- Department of Neurology, West China Hospital, Sichuan University, Guo Xuexiang, Chengdu, China
Background: An increasing number of studies have elucidated a close nexus between COVID-19 phenotypes and neuromyelitis optica spectrum disorder (NMOSD), yet the causality between them remains enigmatic.
Methods: In this study, we conducted a Mendelian randomization (MR) analysis employing summary data sourced from genome-wide association studies (GWAS) pertaining to COVID-19 susceptibility, hospitalization, severity, and NMOSD. The primary MR analysis employed the Inverse variance weighted (IVW) approach, which was supplemented by MR-Egger, weighted median, simple mode, and weighted mode methods. We implemented various sensitivity analyses including Cochran’s Q test, MR-PRESSO method, MR-Egger intercept, leave-one-out analysis, and funnel plot.
Results: The MR results demonstrated a nominal association between COVID-19 susceptibility and the risk of AQP4+ NMOSD, as evidenced by the IVW method (OR = 4.958; 95% CI: 1.322-18.585; P = 0.018). Conversely, no causal association was observed between COVID-19 susceptibility, hospitalization, or severity and the increased risk of NMOSD, AQP4-NMOSD, or AQP4+ NMOSD. The comprehensive sensitivity analyses further bolstered the robustness and consistency of the MR estimates.
Conclusion: Our findings provide compelling evidence for a causal effect of COVID-19 phenotype on AQP4+ NMOSD, shedding new light on the understanding of the comorbidity between COVID-19 and NMOSD.
Introduction
Coronavirus disease 2019 (COVID-19), caused by severe acute respiratory syndrome coronavirus 2 (SARS-CoV-2), has triggered a global panic and posed a significant public health threat (1). The phenotype of COVID-19 including susceptibility, hospitalization and severity showed a high degree of heterogeneity (2). Specifically, most patients presented as asymptomatic, and a small proportion required hospitalization or even death. Changes in the neurological and psychiatric sequelae of COVID-19 survivors were tracked (1). The COVID-19 pandemic spans less than four years to date. Therefore, the physical and mental effects of COVID-19 over a longer timeline are still unknown.
Accumulating evidence suggests that SARS-CoV-2 can penetrate the blood-brain barrier and multiple pro-inflammatory cytokines such as IL-6, IL-10, and IFN-γ, trigger a cytokine storm that induces immune dysregulation leading to CNS demyelinating lesions (3–5). These factors are closely related to neuromyelitis optica spectrum disorder (NMOSD). A growing body of case reports or series has documented the emergence of NMOSD subsequent to COVID-19 infection or COVID-19 vaccination (6–9). Although these studies show a clear chronological sequence, it remains uncertain whether COVID-19 infection caused or triggered latent NMOSD. Clarifying the causal relationship between them is urgent in the COVID-19 era.
Mendelian randomization (MR) is a robust approach employed to evaluate the causal relationship between exposure factors and outcomes, leveraging single nucleotide polymorphisms (SNPs) as instrumental variables (IVs) (10, 11). The utilization of the MR approach offers notable advantages in mitigating residual confounding, as the random allocation of genetic variants during conception ensures minimal association with confounding factors. Moreover, this method effectively mitigates the issue of reverse causality, as the genetic variations utilized to approximate the effects of exposure remain unaffected by the occurrence and progression of the outcome (12). Herein, we applied a two-sample MR approach to investigate the causal effects of COVID-19 susceptibility, hospitalization, and severity on NMOSD. The overall design flow of this study is shown in Figure 1.
