- 1EpiVax, Providence, RI, United States
- 2University of Georgia, Center for Vaccines and Immunology, Athens, GA, United States
Biologics developers are moving beyond antibodies for delivery of a wide range of therapeutic interventions. These non-antibody modalities are often based on ‘natural’ protein scaffolds that are modified to deliver bioactive sequences. Both human-derived and non-human-sourced scaffold proteins have been developed. New types of “non-antibody” scaffolds are still being discovered, as they offer attractive alternatives to monoclonals due to their smaller size, improved stability, and ease of synthesis. They are believed to have low immunogenic potential. However, while several human-sourced protein scaffolds have not been immunogenic in clinical studies, this may not predict their overall performance in other therapeutic applications. A preliminary evaluation of their potential for immunogenicity is warranted. Immunogenicity risk potential has been clearly linked to the presence of T “helper” epitopes in the sequence of biologic therapeutics. In addition, tolerogenic epitopes are present in some human proteins and may decrease their immunogenic potential. While the detailed sequences of many non-antibody scaffold therapeutic candidates remain unpublished, their backbone sequences are available for review and analysis. We assessed 12 example non-antibody scaffold backbone sequences using our epitope-mapping tools (EpiMatrix) for this perspective. Based on EpiMatrix scoring, their HLA DRB1-restricted T cell epitope content appears to be lower than the average protein, and sequences that may act as tolerogenic epitopes are present in selected human-derived scaffolds. Assessing the potential immunogenicity of scaffold proteins regarding self and non-self T cell epitopes may be of use for drug developers and clinicians, as these exciting new non-antibody molecules begin to emerge from the preclinical pipeline into clinical use.
Introduction
Non-antibody molecules known as engineered scaffold proteins emerged in the early 2000’s (see Gebauer and Skerra (1) for a review of the field). While they are currently used for a number of diagnostics and proteomics applications (2), these novel protein drugs provide developers with an attractive alternative to antibodies. They are often derived from repeating domains of natural human proteins (or highly structured non-human proteins) and feature loops that resemble the CDR domains of monoclonal antibodies that can be modified to express targeting sequences. Examples include Anticalins (from lipocalin) (3, 4); DARPins (from Ankyrin) (5); Affilin (from ubiquitin or gamma-B-crystallin) (6); derivatives of Fibronectin such as Monobodies (7) and Adnectins (8, 9); Avimers (10) and Kunitz scaffold proteins (11, 12). Non-human derived scaffold proteins include Affibodies (from protein A of Staphylococcus) (13) and Nanofitins (14). Knottins (15) are derived from eukaryotic organisms but are also present in several plant species. These example non-antibody scaffolds with their source proteins are described in Table 1 and the protein backbone representations of twelve example protein scaffolds are illustrated in Figure 1.
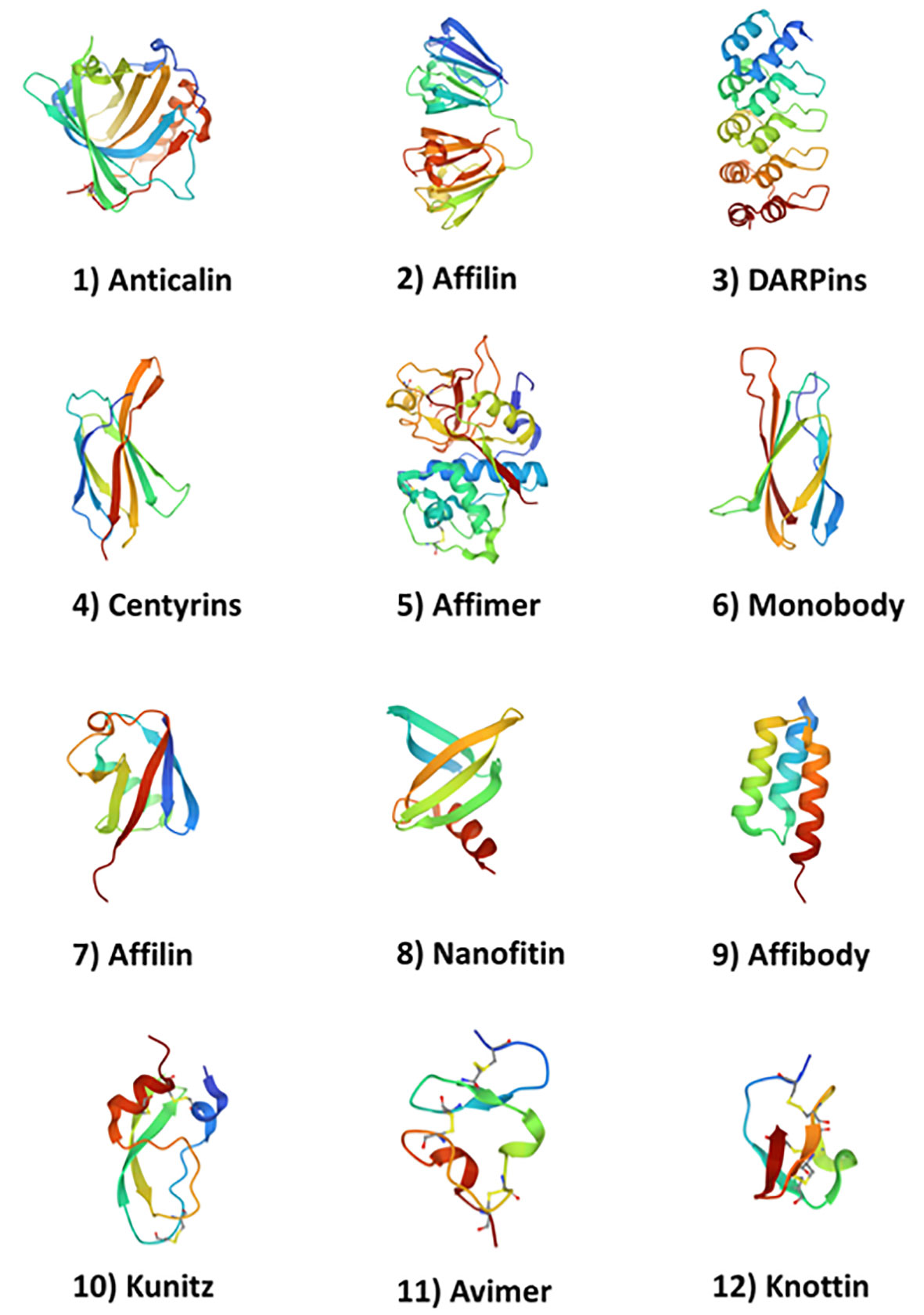
Figure 1 Structures of 12 example protein scaffolds. (1) Anticalins (PDB ID: 4GH7); (2) Affilin (g-B-crystallin based) (PDB ID: 2JDG); (3) DARPins (PDB ID: 1MJ0); (4) Centyrins (PDB ID: 5L2H); (5) Affimer (PDB ID: 1NB5); (6) Adnectin/Monobody (PDB ID: 1TTG); (7) Affilin (ubiquitin based) (PDB ID: 1UBI); (8) Nanofitin (PDB ID: 4CJ2); (9) Affibody (PDB ID: 3MZW); (10) Kunitz (PDB ID: 1KTH); (11) Avimer (PDB ID: 1AJJ); and (12) Knottin (PDB ID: 2IT7. Structures were obtained from the PDB (https://www.rcsb.org/ on April 26th, 2023).
These proteins have attractive features such as stability in circulation, ease of modification, developability and relatively low immunogenicity in preclinical animal models, but their true potential for immunogenicity in clinical applications is not well understood. The number of (HLA DRB1) T helper epitopes that may be present, to drive Anti-Drug-Antibody (ADA) responses has not been quantified and reported. More specifically, modifications to the scaffold to improve affinity for target ligands may introduce new T cell epitopes, contributing to effector (inflammatory) T cell response and ADA. Furthermore, whether or not natural T regulatory epitope sequences (similar to IgG “Tregitopes” previously identified by our group (16) and others (17)) are present in the sequences of scaffold proteins, has yet to be well-defined. These important self-regulatory epitopes may modulate or suppress immunogenic potential of these novel scaffolds.
