- 1Institute of Dermatology, Chinese Academy of Medical Sciences and Peking Union Medical College, Nanjing, China
- 2Key Laboratory of Basic and Translational Research on Immune-Mediated Skin Diseases, Institute of Dermatology, Chinese Academy of Medical Sciences, Nanjing, China
- 3Department of Dermatology, The Second Xiangya Hospital of Central South University, Changsha, China
- 4State Key Laboratory of Natural Medicines, School of Basic Medicine and Clinical Pharmacy, China Pharmaceutical University, Nanjing, China
Three-dimensional (3D) genomics is an emerging field of research that investigates the relationship between gene regulatory function and the spatial structure of chromatin. Chromatin folding can be studied using chromosome conformation capture (3C) technology and 3C-based derivative sequencing technologies, including chromosome conformation capture-on-chip (4C), chromosome conformation capture carbon copy (5C), and high-throughput chromosome conformation capture (Hi-C), which allow scientists to capture 3D conformations from a single site to the entire genome. A comprehensive analysis of the relationships between various regulatory components and gene function also requires the integration of multi-omics data such as genomics, transcriptomics, and epigenomics. 3D genome folding is involved in immune cell differentiation, activation, and dysfunction and participates in a wide range of diseases, including autoimmune diseases. We describe hierarchical 3D chromatin organization in this review and conclude with characteristics of C-techniques and multi-omics applications of the 3D genome. In addition, we describe the relationship between 3D genome structure and the differentiation and maturation of immune cells and address how changes in chromosome folding contribute to autoimmune diseases.
Introduction
Among the most important mechanisms for regulating cellular life is DNA, the genetic material inside the cell. The interaction of DNA and histone octamers in the form of ribosomes results in the development of chromatin fibers. Scientists have thoroughly aligned, interpreted, and annotated the characteristics and functions of various sequences in the genome, including coding sequences, non-coding sequences, transcripts, and non-coding RNAs after the completion of the Human Genome Project (HGP) (1) and the encyclopedia of DNA elements (ENCODE) (2). Gene bodies, promoters, and some important cis-regulatory DNA elements including enhancers, silencers, and insulators are also found in linear chromatin (2). As we know, the chromatin fibers are compressed into the nucleus in an orderly three-dimensional (3D) structure at various levels. The eukaryotic genome has a hierarchical organization instead of being arranged linearly, which allows the transcriptional and regulatory machinery to reside in specific locations, facilitating their efficient function (3). In 2002, Dekker proposed the chromosome conformation capture (3C) technology (4) to study the geographic organization of the genome inside the cell nucleus. In the following years, 3C-based derivative sequencing technologies were rapidly developed, such as chromosome conformation capture-on-chip (4C) (5), chromosome conformation capture carbon copy (5C) (6), high-throughput chromosome conformation capture (Hi-C) (7), and chromatin interaction analysis by paired-end tag sequencing (ChIA-PET) (8). The effects of DNA folding resulting in physical interactions could have a significant impact on gene regulation, allowing regulatory elements to interact with gene promoters mediated by specific chromatin structure-associated proteins like CCCTC-binding factor (CTCF) and cohesin. Furthermore, recent discoveries suggest that 3D chromatin structure is involved in a variety of cell types and biological activities, including embryonic development, immunity, and disease susceptibility (9). For example, the 3D chromatin structure of the fertilized egg is gradually built up during the two-cell stage and, by the time of the inner cell mass, is basically similar to that of embryonic stem cells (10). Chen et al. (11) investigated 8,928 samples from 33 tumor types and discovered that enhancers were activated in the majority of cases, which were generated by particular topologically associating domains (TADs), where regulators that are linearly distant may fold into 3D structures through chromatin to reach spatial closeness, leading to cancer development.
The study of the 3D genome focuses on the fundamental principles of chromatin folding, assembly, and regulation in the nucleus as well as the mechanisms that govern genome regulation on a genome-wide scale. In early genomics research, genome-wide association studies (GWASs) have made significant advancements in identifying variations associated with complicated genetic disorders, including autoimmune diseases (12). Single-nucleotide polymorphism (SNP) and chromatin rearrangements involving deletions, duplications, insertions, inversions, and translocations could potentially interfere with gene expression and protein synthesis. Large-scale GWASs have shown that 80%–90% of variants are in non-coding regions (13). Various genetic variations are believed to affect disease risk by influencing gene expression, which can differ depending on the cell type and cellular state (14). In addition, approximately 60% of the causal variants associated with autoimmune diseases have been mapped to enhancer regions in immune cells (15). The process of DNA looping is responsible for transporting transcription factors (TFs) to gene promoters, which are often positioned on the same chromosome, but at different distances. An important advantage of 3D genomics is the ability to link diverse loci across the genome, which is vital for linking GWAS-identified expression quantitative trait loci (eQTLs) with potential target areas. Several studies have demonstrated that disease-associated SNPs may serve as marker to uncover regulating elements that play a significant role in disease susceptibility (16–20). For example, a risk variant for systemic lupus erythematosus (SLE), rs2280381, is found in an area of the distal enhancer that regulated IRF8 expression by spatially interacting with the IRF8 promoter and influencing methylation levels. DNA methylation, histone post-translational modification, and non-coding RNAs have been extensively explored at the epigenetic level in many autoimmune diseases (21, 22). Despite significant progress in understanding the pathogenesis of these diseases, there have been relatively few 3D genomic studies conducted in autoimmune diseases compared to psychiatric disorders and cancer.
This review summarized current concepts in 3D genome organization and multi-omics research techniques and applications of the 3D genome. We focus on how the 3D organization of genomes has an impact on the feature of immune cells of innate and adaptive immunity. Furthermore, we discuss how variants in non-coding regions, as well as epigenetic changes, might disrupt spatial genomic folding and generate an abnormal state of autoimmune diseases, as well as the functional consequences of autoimmune diseaseassociated variants and chromosomal rearrangements. Furthermore, following changes in gene expression and cellular function regulation throughout disease progression may give clues for developing new biomarkers and designing focused therapy regions.
Hierarchical view of 3D genome organization
Chromosomes are not randomly distributed within the nucleus but tend to occupy distinct, non-overlapping regions known as chromosome territory (CT), representing the highest level of chromatin organization (23). Chromosomes with high gene density are found more centrally in the nucleus, whereas chromosomes of low gene density are predominantly located toward the nuclear periphery (24, 25). The CT organization is critical for nuclear functions, including transcription, replication, RNA processing, and DNA damage repair (25). Mehta et al. showed that DNA damage caused a large-scale spatial repositioning of CT regions, including a partial transfer of CTs from the interior to the exterior of the nucleus. Interestingly, this is a reversible process in which chromosome territories reoccupy positions similar to those in intact control cells after repair, indicating the stability of CTs in the nucleus (26). Chromatin is separated into two multi-megabase (Mb) compartments at the sub-chromosomal level. The A compartments are distinguished by open chromatin areas that are rich in active histone marks and genes with high levels of transcription and are found internally in the nucleus. The B compartments are often located at the nuclear periphery and are associated with low levels of gene expression and histone modifications related to active chromatin, such as acetylation. As a result, the B compartments are transcriptionally repressed (27). Although chromatin compartments are generally permanent in the nucleus, there is significant interconversion between the A and B compartments throughout growth and disease development, suggesting that chromatin compartments are highly malleable and correspond with the expression of cell-specific genes. On a smaller scale, chromosomes are organized into TADs, the sub-megabase regions with a high frequency of self-interactions (28, 29). Normally, TADs are separated from surrounding domains by boundary regions (also known as borders) where local interactions are reduced. TAD borders are enriched in housekeeping genes, transfer RNA, the CTCF–cohesin complex, transcription start site (TSS), and active transcriptional indicators like H3K4me3, H3K36me3, and H3K9me2 (9, 30). Cell-specific TAD boundaries divide adjacent domains and restrict the interactions between promoters and enhancers. After the genetic deletion of a boundary, nearby TADs tend to fuse, resulting in abnormal connections between regulators and genes (31). In some cases, shifting genes to a new TAD will result in ectopic interactions with regulatory elements, leading to an alteration of their expression profile. Additionally, nearly all heterochromatin is found close to the nucleus and nucleolar peripheries, which are known as lamina-associated domains (LADs) and nucleolus-associated domains (NADs), respectively (32). LADs make up approximately 40% of the genome and mainly consist of gene-depleted regions of transcriptionally silenced chromatin enriched in histone H3K27me3 (33). NADs have higher H3K9me2 and lower H3K27me3 contents than regions that only interact with the nuclear lamina (34). Chromatin loops, which are frequently linked promoters and enhancers, are more elaborate structural units than TADs. Gene promoters and enhancers can be either looped together to upregulate gene expression or excluded from loops to initiate borders and suppress gene expression (35). The presence of CTCF and the cohesin subunits RAD21 and SMC3 in more than 86% of the loops suggests that CTCF and cohesin are involved in loop anchor formation (27). In the loop extrusion model, the structural maintenance of the chromosome, like the cohesin complex, pushes the linear DNA outward until the complex comes into contact with the convergent CTCF bound to loop anchor sequences (36, 37). Moreover, certain TFs like SATB1, P300, YY1, EKLF, OCT4, and SOX2 have been reported to play critical roles in chromatin looping control (38–43). The 3D genome structure controlling distal connections allows complex gene regulation networks to form, enabling multiple enhancers to interact with one promoter or a single enhancer to contact numerous promoters (44) (Figure 1).
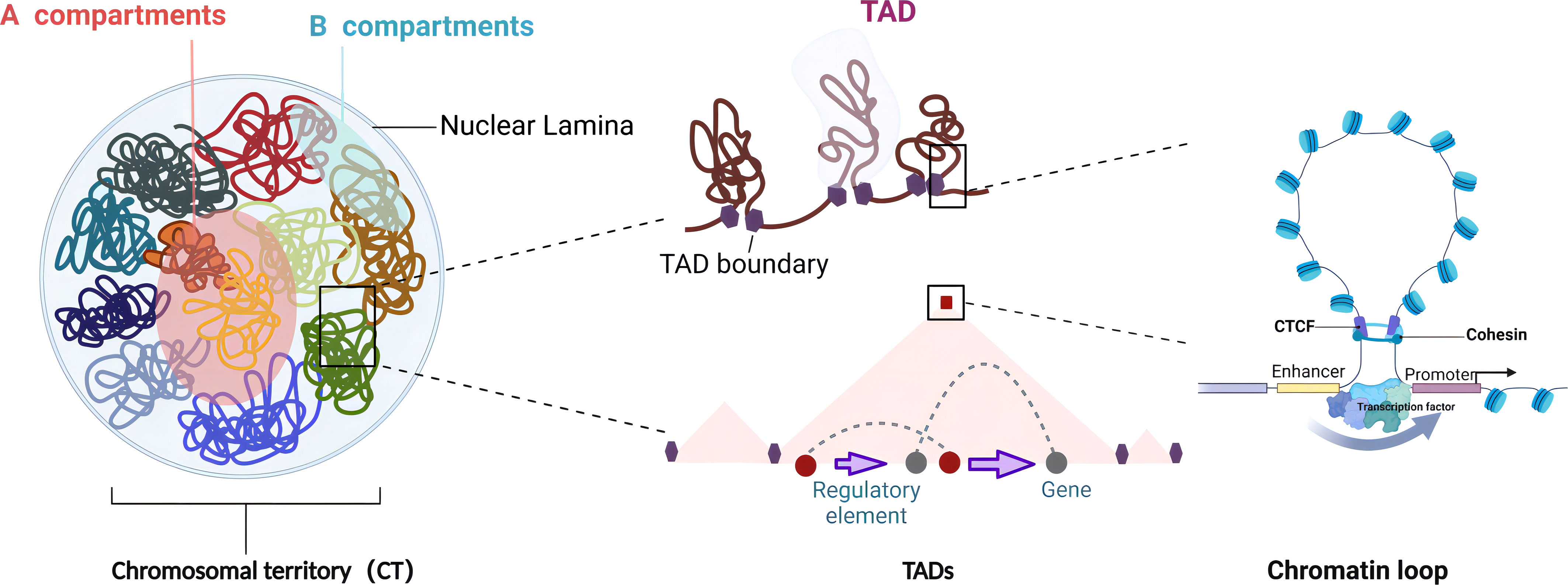
Figure 1 Features of 3D genome organization. The 3D genome hierarchical units of chromatin in the nucleus are in descending order: chromosomal territories (CTs) are the universal domain of the genome, with each chromosome occupying a separate and non-overlapping area in the nucleus. Chromatin compartments are composed of two compartments of A and B with different appearances in the spatial structure of the genome. The A compartments are open chromatin compartments with mostly euchromatin, showing high concentration and expression of genes. The B compartments are closed chromatin compartments of repressed transcription regions with mostly heterochromatin. Topologically associated domains (TADs) are stable spatial structural units in the nucleus, which act as functional units of genome folding and guide the regulation of long-range regulation. Chromatin loops are usually formed by distant interactions between promoters and distal enhancers or regulatory elements, which are the most elaborate structural and functional units directly regulating gene expression (created with BioRender.com).