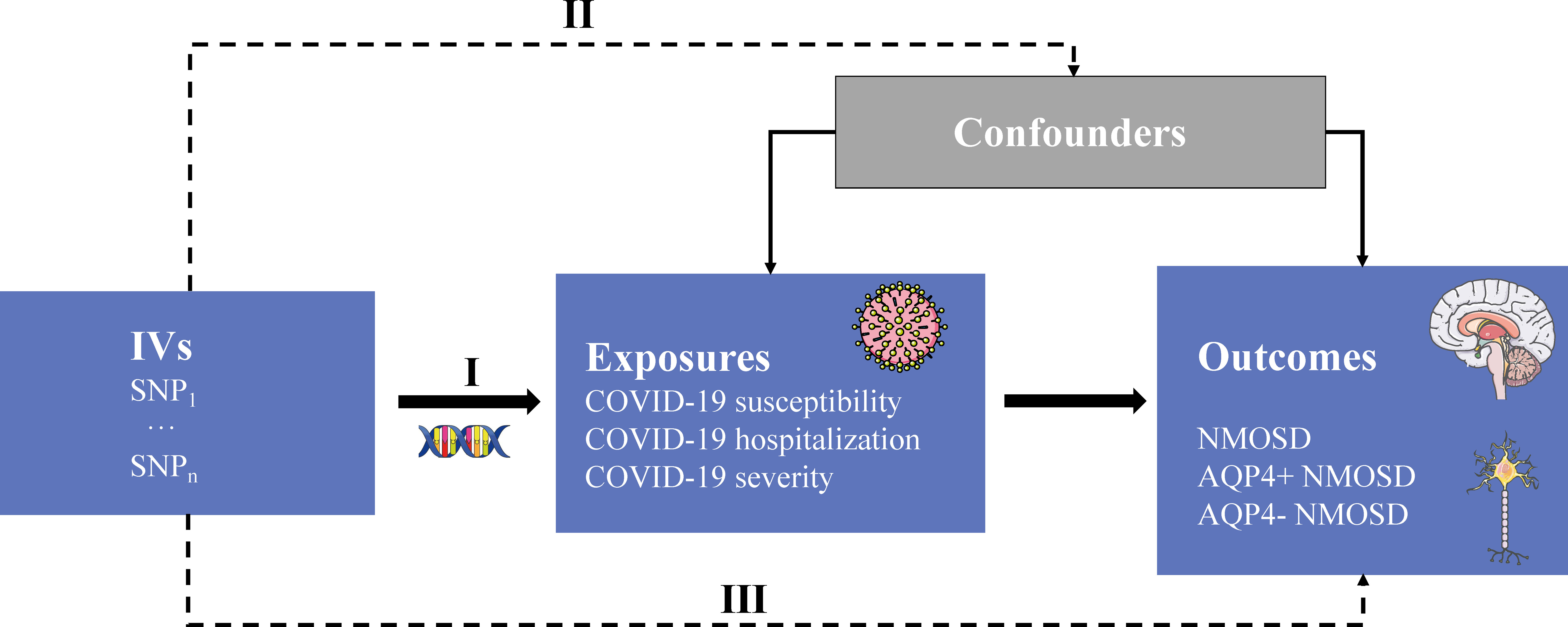
Figure 1 Study overview. IVs, instrumental variables; SNP, single nucleotide polymorphism; AQP4, aquaporin-4 antibody; +/-, positive/negative; NMOSD, neuromyelitis optica spectrum disease. Three core assumptions: (I) genetic variants that are IVs must be strongly associated with the exposure of interest; (II) SNPs should not be associated with any potential confounders; and (III) SNPs can only influence outcome trait through risk factors that represent the exposure not through other causal pathways.
Methods
Data sources and genetic instruments
Exposure sources
COVID-19
We used the most current available data pertaining to COVID-19 phenotypes, sourced from the COVID-19 Host Genetics Initiative (2) (RELEASE 7, updated April 2022). This comprehensive dataset encompasses three distinct components: COVID-19 susceptibility, COVID-19 hospitalization, and COVID-19 severity. Notably, COVID-19 susceptibility involved the analysis of 159,840 cases and 2,782,977 controls, with reliable SARS-CoV-2 infection serving as the defining criterion. The examination of COVID-19 hospitalization encompassed 44,986 cases and 2,356,386 controls, focusing on patients who required hospitalization due to COVID-19. Additionally, the assessment of severe COVID-19 phenotypes entailed 18,152 cases and 1,145,546 controls, specifically targeting individuals who either succumbed to the illness or necessitated respiratory support (13). Detailed information regarding these parameters can be found in Tables 1, 2.
Outcome sources
The NMOSD trait analyzed in this study was derived from an extensive GWAS dataset, comprising 215 individuals diagnosed with NMOSD, including 132 with aquaporin-4 antibody-positive NMOSD (AQP4+NMOSD), 83 with aquaporin-4 antibody-negative NMOSD (AQP4-NMOSD), and a control group of 1,244 healthy individuals (14). The researchers conducted a preliminary study (Stage I) that included 86 individuals diagnosed with NMOSD and a control group of 460 healthy participants. In the subsequent phase (Stage II), they expanded their investigation to include 129 individuals with NMOSD and an additional 784 healthy controls. Following these phases, a comprehensive meta-analysis was conducted. Through meticulous analysis, this study successfully identified two distinct signals within the major histocompatibility complex region that are significantly associated with NMOSD. Notably, the findings revealed that AQP4+NMOSD exhibits a greater genetic resemblance to systemic lupus erythematosus (SLE) than to multiple sclerosis (MS). The clinical characteristics of the NMOSD sample at both stages are shown in Table 3.
