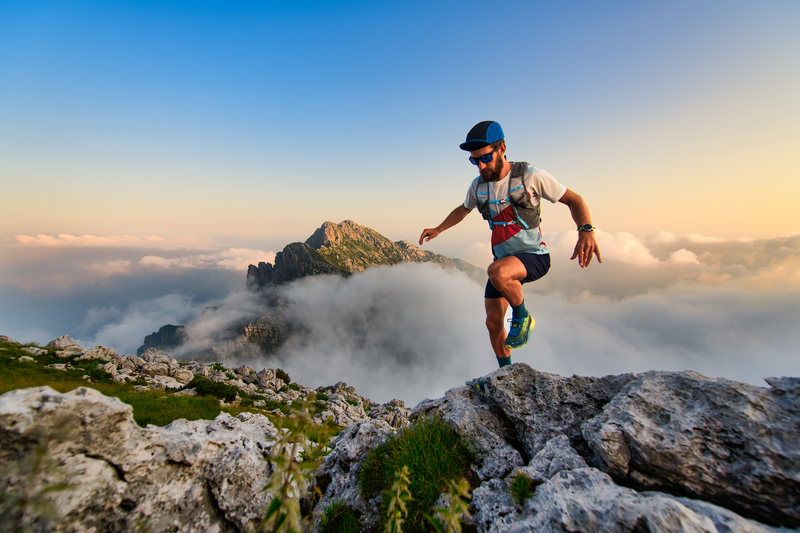
95% of researchers rate our articles as excellent or good
Learn more about the work of our research integrity team to safeguard the quality of each article we publish.
Find out more
ORIGINAL RESEARCH article
Front. Immunol. , 26 July 2023
Sec. Inflammation
Volume 14 - 2023 | https://doi.org/10.3389/fimmu.2023.1194738
This article is part of the Research Topic New Insights of Immune Cells in Cardiovascular and Metabolic Disorders View all 36 articles
Background: Macrophages are considered an essential source of inflammatory cytokines, which play a pivotal role in the development of diabetes and its sequent complications. Therefore, a better understanding of the intersection between the development of diabetes and macrophage is of massive importance.
Objectives: In this study, we performed an informative bibliometric analysis to enlighten relevant research directions, provide valuable metrics for financing decisions, and help academics to gain a quick understanding of the current macrophage-related diabetes studies knowledge domain.
Methods: The Web of Science Core Collection database was used for literature retrieval and dataset export. Bibliometrix R-package was performed to conduct raw data screening, calculating, and visualizing.
Results: Between 2000 and 2022, the annual publication and citation trends steadily increased. Wu Yonggui was the scholar with the most published papers in this field. The institute with the highest number of published papers was the University of Michigan. The most robust academic collaboration was observed between China and the United States of America. Diabetologia was the journal that published the most relevant publications. The author’s keywords with the highest occurrences were “inflammation”, “diabetic nephropathy”, and “obesity”. In addition, “Macrophage polarization” was the current motor topic with potential research prospects.
Conclusions: These comprehensive and visualized bibliometric results summarized the significant findings in macrophage-related diabetes studies over the past 20 years. It would enlighten subsequent studies from a macro viewpoint and is also expected to strengthen investment policies in future macrophage-related diabetes studies.
The prevalence of diabetes is skyrocketing across the globe, which has become a severe public health issue. According to current estimates from International Diabetes Federation, diabetes is a metabolic disorder affecting approximately 537 million adults in 2021, an astonishing increase of 74 million (16%) compared with 2019 (1). Type 2 diabetes(T2D) is the most common type, characterized by insulin resistance and progressive β-cell dysfunction, which gradually deteriorates glucose homeostasis from prediabetes to diabetes. It can be attributed to oxidative stress, ectopic lipid deposition, lipotoxicity, and glucotoxicity leading to long-term micro and macrovascular complications (2–4). Worth noting that each of these potential mechanisms is linked to inflammation (5). A meta-analysis based on prospective studies has revealed that elevated levels of C-reactive protein (relative risk: 1.26 [95% CI, 1.16 to 1.37]) and interleukin-6 (IL-6) (relative risk: 1.31 [95% CI, 1.17 to 1.46]) are associated with an increased risk of T2D (6). Autoimmunity is the hallmark of type 1 diabetes—this multifactorial, organ-specific autoimmune disease results from insulitis by infiltrating immune cells. During insulitis, effector T cells secrete high levels of proinflammatory cytokines like tumor necrosis factor-alpha (TNF-α), IL-1β, and IL-12, which trigger the process of β cell destruction (7, 8).