While many protein scaffold-based therapeutics are in development, only a few have been approved or have reached the phase III clinical stage of development (18, 19), thus it is difficult to determine whether scaffolds are truly advantageous, compared to monoclonal antibodies, in terms of their relative potential for immunogenicity. Nevertheless, it is worth evaluating their potential for immunogenicity using epitope-mapping tools currently used in the industry. Here we used tools that are available in the proprietary ISPRI (Immunogenicity Screening and Protein Re-engineering) toolkit. A similar approach can be performed using publicly-available tools, namely epitope mapping, epitope cluster identification, and ranking with respect to epitope density corrected for potentially tolerogenic epitopes.
Using ISPRI, we assessed the twelve example non-antibody scaffold backbone sequences for this discussion and will focus on their potential for immunogenicity (while understanding that we are not describing the exact immunogenic potential of any specific non-antibody scaffold drug product). While additional studies are clearly needed, this immunoinformatics perspective may provide an initial guide to the assessment of immunogenic potential of scaffold proteins, which might begin with in silico analysis of HLA DRB1-restricted T cell epitope content and a search for potentially tolerogenic epitopes.
Methods for evaluating the immunogenicity risk of scaffold proteins
Both in silico and in vitro methods for evaluating the potential immunogenicity of biologic proteins have been developed and deployed over the past 15 years. It is now well known that fully human-derived biologic products such as Erythropoietin, Thrombopoietin and monoclonal antibodies have the potential to induce unwanted and unexpected immune response (20, 21). Over the years, multiple human-derived biologics were identified as being immunogenic in clinical studies (22).
Drug developers are using both in silico and in vitro methods to define and de-risk inflammatory T helper epitopes in therapeutic proteins prior to advancing candidates to the clinic (see Jawa et al. for a review of current methods (23)). In silico tools that resemble the tools used in this analysis include several, that are available on public websites (24); these can be integrated into internal pipelines. Large and mid-sized companies tend to use secure, web-based commercial platforms such as the ISPRI toolkit developed by De Groot and Martin to assess immunogenicity risk and de-risk monoclonals (25–32), or their own, in house-developed tools. These types of toolkits offer epitope-mapping algorithms such as EpiMatrix (33), as well as other tools, to rank biologic candidates and to compare them with biologics for which the immunogenicity risk profile has been well-established.
T cell epitope-mapping tools are always at the core of immunogenicity risk assessments that are performed using in silico toolkits. ISPRI uses EpiMatrix, a matrix-based algorithm, to define linear nine-to-ten amino acid long peptides that are predicted to bind to human leukocyte antigen (HLA) molecules [also called major histocompatibility complex (MHC)], where they are presented to T cell receptors (TCR) of T cells (CD4+ and CD8+). Since the sequence of T cell epitopes are linear, EpiMatrix uses a set of proprietary matrices to score each amino acid in each nine-mer (or 10 mer) sequence for matches (positive or negative) to well-defined amino acid matrices of possibilities. The final score reflects the probability that a given peptide will bind to a specific MHC (HLA class I or class II) molecule. These scores are normalized across alleles, enabling the comparison of epitopes across proteins and the ranking and comparison of proteins by total T cell epitope content per 1000 9-mer frame assessments, on a normalized immunogenicity scale (see Supplemental Figure 1 and Supplemental Information for a more detailed description of the in silico methods applied to this analysis). Additional features such as the accuracy of the tools (88% for HLA DRB1 alleles, overall) and examples of their use are described in a review of immunoinformatics tools by De Groot et al., 2020 (33).
Of the different types of epitopes that may induce ADA, HLA DRB1-restricted class II epitopes are the most common on antigen-presenting cells. Concentrated predicted HLA DRB1 T cell epitope ‘regions or clusters’ are easily identified in protein sequences (Supplemental Figure 2). These clusters are often found in highly immunogenic vaccine antigens and tend to be associated with immunogenicity when they are identified in protein therapeutics. Once identified, T cell epitope clusters can be compared to sequences of previously published epitopes [and MAPPS data (34)] by searching for homologous MHC-eluted sequences in immune epitope databases such as IEDB (35). The MAPPS approach involves identification of peptides eluted from MHC molecules, followed by mass spectrometry-based analysis. The availability of MAPPS data in online databases has improved the rapid validation of computationally predicted T cell epitopes, as in silico results can be compared to the extensive list of peptides eluted from MHC that is stored online (34). However, it is important to note that while MAPPS assays identify MHC ligands, it does not determine whether the ligands are immunogenic or tolerogenic T-cell epitopes.
Integrating assessment of tolerogenic epitopes
The presence of a T cell epitope does not guarantee that a T helper cell will be activated. In fact, a subset of T cells, called regulatory T cells (Tregs) that are responsible for maintaining immune tolerance and preventing autoimmune diseases can also recognize T cell epitopes in self-proteins such as scaffold proteins. Tregs act as a brake on the immune response by suppressing the activity of other immune cells, including T effector cells that might drive antibody responses (ADA). Tregs are present in the B cell follicle where they control the maturation (and increasing affinity) of antibodies and the development of memory B cells.
In particular, T follicular regulatory cells (Tfr) play a crucial role in modulating B cell selection and affinity maturation within the germinal center (GC) microenvironment, which is relevant to the development of anti-drug-antibodies (ADA) (36). Although many of the mechanisms by which the Tfr regulate the selection process remain to be defined, Tfr cells appear to promote the survival and expansion of B cells with improved affinity for the antigen (37). Thus, the presence or absence of regulatory T cell epitopes in therapeutic proteins [such as Tregitopes (16)], may influence the development of anti-drug antibodies. Identifying and classifying such epitopes in scaffold proteins may inform as to which of the unmodified scaffold protein sequences are less likely to drive ADA, while improving their utility in therapeutic applications.
Tregs (both peripheral and Tfr) are known to require lower antigen concentrations for activation compared to T helper cells. This is because Tregs apparently express T cell receptors (TCRs) with higher affinity for T cell epitope sequences in self-antigens and that have lower affinity for similar sequences in foreign antigens, which allows them to recognize and respond to low levels of self-antigens in the absence of inflammation (38–42). In contrast, T effector cells generally require higher antigen concentrations and co-stimulatory signals for activation, which allows them to respond to foreign antigens during an immune response.
An interesting outcome of this imbalance between Treg and T effector TCR sensitivity is that foreign T cell epitopes that are nearly identical to Treg epitopes that are already present in the human genome, may drive Treg responses (43–45). This observation about foreign antigens that contain self-like sequences contributed to the development of the JanusMatrix tool for defining T reg epitopes in silico. The JanusMatrix tool was developed to predict such T cell epitopes (in foreign proteins, and later, in self-proteins), based on conservation of their TCR-facing residues with similar HLA-binding T helper epitopes in the human genome. This tool is now used to identify potential tolerogenic and tolerated epitopes.
JanusMatrix divides the amino acids present in the linear sequence of T cell epitopes into two sets of amino acids to find matching but not identical epitopes in the human genome. As illustrated in Supplemental Figure 3, T cells interrogate the surface of the peptide-MHC complex using their TCR, but amino acid side chains that are involved in anchoring the peptide to the bottom of the HLA binding groove are invisible to the TCR. Taking advantage of the linear conformation of MHC- (or HLA-) bound T cell epitopes, Moise et al. separated the MHC-binding amino acids from the TCR-facing amino acids, computationally, enabling the prospective identification of T cell epitopes that were conserved with, but not identical to, human proteome self-epitopes (46). The tool facilitates the identification of potentially “immunogenic” and “tolerated” epitopes found in proteins, peptide drugs, and vaccines (47). The JanusMatrix tool was specifically developed to find putative T cell epitopes in the human proteome that are restricted by the same MHC as the input sequence, even when they do not exactly match the input sequence, if they present the same TCR facing residues to the T cell and thus have the potential to generate a cross-conserved immune response. No other tools that are currently available in public toolkits provide this unique search capability, to our knowledge.
