- 1Department of Endocrinology, Shandong Provincial Hospital, Shandong University, Jinan, Shandong, China
- 2Department of Biostatistics, School of Public Health, Cheeloo College of Medicine, Shandong University, Jinan, Shandong, China
- 3Key Laboratory of Endocrine Glucose & Lipids Metabolism and Brain Aging, Ministry of Education, Department of Endocrinology, Shandong Provincial Hospital Affiliated to Shandong First Medical University, Jinan, China
- 4Department of Vascular Surgery, Shandong Provincial Hospital Affiliated to Shandong First Medical University, Jinan, Shandong, China
- 5Shandong Clinical Medical Center of Endocrinology and Metabolism, Jinan, China
- 6Institute of Endocrinology and Metabolism, Shandong Academy of Clinical Medicine, Jinan, China
Background: Accumulating evidence suggests that the gut microbiota and its metabolites may be involved in autoimmune hypothyroidism. However, the causal association between gut microbiota, metabolites and autoimmune hypothyroidism remains to be determined.
Methods: Instrumental variables were screened from the GWAS datasets of 211 gut microbiota taxonomic groups, gut microbiota-derived metabolites, and autoimmune hypothyroidism. Univariable Mendelian randomization (MR) and multivariable Mendelian randomization (MVMR) were used to analyse the potential causal relationship between autoimmune hypothyroidism, these metabolites, or these microbiota. During the MR analysis, we alternated multiple MR methods with different model assumptions to assess the consistency and robustness of the findings: inverse variance weighted (IVW), weighted median, MR pleiotropy residual sum and outlier (MRPRESSO) and MR−Egger methods. Reverse MR analysis was performed to assess the possibility of reverse causality. Finally, enrichment analyses were used to investigate potential biofunctions.
Results: The IVW results of univariable MR showed that the phyla Actinobacteria, genus DefluviitaleaceaeUCG011, genus Eggerthella, family Defluviitaleaceae, genus Subdoligranulum, genus RuminococcaceaeUCG011, and genus Intestinimonas were associated with autoimmune hypothyroidism. After FDR adjustment, the absence of a causal relationship between gut microbiota and autoimmune hypothyroidism (PFDR > 0.05) suggested a possible marginal association. The results on gut metabolites showed that N-(3-furoyl)glycine, pipecolate, phenylalanine, allantoin, indololactate and alanine were associated with autoimmune hypothyroidism. After FDR correction, only indololactate was associated with hypothyroidism (OR=1.592; 95% CI, 1.228-2.065; PFDR= 0.036). Family Defluviitaleaceae and genus DefluviitaleaceaeUCG011 were suggestively significant in the MVMR. The results of reverse MR analysis showed no reverse causality between autoimmune hypothyroidism and the identified gut microbiota. Enrichment analysis revealed that several key regulatory pathways were significantly enriched.
Conclusion: This study supported that there were beneficial or detrimental causal effects of gut microbiota and its metabolites on autoimmune hypothyroidism risk, which provides more theoretical support for mechanistic research on the “thyroid–gut” axis.
1 Introduction
As previous studies have indicated, the human gut microbiota is primarily composed of bacteria, with more than 90% comprising Firmicutes, Bacteroides, Actinomycetes, and Proteobacteria (1). The gut microbiota exerts a significant influence on the absorption of nutrients, regulation of epithelial development, guidance of innate immunity (1) and impact on adaptive immunity (2). In addition, gut microbiota ecology encompasses not only the gut microbiota but also a vast array of metabolic byproducts produced by the gut microbial community (3). The collective metabolic activities of the microbiota can be regarded as a virtual organ within the gut, relying on the human intestine, which plays a vital role in converting nutritional information from the gut into endocrine signals, influencing the surroundings, as well as remote organ metabolism (4), even being described as a “forgotten organ” (5). The gut microbiota could influence host immunity and physiology by producing metabolites (6). Studies have shown that metabolites produced by the gut microbiota, such as short-chain fatty acids, bile acids, and tryptophan metabolites, are the primary driving factors behind the influence of the gut microbiota on hosts (7). Furthermore, once breaching the intestinal barrier and entering the systemic circulation, the microbiota and its metabolites could promote the release of inflammatory factors, which may be one of the mechanisms involved in the activation of Hashimoto’s thyroiditis (HT) inflammation (8, 9).
Autoimmune hypothyroidism is one of the most prevalent thyroid disorders caused by HT, with a global prevalence rate of approximately 10–12% (10, 11). However, the aetiology of autoimmune hypothyroidism remains unclear. Numerous studies have provided evidence of the relationship between the gut microbiota and the thyroid (12). Since the first report of the association between gut microbiota and HT cases 40 years ago, a study had indicated that rats under pathogen-free conditions had a lower level of incidence of autoimmune thyroiditis compared to conventionally raised rats (13). Ishaq et al. illustrated the link between the modified composition and heightened diversity of the microbiota in individuals with HT compared to those in a healthy population, revealing an impaired microbiota in HT patients (14). Zhao et al. reported comparable findings, indicating a correlation between alterations in gut microbiota and thyroid function (15). With a foundation in genetic predisposition, the concept of the thyroid-gut axis (TGA) has emerged as a means to delve into the interconnectedness between the thyroid and the gut (16). While a correlation has been established between the gut microbiota, its metabolites, and hypothyroidism (17), the causal relationship remains unclear.
Due to ethical considerations and challenges in experimental procedures, it is challenging to establish causal relationships between them through experimental means. To achieve this, we utilized Mendelian randomization (MR) methods, which involve the application of instrumental variable (IV) analysis using genetic variants. The design is comparable to that of a randomized controlled trial. Given that there is usually a high degree of association between gut microbiota, we further verified it with multivariate Mendelian randomization (MVMR) after we used univariate MR to screen out meaningful microbiota.
In our study, we conducted a univariable and multivariable MR study using large-scale genome-wide association study (GWAS) datasets to explore the genetic relationship between the gut microbiota, its metabolites, and autoimmune hypothyroidism. This research yields fresh perspectives on the pathogenesis of thyroid disorders and offers potential clinical management strategies.
2 Materials and methods
2.1 Study design
In this study, univariable MR analysis and MVMR analysis were conducted on the overall profile of the gut microbiota, metabolites derived from the gut microbiota, and autoimmune hypothyroidism. MR analysis revealed a potential causal relationship between the gut microbiota and its metabolites and autoimmune hypothyroidism. Finally, reverse MR analysis was performed to assess the possibility of reverse causality.
2.2 Data sources
2.2.1 Gut microbiota
The genetic data of the gut microbiota were derived from the most recent GWAS summary data provided by the MiBioGen Consortium (18). This large-scale GWAS involved 18,340 participants from 24 cohorts, representing multiple ethnicities. It encompassed a total of 211 taxonomic groups, including 131 genera, 35 families, 20 orders, 16 classes, and 9 phyla.
