- 1Department of General Surgery, Renmin Hospital of Wuhan University, Wuhan, China
- 2Key Laboratory of Hubei Province for Digestive System Disease, Wuhan, China
Objective: Our study represents the first meta-analysis conducted to evaluate the prognostic utility of the baseline prognostic nutritional index (PNI) in patients with gastrointestinal cancer (GIC) who received immune checkpoint inhibitor (ICI) therapy.
Methods: We searched PubMed, the Cochrane Library, EMBASE, and Google Scholar until April 23, 2023, to obtain relevant articles for this study. Our analysis examined several clinical outcomes, including overall survival (OS), progression-free survival (PFS), objective response rate (ORR), and disease control rate (DCR).
Results: In this analysis, a total of 17 articles with 2883 patients were included. Our pooled results indicated that patients with high PNI levels had longer OS (HR: 0.530, 95% CI: 0.456-0.616, p < 0.001) and PFS (HR: 0.740, 95% CI: 0.649-0.844, p < 0.001), as well as higher ORR (OR: 1.622, 95% CI: 1.251-2.103, p < 0.004) and DCR (OR: 1.846, 95% CI: 1.428-2.388, p < 0.001). Subgroup analysis showed that PNI cutoff values of 40 to 45 showed greater predictive potential. Subgroup analysis also confirmed that the above findings still hold true in patients with esophageal cancer, gastric cancer, and hepatocellular carcinomas.
Conclusion: The PNI were reliable predictors of outcomes in GIC patients treated with ICIs.
1 Introduction
Approximately one-fourth of all cancer cases and one-third of all cancer-related deaths worldwide can be attributed to gastrointestinal cancers (GIC) (1). Systemic therapy remains the cornerstone of treatment for patients with locally advanced or metastatic GIC (2). However, there is a critical need for strategies to reduce metastasis and improve survival. The introduction of immune checkpoint inhibitors (ICIs) over the past five years has resulted in significant advances in the treatment of advanced GIC patients, achieving durable antitumor immune responses and improvements in overall survival (OS) (3–5). But because of the low response rate, scientists are looking for new potential biomarkers that can predict treatment outcomes (3, 6). The identification of well-characterized and predictive biomarkers would facilitate personalized treatment selection based on the anticipated efficacy of therapy and avoid the cost of ineffective treatment.
Numerous studies have demonstrated the relationship between nutritional status and cancer prognosis (7–11). Patients with GIC are particularly affected by nutritional status due to their anatomical features (12). The prognostic nutrition index (PNI) is an index that utilizes the levels of serum albumin and peripheral blood lymphocyte count, initially created to forecast the probability of postoperative complications in surgical patients by evaluating their nutritional status before the operation (13). Recent studies have demonstrated the high accuracy of PNI in predicting treatment outcomes for various cancers, especially GIC (14, 15). Immune system function plays a critical role in the efficacy of ICIs, and the levels of serum albumin and lymphocytes are significant indicators of immune system function.
Notably, the association between PNI levels and the prognosis of GIC patients treated with ICIs remains controversial, and no meta-analysis has been conducted to date. Hence, the aim of this study was to systematically evaluate the predictive value of PNI in ICI-treated GIC patients. The findings of this study can aid in developing effective treatment strategies that facilitate the administration of precise, cost-effective treatments with minimal adverse effects.
2 Methods
2.1 Literature search strategies
The analysis performed in this study was conducted following the guidelines of the PRISMA statement (16). On April 23, 2023, a comprehensive literature search was carried out using PubMed, EMBASE, and the Cochrane Library. Various search terms, including MeSH terms and keywords, were used to retrieve relevant studies, such as “Immune Checkpoint Inhibitors [MeSH]”, “PD-1 Inhibitors”, “PD-L1 Inhibitors”, “CTLA-4 Inhibitors”, “Pembrolizumab”, “Nivolumab”, “Atezolizumab”, “Ipilimumab”, “Avelumab”, “Tremelimumab”, “Durvalumab”, “Cemiplimab”, “Prognostic Nutritional Index”, “PNI”. Search restricted to English literature. A detailed description of the search strategies is provided in Table S1. Additionally, gray literature was searched using Google Scholar, and the reference lists of eligible studies were screened manually.
2.2 Inclusion and exclusion criteria
In our study, we strictly included research articles that met the following criteria: patients who were diagnosed with GIC underwent treatment with ICIs, and the prognostic value of PNI was evaluated. Furthermore, these articles reported on at least one of the following outcomes: overall survival (OS), progression-free survival (PFS), objective response rate (ORR), and disease control rate (DCR). We excluded conference abstracts, comments, and case reports from our analysis. In situations where studies had overlapping patients, we prioritized those with the most comprehensive data and robust methodology (3).
2.3 Data extraction and quality assessment
In this study, we gathered diverse information from the chosen articles, including the names of the authors, year of publication, duration and location of the study, drugs used for treatment, cancer type, sample size, patient age and gender, and relevant cut-off values and outcomes. We placed greater emphasis on obtaining data from multivariate analyses of hazard ratios (HR) compared to univariate analyses. We also employed the Newcastle-Ottawa Scale (NOS) to appraise the quality of observational studies and classified those with a NOS score of 6 or above as high-quality literature (17). All of the above steps were completed and cross-checked independently by two authors, with decisions sought from the corresponding author on points of dispute.
2.4 Statistical methods
Statistical analyses were performed using Stata 15.0 software. To assess heterogeneity, we utilized the chi-squared test. We employed a random effects model when the p-value < 0.1 or the I2 statistic was > 50%, and a fixed effects model otherwise. We estimated publication bias using both Egger’s and Begg’s tests, and if bias was detected, we utilized the “trim and fill” method to evaluate the influence of the bias on the pooled results. Furthermore, we conducted a sensitivity analysis by excluding each study independently to assess the robustness of the results. p < 0.05 was considered statistically significant.
3 Results
3.1 Characteristics of studies
After excluding duplicates and screening titles and abstracts, we identified 25 articles for full-text evaluation, among which 17 met the eligibility criteria, resulting in a total of 2883 patients (18–34). The PRISMA flowchart in Figure 1 depicts the study selection process. Table 1 provides a comprehensive overview of the characteristics of the eligible studies. We assessed the risk of bias using the Newcastle-Ottawa Scale (NOS), with scores ranging from 6 to 8, indicating a low risk of bias in all included studies. Of the 17 studies, 16 were retrospective, and one was prospective. Four studies were in esophageal squamous cell carcinoma (ESCC) patients; five studies were in gastric cancer (GC) patients; and four studies were in hepatocellular carcinoma (HCC) patients. In addition, there is one study on esophageal cancer (EC) patients, one study on intrahepatic cholangiocarcinoma (ICC) patients, one study on upper gastrointestinal cancer (UGIC) patients and one study on biliary tract cancers (BTC) patients.
3.2 Baseline PNI levels and OS
Through the analysis of data from 14 studies with 2293 patients, we aimed to explore the correlation between PNI levels and OS in ICI-treated GIC patients. Considering no significant heterogeneity across the studies (I2 = 33.2%, p = 0.109), we employed a fixed-effects model to estimate the pooled HR. The results revealed that high PNI levels were significantly related to longer OS (HR: 0.530, 95% CI: 0.456-0.616, p < 0.001), as depicted in Figure 2.