It is widely accepted that multiple immune cell types participate in initiating and maintaining metabolic disorders, including diabetes. Macrophages are considered an essential source of inflammatory cytokines, which play a pivotal role in the development of diabetes and its sequent complications (9, 10). Furthermore, macrophage activation has been commonly used to describe macrophage activity in response to diverse stimuli that include microbial products, damaged cells, and particularly inflammatory cells (11). If the inflammatory conditions are not properly dealt with, it may result in the persistent activation of the immune system, which can contribute to the development of diabetes. However, macrophages are highly plastic, indicating that distinct functional subsets undergoing different phenotypic differentiation can exert their unique biological effects, including anti-inflammatory actions. Therefore, to discover and promote new molecular targets and therapies to improve life expectancy, a better understanding of the intersection between the development of diabetes and macrophage is of massive importance. Notwithstanding, there is no comprehensive elucidation of the current state and research hotspots of macrophage-related diabetes studies (MRDS).
In 1969, Pritchard defined bibliometric analysis as “the application of mathematical and statistical methods to books and other media of communication”. As a quantitative science approach that monitors and evaluates research characteristics and trends based on published data within a specific timeframe, it has gained popularity in medical research (12, 13). Based on powerful algorithms, Bibliometrix R-package is a widely used open-source science mapping application with solid visualization and data analysis capabilities (14). In this study, we performed a bibliometric analysis to inform relevant research directions, provide valuable metrics for financing decisions, and gain a quick understanding of the MRDS knowledge domain for academics through the following steps with visualization:(1) Calculate growth trends of publications and citations; (2) Discover collaboration between core countries, authors, and institutions; (3) Investigate influential journals and top-cited publications; (4) Summarize dynamic changes of research hotspots and detect forefront topics.
As one of the primary sources for scientometric analysis, the Web of Science (WoS) has a well-established citation network in different research fields (15). Dr. Ho Yuh-Shan proposed that the WoS topic search (TS) result in the intersectional knowledge domain may not be consistent with the article’s theme (16). Therefore, to get our bibliometric data more precisely, a systematic search strategy was developed using the following search terms based on title (TI) and author’s keyword (AK) search in the Science Citation Index Expanded (SCIE) of the WoS core collection database, regardless of language or document type: (TI=(diabet*) OR AK=(diabet*)) AND (TI=(macrophag*) OR AK=(macrophag*)). Notably, the raw data of publication records were extracted on a single day (December 4th, 2022) to avoid deviation errors from the WoSCC and saved as a TXT file.
Bibliometrix (version 3.2.1, R version 4.2.0) was performed to conduct raw data screening by two independent investigators. Publications fulfilling the following criteria would be included in our bibliometric analysis: (1) Papers that were published in English; (2) Document type should be review or article; (3) Papers that had no duplicates according to the authorship, publication title, and DOI number. Otherwise, only one of all duplicates would be retained;(4) Publication records must have complete information on authors, institutes, countries, journals, keywords, and citations. Two investigators (S.W. and Z. J.) independently screened each publication record for eligibility, and any discrepancies were settled through consultation with other authors to obtain a more precise data selection. The detailed filtering process is shown in Figure 1.
Figure 1 Flow chart of bibliometric analysis dataset establishment. DOI: Digital Object Unique Identifier.
Bibliometrix R-package was applied to perform statistical and visualizing procedures. In our study, we analyzed and summarized annual publications and citations, the contribution of authors, institutes, countries, journals, and keywords to explore research trends, collaborations, reliable sources, internal structures of research hotspots, and temporal distribution of frontier topics.
Specifically, the Hirsch index (H-index), an acknowledged quantitative metric defined as the maximum value of H such that an author has H publications that have each been cited at least H times, was performed to measure the academic influence of authors (17). The strength of journal influence was assessed by Impact Factor, which was obtained from the 2021 Journal Citation Report (JCR) (http://mjl.clarivate.com/). PageRank score, proposed by Larry Page, the Google company sponsor, is an alternative and newly emerged measurement of academic impact calculated by computer-based algorithms (18, 19). It was designed to embody the relevance and importance of different web pages. In our network analysis, those with a high PageRank score implied more intensive academic connections. Our previous work has proven this quantitative metric more intuitive and efficient (20).
A total of 1272 publications were selected in our bibliometric study, including 1099 original research full-length articles and 173 reviews. An apparent trend in annual publication numbers was observed in the past two decades, indicating a skyrocketed interest of endocrinologists in macrophage research (Figure 2A). Specifically, this growth could be divided into three phases: from 2000 to 2011 was the beginning study phase with less than 50 publications annually. Then, a stable growth period was found between 2012 to 2015. The number of publications has entered a tremendous development phase from 2016 onwards and peaked at 132 in 2021. The total mean citation per year represents the yearly average number of times each publication of MRDS has been cited. This metric can reveal the overall picture of the academic impact of papers published in a certain year. As shown in Figure 2B, literature published in 2005, 2006, 2014, and 2020 with higher citation times.