2.2.2 Gut metabolites
The GWAS of the human metabolome from European populations (TwinsUK and KORA, N = 7824). The GWAS tested all 486 metabolite concentrations present in both datasets at each single-nucleotide polymorphism (SNP). We then obtained a list of 69 gut microbiota-derived metabolite signatures from all quantified metabolites in the GWAS via this URL (https://metacyc.org/).
2.2.3 Autoimmune hypothyroidism
The genetic data regarding autoimmune hypothyroidism were collected from the publicly released DF8 data of the Finngen database, which was made available on December 1, 2022. In this dataset, hypothyroidism is defined as strict autoimmune hypothyroidism, comprising 36,321 cases and 250,926 controls. Detailed information regarding the Finngen database can be accessed at https://r8.risteys.finngen.fi/.
2.3 IV Selection
Figure 1 illustrates a concise study flow chart. In this study, the gut microbiota traits and gut microbiota-derived metabolites were considered as exposures, while autoimmune hypothyroidism was examined as the outcome. The IVs that met the assumptions of MR were strictly chosen. The specific screening steps were as follows: (1) The correlation between IVs and gut microbiota was evaluated using a threshold of P< 1 × 10-5. (2) Screening for independent SNPs (r2< 0.001, physical distance = 10000 kb). (3) Excluded the potentially pleiotropic SNPs that were associated with outcome at a P value less than 0.05 after Bonferroni correction. (4) To satisfy the strong association with exposure, SNPs with F statistic >10 were selected as IV.
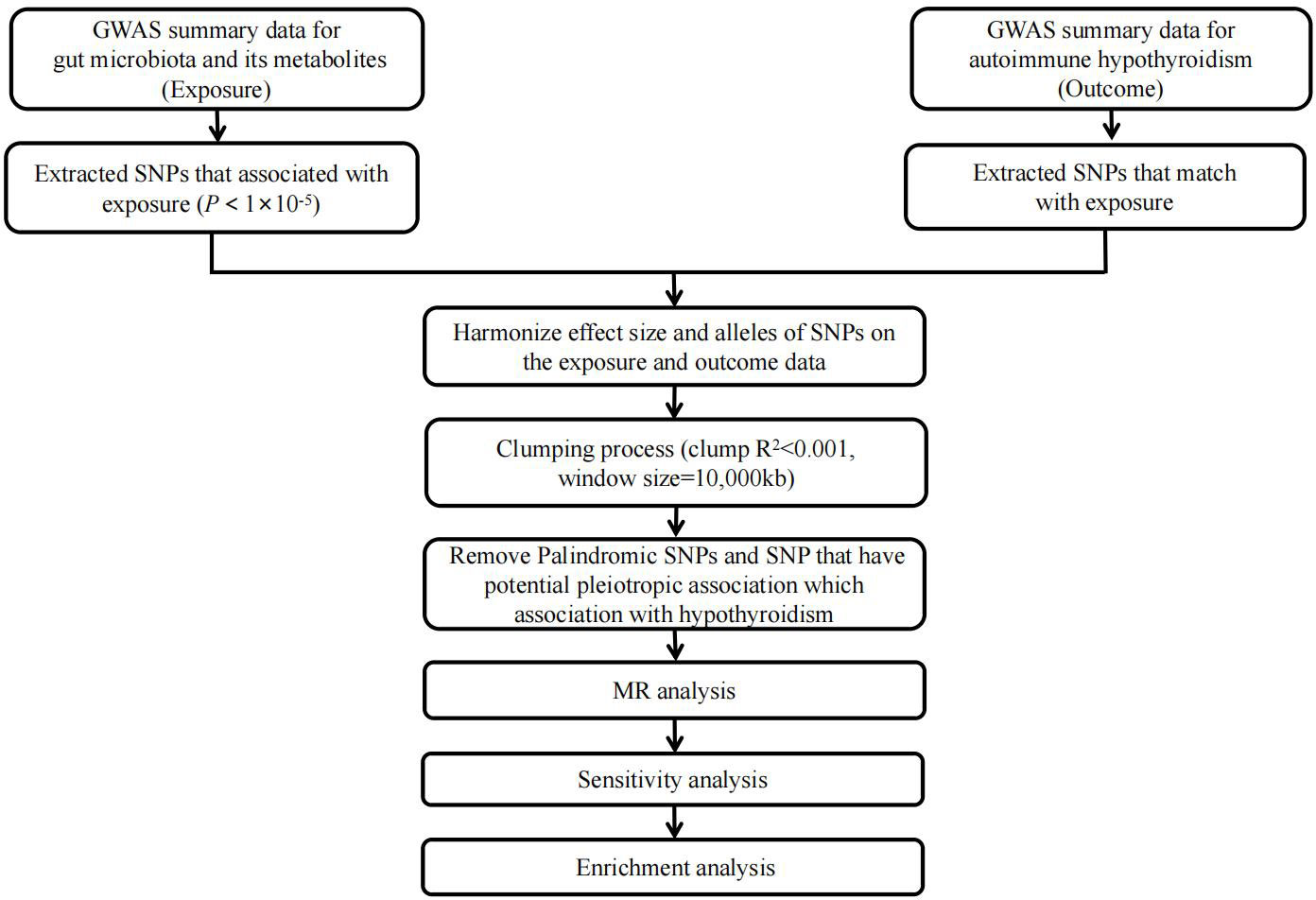
Figure 1 Study flow chart. The entire workflow of MR analysis. GWAS, Genome-wide association studies; MR, Mendelian Randomization.
( means explained by genetic variation Exposure variance proportion of; = effect; N = sample size; k = number of IVs). The F-statistic indicates the strength of the relationship between IVs and exposure (19). (5) Palindromic SNPs with intermediate allele frequencies were removed (20). (6) Checked in PhenoScanner (www.phenoscanner.medschl.cam.ac.uk) (21, 22), a platform with comprehensive information on the association of genotype and phenotype, to see whether these SNPs were associated with potential risk factors, such as other autoimmune diseases, iodine, selenium and other environmental factors.