Selection of instrumental variables
First, we identified SNPs that exhibited strong associations (P < 5E-8) with COVID-19 phenotypes. Second, a clumping procedure was applied to SNPs representing the COVID-19 phenotypes, employing a cut-off value of R2 < 0.001 and a clumping window of 10,000 kb. Third, SNP proxies were used if no IVs were extracted from the outcome trait (minimum LD R2 value 0.8). Fourth, we harmonized the selected IVs and removed palindromic SNPs to avoid potential reverse causality. In addition, the F-statistic was computed for each SNP to address weak instrument bias (10). F-statistic > 10 implies strong instrument strength. The F-statistic was derived using the following formula: R2 × (N-k-1)/[(1-R2) × k], where N represents the sample size of the COVID-19 phenotype GWAS, k denotes the number of SNPs, and R2 signifies the proportion of variability in the COVID-19 phenotype that can be explained by each SNP. Additionally, R2 was computed using the formula: 2 × beta2 × (1-EAF) × EAF, with EAF representing the effect allele frequency and beta representing the estimated genetic effect of each SNP on the COVID-19 phenotype (15, 16).
MR analysis and sensitivity analysis
The primary estimation of causal effects was conducted using the random-effects inverse-variance weighted (IVW) method, which combines the Wald ratio of each SNP pair outcome to generate an overall causal estimate (17). We also employed MR-Egger, weighted median, simple mode, and weighted mode methods to complement the IVW. The Cochran’s Q test of the IVW approach was used to investigate heterogeneity. To detect horizontal pleiotropy, we utilized the MR-Egger intercept test and MR Pleiotropy Residual Sum and Outlier test (MR-PRESSO). Robustness and consistency of the MR results were evaluated through leave-one-out analysis and funnel plot (18). In addition, we utilized a widely used tool (https://shiny.cnsgenomics.com/mRnd/) to evaluate the statistical power of our MR estimates. Although any violations of the MR hypothesis were evaluated in extensive sensitivity analyses, we used the PhenoScanner tool (19, 20) to assess whether genetic variants were associated with potential confounders including age, sex, and autoimmune disease (21–23). Multiple comparisons were corrected by Bonferroni measures (P=0.05/9 = 0.001). P < 0.001 was considered statistically significant. P < 0.05 was regarded as nominally significant. Our study followed the STROBE-MR Statement (24). All statistical analyses were performed using the TwoSampleMR package (v 0.5.6) within the R software (v 4.2.1).
Results
In our MR studies, we obtained strongly correlated (P < 5E-8) independent (R2 < 0.001) SNPs for exposure. All F-statistics were greater than 10 (from 729 to 99007). These SNPs explained between 1.73% and 24.08% of the variance of exposure. All MR estimates of power were greater than 0.8, representing sufficient (Supplementary Table S1).
MR effect of COVID-19 phenotypes on NMOSD
We observed no statistically significant effect of COVID-19 susceptibility on the increased risk of NMOSD or AQP4-NMOSD (NMOSD: OR = 1.915, 95% CI: 0.647 - 5.667, P = 0.240; AQP4-NMOSD: OR = 0.565, 95% CI: 0.099 to 3.212, P = 0.520; Supplementary Figures S1-3). Similarly, we found no evidence to support a causal association between COVID-19 hospitalization and severity on NMOSD, AQP4+NMOSD or AQP4-NMOSD (Figure 2). Interestingly, genetic liability to COVID-19 susceptibility was nominally associated with AQP4+NMOSD by implementing the IVW approach (OR = 4.958, 95% CI: 1.322-18.585, P = 0.018; Figure 2), consistent with MR Egger, weighted median and weighted mode (Supplementary Figures S1-3).
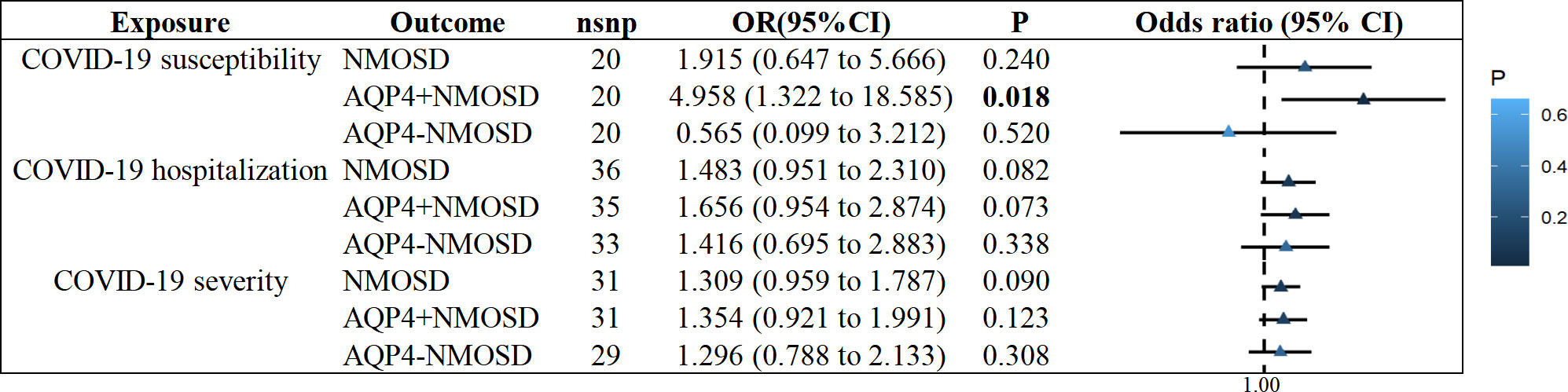
Figure 2 IVW results of COVID−19 phenotypes on risk of NMOSD. IVW, inverse-variance weighted method; NMOSD, neuromyelitis optica spectrum disease.