Figure 2 Annual trend chart of publications and citations between 2000 and 2022. The dotted line represents linear growth with r2 = 0.906 (A) and r2 = 0.226 (B).
In the past two decades, a total of 7520 authors have made their contributions to MRDS. Table 1 listed the top ten most prolific authors: Wu Yonggui was the scholar with the most published papers in this field (23 publications), followed by Stojanovic Ivana (12 publications), Aviram Michael (11 publications), and Gallagher Katherine A. (11 publications). In terms of H-index, Wu Yonggui (H-index:12), Aviram Michael (H-index:10), and Gallagher Katherine A. (H-index:9) and Stojanovic Ivana (H-index:9) ranked in the top three. The collaborations among the top 50 most prolific authors are shown in Figure 3. A total of eight academics, centered on Gallagher Katherine A. (PageRank score: 0.034), constituted the largest collaborative cluster of authors (purple group).
Figure 3 Collaboration network map of the top 50 most prolific authors. Nodes represent different authors, and lines represent connections between them. Ten isolated nodes were removed.
Additionally, we summarized the annual publication numbers and citation times of the top ten high-yielding authors, and Gallagher Katherine A. and Davis Frank M. (both from the purple cluster in Figure 3) have made a considerable contribution to this field in recent years, whose publications were all published in the last five years with higher total citations per year (Figure 4).
Figure 4 Publications and total citations per year of the top ten prolific authors between 2000 and 2022. the size of the circles represents the number of publications, and the color of the circles represents the number of citations per year.
We selected the top 50 countries and institutes that ranked according to the number of publications. The PageRank score of each country or institute was calculated through their connections to others. Figure 5 and Figure 6 showed cooperative network maps of countries and institutes, respectively. From the perspective of national cooperation, the closest academic connection was observed between China and the United States of America. The blue nodes formed the largest cluster (14 countries), centered on Germany (PageRank score: 0.077) and mainly composed of European countries.
Figure 5 Collaboration network map of the 50 top-publishing countries between 2000 and 2022. Nodes represent different countries, colors represent clusters, and lines represent connections between them. Cluster 1: red (10 items); Cluster 2: blue (14 items); Cluster 3: green (8 items); Cluster 4: orange (7 items); Cluster 5: purple (5 items); Cluster 6: brown (3 items). Three isolated nodes were removed.
Figure 6 Collaboration network map of the 50 top-publishing institutes between 2000 and 2022. Nodes represent different institutes, colors represent clusters, and lines represent connections between them. Cluster 1: green (6 items); Cluster 2: red (8 items); Cluster 3: purple (9 items); Cluster 4: orange (7 items); Cluster 5: brown (6 items); Cluster 6: blue (3 items).11 isolated nodes were removed.
We listed the top ten high-producing countries and institutes according to the number of papers published and PageRank scores (Table 2). China was the leader in this field with 315 publications, followed by other top-publishing countries, including the United States of America (293 publications) and Japan (123 publications). The institute with the most published papers was the University of Michigan (75 publications). According to the PageRank scores, the United States of America was the most influential country, and Shanghai Jiao Tong University was the most significant research institute.
All the included publications of the MRDS between 2000 and 2022 were published in 537 journals. According to the 2021 edition of the JCR, seven of the ten most highly published journals were in the Q1 JCR division, and two had an impact factor greater than 10 (Supplementary Table 1). As depicted in Figure 7 and Supplementary Table 1, Diabetologia (publication:45; IF:10.460), Frontiers in Immunology (publication:28; IF:8.787), and International Journal of Molecular (publication:26; IF:6.208) were the journals that published the most relevant publications. Additionally, Diabetes (citation:3365; IF:9.305), Journal of Clinical Investigation (citation:2101; IF:19.477), and Journal of Biological Chemistry (citation:1764; IF:5.485) were journals with the highest number of citations.
Figure 7 Cleveland dot plot of the top 20 journals ranked by publications and citations between 2000 and 2022.
Supplementary Table 2 summarized top-cited papers to reveal essential publications from our bibliometric dataset. To better show the citation trend in relatively recent years, we ranked the top ten top-cited publications between 2000 and 2022 in terms of both total citations and total citations per year, respectively. The top-cited paper with the highest total citations was “Adipocyte Death Defines Macrophage Localization and Function in Adipose Tissue of Obese Mice and Humans”, an original full-length research article that published by Saverio Cinti et al., and “Inflammation as a Link Between Obesity, Metabolic Syndrome and Type 2 Diabetes” by Nathalie Esser et al. had the highest total citations per year. Notably, a review published in 2017 named “Macrophage Phenotypes Regulate Scar Formation and Chronic Wound Healing” (Supplementary Table 2) was the most recent highly cited publication according to total citations per year.