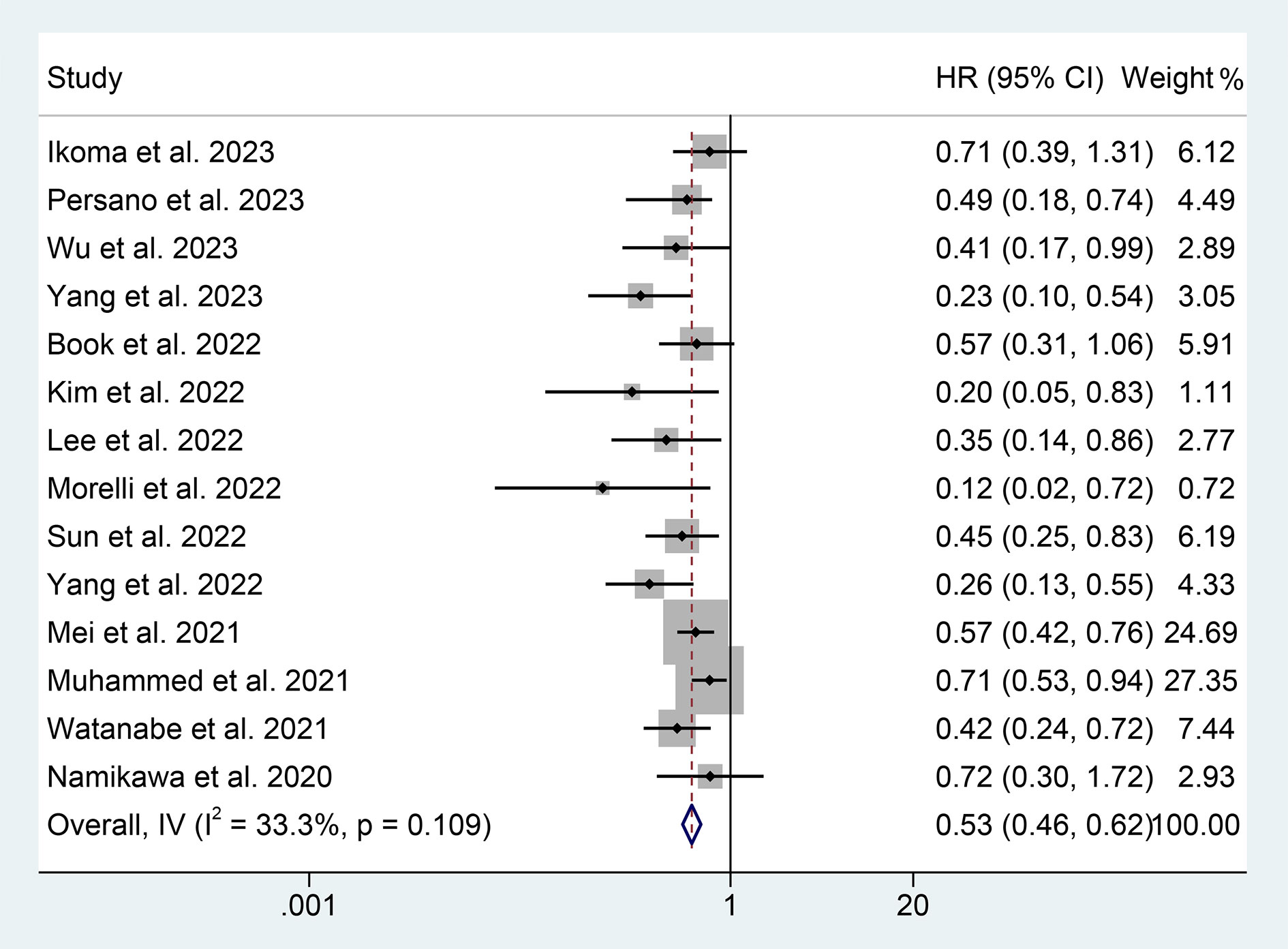
Figure 2 Forest plots of the relationship between prognostic nutritional index and overall survival. HR, hazard ratio; CL, confidence interval.
We performed a subgroup analysis based on cancer type, cut-off values, and the Cox model. We found that a high PNI was associated with a better prognosis in patients with EC, GC, HCC, or BTC (Figure 3). Differences in PNI cutoff values do not affect the correlation between PNI and OS in ICI-treated GIC patients (Figure 4). Both univariate and multivariate analyses confirmed the above findings (Figure S1).
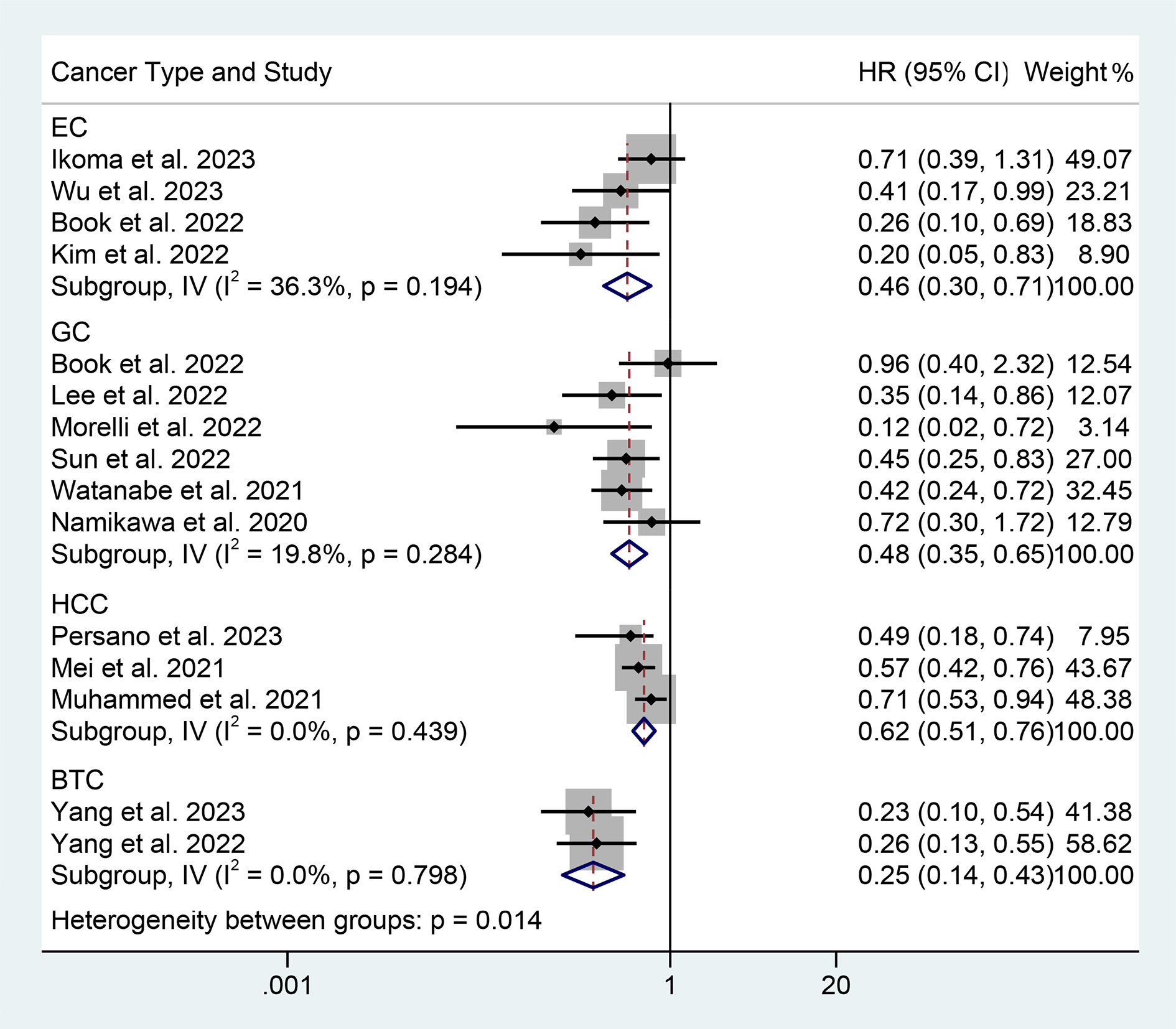
Figure 3 Subgroup analysis of the relationship between prognostic nutritional index and overall survival based on the cancer type. HR, hazard ratio; CL, confidence interval.
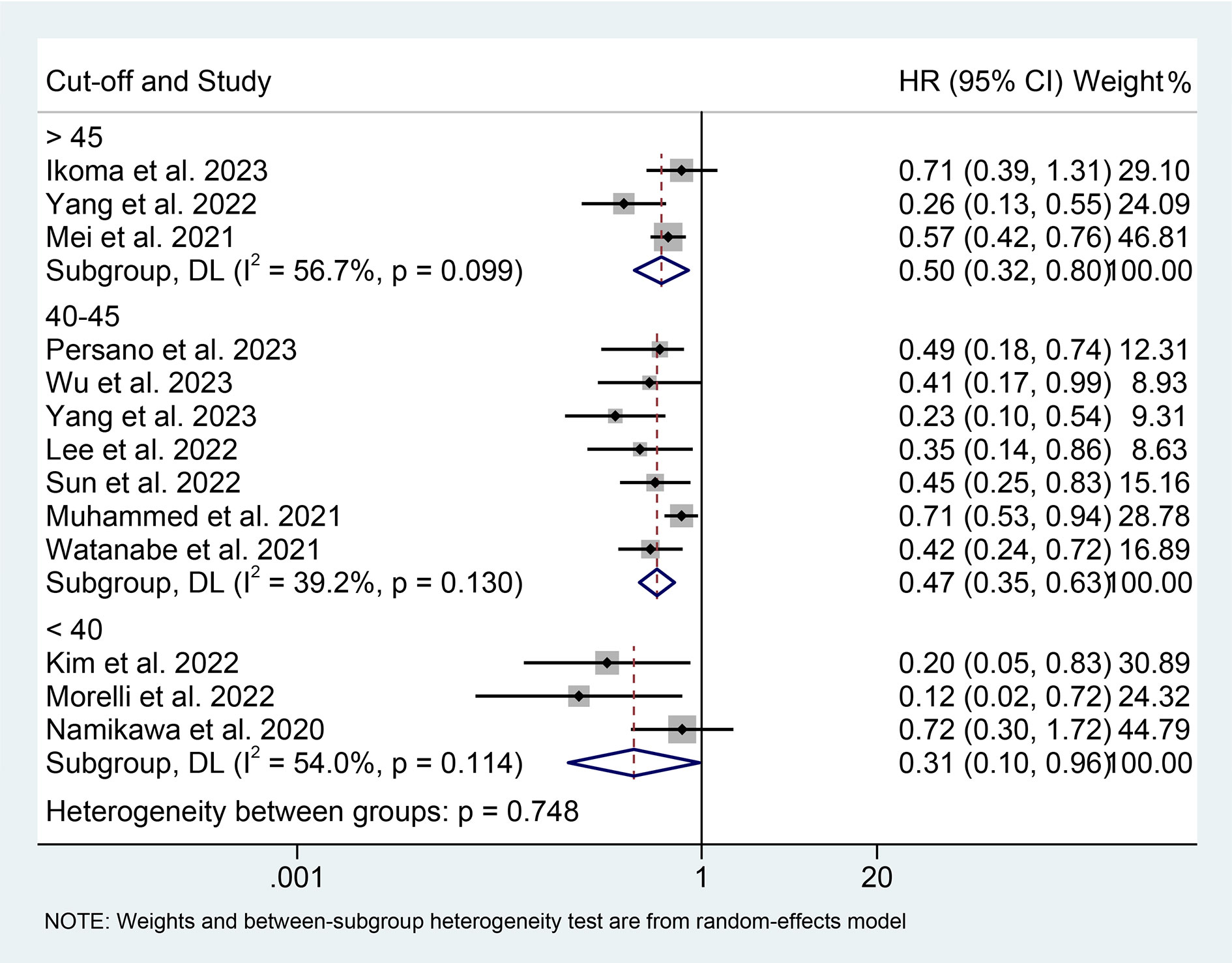
Figure 4 Subgroup analysis of the relationship between prognostic nutritional index and overall survival based on the cut-off. HR, hazard ratio; CL, confidence interval.