Sensitivity analysis
In our comprehensive MR analyses, the Cochran’s Q test yielded p-values greater than 0.05, suggesting the absence of heterogeneity. The Egger intercept, approaching zero with a p-value exceeding 0.05, indicated no potential horizontal pleiotropy. Furthermore, the MR-PRESSO test detected no outliers of horizontal pleiotropy that could compromise our MR estimates (all Global Test p-values > 0.05) (Table 4). The robustness of our findings was confirmed by leave-one-out analyses, which demonstrated that MR estimates were not influenced by individual SNPs. Funnel plots were almost symmetrical with the IVW method (Supplementary Figures S1-3). These results further strengthen the robustness and consistency of MR results.
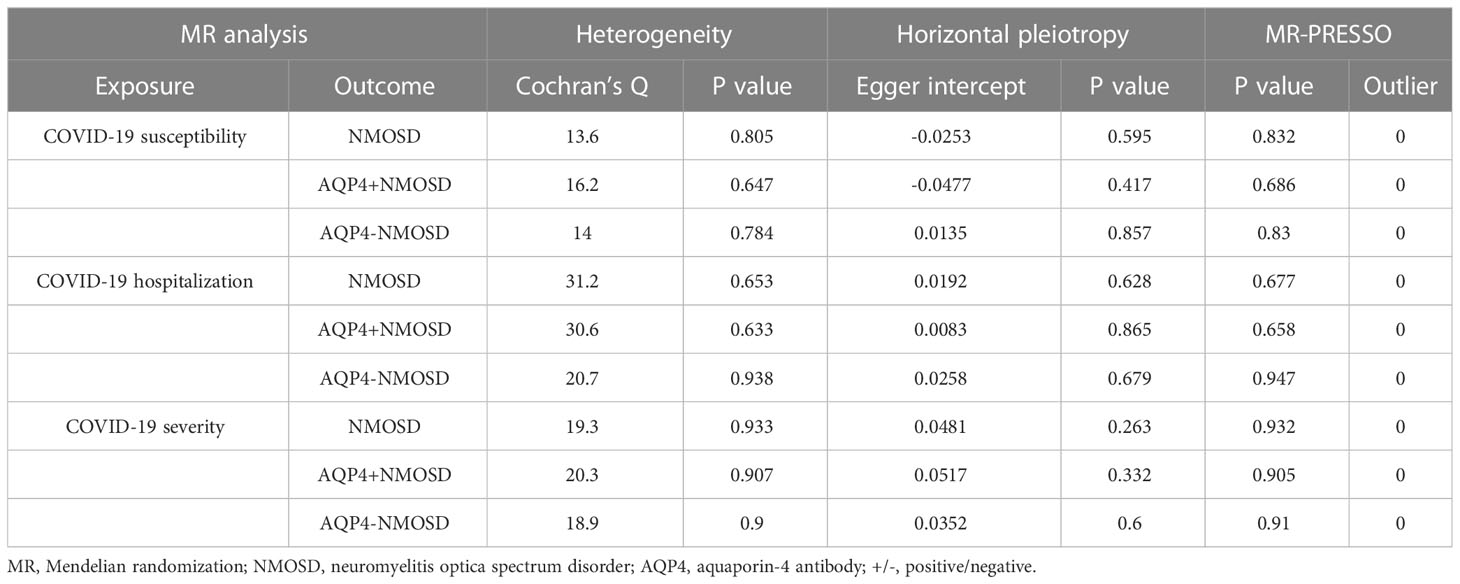
Table 4 Sensitivity analysis of the causal association between COVID-19 phenotype and the risk of NMOSD.
Confounding analysis
Although extensive sensitivity analyses indicated no bias in MR estimates, we manually retrieved potential confounders using the Phenoscanner online tool. We identified associations of rs505922 with Graves’ disease, rs9916158 with ulcerative colitis, rs1634761 and rs1128175 with self-reported MS, and rs13107325, rs914615 and rs646327 with Crohn’s disease. After excluding these SNPs, the causal association of COVID-19 susceptibility with AQP4+NMOSD remained nominally significant (IVW: OR = 5.090, 95% CI: 1.151-22.505, P = 0.032). The remaining MR analyses were consistent with previous results (Supplementary Table S2).
Discussion
To our knowledge, we have comprehensively assessed the causal association between COVID-19 phenotypes and NMOSD for the first time. In this MR study, our findings showed that genetically predicted COVID-19 susceptibility was associated with a high risk of AQP4+ NMOSD. Extensive sensitivity analysis was consistent with the primary results, consolidating the stability and consistency of the MR analysis.