Keywords are well-suited to be selected for exploring research hotspots and trends as they are typically high-level summaries of the publication. In the current study, we identified 2495 authors’ keywords. In order to facilitate co-occurrence visualization, we extracted the top 50 keywords by occurrence frequency and calculated the PageRank score between them. As shown in Figure 8 and Supplementary Table 3, in the exclusion of search terms such as “diabetes” and “macrophage”, the keywords with higher occurrences were “inflammation” (277 times), “diabetic nephropathy” (123 times), “obesity” (114 times), and “insulin” (105 times). These authors’ keywords appeared more than 100 times, indicating their core position in this research field. In Figure 9, all closely related keywords were automatically grouped into four clusters and displayed in different colors. The red one, which was composed of “inflammation”, “metabolism”, “insulin” and “obesity”, was the most closely linked cluster. Among them, the term “inflammation” (PageRank: 0.183) almost intersected with all keywords that appeared in Figure 9, which undoubtedly represents it was the most exciting hotspot in this research field.
Figure 8 Word-cloud map of top 50 authors’ keywords ranked by frequency of occurrence in publications between 2000 and 2022. The font size represents the frequency of occurrence.
Figure 9 Co-occurrence network map of top 50 authors’ keywords ranked by frequency of occurrence in publications between 2000 and 2022. Nodes represent different keywords, colors represent clusters, and lines represent connections between them. Cluster 1: red (11 items); Cluster 2: blue (14 items); Cluster 3: green (13 items); Cluster 4: purple (12 items). None isolated nodes were detected.
After exploring the hotspots in MRDS, we made a further effort on the trend topic analysis based on keywords in combination with the evolution of time to reveal the current and promising forefront research topics. In our study, we identified the authors’ keywords from papers published from 2012 to 2022 that appear at least five times a year. Among them, a maximum of three themes were selected as annual topics. Precisely, 31 buzz topic terms of the year were calculated. The topic term at the top of Figure 10 was “exosome” which was followed by “macrophage polarization” and “epigenetics”, denoting the current motor topics with potential research prospects. While the annual topics at the bottom, such as “atherosclerosis”, “cytokine” and “apoptosis” have been studied in the past with relatively mature development. Therefore, these topics which were listed as research hotspots in Supplementary Table 3 and have not been calculated as forefront research topics.
Figure 10 Trend topics map of Author’s Keyword for publications published between 2012 and 2022. Data and visualization were generated from the Documents menu of the Bibliometrix R-package.
Keyword Plus® are terms frequently appearing in the titles of a publication’s references, automatically extracted by Web of Science via computer algorithms, and have been confirmed with more broadly descriptive (21). Hence, using Keyword Plus® to study the change in research trends from different periods is appropriate and objective. As shown in Figure 11, we performed a thematic evolution map to display the development of MRDS based on summarizing various evolutionary associations via Keyword Plus®. To better reflect the research focus and trends, we divided the collection of publications from the past two decades into five periods: 2000-2006, 2007-2011, 2012-2016, 2017-2020, and 2021-2022. Throughout these five periods, the term “expression” and “gene expression” have been the core theme of such research, while the term “inflammation” became an emerging theme in the third period (2012- 2016), and it is still a research topic in great demand up to now. In the last two years, the fifth period, four new themes have emerged, including “polarization”, “differentiation”, “adipose-tissue” and “dysfunction” which shows the evolution of themes in MRDS has been ongoing and continues to progress.
Figure 11 Thematic evolution map of Keyword Plus® in publications published between 2000 and 2022. Data and visualization were generated from the Conceptual Structure menu of the Bibliometrix R-package. Column 1 (2000-2006): expression, tumor necrosis factor, t cells, glycation end products, low density lipoprotein, gene expression; Column 2 (2007-2011): expression, insulin resistance, gene expression, glucose, apoptotic cells, cells; Column 3 (2012-2016): inflammation, expression, nf kappa b, glucose, gene expression; Column 4 (2017-2020): activation, inflammation, injury, gene expression, insulin resistance, expression; Column 5 (2021-2022): polarization, inflammation, injury, expression, differentiation gene expression, adipose tissue, dysfunction.
To our knowledge, this is the first bibliometric analysis to reveal the structural associations and temporal dynamics in macrophage research related to the diabetes field. Our objective was to conduct a comprehensive bibliometric analysis of the evolution of this field over the past two decades using the Bibliometrix R-package. Through visualized results, the quantitative data on authors, institutes, countries, sources, and keywords provided valuable insights into research hotspots and trends.
The annual publication analysis of 1272 papers published between 2000 and 2022 has shown a steady increase on the whole. After a long phase of knowledge accumulation from 2000 to 2011, identified as the beginning period, MRDS has entered a growth trend since 2012, especially in the past five years. The intense interest in macrophage function in influencing the process and treatment of diabetes reached its peak in 2021. Notwithstanding that our publication was conducted before the end of 2022, the fitting curves in Figure 2 indicated that this popular trend would continue. Macrophage studies were expected to become a future direction and offer enlightening insight into the general landscape of the diabetes domain.