3.3 Baseline PNI levels and PFS
We also investigate the correlation between PNI and PFS in GIC patients treated with ICIs using data from 12 studies with 1733 patients. We demonstrated that patients with high PNI levels had a lower risk of progression (HR: 0.740, 95% CI: 0.649-0.844, p < 0.001, Figure 5). No significant heterogeneity was observed (I2 = 22.1%, p = 0.226); we used a fixed-effects model for our analysis.
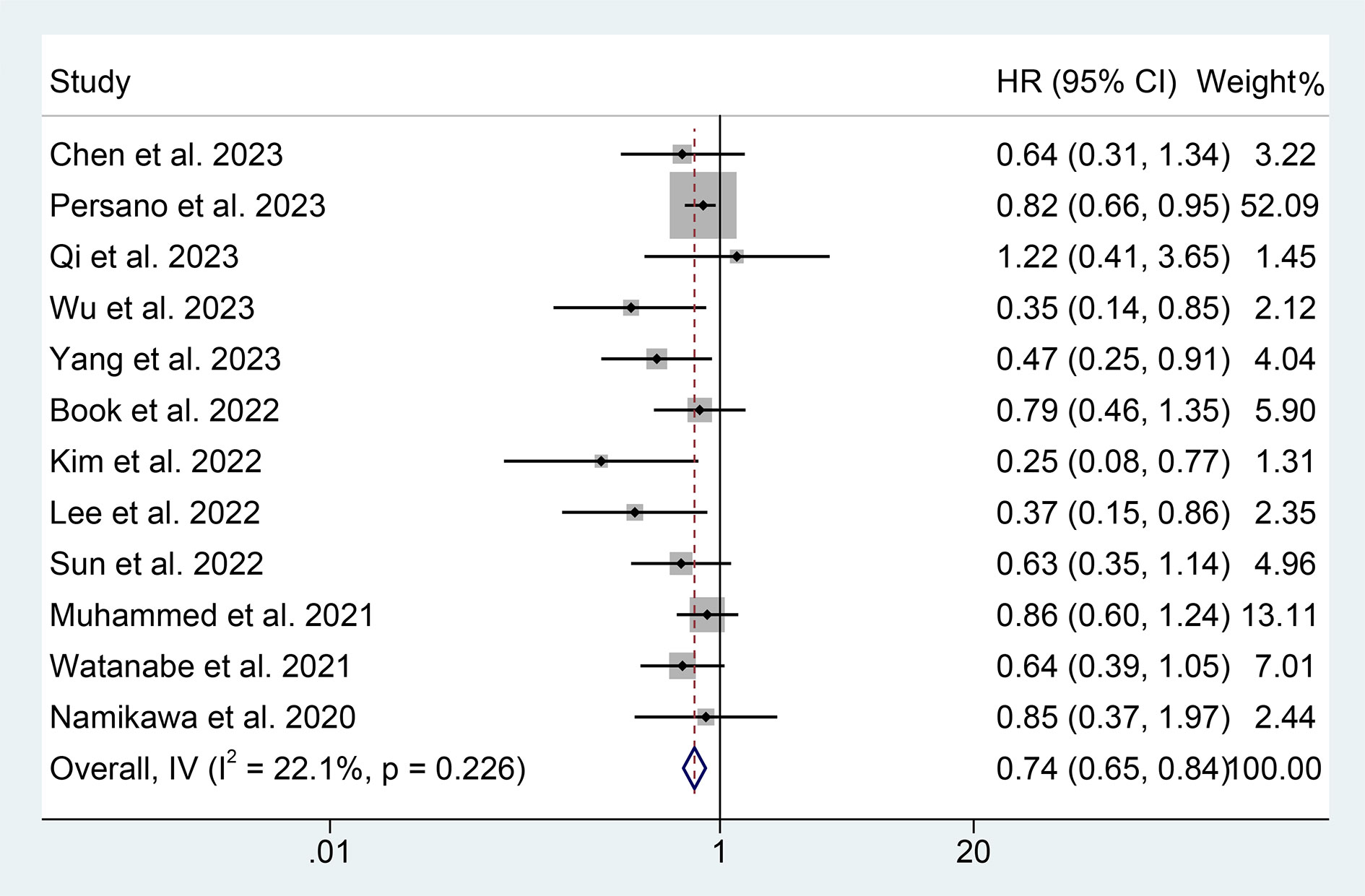
Figure 5 Forest plots of the relationship between prognostic nutritional index and progression-free survival. HR, hazard ratio; CL, confidence interval.
The results of the subgroup analysis showed that the relationship between high PNI levels and longer PFS was consistent in GIC patients with EC, GC, and HCC (Figure 6). Notably, PNI predicted PFS in patients with GIC only when the cut-off value was between 40 and 45 (Figure 7).
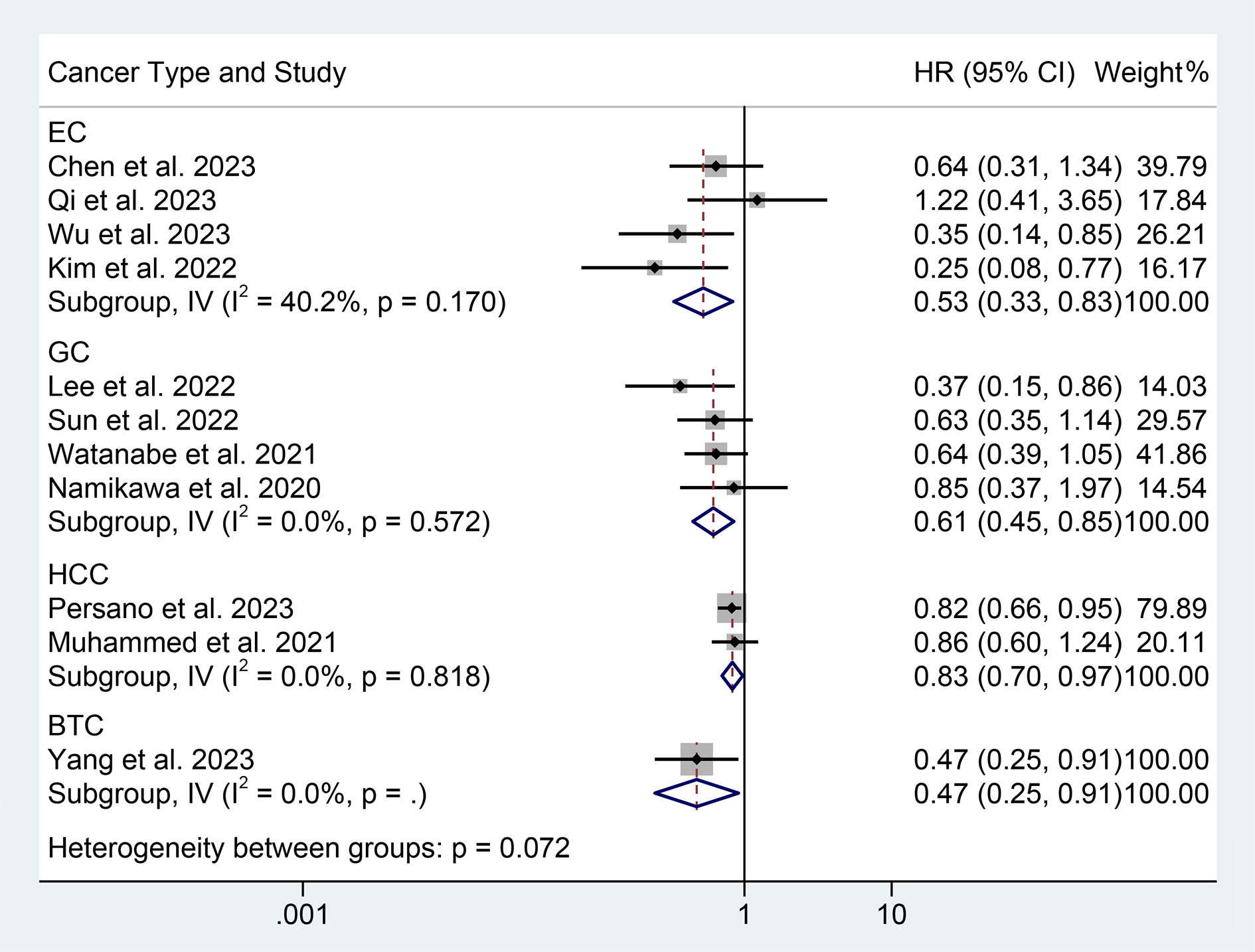
Figure 6 Subgroup analysis of the relationship between prognostic nutritional index and progression-free survival based on the cancer type. HR, hazard ratio; CL, confidence interval.