2.4 Statistical analysis
In this study, univariable MR analysis was conducted to estimate the potential causal relationship between the gut microbiota, its metabolites, and autoimmune hypothyroidism. During the MR analysis, we alternated multiple MR methods with different model assumptions to assess the consistency and robustness of the findings. During the univariable MR analysis, we used the inverse variance weighted (IVW) method (23). As the main analysis method, the weighted median, MR Pleiotropy RESidual Sum and Outlier (MRPRESSO) and MR−Egger methods were used for the sensitivity analysis. At the same time, the MRPRESSO outlier test was used to test whether there were abnormal SNPs. To assess the heterogeneity among SNPs, Cochrane’s Q test was employed. The intercept of the MR−Egger regression test was used to estimate horizontal pleiotropy (24). Leave-one-out analysis was employed to examine whether the causal relationship between the gut microbiota, its metabolites, and autoimmune hypothyroidism was affected by individual SNPs (25). In MVMR analysis, we included the significant gut microbiota from the univariable analysis and tried to identify the independent gut microbiota. To explore whether autoimmune hypothyroidism had any causal effect on the identified important bacterial genera, we also performed a reverse MR analysis using SNPs associated with autoimmune hypothyroidism as IV (i.e., autoimmune hypothyroidism as exposure, identified pathogenic bacterial genera as a result).
To further explore the biological role of gut microbiota and metabolites in autoimmune hypothyroidism, we performed gene ontology (GO) and Kyoto Encyclopedia of Genes and Genomes (KEGG) enrichment analyses based on lead SNPs for all identified gut microbiota and metabolites. We mapped lead SNPs of causal microbiota and metabolites identified in autoimmune hypothyroidism to nearby genes.
All analyses in this study used the TwoSampleMR package (version 0.5.4) (26)MRPRESSO package (version 1.0) and MVMR package (version 0.3) in R software (version 4.1.1). Our results were corrected for multiple hypothesis testing using the false discovery rate (FDR), as the significance threshold was set at FDR-corrected P values < 0.05, while associations with P < 0.05 but not reaching the FDR-controlled threshold were reported as suggestive of association. Enrichment analysis was performed by the website tool “Metascape” (27). Reporting of the study follows the STROBE-MR statement (28) (Supplementary Table 16).
3 Results
3.1 IV selection
SNPs (rs1595463, rs16938435, rs6918730, rs11135366, rs2172426, rs4644504, rs2172426, rs11135366, rs17379710) were identified as potential pleiotropic outliers at the nominal significance level of 0.05 in the MRPRESSO outlier test. Detailed information can be found in the Supplementary Tables. The F-statistics, mostly above 10, suggested that the MR results were less likely to be affected by weak instrument bias. Furthermore, the MR−Egger regression analysis did not indicate any evidence of horizontal pleiotropy for the IVs (MR−Egger regression P > 0.05).
3.2 Univariable Mendelian randomization analysis
3.2.1 Associations between the gut microbiota and autoimmune hypothyroidism
As shown in Figure 2, IVW analysis suggested that genetic prediction of the phylum Actinobacteria (OR=0.871; 95% CI, 0.794-0.956; P = 0.004), genus. DefluviitaleaceaeUCG011 (OR=0.915; 95% CI, 0.847-0.988; P = 0.024), Genus. Eggerthella (OR=0.935; 95% CI, 0.882-0.993; P = 0.027), Family. Defluviitaleaceae (OR=0.927; 95% CI, 0.865-0.994; P = 0.033), Genus. Subdoligranulum (OR=0.903; 95% CI 0.822-0.992; P = 0.033) and genus. RuminococcaceaeUCG011 (OR=0.949; 95% CI, 0.902-0.998; P = 0.041) was associated with a reduced risk of autoimmune hypothyroidism, but the genus. Intestinimonas (OR=1.098; 95% CI, 1.029-1.172; P = 0.005) was associated with an increased risk of autoimmune hypothyroidism. Table 1 demonstrates that all P values in the heterogeneity tests were greater than 0.05, suggesting that our results were unlikely to be affected by heterogeneity bias. The MR−Egger intercept test suggested a minimal likelihood of horizontal pleiotropy (P > 0.05) (Supplementary Table 17). Furthermore, the leave-one-out analysis revealed that none of the potential outlier SNPs were included in the MR analysis (Supplementary Material).
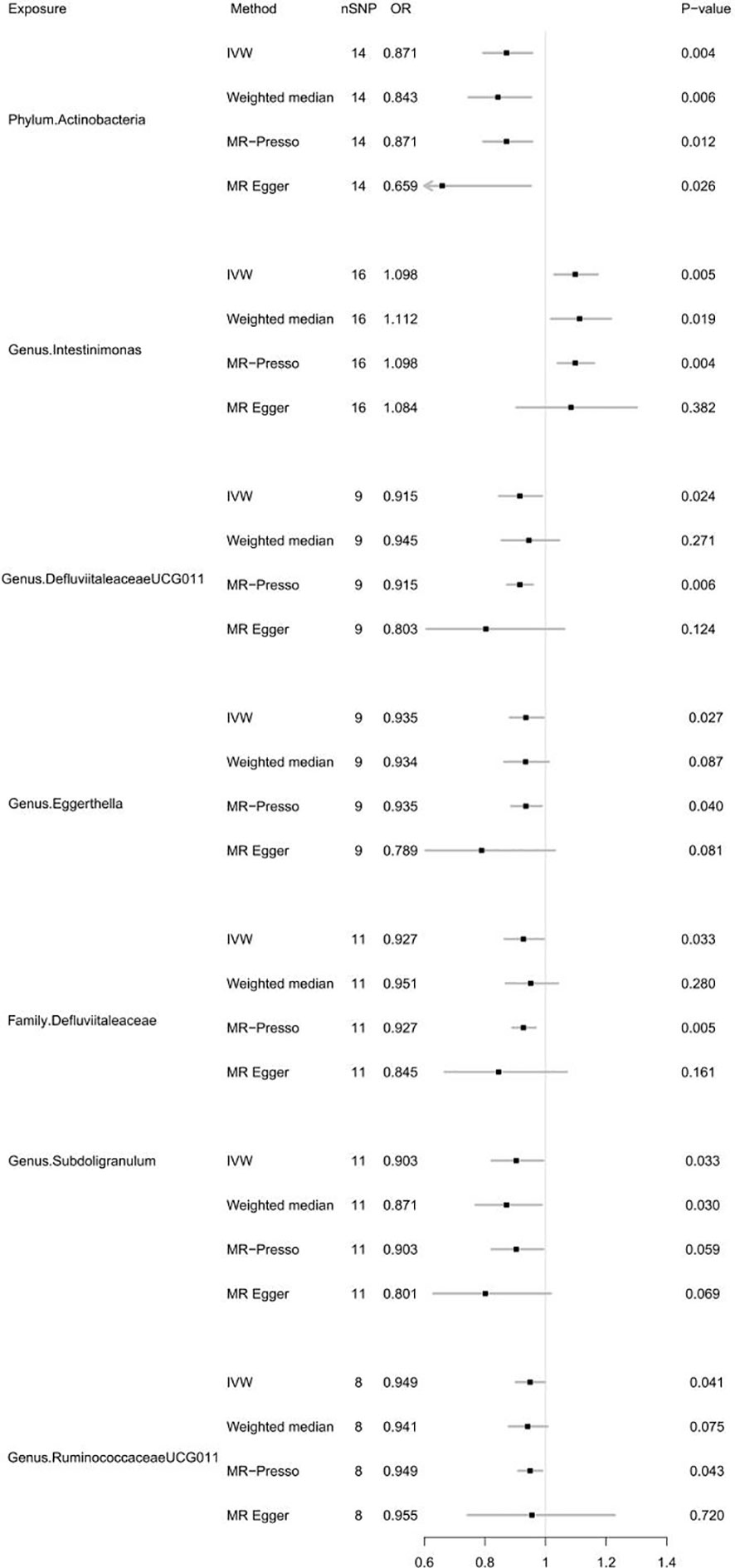
Figure 2 Forest plot of MR results for gut microbiota on autoimmune hypothyroidism. Method, statistical analysis methods; nSNP, number of SNPs; OR, odds ratio; P-value, significance P-value.