Accumulating evidence shows an inextricable association between SARS-CoV-2 infection and neuroimmune diseases (4). The underlying pathogenesis is that SARS-CoV-2 infection of multiple cells, including macrophages, neutrophils, and dendritic cells, induces an immune response in T cells and B cells by producing interferon and drives the accumulation of pro-inflammatory chemokines and cytokines, creating a “cytokine storm” that invades and damages the central and peripheral nervous system, leading to neuroinflammation and demyelination (4, 25). The development of these processes is related to the mechanisms by which viruses trigger autoimmunity including viral mimicry, propagation of epitopes, activation of bystanders, and presentation of cryptogenic antigens (26).
However, current studies on COVID-19 phenotypes in NMOSD are limited to a few case reports or case series, making it difficult to accurately assess the association between them. Several cases of optic neuritis or transverse myelitis accompanying SARS-CoV-2 infection have been reported (27–30). Other individuals have developed MRI manifestations consistent with NMOSD and positive AQP4 IgG after receiving the COVID-19 vaccine (31–33). More importantly, an increasing number of case reports demonstrate the development of NMOSD in patients after COVID-19 infection, with a proportion detecting positive AQP4 antibodies (8, 9, 34–38). Our study found the causal effect of COVID-19 susceptibility on AQP4+NMOSD, which supports previous observational findings. But the underlying mechanisms need to be clarified. Notably, although we did not find evidence for causal effects of COVID-19 phenotypes on NMOSD or AQP4-NMOSD, we had sufficient power (>0.8) to assess these associations in the current study.
To the best of our knowledge, there are currently no studies utilizing MR approach to investigate the causal effects of other infections on NMOSD. However, a recent review has highlighted the association between COVID-19 and central nervous system demyelination, emphasizing the critical importance of establishing a causal relationship (4). MS, as a distinct entity of demyelinating disorders separate from NMOSD, has received considerable attention. Despite several studies using MR methods to investigate the association between various COVID-19 phenotypes and MS, research results have yielded inconsistent conclusions. For example, Shang et al. investigated the causal effect of COVID-19 using phenotype data from the COVID-19 Host Genetics Initiative (Release 6), but found no positive association with MS (39), in agreement with the conclusions of Larsson et al. (40). However, using the largest available dataset from the COVID-19 Host Genetics Initiative (Release 7), Baranova et al. showed that genetic susceptibility to hospitalized COVID-19 had a causal effect on MS (OR: 1.15, 95%CI: 1.02-1.30, p = 0.022, FDR = 0.044) (41). The use of the most comprehensive COVID-19 phenotype data to date by Baranova et al. makes their findings more reliable and consistent with certain case reports or case series observations (6, 42, 43).
It is important to note that although MR studies have genetically confirmed the potential causal relationship between COVID phenotypes and NMOSD or MS, further validation of these findings is needed, particularly in the absence of large cohort studies. This means that MR studies can be considered as preliminary research that to some extent fills these gaps.
The advantage of the current study is that compared to traditional observational studies, we have utilized MR methods to minimize the interference of reverse causality and residual confounders. Sensitivity analysis also supported the robustness of MR estimates.
Nevertheless, several limitations exist in our study. First, our GWAS data were mainly from European populations, so estimates of the overall population still need to be clarified. Second, we could not specify the proportion of participants with a possible overlap in exposure and outcome. Third, due to the different origins of data on different COVID-19 phenotypes, individuals affected by COVID-19 may manifest phenotypic variation influenced by local factors, such as the standard of health care. Fourth, the genetic explanatory power of significant SNPs is not entirely satisfactory, suggesting the potential involvement of other factors in the occurrence of NMOSD. Due to the large sample size, this study achieved an ideal statistical power (>0.8), which implies the reliability of the statistical conclusions derived from the present research. Fifth, due to data limitations, it becomes challenging to present the clinical characteristics of COVID-19 phenotype data, such as age and gender. This limitation may hinder the clinical applicability. Ongoing MR studies have begun preliminary explorations of the causal effects of different COVID-19 phenotypes on NMOSD, which warrant further verification through cohort studies.
In conclusion, we provide strong evidence supporting the genetic liability to COVID-19 susceptibility was positively associated with the high risk of AQP4+ NMOSD. These contribute to our understanding of the prevention, diagnosis, treatment, and potential pathogenesis of NMOSD during the COVID-19 pandemic. Further studies are still warranted.
Data availability statement
The original contributions presented in the study are included in the article/Supplementary Material. Further inquiries can be directed to the corresponding author.
Author contributions
The overall research was conceptualized and designed by HZ. DS performed the data analysis and contributed to the writing and revision of the manuscript. All authors participated in data collection and monitoring. The submitted version of the manuscript was reviewed and approved by all authors.