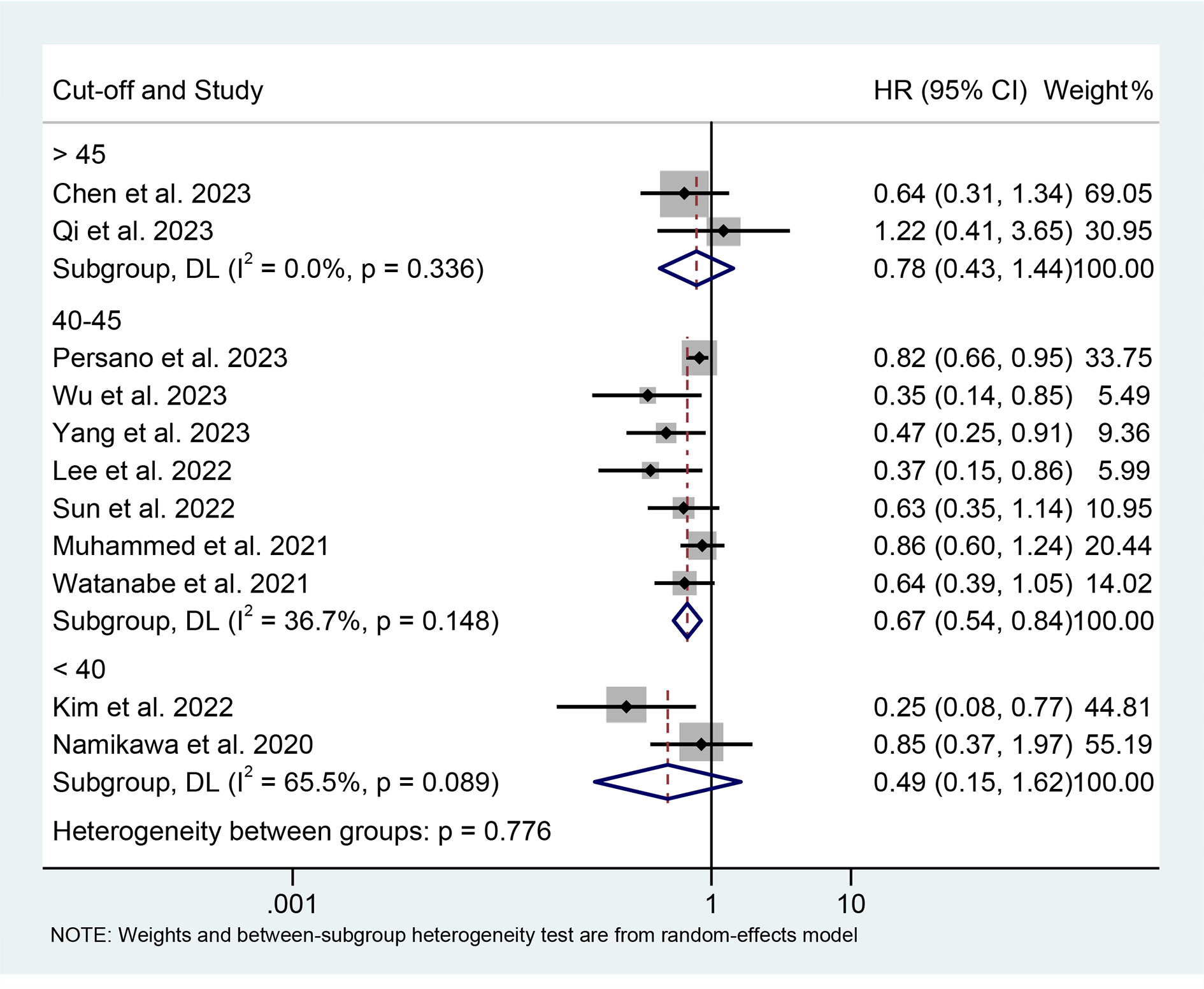
Figure 7 Subgroup analysis of the relationship between prognostic nutritional index and progression-free survival based on the cut-off. HR, hazard ratio; CL, confidence interval.
The findings of the multivariate analysis also support these findings; although the univariate analysis revealed that PNI was not associated with patient PFS, we consider the conclusions drawn from the former to be more reliable due to its more methodologically rigorous nature and larger number of inclusions (Figure S2).
3.4 Baseline PNI levels and ORR and DCR
Subsequently, we conducted an analysis to investigate the correlation between PNI levels and response to ICI therapy in GIC patients. The presence of notable heterogeneity was not observed in the results presented in Figures 8A, B, and hence a fixed-effect model was implemented. Our findings revealed that GIC patients with high PNI levels had a higher ORR (5 studies with 1430 patients, OR: 1.622, 95% CI: 1.251-2.103, p < 0.004, Figure 8A) and DCR (7 studies with 1551 patients, OR: 1.846, 95% CI: 1.428-2.388, p < 0.001, Figure 8B).
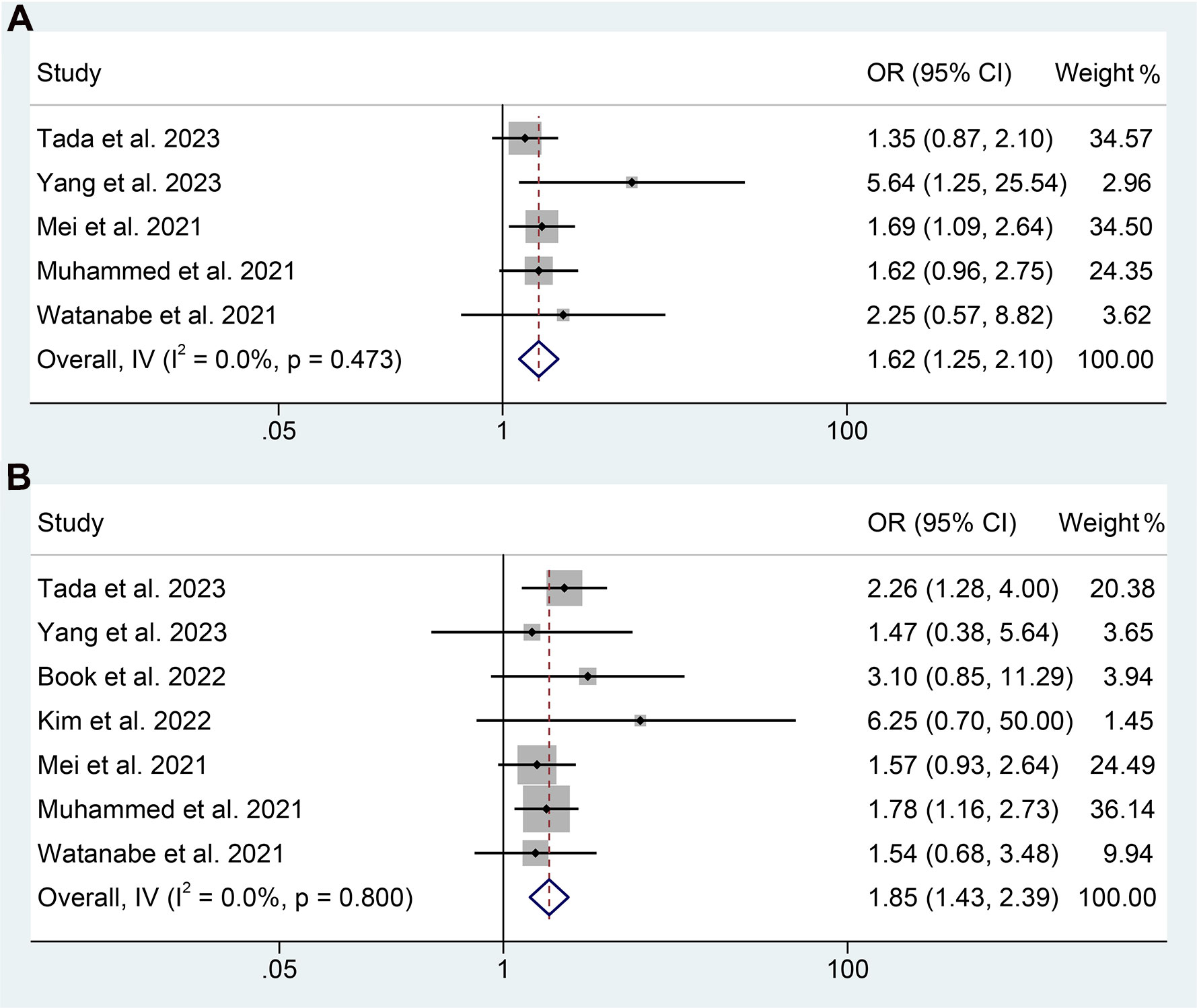
Figure 8 Forest plots of the relationship between prognostic nutritional index and objective response rate (A) and disease control rate (B). OR, odds ratio; CL, confidence interval.
