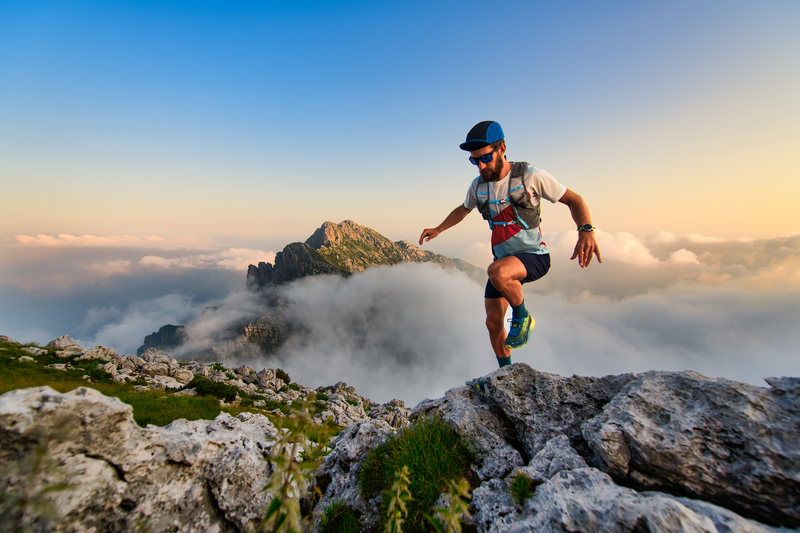
94% of researchers rate our articles as excellent or good
Learn more about the work of our research integrity team to safeguard the quality of each article we publish.
Find out more
ORIGINAL RESEARCH article
Front. Immunol. , 10 August 2023
Sec. Autoimmune and Autoinflammatory Disorders : Autoimmune Disorders
Volume 14 - 2023 | https://doi.org/10.3389/fimmu.2023.1197493
This article is part of the Research Topic COVID-19 and Autoimmunity View all 9 articles
Background: The coronavirus disease (COVID-19) pandemic is a serious threat to public health worldwide. Growing evidence reveals that there are certain links between COVID-19 and autoimmune diseases; in particular, COVID-19 and idiopathic inflammatory myopathies (IIM) have been observed to be clinically comorbid. Hence, this study aimed to elucidate the molecular mechanisms of COVID-19 and IIM from a genomic perspective.
Methods: We obtained transcriptome data of patients with COVID-19 and IIM separately from the GEO database and identified common differentially expressed genes (DEGs) by intersection. We then performed functional enrichment, PPI, machine learning, gene expression regulatory network, and immune infiltration analyses of co-expressed genes.
Results: A total of 91 common genes were identified between COVID-19 and IIM. Functional enrichment analysis revealed that these genes were mainly involved in immune dysregulation, response to external stimuli, and MAPK signaling pathways. The MCODE algorithm recognized two densely linked clusters in the common genes, which were related to inflammatory factors and interferon signaling. Subsequently, three key genes (CDKN1A, IFI27, and STAB1) were screened using machine learning to predict the occurrence of COVID-19 related IIM. These key genes exhibited excellent diagnostic performance in both training and validation cohorts. Moreover, we created TF-gene and miRNA-gene networks to reveal the regulation of key genes. Finally, we estimated the relationship between key genes and immune cell infiltration, of which IFI27 was positively associated with M1 macrophages.
Conclusion: Our work revealed common molecular mechanisms, core genes, potential targets, and therapeutic approaches for COVID-19 and IIM from a genomic perspective. This provides new ideas for the diagnosis and treatment of COVID-19 related IIM in the future.
COVID-19 is a severe contagious illness caused by severe acute respiratory syndrome coronavirus 2 (SARS-CoV-2), which primarily spreads through respiratory droplets (1, 2). Since its emergence in Wuhan at the end of 2019, it has become an epidemic that affects public health worldwide, infecting billions of people (3). COVID-19 is a multisystem disease involving the respiratory, digestive, and musculoskeletal systems (Table 1) (4). The most common symptoms are fever, cough, myalgia, dyspnea, and fatigue (5). Increased research has shown that patients with COVID-19 have several immunological abnormalities that resemble those of autoimmune diseases (6, 7). SARS-CoV-2 infection can cause chronic inflammatory and immune responses that not only directly mediate tissue damage but may also induce serious sequelae of autoimmune disease in susceptible populations (8–10). With the increasing number of people recovering from SARS-CoV-2 infection, the connection between COVID-19 and autoimmune diseases is gaining significance.
Idiopathic inflammatory myopathies (IIM), also known as myositis, represent a group of autoimmune muscle diseases with striking heterogeneity, characterized by myositis, progressive muscle weakness, and inflammatory cell infiltration of the muscle, along with other visceral organ damage (11). Based on clinical serology and pathomorphological features, IIM can be categorized into several disease subtypes, including nonspecific myositis, dermatomyositis, polymyositis, inclusion body myositis and necrotizing myopathy (12). Although there has been dramatic progress in the classification and treatment of IIM, the specific pathogenesis of IIM has not yet been fully elucidated due to the multifactorial nature of the disease. Like other types of autoimmunity, IIM is thought to be a consequence of the interaction between genetic and environmental risk factors in the absence of protective factors (13). Dysregulation of the immune system, especially in the genetic regions of human leukocyte antigens (HLA), has been recognized as an essential genetic risk factor for IIM (14). In addition, adaptive and innate immune mechanisms participate to varying degrees in different subtypes of IIM.
