratory, Lianshui County People’s Hospital, Huai’an, China
- 3Department of Urology, The Second Affiliated Hospital of Nanjing Medical University, Nanjing, China
Purpose: To investigate the impact of N6-methyladenosine- (m6A) and neutrophil extracellular traps- (NETs) related lncRNAs (MNlncRNAs) on the prognosis of hepatocellular carcinoma (HCC).
Methods: We collected m6A and NETs-related genes from published studies. We identified the MNlncRNAs by correlation analysis. Cox regression and the least absolute selection operator (LASSO) method were used to select predictive MNlncRNAs. The expressions of predictive MNlncRNAs were detected by cell and tissue experiments. Survival, medication sensitivity, and immunological microenvironment evaluations were used to assess the model’s prognostic utility. Finally, we performed cellular experiments to further validate the model’s prognostic reliability.
Results: We obtained a total of 209 MNlncRNAs. 7 MNlncRNAs comprised the prognostic model, which successfully stratifies HCC patients, with the area under the curve (AUC) ranging from 0.7 to 0.8. In vitro tests confirmed that higher risk patients had worse prognosis. Risk score, immunological microenvironment, and immune checkpoint gene expression were all significantly correlated with each other in HCC. In the group at high risk, immunotherapy could be more successful. Cellular assays confirmed that HCC cells with high risk scores have a higher proliferation and invasive capacity.
Conclusion: The MNlncRNAs-related prognostic model aided in determining HCC prognosis, revealing novel therapeutic options, notably immunotherapy.
1 Introduction
Liver cancer has a poor prognosis and ranks third in the list of tumor-related causes of death (1). The most frequent kind of liver cancer is hepatocellular carcinoma (HCC) (2). With the development of medicine, great progress has been made in the treatment of HCC (3). Surgery and liver transplantation are currently the common treatment options for HCC (4, 5). However, the prognosis of HCC patients does not increase with the progression of treatment. Five-year survival for patients with advanced HCC is only 10%, with a median survival of fewer than 10 months (6). HCC is a rather complex disease with a high degree of heterogeneity that poses a challenge to the prognostic assessment of patients (7). For immunotherapy of HCC, the identification and validation of predictive biomarkers remain a major unresolved challenge (8).
Long non-coding RNAs (lncRNAs), which are usually not translated into proteins, regulate gene expression involved in cell growth and proliferation (9). According to research, lncRNA is a potential biomarker that plays a critical function in tumor formation (10). Meanwhile, the lncRNA model can reliably predict the outcome of cancer patients and recommend therapeutic therapy (11). Therefore, it is practical to study lncRNA to evaluate the outcome of patients with HCC (12).
N6-methyladenosine (m6A) is the most common RNA modification and a hot issue in the field of cancer research and is abundantly represented in the transcriptome (13, 14). M6A affects almost all aspects of RNA metabolism, providing new ideas for cancer diagnosis, treatment, and prognosis assessment (15). The tumor immune microenvironment is an important element in the growth and spread of malignancies (16). The majority of immune system cells in humans are neutrophils, which serve as tumor patients’ biomarkers for risk classification (17, 18). Especially in HCC, the presence of tumor-associated neutrophils is linked to poor prognosis (19). Neutrophil extracellular traps (NETs) are reticulations whose function is mainly to kill harmful microorganisms (20). Classical NETs are formed by a process known as “NETosis”, which is distinct from programmed cell death and is a certain type of controlled cell death (21). NETs are important for tumor development. By stimulating the immune system, NETs prevent tumor development, whereas tumors can instruct neutrophils to undergo NETosis in order to promote metastasis (22).
Studies had demonstrated that the expression of m6A-related genes was closely related to the immune microenvironment of malignant tumors and can guide patient immunotherapy (23). Meanwhile, m6A genes were mainly enriched in the formation of extracellular traps in neutrophils, suggesting that probing the m6A patterns of tumors can help to understand the diversity and complexity of the tumor microenvironment (23). It has been shown that models that integrate multiple markers into a single model outperform those constructed using a single marker, facilitating individualized patient management (24). Consequently, combining several kinds of biomarkers is acceptable to create a more accurate model.
Hence, we first identified a set of m6A- and NETs-related lncRNAs (MNlncRNAs) linked to m6A genes and NETs-related genes. We developed a predictive model that can reliably predict patients’ survival from HCC based on these MNlncRNAs. Also, the immune microenvironment and drug sensitivity of HCC were correlated with MNlncRNAs. This research lays the groundwork for an HCC treatment plan.
2 Materials and methods
2.1 Data acquisition
Transcriptome and clinical data were obtained from the TCGA database. Counts was the workflow type utilized. All data were log2 transformed. All the HCC tissues and adjacent tissues were gathered from 20 HCC patients who had received curative surgery at Second Affiliated Hospital of Nanjing Medical University between 2020 and 2021. The utilization of human tissues was granted ethical approval by the ethics committee of the Second Affiliated Hospital of Nanjing Medical University. Meanwhile, we collected 23 m6A-related genes and 69 NETs-related genes from the published studies. 23 m6A genes were m6A regulators, including 13 readers, 8 writers and 2 erasers (25). The NETs-related gene set composed of 69 genes summarized the research progress of NETs in immunity and various diseases, mainly covering the ligands and receptors that stimulate the formation of NETs, downstream-related signals, and the molecules identified to adhere to the framework of NETs (26, 27).
2.2 Identification of MNlncRNAs
By Pearson correlation analysis, we identified a set of m6A-related lncRNAs and NETs-related lncRNAs. The p-value was set to 0.001 and the correlation coefficient was set at 0.40. Then, We obtained a set of MNlncRNAs by taking the intersection of these two sets of lncRNAs. These MNlncRNAs were used for subsequent analysis.
2.3 TCGA data process
Before obtaining the count file, the downloaded data were combined and preprocessed with the Perl programming language. The lncRNA symbols were changed using Perl. The TCGA transcriptome data were then matched to MNlncRNAs. Patients with insufficient clinical data and no follow-up days were eliminated. We performed differential analysis (p<0.001) of normal and tumor samples to screen out MNlncRNAs with differential, which were matched to the survival data. In addition, to reduce the effect of noise, patients with zero expression of MNlncRNAs were removed, and a 7:3 ratio of training cohorts (218) to test cohorts (98) was generated from the TCGA dataset at random.
2.4 Prognostic model construction and evaluation
After matching the MNlncRNAs expression data and clinical data, the univariate COX analysis was performed (p <0.001). Then, the LASSO regression method was utilized to narrow down the list of MNlncRNAs with prognostic significance. The risk score was computed according to the model formula. After that, the prognostic model was built. The prognostic model’s performance was validated using the test cohorts.
We divided them into high- and low-risk categories based on the median. Following that, we ran a survival analysis to check if there was any difference in prognosis between the training and test cohorts. Meanwhile, we displayed the sample distribution between the two cohorts to assess the efficacy of stratifying samples. Heatmaps were utilized to compare the expression levels of the model MNlncRNAs. The AUC was used to validate the model’s prediction capabilities. In addition, we compared the predictive performance of risk scores with clinical factors.
2.5 Functional analysis
The Gene Ontology (GO) and Kyoto Encyclopedia of Genes and Genomes (KEGG) pathway studies were performed using the “clusterProfiler” package. To do gene set variation analysis (GSVA), we used the “GSVA” package. The results were shown using bar charts (p<0.05).
2.6 Cell culture
HCC cell lines, including PLC, SK, LM3, HepG2 and HuH-7, and human liver cell LO2 were obtained from the American Type Culture Collection (ATCC) and cultured in DMEM medium containing 10% FBS.
2.7 Quantitative real-time polymerase chain reaction (qRT-PCR)
Utilizing the TRIzol method (Invitrogen, Carlsbad, CA, USA) to extract total RNA from cells or tissues. Reverse transcription followed the instructions of PrimeScriptTM RT reagent kit (TaKaRa, Kyoto, Japan) and qRT-PCR followed the instructions of the CHAMQ SYBR qPCR Master Mix kit (Vazyme, Nanjing, China). The relative gene expression was calculated using the 2-△△CT method. All primers sequences in our research are listed in Supplementary Table 1.
2.8 Transwell migration and invasion assay
Utilizing transwell chambers (8 μm PET; Millipore Corporation, Burlington, MA, USA) to perform transwell assay. Cells (2×105/ml) were resuspended in serum-free DMEM medium. For the migration assay, 200µl of the cell suspension was added to the upper chamber, and 800µl of DMEN medium supplemented with 10% serum was added to lower chamber. For the invasion assay, 200µl of the cell suspension was added to the upper chamber precoated with 0.5 mg/L Matrigel (BD Biosciences, Franklin Lakes, NJ, USA), and 800µl medium containing with 10% serum was added to lower chamber. After 24 hours of incubation, cells were fixed with methanol for 30 minutes and stained with 0.4% crystal violet for an hour at room temperature. The upper layers of cells were gently erased with cotton swabs, and the chambers were washed 3 times with PBS. The cells were counted in 5 random fields.