Regarding research productivity by authors, Wu Yonggui, the most prolific scholar with the highest H-index (23 publications, H-index:12) from Anhui Medicine University, mainly contributed to how herbal extracts regulate the pathological process of diabetic nephropathy (DN) through macrophages (22). The geographical distribution of this field was obtained by exploring productive countries and institutes, which could reflect their degree of influence and research skills. Chinese scholars published the most papers (315 publications), followed by American scholars with 203 publications. This situation might be related to the fact that China has a sizeable population with diabetes, and thus extra emphasis is placed on diabetes-related studies at the bench and clinical (23). As expected, five of the top ten high-yield research institutes were located in the United States of America, and four in China, reflecting the core position of these two countries in this field.
Based on the PageRank algorithm, we visualized the cooperation and influence of authors, countries, and institutes, respectively. This newly emerged bibliometric methodology can more objectively reflect the weight of items through the co-occurrence times (20). The most robust academic collaboration was observed between China and the United States of America in Figure 5. Although Chinese scholars published the most papers on macrophage studies related to diabetes, the United States of America was located in the most central position in this field, which also illustrates, to some extent, that Chinese scholars should pay more attention to international academic collaboration with other countries to break down knowledge barriers. However, no apparent core authors and institutes were observed in Figure 3 and Figure 6 due to no evident difference in PageRank scores. It is worth noting that there still needs to be more in-depth cooperation and communication among these active scholars and institutes.
When evaluating the significance of a publication, it is common to consider the number of citations it receives. Highly-cited papers usually serve as the foundation and framework of the field. Our study enumerated the top ten highly cited papers according to total and annual citations, respectively. The top-cited paper, a full-length original research article ranked by total citations, mainly discussed how macrophage infiltration in white adipose tissue is associated with the metabolic complications of obesity (24). Interestingly, the top-ranked publication by the total citations per year also reviewed the association of inflammation, represented by macrophage infiltration, with obesity, metabolic syndrome, and diabetes (25). The link between inflammation and metabolic disease is a hot topic in macrophage research.
The decrease in insulin-stimulated glucose uptake, known as insulin resistance, is an essential antecedent pathophysiological feature and a significant underlying etiology of T2D (5). Several interrelated mechanisms have been proposed to explain this process, all of which are associated with inflammation or the induction of an inflammatory response. These mechanisms include glucotoxicity, lipotoxicity, oxidative stress, endoplasmic reticulum stress, etc. (2–4). Metabolic disorders, such as obesity and dyslipidemia, increase the risk of overt T2D and its progression through the activation immune system and secondary chronic low-grade inflammation (26). Hence, inflammation is the pathogenic clue that links obesity, insulin resistance, and T2D (27). This association can be observed in the red cluster of Figure 9, and the central position of inflammation in the macrophage research related to diabetes is also well illustrated in Figure 8.
Pro-inflammatory macrophages, as a primary effector cell type, play a role in this phenomenon by its recruitment, accumulation, and activation in the insulin target tissue, in particular adipose tissue, upregulating the production of adipose-derived pro-inflammatory cytokines (25, 28). Adipose tissue is regarded as a highly specialized tissue that exerts endocrine functions based on autocrine and paracrine activities. Instead of solely storing energy, it synthesizes and secretes adipokines (29). Moreover, Adipose tissue inflammation is an important event in obesity and T2D, negatively affecting the transmission of insulin signals, which is mainly attributed to the pro-inflammatory effect of adipose tissue macrophages. Macrophages account for approximately 10% of adipose tissue in lean mice, while in obese and leptin-deficient mice, this proportion increases to more than 50% (30, 31). Additional studies have shown that preventing macrophage accumulation in adipose tissue or pro-inflammatory macrophage signaling can improve glucose metabolism and alleviate insulin resistance in obese mice (32, 33). The major mechanistic evidence can be ascribed to the inflammatory modifications by the enhanced secretion of pro-inflammatory cytokine mediated by macrophages. Hotamisligil et al. found elevated gene expression levels of TNF-α in adipose tissue of insulin resistance and obese rodents (34). In the following years, macrophage-derived TNF-α was identified as the major source (35, 36). Higher TNF-α concentrations in plasma result from macrophage accumulation, which significantly affects the regulation of insulin sensitivity (29, 37).