3.5 Sensitivity analysis
To assess the robustness of the findings, a sensitivity analysis was conducted by iteratively excluding each study and examining the impact on the overall results. Our analysis indicated that the exclusion of any individual study did not significantly affect the pooled HR for OS. Specifically, the HR estimates for OS ranged from 0.474 (95% CI: 0.398-0.566) when excluding Muhammed et al., 2021 (25) to 0.547 (95% CI: 0.469-0.637) when excluding Yang et al., 2022 (34), as depicted in Figure 9A. Furthermore, the sensitivity analysis demonstrated that the removal of any individual study did not significantly impact the overall results for PFS. The range of HR values varied from 0.662 (95% CI: 0.547-0.800) after excluding Persano et al., 2023 (27) to 0.754 (95% CI: 0.659-0.862) after excluding Yang et al., 2022 (33) (Figure 9B). Similarly, sensitivity analysis showed that removing any of the studies did not affect the ORR and DCR results (Figures 10A, B).
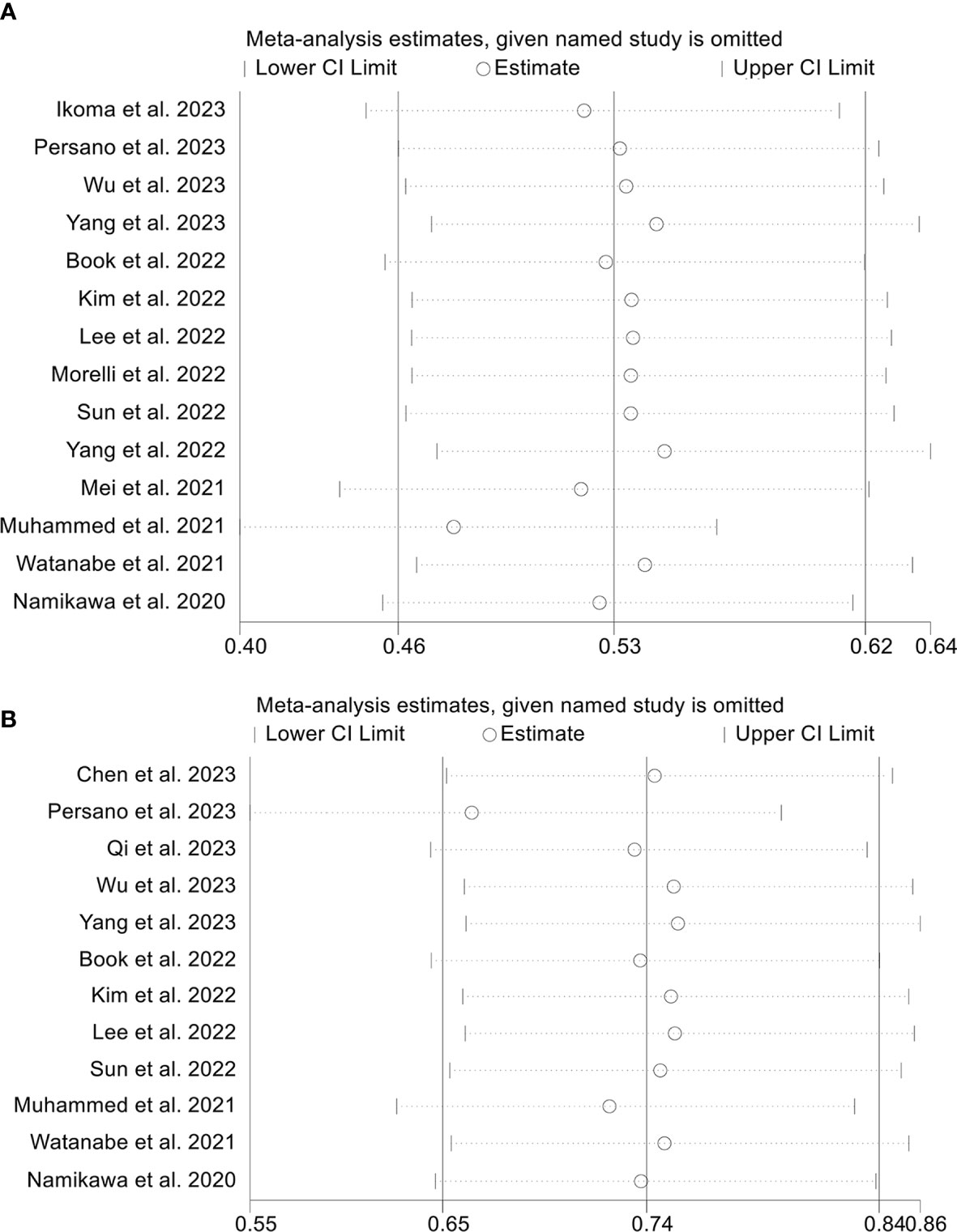
Figure 9 Sensitivity analysis of the association between prognostic nutritional index and overall survival (A) and progression-free survival (B). CL, confidence interval.
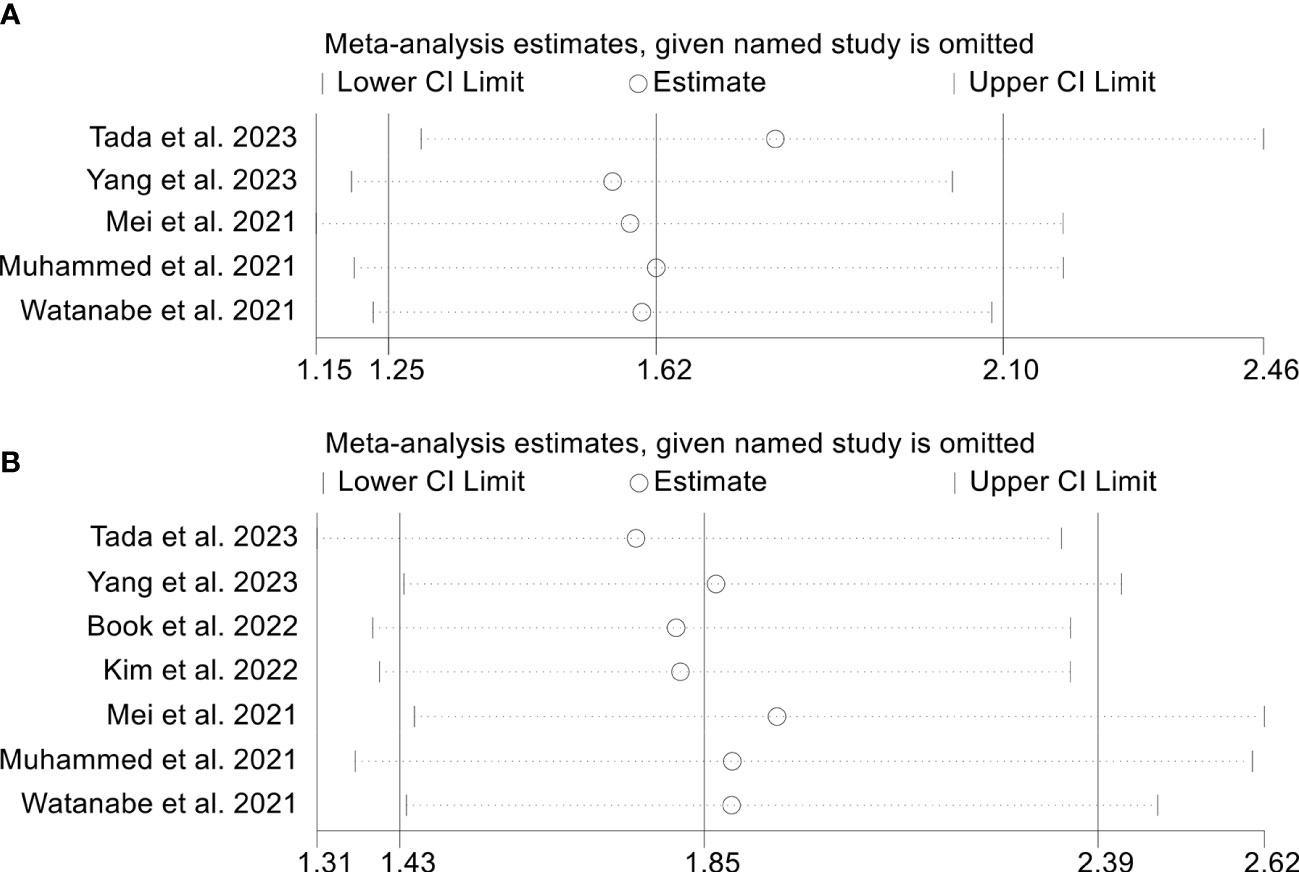
Figure 10 Sensitivity analysis of the association between prognostic nutritional index and objective response rate (A) and disease control rate (B). CL, confidence interval.