Multi-omics research techniques and applications in 3D genome
Imaging-based approaches were used in the majority of early 3D genomics studies (45), including microscopy and fluorescence in situ hybridization (FISH), which makes it possible to visualize the spatial length between two or more loci by hybridizing fluorescently labeled probes to DNA after fixation and moderate denaturation (46, 47). These tools may directly quantify the spatial separations between genomic loci in individual cells, but the throughput, resolution, and genome coverage are limited. Recently, super-resolution and cryogenic electron microscopy allowed us to observe 11-Å structures for 30-nm chromatin fibers (48). Other approaches based on C-technologies have been implemented to specifically measure the physical connection between DNA segments (4). Chromatin is first cross-linked to maintain interactions between the proximal areas of DNA and binding proteins. Subsequently, the cross-linked chromatin is disassembled into DNA fragments by restriction enzymes. The remaining DNA regions that are in close spatial proximity can be enzymatically ligated together to form a chimera DNA strand, a procedure known as proximity ligation. Following de-crosslinking, chimeric DNA is suitable for downstream analysis of 3D chromatin with PCR, DNA microarrays, or DNA sequencing (13). The initial version of the C-technology, known as 3C, could verify interactions between only two pre-determined genomic loci and identify known DNA interactions using fluorescence quantitative PCR (49). 4C technology can determine the interactions between specific genomic baits and several fragments in the form of a ring with only a pair of primers (50). 5C uses a highly multiplexed ligation-mediated amplification that allows the amplification of 3C ligation compounds. Either microarray or high-throughput DNA sequencing is used to examine the resulting 5C library of ligated primers (51). As the many-to-many technique, 5C has been applied to 1% of the human genome to explore long-range interactions between DNA sequences and distal regulators (52). High-resolution chromatin interaction profiles can be produced by Hi-C, which combines biotin enrichment with high-throughput sequencing to examine genome-wide intra- or interchromatin interactions at specific geographical regions (7). The resolution of Hi-C is constrained by sequencing depth. For example, 8.4 million, 2 billion, and 6.5 billion total reads would be needed to attain 1-Mb, 10-kb, and 1-kb resolution, respectively (27, 49). A Hi-C-based method, Micro-C, enables mononucleosome resolution chromosome folding maps using micrococcal nuclease (MNase) rather than restriction enzymes (53). Further, Micro-C XL increases the signal-to-noise ratio by isolating insoluble chromatin and using long cross-linkers (54). Like several high-throughput sequencing methods, different derivative technologies of Hi-C such as DNase Hi-C, in situ Hi-C, and BL-Hi-C have been improved in order to increase the resolution or effectiveness of proximity ligation and also to reduce the bias caused by the use of restriction enzymes (27, 55, 56).
Furthermore, technologies based on combining 3C and Hi-C with oligonucleotide capture, single-cell isolation, and high-throughput sequencing have been developed, which have greatly facilitated the study of the chromatin conformation in the regulation of spatial and temporal specific expression of genes. In the Capture-C or Capture Hi-C (CHi-C) technique, a 3C or Hi-C library is subjected to capture by hybridization with pools of DNA or RNA oligos to concentrate ligation products that fit specific sites (57, 58). With less library complexity, these techniques enable a significant rise in the number of distinguishable interactions within specific areas without ultra-deep sequencing. Chromatin immunoprecipitation-loop (ChIP-loop), a method for studying protein-mediated chromatin interactions, combines ChIP and 3C techniques (59). ChIA-PET is a scale-up of the ChIP-loop technique that identifies genome-wide chromatin connections without preference and is sequenced after immunoprecipitation of DNA–protein complexes with antibodies specific to the target protein (60). In situ Hi-C followed by chromatin immunoprecipitation (HiChIP) is a novel technique that constructs a sequencing library by Tn5 transposase with reduced starting material (Table 1).
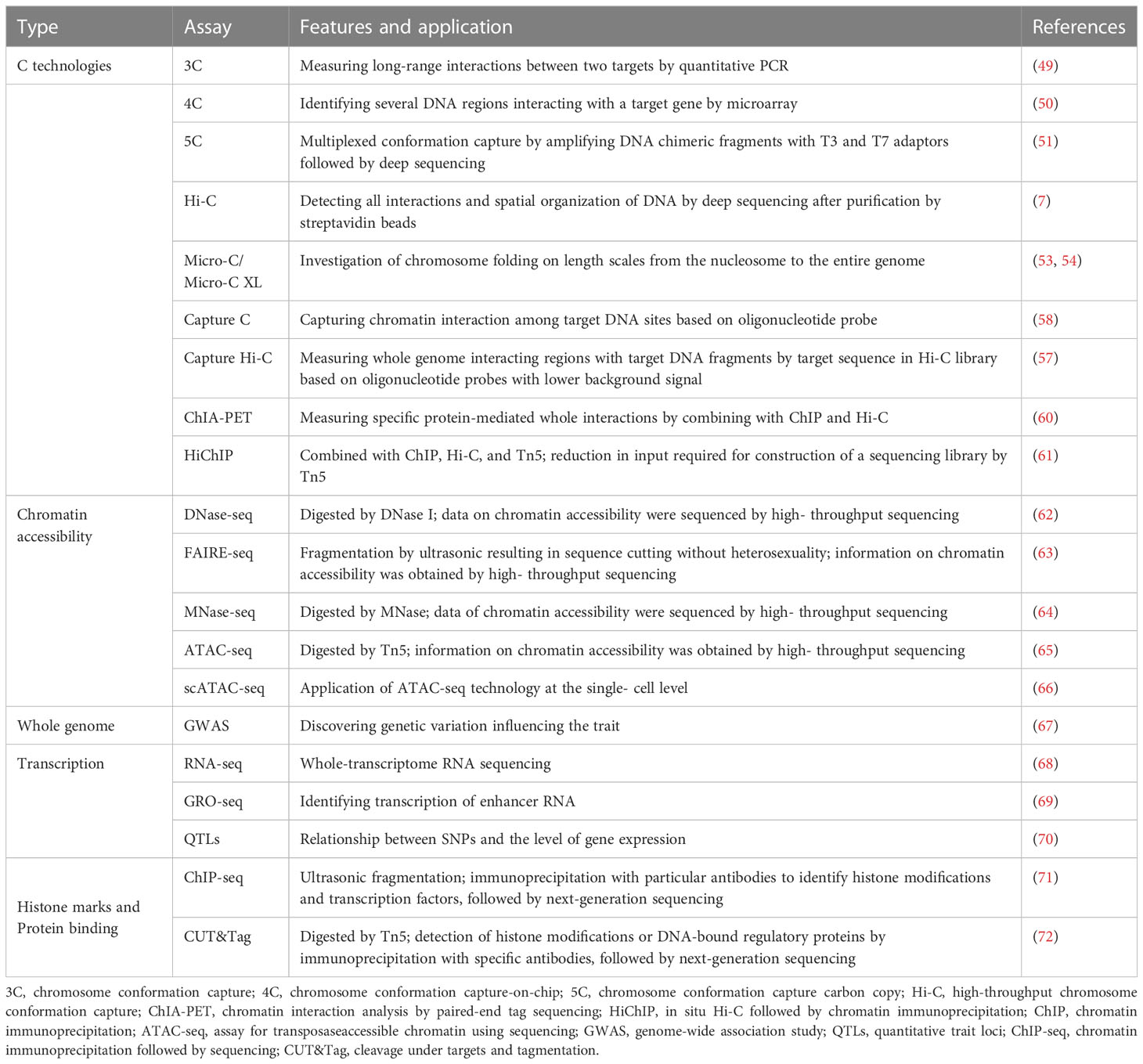
Table 1 The characteristics of multi-omics research techniques and applications in 3D genome chromatin structures.
The degree to which cis-regulatory elements and trans-acting factors can physically interact with chromatinized DNA is referred to as chromatin accessibility (73). Furthermore, post-translational chemical modifications of chromatin are dynamically changing across developmental stages and cell types and are associated with chromatin accessibility, indicating the function of specific genomic regions (74). Deoxyribonuclease I (DNase I) hypersensitive site sequencing (DNaseseq) is a method employing high-throughput sequencing to interrogate DNase hypersensitive sites to detect potentially active chromatin areas, while prior knowledge of histone modifications and TSS are not required (75). FAIRE (formaldehyde-assisted isolation of regulatory elements) combined with high-throughput sequencing (FAIRE-seq) is similar to DNase-seq, whereas DNA fragmentation is performed by sonication instead of ribonuclease and then purified by phenol/chloroform (63). Although the operations of FAIRE-seq are reasonably simple and convenient, they are not favorable to detect sensitive open chromatin areas because of unmanageable ultrasonic mechanical damage and unpredictable accuracy of cleaving. MNase-seq cleaves and eliminates accessible DNA not protected by proteins by using the endo-/exo-nuclease. MNase, a small-molecular-weight enzyme, is widely used to isolate fragments spanning single nucleosomes that are inaccessible to DNase I or Tn5 with a high efficiency of cleaving internucleosomal DNA (64). MNase hypersensitivity sequencing (MH-seq) provides a further improvement in the resolution of open chromatin mapping. Compared to DNase I and Tn5, some specific genomic region- enriched MH-seq reads show unique epigenetic marks H3K27me3 and DNA methylation (76). In addition, an assay for transposaseaccessible chromatin using sequencing (ATAC-seq) introduces sequencing adaptors into open chromatin areas using a hyperactive Tn5 transposase (65). Compared with DNaseseq, FAIRE-Seq, and MNase-Seq, cell requirements for ATAC-seq libraries are reduced from 106 ~107 to 5,000~50,000 (77). Advancements in single-cell sequencing technology have led to the development of various methods for assessing chromatin accessibility in individual cells, which are based on library preparation procedures used in ATAC-seq and DNase-seq. Zhang et al. studied 30 types of adult human tissue using scATAC-seq and identified 111 different cell types based on similarities in chromatin (78). Combining their datasets with single-cell chromatin accessibility data from 15 types of fetal tissue (79), 1.2 million cis-regulatory elements covering 14.8% of the genome for 222 different cell types have been determined, providing a comprehensive overview of gene regulatory programs in human cells in different tissues, life stages, and organ systems. Moreover, a variety of high-resolution chromatin accessibility assays based on 3C or Hi-C, such as HiCAR (Hi-C on accessible regulatory DNA) (80), DNase Hi-C (81), HiCoP (82), and Ocean-C (83), have been developed to profile accessible chromatin regions and to reveal the interaction between cis-regulatory elements.
To accurately assess the interrelationships between different regulatory components and gene function during cell development and differentiation, the integration of multi-omics data to define the biological effects of associated genes, transcriptional regulatory elements, and other biomolecules involved in the transcriptional regulation of specific genes is required. Numerous loci have been identified as statistically associated with various diseases and traits based on GWASs. However, because more than 80% of disease-associated variants are found in non-coding areas, it is difficult to ascertain the biological mechanism behind a genetic risk factor (14). Generally, QTLs indicate genes that are involved in quantitative traits (97). Several QTL analyses have been conducted to assess the polygenic nature of phenotypes and intermediate phenotypes, like gene expression. Recent advances in next-generation sequencing techniques have enabled the analysis of histone modifications and chromatin structures. For instance, RNA-seq reveals the level of gene expression. Chromatin immunoprecipitation followed by sequencing (ChIP-seq) and cleavage under targets and tagmentation (CUT&Tag) identifies distinct nucleosome properties or TSS. GWAS variants in non-coding or intergenic genomic regions can be linked more precisely to the genes they affect (98) (Figure 2).
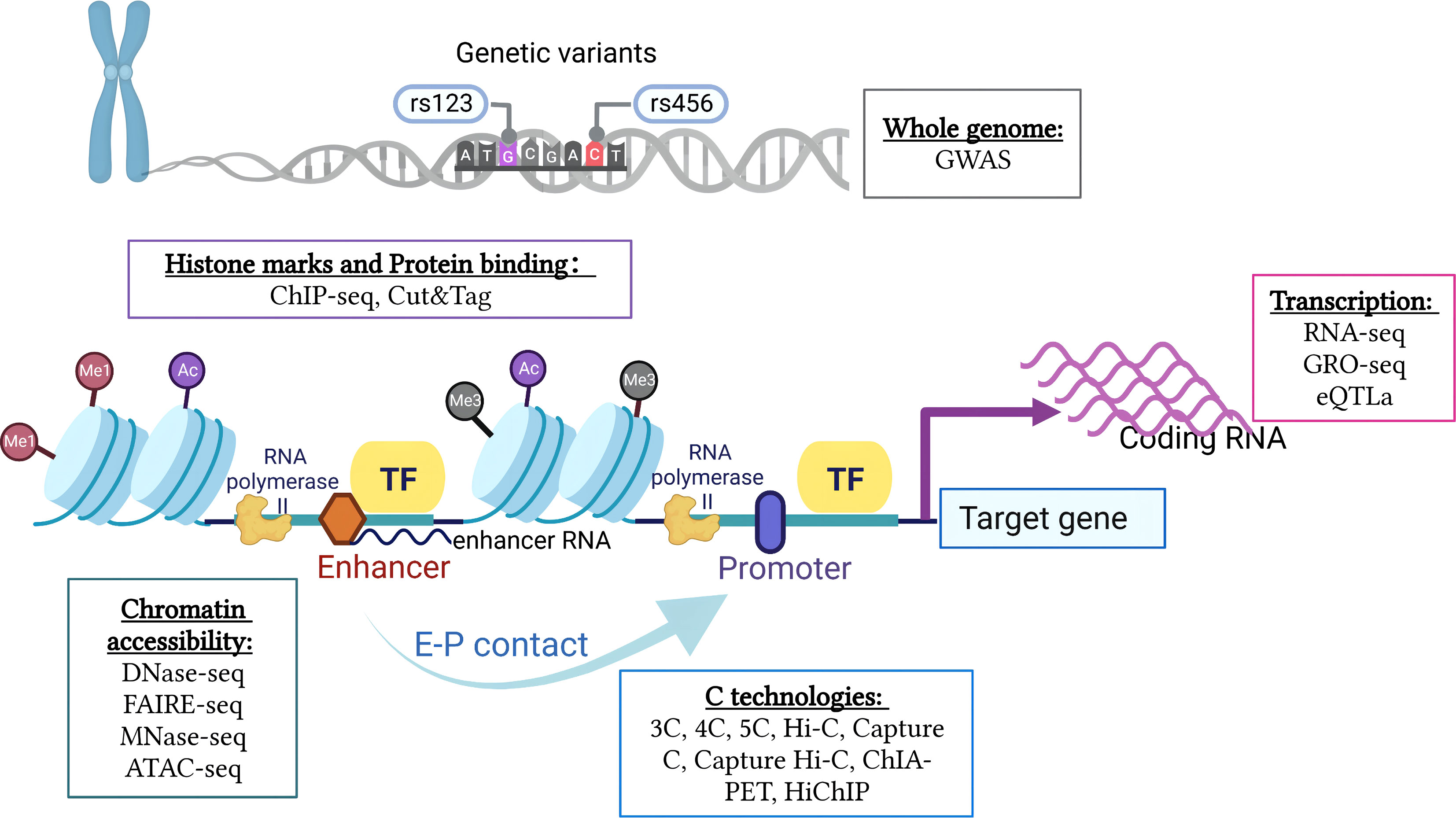
Figure 2 Integration of multi-omics research techniques of 3D chromatin and genome organization. A comprehensive analysis of the interrelationships between different regulatory components and gene function requires integration of multi-omics data, including genetic variation of the whole genome, chromatin accessibility, histone modifications and protein binding, gene transcription levels, and chromatin conformation (created with BioRender.com).