Kidney inflammation is a critical factor in the pathogenesis of DN, which is characterized by excessive extracellular matrix deposition (38). In the context of DN, elevated glucose levels and metabolites contribute to cellular inflammation, glomerular sclerosis, and tubulointerstitial fibrosis (39). The role of macrophages in the pathogenesis of DN has attracted mounting attention. Specifically, the activation and infiltration of macrophages in this process play a crucial role in promoting renal inflammation and fibrosis in both glomeruli and the tubulointerstitium (40, 41). Macrophage infiltration has been confirmed to be responsible for multiple pathological changes, including renal interstitial proliferation and irreversible pathological changes in glomeruli, aggravating the development of DN (42–44). The NADPH oxidase Nox4 is an isoform of the catalytic subunit of NADPH oxidase and is primarily responsible for generating renal reactive oxygen species in rodent models of DN induced by streptozotocin. Removing Nox4 can reduce glomerular macrophage infiltration and provide renal protection against glomerular injury (45).
Additionally, it is worth mentioning that the green cluster in Figure 9 reflects metabolic disease augmentation on vascular disease mediated by macrophages and common mechanisms underlying atherosclerosis, diabetes, and obesity. As the primary cause of coronary artery disease, a common comorbidity of obesity and diabetes, atherosclerosis is a chronic inflammatory disease that has received considerable attention (46). Compared with purely inflammatory vascular disease individuals, macrophages from patients with coronary artery disease show significantly higher levels of glucose utilization and expression of pro-inflammatory cytokines, particularly TNF-α (47). Macrophage substrate metabolism is sensitive to extracellular glucose and lipid levels due to hyperglycemia further promotes lipid accumulation and lipotoxicity by increasing the expression of long-chain acyl CoA synthetase-1, and this glycemic condition could also lead to protein glycosylation and formation of advanced glycation end products producing massive pro-inflammatory cytokines (48, 49).
The classical view of the macrophage polarization model describes two opposite phenotypic states: pro-inflammatory M1-like macrophages, the classic phenotype, which can be triggered by bacterial lipopolysaccharide, interferon-γ, and secret several pro-inflammatory cytokines including TNF-α, IL-1β, IL-6. In contrast, M2-like macrophages, the alternative phenotype induced by IL-4 and IL-13, play a role in anti-inflammation by producing IL-10 (50, 51). Multiple stimulating factors determine the phenotype of macrophages, and new subtypes are also being discovered (31). Although it is too polarized to generalize different subtypes of macrophages through highly simplified phenotype dichotomy, we mainly mentioned macrophages as either M1 or M2 for clarity purposes in this work.
Epigenetics, a fast-growing research field, is currently defined as an alteration in gene function in response to external stimuli that modify gene expression with no change in DNA sequence via DNA methylation, histone modifications, and non-coding RNAs (51). Due to the remarkable plasticity of macrophages, M1/M2 polarization is a dynamic process that can be reversed under different pathophysiological conditions and is associated with epigenetic modification (52). The predominance of these phenotypically distinct macrophages at specific times plays different roles. Figure 10 and Figure 11 showed that macrophage polarization could be a breakthrough point in this field. Here we discuss the current understanding of macrophage polarization in diabetic complications.
Wound healing involves four classical phases: hemostasis, inflammation, proliferation, and remodeling. This complex and well-coordinated process is promoted by multiple factors, among which the convergence of macrophages at the wound site is a decisive step in the healing process (53, 54). Specifically, the early inflammatory phase is dominated by the M1 macrophages, which are responsible for events such as bacterial phagocytosis, tissue debris, and the release of cytokines (e.g., TNF-α, IL-1β, nitric oxide synthase, IL-6) (55). Over time, the M2-like macrophage subtype becomes dominant, triggering the proliferative phase. The function of M1-like macrophages transforming into anti-inflammatory phenotype plays a vital role during normal wound repairment (51). However, under the influence of diabetic circumstances, this transition is impeded, leading to excessive wound inflammation and problematic wound resolution (56–58). Diabetic wounds tend to experience a higher presence of M1 macrophages, which can lead to delayed healing (59). Gallagher Katherine A. from the University of Michigan, USA, who made outstanding contributions to diabetic wound repair in the past five years (Figure 4), reversed the inflammatory macrophage phenotype and alienated wound repair by inhibiting the cyclooxygenase 2/prostaglandin E2 pathway (60).
As mentioned, macrophages are regarded as an indispensable source of TNF-α. Increased TNF-α levels in the kidney have been well-validated in models of DN (61). To support this, recent evidence has pointed out that compared with control mice, conditional ablation of TNF-α in macrophages significantly reduced albuminuria, alleviated the increase of plasma creatinine and blood urea nitrogen, and improved histopathological changes (62). M1 is the primary macrophage type located at the site of diabetic renal injury, producing inflammatory cytokines, such as IL-1β and TNF-α, which are aggravated by hyperglycemia (10). More importantly, the number of M1 macrophages that infiltrated the kidneys of mice with diabetes could gradually increase over time (63). Evidence from experimental models of diabetes also has shown a positive correlation between increased M1 polarization and renal injury (64). However, M2 therapies would become profibrotic at some point because M2 macrophages could release high levels of transforming growth factor-β1 to enhance kidney fibrosis (65). Although the exact M2 repolarization phenotype remains to be further elucidated, inhibiting the activation of M1 macrophages and promoting the polarization of M2 macrophages have the potential to prevent podocyte damage, reduce proinflammatory cytokines, and reduce profibrotic proteins against DN (66–69).