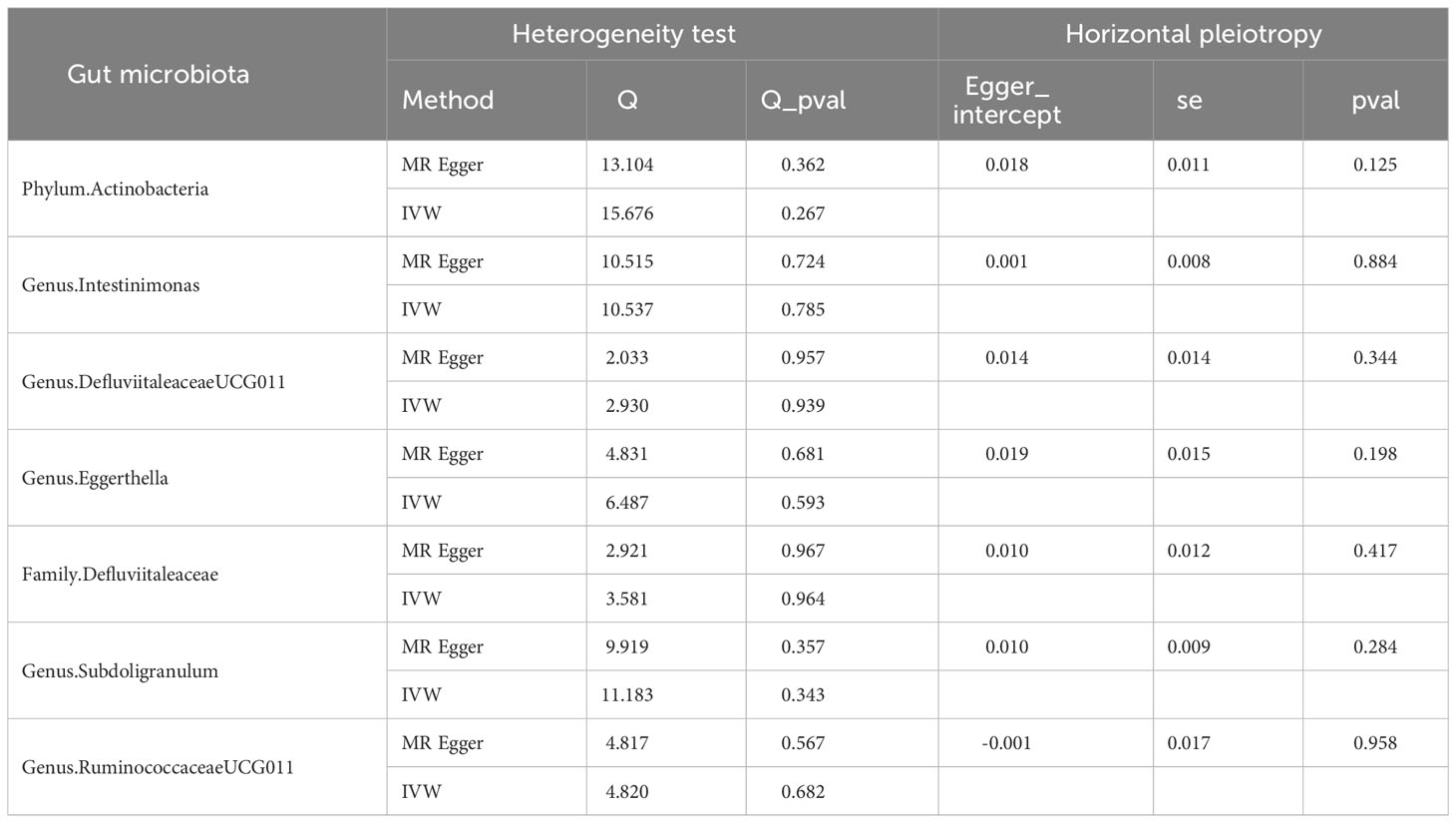
Table 1 Results of heterogeneity and horizontal pleiotropy in the MR analysis of gut microbiota for autoimmune hypothyroidism.
After FDR correction, the causal link between gut microbiota and autoimmune hypothyroidism was absent (PFDR > 0.05). In summary, phylum Actinobacteria, genus DefluviitaleaceaeUCG011, genus Eggerthella, family Defluviitaleaceae, genus Subdoligranulum and genus RuminococcaceaeUCG011 may have a borderline negative correlation with autoimmune hypothyroidism risk, and the genus Intestinimonas may have a borderline positive correlation with autoimmune hypothyroidism risk. For detailed FDR results, please refer to Supplementary Table 8.
3.2.2 Associations between gut metabolites and autoimmune hypothyroidism
For the gut microbiota-derived metabolites included in the MR analysis, we observed suggestive estimated effects for 6 gut metabolites on autoimmune hypothyroidism. Our study revealed that gut metabolites, including indololactate and alanine, were associated with an increased risk of autoimmune hypothyroidism. The odds ratio (OR) values, estimated using the IVW method, were 1.592 (95% CI, 1.228-2.065; P = 4.55×10-4) and 1.518 (95% CI, 1.033-2.229; P = 0.033). N-(3-Furoyl)glycine, pipecolate, phenylalanine and allantoin were associated with a lower risk of autoimmune hypothyroidism, and their OR values by the IVW method were 0.947 (95% CI, 0.904-0.993; P =0.024), 0.765 (95% CI, 0.598-0.977; P =0.032), 0.287 (95% CI, 0.089-0.931; P =0.038), and 0.815 (95% CI, 0.667-0.996; P =0.045), respectively. Figure 3 illustrates the relationship of these metabolites to autoimmune hypothyroidism. However, after FDR adjustment, only indololactate was positively associated with autoimmune hypothyroidism (PFDR= 0.036). Supplementary Table 15 displays the precise FDR outcomes, while Table 2 shows the outcomes of the heterogeneity test and horizontal pleiotropic test. Supplementary Table 18 presents detailed results of horizontal pleiotropy testing of gut metabolites and autoimmune hypothyroidism.