Insights into lymphocyte development and immune response from dynamic 3D genome
Different types of cells make up the immune system with specialized functions, concluding rapidly responding innate immune cells and highly specialized lymphocytes (99). It is noteworthy that impaired transcriptional control in lymphocytes could have a detrimental effect on immune cell development, which has been observed in a variety of diseases such as cancers, immunodeficiencies, and autoimmune disorders (100). In addition, 3D genome folding is involved in the regulation of gene expression, immune cell differentiation, activation, and dysfunction (101).
B-cell receptors (BCRs), consisting of pairs of immunoglobulin heavy (IgH) and immunoglobulin light (IgL) chains, and T-cell receptors (TCRs), composed of either an α and β chain or a γ and δ chain, confer the ability to detect foreign antigens (102). The antigen receptor segments composed of variable (V), diversity (D), and joining (J) gene segments are recombined during B- and T- cell development, in order to create the antigen-specific variable domains of the Ig/TCR chains (103). Chromosome openness reorganizes locally at recombination signal sequences (RSSs) and globally across antigen receptor loci with V (D)J recombination in a time-dependent manner. V (D)J recombination is initiated by the protein complex derived from the recombination activating gene 1 (RAG1) and RAG2 in recombination centers, where gene segments become accessible to the complex. DNA is recombined RSSs adjacent to segments of V, D, and J genes by RAG recombinase (104). Given that diverse V segments are scattered across 0.6–3 Mb of linear genomic space at IgH and TCR sites, rearrangements of these segments can be difficult. 3C-based methods have shown that the looping of DNA sequences underlies cell compaction and enhances interactions between V and (D)J segments (105). CTCF binding sites in the V region are forward- oriented, while reverse-oriented CTCF binding sites flank the recombination center (37). Through the cohesin-mediated loop extrusion constrained by convergent CTCF sites, diffusion-based synapsis of accessible V RSSs by D/J RSSs bound to RAG would be enhanced in the compacted structure (37, 106).
Significant alterations of genomic topology accompany early B- cell development (107). It has been shown that a transcriptionally inactive gene that encodes early B-cell TF (Ebf1) is sequestered in the nuclear lamina of progenitor cells switching between an inactive B compartment and an active A compartment. By developing new intradomain and interdomain interactions at the pro-B- cell stage, B-lineage transcriptional signatures are developed (108). In addition to regulating gene expression and chromatin status, TFs appear to reconfigure the 3D genome in compartments of early B cells. TF binding sites’ co-localization forms two interaction hubs within nuclear space. First, a large portion of the cell-type-invariant structural loops in the genome is produced by short-range contacts between CTCF and the cohesin complex. Second, long-range interactions between TFs such as E2A and PU.1 with enhancer-binding histone acetyltransferases P300 acted within and between chromatin domains (108). Paired box 5 (PAX5), an example of a TF that specifies a particular lineage, is crucial for establishing the B- cell lineage by triggering B cell-specific genes and suppressing other immune cell genes (109). PAX5 binding sites were supposed to regulate global 3D genomic organization because only 47% of these sites were located in genes or promoters (107). A total of 7,810 differential chromatin interactions were identified between the wild-type (WT) and Pax5−/− pro-B cells, while 83% of differential interactions are weakened or abolished in the absence of Pax5 (107). In addition, PAX5 enhances cohesin’s residence time on chromatin and facilitates extended loop extrusion by suppressing the expression of WAPL, specifically in pro-B and pre-B cells (110).
The thymus serves as the primary site for T- cell development, where the double-negative stage of growth takes place, during which neither CD4 nor CD8 is expressed. T cells follow a stepwise differentiation pathway monitored by Notch signals and combinations of TFs (such as BCL11B and RUNX), resulting in naïve CD4+ and CD8+ T cells (111). BCL11B is crucial for silencing alternative lineage programs in double-negative thymocytes, as revealed by BCL11B binding to regions with repressive H3K27me3 enrichment. Substantial intra-TAD interaction was also mediated by BCL11B as cells differentiate toward the double-positive stage (112). Similar to Ebf1 in B- cell development, the Bcl11b locus is repositioned from the B compartment to the A compartment during T-cell differentiation, which is mediated through transcription of a non-coding RNA known as thymocyte differentiation factor (ThymoD). ThymoD transcription stimulates CpG residue demethylation and enhances the recruitment of cohesin, bringing the Bcl11b promoter and enhancer together into a loop domain (113). Recently, another critical TF, T- cell factor 1 (TCF1), has been implicated to weaken or disrupt the TAD border insulation through recruiting NIPBL and cohesin complex at active enhancers. This mechanism improves the interplay of target genes and regulatory elements on insulated domains in T- cell progenitors (114). Taken together, the aforementioned investigations demonstrate the critical role of chromatin-associated protein binding, transcription factor-mediated transcriptional regulation, and 3D chromatin rearrangement in lymphocyte development.
Inflammatory response genes are rapidly upregulated when receptors recognize specific molecules associated with pathogens, which are associated with a network of enhancers established by lineage-determining transcription factors (115). While there is little change in TADs and compartments in response to innate immune triggers, enhancer–promoter contacts in the 3D genome are essential for the rapid activation of target genes upon stimulation (101, 116, 117). Unlike innate immune cells, naïve T cells and B cells need more time to establish an inflammatory gene expression program after accepting antigen recognition and co-stimulatory signals (101). In naïve T cells, TCF1 and CTCF modulate the 3D genomic architecture to control a similar set of genes (118). Dynamic 3D chromatin interactions are thought to be involved in the activation of T and B cells and the initiation of adaptive immune response in several studies. TAD architecture of human T cells remains generally constant for initial periods, but 72 h after activation, TAD partition displayed smaller chromatin domains than those in resting cells (119). CD4+ T cells are at the center of the adaptive immune system, which is phenotypically specialized in various ways by cytokine stimulation, for example, Th1, Th2, and Th17 cells. T-bet TF regulates the differentiation of naïve CD4+ T helper lymphocytes by increasing transcription of Th1-specific genes (such as IFN-γ) and suppressing transcription of Th2-specific genes (such as IL-4) (120). 3C experiments demonstrate that T-bet is necessary for the direct development of chromatin loops or tethers at the IFN-γ gene locus (121). Th1 and Th2 cell subset-specific interactions of Ifng and Il4 genes appear to be regulated by stimulation-induced signal transducer and activator of transcription (STAT) TFs across the genome. STAT4-deficient cells are unable to produce Th1-selective Ifng contact due to the prevented 3D genome reorganization (122). The basic leucine zipper TF activating transcription factor-like (BATF), a necessary TF for the generation of Th17 and follicular helper T cell (TFH) cells, recruits CTCF to lineage-specifying gene loci to form a chromatin looping in the transcriptional programming of various effector T cells (123). Naïve B cells are activated by encountering cognate antigens and migrate to the germinal center, where they undergo T cell-mediated co-stimulation and eventually specialize into antibody-secreting plasma cells. An estimated 30% of the compartment status of the genome has been changed in B- cell specification, most of which are in regions enriched for genes and TFs relevant to germinal center development (124). It appears that BCL6, which represses cell cycle checkpoint genes and is specifically upregulated in germinal center B cells, is coordinated with other genes in a way that facilitates proliferation by merging the 3D gene neighborhoods on chromosomes (125).
In summary, the activation of lymphocytes is followed by comprehensive, multistep 3D genome organization dynamics that enable cell proliferation and the formation of an inflammatory transcription program.
3D genome disorganization in autoimmune diseases
Immune cell dysfunction is responsible for a number of human morbidities, especially autoimmune diseases. When self or harmless antigens cause aberrant activation of lymphocytes, autoimmunity may be the result (126). Disruption of gene expression through altered 3D architecture is frequently associated with immune disorders and could be identified in two scenarios (101) (Figure 3). First, the alteration of non-coding sequences disrupts the binding of TFs or loop extrusion proteins, resulting in changes in 3D genome conformation and disrupting genomic conformation and transcriptional control. As GWAS variants are enriched in largely uncharacterized enhancer regions, it is essential to analyze their functional significance in the context of the 3D genome in order to understand how they influence disease pathology by reducing or enhancing interactions with the enhancer–promoter regulatory network (13). For instance, SNPs in non-coding regions interacting with tumor necrosis factor alpha-induced protein 3 (TNFAIP3) hindered TF binding and disrupted 3D chromatin folding in human CD4+ T cells (127). Second, variants in the genes that encode proteins (such as TFs) can also alter their function and alter the order of the 3D genome. For example, SATB1, a chromatin assembly protein and tissue-specific TF, is associated with T- cell development (128). Conditional depletion of SATB1 results in an autoimmune-like phenotype in mice because the obstructed promoter–enhancer loops in Satb1 cKO T cells result in downregulated expression of genes encoding for master regulators (such as Bcl6, Ets2, and Cd8b) and T- cell receptor locus (129, 130).
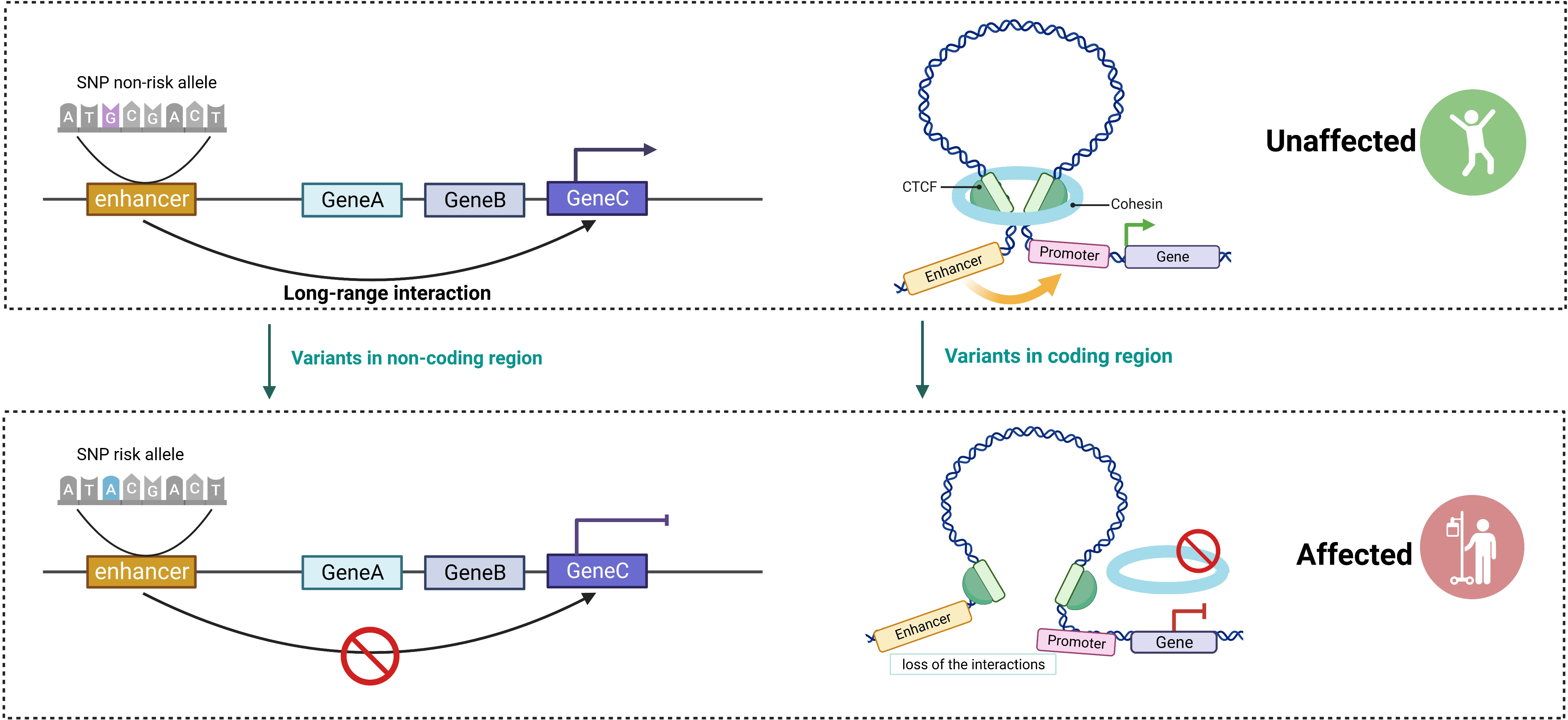
Figure 3 The critical role of 3D genome in the regulation of gene expression. Disruptions in gene expression caused by altered 3D architecture are frequently associated with immune disorders and can be classified into two scenarios. Altering non-coding sequences disrupts the binding of transcription factors (TFs) and impairs long-range interactions, resulting in reduced delivery of TFs to the promoter and hindering gene expression. Variants in the genes that encode proteins (such as TFs and cohesin complex) lead to changes in genome conformation (created with BioRender.com).