Initiation and maintenance of atherosclerosis (AS) are well acknowledged as a chronic inflammatory state of the arterial wall in which several immune cells participate, and atherosclerotic plaque formation and progression are highly associated with the epigenetic regulation of macrophages, particularly macrophage-produced cytokines (70, 71). According to histological analysis of human atherosclerotic plaques, compared with M2 macrophages, M1 macrophages were most located in lipid-enrich areas. In vivo, the increase of M1 macrophages could accelerate the progression of AS contributing to necrotic core formation, plaque rupture, and coagulation cascade activation. In contrast, the rise in M2 macrophage polarization could suppress this process (72, 73).
Patients with diabetes have a high risk of complicating coronary artery disease, which leads to more diffuse AS under the influence of increased infiltration of inflammatory macrophages (74). Macrophages can be polarized toward the M1 phenotype within the atherosclerotic plaque in response to the exposition of various oxidized derivatives and growth factors. As a representative pathological toxic metabolite of diabetes, advanced glycation end products could trigger macrophages to polarize toward M1 by interacting with its receptors via RAGE/TLR4 signaling, leading to the inducement and aggravation of AS, and specifically inhibiting the activation of TLR4 signaling could effectively reduce plaque M1 macrophage infiltration and arterial stenosis (70, 75). Another key finding was that the overexpression of brain-derived neurotrophic factors (BDNF) could promote the M2 macrophage polarization to alleviate diabetes-related AS (76). As a critical regulator of the survival, differentiation, and growth of neurons, expression of BDNF is observed in activated macrophages and promotes the transformation of macrophages from M1 to M2 (77). In the acute phase of human coronary artery AS, the plasma level of BDNF decreases, which can also be observed in individuals with diabetes (78, 79). Taken together, it reflects diabetes augmentation on vascular disease mediated by macrophages. Promoting the polarization of M1 macrophages to M2 can be a breakthrough to better interpret and alleviate inflammatory diseases, including diabetes-related conditions.
Notably, exosomes, a type of cell vesicle with lipid bilayer membranes containing functional protein and nucleic acids, have been attractive in macrophage-related diabetes studies (Figure 9). As mentioned, macrophages are the predominant immune cells, and their polarization can mediate the inflammatory process. In this scenario, exosomes can further control the inflammatory conditions in various inflammatory diseases by regulating the differentiation of macrophages (80). Through this mechanism, exosomes which were originated from stem cells transferred into macrophages to induce the anti-inflammatory M2 macrophage polarization through the transactivation of arginase-1 with remarkably increased anti-inflammatory factors including IL-10, but no significant changes in those of M1-related cytokines which in turn evoked inflammatory responses and enhanced insulin sensitivity (81). The development of neuronal injury in rats could be accelerated by the miR−21−5p, carried by exosomes derived from dorsal root ganglia neurons, inducing microglia, which served as brain macrophage, differentiated into the proinflammatory M1 phenotype (82). Lv et al. found that high levels of miR-19b-3p positively correlated with the severity of tubulointerstitial inflammation in patients with DN and demonstrated that exosomal miR-19b-3p mediated the communication between injured tubular epithelial cells and macrophages, leading to M1 macrophage activation and further tubulointerstitial inflammation (83). In addition, exosomes secreted from human serum albumin−treated HK−2 cells released miR−199a−5p, which targeted the TLR4 pathway to induce M1 polarization, resulting in the progression of DN (84).
Admittedly, our work has some common limitations of bibliometric analysis. First, Boolean search terms (OR/AND) were performed to establish our bibliometric database by merging the publication information of diabetes and macrophage fields. However, this process would inevitably result in an amount of included literature that did not fit our research topic. To address this issue, we refined our search strategies and manually screened each publication record. Second, due to the limited number of publications included, co-citation analysis could not be conducted to obtain interpreted results. In addition, our research mainly focused on analyzing and mining quantitative metrics to describe the current state and temporal dynamics in MRDS systematically. However, qualitative analyses were not performed and the final results should be explained cautiously (85).