Funding
This work received funding support from the Department of Science and Technology of Sichuan Province (2020YFS0219 and 2021YFS0173), as well as the 1·3·5 project for disciplines of excellence–Clinical Research Incubation Project, West China Hospital, Sichuan University (Grant No. 21HXFH041).
Acknowledgments
The authors express their sincere appreciation to the participants in the GWAS study for their valuable contributions. Figure 1 was created in part using Servier Medical Art, provided by Servier and licensed under a Creative Commons Attribution 3.0 unported license. Special thanks are extended to Dr. Yi Liu from the Emergency Department, West China Hospital, Sichuan University, for his assistance in image processing.
Conflict of interest
The authors declare that the research was conducted in the absence of any commercial or financial relationships that could be construed as a potential conflict of interest.
Publisher’s note
All claims expressed in this article are solely those of the authors and do not necessarily represent those of their affiliated organizations, or those of the publisher, the editors and the reviewers. Any product that may be evaluated in this article, or claim that may be made by its manufacturer, is not guaranteed or endorsed by the publisher.
Supplementary material
The Supplementary Material for this article can be found online at: https://www.frontiersin.org/articles/10.3389/fimmu.2023.1207514/full#supplementary-material
Supplementary Table S1 | Variance and power of COVID-19 phenotypes and neuromyelitis optica spectrum disorder.
Supplementary Table S2 | MR reanalysis after removal of confounding factors.
Supplementary Figure S1 | MR estimation and sensitivity analysis of COVID-19 phenotypes on neuromyelitis optica spectrum disorder (NMOSD).
Supplementary Figure S2 | MR estimation and sensitivity analysis of COVID-19 phenotypes on aquaporin-4 antibody-positive NMOSD (AQP4+NMOSD).
Supplementary Figure S3 | MR estimation and sensitivity analysis of COVID-19 phenotypes on aquaporin-4 antibody-negative NMOSD (AQP4-NMOSD).
References
1. Taquet M, Sillett R, Zhu L, Mendel J, Camplisson I, Dercon Q, et al. Neurological and psychiatric risk trajectories after SARS-CoV-2 infection: an analysis of 2-year retrospective cohort studies including 1 284 437 patients. Lancet Psychiatry (2022) 9:815–27. doi: 10.1016/S2215-0366(22)00260-7
2. The COVID-19 Host Genetics Initiative, a global initiative to elucidate the role of host genetic factors in susceptibility and severity of the SARS-CoV-2 virus pandemic. Eur J Hum Genet (2020) 28:715–8. doi: 10.1038/s41431-020-0636-6
3. Gu J, Gong E, Zhang B, Zheng J, Gao Z, Zhong Y, et al. Multiple organ infection and the pathogenesis of SARS. J Exp Med (2005) 202:415–24. doi: 10.1084/jem.20050828
4. Ismail II, Salama S. Association of CNS demyelination and COVID-19 infection: an updated systematic review. J Neurol (2022) 269:541–76. doi: 10.1007/s00415-021-10752-x
5. Kuhlmann T, Moccia M, Coetzee T, Cohen JA, Correale J, Graves J, et al. Multiple sclerosis progression: time for a new mechanism-driven framework. Lancet Neurol (2022) 22: 78–88. doi: 10.1016/S1474-4422(22)00289-7
6. Moore L, Ghannam M, Manousakis G. A first presentation of multiple sclerosis with concurrent COVID-19 infection. eNeurologicalSci (2021) 22:100299. doi: 10.1016/j.ensci.2020.100299
7. Restivo DA, Centonze D, Alesina A, Marchese-Ragona R. Myasthenia gravis associated with SARS-CoV-2 infection. Ann Intern Med (2020) 173:1027–8. doi: 10.7326/L20-0845
8. Shaw VC, Chander G, Puttanna A. Neuromyelitis optica spectrum disorder secondary to COVID-19. Br J Hosp Med (Lond) (2020) 81:1–3. doi: 10.12968/hmed.2020.0401
9. de Ruijter NS, Kramer G, Gons RAR, Hengstman GJD. Neuromyelitis optica spectrum disorder after presumed coronavirus (COVID-19) infection: A case report. Mult Scler Relat Disord (2020) 46:102474. doi: 10.1016/j.msard.2020.102474
10. Cai J, Chen X, Wang H, Wei Z, Li M, Rong X, et al. Iron status may not affect amyotrophic lateral sclerosis: A mendelian randomization study. Front Genet (2021) 12:617245. doi: 10.3389/fgene.2021.617245