2.9 Colony formation assay
200 untreated cells were seeded in six-well plate and cultivated for 2 weeks. Afterwards, cells were fixed with methanol for 15 minutes and stained with 0.4% crystal violet for 30 minutes at room temperature, and the colonies were analyzed.
2.10 EDU proliferation assay
20,000 untreated cells were seeded in 96-well plate and cultivated for 24 hours. EDU proliferation assay was performed following the instruction of BeyoClick™ EdU Cell Proliferation Kit with DAB (Beyotime, Nanjing, China), and the cells were then observed using fluorescent microscope.
2.11 Immunoassay analysis
Utilizing heatmaps for immune infiltration and correlation maps, the degree of tumor invasion was compared to the model. CIBERSORT and XCELL methods were mainly refered (28, 29). A list of genes related to immune checkpoints was discovered (30–33). The boxplots depicted the results of the analyses.
2.12 Drug sensitivity analysis
We received expression matrices and medication processing data from the Cancer Genome Project. The drugs associated with the prognosis model were derived using the “pRROpheticPredict” tool (p<0.001) (34).
3 Results
3.1 Data processing
After screening, 365 patients with HCC were included in the study. Using Pearson correlation analysis, we obtained 415 m6A-associated lncRNAs and 488 NETs-related lncRNAs. Then, we obtained a total of 209 MNlncRNAs for subsequent analysis after taking the intersection of the two sets of lncRNAs.
3.2 Prognostic model construction
First, we performed a different analysis and 148 MNlncRNAs with differences in the normal and tumor groups were screened out. After matching the MNlncRNAs expression data and clinical data, we performed the univariate COX analysis and selected 17 MNlncRNAs (Figure 1A). Then through Lasso regression analysis, we selected out 7 MNlncRNAs and built the prognostic model (Figures 1B, C). The model was calculated as follows: risk score = AC074117.1* 0.10679103 + AC026401.3* 0.00817401 + AL355574.1* 0.08725795 + ZEB1.AS1* 0.11541016 + AL031985.3* 0.24377726 + NRAV* 0.11141409 + AC107959.3* 0.03665612.
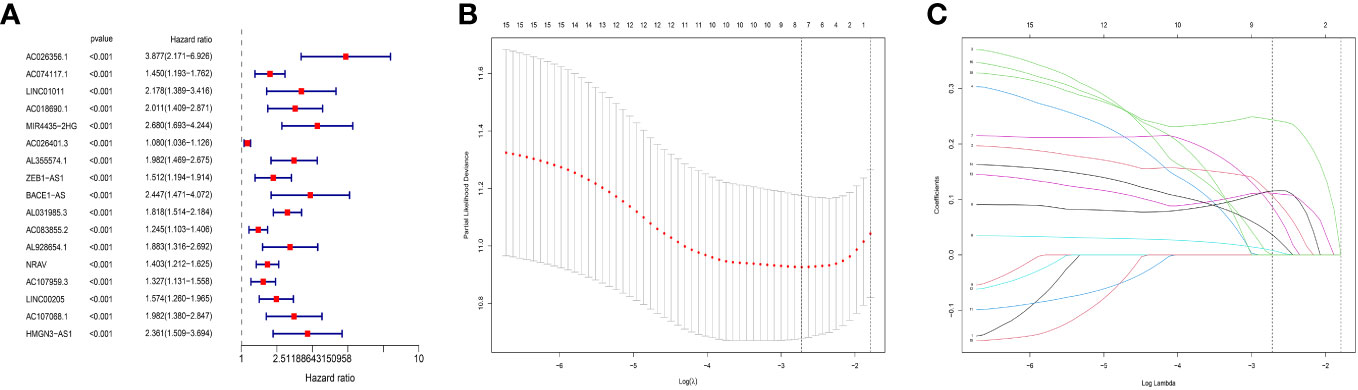
Figure 1 Prognostic model construction. (A) Univariate COX analysis. 17 predictive N6-methyladenosine- and neutrophil extracellular traps- related long non-coding RNA (MNlncRNAs) were chosen. MNlncRNAs in red are classified as high risk. (B, C) The least absolute selection operator (LASSO) regression analysis. LASSO regression analysis was used to select additional predictive MNlncRNAs.
Figures 2A, B demonstrated how we classified HCC samples as high- or low-risk. The risk score increased the percentage of patients who died (Figures 2C, D). Besides, among those at high risk, all the model MNlncRNAs were substantially expressed in both cohorts (Figures 2E, F). The results of the survival research showed that the high-risk group’s outcome was much poorer (Figures 3A, B). For the training cohort, the AUCs were 0.740, 0.755, and 0.749 at 1, 3, and 5 years, respectively (Figure 3C). For the test cohort, the AUCs were 0.795, 0.775, and 0.863 at 1, 3, and 5 years, respectively (Figure 3D). Finally, to further evaluate the prognostic value of the model, we compared it with clinical characteristics (TNM stage, age, grade and gender) and found that the model had the highest predictive accuracy (Figures 3E, F).
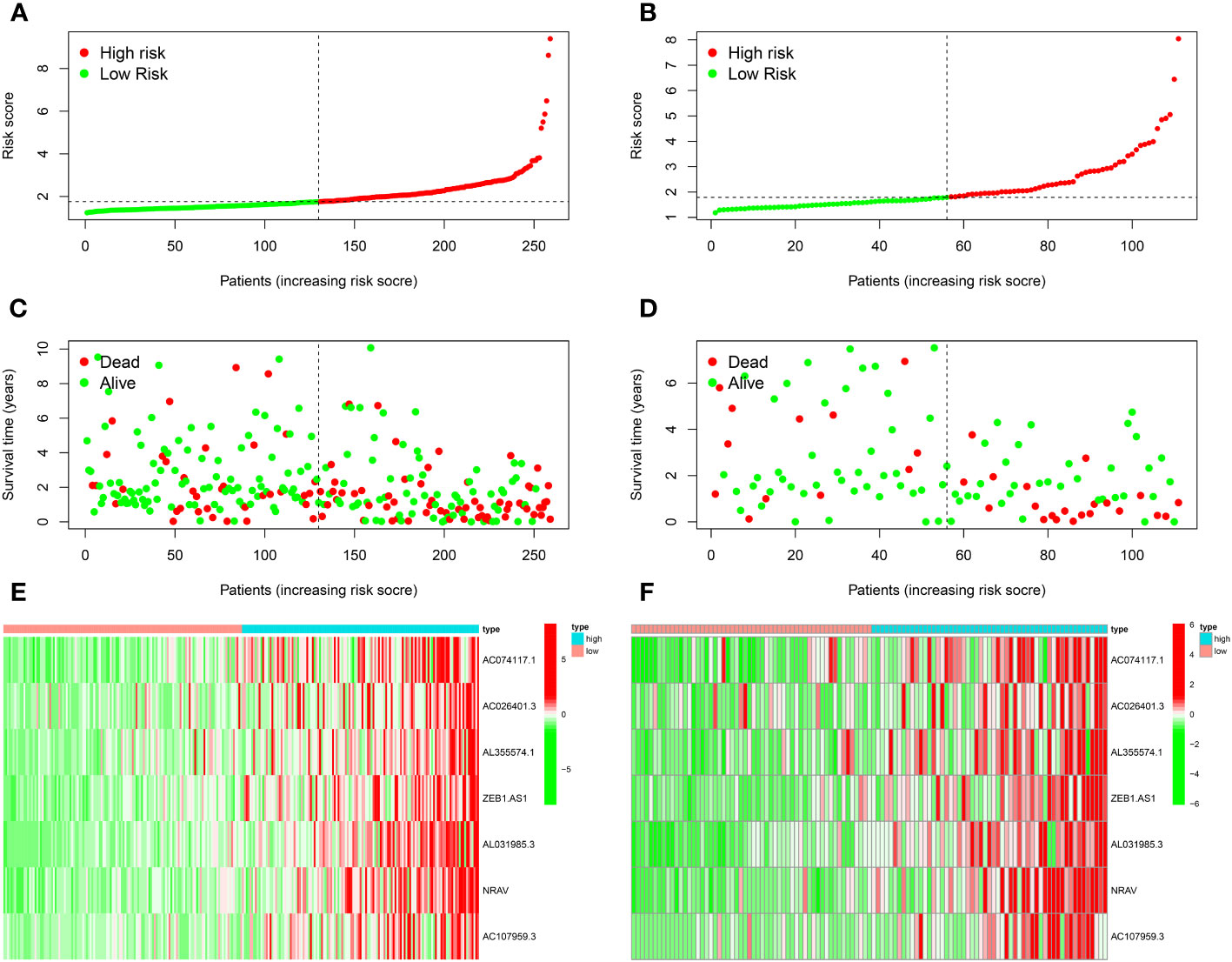
Figure 2 Prognostic model evaluation. (A, B) The risk score of training (A) and test (B) cohorts. (C, D) The correlation between survival status and riskscore. (E, F) Expression heatmap of 7 model MNlncRNAs.