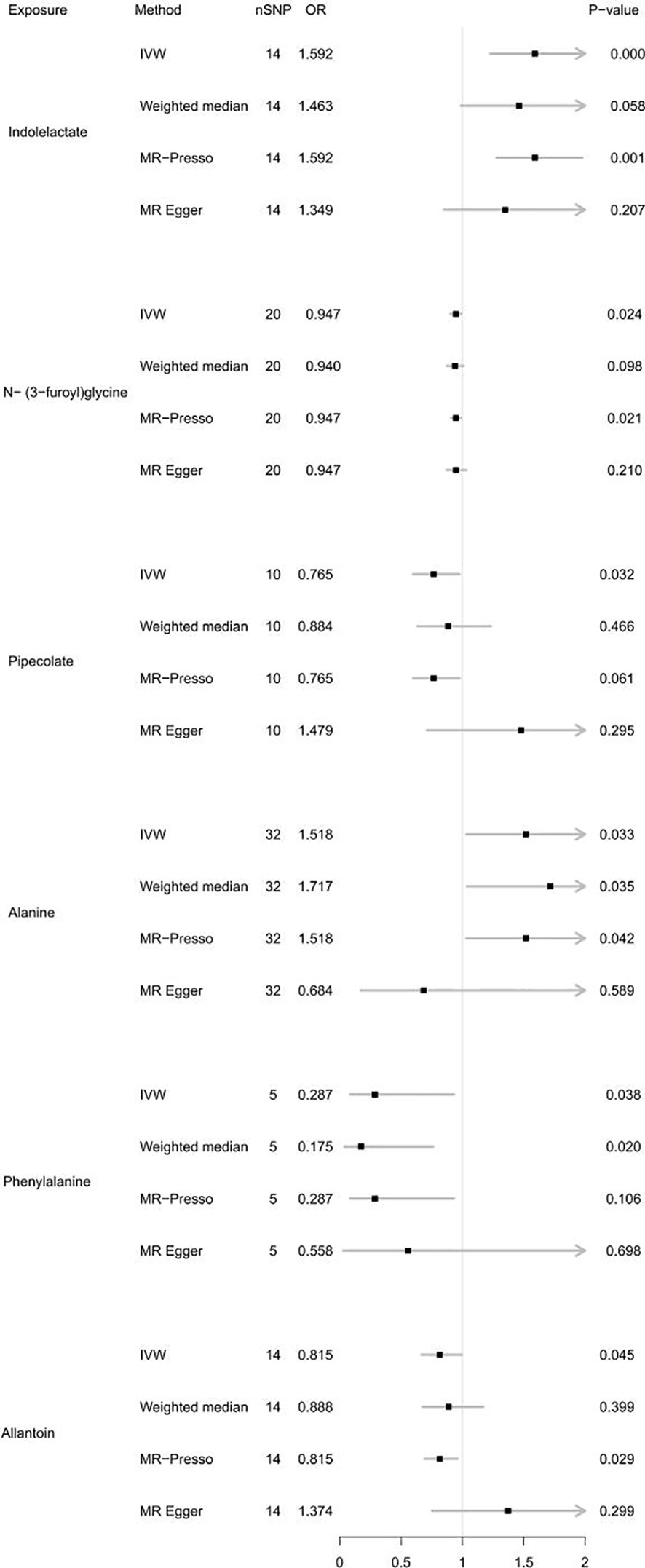
Figure 3 Forest plot of MR results for gut metabolites on autoimmune hypothyroidism. Method, statistical analysis methods; nSNP, number of SNPs; OR, odds ratio; P-value, significance P-value.
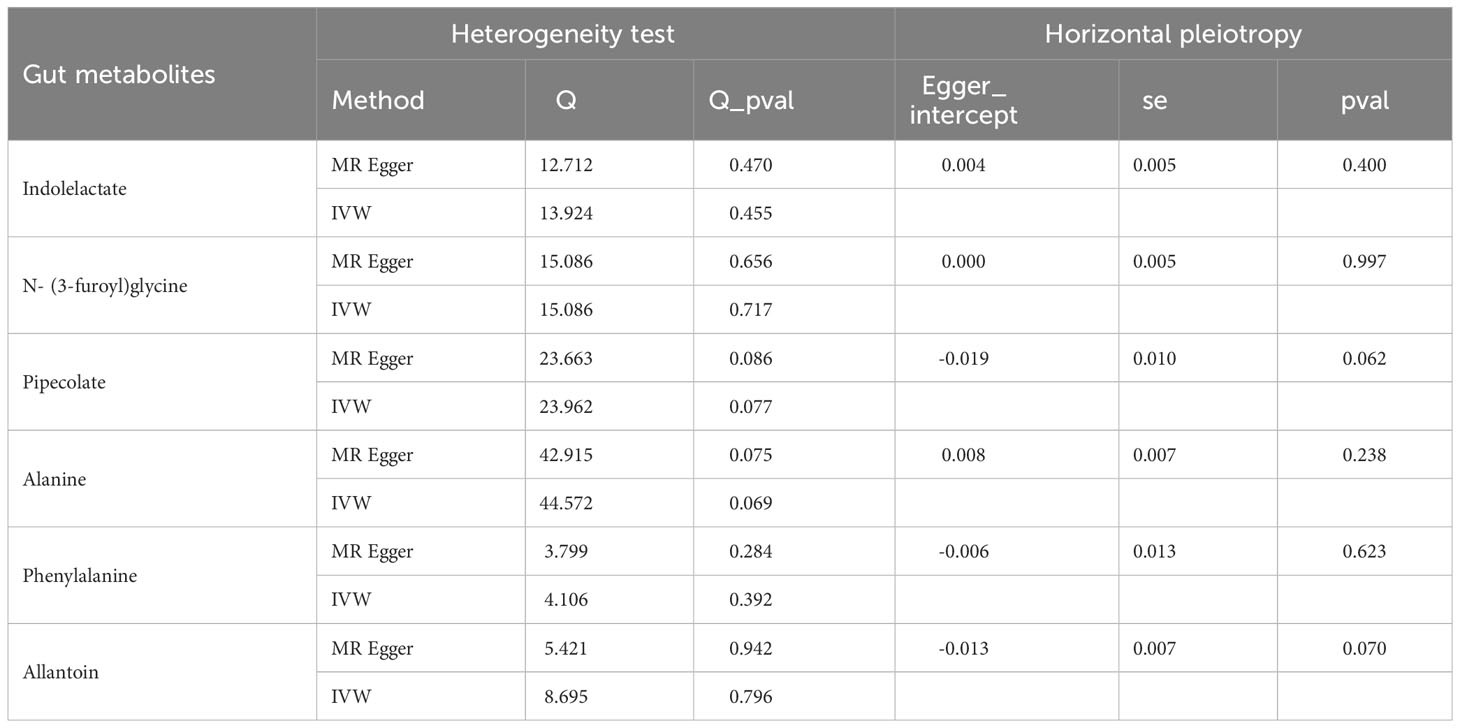
Table 2 Results of heterogeneity and horizontal pleiotropy in the MR analysis of gut metabolites for autoimmune hypothyroidism.