CD4+ T cells are at the center of the adaptive immune system, and activation of T cells is a key etiological pathway involved in many autoimmune disorders (131). A total of 245 candidate disease genes for autoimmune diseases were identified based on promoter CHi-C data in activated CD4+ T cells and genetic associations for five autoimmune diseases. An example was IL2RA, in which allele-specific expression analyses were consistent with its interaction-mediated regulation (132). Functional genomic analysis not only helps translate GWAS results into biologically meaningful disease mechanisms but also can be used to validate or develop new therapeutic targets based on disease risk loci. Martin et al. exploited CHi-C to identify prospective therapeutic targets in rheumatoid arthritis (RA), juvenile idiopathic arthritis, and psoriatic arthritis in T-helper and B-cell lines. They have discovered that 48 out of 454 potential illness genes were pharmacological targets; however, only 11 were addressed by rheumatic disease medications, providing an approach for repurposing current treatments (133). Similarly, H3K27ac HiChIP was used to identify genes associated with chromatin interactions with disease-associated SNPs of some inflammatory skin diseases. Therefore, by identifying genes enriched for disease-relevant pathways, drugs could potentially be repurposed and developed to target these pathways (134).
In this part, we summarize recent research on how the 3D genome affects gene regulatory processes in autoimmune diseases.
Systemic lupus erythematosus
Autoantibodies, abnormal T and B lymphocyte function, and immune complex development are features of SLE, an autoimmune disease that causes damage to multiple organs. Analysis of coordinated gene expression patterns in SLE patients and healthy controls revealed deregulation in the IFN pathway that was dependent on disease activity, indicating that genome organization and structure play a significant role in gene expression and disease activity (135). In our previous study, we analyzed the 3D chromosome structure of CD4+ T cells from SLE patients, finding that it differed considerably from that of healthy controls and was closely related to the severity of the illness (136). By using Hi-C, RNA-seq, ATAC-seq motif enrichment analysis, and histone modifications, we discovered SLE-associated loops with differentially expressed genes (DEGs) in CD4+ T cells correlated with disease activity. Over 90% of SLE-associated loops are based on enhancer–promoter contacts. Moreover, it was found that the promoter area of the DDX60L gene overlapped with two SLE-associated loops. DDX60L is an ATP-dependent helicase gene that participated in the innate immune IFN antiviral response. SLE patients with higher SLE disease activity index scores have a stronger frequency of interactions on DDX60L. We also found that the active histone marker and TF SPI1 were enriched at the two loop anchors. When ChIP-qPCR and 3C-qPCR were performed in SPI1 knockdown CD4+ T cells, silencing of SPI1 reduced the binding of SPI1 to the DDX60L promoter and decreased the association between the distal looping region and the DDX60L promoter. In addition, some SLE-associated SNPs, such as rs13385731, rs2732549, and rs2245214, were found to overlap with activating enhancers based on the enrichment of ChIP-seq data from ENCODE. For example, the enhancer region containing rs13385731 forms a long interaction loop, which may affect LTBP1 local chromatin conformation and gene expression (136). GWASs and fine-mapping identified a number of genetic loci linked to increased susceptibility to SLE in the chromosome 6q23 region, including rs148314165 and rs200820567, which were found in a potential enhancer region revealed by ENCODE that is approximately 42 kb downstream of the gene TNFAIP3 (84). The gene TNFAIP3 is a likely target of the discovered enhancer, as it is a major negative regulator of pro-inflammatory nuclear factor kappa B (NF-κB) signaling, which is associated with many autoimmune diseases (137). Moreover, the risk allele (A/A) in the enhancer dramatically disrupts NF-κB binding and inhibits long-range DNA looping of the enhancer to the TNFAIP3 promoter, effectively decreasing TNFAIP3 expression and increasing IL20RA and IFNGR1 expression through TALEN (transcription activation-like effector nuclease)-mediated genome editing, HiChIP, 3C-qPCR, and dual-luciferase reporter assays (85, 138). TNFAIP3 loss-of-function mutations result in elevated inflammatory responses, autoantibody production, and inflammatory arthritis in humanized mouse models (86, 139). Genome-wide investigation into the function of autoimmune risk polymorphisms is made possible by the combination of transcriptomic and epigenomic data with GWASs (67). A recent study found that the allele rs13239597-A is strongly associated with SLE and able to regulate IRF5 expression. This was performed using CRISPR/Cas9 and luciferase assays as well as Hi-C and CHi-C data across multiple blood cell lines (90). A cell type-specific epigenetic landscape of SLE SNPs in adult immune cells was examined using ENCODE and Roadmap Epigenomics data from CD4+ T cells and CD19+ B cells, together with ChIP-seq data from neutrophils (140). Super-enhancers (SEs) are a wide region of clustered enhancers with a high and widespread enrichment of TFs, H3K4me1, and H3K27ac, resulting in a high capacity to increase the gene expression program (141). Abnormal SE activity mediated by STAT3 has been discovered in memory B- cell subsets and is crucial for B- cell maturation and differentiation (87).
TFH cells are a type of cell that is necessary for the production of anti-nuclear antibodies, which are characteristic of SLE. Su et al. produced detailed and precise maps of the open chromatin–promoter connections in human TFH cells by applying promoter-focused Capture C and ATAC-seq analysis, providing a way to link non-coding open chromatin regions and SNPs to specific regions. The majority of SLE-associated variants do not interact with the closest gene but rather with distant promoters, many of which have been implicated in TFH and SLE, including BCL6 and CXCR5. Additionally, genes whose role in TFH/SLE disease biology was previously unknown were discovered through the combination of GWASs and 3D genomics. For example, the kinases HIPK1 and MINK1 were essential for the production of IL-21, which was required for T cell-mediated help for B- cell antibody production in SLE patients (142).
In studies of monocytes, the genomic region containing rs2431697 was identified to be a cell-type-specific enhancer through the combination of genetic, epigenomic, and high-throughput sequencing technologies, such as meta-analysis GWASs, ChIP-seq for H3K4me1 and H3K27ac from Roadmap, and ATAC-seq data. Moreover, CRISPR gene editing techniques were used to edit these disease susceptibility loci in cell lines, primary immune cells, and humanized mice. The DNA sequence containing the non-risk allele (rs2431697 C) binds NF-κB with a greater affinity and is more accessible that the risk allele (T), leading to an increase in miR-146a production in monocytes through a special chromosomal 3D structure proved by 4C-seq. It has been determined how miR-146a controls the inflammatory response in SLE (143). Different alleles have influenced the binding ability of TFs and chromatin accessibility, which could regulate the expression of susceptibility genes of lupus (19). GWASs have demonstrated at least 16 genetic variants significantly associated with autoimmune disorders in the IRF8 locus, but it is difficult to identify the functional loci due to linkage disequilibrium (LD) (15). To address this issue, a high-throughput screen for the disease-related susceptibility loci through the CRISPR array has identified a genomic region located approximately 64 kb downstream of IRF8 (rs2280381) as an enhancer that specifically regulates IRF8 expression in monocytes. The spatial structure of the enhancer region and IRF8 promoter was examined using 4C. It is interesting to note that the enhancer RNA AC092723.1 is necessary for the development of this spatial structure because it recruits TET1 to the IRF8 promoter by changing methylation levels and chromatin state (20). Recently, an analysis of innate immunity was conducted among human primary monocytes from SLE patients based on a high-resolution 3D landscape by integrated datasets from Hi-C, RNA-seq, ATAC-seq, and ChIP-seq. High-resolution chromatin interaction maps of human primary monocyte samples show differences from those of the THP1 cell line. In addition, the 3D chromatin maps revealed a great deal of diversity in the HLA region among individuals (144).
Rheumatoid arthritis
RA is an inflammatory autoimmune disease that damages bone and cartilage through the release of cytokines by activated T cells, B cells, and monocytes in the synovial membranes (145). Most of the research on disease-associated alleles at region 6q23 has been conducted in relation to the gene TNFAIP3. McGovern et al. identified causal genes and refined the possible functional SNPs at the locus 6q23 by searching for distal chromatin interactions with CHi-C, validating with 3C, and further proving with cell type- and genotype-specific eQTLs and ChIP. The result showed that the variant-associated DNA fragment interacts via a chromatin loop not only with TNFAIP3 but also with the more distant IL20RA instead of OLIG3 in B and T cells. Furthermore, there are more interactions between enhancer and IL20RA in patients with the risk G allele of the SNP rs6927172 compared to those with the non-risk allele (91). The JAK–STAT pathway is activated by IL20RA, which also includes IL-19, IL-20, and IL-24. Constitutive JAK–STAT activation stimulates the formation of cytokines and causes the mobilization of immune cells in an inflammatory environment, resulting in damage to the underlying cartilage due to chronic joint inflammation and proliferative synovitis (146). ATAC-seq, RNA-seq, Hi-C, and Chi-C analyses were performed in human CD4+ T cells stimulated with anti-CD3/anti-CD28 for 24 h. This showed that approximately 30% of the long-range chromatin junctions undergo significant changes, while compartments or TADs remain unchanged after 24 h of TCR activation. By collecting nuclear RNA-seq data, genes such as MYC and FOXO1 not previously implicated by GWASs in the RA pathophysiology have been found and also confirmed using CRISPR/Cas9 (147).
Although fibroblast-like synoviocytes (FLS) are crucial for the development of RA, there are few functional genetics databases for these cells. FLS samples from patients with RA were studied to characterize 3D chromatin interactions, DNA accessibility, and gene expression (148). Epigenetic and transcriptomic datasets of primary FLS samples were generated using ChIP-seq, ATAC-seq, RNA-seq, cap analysis gene expression sequencing (CAGE-seq), and chromatin conformation analysis (HiC and Chi-C). Up to 24% of RA heritability can be attributed to FLS, suggesting FLS’s role in influencing RA genetic risk independently. Moreover, the topological organization, chromatin state, and FLS samples from patients with RA were studied to characterize 3D chromatin interactions; the DNA LS genome was found to be significantly altered following TNF stimulation. RBPJ is a candidate causal and functional FLS gene based on epigenetic and functional analyses. Three SNPs (rs7441808, rs35944082, and rs874040) within the RBPJ region were selected to confirm enhancer activity by luciferase reporter assay and CRISPRi, which revealed that rs874040-containing enhancers regulate RBPJ expression (149).
Multiple sclerosis
Multiple sclerosis (MS), which is characterized by persistent demyelination, oligodendrocyte mortality, and loss of axons and neurons, is an excellent illustration of an autoimmune disorder driven by abnormal activation of tissue-derived immune cells (150). Some chromosomal regions (such as 6q23 and 16p13) have been found to be associated with MS predisposition through GWASs (92, 151). The region rs11154801 and a region with the other three independent MS associations (rs17066096, rs7769192, and rs67297943) appeared to form the majority of chromosomal interactions in 6q23. The SNP rs11154801 within the introns of AHI1 interacts with the promoter region and correlates with expression, supporting the gene candidacy of AHI. The CHi-C results indicated that the other interaction was linked to multiple immune-related genes, such as IL20RA, IL22RA2, IFNGR1, and TNFAIP3, suggesting that these variants could play a role in regulating numerous immune pathways (92). SNPs at or close to CLEC16A at chromosome 16p13.13 including rs12927355, rs4780346, rs12708716, and rs8062322 serve as expression quantitative trait loci for CIITA, SOCS1, and DEXI in peripheral T cells. In samples homozygous for the CLEC16A rs12927355 risk allele, SOCS1 and CLEC16A were expressed at a higher level on CD4+ T cells (152). The functions of CIITA and SOCS1 in immune cells have been established. CIITA encodes the MHC class II transactivator, which is an important genetic factor that determines susceptibility to autoimmune diseases (153). SOCS1 plays a role in inhibiting type I interferon signaling and regulating inflammation and immune cell homeostasis (154). Nevertheless, DEXI is a gene considered to respond to dexamethasone, a pro-inflammatory drug, and DEXI probably regulates inflammation (151).