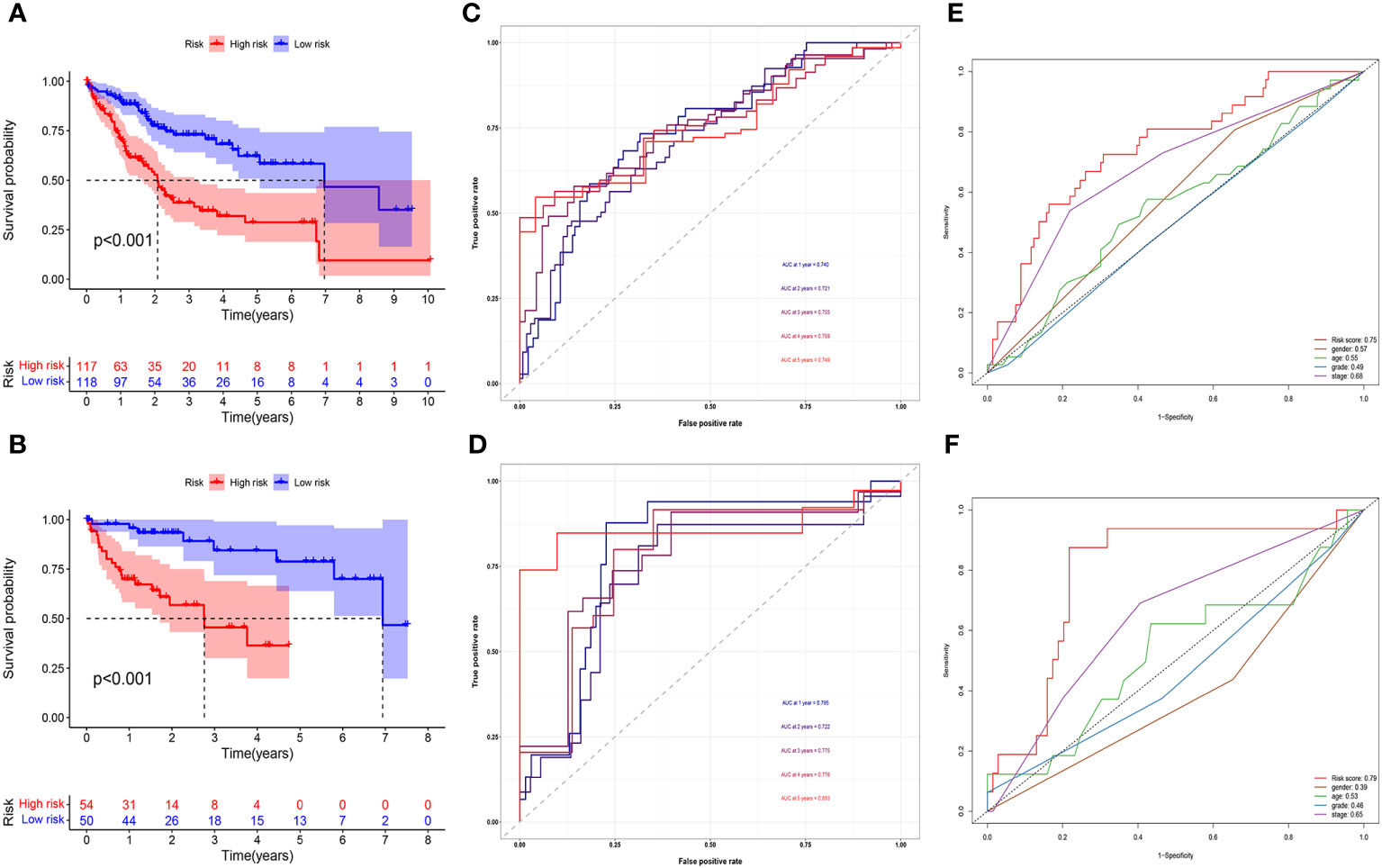
Figure 3 Prognostic model evaluation. (A, B) High-risk patients did poorly in both the training (A) and test cohorts (B). (C, D) The AUC in both cohorts was essentially in the 0.7–0.9 range. (E, F) Risk score had the highest predictive accuracy compared with clinical characteristics in both cohorts.
3.3 Functional analysis
The key roles of these genes, as determined by the results of the GO enrichment study, were stimulation of mononuclear migration and leukocyte migration in BP, tertiary granule and secretory granule membrance in CC, and immune receptor activity and receptor activity in MF (Figure 4A). Besides, they mostly contributed to neutrophil extracellular trap formation, immune-related signaling pathways, and cytokine−cytokine receptor interaction by the KEGG analysis (Figure 4B). The GSVA analysis showed that they also contributed to leukocyte migration, signal transmission, and apoptosis (Figure 4C).
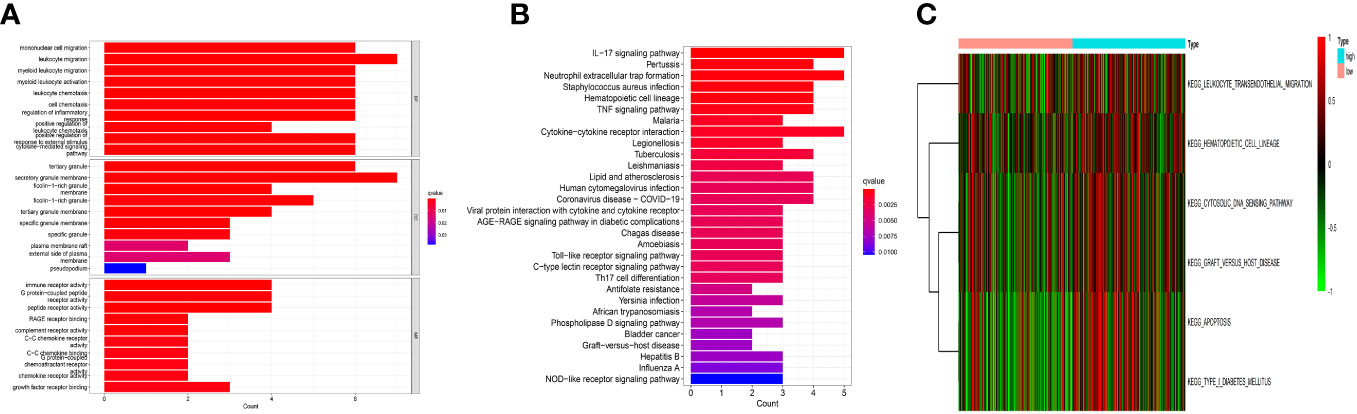
Figure 4 Functional analysis. (A) The Gene Ontology (GO) analysis. These genes functions were stimulation of mononuclear migration and leukocyte migration in BP, tertiary granule and secretory granule membrance in CC, and immune receptor activity and receptor activity in MF. (B) The Kyoto Encyclopedia of Genes and Genomes (KEGG) analysis. They were mainly involved in cytokine−cytokine receptor interaction, neutrophil extracellular trap formation, and immune-related signaling pathways. (C) The gene set variation analysis (GSVA). They were mainly involved in leukocyte migration, signal transmission, and apoptosis.
3.4 Model validation in vitro and vivo
To further verify the reliability of the risk model, we detected the expression of the 7 genes by using qRT-PCR. The results showed significantly increased expression of these genes in HCC cells (PLC, SK, LM3, HepG2 and HuH-7) (Figures 5A–G) and tumor tissues from 20 patients diagnosed with hepatocellular carcinoma (Figures 5H–N), consistent with these of the bioinformatics analysis. Afterwards, we calculated the risk score of hepatocellular carcinoma cell lines according above calculation method. As shown in Figure 6A, the risk scores of HepG2 (2.4662) and LM3 (2.655) were relatively close. Similarly, the risk scores of PLC (1.5669), SK (1.5028) and HuH-7 (1.3849) were also relatively close. Based on our model, we believe that cells with similar risk scores have little difference in biological characteristics, so we selected cells with the highest and lowest risk scores for functional experiments. Subsequently, we further compared the ability of proliferation and metastasis of LM3 and HuH-7. Colony formation and EDU proliferation assay indicted that LM3 cells proliferation rate was significantly higher than HuH-7 cells (Figures 6B, C). Meanwhile transwell migration and invasion assay was performed, which showed that LM3 cells had higher metastatic potential (Figure 6D). The results confirmed that LM3 had relatively higher malignancy than other cell lines, suggesting that patients with higher risk score had worse prognosis.