Notably, viral infection and vaccination have been established as critical triggers of autoimmunity in patients with IIM (15–18). Patients with COVID-19 frequently exhibit immune dysregulation, which can contribute to multiple autoimmune diseases, including IIM (19). Kharouf et al. observed that the incidence of IIM increased significantly during the COVID-19 pandemic and that these patients exhibited unique characteristics and more severe symptoms (20). The presentation of COVID-19 induced myositis may vary from significant muscle weakness to typical dermatomyositis or simply back pain with muscle disease. The pathophysiological process of the hyperinflammatory response triggered by COVID-19, leading to extensive endothelial dysfunction, vasculopathy, and thrombosis is highly similar to anti-MDA5 myositis (21). A few case reviews have also reported the risk of anti-MDA5 myositis shortly after COVID-19 vaccination (22, 23). Moreover, patients with autoimmune diseases are more susceptible to SARS-CoV-2 infections. IIM patients are more readily hospitalized for COVID-19 all-cause than healthy individuals, the course of COVID-19 is more severe, and disease activity and drug exposure are strongly linked to its severity of COVID-19 (24, 25). Additionally, following recovery from acute SARS-CoV-2 infection, a large proportion of patients develop a range of persistent symptoms and complications, also known as Long COVID (26). Long COVID accumulates in all systems and organs of the body, with common symptoms including fatigue, shortness of breath, dyspnea, muscle pain, and joint pain. Certain symptoms are typical of IIM, causing serious physical and mental health impacts (27). Growing evidence suggests that Long COVID seems to be an autoimmune disease in which the infected patient produces an “autoantibody” that attacks their own tissues, resulting in a chronic, persistent inflammatory response in recovering patients (28). Although the link between COVID-19 and autoimmune diseases has begun to attract the attention of researchers, few studies have shed light on the common molecular mechanisms of COVID-19 and IIM.
Here, we aimed to decipher the crosstalk between COVID-19 and IIM from a genetic perspective using bioinformatics analysis. Moreover, we applied machine learning to identify key biomarkers for the occurrence of COVID-19-related IIM and evaluated the effect of these markers on immune infiltration of IIM. These studies provide a deeper understanding of the comorbidity mechanisms of COVID-19 and IIM.
The COVID-19 and IIM datasets (GSE171110, GSE128470, and GSE39454) were retrieved from the GEO database. The GSE171110 dataset consisted of whole-blood gene expression profiles of 44 COVID-19 patients and 10 healthy controls (HC). The GSE128470 dataset included gene expression profiles of muscle tissues from 65 patients with IIM and 12 HCs. The GSE39454 dataset included gene expression profiles of muscle tissues from 31 patients with IIM and five HCs. Subsequently, the R package “limma” was used to analyze differentially expressed genes (DEGs). In the GSE171110 dataset, |log2FoldChange| ≥1 and |adj.P.Val.| <0.05 were set as the threshold. The GSE128470 dataset was used as the test set, with |log2FoldChange| ≥0.58496 and |adj.P.Val.| <0.05 set as the threshold value. The GSE39454 dataset was used as a validation cohort to verify key diagnostic genes. Subtype classifications of the IIM cohort are summarized in Table 2.
To determine the biological functions and pathways involved in common genes, we performed and visualized Wikipathway, KEGG pathway, GO terms, and hallmark gene sets for common genes using the R package “clusterProfiler.”
A PPI network of common genes was established in STRING (version 11.0), with confidence scores >0.15 set as the threshold, and visualized using Cytoscape (29, 30). In addition, the Molecular Complex Detection (MCODE) algorithm was used to recognize densely linked network components in Metascape (31).
To identify key biomarkers for the occurrence of COVID-19 related IIM, we used two machine learning methods for screening (LASSO and Random forest) (32–35). LASSO was performed by R package “glmnet,” and the minimum lamba value was set as the threshold. Random forest algorithm was executed with the R package “randomForest” with the relative importance score >0.3 set as a threshold. Finally, the intersection of the outputs of the two algorithms was considered as the key gene.
We further validated and evaluated the expression levels of these key genes in the GSE39454 cohort. Subsequently, ROC curves were used to evaluate the diagnostic performance of these key genes by the R package “pROC.”
Transcription factors and miRNAs play essential roles in the regulation of gene expression (36). Subsequently, we established TF-gene and miRNA-gene networks based on the NetworkAnalyst database (https://www.networkanalyst.ca/) (37).
The CIBERSORT algorithm was used to determine the infiltration abundance of 22 immune cells in each sample of the IIM cohort. Spearman’s correlation analysis was used to assess the relationship between key genes and immune cells.
The DSigDB tool was utilized to screen for candidate agents interacting with key genes (38). The top 11 agents were identified using p-values.
R 4.0.5 software and SPSS 21.0 software were used for statistical analysis. Wilcoxon was employed to determine the differences between groups, and p-value <0.05 was set as the threshold (*p <0.05, **p <0.01, ***p <0.001).
A flowchart of the study is presented in Figure 1. Using principal component analysis, we found that the disease and HC groups could be separated distinctly in the COVID-19 and IIM cohorts, respectively (Figures 2A, B). In the COVID-19 dataset, 3,803 DEGs were identified, of which 2,020 were upregulated and 1,783 were downregulated (Figure 2C). In the IIM dataset, 1,040 DEGs were identified, including 650 upregulated and 390 downregulated genes (Figure 2D).
Figure 2 Analysis of DEGs in patients with COVID-19 and IIM. (A) PCA plot for the COVID-19 dataset; (B) PCA plot for the IIM dataset; (C) volcano diagram of DEGs in the COVID-19 dataset; (D) volcano diagram of DEGs in the IIM dataset.
Subsequently, Wayne analysis was performed to intersect DEGs in the two datasets (Figure 3A). The results revealed 68 common upregulated expression genes and 23 common downregulated expression genes between the two diseases (Table S1). Heatmaps showing the expression levels of these genes in the COVID-19 and IIM cohorts, respectively (Figures 3B, C).