Type 1 diabetes
Even though diabetes is regarded as a metabolic disorder, exogenous insulin dependency is typically caused by the autoimmune destruction of insulin-producing cells of the pancreas in type 1 diabetes (T1D). GWASs have found over 60 loci associated with T1D across the human genome (155). To identify genes regulated by T1D-associated genetic variants, Nyaga et al. performed a combined spatial and functional eQTL analysis using the CoDeS3D algorithm. A total of 246 spatially regulated genes, including HLA-DRB1, LAT, CTLA4, and NOTCH1, which exhibit tissue-specific effects in multiple tissues, were found based on the Genotype-Tissue Expression database. Moreover, T1D-associated variants are interconnected through networks that comprise immunological regulatory mechanisms, including immune-cell activation, cytokine, and programmed cell death protein-1 (PD-1) (156). Genetic variation in an intron of CLEC16A acts at a distance to regulate the promoter region of DEXI, and decreased DEXI expression is associated with an increased risk for T1D (157). The non-obese diabetic (NOD) mouse strain offers an excellent model for understanding the complex processes associated with autoimmune diseases. In T cells from T1D mice, H3K27ac and Smc1 HiChIP revealed hyperconnected clusters in T1D-associated regions, including genes associated with autoimmunity, including BCL11B and ETS1, but these clusters were not found in WT mice. However, when NOD mice were backcrossed with WT mice, decreased 3D chromatin interaction was identified in disease-related regions, suggesting that genomic variants deregulating enhancer function were responsible. The 3DFAACTS-SNP workflow identified 47 new candidate genes associated with variants in 12 T1D risk loci related to loss of immune tolerance in regulatory T cells. For example, SNP rs614120 located in the first intron of the BACH2 was predicted to inhibit the binding of FOXA2 (a member of the Forkhead Transcription factor family). It is noteworthy, however, that rs614120 may interfere with the binding of other members of the forkhead family, such as FOXP3, to similar DNA sequences due to the lack of expression of FOXA2 in T cells. The 3DFAACTS-SNP procedure also reveals that this rs614120-containing enhancer region interacts with the BACH2 promoter to create a distal promoter–enhancer interaction. This suggests that rs614120 can interfere with the binding of FOXP3 to the enhancer and lead to dysregulated BACH2 production (93).
Other diseases
CD4+ T cells are one of the principal drivers of pathogenesis in systemic sclerosis (SSc), a highly complex autoimmune disease regulated by the interaction of hereditary and epigenetic factors (158). DNA methylation surrounding HLA gene clusters and genes enriched in inflammatory pathways differed between SSc patients and healthy controls in CD4+ T cells. A study linking genetic risk to epigenome and transcriptome dysregulations has identified four significant risk genes for SSc, TNIP1 (rs3792783), GSDMB (rs9303277), IL12RB1 (rs2305743), and CSK (rs1378942), which potentially interact with DMP-DEG (differentially methylated CpG position–differentially expressed gene) pairs cg17239269–ANXA6, cg19458020–CCR7, cg10808810–JUND, and cg11062629–ULK3, respectively (159). Moreover, CTCF binding was enriched in DMPs in CD4+ cells from SSc patients, which may be a result of abnormal overexpression of the CTCF gene (159). CTCF recruitment, previously characterized as being DNA methylation-dependent, would be deregulated by aberrant DNA methylation and also participate in long-distance contacts that affect aberrant gene regulation (160). Takayasu arteritis is linked to genetic susceptibility loci primarily in non-coding regions, similar to other autoimmune diseases. To identify chromatin looping in the IL-6 locus, DNA-affinity pulldown assay was applied, followed by mass spectrometry and Western blotting, luciferase reporter assays, and 3C. A change in the intron domain of IL-6 (rs2069837) could disrupt a chromatin loop and recruit the repressive HDAC–MEF2 complex, which inhibits the anti-inflammatory monocyte gene GPNMB, mediating increased susceptibility to Takayasu arteritis and promoting persistent infections and cancer (94). Inflammatory bowel diseases (IBDs), including ulcerative colitis (UC) and Crohn’s disease (CD), are chronic inflammatory disorders that affect the colonic mucosa and gastrointestinal tract, respectively. Genetics participates in the pathogenesis of IBD, as demonstrated by twin, family, and population-based studies (161). Based on H3K27ac analysis, 92 of 163 IBD risk loci are localized to DNA regulatory elements (DREs), particularly enhancer elements in the intestinal epithelium and immune cells (162). Following 4C-seq in monocytes, lymphocytes, and intestinal epithelial cells, 902 new IBD candidate genes interacting with 92 DRE were discovered. In monocytes and lymphocytes, these genes were enriched for signaling in immune pathways such as JAK–STAT and interferon. I ntestinal epithelial cells, however, were enriched for signaling in IL-10, RHO, and RAC (163). In CD patients, SNP rs6651252 is associated with Wnt-responsive DNA enhancer elements, and its disease-associated allele boosts TF TCF7L2 binding. By employing CRISPR/Cas9 and epigenetic modulation, the rs6651252 enhancer was found to regulate the expression of the c-MYC proto-oncogene (95). By performing systematic annotation, prediction, and prioritization of the known pleiotropic variants associated with autoimmune diseases from 12 genome-wide studies, rs4728142 was identified as a top causal regulatory variant that was located upstream of the pro-inflammatory gene IRF5. ZBTB3, a putative structural regulator, mediates the formation of site-specific chromatin loops in the chromosomal rs4728142 locus. The presence of the rs4728142-A risk allele facilitated the tight binding of ZBTB3 and regulated the upregulation of IRF5-induced genes related to autoimmunity. This process leads to the polarization of M1 macrophages, contributing to the occurrence and development of autoimmune diseases by improving the inflammatory and immune response (96) (Table 2).
Conclusion and future perspective
As previously mentioned, the application of 3D genomics has significantly advanced our knowledge of the genetics of lymphocyte development and the etiology of autoimmune diseases. The 3D genome can help to determine the functional effect of disease genetics on the associated target genes, involving the gene expression and regulatory networks or pathways, which provides a great grasp of the mechanisms of spatial conformational changes in chromatin, transcriptional regulation, and the generation of biological features. Our previous research identified the affecting gene regulatory elements, such as active enhancers and promoters within the genome loops, revealing the new mechanisms of SLE from the 3D genome landscape (136).
GWASs are based on the assumption that common diseases are often influenced by a large number of genetic variants. After analyzing information from Hi-C sequencing, histone markers, and chromatin accessibility, some risk-associated SNPs were found to be located in the promoter or enhancer region. Risk variants can affect gene expression by interacting with distal genomic regions through 3D chromatin folding. These interactions can bring the regulatory elements closer to the target gene and increase or decrease its expression levels, depending on the nature of the interaction and the specific regulatory elements involved. However, it is especially challenging to demonstrate the functions of enhancers through experiments. Through targeting genomic deletions and mutations, CRISPR/Cas9 and CRISPR-based approaches, such as CRISPRi and CRISPRa, were used to identify the function of potential regulatory regions coupled with transcriptional quantification or RNA-seq. The integration of GWASs and omics data allows the elucidation of the function of individual risk variants and the identification of disease and tissue specificity. This helps to elucidate the detailed pathogenesis process of autoimmune diseases and thus leads to precise diagnosis and treatment.
Although 3C and its derivatives are unquestionably powerful tools for structural and functional genomics research, those technologies still face challenges on several fronts, such as difficulties in detecting weak interactions between chromatin, long experimental cycles that do not allow for a rapid and realistic response to interactions and a low ratio of signal to noise. Moreover, current visualization tools are incapable of integrating genomic information across the interaction intensity, spatial conformation, and gene synergy, as 3D genome data indicate the spatial composition and functional interconnections in multiple dimensions. The spatial conformation of the genome in the nucleus varies with time in order to sustain proper cell growth and development. As the 4D nucleosome project proceeds, it will be feasible to map the spatial and temporal organization and dynamics of the genomic structure of humans (164). There are still some challenges in the clinical application. For example, how to manipulate the structure of the chromatin or alter the genetic loci to investigate their impact on the disease process is still a problem. It is also necessary to create novel treatments for illnesses based on genomic information.
Author contributions
YQ, QL, and MZ made substantial contributions to the study’s conception and design. All authors drafted the article or revised it critically for important intellectual content. All authors gave their final approval of the version to be submitted and any revised versions.
Funding
This work was supported by the Non-profit Central Research Institute Fund of the Chinese Academy of Medical Sciences (2022-RC310-04), the National Natural Science Foundation of China (No. 82030097), and the National Key R&D Program of China (2022YFC3601803).
Conflict of interest
The authors declare that the research was conducted in the absence of any commercial or financial relationships that could be construed as a potential conflict of interest.
Publisher’s note
All claims expressed in this article are solely those of the authors and do not necessarily represent those of their affiliated organizations, or those of the publisher, the editors and the reviewers. Any product that may be evaluated in this article, or claim that may be made by its manufacturer, is not guaranteed or endorsed by the publisher.
References
1. International Human Genome Sequencing Consortium. Finishing the euchromatic sequence of the human genome. Nature (2004) 431(7011):931–45. doi: 10.1038/nature03001
2. ENCODE Project Consortium. An integrated encyclopedia of DNA elements in the human genome. Nature (2012) 489(7414):57–74. doi: 10.1038/nature11247
3. Taberlay PC, Achinger-Kawecka J, Lun ATL, Buske FA, Sabir K, Gould CM, et al. Three-dimensional disorganization of the cancer genome occurs coincident with long-range genetic and epigenetic alterations. Genome Res (2016) 26(6):719–31. doi: 10.1101/gr.201517.115
4. Dekker J, Rippe K, Dekker M, Kleckner N. Capturing chromosome conformation. Science (2002) 295(5558):1306–11. doi: 10.1126/science.1067799
5. Simonis M, Klous P, Splinter E, Moshkin Y, Willemsen R, de Wit E, et al. Nuclear organization of active and inactive chromatin domains uncovered by chromosome conformation capture-on-chip (4C). Nat Genet (2006) 38(11):1348–54. doi: 10.1038/ng1896
6. Dostie J, Richmond TA, Arnaout RA, Selzer RR, Lee WL, Honan TA, et al. Chromosome conformation capture carbon copy (5C): a massively parallel solution for mapping interactions between genomic elements. Genome Res (2006) 16(10):1299–309. doi: 10.1101/gr.5571506