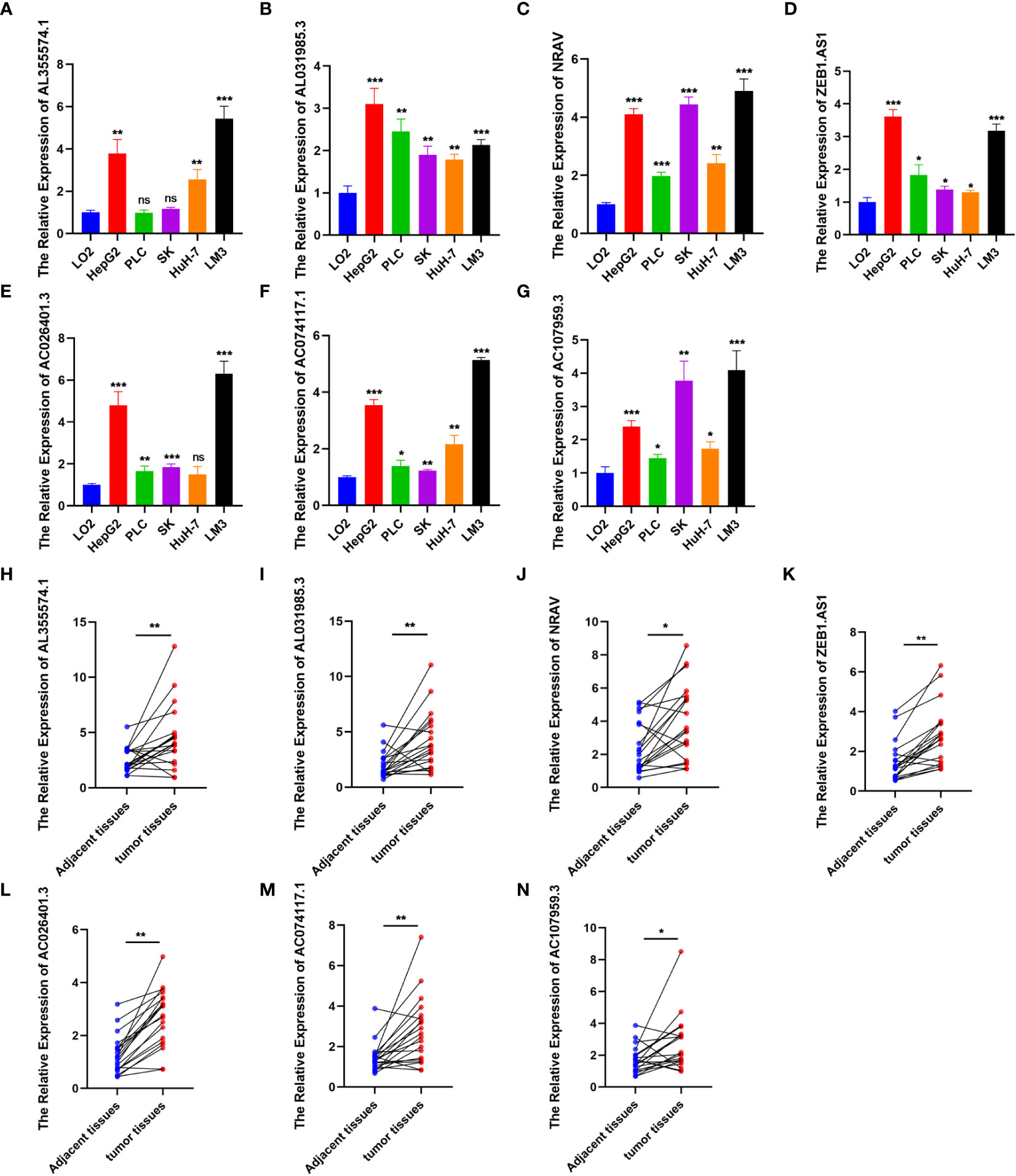
Figure 5 Expression level analysis. (A–G) The expression of AL355574.1, AL031985.3, NRAV, ZEB1.AS1, AC026401.3, AC074117.1 and AC107959.3 in normal liver cell and HCC cells were detected by RT-qPCR. (H–N) Paired comparison of the differential expression of the 7 lncRNAs between 20 HCC tissues and adjacent tissues. *p<0.05; **p<0.01; ***p<0.001; ns, not significant.
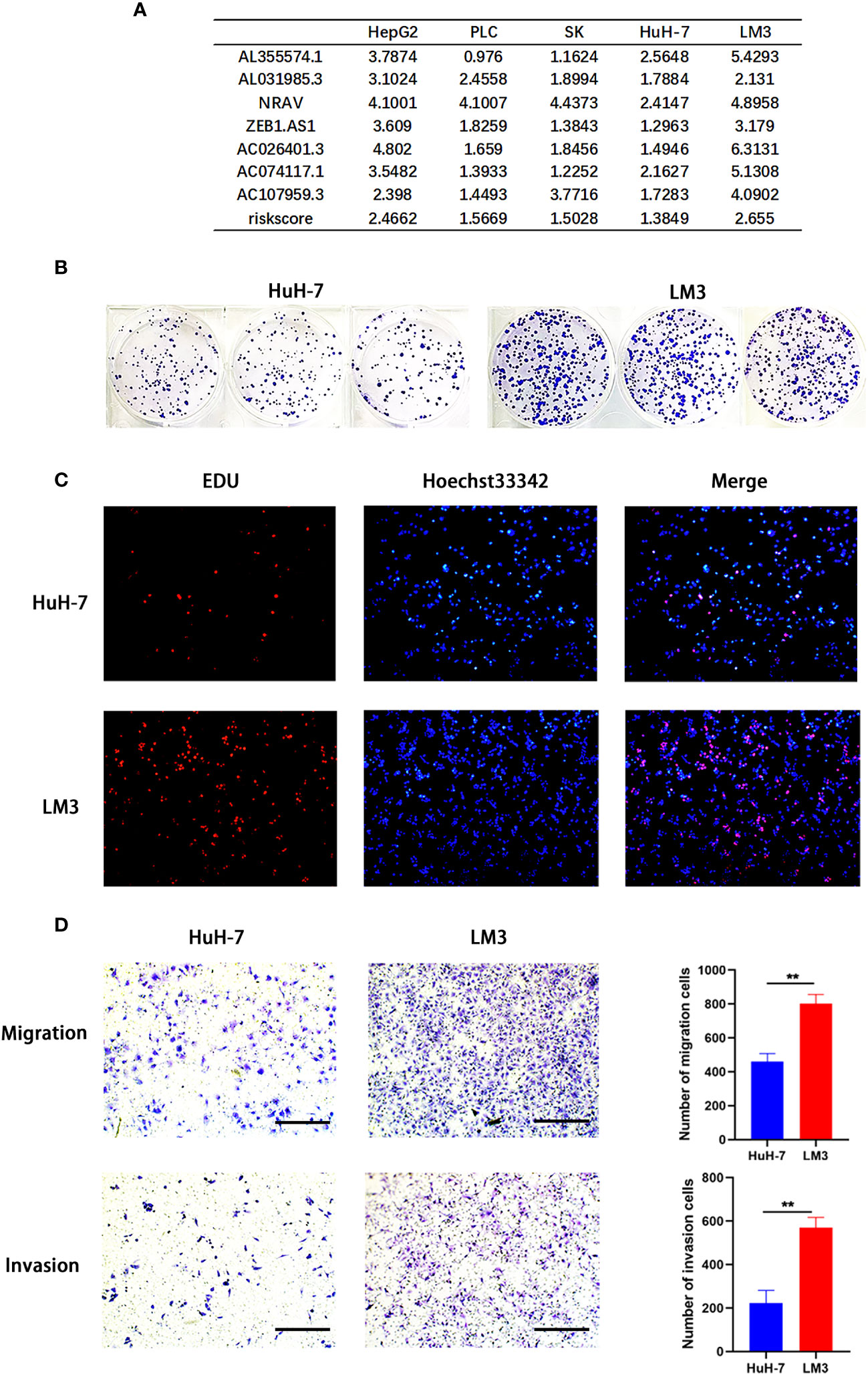
Figure 6 Model validation in vitro. (A) Application of risk model to hepatocellular carcinoma (HCC) cell lines. (B–D) Colony formation assay, EDU assay, and transwell migration and invasion assay (Scale bar: 100mm) showing that LM3 had relatively higher malignancy than HuH-7. **p<0.01.