3.6 Publication bias
We performed Begg’s and Egger’s tests to evaluate the potential publication bias in our meta-analysis. The findings indicated no considerable publication bias for ORR (Egger’s test: p = 0.241, Begg’s test: p = 0.230) and DCR (Egger’s test: p = 0.086, Begg’s test: p = 0.081). Nevertheless, we detected publication bias in OS (Egger’s test: p = 0.003, Begg’s test: p = 0.021) and PFS (Egger’s test: p = 0.027, Begg’s test: p = 0.064) based on Egger’s test. To address this issue, we utilized the trim and fill method to estimate the number of potential missing studies in OS. The results showed no change in pooled HR without the missing study being incorporated (Figure 11).
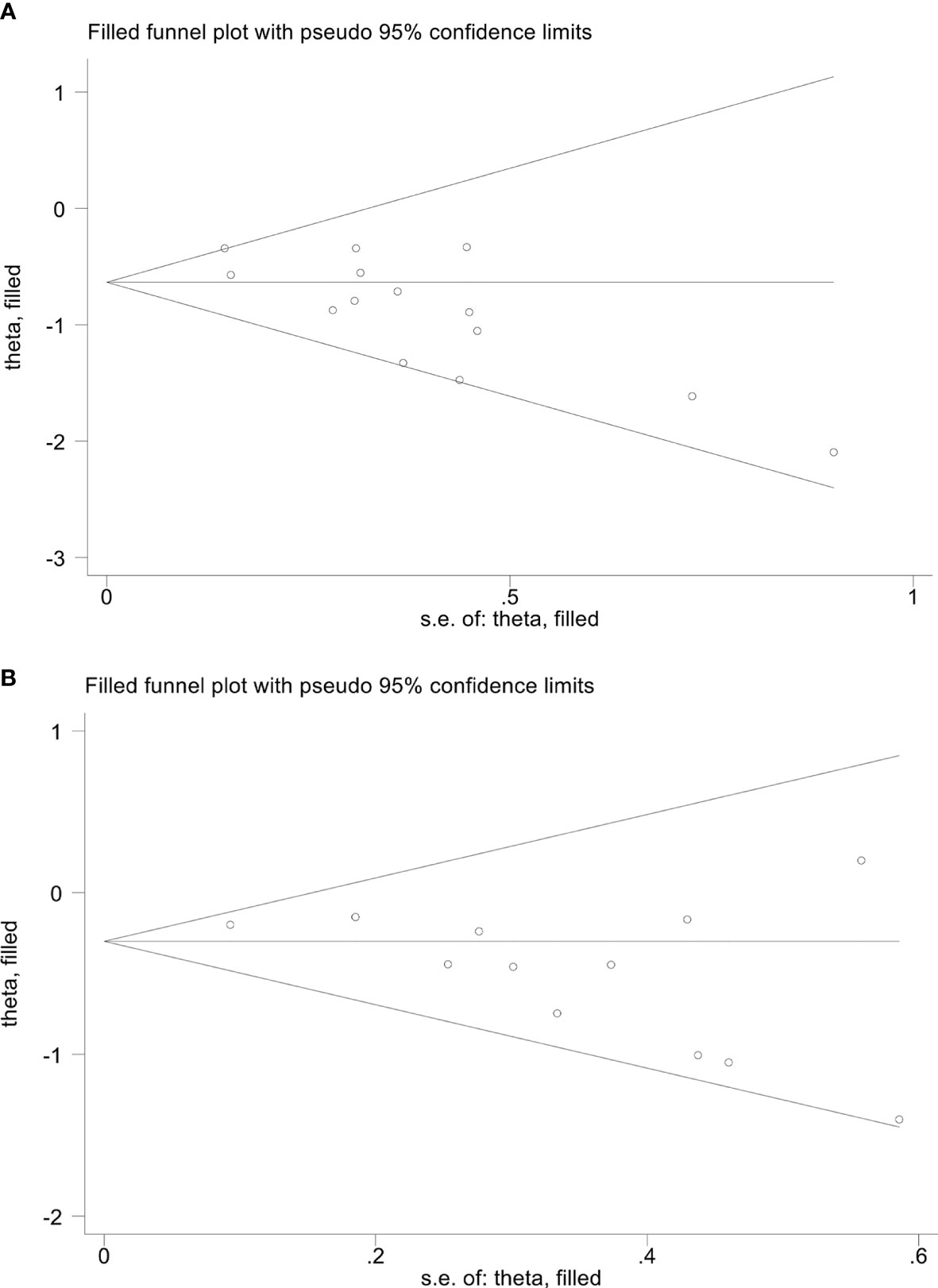
Figure 11 The picture of the trim-and-fill method terms of overall survival (A) and progression-free survival (B). Theta, the effect estimate; S.e. of: theta, the corresponding standard error.
4 Discussion
Our investigation aimed to explore the prognostic significance of PNI in GIC patients receiving ICI therapy. Through a meta-analysis of relevant studies, we established a strong association between elevated PNI levels and favorable OS and PFS and higher ORR and DCR. Furthermore, subgroup analysis showed that PNI cutoff values of 40 to 45 showed greater predictive potential.
While ICIs have emerged as a promising treatment for GIC patients, the factors affecting their efficacy remain unclear. Multiple biomarkers have been proposed for predicting response to ICIs, including tumor mutation burden, microsatellite instability/mismatch repair deficiency, tertiary lymphoid structures, and tumor-infiltrating lymphocytes (35). However, the application of these biomarkers in clinical practice is limited by challenges such as immature detection technology, difficulty obtaining specimens, and high costs.
PNI is calculated as 5 × peripheral lymphocyte count (109/L) +serum albumin (g/L), which includes albumin and lymphocyte count, reflecting nutritional and immune status, respectively. In upper gastrointestinal cancer patients, nutritional problems are prevalent in up to 90% of cases, mainly due to reduced food intake and increased nutrition consumption by tumors (36). Long-standing research has linked malnutrition to a worse tumor prognosis (37, 38). While low albumin levels are indicative of malnutrition, they can also serve as a biomarker for systemic inflammation (39). Inflammatory factors have been shown to inhibit albumin synthesis, while oxidative stress can result in albumin denaturation, contributing to a rapid decrease in serum albumin levels in patients with an inflammatory state (40, 41). Another crucial element in the development of tumors is the tumor microenvironment. Through the attraction of T lymphocytes, tumor-associated macrophages, and circulating cytokines, inflammatory factors can significantly impact tumor cell proliferation, angiogenesis, and tumor invasion/metastasis (42, 43). A crucial component of adaptive immunity is the lymphocyte. The immune system’s capacity to prevent tumor cell growth and metastasis may decline when lymphocyte numbers drop, hastening the development of tumors (44, 45). Therefore, the combination of albumin and lymphocyte counts in the PNI can provide a more comprehensive reflection of the host condition.
Initially used to evaluate the immunotrophic status and surgical risks of gastrointestinal surgery patients, PNI has since been applied to other cancer types, including EC (46), GC (26), HCC (47), and pancreatic cancer (48). The success of ICIs in combating tumors is attributed to their ability to alleviate the suppression of tumor cells by the immune system. Hence, the nutritional and immune statuses of patients are critical determinants of the efficacy of ICIs (49). In this study, we performed the first meta-analysis to confirm that PNI predicted the response of GIC patients to ICI therapy. PNI possesses several benefits that make it convenient for daily clinical practice. It is readily available, easily quantifiable, repeatable, and relatively cost-effective to assess (50). As a result, due to its well-established impact on the host’s nutritional and immune status as well as cancer, the PNI could serve as a useful tool in predicting the therapeutic outcomes of ICIs in GIC patients. Individualized and timely nutritional and immunological interventions may improve the prognosis of patients with low baseline PNI.
It is noteworthy that the majority of studies included in this analysis were retrospective cohort studies, which may limit their statistical validity. In addition, the types of ICIs used in each study are not entirely consistent. Therefore, it is imperative to perform additional high-quality investigations with larger sample sizes, particularly multicenter prospective studies, to corroborate and refine our findings.