JanusMatrix scores are calculated by determining how extensive the cross-conservation of each potential epitope in a peptide is with peptides from the human genome that bind to the same MHC. The JanusMatrix score of a sequence reflects the depth of T cell epitope conservation with the sequence within the human proteome, giving each individually cross-conserved T cell epitope equal weight and dividing by the number of epitopes that have the potential to be cross-conserved in the parent peptide. Higher JanusMatrix scores denote greater degrees of conservation with the human proteome. Following a series of retrospective studies of existing epitope databases, Moise et al. identified a JanusMatrix score threshold of three, as defining potentially tolerated epitope (46), while T cell epitopes with JanusMatrix scores greater than five, are more likely to be actively tolerogenic (activate natural regulatory T cell responses) (48, 49). Not all high-scoring JanusMatrix epitopes are tolerogenic in vitro. Additional in vitro studies are generally carried out to confirm that the epitopes actively suppress T effector responses. The prevalence of the cross-conserved human protein, and/or the fact that it is secreted, may also have an impact on tolerance. It is notable that JanusMatrix enabled the identification of Treg epitopes in immunoglobulin (50), Factor V (51), and others have identified Treg epitopes in heat shock proteins (52).
One of the first applications of the JanusMatrix tool was to assess the cross-reactivity of T cell epitopes between 151 human pathogens and host (human) proteome epitope sequences. Cross-conservation was found to be more common in commensal pathogens (e.g. Herpes Simplex Virus, HSV) and less common in pathogens that had not adapted to humans (53, 54). This led to the concept of ‘immune camouflage’ and suggested that pathogens may evolve to escape immune responses. Furthermore, the epitopes that were the target of the pathogen camouflage epitope appeared to be extensively cross-conserved within the human genome, a feature of T cell epitopes that induced active tolerance (55, 56). The tool has been used to identify Treg epitopes in human pathogens such as Hepatitis C virus (55), and avian influenza virus H7N9 (57).
JanusMatrix has also helped to define tolerogenic epitopes in immunoglobulin G (IgG) (50) and blood coagulation factor V (51). The JanusMatrix tool has also been used to evaluate neo-antigen epitopes in cancer genomes (58). Recent publications describing in vitro methods to confirm predicted T reg epitopes, see references (51, 59), make it possible to validate new Tregitopes that have been defined using specialized in silico tools.
Since the prevalence of human proteome cross-conserved T cell epitopes appears to be relevant to tolerance, at least for Tregitopes and monoclonal antibodies (60, 61), it seems timely to explore epitope cross-conservation in commonly used “non-antibody” scaffold proteins to determine if the balance of immunogenic and tolerogenic epitopes is correlated with clinical immunogenicity. Natural scaffold proteins also contain HLA DRB1-binding peptides that have been eluted from HLA molecules and published in online databases such as the “HLA ligand Atlas” [see IEDB (35) and the HLA Ligand Atlas (62)], making it possible to confirm in silico predictions using published data, as described above for MAPPS data., and illustrated here.
The integration of this type of in silico analysis and data from publicly available HLA ligand databases, and in vitro studies using the regulatory T cell bystander suppression assay (51) and studies of responding T cell phenotypes, may help to further elucidate the relationship between the potential for immunogenicity and the observed immunogenicity of scaffold proteins.
In silico approach to assessing immunogenicity risk
In silico assessment with epitope-mapping tools
EpiMatrix (and other epitope mapping tools) are used to identify putative T cell epitopes in protein sequences. EpiMatrix is based on the pocket profile method developed by Hammer et al. as originally described in (63) and has improved over 25 years of continuous use by EpiVax experts through refinement of the epitope prediction matrices (23). It is used to predict HLA ligands for thousands of HLA class I and class II alleles, covering up to 95% of global populations (64). Scores higher than positive 20 on a normalized scale (the EpiMatrix Immunogenicity Scale, Supplemental Figure 1) indicate significant potential for immunogenicity. Based on an assessment of 10,000 randomly generated protein sequences, EpiMatrix Scores are normalized on a per 1000 assessments scale so that the expected score of a randomly generated protein sequence of a given length is set to zero. Positive scores denote more putative T cell epitope content than random chance and negative scores denote fewer putative T cell epitopes than random chance. The average human genome protein score is negative nine (–9) on the scale, and the average secreted protein score is negative 23 (–23). Standard vaccine antigens score from positive 20 (influenza HA) to greater than 80 (Hepatitis B Virus Surface Antigen (HBVsAg)). (See Supplemental data for additional details).
JanusMatrix used for human homology and tolerance
JanusMatrix, searches HLA/epitope complexes for a human-like outer contour (TCR face) that can be recognized by T cells trained on self-epitopes from other prevalent and circulating human proteins. Improvements to in silico immunogenicity risk predictions using JanusMatrix lead to better definition and discrimination between immunogenic and tolerated T cell epitopes (as described above). JanusMatrix thresholds have been defined for epitopes that are more likely to generate tolerogenic responses or immunogenic responses based on the extent of cross-conservation between a given T cell epitope and similar HLA-binding T cell epitopes in the human genome. For each positive HLA-binding score in the source protein, JanusMatrix calculates the number of like-binding, TCR conserved sequences in the human proteome. The JanusMatrix score represents ‘depth of coverage” or, essentially, how many like-binding epitopes with similar TCR facing residues are found in the human genome. High (>3) JanusMatrix scores indicate high self-epitope conservation. In general, even higher JanusMatrix scores (>5) are found for regulatory T cell epitopes from IgG (65) and Factor V (51), and are rare. While higher JanusMatrix scores are correlated with tolerance in vivo (46, 51, 55, 57, 66, 67), EpiVax has also observed that some T cell epitopes that are conserved with self (but have JanusMatrix scores less than 3) are immunogenic. There are clearly aspects of “cross-conservation with self” that remain to be defined before JanusMatrix can be highly accurate, such as defining whether the prevalence of the peptide sequence or the protein that contains it is relevant to its tolerogenicity. Thus, JanusMatrix scores can be taken as a guide, and putatively tolerogenic epitopes that have high JanusMatrix scores should be carefully evaluated in vitro for tolerogenic activity to better assess their true immunomodulatory potential.
Validation using in vitro - HLA binding
Binding assays (68) are used as a second orthogonal (independent) method in addition to the in silico predictions to assess the HLA binding potential of a given epitope sequence. EpiVax performs soluble HLA DRB1 binding assays using typically seven to nine HLA DRB1 class II alleles that cover >85% of the human population (30) (see Supplemental information for discussion of the HLA Class II supertype alleles). HLA binding assays provide important validation of the HLA binding, although the phenotype of the immune response usually is determined using two types of T cell assays, described below.
In vitro assays to evaluate T cell response
T cell response (measured in vitro) is another independent method of assessment and is generally combined with HLA binding results to further support in silico predictions. Most biotech groups are using in vitro confirmation of immunogenicity to evaluate their candidate molecules (69). In vitro T cell assays use peptides representing predicted epitopes. T cell assays provide information about the ability of defined sequences to drive a T cell response (including an off-target immune response) and may be able to define the type of immune response elicited by the T cell epitope (effector or inflammatory, null, or regulatory). This is an essential step in the epitope validation process because in silico epitope prediction and in vitro HLA binding are not sufficient on their own to confirm T cell response.
(A) In vitro T cell assay (in vitro immunogenicity protocol)
Over the past 10 years, therapeutic protein developers have adapted an in vitro immunogenicity (IVIP) protocol from Wullner et al. (70) to test T cell response to biologics, using human PBMC. Blood from HLA-typed healthy individuals can be obtained from local blood banks. B cells, T cells, and antigen-presenting cells are separated from red blood cells for use in the assay. Epitopes are incubated with the cells to drive T cell responses. In general, overnight incubation is all that is required to generate a T cell response that is due to prior exposure (memory T cell response), while naïve T cell response can be generated in ten to 14 days. ELISpot assays are used to confirm the presence of responding T cells and flow cytometry assays can also be used, to better define the regulatory and effector T cell responses. Alternative (orthogonal) assays include dendritic cell assays developed by Laurent Malherbe (71) and MAPPS assays (72).