Figure 3 Identification of common molecules in COVID-19 and IIM patients. (A) Intersection of the COVID-19 and IIM DEGs; (B) heat map of common gene expression in the COVID-19 dataset; (C) heat map of common gene expression in the IIM dataset.
We performed an enrichment analysis of these common genes to reveal the comorbidity mechanism between COVID-19 and IIM. GO terms found that these common genes were mostly involved in response to external stimuli, immune response, defense response, and other functions; hallmark gene sets found that these common genes were predominantly involved in apoptosis, interferon gamma response, complement and so on; Wikipathways identified these common genes as involved in complement, and coagulation cascades, oxidative damage, host–pathogen interaction of human corona viruses MAPK signaling, etc. (Figure 4).
Figure 4 Functional enrichment analysis of common genes. (A) GO term enrichment; (B) hallmark gene set enrichment; (C) Wikipathway enrichment.
A PPI network of common genes was constructed using Cytoscape, which contained 89 nodes and 460 edges (Figure 5A). Moreover, the MCODE algorithm recognized two densely linked clusters (Figure 5B). Cluster 1 was mostly involved in ossification, collagen metabolic process and lnterleukin-4 and Interleukin-13 signaling; Cluster 2 was primary involvement in defense response to virus and type I interferon-mediated signaling pathway (Figures 5C, D).
Figure 5 Construction of PPI network to identify core clusters. (A) PPI network of the common genes; (B) MCODE algorithm recognizes core clusters; (C, D) functional enrichment analysis of core clusters.
Given that the interferon pathway is known to be significantly upregulated in dermatomyositis, to reduce the bias of our results, we divided the IIM patients into subgroups of patients with and without dermatomyositis for further analysis. A total of 68 common upregulated genes and 11 common downregulated genes were identified between dermatomyositis and COVID-19 (Table S2), in which two densely linked clusters were identified (Figures S1A, B). Cluster 1 was correlated with defense response to virus, type I interferon-mediated signaling pathway, negative regulation of viral genome replication and organelle inner membrane; Cluster 2 was related to protein processing in endoplasmic reticulum (Figure S1C). In addition, 64 common upregulated genes and 29 common downregulated genes were identified between other IIMs and COVID-19 (Table S3), in which two densely linked clusters were also identified (Figures S1D, E). Cluster 1 was mostly involved in proteoglycans in cancer, ossification, regulation of vascular associated smooth muscle cell proliferation, and regulation of lymphocyte activation; Cluster 2 was related to defense response to virus and type I interferon-mediated signaling pathway (Figure S1F). These findings reduce the bias of our analysis and further increase the robustness of the results.
To identify the key genes involved in the occurrence of COVID-19 related IIM, we screened for common genes using two machine learning methods. The LASSO algorithm confirmed four common genes and the random forest algorithm confirmed 19 common genes (Figures 6A–D). Finally, we intersected the two algorithms and obtained a total of four genes, which were considered key genes for COVID-19 related IIM (Figure 6E).
Figure 6 Machine learning was used to identify key genes. (A, B). LASSO algorithm; (C, D) Random forest algorithm; (E) Intersection between the two algorithms.
Subsequently, we verified the expression of key genes in the validation set. The expression levels of these key genes were elevated in IIM compared to HC, which was consistent with the results of the test set (Figures 7A–C). ROC curves revealed that the AUC values of these genes were higher than 0.8 in both the training and validation sets, indicating that these genes exhibited excellent diagnostic performance (Figures 7D, E).
Figure 7 Validation of key genes and evaluation of diagnostic performance evaluation. (A–C) Expression levels of key genes in the validation set; (D, E) ROC curves were used to evaluate the diagnostic performance of the key genes in the training and validation sets. **p < 0.01, and ***p < 0.001.
To reveal the regulatory mechanisms of key genes, we predicted the upstream and downstream TFs and miRNAs of key genes. It was revealed that 14 TFs can regulate CDKN1A, five TFs can regulate STAB1, four TFs can regulate IFI27 (Figure 8A). As for miRNAs, we found that 187 miRNAs could interact with CDKN1A, 31 miRNAs could interact with IFI27, and seven miRNAs could interact with STAB1 (Figure 8B). TFs can regulate the expression of key genes by binding to specific DNA sequences to control the transcription of target genes. MiRNAs are important post-transcriptional regulators that regulate key gene expression by binding to specific messenger RNA (mRNA) molecules, which can lead to mRNA degradation or inhibit protein translation. Our results provide new insights into the upstream and downstream regulatory mechanisms of key gene expression levels.
Figure 8 Building a regulatory network for key gene expression. (A) TF-genes networks; (B) miRNA-gene networks.
Considering that immune cell infiltration is an essential feature in the pathogenesis of IIM, we estimated the infiltration abundance of 22 immune cell types in the immune microenvironment of IIM using the CIBERSORT algorithm (Figure 9A). The results revealed that T cell gamma delta and M1 macrophage showed markedly increased infiltration abundance in IIM, whereas T cell CD8, Tregs, NK cell resting, M0 macrophage, and neutrophils exhibited opposite results (Figure 9B). Correlation analysis showed that the key genes were related to different infiltrating immune cells (Figure 9C). In particular, IFI27 was positively linked to M1 macrophage, which is considered an active mediator of virus infection-associated myositis (Figure 9D).
Figure 9 Immuno-infiltration analysis. (A, B) Abundance of 22 immune-infiltrating cells in IIM; (C) Correlation heat map of key genes and immune-infiltrating cells; (D) Correlation scatter plot of key genes with M1 macrophages. *p < 0.05, **p < 0.01, and ***p < 0.001.
To identify potential agents for the treatment of COVID-19 related IIM, we predicted the agents that interact with these key genes using the DSigDB database. We found that 11 agents interacted with CDKN1A, five agents interacted with IFI27, and 1 agent interacted with STAB1 (Table 3).