3.5 Immunoassay analysis
The immune microenvironment had a significant impact on tumor development. B cell, monocyte, T cell, and macrophage immunocorrelation research revealed a high correlation between risk scores and each of these cell types (Figure 7A). In the low-risk group, the Cytolytic_activity, the Type-II-IFN_Reponse, and Type-I-IFN-Response were more activate; In the high-risk group, the MHC_class-I were more activated (Figure 7B). Significant differences in immunological checkpoint gene expression were found between the two groups, as demonstrated by Figure 7C. In the low-risk group, the immune system was more active, indicating that immunotherapy could be beneficial for these individuals. Furthermore, the high-risk group had higher levels of the vast majority of immune checkpoint genes, which raises the possibility that the immunological milieu may differ between the two groups.
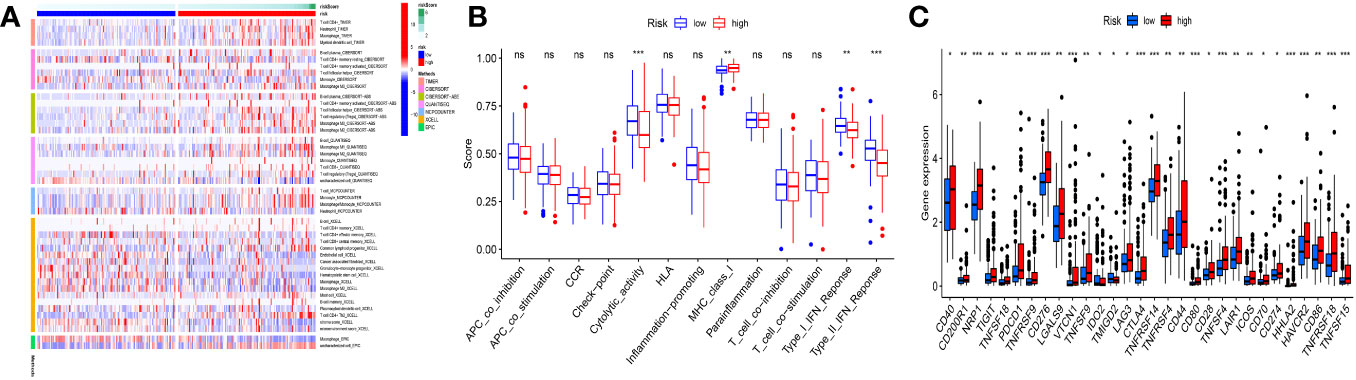
Figure 7 Immunoassay analysis. (A) Significant correlations existed between risk scores and B cells, T cells, monocyte cells, and macrophage cells. (B) Low-risk populations have more active immune systems. (C) Most immune checkpoint genes had increased expression in the high-risk group. *p<0.05; **p<0.01; ***p<0.001; ns, not significant.
3.6 Drug sensitivity analysis
We carry out drug susceptibility testing to find effective medications for targeted therapy. After analysis, in the high-risk group, Camptothecin and Mitomycin.C, were more sensitive (Figures 8A, B). Doxorubicin, Refametinib, and Sorafenib were more sensitive in the low-risk group (Figures 8C–E).
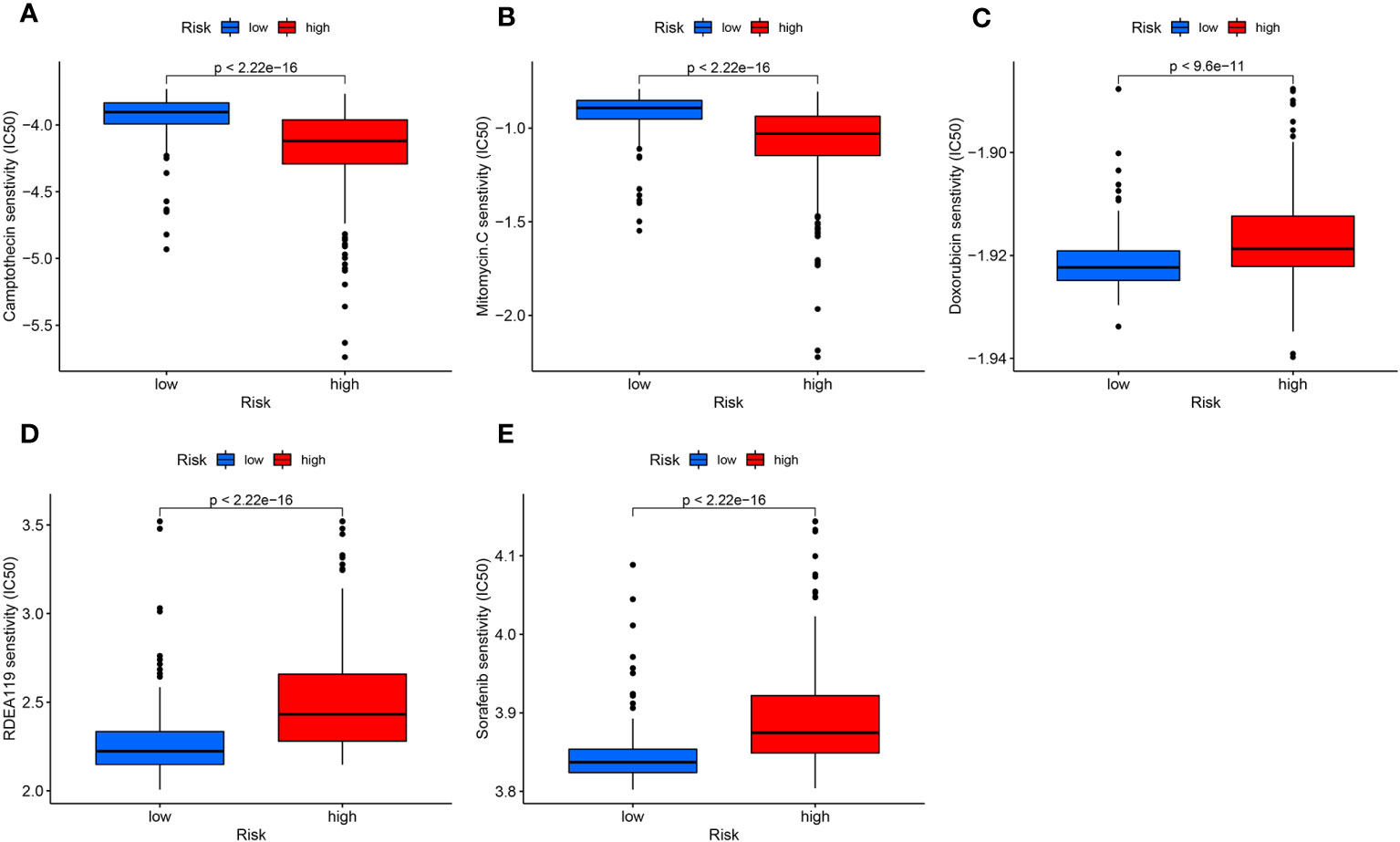
Figure 8 Drug sensitivity analysis. (A, B) Camptothecin (A) and Mitomycin.C (B) sensitivity was higher in the high-risk group. (C-E) Doxorubicin (C), Refametinib (D), and Sorafenib (E) were more toxic to the low-risk group.
4 Discussion
We used in-depth bioinformatics analysis to investigate the significance of lncRNAs associated with m6A and NETs in HCC in this work. Based on the TCGA database, we constructed a prognostic model based on MNlncRNAs expression, which can accurately predict their prognosis and stratify HCC patients. Additionally, our research revealed substantial variations in the function of MNlncRNAs in the immunological milieu of HCC, which may provide HCC patients a new therapy predictor. By finding more sensitive drugs, drug sensitivity analysis helps stratify the treatment of HCC.
LncRNAs are connected to carcinogenesis and metastasis through aberrant expression, and they are a hub in the area of cancer diagnosis, therapy, and prognosis evaluation (35, 36). LncRNA can promote or inhibit tumor progression. There have been reports that certain lncRNAs might serve as biomarkers for HCC patients with high sensitivity or be involved in important pathways of tumor regulation (37). It was shown that lncRNA-PDPK2P promotes the progression of HCC by interacting with PDK1, thereby affecting the value-added and migration of HCC (38). Prognostic markers of lncRNAs can be used to differentiate immunotherapy responses in cancer patients (39). Although lncRNA-based prognostic models are beneficial for the prognostic assessment of HCC patients, they have the disadvantage of many variables and lack accuracy (37). To increase the precision of lncRNA prognostic models, we tried to combine m6A and NETs inspired by the study of Huang et al (24). Thus, we first identified a set of specific lncRNAs linked to m6A and NETs genes in our study, which were used to construct MNlncRNAs markers. After combining the two prognostic biomarkers, we constructed a prognostic model with superior accuracy, with an AUC value for survival prediction essentially greater than 0.7. In conclusion, the construction of MNlncRNAs-based prognostic models is a great innovation that has superior predictive power than common clinical prognostic models.