(B) Treg assay (Tetanus Toxoid Bystander Suppression Assay)
To evaluate the regulatory potential of highly cross-conserved epitope peptides, our group has adapted a previously published Tetanus Toxoid Bystander Suppression Assay (TTBSA) that measures the inhibitory capacity of potential regulatory peptides on the recall response of human CD4 T cells to the tetanus toxoid (TT) antigen (51, 73). We have set the TT-induced response (proliferation of memory CD4 T cells) in healthy donors at >10%, and suppression of response by the putative regulatory peptide must be statistically significant when compared to response to the TT antigen alone. These assays have been used to validate JanusMatrix predicted Treg epitopes. For example, we selected 22 peptides from GAA (the enzyme that is deficient in Pompe disease patients) with high JanusMatrix scores. The TTBSA confirmed that 4 of the 22 peptides were validated Treg peptides demonstrating greater than 40% suppression in the TTBSA (59). This assay has been used to define additional T cell epitopes that are regulatory in nature including some that are derived from human proteins such as IgG, coagulation factor V and alpha glucosidase (GAA) (51, 59). The assay was used to confirm Treg epitopes in the NSP7 protein of SARS-COV-2 (74) and avian influenza virus H7N9 (57).
Potential immunogenicity and tolerogenicity of scaffold proteins
In Silico risk assessment of non-antibody scaffold proteins
To our knowledge, no in silico studies have been published for non-antibody scaffold proteins. Since many of these products are now approaching clinical use, it may be of interest to report the in silico risk assessment of these sequences, especially as some non-antibody scaffolds are human in origin and some are derived from bacterial proteins.
We therefore performed an Epi Matrix and JanusMatrix evaluation of the 12 scaffold proteins listed in Table 1. The protein sequences identified in the original publications (see Table 1 for citations) were uploaded to the ISPRI toolkit. Methods are described in Supplemental Material, Part 1. EpiMatrix was used to parse each of the proteins into overlapping nine-mer frames for evaluation of HLA binding potential. Each nine-mer frame was evaluated for potential binding to one of nine HLA Class II alleles using the EpiMatrix algorithm, and the overall likelihood of binding was summed for each of the proteins and normalized on the EpiMatrix immunogenicity scale (Supplemental Figure 1), for comparison across different length proteins. ClustiMer was used to identify high-density clusters of predicted T cell epitopes that might generate a promiscuous T cell response (67). (See Supplemental Figure 2).
These clusters were then compared to the human genome and the degree of cross-conservation with self-epitopes was defined using JanusMatrix (Supplemental Figure 3). A whole protein JanusMatrix score was generated by summing the tolerogenic potential of self-like conserved T cell epitopes over the length of the entire protein. The EpiMatrix and JanusMatrix scores for each of these scaffold proteins are listed in Table 2 and illustrated in Figure 2. The number of potentially immunogenic clusters (JanusMatrix less than 3) and potentially tolerogenic clusters (JanusMatrix greater than 5) is also noted for each protein.
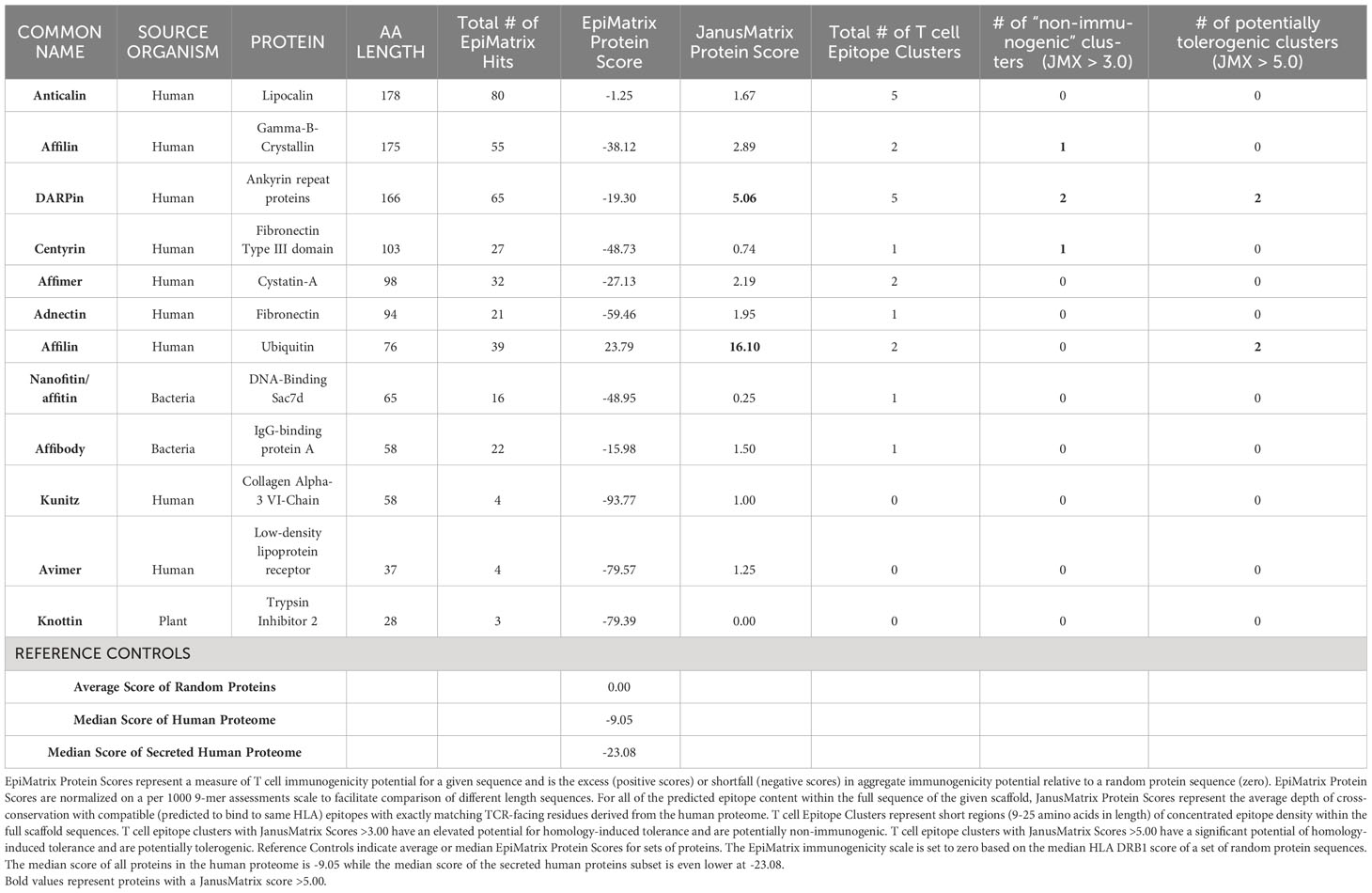
Table 2 In Silico Analysis of 12 Scaffold Proteins. Total # of EpiMatrix Hits is the number of predicted HLA DRB1 ligands or T cell epitopes within the full length of the scaffold sequence.