7. Lieberman-Aiden E, van Berkum NL, Williams L, Imakaev M, Ragoczy T, Telling A, et al. Comprehensive mapping of long-range interactions reveals folding principles of the human genome. Science (2009) 326(5950):289–93. doi: 10.1126/science.1181369
8. Fullwood MJ, Liu MH, Pan YF, Liu J, Xu H, Mohamed YB, et al. An oestrogen-receptor-alpha-bound human chromatin interactome. Nature (2009) 462(7269):58–64. doi: 10.1038/nature08497
9. Anania C, Lupiáñez DG. Order and disorder: abnormal 3D chromatin organization in human disease. Brief Funct Genomics (2020) 19(2):128–38. doi: 10.1093/bfgp/elz028
10. Wang L, Zhang J, Duan J, Gao X, Zhu W, Lu X, et al. Programming and inheritance of parental DNA methylomes in mammals. Cell (2014) 157(4):979–91. doi: 10.1016/j.cell.2014.04.017
11. Chen H, Li C, Peng X, Zhou Z, Weinstein JN, Liang H. A pan-cancer analysis of enhancer expression in nearly 9000 patient samples. Cell (2018) 173(2):386–399.e12. doi: 10.1016/j.cell.2018.03.027
12. Gutierrez-Arcelus M, Rich SS, Raychaudhuri S. Autoimmune diseases - connecting risk alleles with molecular traits of the immune system. Nat Rev Genet (2016) 17(3):160–74. doi: 10.1038/nrg.2015.33
13. Fu Y, Tessneer KL, Li C, Gaffney PM. From association to mechanism in complex disease genetics: the role of the 3D genome. Arthritis Res Ther (2018) 20(1):216. doi: 10.1186/s13075-018-1721-x
14. Maurano MT, Humbert R, Rynes E, Thurman RE, Haugen E, Wang H, et al. Systematic localization of common disease-associated variation in regulatory DNA. Science (2012) 337(6099):1190–5. doi: 10.1126/science.1222794
15. Farh KK-H, Marson A, Zhu J, Kleinewietfeld M, Housley WJ, Beik S, et al. Genetic and epigenetic fine mapping of causal autoimmune disease variants. Nature (2015) 518(7539):337–43. doi: 10.1038/nature13835
16. Claussnitzer M, Dankel SN, Kim K-H, Quon G, Meuleman W, Haugen C, et al. FTO obesity variant circuitry and adipocyte browning in humans. N Engl J Med (2015) 373(10):895–907. doi: 10.1056/NEJMoa1502214
17. Gupta RM, Hadaya J, Trehan A, Zekavat SM, Roselli C, Klarin D, et al. A genetic variant associated with five vascular diseases is a distal regulator of endothelin-1 gene expression. Cell (2017) 170(3):522–533.e15. doi: 10.1016/j.cell.2017.06.049
18. Soldner F, Stelzer Y, Shivalila CS, Abraham BJ, Latourelle JC, Barrasa MI, et al. Parkinson-Associated risk variant in distal enhancer of α-synuclein modulates target gene expression. Nature (2016) 533(7601):95–9. doi: 10.1038/nature17939
19. Hou G, Harley ITW, Lu X, Zhou T, Xu N, Yao C, et al. SLE non-coding genetic risk variant determines the epigenetic dysfunction of an immune cell specific enhancer that controls disease-critical microRNA expression. Nat Commun (2021) 12(1):135. doi: 10.1038/s41467-020-20460-1
20. Zhou T, Zhu X, Ye Z, Wang Y-F, Yao C, Xu N, et al. Lupus enhancer risk variant causes dysregulation of IRF8 through cooperative lncRNA and DNA methylation machinery. Nat Commun (2022) 13(1):1855. doi: 10.1038/s41467-022-29514-y
21. Imgenberg-Kreuz J, Carlsson Almlöf J, Leonard D, Alexsson A, Nordmark G, Eloranta M-L, et al. DNA Methylation mapping identifies gene regulatory effects in patients with systemic lupus erythematosus. Ann Rheum Dis (2018) 77(5):736–43. doi: 10.1136/annrheumdis-2017-212379
22. Ding S, Zhang Q, Luo S, Gao L, Huang J, Lu J, et al. BCL-6 suppresses miR-142-3p/5p expression in SLE CD4 T cells by modulating histone methylation and acetylation of the miR-142 promoter. Cell Mol Immunol (2020) 17(5):474–82. doi: 10.1038/s41423-019-0268-3
23. Cremer T, Kurz A, Zirbel R, Dietzel S, Rinke B, Schröck E, et al. Role of chromosome territories in the functional compartmentalization of the cell nucleus. Cold Spring Harb Symp Quant Biol (1993) 58:777–92. doi: 10.1101/SQB.1993.058.01.085
24. Tanabe H, Müller S, Neusser M, von Hase J, Calcagno E, Cremer M, et al. Evolutionary conservation of chromosome territory arrangements in cell nuclei from higher primates. Proc Natl Acad Sci USA (2002) 99(7):4424–9. doi: 10.1073/pnas.072618599
25. Umlauf D, Mourad R. The 3D genome: from fundamental principles to disease and cancer. Semin Cell Dev Biol (2019) 90:128–37. doi: 10.1016/j.semcdb.2018.07.002
26. Mehta IS, Kulashreshtha M, Chakraborty S, Kolthur-Seetharam U, Rao BJ. Chromosome territories reposition during DNA damage-repair response. Genome Biol (2013) 14(12):R135. doi: 10.1186/gb-2013-14-12-r135
27. Rao SSP, Huntley MH, Durand NC, Stamenova EK, Bochkov ID, Robinson JT, et al. A 3D map of the human genome at kilobase resolution reveals principles of chromatin looping. Cell (2014) 159(7):1665–80. doi: 10.1016/j.cell.2014.11.021
28. Hnisz D, Day DS, Young RA. Insulated neighborhoods: structural and functional units of mammalian gene control. Cell (2016) 167(5):1188–200. doi: 10.1016/j.cell.2016.10.024
29. Nora EP, Lajoie BR, Schulz EG, Giorgetti L, Okamoto I, Servant N, et al. Spatial partitioning of the regulatory landscape of the X-inactivation center. Nature (2012) 485(7398):381–5. doi: 10.1038/nature11049
30. Madani Tonekaboni SA, Haibe-Kains B, Lupien M. Large Organized chromatin lysine domains help distinguish primitive from differentiated cell populations. Nat Commun (2021) 12(1):499. doi: 10.1038/s41467-020-20830-9
31. Rodríguez-Carballo E, Lopez-Delisle L, Zhan Y, Fabre PJ, Beccari L, El-Idrissi I, et al. The cluster is a dynamic and resilient TAD boundary controlling the segregation of antagonistic regulatory landscapes. Genes Dev (2017) 31(22):2264–81. doi: 10.1101/gad.307769.117
32. Vertii A, Ou J, Yu J, Yan A, Pagès H, Liu H, et al. Two contrasting classes of nucleolus-associated domains in mouse fibroblast heterochromatin. Genome Res (2019) 29(8):1235–49. doi: 10.1101/gr.247072.118
33. van Schaik T, Liu NQ, Manzo SG, Peric-Hupkes D, de Wit E, van Steensel B. CTCF and cohesin promote focal detachment of DNA from the nuclear lamina. Genome Biol (2022) 23(1):185. doi: 10.1186/s13059-022-02754-3
34. Bersaglieri C, Kresoja-Rakic J, Gupta S, Bär D, Kuzyakiv R, Panatta M, et al. Genome-wide maps of nucleolus interactions reveal distinct layers of repressive chromatin domains. Nat Commun (2022) 13(1):1483. doi: 10.1038/s41467-022-29146-2
35. Krijger PHL, de Laat W. Regulation of disease-associated gene expression in the 3D genome. Nat Rev Mol Cell Biol (2016) 17(12):771–82. doi: 10.1038/nrm.2016.138
36. Rowley MJ, Corces VG. Organizational principles of 3D genome architecture. Nat Rev Genet (2018) 19(12):789–800. doi: 10.1038/s41576-018-0060-8
37. Davidson IF, Peters J-M. Genome folding through loop extrusion by SMC complexes. Nat Rev Mol Cell Biol (2021) 22(7):445–64. doi: 10.1038/s41580-021-00349-7
38. Drissen R, Palstra R-J, Gillemans N, Splinter E, Grosveld F, Philipsen S, et al. The active spatial organization of the beta-globin locus requires the transcription factor EKLF. Genes Dev (2004) 18(20):2485–90. doi: 10.1101/gad.317004
39. Deng W, Lee J, Wang H, Miller J, Reik A, Gregory PD, et al. Controlling long-range genomic interactions at a native locus by targeted tethering of a looping factor. Cell (2012) 149(6):1233–44. doi: 10.1016/j.cell.2012.03.051
40. Denholtz M, Bonora G, Chronis C, Splinter E, de Laat W, Ernst J, et al. Long-range chromatin contacts in embryonic stem cells reveal a role for pluripotency factors and polycomb proteins in genome organization. Cell Stem Cell (2013) 13(5):602–16. doi: 10.1016/j.stem.2013.08.013
41. Liu NQ, Maresca M, van den Brand T, Braccioli L, Schijns MMGA, Teunissen H, et al. WAPL maintains a cohesin loading cycle to preserve cell-type-specific distal gene regulation. Nat Genet (2021) 53(1):100–9. doi: 10.1038/s41588-020-00744-4
42. Beagan JA, Duong MT, Titus KR, Zhou L, Cao Z, Ma J, et al. YY1 and CTCF orchestrate a 3D chromatin looping switch during early neural lineage commitment. Genome Res (2017) 27(7):1139–52. doi: 10.1101/gr.215160.116
43. Jiao W, Chen Y, Song H, Li D, Mei H, Yang F, et al. HPSE enhancer RNA promotes cancer progression through driving chromatin looping and regulating hnRNPU/p300/EGR1/HPSE axis. Oncogene (2018) 37(20):2728–45. doi: 10.1038/s41388-018-0128-0
44. Di Giammartino DC, Polyzos A, Apostolou E. Transcription factors: building hubs in the 3D space. Cell Cycle (2020) 19(19):2395–410. doi: 10.1080/15384101.2020.1805238
45. Cremer T, Cremer C. Chromosome territories, nuclear architecture and gene regulation in mammalian cells. Nat Rev Genet (2001) 2(4):292–301. doi: 10.1038/35066075
46. Cremer M, Grasser F, Lanctôt C, Müller S, Neusser M, Zinner R, et al. Multicolor 3D fluorescence in situ hybridization for imaging interphase chromosomes. Methods Mol Biol (2008) 463:205–39. doi: 10.1007/978-1-59745-406-3_15
47. Lakadamyali M, Cosma MP. Visualizing the genome in high resolution challenges our textbook understanding. Nat Methods (2020) 17(4):371–9. doi: 10.1038/s41592-020-0758-3
48. Song F, Chen P, Sun D, Wang M, Dong L, Liang D, et al. Cryo-EM study of the chromatin fiber reveals a double helix twisted by tetranucleosomal units. Science (2014) 344(6182):376–80. doi: 10.1126/science.1251413
49. Yu M, Ren B. The three-dimensional organization of mammalian genomes. Annu Rev Cell Dev Biol (2017) 33:265–89. doi: 10.1146/annurev-cellbio-100616-060531
50. Zhao Z, Tavoosidana G, Sjölinder M, Göndör A, Mariano P, Wang S, et al. Circular chromosome conformation capture (4C) uncovers extensive networks of epigenetically regulated intra- and interchromosomal interactions. Nat Genet (2006) 38(11):1341–7. doi: 10.1038/ng1891
51. Cameron CJF, Wang XQD, Dostie J, Blanchette M. LAMPS: an analysis pipeline for sequence-specific ligation-mediated amplification reads. BMC Res Notes (2020) 13(1):273. doi: 10.1186/s13104-020-05106-1
52. Sanyal A, Lajoie BR, Jain G, Dekker J. The long-range interaction landscape of gene promoters. Nature (2012) 489(7414):109–13. doi: 10.1038/nature11279
53. Hsieh T-HS, Weiner A, Lajoie B, Dekker J, Friedman N, Rando OJ. Mapping nucleosome resolution chromosome folding in yeast by micro-c. Cell (2015) 162(1):108–19. doi: 10.1016/j.cell.2015.05.048
54. Hsieh T-HS, Fudenberg G, Goloborodko A, Rando OJ. Micro-c XL: assaying chromosome conformation from the nucleosome to the entire genome. Nat Methods (2016) 13(12):1009–11. doi: 10.1038/nmeth.4025
55. Ma W, Ay F, Lee C, Gulsoy G, Deng X, Cook S, et al. Fine-scale chromatin interaction maps reveal the cis-regulatory landscape of human lincRNA genes. Nat Methods (2015) 12(1):71–8. doi: 10.1038/nmeth.3205
56. Liang Z, Li G, Wang Z, Djekidel MN, Li Y, Qian M-P, et al. BL-Hi-C is an efficient and sensitive approach for capturing structural and regulatory chromatin interactions. Nat Commun (2017) 8(1):1622. doi: 10.1038/s41467-017-01754-3
57. Mifsud B, Tavares-Cadete F, Young AN, Sugar R, Schoenfelder S, Ferreira L, et al. Mapping long-range promoter contacts in human cells with high-resolution capture Hi-c. Nat Genet (2015) 47(6):598–606. doi: 10.1038/ng.3286
58. Hughes JR, Roberts N, McGowan S, Hay D, Giannoulatou E, Lynch M, et al. Analysis of hundreds of cis-regulatory landscapes at high resolution in a single, high-throughput experiment. Nat Genet (2014) 46(2):205–12. doi: 10.1038/ng.2871
59. Fullwood MJ, Ruan Y. ChIP-based methods for the identification of long-range chromatin interactions. J Cell Biochem (2009) 107(1):30–9. doi: 10.1002/jcb.22116
60. Fullwood MJ, Wei C-L, Liu ET, Ruan Y. Next-generation DNA sequencing of paired-end tags (PET) for transcriptome and genome analyses. Genome Res (2009) 19(4):521–32. doi: 10.1101/gr.074906.107
61. Mumbach MR, Rubin AJ, Flynn RA, Dai C, Khavari PA, Greenleaf WJ, et al. HiChIP: efficient and sensitive analysis of protein-directed genome architecture. Nat Methods (2016) 13(11):919–22. doi: 10.1038/nmeth.3999