According to preliminary results, the prognostic model’s model lncRNAs have been linked to the illness’s onset and progression. AC074117.1 was regulated by super-enhancers, and its silencing significantly slowed the growth of lung cancer cells (40). Yao et al. constructed a 4-lncRNA model to estimate the prognosis of individuals with breast cancer in which AC026401.3 played a key role (41). In the 7-lncRNA prognostic model developed by Yuan et al., AL355574.1 was a key component to assess the prognosis of gastric cancer (42). It has been demonstrated that increased ZEB1.AS1 expression is linked to tumor development and metastasis (43). The study by Li et al. screened 15 lncRNAs to estimate the prognosis of individuals with colorectal cancer, and AL031985.3 played a key role in this (44). NRAV promoted pancreatic cancer progression by targeting miR-299-3p (45). Huang et al. constructed an 8-lncRNA model to estimate the prognosis of individuals with HCC in which AC107959.3 played an important role (46). These 7 lncRNAs were included in the prognostic model used in this work, which can help us better understand tumor development.
Death in patients with tumors is more often attributed to metastases than to the primary tumor. The majority of patients die as a result of organ failure, cancer-associated thrombosis, or other problems connected to tumor spread (47). There is growing evidence that heterogeneity remains between tumors in the same tissue. The energy and nourishment that the tumor microenvironment provides to sustain tumor development and spread is essential for tumor growth (48). Neutrophils, the most common cells in the human immune system, may serve as a bridge between the tumor parenchyma and the immunological microenvironment. Neutrophil extracellular traps are an important mechanism in innate immunity that is implicated in cancer development and has recently emerged as a hotspot (49). NETs are expected to play an important function in the tumor immune microenvironment. NETs are a distinct kind of neutrophil that protects tumor cells from immune assault, activates dormant tumor cells, and promotes tumor invasion and metastasis (50). The colocalization between tumor cells and NETs is closely linked, which would also promote tumor progression (50). Recent studies have provided a preliminary exploration of the mechanisms by which NETs promote tumor metastasis. NETs can upregulate the TLR9 pathway to promote the progression of diffuse large B lymphoma (51). CTSC enzymes released by breast cancer cells can control NET development, thus promoting lung metastasis of breast cancer (52). The tumor microenvironment of HCC can promote early metastasis of HCC by facilitating tumor cell invasion and migration (53). In recent years, advances in epigenetic techniques provide researchers with new insights into tumor research (54). An increasing number of studies have focused on tumorigenic progression and m6A modifications of lncRNAs in innate immunity (55). The most prevalent RNA modification, M6A, is crucial for many cellular processes and biological functions (56, 57). Factors that affect m6A alteration are linked to both specific malignancies and abnormal immune regulation (58, 59). The tumor microenvironment is highly correlated with M6A alterations (60). Additionally, tumor immunotherapy benefits from aspects of tumor microenvironment cell invasion mediated by m6A regulator (61). Our findings revealed a set of lncRNA signatures associated with m6A and NETs that helped us understand the progression of HCC. The risk score classified all HCC patients into two groups. The majority of cancer progression occurred in high-risk individuals, whereas other patients lived longer.
HCC is a disease that is fueled by inflammation, and a sizable portion of HCC patients show indicators of the inflammatory response (62). Stromal and tumor cells can produce immunosuppression associated with chronic inflammatory factors (63). Cells of bone marrow origin, including tumor-associated neutrophils (TANs), promote tumor progression (64). It has been demonstrated that TANs encourage HCC development, progression, and sorafenib resistance by enlisting macrophages and T cells into the tumor’s microenvironment (65). TAN-induced HCC stem-like cells are active in signaling, CXCL5 secretion, and recruitment of more TAN infiltration (66). Also, a poor prognosis is linked to the presence of neutrophils in the tumor microenvironment (67). Importantly, the knockdown of CXCR2, a key chemotactic receptor for neutrophils, to inhibit immune infiltration of neutrophils can lead to a T cell-dependent suppression of tumor growth (68). Therefore, understanding the immune infiltration of neutrophils is crucial to the diagnosis and management of HCC. Our study stratified patients according to modeled risk scores. The high-risk patients had more immune infiltrating cells, and the majority of immunological checkpoint-related genes have increased expression, indicating that immunotherapy may help the high-risk patients more. For patients in the two groups, we screened for drugs that are sensitive to their treatment, which helped to individualize the treatment for patients.
However, our study possesses certain limitations. The first limitation pertains to the inadequate elucidation of the mechanisms underlying the functionality of these m6A and neutrophil extracellular traps-related lncRNAs. Their role in shaping the tumor microenvironment and tumor growth and progression remains ambiguous, necessitating further investigation. Furthermore, this model is constructed based on the TCGA public database and lacks validation with a larger sample size. Despite these limitations, it is the initial model created incorporating lncRNAs associated with m6A and NETs. It provides information for studying the metabolism of HCC tumors and aids in the treatment of HCC patients.
5 Conclusion
Based on m6A- and NETs-related lncRNAs, a prognostic model of HCC was established. This model can accurately predict the immune microenvironment and prognosis of HCC patients. In addition, our findings could result in new approaches.
Data availability statement
The original contributions presented in the study are included in the article/Supplementary Material. Further inquiries can be directed to the corresponding authors.
Ethics statement
The studies involving humans were approved by The Second Affiliated Hospital of Nanjing Medical University. The studies were conducted in accordance with the local legislation and institutional requirements. The participants provided their written informed consent to participate in this study.
Author contributions
TZ, WW, and XG designed the study. XG and WB were involved in database search and statistical analyses. TZ and NL were involved in the writing of the manuscript. NL and JZ were responsible for the submission of the final version of the paper. All authors contributed to the article and approved the submitted version.
Conflict of interest
The authors declare that the research was conducted in the absence of any commercial or financial relationships that could be construed as a potential conflict of interest.
Publisher’s note
All claims expressed in this article are solely those of the authors and do not necessarily represent those of their affiliated organizations, or those of the publisher, the editors and the reviewers. Any product that may be evaluated in this article, or claim that may be made by its manufacturer, is not guaranteed or endorsed by the publisher.