3.3 Multivariable Mendelian randomization analysis
To determine the influence of the gut microbiota on the risk of autoimmune hypothyroidism, we further performed MVMR analysis. The consistent direction and magnitude of the different MR models further supported causal inference. It should be noted that both the family Defluviitaleaceae and genus DefluviitaleaceaeUCG011 were also suggestively significant in the MVMR (Family Defluviitaleaceae OR =0.158; 95% CI, 0.027-0.928; P =0.041; DefluviitaleaceaeUCG011 OR =0.164; 95% CI, 0.028-0.967; P =0.046) (Table 3), although failing to pass FDR correction. The MVMR results showed no independent relationship between gut metabolites and autoimmune hypothyroidism (Table 4).
3.4 Reverse MR analysis
In the reverse MR analysis, there was no evidence of a causal influence between autoimmune hypothyroidism and the identified gut microbiota (Table 5).
3.5 Enrichment analysis
Enrichment analysis of gut microbiota, metabolites and autoimmune hypothyroidism found that several key regulatory pathways were significantly enriched. GO enrichment analysis showed that 20 GO biological processes (e.g., transcription factor TFIID complex, collagen catabolic process, aminoacyltransferase activity, and disruption of plasma membrane integrity in another organism) were associated with autoimmune hypothyroidism (Supplementary Figure 14). KEGG enrichment analysis showed that the highest enrichments were Taurine and hypotaurine metabolism (P=5.17E-5), Transcriptional misregulation in cancer (P=9.00E-5), NOD-like receptor signaling pathway (P=2.00E-4), Calcium signaling pathway (P=6.64E-4), as well as MAPK signaling pathway (P=7.07E-4) (Supplementary Figure 15).
4 Discussion
4.1 Principal findings
This is the first MR analysis to examine the genetically predictive ability of gut microbiota and metabolites on autoimmune hypothyroidism. In the present MR study, we found borderline causal associations of phylum Actinobacteria, genus DefluviitaleaceaeUCG011, genus Eggerthella, family Defluviitaleaceae, genus Subdoligranulum and genus RuminococcaceaeUCG011 with a lower risk of hypothyroidism, while the genus Intestinimonas was borderline positively associated with autoimmune hypothyroidism. Additionally, in gut metabolites, indololactate was significantly positively associated with autoimmune hypothyroidism. The gut microbiota and its metabolites, also referred to as the thyroid-gut axis, may act directly or indirectly on the thyroid by influencing intestinal microelement uptake, iodothyronine conversion and storage, and immune regulation (29).
4.2 The association between the gut microbiota and host inflammatory regulation
The widely known fact is that a dysfunctional microbiota could adversely impact the immune system and host inflammatory regulation, potentially contributing to the development of autoimmune diseases such as autoimmune thyroid diseases (AITD) (30). HT, as the most common AITD worldwide, is characterized by chronic inflammation and autoantibodies against thyroid peroxidase (TPO) and thyroglobulin (TG). Previous research has demonstrated that the majority of HT cases eventually progress to autoimmune hypothyroidism (10).
4.3 The mechanisms of action for gut microbiota and gut metabolites
Moreover, gut dysbiosis could lead to alterations in the circulating levels of gut metabolites, which could exert an influence on the absorption and utilization of vital micronutrients for the thyroid gland (31). Short-chain fatty acids (SCFAs), being one of the primary components among these metabolites (32), are predominantly produced by the gut microbiota through the fermentation of dietary fibres. They held significant importance for the intestine. On the one hand, SCFAs serve as a major energy source for enterocytes (16). On the other hand, SCFAs, especially butyrate, exert a significant influence on immune regulation and possess anti-inflammatory properties (33, 34).
Notably, the uptake of iodine was primarily facilitated by the sodium/iodine symporter (NIS). Based on previous research, it was hypothesized that altering the release of SCFAs might be a potential mechanism through which the gut microbiota can influence the expression and activity of NIS and subsequently impact thyroid iodine metabolism (29). Furthermore, the microbiota could also influence the uptake and utilization of trace elements, such as iron and zinc. Particularly crucial was the fact that these trace elements played essential roles in regulating thyroid hormone synthesis and conversion: iron was indispensable for thyroid hormone synthesis, and zinc was needed for converting T4 to T3 (30).
The tryptophan transport system T was implicated in the counter transport of tri-iodothyronine and aromatic amino acids (35). Tryptophan is metabolized by the microbiota into indole derivatives, as well as tryptamine and kynurenine metabolites (36), which reduces the amount of tryptophan in vivo. Furthermore, our finding of indolelactate as a risk factor for autoimmune hypothyroidism was consistent with previous studies. Moreover, we identified N-(3-furoyl)glycine, pipecolate, phenylalanine and allantoin as microbial metabolites that served as protective factors against autoimmune hypothyroidism. These findings may offer novel insights for potential disease interventions.
4.4 Enrichment analysis
Furthermore, GO enrichment analysis found that larger number of GO biologic processes play key role in relationship between gut microbiota, metabolites and autoimmune hypothyroidism, which have been supported by previous studies. For example, collagen catabolic process was observed to be involved in the effects of gut microbiota/metabolites on autoimmune hypothyroidism, which had been proved that hypothyroidism leads to increased collagen-based stiffness (37). MAPK signaling pathway was also observed to be involved it, which had been demonstrated that the rat thyroid proliferation induced by TSH may involve an increase in MAPK signaling (38).