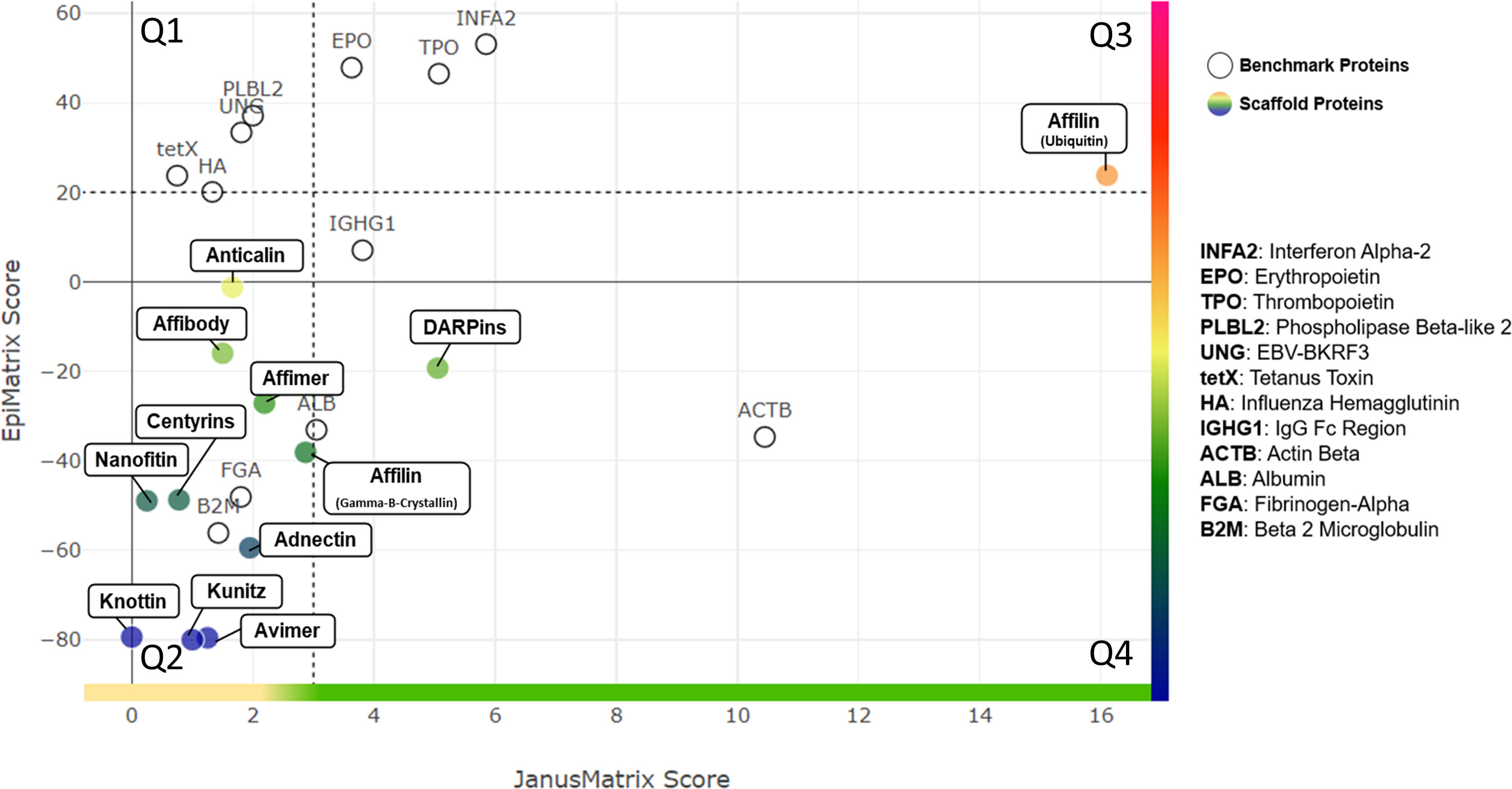
Figure 2 Quadrant Plot Analysis. EpiMatrix Protein Score (measure of epitope content) and JanusMatrix Protein Score (measure of humanness) are plotted for each scaffold protein. The Y-axis represents total epitope content or immunogenic potential. The JanusMatrix Score of a given protein indicates the average depth of coverage (in the human genome) for the HLA-binding peptides contained within that sequence. High JanusMatrix scores indicate high ‘Humanness” and are shown on the X axis. The plot is divided into quadrants that enable the categorization of proteins into one of four broad potential immunogenicity risk quadrants: (Q1) Highest Risk - Epitope Dense, Less Common in Human Proteins; (Q2) Moderate to High Risk: Epitope Sparse, Less Common in Human Proteins; (Q3) Moderate to Low Risk: Epitope Dense, More Common in Human Proteins; and (Q4) Lowest Risk: Epitope Sparse, More Common in Human Proteins. A set of proteins with known immunogenicity profiles are shown on the quadrant plot for comparison.
While this is far from a comprehensive list of scaffold proteins, and the analysis that has been performed still needs to be examined in the context of clinical studies (when those are available), the information provided illustrates the concept that non-antibody scaffold proteins, like immunoglobulins, contain T cell epitope clusters in their backbone sequences that are likely to have an impact on the immunogenicity risk of drug candidates derived from these proteins in the clinic.
As illustrated in Table 2, the backbone sequence of non-antibody scaffolds Anticalin, Affilin (Gamma-B-Crystallin), DARPins, Centyrins, Affimers and Adnectins had high overall numbers of T cell epitope “hits” as well as epitope clusters, although these clusters were distributed over large sequence space resulting in low overall EpiMatrix protein scores. Several epitope clusters were highly homologous with human epitopes (JanusMatrix score >5) in the case of Affilin (ubiquitin), DARPin, and slightly less so for Anticalin and Centyrin (JanusMatrix score >3). The human ubiquitin-based Affilin is striking for its higher overall T cell epitope content, which might otherwise indicate a high risk of immunogenicity, if not for the fact that the protein sequence contains quite a few clusters that are extensively conserved within the human genome.
The bacterial-origin scaffold nanofitin and affibody proteins had more limited cross-conservation with the human genome as demonstrated by their low JanusMatrix scores. Avimer and Knottin are notable for the near absence of T cell epitope ‘hits’, resulting in extremely low scores, which lower their potential for immunogenic response, even though their cross-conservation with the human genome is limited.
A more detailed discussion of the immunogenicity assessment for each of the individual scaffold proteins follows in the Supplemental Information, Part 2. While the overall potential of each of these proteins can be estimated from their overall EpiMatrix score and their overall JanusMatrix score, there is no combined EpiMatrix and JanusMatrix score that can be generated for these proteins, as methods for combining the scores have not yet been validated in prospective studies. Rather than combining the scores, it is possible to visualize the combined scores on a quadrant plot as illustrated in Figure 2.
Discussion
In answer to the question posed in the title of this perspective, human homology may well reduce the potential immunogenicity of selected scaffolds, especially in the case of DARPins and ubiquitin-based Affillin. In addition, most of these non-antibody scaffold proteins have fewer T cell epitopes than the average human protein, which further reduces their potential to drive B cell response and anti-drug antibodies. However, the introduction of novel epitopes in the engineered domains may drive new T cell responses, leading to the development of ADA.
It is important to note that the intended mechanism of action of the scaffold protein therapeutic may have an impact on immunogenicity. At steady states, Treg cells require lower amounts of MHC-bound T cell epitopes to be activated, due to their higher affinity TCR, as compared to conventional T cells that may be specific for the same MHC-peptide complex (75). However, in the context of either acute or chronic inflammatory conditions or an environment in which pro-inflammatory cytokine levels are elevated (such as can be found in autoimmune diseases, or induced by check-point inhibitors such as those used in cancer therapy), conventional effector T cells can be activated and overcome the suppression by Tregs (76, 77).
Therefore, under certain inflammatory conditions, the countervailing balance of tolerogenic epitopes may be overcome, which will increase the potential immunogenicity of scaffold proteins that contain such epitopes. That is exactly why it is even more crucial to validate the presence of putatively tolerogenic epitopes in scaffold proteins, and to define whether the candidate will be used in a clinical context that risks induction of unexpected immunogenicity.
There are additional factors that can impact observed immunogenicity and lead to deviation from predicted values. These additional factors include differences in formulation, the presence or absence of impurities and/or host cell proteins, differences in dose, route of administration, target, mechanism of action, and the immune status of the patient population. The observed incidence of antibody (including neutralizing antibody) positivity in an assay may be influenced by several other factors including assay methodology, sample handling, timing of sample collection, concomitant medications, and underlying disease.
The degree to which any individual being treated with a therapeutic such as a scaffold protein may react to any of the T cell epitopes present in that therapeutic protein will also be dependent on which T cell epitopes are presented by their HLA, and whether those epitopes are cross-reactive to self-epitopes that are also restricted by the same HLA. This concept is discussed at greater length in an analysis of Infantile Pompe Disease subjects’ anti-therapeutic antibody responses to the replacement enzyme GAA. That article describes a method for assessing immunogenicity risk for individual patients by estimating the regulatory to effector ratio of T cell epitopes defined in the ERT as compared to their own residual GAA gene (59). Thus, future investigations into the immunogenicity risk of any therapeutic protein in an individual patient may benefit from approaches that can assess ‘personalized’ immunogenicity risk.