11. Zhang H, Zhou Z. COVID-19 and the risk of Alzheimer's disease, amyotrophic lateral sclerosis, and multiple sclerosis. Ann Clin Transl Neurol (2022) 9: 1953–61. doi: 10.1002/acn3.51688
12. Burgess S, Small DS, Thompson SG. A review of instrumental variable estimators for Mendelian randomization. Stat Methods Med Res (2017) 26:2333–55. doi: 10.1177/0962280215597579
13. Butler-Laporte G, Nakanishi T, Mooser V, Morrison DR, Abdullah T, Adeleye O, et al. Vitamin D and COVID-19 susceptibility and severity in the COVID-19 Host Genetics Initiative: A Mendelian randomization study. PloS Med (2021) 18:e1003605. doi: 10.1371/journal.pmed.1003605
14. Estrada K, Whelan CW, Zhao F, Bronson P, Handsaker RE, Sun C, et al. A whole-genome sequence study identifies genetic risk factors for neuromyelitis optica. Nat Commun (2018) 9:1929. doi: 10.1038/s41467-018-04332-3
15. Burgess S, Thompson SG. Avoiding bias from weak instruments in Mendelian randomization studies. Int J Epidemiol (2011) 40:755–64. doi: 10.1093/ije/dyr036
16. Pierce BL, Ahsan H, Vanderweele TJ. Power and instrument strength requirements for Mendelian randomization studies using multiple genetic variants. Int J Epidemiol (2011) 40:740–52. doi: 10.1093/ije/dyq151
17. Burgess S, Butterworth A, Thompson SG. Mendelian randomization analysis with multiple genetic variants using summarized data. Genet Epidemiol (2013) 37:658–65. doi: 10.1002/gepi.21758
18. Chen X, Hong X, Gao W, Luo S, Cai J, Liu G, et al. Causal relationship between physical activity, leisure sedentary behaviors and COVID-19 risk: a Mendelian randomization study. J Transl Med (2022) 20:216. doi: 10.1186/s12967-022-03407-6
19. Kamat MA, Blackshaw JA, Young R, Surendran P, Burgess S, Danesh J, et al. PhenoScanner V2: an expanded tool for searching human genotype-phenotype associations. Bioinformatics (2019) 35:4851–3. doi: 10.1093/bioinformatics/btz469
20. Staley JR, Blackshaw J, Kamat MA, Ellis S, Surendran P, Sun BB, et al. PhenoScanner: a database of human genotype-phenotype associations. Bioinformatics (2016) 32:3207–9. doi: 10.1093/bioinformatics/btw373
21. Ma X, Kermode AG, Hu X, Qiu W. Risk of relapse in patients with neuromyelitis optica spectrum disorder: Recognition and preventive strategy. Mult Scler Relat Disord (2020) 46:102522. doi: 10.1016/j.msard.2020.102522
22. Akaishi T, Takahashi T, Fujihara K, Misu T, Abe M, Ishii T, et al. Risk factors of attacks in neuromyelitis optica spectrum disorders. J Neuroimmunol (2020) 343:577236. doi: 10.1016/j.jneuroim.2020.577236
23. Li Y, Zhang J, Zhou Y, Xie H, Duan R, Jing L, et al. Analysis of predictive risk factors in Aquaporin-4-IgG positive highly active neuromyelitis optica spectrum disorders. Front Neurol (2021) 12:731835. doi: 10.3389/fneur.2021.731835
24. Skrivankova VW, Richmond RC, Woolf BAR, Yarmolinsky J, Davies NM, Swanson SA, et al. Strengthening the reporting of observational studies in epidemiology using mendelian randomization: the STROBE-MR statement. Jama (2021) 326:1614–21. doi: 10.1001/jama.2021.18236
25. Amruta N, Chastain WH, Paz M, Solch RJ, Murray-Brown IC, Befeler JB, et al. SARS-CoV-2 mediated neuroinflammation and the impact of COVID-19 in neurological disorders. Cytokine Growth Factor Rev (2021) 58:1–15. doi: 10.1016/j.cytogfr.2021.02.002
26. Mirmosayyeb O, Ghaffary EM, Bagherieh S, Barzegar M, Dehghan MS, Shaygannejad V. Post COVID-19 infection neuromyelitis optica spectrum disorder (NMOSD): A case report-based systematic review. Mult Scler Relat Disord (2022) 60:103697. doi: 10.1016/j.msard.2022.103697
27. Rodríguez-Rodríguez MS, Romero-Castro RM, Alvarado-de la Barrera C, González-Cannata MG, García-Morales AK, Ávila-Ríos S. Optic neuritis following SARS-CoV-2 infection. J Neurovirol (2021) 27:359–63. doi: 10.1007/s13365-021-00959-z
28. Munz M, Wessendorf S, Koretsis G, Tewald F, Baegi R, Krämer S, et al. Acute transverse myelitis after COVID-19 pneumonia. J Neurol (2020) 267:2196–7. doi: 10.1007/s00415-020-09934-w