62. Sabo PJ, Kuehn MS, Thurman R, Johnson BE, Johnson EM, Cao H, et al. Genome-scale mapping of DNase I sensitivity in vivo using tiling DNA microarrays. Nat Methods (2006) 3(7):511–8. doi: 10.1038/nmeth890
63. Bianco S, Rodrigue S, Murphy BD, Gévry N. Global mapping of open chromatin regulatory elements by formaldehyde-assisted isolation of regulatory elements followed by sequencing (FAIRE-seq). Methods Mol Biol (2015) 1334:261–72. doi: 10.1007/978-1-4939-2877-4_17
64. Lorzadeh A, Bilenky M, Hammond C, Knapp DJHF, Li L, Miller PH, et al. Nucleosome density ChIP-seq identifies distinct chromatin modification signatures associated with MNase accessibility. Cell Rep (2016) 17(8):2112–24. doi: 10.1016/j.celrep.2016.10.055
65. Thurman RE, Rynes E, Humbert R, Vierstra J, Maurano MT, Haugen E, et al. The accessible chromatin landscape of the human genome. Nature (2012) 489(7414):75–82. doi: 10.1038/nature11232
66. Cusanovich DA, Daza R, Adey A, Pliner HA, Christiansen L, Gunderson KL, et al. Multiplex single cell profiling of chromatin accessibility by combinatorial cellular indexing. Science (2015) 348(6237):910–4. doi: 10.1126/science.aab1601
67. Mehra P, Wells AD. Variant to gene mapping to discover new targets for immune tolerance. Front Immunol (2021) 12:633219. doi: 10.3389/fimmu.2021.633219
68. Xu L, Yin L, Qi Y, Tan X, Gao M, Peng J. 3D disorganization and rearrangement of genome provide insights into pathogenesis of NAFLD by integrated Hi-c, nanopore, and RNA sequencing. Acta Pharm Sin B (2021) 11(10):3150–64. doi: 10.1016/j.apsb.2021.03.022
69. Pan C-W, Wen S, Chen L, Wei Y, Niu Y, Zhao Y. Functional roles of antisense enhancer RNA for promoting prostate cancer progression. Theranostics (2021) 11(4):1780–94. doi: 10.7150/thno.51931
70. Battle A, Brown CD, Engelhardt BE, Montgomery SB. Genetic effects on gene expression across human tissues. Nature (2017) 550(7675):204–13. doi: 10.1038/nature24277
71. Nakato R, Sakata T. Methods for ChIP-seq analysis: a practical workflow and advanced applications. Methods (2021) 187:44–53. doi: 10.1016/j.ymeth.2020.03.005
72. Kaya-Okur HS, Wu SJ, Codomo CA, Pledger ES, Bryson TD, Henikoff JG, et al. CUT&Tag for efficient epigenomic profiling of small samples and single cells. Nat Commun (2019) 10(1):1930. doi: 10.1038/s41467-019-09982-5
73. Klemm SL, Shipony Z, Greenleaf WJ. Chromatin accessibility and the regulatory epigenome. Nat Rev Genet (2019) 20(4):207–20. doi: 10.1038/s41576-018-0089-8
74. Minnoye L, Marinov GK, Krausgruber T, Pan L, Marand AP, Secchia S, et al. Chromatin accessibility profiling methods. Nat Rev Methods Primers (2021) 1(1):10. doi: 10.1038/s43586-020-00008-9
75. Cho JS, Blitz IL, Cho KWY. DNase-seq to study chromatin accessibility in early embryos. Cold Spring Harb Protoc (2019) 2019(4). doi: 10.1101/pdb.prot098335
76. Zhao H, Zhang W, Zhang T, Lin Y, Hu Y, Fang C, et al. Genome-wide MNase hypersensitivity assay unveils distinct classes of open chromatin associated with H3K27me3 and DNA methylation in arabidopsis thaliana. Genome Biol (2020) 21(1):24. doi: 10.1186/s13059-020-1927-5
77. Corces MR, Trevino AE, Hamilton EG, Greenside PG, Sinnott-Armstrong NA, Vesuna S, et al. An improved ATAC-seq protocol reduces background and enables interrogation of frozen tissues. Nat Methods (2017) 14(10):959–62. doi: 10.1038/nmeth.4396
78. Zhang K, Hocker JD, Miller M, Hou X, Chiou J, Poirion OB, et al. A single-cell atlas of chromatin accessibility in the human genome. Cell (2021) 184(24):5985–6001.e19. doi: 10.1016/j.cell.2021.10.024
79. Domcke S, Hill AJ, Daza RM, Cao J, O'Day DR, Pliner HA, et al. A human cell atlas of fetal chromatin accessibility. Science (2020) 370(6518):eaba7612. doi: 10.1126/science.aba7612
80. Wei X, Xiang Y, Peters DT, Marius C, Sun T, Shan R, et al. HiCAR is a robust and sensitive method to analyze open-chromatin-associated genome organization. Mol Cell (2022) 82(6):1225–1238.e6. doi: 10.1016/j.molcel.2022.01.023
81. Duan Z. Targeted DNase Hi-c. Methods Mol Biol (2021) 2157:65–83. doi: 10.1007/978-1-0716-0664-3_5
82. Zhang Y, Li Z, Bian S, Zhao H, Feng D, Chen Y, et al. HiCoP, a simple and robust method for detecting interactions of regulatory regions. Epigenet Chromatin (2020) 13(1):27. doi: 10.1186/s13072-020-00348-6
83. Li T, Jia L, Cao Y, Chen Q, Li C. OCEAN-c: mapping hubs of open chromatin interactions across the genome reveals gene regulatory networks. Genome Biol (2018) 19(1):54. doi: 10.1186/s13059-018-1430-4
84. Wang S, Wen F, Wiley GB, Kinter MT, Gaffney PM. An enhancer element harboring variants associated with systemic lupus erythematosus engages the TNFAIP3 promoter to influence A20 expression. PloS Genet (2013) 9(9):e1003750. doi: 10.1371/journal.pgen.1003750
85. Pasula S, Gopalakrishnan J, Fu Y, Tessneer KL, Wiley MM, Pelikan RC, et al. Systemic lupus erythematosus variants modulate the function of an enhancer upstream of TNFAIP3. Front Genet (2022) 13:1011965. doi: 10.3389/fgene.2022.1011965
86. Pasula S, Tessneer KL, Fu Y, Gopalakrishnan J, Pelikan RC, Kelly JA, et al. Role of systemic lupus erythematosus risk variants with opposing functional effects as a driver of hypomorphic expression of TNIP1 and other genes within a three-dimensional chromatin network. Arthritis Rheumatol (2020) 72(5):780–90. doi: 10.1002/art.41188
87. Zhang Y, Day K, Absher DM. STAT3-mediated allelic imbalance of novel genetic variant Rs1047643 and b-cell-specific super-enhancer in association with systemic lupus erythematosus. Elife (2022) 11:e72837. doi: 10.7554/eLife.72837
88. Luo X, Yang W, Ye D-Q, Cui H, Zhang Y, Hirankarn N, et al. A functional variant in microRNA-146a promoter modulates its expression and confers disease risk for systemic lupus erythematosus. PloS Genet (2011) 7(6):e1002128. doi: 10.1371/journal.pgen.1002128
89. Hou G, Zhou T, Xu N, Yin Z, Zhu X, Zhang Y, et al. Integrative functional genomics identifies SLE causal genetic variant in the IRF5 risk locus. Arthritis Rheumatol (2022) 75(4):574–585. doi: 10.1002/art.42390
90. Thynn HN, Chen X-F, Hu W-X, Duan Y-Y, Zhu D-L, Chen H, et al. An allele-specific functional SNP associated with two systemic autoimmune diseases modulates IRF5 expression by long-range chromatin loop formation. J Invest Dermatol (2020) 140(2):348–360.e11. doi: 10.1016/j.jid.2019.06.147
91. McGovern A, Schoenfelder S, Martin P, Massey J, Duffus K, Plant D, et al. Capture Hi-c identifies a novel causal gene, IL20RA, in the pan-autoimmune genetic susceptibility region 6q23. Genome Biol (2016) 17(1):212. doi: 10.1186/s13059-016-1078-x
92. Martin P, McGovern A, Massey J, Schoenfelder S, Duffus K, Yarwood A, et al. Identifying causal genes at the multiple sclerosis associated region 6q23 using capture Hi-c. PloS One (2016) 11(11):e0166923. doi: 10.1371/journal.pone.0166923
93. Liu N, Sadlon T, Wong YY, Pederson S, Breen J, Barry SC. 3DFAACTS-SNP: using regulatory T cell-specific epigenomics data to uncover candidate mechanisms of type 1 diabetes (T1D) risk. Epigenet Chromatin (2022) 15(1):24. doi: 10.1186/s13072-022-00456-5
94. Kong X, Sawalha AH. Takayasu arteritis risk locus in represses the anti-inflammatory gene through chromatin looping and recruiting MEF2-HDAC complex. Ann Rheum Dis (2019) 78(10):1388–97. doi: 10.1136/annrheumdis-2019-215567
95. Matthews SM, Eshelman MA, Berg AS, Koltun WA, Yochum GS. The crohn's disease associated SNP rs6651252 impacts MYC gene expression in human colonic epithelial cells. PloS One (2019) 14(2):e0212850. doi: 10.1371/journal.pone.0212850
96. Wang Z, Liang Q, Qian X, Hu B, Zheng Z, Wang J, et al. An autoimmune pleiotropic SNP modulates IRF5 alternative promoter usage through ZBTB3-mediated chromatin looping. Nat Commun (2023) 14 1):1208. doi: 10.1038/s41467-023-36897-z
97. Garrido-Cardenas JA, Mesa-Valle C, Manzano-Agugliaro F. Trends in plant research using molecular markers. Planta (2018) 247(3):543–57. doi: 10.1007/s00425-017-2829-y
98. Ulirsch JC, Lareau CA, Bao EL, Ludwig LS, Guo MH, Benner C, et al. Interrogation of human hematopoiesis at single-cell and single-variant resolution. Nat Genet (2019) 51(4):683–93. doi: 10.1038/s41588-019-0362-6
100. Smale ST, Natoli G. Transcriptional control of inflammatory responses. Cold Spring Harb Perspect Biol (2014) 6(11):a016261. doi: 10.1101/cshperspect.a016261
101. Cuartero S, Stik G, Stadhouders R. Three-dimensional genome organization in immune cell fate and function. Nat Rev Immunol (2022) 23(4):206–21. doi: 10.1038/s41577-022-00774-5
102. Bashford-Rogers RJM, Smith KGC, Thomas DC. Antibody repertoire analysis in polygenic autoimmune diseases. Immunology (2018) 155(1):3–17. doi: 10.1111/imm.12927
103. Chi X, Li Y, Qiu XV. (D)J recombination, somatic hypermutation and class switch recombination of immunoglobulins: mechanism and regulation. Immunology (2020) 160(3):233–47. doi: 10.1111/imm.13176
104. Schatz DG, Ji Y. Recombination centers and the orchestration of V (D)J recombination. Nat Rev Immunol (2011) 11(4):251–63. doi: 10.1038/nri2941
105. Dekker J, Marti-Renom MA, Mirny LA. Exploring the three-dimensional organization of genomes: interpreting chromatin interaction data. Nat Rev Genet (2013) 14(6):390–403. doi: 10.1038/nrg3454
106. Lucas JS, Zhang Y, Dudko OK, Murre C. 3D trajectories adopted by coding and regulatory DNA elements: first-passage times for genomic interactions. Cell (2014) 158(2):339–52. doi: 10.1016/j.cell.2014.05.036
107. Johanson TM, Lun ATL, Coughlan HD, Tan T, Smyth GK, Nutt SL, et al. Transcription-factor-mediated supervision of global genome architecture maintains b cell identity. Nat Immunol (2018) 19(11):1257–64. doi: 10.1038/s41590-018-0234-8
108. Lin YC, Benner C, Mansson R, Heinz S, Miyazaki K, Miyazaki M, et al. Global changes in the nuclear positioning of genes and intra- and interdomain genomic interactions that orchestrate b cell fate. Nat Immunol (2012) 13(12):1196–204. doi: 10.1038/ni.2432
109. Holmes ML, Carotta S, Corcoran LM, Nutt SL. Repression of Flt3 by Pax5 is crucial for b-cell lineage commitment. Genes Dev (2006) 20(8):933–8. doi: 10.1101/gad.1396206
110. Hill L, Ebert A, Jaritz M, Wutz G, Nagasaka K, Tagoh H, et al. Wapl repression by Pax5 promotes V gene recombination by igh loop extrusion. Nature (2020) 584(7819):142–7. doi: 10.1038/s41586-020-2454-y
111. Hosokawa H, Rothenberg EV. Cytokines, transcription factors, and the initiation of T-cell development. Cold Spring Harb Perspect Biol (2018) 10(5):a028621. doi: 10.1101/cshperspect.a028621
112. Hu G, Cui K, Fang D, Hirose S, Wang X, Wangsa D, et al. Transformation of accessible chromatin and 3D nucleome underlies lineage commitment of early T cells. Immunity (2018) 48(2):227–242.e8. doi: 10.1016/j.immuni.2018.01.013
113. Isoda T, Moore AJ, He Z, Chandra V, Aida M, Denholtz M, et al. Non-coding transcription instructs chromatin folding and compartmentalization to dictate enhancer-promoter communication and T cell fate. Cell (2017) 171(1):103–119.e18. doi: 10.1016/j.cell.2017.09.001
114. Wang W, Chandra A, Goldman N, Yoon S, Ferrari EK, Nguyen SC, et al. TCF-1 promotes chromatin interactions across topologically associating domains in T cell progenitors. Nat Immunol (2022) 23(7):1052–62. doi: 10.1038/s41590-022-01232-z
115. Troutman TD, Kofman E, Glass CK. Exploiting dynamic enhancer landscapes to decode macrophage and microglia phenotypes in health and disease. Mol Cell (2021) 81(19):3888–903. doi: 10.1016/j.molcel.2021.08.004
116. Platanitis E, Gruener S, Ravi Sundar Jose Geetha A, Boccuni L, Vogt A, Novatchkova M, et al. Interferons reshape the 3D conformation and accessibility of macrophage chromatin. iScience (2022) 25(3):103840. doi: 10.1016/j.isci.2022.103840
117. Stadhouders R, Filion GJ, Graf T. Transcription factors and 3D genome conformation in cell-fate decisions. Nature (2019) 569(7756):345–54. doi: 10.1038/s41586-019-1182-7