4.5 Strengths and limitations
One strength of our study was that we are the first to demonstrate causal associations among gut microbiota, metabolites and autoimmune hypothyroidism using univariate and multivariate MR, with various sensitivity analyses employed to ensure the consistency and robustness of our results. Second, the most recent extensive GWAS enables the acquisition and analysis of genetic data from sizable sample populations, thereby enhancing the robustness of findings in contrast to smaller randomized controlled studies. Finally, our examination goes a step further by refining the gut microbiota taxa and scrutinizing the causal impact of each taxon on autoimmune hypothyroidism from the genus to the phylum level. This establishes a theoretical foundation for understanding the subsequent mechanisms of specific bacterial strains on autoimmune hypothyroidism and aids in the identification of novel biomarkers. However, this study had several limitations. First, the sample size for autoimmune hypothyroidism was not very large. Second, although the majority of the data used in our study were European, a small number of the microbiological data were of other races, which may have confounded our estimates to some extent. Last, limited by the public database, we only verified the reverse causality between gut microbiota and autoimmune hypothyroidism and could not conduct a reverse causality study on gut metabolites. Further studies are certainly needed to validate our findings to provide more theoretical support for mechanistic research on the “thyroid–gut” axis.
Data availability statement
Publicly available datasets were analyzed in this study. This data can be found in the article.
Ethics statement
This study was approved by the Biomedical Research Ethics Committee of Shandong Provincial Hospital (SWYX2020-187).
Author contributions
QG and HZ conceived the presented idea. XL and JY performed the computations and manuscript writing. SL, MT, XM, XW and YL were involved in the acquisition of data. YC, CK, QY, JL and LZ were involved in the interpretation of the data. All authors contributed to the article and approved the submitted version.
Funding
The author(s) declare financial support was received for the research, authorship, and/or publication of this article. This work was supported by grants from the National Natural Science Foundation of China (81670721 and 82370793).
Acknowledgments
We thank all the GWAS consortiums for making the summary statistics publicly available and are grateful to all the investigators and participants who contributed to those studies.
Conflict of interest
The authors declare that the research was conducted in the absence of any commercial or financial relationships that could be construed as a potential conflict of interest.
Publisher’s note
All claims expressed in this article are solely those of the authors and do not necessarily represent those of their affiliated organizations, or those of the publisher, the editors and the reviewers. Any product that may be evaluated in this article, or claim that may be made by its manufacturer, is not guaranteed or endorsed by the publisher.
Supplementary material
The Supplementary Material for this article can be found online at: https://www.frontiersin.org/articles/10.3389/fimmu.2023.1213159/full#supplementary-material
References
1. Eckburg PB, Bik EM, Bernstein CN, Purdom E, Dethlefsen L, Sargent M, et al. Diversity of the human intestinal microbial flora. Science (2005) 308(5728):1635–8. doi: 10.1126/science.1110591
2. Febbraio MA, Reibe S, Shalapour S, Ooi GJ, Watt MJ, Karin M. Preclinical models for studying NASH-driven HCC: how useful are they? Cell Metab (2019) 29(1):18–26. doi: 10.1016/j.cmet.2018.10.012
3. Chen C, Yin Q, Wu H, Cheng L, Kwon JI, Jin J, et al. Different effects of premature infant formula and breast milk on intestinal microecological development in premature infants. Front Microbiol (2019) 10:3020. doi: 10.3389/fmicb.2019.03020
4. Lerner A, Jeremias P, Matthias T. Gut-thyroid axis and celiac disease. Endocr Connect (2017) 6(4):R52–r58. doi: 10.1530/ec-17-0021
5. O'Hara AM, Shanahan F. The gut flora as a forgotten organ. EMBO Rep (2006) 7(7):688–93. doi: 10.1038/sj.embor.7400731
6. Kubinyi E, Bel Rhali S, Sándor S, Szabó A, Felföldi T. Gut microbiome composition is associated with age and memory performance in pet dogs. Anim (Basel) (2020) 10(9):1488. doi: 10.3390/ani10091488
7. Peyrottes A, Coquant G, Brot L, Rainteau D, Seksik P, Grill JP, et al. Anti-inflammatory effects of analogues of N-Acyl homoserine lactones on eukaryotic cells. Int J Mol Sci (2020) 21(24):9448. doi: 10.3390/ijms21249448
8. Vitale G, Dicitore A, Barrea L, Sbardella E, Razzore P, Campione S, et al. From microbiota toward gastro-enteropancreatic neuroendocrine neoplasms: Are we on the highway to hell? Rev Endocr Metab Disord (2021) 22(3):511–25. doi: 10.1007/s11154-020-09589-y
9. Lv LX, Fang DQ, Shi D, Chen DY, Yan R, Zhu YX, et al. Alterations and correlations of the gut microbiome, metabolism and immunity in patients with primary biliary cirrhosis. Environ Microbiol (2016) 18(7):2272–86. doi: 10.1111/1462-2920.13401
10. Caturegli P, De Remigis A, Rose NR. Hashimoto thyroiditis: clinical and diagnostic criteria. Autoimmun Rev (2014) 13(4-5):391–7. doi: 10.1016/j.autrev.2014.01.007
11. Hiromatsu Y, Satoh H, Amino N. Hashimoto's thyroiditis: history and future outlook. Hormones (Athens) (2013) 12(1):12–8. doi: 10.1007/bf03401282
12. Virili C, Stramazzo I, Centanni M. Gut microbiome and thyroid autoimmunity. Best Pract Res Clin Endocrinol Metab (2021) 35(3):101506. doi: 10.1016/j.beem.2021.101506
13. Penhale WJ, Young PR. The influence of the normal microbial flora on the susceptibility of rats to experimental autoimmune thyroiditis. Clin Exp Immunol (1988) 72(2):288–92.