Supplementary material
The Supplementary Material for this article can be found online at: https://www.frontiersin.org/articles/10.3389/fimmu.2023.1231543/full#supplementary-material
References
1. Sung H, Ferlay J, Siegel RL, Laversanne M, Soerjomataram I, Jemal A, et al. Global cancer statistics 2020: GLOBOCAN estimates of incidence and mortality worldwide for 36 cancers in 185 countries. CA: Cancer J Clin (2021) 71(3):209–49. doi: 10.3322/caac.21660
2. Kulik L, El-Serag HB. Epidemiology and management of hepatocellular carcinoma. Gastroenterology (2019) 156(2):477–491.e1. doi: 10.1053/j.gastro.2018.08.065
3. Anwanwan D, Singh SK, Singh S, Saikam V, Singh R. Challenges in liver cancer and possible treatment approaches. Biochim Biophys Acta Rev Cancer (2020) 1873(1):188314. doi: 10.1016/j.bbcan.2019.188314
4. Vogel A, Cervantes A, Chau I, Daniele B, Llovet JM, Meyer T, et al. Hepatocellular carcinoma: ESMO Clinical Practice Guidelines for diagnosis, treatment and follow-up. Ann Oncol Off J Eur Soc Med Oncol (2018) 29(Suppl 4):iv238–55. doi: 10.1093/annonc/mdy308
5. European Association for the Study of the Liver. EASL Clinical Practice Guidelines: Management of hepatocellular carcinoma. J Hepatol (2018) 69(1):182–236. doi: 10.1016/j.jhep.2018.03.019
6. Wei L, Lee D, Law CT, Zhang MS, Shen J, Chin DW, et al. Genome-wide CRISPR/Cas9 library screening identified PHGDH as a critical driver for Sorafenib resistance in HCC. Nat Commun (2019) 10(1):4681. doi: 10.1038/s41467-019-12606-7
7. Yang JD, Hainaut P, Gores GJ, Amadou A, Plymoth A, Roberts LR. A global view of hepatocellular carcinoma: trends, risk, prevention and management. Nat Rev Gastroenterol Hepatol (2019) 16(10):589–604. doi: 10.1038/s41575-019-0186-y
8. Sangro B, Sarobe P, Hervás-Stubbs S, Melero I. Advances in immunotherapy for hepatocellular carcinoma. Nat Rev Gastroenterol Hepatol (2021) 18(8):525–43. doi: 10.1038/s41575-021-00438-0
9. Chi Y, Wang D, Wang J, Yu W, Yang J. Long Non-Coding RNA Pathogenesis Cancers. Cells (2019) 8(9):1015. doi: 10.3390/cells8091015
10. Quinn JJ, Chang HY. Unique features of long non-coding RNA biogenesis and function. Nat Rev Genet (2016) 17(1):47–62. doi: 10.1038/nrg.2015.10
11. Liu S, Cao Q, An G, Yan B, Lei L. Identification of the 3-lncRNA signature as a prognostic biomarker for colorectal cancer. Int J Mol Sci (2020) 21(24):9359. doi: 10.3390/ijms21249359
12. Ping H, Jia X, Ke H. A novel ferroptosis-related lncRNAs signature predicts clinical prognosis and is associated with immune landscape in pancreatic cancer. Front Genet (2022) 13:786689. doi: 10.3389/fgene.2022.786689
13. Lan Y, Liu B, Guo H. The role of M(6)A modification in the regulation of tumor-related lncRNAs. Mol Ther Nucleic Acids (2021) 24:768–79. doi: 10.1016/j.omtn.2021.04.002
14. Chen M, Nie ZY, Wen XH, Gao YH, Cao H, Zhang SF. m6A RNA methylation regulators can contribute to malignant progression and impact the prognosis of bladder cancer. Bioscience Rep (2019) 39(12):BSR20192892. doi: 10.1042/bsr20192892
15. Sun T, Wu R, Ming L. The role of m6A RNA methylation in cancer. Biomedicine pharmacotherapy = Biomedecine pharmacotherapie (2019) 112:108613. doi: 10.1016/j.biopha.2019.108613
16. Bruni D, Angell HK, Galon J. The immune contexture and Immunoscore in cancer prognosis and therapeutic efficacy. Nat Rev Cancer (2020) 20(11):662–80. doi: 10.1038/s41568-020-0285-7
17. Giese MA, Hind LE, Huttenlocher A. Neutrophil plasticity in the tumor microenvironment. Blood (2019) 133(20):2159–67. doi: 10.1182/blood-2018-11-844548
18. Liang W, Ferrara N. The complex role of neutrophils in tumor angiogenesis and metastasis. Cancer Immunol Res (2016) 4(2):83–91. doi: 10.1158/2326-6066.Cir-15-0313
19. Arvanitakis K, Mitroulis I, Germanidis G. Tumor-associated neutrophils in hepatocellular carcinoma pathogenesis, prognosis, and therapy. Cancers (2021) 13(12):2899. doi: 10.3390/cancers13122899
20. Pruchniak MP, Demkow U. Potent NETosis inducers do not show synergistic effects in vitro. Central-European J Immunol (2019) 44(1):51–8. doi: 10.5114/ceji.2019.84017
21. Manda-Handzlik A, Bystrzycka W, Cieloch A, Glodkowska-Mrowka E, Jankowska-Steifer E, Heropolitanska-Pliszka E, et al. Nitric oxide and peroxynitrite trigger and enhance release of neutrophil extracellular traps. Cell Mol Life Sci CMLS (2020) 77(15):3059–75. doi: 10.1007/s00018-019-03331-x
22. Hu W, Lee SML, Bazhin AV, Guba M, Werner J, Nieß H. Neutrophil extracellular traps facilitate cancer metastasis: cellular mechanisms and therapeutic strategies. J Cancer Res Clin Oncol (2023) 149(5):2191–210. doi: 10.1007/s00432-022-04310-9
23. Qiu Y, Li J, Yao J, Meng J, Huang X, Zheng X, et al. Correlative study on the relationship between the expression of M6a-related genes and the prognosis and immunotherapy of soft tissue sarcoma. BioMed Res Int (2022) 2022:e5439023. doi: 10.1155/2022/5439023
24. Huang YQ, Liang CH, He L, Tian J, Liang CS, Chen X, et al. Development and validation of a radiomics nomogram for preoperative prediction of lymph node metastasis in colorectal cancer. J Clin Oncol (2016) 34(18):2157–64. doi: 10.1200/jco.2015.65.9128
25. Feng Z-H, Liang Y-P, Cen J-J, Yao H-H, Lin H-S, Li J-Y, et al. M6A-immune-related lncRNA prognostic signature for predicting immune landscape and prognosis of bladder cancer. J Transl Med (2022) 20(1):492. doi: 10.1186/s12967-022-03711-1
26. Ding W, Li B, Zhang Y, He L, Su J. A neutrophil extracellular traps-associated lncRNA signature predicts the clinical outcomes in patients with lung adenocarcinoma. Front Genet (2022) 13:1047231. doi: 10.3389/fgene.2022.1047231
27. Zhang Y, Guo L, Dai Q, Shang B, Xiao T, Di X, et al. A signature for pan-cancer prognosis based on neutrophil extracellular traps. J Immunother. Cancer (2022) 10(6):e004210. doi: 10.1136/jitc-2021-004210
28. Newman AM, Liu CL, Green MR, Gentles AJ, Feng W, Xu Y, et al. Robust enumeration of cell subsets from tissue expression profiles. Nat Methods (2015) 12(5):453–7. doi: 10.1038/nmeth.3337
29. Aran D, Hu Z, Butte AJ. xCell: digitally portraying the tissue cellular heterogeneity landscape. Genome Biol (2017) 18(1):220. doi: 10.1186/s13059-017-1349-1
30. Shimada K, Yoshida K, Suzuki Y, Iriyama C, Inoue Y, Sanada M, et al. Frequent genetic alterations in immune checkpoint-related genes in intravascular large B-cell lymphoma. Blood (2021) 137(11):1491–502. doi: 10.1182/blood.2020007245
31. Song D, Tian J, Han X, Li X. A model of seven immune checkpoint-related genes predicting overall survival for head and neck squamous cell carcinoma. Eur Arch oto-rhino-laryngology Off J Eur Fed Oto-Rhino-Laryngological Societies (EUFOS) affiliated German Soc Oto-Rhino-Laryngology - Head Neck Surg (2021) 278(9):3467–77. doi: 10.1007/s00405-020-06540-4
32. Jiang F, Wang XY, Wang MY, Mao Y, Miao XL, Wu CY, et al. An immune checkpoint-related gene signature for predicting survival of pediatric acute myeloid leukemia. J Oncol (2021) 2021:5550116. doi: 10.1155/2021/5550116
33. Tian M, Yang J, Han J, He J, Liao W. A novel immune checkpoint-related seven-gene signature for predicting prognosis and immunotherapy response in melanoma. Int Immunopharmacol (2020) 87:106821. doi: 10.1016/j.intimp.2020.106821
34. Geeleher P, Cox N, Huang RS. pRRophetic: an R package for prediction of clinical chemotherapeutic response from tumor gene expression levels. PloS One (2014) 9(9):e107468. doi: 10.1371/journal.pone.0107468
35. Danaeifar M. Recent advances in gene therapy: genetic bullets to the root of the problem. Clin Exp Med (2023) 23(4):1107–21. doi: 10.1007/s10238-022-00925-x
36. Bhan A, Soleimani M, Mandal SS. Long noncoding RNA and cancer: A new paradigm. Cancer Res (2017) 77(15):3965–81. doi: 10.1158/0008-5472.Can-16-2634