In the present study, we identified 86 common genes between COVID-19 and IIM using differential expression analysis, of which 56 were upregulated and 16 were downregulated. Functional enrichment analysis of common genes showed that these genes were primarily linked to response to stress, MAPK signaling pathways and immune dysregulation-related pathways such as complement and coagulation cascades, interferon response, and others. MAPK pathways have been recognized as an efficient transmitter of signals from the cell surface to the nucleus. MAPK can respond to different extracellular stimuli and thus generate contingency responses to trigger cascade signals to regulate cell proliferation, differentiation, and apoptosis (39, 40). Further analysis revealed that two closely linked clusters of common genes were related to inflammatory factors and interferon signaling. During SARS-CoV2 infection, the body produces large amounts of inflammatory mediators and chemokines that recruit immune cell infiltration to mediate inflammatory injury (41). An over-activated immune response of the organism induces a cytokine storm, which is positively related to the severity of COVID-19 (42). Immune dysregulation, especially interferon response, is considered as an essential sign of the progression and worsening of COVID-19 and IIM (43, 44). Interferons are potent cytokines and components of the first line of defense against viral infections (45). According to their distinct molecular characteristics and intracellular recognition receptors, interferons are classified as types I, II, and III, which induce hundreds of interferon-stimulated effector genes (ISGs) with dual roles in antiviral and immunomodulatory functions (46). Both the early stages of interferons deficiency and the late stages of interferons persistence can be indicators of severe COVID-19 (47, 48). Similarly, apart from genetic susceptibility and environmental factors, adaptive and innate immune mechanisms have recently been shown to be involved in the pathogenesis of IIM via coordinated interactions (49). Interferon signaling is highly represented in the muscle and skin of IIM patients, together with complement cascade activation, exerting various inflammatory effects that result in muscle fibrosis (50). In particular, interferon I signaling is increased in anti-MDA5+ dermatomyositis, which greatly correlates with disease activity and can be used to predict patient mortality (51, 52). Therefore, we hypothesized that after COVID-19 infection, myocytes respond to external stimuli to activate complement and interferon signaling via MAPK signaling, which releases large amounts of inflammatory factors. Ultimately, persistent inflammatory muscle damage can result in the development of IIM.
The extrapulmonary manifestation of COVID-19 is thought to occur through ACE-2 receptor-mediated viral attack (53). Similar to most other SARS-CoV-2 susceptible regions, the muscle tissue also highly expresses ACE-2 receptors (54). Viral stinger proteins can attach to the ACE-2 receptor, allowing the SARS-CoV-2 viral envelope to bind to the host cell membrane and transfer hereditary materials into the cell to strike it (55). This indicates that SARS-CoV-2 may be directly responsible for infecting muscle cells to activate the immune response (56). Altogether, the integration of our results may provide a new perspective for understanding the common pathogenesis of COVID-19 and IIM.
To shed further light on the key targets for the emergence of COVID-19-associated IIM, we used machine learning to screen four key genes for predicting the occurrence of COVID-19 related IIM. Interferon alpha-inducible protein 27 (IFI27) acts upstream of the negative regulation of RNA polymerase II transcription and regulates the export of proteins from the nucleus. It is involved in several cellular processes that mediate cytokine signaling in innate and adaptive immunity (57). An observational multi-cohort study found that IFI27 is highly expressed in the lower respiratory airways of COVID-19 patients and is associated with the presence of a high viral load (58). The upregulation of IFI27 expression in blood could be a predictor of respiratory failure in COVID-19 patients. The aberrant expression of IFI27 is also involved in the development of several autoimmune diseases (59). CDKN1A encodes a potent cyclin-dependent kinase inhibitor that functions in DNA damage repair and execution of apoptosis after caspase activation. CDKN1A is markedly upregulated in COVID-19 dependent muscle loss (60). Wang et al. (61) revealed that CDKN1A can be used for the early diagnosis of dermatomyositis as well as promoting the development of dermatomyositis by regulating immune cell infiltration. STAB1 is a type l transmembrane receptor, also known as a multifunctional scavenger receptor, that is highly expressed on macrophage endothelial cells (62). STAB1 mediates endocytose ligands and is critical for cell adhesion in chronic inflammation and tumor metastasis (63). Sotzny et al. (64) found that STAB1 could stratify patients with post-COVID syndrome and predict the development of myalgic encephalomyelitis/chronic fatigue syndrome. ROC curves showed that these genes exhibited high diagnostic value for the development of IIM and may be expected to be potential targets for intervention in COVID-19 related IIM.
Transcription factors and miRNAs are pivotal factors that regulate gene expression in organisms and are involved in transcriptional regulation and post-transcriptional regulation of genes, respectively (36). We constructed an interaction network to elucidate the regulatory network of TFs and miRNAs involving key genes, which revealed upstream and downstream expression regulation of key genes. Moreover, we characterized the immune microenvironment of IIM. Consistent with previous findings, there was a substantial infiltration of pro-inflammatory immune cells in the IIM group (follicular helper T cells, gamma delta T cells, and M1 macrophages) and a clear lack of suppressive inflammatory cells (Tregs) compared to the HC group (65–67). Correlation analysis revealed that key genes were linked to multiple immune-infiltrating cells, indicating that key genes may be involved in the progression of IIM by regulating immune cells. Notably, the expression level of IFI27 was positively correlated with M1 macrophage. Macrophages can be classified into classically activated M1 macrophages and selectively activated M2 macrophages (68). M1 macrophages are generally polarized by interferon-gamma, secrete large amounts of pro-inflammatory factors, and play an essential role in the early stages of inflammation. M2 macrophage express suppressive inflammatory factors that inhibit the inflammatory response and tissue repair (34, 35). Watson et al. (69) showed that M1 macrophage were essential mediators of virus-induced myopathy and that blocking SHP-1 activation in macrophages could prevent virus-induced myofiber degeneration. Finally, several potential agents which interact with these key genes were screened. These agents mainly exert their therapeutic effects by inhibiting the release of inflammatory mediators and modulating immune cell activity. Thus, we suggest that these agents target key genes in the treatment of COVID-19 related IIM. Collectively, our findings provide a comprehensive analysis to understand the pathogenesis of these key genes in COVID-19 related IIM.