14. Ishaq HM, Mohammad IS, Guo H, Shahzad M, Hou YJ, Ma C, et al. Molecular estimation of alteration in intestinal microbial composition in Hashimoto's thyroiditis patients. BioMed Pharmacother (2017) 95:865–74. doi: 10.1016/j.biopha.2017.08.101
15. Zhao F, Feng J, Li J, Zhao L, Liu Y, Chen H, et al. Alterations of the gut microbiota in Hashimoto's thyroiditis patients. Thyroid (2018) 28(2):175–86. doi: 10.1089/thy.2017.0395
16. Knezevic J, Starchl C, Tmava Berisha A, Amrein K. Thyroid-gut-axis: how does the microbiota influence thyroid function? Nutrients (2020) 12(6):1769. doi: 10.3390/nu12061769
17. Su X, Zhao Y, Li Y, Ma S, Wang Z. Gut dysbiosis is associated with primary hypothyroidism with interaction on gut-thyroid axis. Clin Sci (Lond) (2020) 134(12):1521–35. doi: 10.1042/cs20200475
18. Kurilshikov A, Medina-Gomez C, Bacigalupe R, Radjabzadeh D, Wang J, Demirkan A, et al. Large-scale association analyses identify host factors influencing human gut microbiome composition. Nat Genet (2021) 53(2):156–65. doi: 10.1038/s41588-020-00763-1
19. Burgess S, Thompson SG. Avoiding bias from weak instruments in Mendelian randomization studies. Int J Epidemiol (2011) 40(3):755–64. doi: 10.1093/ije/dyr036
20. Cao Z, Wu Y, Li Q, Li Y, Wu J. A causal relationship between childhood obesity and risk of osteoarthritis: results from a two-sample Mendelian randomization analysis. Ann Med (2022) 54(1):1636–45. doi: 10.1080/07853890.2022.2085883
21. Kamat MA, Blackshaw JA, Young R, Surendran P, Burgess S, Danesh J, et al. PhenoScanner V2: an expanded tool for searching human genotype-phenotype associations. Bioinformatics (2019) 35(22):4851–3. doi: 10.1093/bioinformatics/btz469
22. Staley JR, Blackshaw J, Kamat MA, Ellis S, Surendran P, Sun BB, et al. PhenoScanner: a database of human genotype-phenotype associations. Bioinformatics (2016) 32(20):3207–9. doi: 10.1093/bioinformatics/btw373
23. Burgess S, Butterworth A, Thompson SG. Mendelian randomization analysis with multiple genetic variants using summarized data. Genet Epidemiol (2013) 37(7):658–65. doi: 10.1002/gepi.21758
24. Bowden J, Davey Smith G, Burgess S. Mendelian randomization with invalid instruments: effect estimation and bias detection through Egger regression. Int J Epidemiol (2015) 44(2):512–25. doi: 10.1093/ije/dyv080
25. Lee YH. Causal association between smoking behavior and the decreased risk of osteoarthritis: a Mendelian randomization. Z Rheumatol (2019) 78(5):461–6. doi: 10.1007/s00393-018-0505-7. Kausalzusammenhang zwischen Rauchen und dem verminderten Risiko für Arthrose: eine Mendel-Randomisierung.
26. Hemani G, Zheng J, Elsworth B, Wade KH, Haberland V, Baird D, et al. The MR-Base platform supports systematic causal inference across the human phenome. Elife (2018) 7:e34408. doi: 10.7554/eLife.34408
27. Zhou Y, Zhou B, Pache L, Chang M, Khodabakhshi AH, Tanaseichuk O, et al. Metascape provides a biologist-oriented resource for the analysis of systems-level datasets. Nat Commun (2019) 10(1):1523. doi: 10.1038/s41467-019-09234-6
28. Skrivankova VW, Richmond RC, Woolf BAR, Yarmolinsky J, Davies NM, Swanson SA, et al. Strengthening the reporting of observational studies in epidemiology using Mendelian randomization: the STROBE-MR statement. JAMA (2021) 326(16):1614–21. doi: 10.1001/jama.2021.18236
29. Jiang W, Lu G, Gao D, Lv Z, Li D. The relationships between the gut microbiota and its metabolites with thyroid diseases. Front Endocrinol (Lausanne) (2022) 13:943408. doi: 10.3389/fendo.2022.943408
30. Fröhlich E, Wahl R. Microbiota and thyroid interaction in health and disease. Trends Endocrinol Metab (2019) 30(8):479–90. doi: 10.1016/j.tem.2019.05.008
31. Chen YH, Yuan W, Meng LK, Zhong JC, Liu XY. The role and mechanism of gut microbiota in pulmonary arterial hypertension. Nutrients (2022) 14(20):4278. doi: 10.3390/nu14204278
32. Sam QH, Ling H, Yew WS, Tan Z, Ravikumar S, Chang MW, et al. The divergent immunomodulatory effects of short chain fatty acids and medium chain fatty acids. Int J Mol Sci (2021) 22(12):6453. doi: 10.3390/ijms22126453
33. Köhling HL, Plummer SF, Marchesi JR, Davidge KS, Ludgate M. The microbiota and autoimmunity: Their role in thyroid autoimmune diseases. Clin Immunol (2017) 183:63–74. doi: 10.1016/j.clim.2017.07.001
34. Mori K, Nakagawa Y, Ozaki H. Does the gut microbiota trigger Hashimoto's thyroiditis? Discovery Med (2012) 14(78):321–6. doi: 10.1055/a-2190-3847
35. Zhou Y, Samson M, Francon J, Blondeau JP. Thyroid hormone concentrative uptake in rat erythrocytes. Involvement of the tryptophan transport system T in countertransport of tri-iodothyronine and aromatic amino acids. Biochem J (1992) 281(Pt 1):81–6. doi: 10.1042/bj2810081
36. Ning J, Huang SY, Chen SD, Zhang YR, Huang YY, Yu JT. Investigating casual associations among gut microbiota, metabolites, and neurodegenerative diseases: A Mendelian randomization study. J Alzheimers Dis (2022) 87(1):211–22. doi: 10.3233/jad-215411
37. Wu Y, Peng J, Campbell KB, Labeit S, Granzier H. Hypothyroidism leads to increased collagen-based stiffness and re-expression of large cardiac titin isoforms with high compliance. J Mol Cell Cardiol (2007) 42(1):186–95. doi: 10.1016/j.yjmcc.2006.09.017
Keywords: gut microbiota, gut metabolites, autoimmune hypothyroidism, mendelian randomization, thyroid-gut axis
Citation: Liu X, Yuan J, Liu S, Tang M, Meng X, Wang X, Li Y, Chai Y, Kou C, Yang Q, Li J, Zhang L, Guan Q and Zhang H (2024) Investigating causal associations among gut microbiota, metabolites and autoimmune hypothyroidism: a univariable and multivariable Mendelian randomization study. Front. Immunol. 14:1213159. doi: 10.3389/fimmu.2023.1213159
Received: 27 April 2023; Accepted: 04 December 2023;
Published: 04 January 2024.
Edited by:
Ger Rijkers, University College Roosevelt, NetherlandsReviewed by:
An Jin Zhang, Shanghai University of Medicine and Health Sciences, ChinaPing Zeng, Xuzhou Medical University, China
Copyright © 2024 Liu, Yuan, Liu, Tang, Meng, Wang, Li, Chai, Kou, Yang, Li, Zhang, Guan and Zhang. This is an open-access article distributed under the terms of the Creative Commons Attribution License (CC BY). The use, distribution or reproduction in other forums is permitted, provided the original author(s) and the copyright owner(s) are credited and that the original publication in this journal is cited, in accordance with accepted academic practice. No use, distribution or reproduction is permitted which does not comply with these terms.
*Correspondence: Qingbo Guan, doctorguanqingbo@163.com; Haiqing Zhang, zhanghq@sdu.edu.cn
†These authors have contributed equally to this work and share first authorship
‡ORCID: Haiqing Zhang, orcid.org/0000-0003-4396-4793