These discoveries are in line with research by other groups, in which the concept of the yin-and-yang balance of Treg and Teffector (or T helper) cells that recognize the same antigen is being identified [see excellent review by Santambroglio (78)]. There is now sufficient evidence to suggest that the peripheral T cell repertoire includes T cells with different phenotypes and different TCRs, that recognize the same MHC-self peptide complex, resulting in opposite immunological outcomes. When immune homeostasis is present, tolerance results. However, under inflammatory conditions associated with increased T cell activation, changes in the balance of signals can tilt the immune response towards the expansion of pro-inflammatory T cells.
Further studies will help elucidate these hypotheses, and much is to be learned from the experiments being conducted by non-antibody scaffold protein developers, as these exciting new molecules begin to enter clinical development.
Data availability statement
All relevant data generated for this study are included in this article (please see Supplemental Material for additional details on methods and detailed scores). The raw data supporting the conclusions of this article will be made available by the authors to any qualified researcher upon request.
Author contributions
ADG, AM, and WM conceptualized and designed the immunogenicity assessment of the non-antibody scaffold proteins. In silico implementation of algorithms were performed by AM, SK, and SL. All authors were involved in data analysis and also participated in drafting or revising the manuscript. All authors approved the submitted version.
Conflict of interest
ADG and WM are senior officers and majority shareholders of EpiVax, Inc, a privately owned immunoinformatics and vaccine design company. SK, SL, and AM are currently employees of EpiVax, Inc.
The authors declare that this study received funding from EpiVax, Inc. The funder had the following involvement in the study: study design, collection, analysis, interpretation of data, the writing of this article, and the decision to submit it for publication.
Publisher’s note
All claims expressed in this article are solely those of the authors and do not necessarily represent those of their affiliated organizations, or those of the publisher, the editors and the reviewers. Any product that may be evaluated in this article, or claim that may be made by its manufacturer, is not guaranteed or endorsed by the publisher.
Author disclaimer
The authors attest that the work contained in this perspective article is free of any bias that might be associated with the commercial goals of the company.
Supplementary material
The Supplementary Material for this article can be found online at: https://www.frontiersin.org/articles/10.3389/fimmu.2023.1215939/full#supplementary-material
Abbreviations
ADA, Anti-Drug Antibodies; DARPins, Designed ankyrin repeat proteins; MAPPs, MHC associated peptide proteomics; MHC, Major Histocompatibility Complex also referred to Human Leukocyte Antigen (HLA) complex in humans; TCR, T Cell Receptor; Tregs, Regulatory T Cells; Tregitope, Regulatory T Cell epitope.
References
1. Gebauer M, Skerra A. Engineered protein scaffolds as next-generation therapeutics. Annu Rev Pharmacol Toxicol (2020) 60:391–415. doi: 10.1146/annurev-pharmtox-010818-021118
2. Olaleye O, Govorukhina N, van de Merbel NC, Bischoff R. Non-antibody-based binders for the enrichment of proteins for analysis by mass spectrometry. Biomolecules (2021) 11. doi: 10.3390/biom11121791
3. Schlehuber S, Skerra A. Lipocalins in drug discovery: From natural ligand-binding proteins to A’nticalins’. Drug Discovery Today (2005) 10:23–33. doi: 10.1016/S1359-6446(04)03294-5
4. Rothe C, Skerra A. Anticalin® Proteins as therapeutic agents in human diseases. BioDrugs (2018) 32:233–43. doi: 10.1007/s40259-018-0278-1
5. Forrer P, Stumpp MT, Binz HK, Plückthun A. A novel strategy to design binding molecules harnessing the modular nature of repeat proteins. FEBS Lett (2003) 539:2–6. doi: 10.1016/s0014-5793(03)00177-7
6. Settele F, Zwarg M, Fiedler S, Koscheinz D, Bosse-Doenecke E. Construction and selection of affilin® Phage display libraries. Clifton, NJ: Methods in molecular biology (2018) pp. 205–38. doi: 10.1007/978-1-4939-7447-4_11.
7. Chandler PG, Buckle AM. Development and differentiation in monobodies based on the fibronectin type 3 domain. Cells (2020) 9. doi: 10.3390/cells9030610
8. Lipovsek D. Adnectins: engineered target-binding protein therapeutics. Protein Eng Des Sel (2011) 24:3–9. doi: 10.1093/protein/gzq097
9. Hantschel O, Biancalana M, Koide S. Monobodies as enabling tools for structural and mechanistic biology. Curr Opin Struct Biol (2020) 60:167–74. doi: 10.1016/j.sbi.2020.01.015
10. Silverman J, Lu Q, Bakker A, To W, Duguay A, Alba BM, et al. Multivalent avimer proteins evolved by exon shuffling of a family of human receptor domains. Nat Biotechnol (2005) 23:1556–61. doi: 10.1038/nbt1166
11. Simeon R, Chen Z. In vitro-engineered non-antibody protein therapeutics. Protein Cell (2018) 9:3–14. doi: 10.1007/s13238-017-0386-6
12. Hosse RJ. A new generation of protein display scaffolds for molecular recognition. Protein Sci (2006) 15:14–27. doi: 10.1110/ps.051817606
13. Eklund M, Axelsson L, Uhlén M, Nygren P-A. Anti-idiotypic protein domains selected from protein A-based affibody libraries. Proteins (2002) 48:454–62. doi: 10.1002/prot.10169
14. Garlich J, Cinier M, Chevrel A, Perrocheau A, Eyerman DJ, Orme M, et al. Discovery of APL-1030, a novel, high-affinity nanofitin inhibitor of C3-mediated complement activation. Biomolecules (2022) 12. doi: 10.3390/biom12030432
15. Gracy J, Chiche L. Structure and modeling of knottins, a promising molecular scaffold for drug discovery. Curr Pharm Des (2011) 17:4337–50. doi: 10.2174/138161211798999339
16. De Groot AS, Moise L, McMurry JA, Wambre E, Van Overtvelt L, Moingeon P, et al. Activation of natural regulatory T cells by IgG Fc-derived peptide “Tregitopes”. Blood (2008) 112:3303–11. doi: 10.1182/blood-2008-02-138073
17. Kedzierska AE, Lorek D, Slawek A, Chelmonska-Soyta A. Tregitopes regulate the tolerogenic immune response and decrease the foetal death rate in abortion-prone mouse matings. Sci Rep (2020) 10:10531. doi: 10.1038/s41598-020-66957-z
18. Mamluk R, Carvajal IM, Morse BA, Wong H, Abramowitz J, Aslanian S, et al. Anti-tumor effect of CT-322 as an adnectin inhibitor of vascular endothelial growth factor receptor-2. MAbs (2010) 2:199–208. doi: 10.4161/mabs.2.2.11304
19. Baird RD, Linossi C, Middleton M, Lord S, Harris A, Rodón J, et al. First-in-human phase I study of MP0250, a first-in-class DARPin drug candidate targeting VEGF and HGF, in patients with advanced solid tumors. J Clin Oncol (2021) 39:145–54. doi: 10.1200/JCO.20.00596
20. Casadevall N, Dupuy E, Molho-Sabatier P, Tobelem G, Varet B, Mayeux P. Autoantibodies against erythropoietin in a patient with pure red-cell aplasia. N Engl J Med (1996) 334:630–3. doi: 10.1056/NEJM199603073341004