This study had some limitations. First, this study was based on the bioinformatics analysis of public databases, and extensive experiments are necessary to validate the value of these molecules, pathways, and drugs. Second, this study was a retrospective analysis based on a public database, with a small amount of data and the absence of clinical information. Many patients, especially those infected with SARS-CoV-2 and IIM, will need to be acquired in the future to further validate the clinical applicability of our findings.
In conclusion, our study revealed for the first time the critical role of MAPK signaling and inflammatory responses in the link between COVID-19 and IIM comorbidity development. Moreover, four key genes were screened using machine learning for early diagnosis and treatment of COVID-19 related IIM. These findings provide thorough insight into the pathogenesis of comorbid IIM in patients with COVID-19. As the COVID-19 pandemic continues, a great deal of work is needed in the future to focus on the crosstalk between COVID-19 and IIM.
The original contributions presented in the study are included in the article/Supplementary Material. Further inquiries can be directed to the corresponding authors.
Conception: DL and JF. Interpretation or analysis of data: ZZ, WT, and DC. Preparation of the manuscript: ZZ, WT, and DC. Revision for important intellectual content: MQ. Supervision: DL and JF. All authors listed have made a substantial, direct, and intellectual contribution to the work and approved it for publication.
This work was supported by the National Natural Science Foundation of China (31971272) and Natural Science Foundation of Shannxi Province (2022SF-054).
The authors declare that the research was conducted in the absence of any commercial or financial relationships that could be construed as a potential conflict of interest.
All claims expressed in this article are solely those of the authors and do not necessarily represent those of their affiliated organizations, or those of the publisher, the editors and the reviewers. Any product that may be evaluated in this article, or claim that may be made by its manufacturer, is not guaranteed or endorsed by the publisher.
The Supplementary Material for this article can be found online at: https://www.frontiersin.org/articles/10.3389/fimmu.2023.1197493/full#supplementary-material
COVID-19, Corona Virus Disease 2019; IIM, idiopathic inflammatory myopathies; GEO, gene expression omnibus; HLA, human leukocyte antigens; GO, Gene Ontology; AUC, Area Under Curve; ROC, receiver operating characteristic.
1. Wang CC, Prather KA, Sznitman J, Jimenez JL, Lakdawala SS, Tufekci Z, et al. Airborne transmission of respiratory viruses. Science (2021) 373(6558). doi: 10.1126/science.abd9149
2. Pelosi P, Tonelli R, Torregiani C, Baratella E, Confalonieri M, Battaglini D, et al. Different methods to improve the monitoring of noninvasive respiratory support of patients with severe pneumonia/ARDS due to COVID-19: an update. J Clin Med (2022) 11(6). doi: 10.3390/jcm11061704
3. Kim S, Lee JH. Current advances in paper-based biosensor technologies for rapid COVID-19 diagnosis. Biochip J (2022) 16(4):376–96. doi: 10.1007/s13206-022-00078-9
4. Nie X, Qian L, Sun R, Huang B, Dong X, Xiao Q, et al. Multi-organ proteomic landscape of COVID-19 autopsies. Cell (2021) 184(3):775–791.e714. doi: 10.1016/j.cell.2021.01.004
5. Vahey GM, Marshall KE, McDonald E, Martin SW, Tate JE, Midgley CM, et al. Symptom profiles and progression in hospitalized and nonhospitalized patients with coronavirus disease, Colorado, USA 2020. Emerg Infect Dis (2021) 27(2):385–95. doi: 10.3201/eid2702.203729
6. Liu Y, Sawalha AH, Lu Q. COVID-19 and autoimmune diseases. Curr Opin Rheumatol (2021) 33(2):155–62. doi: 10.1097/bor.0000000000000776
7. Zebardast A, Hasanzadeh A, Ebrahimian Shiadeh SA, Tourani M, Yahyapour Y. COVID-19: A trigger of autoimmune diseases. Cell Biol Int (2023). doi: 10.1002/cbin.11997
8. Lee PI, Hsueh PR. Multisystem inflammatory syndrome in children: A dysregulated autoimmune disorder following COVID-19. J Microbiol Immunol Infect (2023) 56(2):236–45. doi: 10.1016/j.jmii.2023.01.001
9. Votto M, Castagnoli R, Marseglia GL, Licari A, Brambilla I. COVID-19 and autoimmune diseases: is there a connection? Curr Opin Allergy Clin Immunol (2023) 23(2):185–92. doi: 10.1097/aci.0000000000000888
10. Zhang Z, Zhu Z, Liu D, Mi Z, Tao H, Fan H. Blood transcriptome and machine learning identified the crosstalk between COVID-19 and fibromyalgia: a preliminary study. Clin Exp Rheumatol (2023). doi: 10.55563/clinexprheumatol/tz9i6y