29. Kaur H, Mason JA, Bajracharya M, McGee J, Gunderson MD, Hart BL, et al. Transverse myelitis in a child with COVID-19. Pediatr Neurol (2020) 112:5–6. doi: 10.1016/j.pediatrneurol.2020.07.017
30. Zachariadis A, Tulbu A, Strambo D, Dumoulin A, Di Virgilio G. Transverse myelitis related to COVID-19 infection. J Neurol (2020) 267:3459–61. doi: 10.1007/s00415-020-09997-9
31. Badrawi N, Kumar N, Albastaki U. Post COVID-19 vaccination neuromyelitis optica spectrum disorder: Case report & MRI findings. Radiol Case Rep (2021) 16:3864–7. doi: 10.1016/j.radcr.2021.09.033
32. Chen S, Fan XR, He S, Zhang JW, Li SJ. Watch out for neuromyelitis optica spectrum disorder after inactivated virus vaccination for COVID-19. Neurol Sci (2021) 42:3537–9. doi: 10.1007/s10072-021-05427-4
33. Anamnart C, Tisavipat N, Owattanapanich W, Apiwattanakul M, Savangned P, Prayoonwiwat N, et al. Newly diagnosed neuromyelitis optica spectrum disorders following vaccination: Case report and systematic review. Mult Scler Relat Disord (2022) 58:103414. doi: 10.1016/j.msard.2021.103414
34. Das D, Bhattacharjee H, Rehman O, Deori N, Magdalene D, Bharali G, et al. Neuromyelitis optica spectrum disorder post-COVID-19 infection: A rare case report from Northeast India. Indian J Ophthalmol (2022) 70:1833–6. doi: 10.4103/ijo.IJO_61_22
35. Antonescu Ghelmez D, Moraru A, Antonescu F, Chelmambet AS, Bucur AI, Tuţă S. Double seropositive neuromyelitis optica associated with COVID-19: A case report. Front Neurol (2022) 13:1004132. doi: 10.3389/fneur.2022.1004132
36. Batum M, Kisabay Ak A, Mavioğlu H. Covid-19 infection-induced neuromyelitis optica: a case report. Int J Neurosci (2022) 132:999–1004. doi: 10.1080/00207454.2020.1860036
37. Avila M, Tan Y, Hernandez R, Zuberi H, Rivera VM. Multiple sclerosis and neuromyelitis optica spectrum disorder: onset following acute COVID-19 infection, a case series. Neurol Ther (2022) 2022:1–9. doi: 10.1007/s40120-022-00418-9
38. Corrêa DG, de Souza Lima FC, da Cruz Bezerra D, Coutinho ACJ, Hygino da Cruz LCJ. COVID-19 associated with encephalomyeloradiculitis and positive anti-aquaporin-4 antibodies: Cause or coincidence? Mult Scler (2021) 27:973–6. doi: 10.1177/1352458520949988
39. Li C, Liu J, Lin J, Shang H. COVID-19 and risk of neurodegenerative disorders: A Mendelian randomization study. Transl Psychiatry (2022) 12:283. doi: 10.1038/s41398-022-02052-3
40. Li S, Yuan S, Schooling CM, Larsson SC. A Mendelian randomization study of genetic predisposition to autoimmune diseases and COVID-19. Sci Rep (2022) 12:17703. doi: 10.1038/s41598-022-22711-1
41. Baranova A, Cao H, Teng S, Su KP, Zhang F. Shared genetics and causal associations between COVID-19 and multiple sclerosis. J Med Virol (2023) 95:e28431. doi: 10.1002/jmv.28431
42. Avila M, Tan Y, Hernandez R, Zuberi H, Rivera VM. Multiple sclerosis and neuromyelitis optica spectrum disorder: onset following acute COVID-19 infection, a case series. Neurol Ther (2023) 12:319–27. doi: 10.1007/s40120-022-00418-9
Keywords: COVID-19, causal effect, Mendelian randomization, NMOSD COVID-19, NMOSD
Citation: Sun D, Du Q, Wang R, Shi Z, Chen H and Zhou H (2023) COVID-19 and the risk of neuromyelitis optica spectrum disorder: a Mendelian randomization study. Front. Immunol. 14:1207514. doi: 10.3389/fimmu.2023.1207514
Received: 17 April 2023; Accepted: 12 July 2023;
Published: 27 July 2023.
Edited by:
Pier Paolo Sainaghi, University of Eastern Piedmont, ItalyReviewed by:
Sasitorn Siritho, Bumrungrad International Hospital, ThailandGiacomo Tondo, Vita-Salute San Raffaele University, Italy
Copyright © 2023 Sun, Du, Wang, Shi, Chen and Zhou. This is an open-access article distributed under the terms of the Creative Commons Attribution License (CC BY). The use, distribution or reproduction in other forums is permitted, provided the original author(s) and the copyright owner(s) are credited and that the original publication in this journal is cited, in accordance with accepted academic practice. No use, distribution or reproduction is permitted which does not comply with these terms.
*Correspondence: Hongyu Zhou, zhouhy@scu.edu.cn