Data availability statement
The original contributions presented in the study are included in the article/Supplementary Material. Further inquiries can be directed to the corresponding authors.
Author contributions
LZ, WM, BH, and WW conceived and designed the study. LZ, WM, ZQ, TK, and KW were responsible for the collection and assembly of data, data analysis, and interpretation. LZ, ZQ, and WM were involved in writing the manuscript. LZ, QZ, WM, BH, and WW revised the manuscript. All authors contributed to the article and approved the submitted version.
Conflict of interest
The authors declare that the research was conducted in the absence of any commercial or financial relationships that could be construed as a potential conflict of interest.
Publisher’s note
All claims expressed in this article are solely those of the authors and do not necessarily represent those of their affiliated organizations, or those of the publisher, the editors and the reviewers. Any product that may be evaluated in this article, or claim that may be made by its manufacturer, is not guaranteed or endorsed by the publisher.
Supplementary material
The Supplementary Material for this article can be found online at: https://www.frontiersin.org/articles/10.3389/fimmu.2023.1219929/full#supplementary-material
Supplementary Table 1 | The detailed search strategies for Pubmed.
Supplementary Figure 1 | Subgroup analysis of the relationship between prognostic nutritional index and overall survival based on the Cox model. HR, hazard ratio; CL, confidence interval.
Supplementary Figure 2 | Subgroup analysis of the relationship between prognostic nutritional index and progression-free survival based on the Cox model. HR, hazard ratio; CL, confidence interval.
References
1. Arnold M, Abnet CC, Neale RE, Vignat J, Giovannucci EL, McGlynn KA, et al. Global burden of 5 major types of gastrointestinal cancer. Gastroenterology (2020) 159(1):335–349.e315. doi: 10.1053/j.gastro.2020.02.068
2. Wang Y, Zou J, Li Y, Jiao X, Wang Y, Zhuo N, et al. Serological biomarkers predict immune-related adverse events and clinical benefit in patients with advanced gastrointestinal cancers. Front Immunol (2022) 13:987568. doi: 10.3389/fimmu.2022.987568
3. Zhang L, Chen C, Chai D, Li C, Guan Y, Liu L, et al. The association between antibiotic use and outcomes of HCC patients treated with immune checkpoint inhibitors. Front Immunol (2022) 13:956533. doi: 10.3389/fimmu.2022.956533
4. Boku N, Ryu MH, Kato K, Chung HC, Minashi K, Lee KW, et al. Safety and efficacy of nivolumab in combination with s-1/capecitabine plus oxaliplatin in patients with previously untreated, unresectable, advanced, or recurrent gastric/gastroesophageal junction cancer: interim results of a randomized, phase II trial (ATTRACTION-4). Ann Oncol (2019) 30(2):250–8. doi: 10.1093/annonc/mdy540
5. Santini D, Zeppola T, Russano M, Citarella F, Anesi C, Buti S, et al. PD-1/PD-L1 checkpoint inhibitors during late stages of life: an ad-hoc analysis from a large multicenter cohort. J Transl Med (2021) 19(1):270. doi: 10.1186/s12967-021-02937-9
6. Zhang L, Jin Q, Chai D, Kuang T, Li C, Guan Y, et al. The correlation between probiotic use and outcomes of cancer patients treated with immune checkpoint inhibitors. Front Pharmacol (2022) 13:937874. doi: 10.3389/fphar.2022.937874
7. Ding P, Lv J, Sun C, Chen S, Yang P, Tian Y, et al. Combined systemic inflammatory immunity index and prognostic nutritional index scores as a screening marker for sarcopenia in patients with locally advanced gastric cancer. Front Nutr (2022) 9:981533. doi: 10.3389/fnut.2022.981533
8. Ding P, Guo H, He X, Sun C, Lowe S, Bentley R, et al. Effect of skeletal muscle loss during neoadjuvant imatinib therapy on clinical outcomes in patients with locally advanced GIST. BMC Gastroenterol (2022) 22(1):399. doi: 10.1186/s12876-022-02479-4
9. Ding P, Guo H, Sun C, Yang P, Kim NH, Tian Y, et al. Combined systemic immune-inflammatory index (SII) and prognostic nutritional index (PNI) predicts chemotherapy response and prognosis in locally advanced gastric cancer patients receiving neoadjuvant chemotherapy with PD-1 antibody sintilimab and XELOX: a prospective study. BMC Gastroenterol (2022) 22(1):121. doi: 10.1186/s12876-022-02199-9
10. Kim JH, Bae YJ, Jun KH, Chin HM. Long-term trends in hematological and nutritional status after gastrectomy for gastric cancer. J Gastrointest Surg (2017) 21(8):1212–9. doi: 10.1007/s11605-017-3445-7
11. Kim YN, Choi YY, An JY, Choi MG, Lee JH, Sohn TS, et al. Comparison of postoperative nutritional status after distal gastrectomy for gastric cancer using three reconstructive methods: a multicenter study of over 1300 patients. J Gastrointest Surg (2020) 24(7):1482–8. doi: 10.1007/s11605-019-04301-1
12. Yu J, Huang C, Sun Y, Su X, Cao H, Hu J, et al. Effect of laparoscopic vs open distal gastrectomy on 3-year disease-free survival in patients with locally advanced gastric cancer: the CLASS-01 randomized clinical trial. JAMA (2019) 321(20):1983–92. doi: 10.1001/jama.2019.5359
13. Mohri Y, Inoue Y, Tanaka K, Hiro J, Uchida K, Kusunoki M. Prognostic nutritional index predicts postoperative outcome in colorectal cancer. World J Surg (2013) 37(11):2688–92. doi: 10.1007/s00268-013-2156-9
14. Park SH, Lee S, Song JH, Choi S, Cho M, Kwon IG, et al. Prognostic significance of body mass index and prognostic nutritional index in stage II/III gastric cancer. Eur J Surg Oncol (2020) 46(4 Pt A):620–5. doi: 10.1016/j.ejso.2019.10.024
15. Liu JY, Dong HM, Wang WL, Wang G, Pan H, Chen WW, et al. The effect of the prognostic nutritional index on the toxic side effects of radiochemotherapy and prognosis after radical surgery for gastric cancer. Cancer Manag Res (2021) 13:3385–92. doi: 10.2147/CMAR.S301140
16. Liberati A, Altman DG, Tetzlaff J, Mulrow C, Gøtzsche PC, Ioannidis JP, et al. The PRISMA statement for reporting systematic reviews and meta-analyses of studies that evaluate health care interventions: explanation and elaboration. PloS Med (2009) 6(7):e1000100. doi: 10.1371/journal.pmed.1000100
17. Zhang L, Feng J, Kuang T, Chai D, Qiu Z, Deng W, et al. Blood biomarkers predict outcomes in patients with hepatocellular carcinoma treated with immune checkpoint inhibitors: a pooled analysis of 44 retrospective sudies. Int Immunopharmacol (2023) 118:110019. doi: 10.1016/j.intimp.2023.110019
18. Booka E, Kikuchi H, Haneda R, Soneda W, Kawata S, Murakami T, et al. Neutrophil-to-Lymphocyte ratio to predict the efficacy of immune checkpoint inhibitor in upper gastrointestinal cancer. Anticancer Res (2022) 42(6):2977–87. doi: 10.21873/anticanres.15781
19. Chen W, Li D, Bian X, Wu Y, Xu M, Wu M, et al. Peripheral blood markers predictive of progression-free survival in advanced esophageal squamous cell carcinoma patients treated with PD-1 inhibitors plus chemotherapy as first-line therapy. Nutr Cancer (2023) 75(1):207–18. doi: 10.1080/01635581.2022.2123533
20. Ikoma T, Shimokawa M, Matsumoto T, Boku S, Yasuda T, Shibata N, et al. Inflammatory prognostic factors in advanced or recurrent esophageal squamous cell carcinoma treated with nivolumab. Cancer Immunol Immunother (2023) 72(2):427–35. doi: 10.1007/s00262-022-03265-7
21. Kim JH, Ahn B, Hong SM, Jung HY, Kim DH, Choi KD, et al. Real-world efficacy data and predictive clinical parameters for treatment outcomes in advanced esophageal squamous cell carcinoma treated with immune checkpoint inhibitors. Cancer Res Treat (2022) 54(2):505–16. doi: 10.4143/crt.2020.1198
22. Lee J, Choi SH, Baek JH, Baek DW, Kim JG, Kang BW. Clinical impact of prognostic nutrition index for advanced gastric cancer patients with peritoneal metastases treated nivolumab monotherapy. Chonnam Med J (2022) 58(1):24–8. doi: 10.4068/cmj.2022.58.1.24