21. Scott DW, De Groot AS. Can we prevent immunogenicity of human protein drugs? Ann Rheum Dis (2010) 69 Suppl 1:i72–76. doi: 10.1136/ard.2009.117564
22. Jawa V, Cousens LP, Awwad M, Wakshull E, Kropshofer H, De Groot AS. T-cell dependent immunogenicity of protein therapeutics: Preclinical assessment and mitigation. Clin Immunol (2013) 149:534–55. doi: 10.1016/j.clim.2013.09.006
23. Jawa V, Terry F, Gokemeijer J, Mitra-Kaushik S, Roberts BJ, Tourdot S, et al. T-cell dependent immunogenicity of protein therapeutics pre-clinical assessment and mitigation-updated consensus and review 2020. Front Immunol (2020) 11:1301. doi: 10.3389/fimmu.2020.01301
24. Soria-Guerra RE, Nieto-Gomez R, Govea-Alonso DO, Rosales-Mendoza S. An overview of bioinformatics tools for epitope prediction: Implications on vaccine development. J BioMed Inform (2015) 53:405–14. doi: 10.1016/j.jbi.2014.11.003
25. Venkataramani S, Ernst R, Derebe MG, Wright R, Kopenhaver J, Jacobs SA, et al. In pursuit of stability enhancement of a prostate cancer targeting antibody derived from a transgenic animal platform. Sci Rep (2020) 10:9722. doi: 10.1038/s41598-020-66636-z
26. Brinth AR, Svenson K, Mosyak L, Cunningham O, Hickling T, Lambert M. Crystal structure of ultra-humanized anti-pTau Fab reveals how germline substitutions humanize CDRs without loss of binding’. Sci Rep (2022) 12:8699. doi: 10.1038/s41598-022-12838-6
27. Koren E, De Groot AS, Jawa V, Beck KD, Boone T, Rivera D, et al. Clinical validation of the “in silico” prediction of immunogenicity of a human recombinant therapeutic protein. Clin Immunol (2007) 124:26–32. doi: 10.1016/j.clim.2007.03.544
28. Bone R, Fennell BJ, Tam A, Sheldon R, Nocka K, Varghese S, et al. Discovery and multi-parametric optimization of a high-affinity antibody against interleukin-25 with neutralizing activity in a mouse model of skin inflammation. Antib Ther (2022) 5:258–67. doi: 10.1093/abt/tbac022
29. Tolcher AW, Sweeney CJ, Papadopoulos K, Patnaik A, Chiorean EG, Mita AC, et al. Phase I and pharmacokinetic study of CT-322 (BMS-844203), a targeted adnectin inhibitor of VEGFR-2 based on a domain of human fibronectin. Clin Cancer Res (2011) 17:363–71. doi: 10.1158/1078-0432.CCR-10-1411
30. Kotraiah V, Phares TW, Terry FE, Hindocha P, Silk SE, Nielsen CM, et al. Identification and immune assessment of T cell epitopes in five plasmodium falciparum blood stage antigens to facilitate vaccine candidate selection and optimization. Front Immunol (2021) 12:690348. doi: 10.3389/fimmu.2021.690348
31. Jawa V, Cousens L, De Groot AS. Immunogenicity of therapeutic fusion proteins: contributory factors and clinical experience. In: Fusion Protein Technologies for Biopharmaceuticals. Hoboken, NJ, USA: John Wiley & Sons, Inc. (2013) p. 75–90. doi: 10.1002/9781118354599.ch5
32. Eickhoff CS, Terry FE, Peng L, Meza KA, Sakala IG, Van Aartsen D, et al. Highly conserved influenza T cell epitopes induce broadly protective immunity. Vaccine (2019) 37:5371–81. doi: 10.1016/j.vaccine.2019.07.033
33. De Groot AS, Moise L, Terry F, Gutierrez AH, Hindocha P, Richard G, et al. Better epitope discovery, precision immune engineering, and accelerated vaccine design using immunoinformatics tools. Front Immunol (2020) 11:442. doi: 10.3389/fimmu.2020.00442
34. Garde C, Ramarathinam SH, Jappe EC, Nielsen M, Kringelum JV, Trolle T, et al. Improved peptide-MHC class II interaction prediction through integration of eluted ligand and peptide affinity data. Immunogenetics (2019) 71:445–54. doi: 10.1007/s00251-019-01122-z
35. Vita R, Mahajan S, Overton JA, Dhanda SK, Martini S, Cantrell JR, et al. The immune epitope database (IEDB): 2018 update. Nucleic Acids Res (2019) 47:D339–43. doi: 10.1093/nar/gky1006
36. Ribeiro F, Perucha E, Graca L. T follicular cells: The regulators of germinal center homeostasis. Immunol Lett (2022) 244:1–11. doi: 10.1016/j.imlet.2022.02.008
37. Maceiras AR, Fonseca VR, Agua-Doce A, Graca L. T follicular regulatory cells in mice and men. Immunology (2017) 152:25–35. doi: 10.1111/imm.12774
38. Hsieh C-S, Lee H-M, Lio C-WJ. Selection of regulatory T cells in the thymus. Nat Rev Immunol (2012) 12:157–67. doi: 10.1038/nri3155
39. Wing K, Sakaguchi S. Regulatory T cells exert checks and balances on self tolerance and autoimmunity. Nat Immunol (2010) 11:7–13. doi: 10.1038/ni.1818
40. Sakaguchi S, Wing K, Yamaguchi T. Dynamics of peripheral tolerance and immune regulation mediated by Treg. Eur J Immunol (2009) 39:2331–6. doi: 10.1002/eji.200939688
41. O’Hagan KL, Choi J, Pryshchep O, Chernoff J, Phee H. Pak2 links TCR signaling strength to the development of regulatory T cells and maintains peripheral tolerance. J Immunol (2015) 195:1564–77. doi: 10.4049/jimmunol.1500843
42. Morel PA. Differential T -cell receptor signals for T helper cell programming. Immunology (2018) 155:63–71. doi: 10.1111/imm.12945
43. Jiang H, Chess L. How the immune system achieves self-nonself discrimination during adaptive immunity. Adv Immunol (2009) 102:95–133. doi: 10.1016/S0065-2776(09)01202-4
44. De Groot AS, Ardito M, Terry F, Levitz L, Ross T, Moise L, et al. Low immunogenicity predicted for emerging avian-origin H7N9: implication for influenza vaccine design. Hum Vaccin Immunother (2013) 9:950–6. doi: 10.4161/hv.24939
45. Mishra S, Losikoff PT, Self AA, Terry F, Ardito MT, Tassone R, et al. Peptide-pulsed dendritic cells induce the hepatitis C viral epitope-specific responses of naïve human T cells. Vaccine (2014) 32:3285–92. doi: 10.1016/j.vaccine.2014.03.083
46. Moise L, Gutierrez AH, Bailey-Kellogg C, Terry F, Leng Q, Abdel Hady KM, et al. The two-faced T cell epitope: examining the host-microbe interface with JanusMatrix. Hum Vaccin Immunother (2013) 9:1577–86. doi: 10.4161/hv.24615
47. Moise L, Beseme S, Tassone R, Liu R, Kibria F, Terry F, et al. T cell epitope redundancy: cross-conservation of the TCR face between pathogens and self and its implications for vaccines and autoimmunity. Expert Rev Vaccines (2016) 15:607–17. doi: 10.1586/14760584.2016.1123098
48. De Groot AS, Moise L, Liu R, Gutierrez AH, Tassone R, Bailey-Kellogg C, et al. Immune camouflage: relevance to vaccines and human immunology. Hum Vaccin Immunother (2014) 10:3570–5. doi: 10.4161/hv.36134
49. Losikoff PT, Self AA, Gregory SH. Dendritic cells, regulatory T cells and the pathogenesis of chronic hepatitis C. Virulence (2012) 3:610–20. doi: 10.4161/viru.21823
50. De Groot AS, Cousens L, Mingozzi F, Martin W. Tregitope peptides: the active pharmaceutical ingredient of IVIG? Clin Dev Immunol (2013) 2013:1–6. doi: 10.1155/2013/493138
51. De Groot AS, Rosenberg AS, Miah SMS, Skowron G, Roberts BJ, Lélias S, et al. Identification of a potent regulatory T cell epitope in factor V that modulates CD4+ and CD8+ memory T cell responses. Clin Immunol (2021) 224:108661. doi: 10.1016/j.clim.2020.108661
52. van Eden W, Jansen MAA, Ludwig I, van Kooten P, van der Zee R, Broere F. The enigma of heat shock proteins in immune tolerance. Front Immunol (2017) 8:1599. doi: 10.3389/fimmu.2017.01599