11. Barsotti S, Dastmalchi M, Notarnicola A, Leclaire V, Dani L, Gheorghe K, et al. Performance of the new EULAR/ACR classification criteria for idiopathic inflammatory myopathies (IIM) in a large monocentric IIM cohort. Semin Arthritis Rheum (2020) 50(3):492–7. doi: 10.1016/j.semarthrit.2019.12.001
12. Carstens PO, Schmidt J. Diagnosis, pathogenesis and treatment of myositis: recent advances. Clin Exp Immunol (2014) 175(3):349–58. doi: 10.1111/cei.12194
13. O'Hanlon TP, Miller FW. Genetic risk and protective factors for the idiopathic inflammatory myopathies. Curr Rheumatol Rep (2009) 11(4):287–94. doi: 10.1007/s11926-009-0040-2
14. Haq SA, Tournadre A. Idiopathic inflammatory myopathies: from immunopathogenesis to new therapeutic targets. Int J Rheum Dis (2015) 18(8):818–25. doi: 10.1111/1756-185x.12736
15. Lundberg IE, Fujimoto M, Vencovsky J, Aggarwal R, Holmqvist M, Christopher-Stine L, et al. Idiopathic inflammatory myopathies. Nat Rev Dis Primers (2021) 7(1):86. doi: 10.1038/s41572-021-00321-x
16. Ding Y, Ge Y. Inflammatory myopathy following coronavirus disease 2019 vaccination: A systematic review. Front Public Health (2022) 10:1007637. doi: 10.3389/fpubh.2022.1007637
17. Hannah JR, Ali SS, Nagra D, Adas MA, Buazon AD, Galloway JB, et al. Skeletal muscles and Covid-19: a systematic review of rhabdomyolysis and myositis in SARS-CoV-2 infection. Clin Exp Rheumatol (2022) 40(2):329–38. doi: 10.55563/clinexprheumatol/mkfmxt
18. Shimizu H, Matsumoto H, Sasajima T, Suzuki T, Okubo Y, Fujita Y, et al. New-onset dermatomyositis following COVID-19: A case report. Front Immunol (2022) 13:1002329. doi: 10.3389/fimmu.2022.1002329
19. Gracia-Ramos AE, Martin-Nares E, Hernández-Molina G. New onset of autoimmune diseases following COVID-19 diagnosis. Cells (2021) 10(12). doi: 10.3390/cells10123592
20. Kharouf F, Kenig A, Bohbot E, Rubin L, Peleg H, Shamriz O. Increased rates of idiopathic inflammatory myopathies during the COVID-19 pandemic: a single-centre experience. Clin Exp Rheumatol (2023) 41(2):316–21. doi: 10.55563/clinexprheumatol/970881
21. Mehta P, MaChado PM, Gupta L. Understanding and managing anti-MDA 5 dermatomyositis, including potential COVID-19 mimicry. Rheumatol Int (2021) 41(6):1021–36. doi: 10.1007/s00296-021-04819-1
22. Gonzalez D, Gupta L, Murthy V, Gonzalez EB, Williamson KA, Makol A, et al. Anti-MDA5 dermatomyositis after COVID-19 vaccination: a case-based review. Rheumatol Int (2022) 42(9):1629–41. doi: 10.1007/s00296-022-05149-6
23. Tonutti A, Motta F, Ceribelli A, Isailovic N, Selmi C, De Santis M. Anti-MDA5 antibody linking COVID-19, type I interferon, and autoimmunity: A case report and systematic literature review. Front Immunol (2022) 13:937667. doi: 10.3389/fimmu.2022.937667
24. Yeoh SA, GianFrancesco M, Lawson-Tovey S, Hyrich KL, Strangfeld A, Gossec L, et al. Factors associated with severe COVID-19 in people with idiopathic inflammatory myopathy: results from the COVID-19 Global Rheumatology Alliance physician-reported registry. RMD Open (2022) 8(2). doi: 10.1136/rmdopen-2022-002508
25. Hoff LS, Ravichandran N, Shinjo SK, Day J, Sen P, Junior JG, et al. COVID-19 severity and vaccine breakthrough infections in idiopathic inflammatory myopathies, other systemic autoimmune and inflammatory diseases, and healthy controls: a multicenter cross-sectional study from the COVID-19 Vaccination in Autoimmune Diseases (COVAD) survey. Rheumatol Int (2023) 43(1):47–58. doi: 10.1007/s00296-022-05229-7
26. Healey Q, Sheikh A, Daines L, Vasileiou E. Symptoms and signs of long COVID: A rapid review and meta-analysis. J Glob Health (2022) 12:5014. doi: 10.7189/jogh.12.05014
27. Karaarslan F, Güneri FD, Kardeş S. Long COVID: rheumatologic/musculoskeletal symptoms in hospitalized COVID-19 survivors at 3 and 6 months. Clin Rheumatol (2022) 41(1):289–96. doi: 10.1007/s10067-021-05942-x
28. Lavi Y, Vojdani A, Halpert G, Sharif K, Ostrinski Y, Zyskind I, et al. Dysregulated levels of circulating autoantibodies against neuronal and nervous system autoantigens in COVID-19 patients. Diagnostics (Basel) (2023) 13(4). doi: 10.3390/diagnostics13040687
29. Shannon P, Markiel A, Ozier O, Baliga NS, Wang JT, Ramage D, et al. Cytoscape: a software environment for integrated models of biomolecular interaction networks. Genome Res (2003) 13(11):2498–504. doi: 10.1101/gr.1239303
30. Szklarczyk D, Gable AL, Lyon D, Junge A, Wyder S, Huerta-Cepas J, et al. STRING v11: protein-protein association networks with increased coverage, supporting functional discovery in genome-wide experimental datasets. Nucleic Acids Res (2019) 47(D1):D607–d613. doi: 10.1093/nar/gky1131
31. Zhou Y, Zhou B, Pache L, Chang M, Khodabakhshi AH, Tanaseichuk O, et al. Metascape provides a biologist-oriented resource for the analysis of systems-level datasets. Nat Commun (2019) 10(1):1523. doi: 10.1038/s41467-019-09234-6