23. Mei J, Sun XQ, Lin WP, Li SH, Lu LH, Zou JW, et al. Comparison of the prognostic value of inflammation-based scores in patients with hepatocellular carcinoma after anti-pd-1 therapy. J Inflamm Res (2021) 14:3879–90. doi: 10.2147/JIR.S325600
24. Morelli C, Formica V, Patrikidou A, Rofei M, Shiu KK, Riondino S, et al. Nutritional index for immune-checkpoint inhibitor in patients with metastatic gastro-esophageal junction/gastric cancer. J Gastrointest Oncol (2022) 13(5):2072–81. doi: 10.21037/jgo-22-217
25. Muhammed A, Fulgenzi CAM, Dharmapuri S, Pinter M, Balcar L, Scheiner B, et al. The systemic inflammatory response identifies patients with adverse clinical outcome from immunotherapy in hepatocellular carcinoma. Cancers (Basel) (2021) 14(1):186. doi: 10.3390/cancers14010186
26. Namikawa T, Yokota K, Tanioka N, Fukudome I, Iwabu J, Munekage M, et al. Systemic inflammatory response and nutritional biomarkers as predictors of nivolumab efficacy for gastric cancer. Surg Today (2020) 50(11):1486–95. doi: 10.1007/s00595-020-02048-w
27. Persano M, Rimini M, Tada T, Suda G, Shimose S, Kudo M, et al. Role of the prognostic nutritional index in predicting survival in advanced hepatocellular carcinoma treated with atezolizumab plus bevacizumab. Oncology (2023) 101(5):283–91. doi: 10.1016/j.iotech.2022.100212
28. Qi WX, Wang X, Li C, Li S, Li H, Xu F, et al. Pretreatment absolute lymphocyte count is an independent predictor for survival outcomes for esophageal squamous cell carcinoma patients treated with neoadjuvant chemoradiotherapy and pembrolizumab: an analysis from a prospective cohort. Thorac Cancer (2023) 14(17):1556–66. doi: 10.1111/1759-7714.14898
29. Sun H, Chen L, Huang R, Pan H, Zuo Y, Zhao R, et al. Prognostic nutritional index for predicting the clinical outcomes of patients with gastric cancer who received immune checkpoint inhibitors. Front Nutr (2022) 9:1038118. doi: 10.3389/fnut.2022.1038118
30. Tada T, Kumada T, Hiraoka A, Kariyama K, Tani J, Hirooka M, et al. Nutritional status is associated with prognosis in patients with advanced unresectable hepatocellular carcinoma treated with atezolizumab plus bevacizumab. Oncology (2023) 101(4):270–82. doi: 10.1159/000527676
31. Watanabe H, Yamada T, Komori K, Hara K, Kano K, Takahashi K, et al. Effect of prognostic nutrition index in gastric or gastro-oesophageal junction cancer patients undergoing nivolumab monotherapy. In Vivo (2021) 35(1):563–9. doi: 10.21873/invivo.12292
32. Wu M, Zhu Y, Chen X, Wang X, Lin X, Yan X, et al. Prognostic nutritional index predicts the prognosis of patients with advanced esophageal cancer treated with immune checkpoint inhibitors: a retrospective cohort study. J Gastrointest Oncol (2023) 14(1):54–63. doi: 10.21037/jgo-23-48
33. Yang L, Zhong J, Wang W, Zhou F. Prognostic nutritional index associates with immunotherapy response in patients with metastatic biliary tract cancer. Nutr Cancer (2023) 75(2):696–706. doi: 10.1080/01635581.2022.2153880
34. Yang Z, Zhang D, Zeng H, Fu Y, Hu Z, Pan Y, et al. Inflammation-based scores predict responses to PD-1 inhibitor treatment in intrahepatic cholangiocarcinoma. J Inflamm Res (2022) 15:5721–31. doi: 10.2147/JIR.S385921
35. Grecea M, Soritau O, Dulf D, Ciuleanu TE, Zdrenghea M. Potential biomarkers for the efficacy of PD-1-PD-L blockade in cancer. Onco Targets Ther (2021) 14:5275–91. doi: 10.2147/OTT.S283892
36. Furness K, Silvers MA, Savva J, Huggins CE, Truby H, Haines T. Long-term follow-up of the potential benefits of early nutritional intervention in adults with upper gastrointestinal cancer: a pilot randomised trial. Support Care Cancer (2017) 25(11):3587–93. doi: 10.1007/s00520-017-3789-2
37. Fearon K, Strasser F, Anker SD, Bosaeus I, Bruera E, Fainsinger RL, et al. Definition and classification of cancer cachexia: an international consensus. Lancet Oncol (2011) 12(5):489–95. doi: 10.1016/S1470-2045(10)70218-7
38. Yuan Q, Zhang W, Shang W. Identification and validation of a prognostic risk-scoring model based on sphingolipid metabolism-associated cluster in colon adenocarcinoma. Front Endocrinol (Lausanne) (2022) 13:1045167. doi: 10.3389/fendo.2022.1045167
39. Evans DC, Corkins MR, Malone A, Miller S, Mogensen KM, Guenter P, et al. The use of visceral proteins as nutrition markers: an ASPEN position paper. Nutr Clin Pract (2021) 36(1):22–8. doi: 10.1002/ncp.10588
40. Coffelt SB, de Visser KE. Cancer: inflammation lights the way to metastasis. Nature (2014) 507(7490):48–9. doi: 10.1038/nature13062
41. Bito R, Hino S, Baba A, Tanaka M, Watabe H, Kawabata H. Degradation of oxidative stress-induced denatured albumin in rat liver endothelial cells. Am J Physiol Cell Physiol (2005) 289(3):C531–542. doi: 10.1152/ajpcell.00431.2004
42. Condeelis J, Pollard JW. Macrophages: obligate partners for tumor cell migration, invasion, and metastasis. Cell (2006) 124(2):263–6. doi: 10.1016/j.cell.2006.01.007
43. Yuan Q, Deng D, Pan C, Ren J, Wei T, Wu Z, et al. Integration of transcriptomics, proteomics, and metabolomics data to reveal HER2-associated metabolic heterogeneity in gastric cancer with response to immunotherapy and neoadjuvant chemotherapy. Front Immunol (2022) 13:951137. doi: 10.3389/fimmu.2022.951137
44. Dunn GP, Old LJ, Schreiber RD. The immunobiology of cancer immunosurveillance and immunoediting. Immunity (2004) 21(2):137–48. doi: 10.1016/j.immuni.2004.07.017
45. Kitayama J, Yasuda K, Kawai K, Sunami E, Nagawa H. Circulating lymphocyte number has a positive association with tumor response in neoadjuvant chemoradiotherapy for advanced rectal cancer. Radiat Oncol (2010) 5:47. doi: 10.1186/1748-717X-5-47
46. Nakatani M, Migita K, Matsumoto S, Wakatsuki K, Ito M, Nakade H, et al. Prognostic significance of the prognostic nutritional index in esophageal cancer patients undergoing neoadjuvant chemotherapy. Dis Esophagus (2017) 30(8):1–7. doi: 10.1093/dote/dox020
47. Wang D, Hu X, Xiao L, Long G, Yao L, Wang Z, et al. Prognostic nutritional index and systemic immune-inflammation index predict the prognosis of patients with HCC. J Gastrointest Surg (2021) 25(2):421–7. doi: 10.1007/s11605-019-04492-7
48. Fu M, Yu L, Yang L, Chen Y, Chen X, Hu Q, et al. Predictive value of the preoperative prognostic nutritional index for postoperative progression in patients with pancreatic neuroendocrine neoplasms. Front Nutr (2022) 9:945833. doi: 10.3389/fnut.2022.945833
49. Yang Y. Cancer immunotherapy: harnessing the immune system to battle cancer. J Clin Invest (2015) 125(9):3335–7. doi: 10.1172/JCI83871
Keywords: prognostic nutrition index, immune checkpoint inhibitors, gastrointestinal cancers, esophageal cancer, gastric cancer, hepatocellular carcinoma
Citation: Zhang L, Ma W, Qiu Z, Kuang T, Wang K, Hu B and Wang W (2023) Prognostic nutritional index as a prognostic biomarker for gastrointestinal cancer patients treated with immune checkpoint inhibitors. Front. Immunol. 14:1219929. doi: 10.3389/fimmu.2023.1219929
Received: 09 May 2023; Accepted: 19 June 2023;
Published: 21 July 2023.
Edited by:
Qun Zhao, Fourth Hospital of Hebei Medical University, ChinaReviewed by:
Ye Miao, First Affiliated Hospital of Jinzhou Medical University, ChinaKangyu Jin, Zhejiang University, China
Xiaochen Qi, Dalian Medical University, China
Yunfei Liu, Ludwig Maximilian University of Munich, Germany
Copyright © 2023 Zhang, Ma, Qiu, Kuang, Wang, Hu and Wang. This is an open-access article distributed under the terms of the Creative Commons Attribution License (CC BY). The use, distribution or reproduction in other forums is permitted, provided the original author(s) and the copyright owner(s) are credited and that the original publication in this journal is cited, in accordance with accepted academic practice. No use, distribution or reproduction is permitted which does not comply with these terms.
*Correspondence: Baohong Hu, NDc5NzIzNTk5QHFxLmNvbQ==; Weixing Wang, c2F0ZS5sbGl0ZUAxNjMuY29t
†These authors have contributed equally to this work