37. Huang Z, Zhou JK, Peng Y, He W, Huang C. The role of long noncoding RNAs in hepatocellular carcinoma. Mol Cancer (2020) 19(1):77. doi: 10.1186/s12943-020-01188-4
38. Pan W, Li W, Zhao J, Huang Z, Zhao J, Chen S, et al. lncRNA-PDPK2P promotes hepatocellular carcinoma progression through the PDK1/AKT/Caspase 3 pathway. Mol Oncol (2019) 13(10):2246–58. doi: 10.1002/1878-0261.12553
39. Liu X, Wang D, Han S, Wang F, Zang J, Xu C, et al. Signature of m5C-Related lncRNA for Prognostic Prediction and Immune Responses in Pancreatic Cancer. J Oncol (2022) 2022:7467797. doi: 10.1155/2022/7467797
40. Li M, Yang B, Li X, Ren H, Zhang L, Li L, et al. Identification of prognostic factors related to super enhancer-regulated ceRNA network in metastatic lung adenocarcinoma. Int J Gen Med (2021) 14:6261–75. doi: 10.2147/ijgm.S332317
41. Yao ZY, Xing C, Cai S, Xing XL. Development and validation of ferroptosis-related lncRNAs as prognosis and diagnosis biomarkers for breast cancer. BioMed Res Int (2022) 2022:2390764. doi: 10.1155/2022/2390764
42. Yuan M, Jia Y, Xing Y, Wang Y, Liu Y, Liu X, et al. Screening and validation of platelet activation-related lncRNAs as potential biomarkers for prognosis and immunotherapy in gastric cancer patients. Front Genet (2022) 13:965033. doi: 10.3389/fgene.2022.965033
43. Chen C, Feng Y, Wang X. LncRNA ZEB1-AS1 expression in cancer prognosis: Review and meta-analysis. Clinica chimica acta; Int J Clin Chem (2018) 484:265–71. doi: 10.1016/j.cca.2018.06.007
44. Li N, Shen J, Qiao X, Gao Y, Su HB, Zhang S. Long non-coding RNA signatures associated with ferroptosis predict prognosis in colorectal cancer. Int J Gen Med (2022) 15:33–43. doi: 10.2147/ijgm.S331378
45. Wang HQ, Qian CH, Guo ZY, Li PM, Qiu ZJ. Long noncoding RNA negative regulator of antiviral response contributes to pancreatic ductal adenocarcinoma progression via targeting miR-299-3p. World J Gastroenterol (2022) 28(35):5141–53. doi: 10.3748/wjg.v28.i35.5141
46. Huang ZL, Li W, Chen QF, Wu PH, Shen LJ. Eight key long non-coding RNAs predict hepatitis virus positive hepatocellular carcinoma as prognostic targets. World J gastrointestinal Oncol (2019) 11(11):983–97. doi: 10.4251/wjgo.v11.i11.983
47. Cedervall J, Zhang Y, Olsson AK. Tumor-induced NETosis as a risk factor for metastasis and organ failure. Cancer Res (2016) 76(15):4311–5. doi: 10.1158/0008-5472.Can-15-3051
48. Di Donato M, Zamagni A, Galasso G, Di Zazzo E, Giovannelli P, Barone MV, et al. The androgen receptor/filamin A complex as a target in prostate cancer microenvironment. Cell Death Dis (2021) 12(1):127. doi: 10.1038/s41419-021-03402-7
49. Demkow U. Neutrophil extracellular traps (NETs) in cancer invasion, evasion and metastasis. Cancers (2021) 13(17):4495. doi: 10.3390/cancers13174495
50. Herre M, Cedervall J, Mackman N, Olsson AK. Neutrophil extracellular traps in the pathology of cancer and other inflammatory diseases. Physiol Rev (2023) 103(1):277–312. doi: 10.1152/physrev.00062.2021
51. Nie M, Yang L, Bi X, Wang Y, Sun P, Yang H, et al. Neutrophil extracellular traps induced by IL8 promote diffuse large B-cell lymphoma progression via the TLR9 signaling. Clin Cancer Res (2019) 25(6):1867–79. doi: 10.1158/1078-0432.Ccr-18-1226
52. Xiao Y, Cong M, Li J, He D, Wu Q, Tian P, et al. Cathepsin C promotes breast cancer lung metastasis by modulating neutrophil infiltration and neutrophil extracellular trap formation. Cancer Cell (2021) 39(3):423–437.e7. doi: 10.1016/j.ccell.2020.12.012
53. Fu Y, Liu S, Zeng S, Shen H. From bench to bed: the tumor immune microenvironment and current immunotherapeutic strategies for hepatocellular carcinoma. J Exp Clin Cancer Res CR (2019) 38(1):396. doi: 10.1186/s13046-019-1396-4
54. Shen GQ, Aleassa EM, Walsh RM, Morris-Stiff G. Next-generation sequencing in pancreatic cancer. Pancreas (2019) 48(6):739–48. doi: 10.1097/mpa.0000000000001324
55. Yi YC, Chen XY, Zhang J, Zhu JS. Novel insights into the interplay between m(6)A modification and noncoding RNAs in cancer. Mol Cancer (2020) 19(1):121. doi: 10.1186/s12943-020-01233-2
56. Wei W, Ji X, Guo X, Ji S. Regulatory role of N(6) -methyladenosine (m(6) A) methylation in RNA processing and human diseases. J Cell Biochem (2017) 118(9):2534–43. doi: 10.1002/jcb.25967
57. Chen M, Wei L, Law CT, Tsang FH, Shen J, Cheng CL, et al. RNA N6-methyladenosine methyltransferase-like 3 promotes liver cancer progression through YTHDF2-dependent posttranscriptional silencing of SOCS2. Hepatol (Baltimore Md.) (2018) 67(6):2254–70. doi: 10.1002/hep.29683
58. Gu Y, Wu X, Zhang J, Fang Y, Pan Y, Shu Y, et al. The evolving landscape of N(6)-methyladenosine modification in the tumor microenvironment. Mol Ther (2021) 29(5):1703–15. doi: 10.1016/j.ymthe.2021.04.009
59. Uddin MB, Wang Z, Yang C. The m(6)A RNA methylation regulates oncogenic signaling pathways driving cell Malignant transformation and carcinogenesis. Mol Cancer (2021) 20(1):61. doi: 10.1186/s12943-021-01356-0
60. Tang R, Zhang Y, Liang C, Xu J, Meng Q, Hua J, et al. The role of m6A-related genes in the prognosis and immune microenvironment of pancreatic adenocarcinoma. PeerJ (2020) 8:e9602. doi: 10.7717/peerj.9602
61. Finotello F, Trajanoski Z. Quantifying tumor-infiltrating immune cells from transcriptomics data. Cancer immunology immunotherapy CII (2018) 67(7):1031–40. doi: 10.1007/s00262-018-2150-z
62. Giraud J, Chalopin D, Blanc JF, Saleh M. Hepatocellular carcinoma immune landscape and the potential of immunotherapies. Front Immunol (2021) 12:655697. doi: 10.3389/fimmu.2021.655697
63. Liu B, Salgado OC, Singh S, Hippen KL, Maynard JC, Burlingame AL, et al. The lineage stability and suppressive program of regulatory T cells require protein O-GlcNAcylation. Nat Commun (2019) 10(1):354. doi: 10.1038/s41467-019-08300-3
64. Kitamura T, Qian BZ, Pollard JW. Immune cell promotion of metastasis. Nat Rev Immunology (2015) 15(2):73–86. doi: 10.1038/nri3789
65. Zhou SL, Zhou ZJ, Hu ZQ, Huang XW, Wang Z, Chen EB, et al. Tumor-associated neutrophils recruit macrophages and T-regulatory cells to promote progression of hepatocellular carcinoma and resistance to sorafenib. Gastroenterology (2016) 150(7):1646–1658.e17. doi: 10.1053/j.gastro.2016.02.040
66. Zhou SL, Yin D, Hu ZQ, Luo CB, Zhou ZJ, Xin HY, et al. A positive feedback loop between cancer stem-like cells and tumor-associated neutrophils controls hepatocellular carcinoma progression. Hepatol (Baltimore Md.) (2019) 70(4):1214–30. doi: 10.1002/hep.30630
67. Shen M, Hu P, Donskov F, Wang G, Liu Q, Du J. Tumor-associated neutrophils as a new prognostic factor in cancer: a systematic review and meta-analysis. PloS One (2014) 9(6):e98259. doi: 10.1371/journal.pone.0098259
Keywords: long non-coding RNA, N6-methyladenosine, neutrophil extracellular traps, hepatocellular carcinoma, bioinformatics
Citation: Zhan T, Wang W, Guan X, Bao W, Lu N and Zhang J (2023) Construction of an m6A- and neutrophil extracellular traps-related lncRNA model to predict hepatocellular carcinoma prognosis and immune landscape. Front. Immunol. 14:1231543. doi: 10.3389/fimmu.2023.1231543
Received: 30 May 2023; Accepted: 21 September 2023;
Published: 05 October 2023.
Edited by:
Jadwiga Jablonska, Essen University Hospital, GermanyCopyright © 2023 Zhan, Wang, Guan, Bao, Lu and Zhang. This is an open-access article distributed under the terms of the Creative Commons Attribution License (CC BY). The use, distribution or reproduction in other forums is permitted, provided the original author(s) and the copyright owner(s) are credited and that the original publication in this journal is cited, in accordance with accepted academic practice. No use, distribution or reproduction is permitted which does not comply with these terms.
*Correspondence: Jianping Zhang, ZHJ6aGFuZ2pwQG5qbXUuZWR1LmNu; Na Lu, bG4yMjQ4NDU3MzA2QDE2My5jb20=
†These authors have contributed equally to this work