32. Díaz-Uriarte R, Alvarez de Andrés S. Gene selection and classification of microarray data using random forest. BMC Bioinf (2006) 7:3. doi: 10.1186/1471-2105-7-3
33. Wang H, Lengerich BJ, Aragam B, Xing EP. Precision Lasso: accounting for correlations and linear dependencies in high-dimensional genomic data. Bioinformatics (2019) 35(7):1181–7. doi: 10.1093/bioinformatics/bty750
34. Zhang Z, Shi Y, Zhu Z, Fu J, Liu D, Liu X, et al. Characterization of myeloid signature genes for predicting prognosis and immune landscape in Ewing sarcoma. Cancer Sci (2022). doi: 10.1111/cas.15688
35. Zhang Z, Zhu Z, Wang X, Liu D, Liu X, Mi Z, et al. Comprehensive landscape of immune-based classifier related to early diagnosis and macrophage M1 in spinal cord injury. Aging (Albany NY) (2023) 15(4):1158–76. doi: 10.18632/aging.204548
36. Arora S, Rana R, Chhabra A, Jaiswal A, Rani V. miRNA-transcription factor interactions: a combinatorial regulation of gene expression. Mol Genet Genomics (2013) 288(3-4):77–87. doi: 10.1007/s00438-013-0734-z
37. Zhou G, Soufan O, Ewald J, Hancock REW, Basu N, Xia J. NetworkAnalyst 3.0: a visual analytics platform for comprehensive gene expression profiling and meta-analysis. Nucleic Acids Res (2019) 47(W1):W234–w241. doi: 10.1093/nar/gkz240
38. Yoo M, Shin J, Kim J, Ryall KA, Lee K, Lee S, et al. DSigDB: drug signatures database for gene set analysis. Bioinformatics (2015) 31(18):3069–71. doi: 10.1093/bioinformatics/btv313
39. Braicu C, Buse M, Busuioc C, Drula R, Gulei D, Raduly L, et al. A comprehensive review on MAPK: A promising therapeutic target in cancer. Cancers (Basel) (2019) 11(10). doi: 10.3390/cancers11101618
40. Liang YJ, Yang WX. Kinesins in MAPK cascade: How kinesin motors are involved in the MAPK pathway? Gene (2019) 684:1–9. doi: 10.1016/j.gene.2018.10.042
41. Coperchini F, Chiovato L, Croce L, Magri F, Rotondi M. The cytokine storm in COVID-19: An overview of the involvement of the chemokine/chemokine-receptor system. Cytokine Growth Factor Rev (2020) 53:25–32. doi: 10.1016/j.cytogfr.2020.05.003
42. Ye Q, Wang B, Mao J. The pathogenesis and treatment of the `Cytokine Storm' in COVID-19. J Infect (2020) 80(6):607–13. doi: 10.1016/j.jinf.2020.03.037
43. Gallay L, Mouchiroud G, Chazaud B. Interferon-signature in idiopathic inflammatory myopathies. Curr Opin Rheumatol (2019) 31(6):634–42. doi: 10.1097/bor.0000000000000653
44. Dean MJ, Ochoa JB, Sanchez-Pino MD, Zabaleta J, Garai J, Del Valle L, et al. Severe COVID-19 is characterized by an impaired type I interferon response and elevated levels of arginase producing granulocytic myeloid derived suppressor cells. Front Immunol (2021) 12:695972. doi: 10.3389/fimmu.2021.695972
45. Rouchka EC, Chariker JH, Alejandro B, Adcock RS, Singhal R, Ramirez J, et al. Induction of interferon response by high viral loads at early stage infection may protect against severe outcomes in COVID-19 patients. Sci Rep (2021) 11(1):15715. doi: 10.1038/s41598-021-95197-y
46. Walter MR. The role of structure in the biology of interferon signaling. Front Immunol (2020) 11:606489. doi: 10.3389/fimmu.2020.606489
47. Hadjadj J, Yatim N, Barnabei L, Corneau A, Boussier J, Smith N, et al. Impaired type I interferon activity and inflammatory responses in severe COVID-19 patients. Science (2020) 369(6504):718–24. doi: 10.1126/science.abc6027
48. Cantwell AM, Singh H, Platt M, Yu Y, Lin YH, Ikeno Y, et al. Kinetic multi-omic analysis of responses to SARS-coV-2 infection in a model of severe COVID-19. J Virol (2021) 95(20):e0101021. doi: 10.1128/jvi.01010-21
49. Miller FW, Lamb JA, Schmidt J, Nagaraju K. Risk factors and disease mechanisms in myositis. Nat Rev Rheumatol (2018) 14(5):255–68. doi: 10.1038/nrrheum.2018.48
50. Bolko L, Jiang W, Tawara N, Landon-Cardinal O, Anquetil C, Benveniste O, et al. The role of interferons type I, II and III in myositis: A review. Brain Pathol (2021) 31(3):e12955. doi: 10.1111/bpa.12955
51. Gasparotto M, Franco C, Zanatta E, Ghirardello A, Zen M, Iaccarino L, et al. The interferon in idiopathic inflammatory myopathies: Different signatures and new therapeutic perspectives. A literature review. Autoimmun Rev (2023) 22(6):103334. doi: 10.1016/j.autrev.2023.103334
52. Qian J, Li R, Chen Z, Cao Z, Lu L, Fu Q. Type I interferon score is associated with the severity and poor prognosis in anti-MDA5 antibody-positive dermatomyositis patients. Front Immunol (2023) 14:1151695. doi: 10.3389/fimmu.2023.1151695
53. Dong M, Zhang J, Ma X, Tan J, Chen L, Liu S, et al. ACE2, TMPRSS2 distribution and extrapulmonary organ injury in patients with COVID-19. BioMed Pharmacother (2020) 131:110678. doi: 10.1016/j.biopha.2020.110678
54. Yamamoto K, Takeshita H, Rakugi H. ACE2, angiotensin 1-7 and skeletal muscle: review in the era of COVID-19. Clin Sci (Lond) (2020) 134(22):3047–62. doi: 10.1042/cs20200486