118. Shan Q, Zhu S, Chen X, Liu J, Yuan S, Li X, et al. Tcf1-CTCF cooperativity shapes genomic architecture to promote CD8 T cell homeostasis. Nat Immunol (2022) 23(8):1222–35. doi: 10.1038/s41590-022-01263-6
119. Bediaga NG, Coughlan HD, Johanson TM, Garnham AL, Naselli G, Schröder J, et al. Multi-level remodelling of chromatin underlying activation of human T cells. Sci Rep (2021) 11(1):528. doi: 10.1038/s41598-020-80165-9
120. Avni O, Lee D, Macian F, Szabo SJ, Glimcher LH, Rao AT. (H) cell differentiation is accompanied by dynamic changes in histone acetylation of cytokine genes. Nat Immunol (2002) 3(7):643–51. doi: 10.1038/ni808
121. Liu CF, Brandt GS, Hoang QQ, Naumova N, Lazarevic V, Hwang ES, et al. Crystal structure of the DNA binding domain of the transcription factor T-bet suggests simultaneous recognition of distant genome sites. Proc Natl Acad Sci USA (2016) 113(43):E6572–81. doi: 10.1073/pnas.1613914113
122. Hakim O, Sung M-H, Nakayamada S, Voss TC, Baek S, Hager GL. Spatial congregation of STAT binding directs selective nuclear architecture during T-cell functional differentiation. Genome Res (2013) 23(3):462–72. doi: 10.1101/gr.147652.112
123. Pham D, Moseley CE, Gao M, Savic D, Winstead CJ, Sun M, et al. Batf pioneers the reorganization of chromatin in developing effector T cells via Ets1-dependent recruitment of ctcf. Cell Rep (2019) 29(5):1203–1220.e7. doi: 10.1016/j.celrep.2019.09.064
124. Vilarrasa-Blasi R, Soler-Vila P, Verdaguer-Dot N, Russiñol N, Di Stefano M, Chapaprieta V, et al. Dynamics of genome architecture and chromatin function during human b cell differentiation and neoplastic transformation. Nat Commun (2021) 12(1):651. doi: 10.1038/s41467-020-20849-y
125. Bunting KL, Soong TD, Singh R, Jiang Y, Béguelin W, Poloway DW, et al. Multi-tiered reorganization of the genome during b cell affinity maturation anchored by a germinal center-specific locus control region. Immunity (2016) 45(3):497–512. doi: 10.1016/j.immuni.2016.08.012
126. Stadhouders R, Lubberts E, Hendriks RW. A cellular and molecular view of T helper 17 cell plasticity in autoimmunity. J Autoimmun (2018) 87:1–15. doi: 10.1016/j.jaut.2017.12.007
127. Bourges C, Groff AF, Burren OS, Gerhardinger C, Mattioli K, Hutchinson A, et al. Resolving mechanisms of immune-mediated disease in primary CD4 T cells. EMBO Mol Med (2020) 12(5):e12112. doi: 10.15252/emmm.202012112
128. Zelenka T, Spilianakis C. SATB1-mediated chromatin landscape in T cells. Nucleus (2020) 11(1):117–31. doi: 10.1080/19491034.2020.1775037
129. Hao B, Naik AK, Watanabe A, Tanaka H, Chen L, Richards HW, et al. An anti-silencer- and SATB1-dependent chromatin hub regulates Rag1 and Rag2 gene expression during thymocyte development. J Exp Med (2015) 212(5):809–24. doi: 10.1084/jem.20142207
130. Feng D, Chen Y, Dai R, Bian S, Xue W, Zhu Y, et al. Chromatin organizer SATB1 controls the cell identity of CD4+ CD8+ double-positive thymocytes by regulating the activity of super-enhancers. Nat Commun (2022) 13(1):5554. doi: 10.1038/s41467-022-33333-6
131. Benacerraf B, McDevitt HO. Histocompatibility-linked immune response genes. Science (1972) 175(4019):273–9. doi: 10.1126/science.175.4019.273
132. Burren OS, Rubio García A, Javierre B-M, Rainbow DB, Cairns J, Cooper NJ, et al. Chromosome contacts in activated T cells identify autoimmune disease candidate genes. Genome Biol (2017) 18(1):165. doi: 10.1186/s13059-017-1285-0
133. Martin P, Ding J, Duffus K, Gaddi VP, McGovern A, Ray-Jones H, et al. Chromatin interactions reveal novel gene targets for drug repositioning in rheumatic diseases. Ann Rheum Dis (2019) 78(8):1127–34. doi: 10.1136/annrheumdis-2018-214649
134. Shi C, Ray-Jones H, Ding J, Duffus K, Fu Y, Gaddi VP, et al. Chromatin looping links target genes with genetic risk loci for dermatological traits. J Invest Dermatol (2021) 141(8):1975–84. doi: 10.1016/j.jid.2021.01.015
135. Ntasis VF, Panousis NI, Tektonidou MG, Dermitzakis ET, Boumpas DT, Bertsias GK, et al. Extensive fragmentation and re-organization of transcription in systemic lupus erythematosus. Sci Rep (2020) 10(1):16648. doi: 10.1038/s41598-020-73654-4
136. Zhao M, Feng D, Hu L, Liu L, Wu J, Hu Z, et al. 3D genome alterations in T cells associated with disease activity of systemic lupus erythematosus. Ann Rheum Dis (2023) 82(2):226–234. doi: 10.1136/ard-2022-222653
137. Graham RR, Cotsapas C, Davies L, Hackett R, Lessard CJ, Leon JM, et al. Genetic variants near TNFAIP3 on 6q23 are associated with systemic lupus erythematosus. Nat Genet (2008) 40(9):1059–61. doi: 10.1038/ng.200
138. Wang S, Wen F, Tessneer KL, Gaffney PM. TALEN-mediated enhancer knockout influences TNFAIP3 gene expression and mimics a molecular phenotype associated with systemic lupus erythematosus. Genes Immun (2016) 17(3):165–70. doi: 10.1038/gene.2016.4
139. Sokhi UK, Liber MP, Frye L, Park S, Kang K, Pannellini T, et al. Dissection and function of autoimmunity-associated TNFAIP3 (A20) gene enhancers in humanized mouse models. Nat Commun (2018) 9(1):658. doi: 10.1038/s41467-018-03081-7
140. Hui-Yuen JS, Zhu L, Wong LP, Jiang K, Chen Y, Liu T, et al. Chromatin landscapes and genetic risk in systemic lupus. Arthritis Res Ther (2016) 18(1):281. doi: 10.1186/s13075-016-1169-9
141. Vahedi G, Kanno Y, Furumoto Y, Jiang K, Parker SCJ, Erdos MR, et al. Super-enhancers delineate disease-associated regulatory nodes in T cells. Nature (2015) 520(7548):558–62. doi: 10.1038/nature14154
142. Su C, Johnson ME, Torres A, Thomas RM, Manduchi E, Sharma P, et al. Mapping effector genes at lupus GWAS loci using promoter capture-c in follicular helper T cells. Nat Commun (2020) 11(1):3294. doi: 10.1038/s41467-020-17089-5
143. Zhou C, Zhao L, Wang K, Qi Q, Wang M, Sun P, et al. MicroRNA−146a inhibits NF−κB activation and pro−inflammatory cytokine production by regulating IRAK1 expression in THP−1 cells. Exp Ther Med (2019) 18(4):3078–84. doi: 10.3892/etm.2019.7881
144. Xia Y, Liu X, Mu W, Ma C, Wang L, Jiao Y, et al. Capturing 3D chromatin maps of human primary monocytes: insights from high-resolution Hi-c. Front Immunol (2022) 13:837336. doi: 10.3389/fimmu.2022.837336
145. McInnes IB, Schett G. The pathogenesis of rheumatoid arthritis. N Engl J Med (2011) 365(23):2205–19. doi: 10.1056/NEJMra1004965
146. Kragstrup TW, Andersen T, Heftdal LD, Hvid M, Gerwien J, Sivakumar P, et al. The IL-20 cytokine family in rheumatoid arthritis and spondyloarthritis. Front Immunol (2018) 9:2226. doi: 10.3389/fimmu.2018.02226
147. Yang J, McGovern A, Martin P, Duffus K, Ge X, Zarrineh P, et al. Analysis of chromatin organization and gene expression in T cells identifies functional genes for rheumatoid arthritis. Nat Commun (2020) 11(1):4402. doi: 10.1038/s41467-020-18180-7
148. Orozco G. Fine mapping with epigenetic information and 3D structure. Semin Immunopathol (2022) 44(1):115–25. doi: 10.1007/s00281-021-00906-4
149. Ge X, Frank-Bertoncelj M, Klein K, McGovern A, Kuret T, Houtman M, et al. Functional genomics atlas of synovial fibroblasts defining rheumatoid arthritis heritability. Genome Biol (2021) 22(1):247. doi: 10.1186/s13059-021-02460-6
150. McGinley MP, Goldschmidt CH, Rae-Grant AD. Diagnosis and treatment of multiple sclerosis: a review. JAMA (2021) 325(8):765–79. doi: 10.1001/jama.2020.26858
151. Davison LJ, Wallace C, Cooper JD, Cope NF, Wilson NK, Smyth DJ, et al. Long-range DNA looping and gene expression analyses identify DEXI as an autoimmune disease candidate gene. Hum Mol Genet (2012) 21(2):322–33. doi: 10.1093/hmg/ddr468
152. Leikfoss IS, Keshari PK, Gustavsen MW, Bjølgerud A, Brorson IS, Celius EG, et al. Multiple sclerosis risk allele in CLEC16A acts as an expression quantitative trait locus for CLEC16A and SOCS1 in CD4+ T cells. PloS One (2015) 10(7):e0132957. doi: 10.1371/journal.pone.0132957
153. Gyllenberg A, Asad S, Piehl F, Swanberg M, Padyukov L, Van Yserloo B, et al. Age-dependent variation of genotypes in MHC II transactivator gene (CIITA) in controls and association to type 1 diabetes. Genes Immun (2012) 13(8):632–40. doi: 10.1038/gene.2012.44
154. Fenner JE, Starr R, Cornish AL, Zhang J-G, Metcalf D, Schreiber RD, et al. Suppressor of cytokine signaling 1 regulates the immune response to infection by a unique inhibition of type I interferon activity. Nat Immunol (2006) 7(1):33–9. doi: 10.1038/ni1287
155. Ram R, Mehta M, Nguyen QT, Larma I, Boehm BO, Pociot F, et al. Systematic evaluation of genes and genetic variants associated with type 1 diabetes susceptibility. J Immunol (2016) 196(7):3043–53. doi: 10.4049/jimmunol.1502056
156. Nyaga DM, Vickers MH, Jefferies C, Perry JK, O'Sullivan JM. Type 1 diabetes mellitus-associated genetic variants contribute to overlapping immune regulatory networks. Front Genet (2018) 9:535. doi: 10.3389/fgene.2018.00535
157. Tomlinson MJ, Pitsillides A, Pickin R, Mika M, Keene KL, Hou X, et al. Fine mapping and functional studies of risk variants for type 1 diabetes at chromosome 16p13.13. Diabetes (2014) 63(12):4360–8. doi: 10.2337/db13-1785
158. Frieri M, Angadi C, Paolano A, Oster N, Blau SP, Yang S, et al. Altered T cell subpopulations and lymphocytes expressing natural killer cell phenotypes in patients with progressive systemic sclerosis. J Allergy Clin Immunol (1991) 87(4):773–9. doi: 10.1016/0091-6749(91)90121-4
159. Li T, Ortiz-Fernández L, Andrés-León E, Ciudad L, Javierre BM, López-Isac E, et al. Epigenomics and transcriptomics of systemic sclerosis CD4+ T cells reveal long-range dysregulation of key inflammatory pathways mediated by disease-associated susceptibility loci. Genome Med (2020) 12(1):81. doi: 10.1186/s13073-020-00779-6
160. Wiehle L, Thorn GJ, Raddatz G, Clarkson CT, Rippe K, Lyko F, et al. DNA (de)methylation in embryonic stem cells controls CTCF-dependent chromatin boundaries. Genome Res (2019) 29(5):750–61. doi: 10.1101/gr.239707.118
161. Kaser A, Zeissig S, Blumberg RS. Inflammatory bowel disease. Annu Rev Immunol (2010) 28:573–621. doi: 10.1146/annurev-immunol-030409-101225
162. Mokry M, Middendorp S, Wiegerinck CL, Witte M, Teunissen H, Meddens CA, et al. Many inflammatory bowel disease risk loci include regions that regulate gene expression in immune cells and the intestinal epithelium. Gastroenterology (2014) 146(4):1040–7. doi: 10.1053/j.gastro.2013.12.003
163. Meddens CA, Harakalova M, van den Dungen NAM, Foroughi Asl H, Hijma HJ, Cuppen EPJG, et al. Systematic analysis of chromatin interactions at disease associated loci links novel candidate genes to inflammatory bowel disease. Genome Biol (2016) 17(1):247. doi: 10.1186/s13059-016-1100-3
Keywords: 3D genome, autoimmune disease, GWAS, gene expression, SNP, epigenetic
Citation: Qiu Y, Feng D, Jiang W, Zhang T, Lu Q and Zhao M (2023) 3D genome organization and epigenetic regulation in autoimmune diseases. Front. Immunol. 14:1196123. doi: 10.3389/fimmu.2023.1196123
Received: 29 March 2023; Accepted: 17 May 2023;
Published: 06 June 2023.
Edited by:
Kerstin Klein, University Hospital Bern, SwitzerlandReviewed by:
Charalampos Spilianakis, University of Crete, GreeceAndrew D. Wells, Children’s Hospital of Philadelphia, United States
Copyright © 2023 Qiu, Feng, Jiang, Zhang, Lu and Zhao. This is an open-access article distributed under the terms of the Creative Commons Attribution License (CC BY). The use, distribution or reproduction in other forums is permitted, provided the original author(s) and the copyright owner(s) are credited and that the original publication in this journal is cited, in accordance with accepted academic practice. No use, distribution or reproduction is permitted which does not comply with these terms.
*Correspondence: Ming Zhao, emhhb21pbmczMDFAcHVtY2Rlcm0uY2Ftcy5jbg==; Qianjin Lu, cWlhbmx1NTg2MEBwdW1jZGVybS5jYW1zLmNu