53. He L, De Groot AS, Gutierrez AH, Martin WD, Moise L, Bailey-Kellogg C. Integrated assessment of predicted MHC binding and cross-conservation with self reveals patterns of viral camouflage. BMC Bioinf (2014) 15 Suppl 4:S1. doi: 10.1186/1471-2105-15-S4-S1
54. He L, De Groot AS, Bailey-Kellogg C. Hit-and-run, hit-and-stay, and commensal bacteria present different peptide content when viewed from the perspective of the T cell. Vaccine (2015) 33:6922–9. doi: 10.1016/j.vaccine.2015.08.099
55. Losikoff PT, Mishra S, Terry F, Gutierrez A, Ardito MT, Fast L, et al. HCV epitope, homologous to multiple human protein sequences, induces a regulatory T cell response in infected patients. J Hepatol (2015) 62:48–55. doi: 10.1016/j.jhep.2014.08.026
56. Richard G, Biron B, Boyle C, Ardito M, Moise L, Martin W, et al. Abstract 943: Filtering out self-like neoantigens improves immune response to cancer vaccines. Cancer Res (2019) 79:943–3. doi: 10.1158/1538-7445.AM2019-943
57. Liu R, Moise L, Tassone R, Gutierrez AH, Terry FE, Sangare K, et al. H7N9 T-cell epitopes that mimic human sequences are less immunogenic and may induce Treg-mediated tolerance. Hum Vaccin Immunother (2015) 11:2241–52. doi: 10.1080/21645515.2015.1052197
58. Maine CJ, Richard G, Spasova DS, Miyake-Stoner SJ, Sparks J, Moise L, et al. Self-replicating RNAs drive protective anti-tumor T cell responses to neoantigen vaccine targets in a combinatorial approach. Mol Ther (2021) 29:1186–98. doi: 10.1016/j.ymthe.2020.11.027
59. De Groot AS, Desai AK, Lelias S, Miah SMS, Terry FE, Khan S, et al. Immune tolerance-adjusted personalized immunogenicity prediction for pompe disease. Front Immunol (2021) 12:636731. doi: 10.3389/fimmu.2021.636731
60. Arneth BM. Activation of CD4 and CD8 T cell receptors and regulatory T cells in response to human proteins. PeerJ (2018) 6:e4462. doi: 10.7717/peerj.4462
61. Su Y, Rossi R, De Groot AS, Scott DW. Regulatory T cell epitopes (Tregitopes) in IgG induce tolerance in vivo and lack immunogenicity per se. J Leukoc Biol (2013) 94:377–83. doi: 10.1189/jlb.0912441
62. Marcu A, Bichmann L, Kuchenbecker L, Kowalewski DJ, Freudenmann LK, Backert L, et al. The HLA Ligand Atlas - a resource of natural HLA ligands presented on benign tissues. bioRxiv:778944v2 (2020). doi: 10.1101/778944
63. Hammer J, Sturniolo T, Sinigaglia F. HLA class II peptide binding specificity and autoimmunity. Adv Immunol (1997) 66:67–100. doi: 10.1016/s0065-2776(08)60596-9
64. Southwood S, Sidney J, Kondo A, del Guercio MF, Appella E, Hoffman S, et al. Several common HLA-DR types share largely overlapping peptide binding repertoires. J Immunol (1998) 160:3363–73. doi: 10.4049/jimmunol.160.7.3363
65. Cousens LP, Najafian N, Mingozzi F, Elyaman W, Mazer B, Moise L, et al. In vitro and in vivo studies of IgG-derived Treg epitopes (Tregitopes): a promising new tool for tolerance induction and treatment of autoimmunity. J Clin Immunol (2013) 33 Suppl 1:S43–9. doi: 10.1007/s10875-012-9762-4
66. De Groot AS, Martin W. Reducing risk, improving outcomes: bioengineering less immunogenic protein therapeutics. Clin Immunol (2009) 131:189–201. doi: 10.1016/j.clim.2009.01.009
67. Weber CA, Mehta PJ, Ardito M, Moise L, Martin B, De Groot AS. T cell epitope: friend or foe? Immunogenicity of biologics in context. Adv Drug Delivery Rev (2009) 61:965–76. doi: 10.1016/j.addr.2009.07.001
68. Steere AC, Klitz W, Drouin EE, Falk BA, Kwok WW, Nepom GT, et al. Antibiotic-refractory Lyme arthritis is associated with HLA-DR molecules that bind a Borrelia burgdorferi peptide. J Exp Med (2006) 203:961–71. doi: 10.1084/jem.20052471
69. Gokemeijer J, Wen Y, Jawa V, Mitra-Kaushik S, Chung S, Goggins A, et al. Survey outcome on immunogenicity risk assessment tools for biotherapeutics: an insight into consensus on methods, application, and utility in drug development. AAPS J (2023) 25:55. doi: 10.1208/s12248-023-00820-7
70. Wullner D, Zhou L, Bramhall E, Kuck A, Goletz TJ, Swanson S, et al. Considerations for optimization and validation of an in vitro PBMC derived T cell assay for immunogenicity prediction of biotherapeutics. Clin Immunol (2010) 137:5–14. doi: 10.1016/j.clim.2010.06.018
71. Walsh RE, Lannan M, Wen Y, Wang X, Moreland CA, Willency J, et al. Post-hoc assessment of the immunogenicity of three antibodies reveals distinct immune stimulatory mechanisms. MAbs (2020) 12. doi: 10.1080/19420862.2020.1764829
72. Karle AC. Applying MAPPs assays to assess drug immunogenicity. Front Immunol (2020) 11:698. doi: 10.3389/fimmu.2020.00698
73. Barbey C, Pradervand E, Barbier N, Spertini F. Ex vivo monitoring of antigen-specific CD4<sup<+</sup< T cells after recall immunization with tetanus toxoid. Clin Vaccine Immunol (2007) 14:1108 LP – 1116. doi: 10.1128/CVI.00004-07
74. Miah SMS, Gutierrez A, Lelias S, Boyle CM, Moise L, De Groot AS. A SARS-CoV-2 T cell epitope homologous to a validated human Treg epitope (Tregitope) suppresses CD4 and CD8 T cell memory responses. International Society for Vaccines, Quebec City (2022). Available at: https://epivax.com/wp-content/uploads/2022/08/ISV_poster-2022-Shah-1.pdf.
75. Josefowicz SZ, Lu L-F, Rudensky AY. Regulatory T cells: mechanisms of differentiation and function. Annu Rev Immunol (2012) 30:531–64. doi: 10.1146/annurev.immunol.25.022106.141623
76. Mercadante ER, Lorenz UM. Breaking free of control: how conventional T cells overcome regulatory T cell suppression. Front Immunol (2016) 7:193. doi: 10.3389/fimmu.2016.00193
77. McLaughlin T, Liu L-F, Lamendola C, Shen L, Morton J, Rivas H, et al. T-cell profile in adipose tissue is associated with insulin resistance and systemic inflammation in humans. Arterioscler Thromb Vasc Biol (2014) 34:2637–43. doi: 10.1161/ATVBAHA.114.304636
Keywords: Tregitope, JanusMatrix, tolerance, immunogenicity, protein therapeutics, non-antibody scaffold proteins
Citation: De Groot AS, Khan S, Mattei AE, Lelias S and Martin WD (2023) Does human homology reduce the potential immunogenicity of non-antibody scaffolds? Front. Immunol. 14:1215939. doi: 10.3389/fimmu.2023.1215939
Received: 02 May 2023; Accepted: 20 October 2023;
Published: 07 November 2023.
Edited by:
Dawn Elaine Smilek, UCSF, United StatesReviewed by:
Kathleen P. Pratt, Uniformed Services University of the Health Sciences, United StatesMichael Gutknecht, Novartis, Switzerland
Copyright © 2023 De Groot, Khan, Mattei, Lelias and Martin. This is an open-access article distributed under the terms of the Creative Commons Attribution License (CC BY). The use, distribution or reproduction in other forums is permitted, provided the original author(s) and the copyright owner(s) are credited and that the original publication in this journal is cited, in accordance with accepted academic practice. No use, distribution or reproduction is permitted which does not comply with these terms.
*Correspondence: Anne S. De Groot, YW5uaWVkQGVwaXZheC5jb20=