55. Yesudhas D, Srivastava A, Gromiha MM. COVID-19 outbreak: history, mechanism, transmission, structural studies and therapeutics. Infection (2021) 49(2):199–213. doi: 10.1007/s15010-020-01516-2
56. Suh J, Mukerji SS, Collens SI, Padera RF Jr., Pinkus GS, Amato AA, et al. Skeletal muscle and peripheral nerve histopathology in COVID-19. Neurology (2021) 97(8):e849–58. doi: 10.1212/wnl.0000000000012344
57. Huang S, Zhao J, Song J, Li Y, Zuo R, Sa Y, et al. Interferon alpha-inducible protein 27 (IFI27) is a prognostic marker for pancreatic cancer based on comprehensive bioinformatics analysis. Bioengineered (2021) 12(1):8515–28. doi: 10.1080/21655979.2021.1985858
58. Shojaei M, Shamshirian A, Monkman J, Grice L, Tran M, Tan CW, et al. IFI27 transcription is an early predictor for COVID-19 outcomes, a multi-cohort observational study. Front Immunol (2022) 13:1060438. doi: 10.3389/fimmu.2022.1060438
59. Kim H, Gunter-Rahman F, McGrath JA, Lee E, de Jesus AA, Targoff IN, et al. Expression of interferon-regulated genes in juvenile dermatomyositis versus Mendelian autoinflammatory interferonopathies. Arthritis Res Ther (2020) 22(1):69. doi: 10.1186/s13075-020-02160-9
60. Cantu N, Vyavahare S, Kumar S, Chen J, Kolhe R, Isales CM, et al. Synergistic effects of multiple factors involved in COVID-19-dependent muscle loss. Aging Dis (2022) 13(2):344–52. doi: 10.14336/ad.2021.0817
61. Wang L, Fang D, Liu Y. Autophagy-related genes are potential diagnostic biomarkers for dermatomyositis. Ann Transl Med (2022) 10(4):228. doi: 10.21037/atm-22-70
62. Kzhyshkowska J. Multifunctional receptor stabilin-1 in homeostasis and disease. ScientificWorldJournal (2010) 10:2039–53. doi: 10.1100/tsw.2010.189
63. Kzhyshkowska J, Gratchev A, Goerdt S. Stabilin-1, a homeostatic scavenger receptor with multiple functions. J Cell Mol Med (2006) 10(3):635–49. doi: 10.1111/j.1582-4934.2006.tb00425.x
64. Sotzny F, Filgueiras IS, Kedor C, Freitag H, Wittke K, Bauer S, et al. Dysregulated autoantibodies targeting vaso- and immunoregulatory receptors in Post COVID Syndrome correlate with symptom severity. Front Immunol (2022) 13:981532. doi: 10.3389/fimmu.2022.981532
65. Hohlfeld R, Engel AG, Ii K, Harper MC. Polymyositis mediated by T lymphocytes that express the gamma/delta receptor. N Engl J Med (1991) 324(13):877–81. doi: 10.1056/nejm199103283241303
66. Espinosa-Ortega F, Gómez-Martin D, Santana-De Anda K, Romo-Tena J, Villaseñor-Ovies P, Alcocer-Varela J. Quantitative T cell subsets profile in peripheral blood from patients with idiopathic inflammatory myopathies: tilting the balance towards proinflammatory and pro-apoptotic subsets. Clin Exp Immunol (2015) 179(3):520–8. doi: 10.1111/cei.12475
67. Zhang L, Li W, Cai Y, Liu X, Peng Q, Liang L. Aberrant expansion of circulating CD4(+) CXCR5(+) CCR7(lo) PD1(hi) Tfh precursor cells in idiopathic inflammatory myopathy. Int J Rheum Dis (2020) 23(3):397–405. doi: 10.1111/1756-185x.13782
68. Mills CD. Anatomy of a discovery: m1 and m2 macrophages. Front Immunol (2015) 6:212. doi: 10.3389/fimmu.2015.00212
Keywords: COVID-19, idiopathic inflammatory myopathies, molecular mechanisms, immune dysregulation, biomarker
Citation: Zhang Z, Tao W, Cheng D, Qin M, Fu J and Liu D (2023) Deciphering the crosstalk of immune dysregulation between COVID-19 and idiopathic inflammatory myopathy. Front. Immunol. 14:1197493. doi: 10.3389/fimmu.2023.1197493
Received: 31 March 2023; Accepted: 24 July 2023;
Published: 10 August 2023.
Edited by:
Barbara Ruaro, University of Trieste, ItalyReviewed by:
Liliana Trotta, Ospedale di Cattinara, ItalyCopyright © 2023 Zhang, Tao, Cheng, Qin, Fu and Liu. This is an open-access article distributed under the terms of the Creative Commons Attribution License (CC BY). The use, distribution or reproduction in other forums is permitted, provided the original author(s) and the copyright owner(s) are credited and that the original publication in this journal is cited, in accordance with accepted academic practice. No use, distribution or reproduction is permitted which does not comply with these terms.
*Correspondence: Dong Liu, c2RvbmdqaWFAMTYzLmNvbQ==; Jun Fu, ZnVqdW5AZm1tdS5lZHUuY24=
†These authors have contributed equally to this work
Disclaimer: All claims expressed in this article are solely those of the authors and do not necessarily represent those of their affiliated organizations, or those of the publisher, the editors and the reviewers. Any product that may be evaluated in this article or claim that may be made by its manufacturer is not guaranteed or endorsed by the publisher.
Research integrity at Frontiers
Learn more about the work of our research integrity team to safeguard the quality of each article we publish.