With the help of the bibliometrix R-package, a comprehensive bibliometric analysis was performed to visualize the temporal dynamics and research trends of the MRDS knowledge domain from 2000 to 2022. Overall, the annual quantity of publications has increased steadily. China, the University of Michigan, and the Diabetologia journal have the highest volume of publications compared to other countries, institutes, and journals. Our findings delineate the mainstream of MRDS mainly focused on inflammation and its related mechanisms. Macrophage polarization is currently the most cutting-edge topic. This pathophysiological mechanism has been explored in diabetes and its complications which has the potential as a fulcrum for future diabetes-related translational research. We aimed to provide valuable insights and clues for endocrinologists and academics to conduct further studies. Furthermore, the results of this study are also expected to strengthen investment policies in future macrophage-related diabetes research.
The original contributions presented in the study are included in the article/Supplementary Material. Further inquiries can be directed to the corresponding authors.
LZhao and BZ designed and supervised the study. SW and LZhang wrote the first draft of the manuscript. ZJ and YW extracted the data, performed analyses, and interpreted the data. LZhao and BZ contributed to the polishing and revising the manuscript and resolved all discrepancies. All authors contributed to the article and approved the submitted version.
This research was funded by the National Natural Science Foundation of China (82274343); Beijing Natural Science Foundation (7212189); the Scientific and Technological Innovation Project of China Academy of Chinese Medical Sciences (CI2021A01605); the Innovation Team and Talents Cultivation Program of National Administration of Traditional Chinese Medicine (ZYYCXTD-D-202001); the Clinical Research Center Construction Project of Guang’anmen Hospital, CACMS (2022LYJSZX28); and the China Postdoctoral Science Foundation (2021M693542).
The authors declare that the research was conducted in the absence of any commercial or financial relationships that could be construed as a potential conflict of interest.
All claims expressed in this article are solely those of the authors and do not necessarily represent those of their affiliated organizations, or those of the publisher, the editors and the reviewers. Any product that may be evaluated in this article, or claim that may be made by its manufacturer, is not guaranteed or endorsed by the publisher.
The Supplementary Material for this article can be found online at: https://www.frontiersin.org/articles/10.3389/fimmu.2023.1194738/full#supplementary-material
1. International Diabetes Federation. IDF diabetes atlas. 10th ed. Brussels: International Diabetes Federation (2021).
2. Donath MY, Schumann DM, Faulenbach M, Ellingsgaard H, Perren A, Ehses JA. Islet inflammation in type 2 diabetes from metabolic stress to therapy. Diabetes Care (2008) 31(Supplement 2):S161–4. doi: 10.2337/dc08-s243
3. Hotamisligil GS, Erbay E. Nutrient sensing and inflammation in metabolic diseases. Nat Rev Immunol (2008) 8(12):923–34. doi: 10.1038/nri2449
4. Robertson RP, Harmon J, Tran POT, Poitout V. beta-cell glucose toxicity, lipotoxicity, and chronic oxidative stress in type 2 diabetes. Diabetes (2004) 53(Supplement 1):S119–24. doi: 10.2337/diabetes.53.2007.S119
5. Donath M, Shoelson S. Type 2 diabetes as an inflammatory disease. Nat Rev Immunol (2011) 11(2):98–107. doi: 10.1038/nri2925
6. Wang X, Bao W, Liu J, OuYang YY, Wang D, Rong S, et al. Inflammatory markers and risk of type 2 diabetes a systematic review and meta-analysis. Diabetes Care (2013) 36(1):166–75. doi: 10.2337/dc12-0702
7. Baumann B, Salem H, Boehm B. Anti-inflammatory therapy in type 1 diabetes. Curr Diabetes Rep (2012) 12(5):499–509. doi: 10.1007/s11892-012-0299-y
8. Sanchez-Zamora Y, Rodriguez-Sosa M. The role of MIF in type 1 and type 2 diabetes mellitus. J Diabetes Res (2014) 2014:804519. doi: 10.1155/2014/804519
9. Hamidzadeh K, Christensen SM, Dalby E, Chandrasekaran P, Mosser DM. Macrophages and the recovery from acute and chronic inflammation. Annu Rev Physiol (2017) 79:567–92. doi: 10.1146/annurev-physiol-022516-034348
10. Ma TK, Li X, Zhu YH, Yu SF, Liu TY, Zhang XD, et al. Excessive activation of notch signaling in macrophages promote kidney inflammation, fibrosis, and necroptosis. Front Immunol (2022) 13:835879. doi: 10.3389/fimmu.2022.835879
11. Parisi L, Gini E, Baci D, Tremolati M, Fanuli M, Bassani B, et al. Macrophage polarization in chronic inflammatory diseases: killers or builders. J Immunol Res (2018) 2018:8917804. doi: 10.1155/2018/8917804
12. Kokol P, Vošner HB, Završnik J. Application of bibliometrics in medicine: a historical bibliometrics analysis. Health Inf Libraries J (2020) 38(2):125–38. doi: 10.1111/hir.12295
13. Chen C. Science Mapping: a systematic review of the literature. J Data Inf Sci (2017) 2(2):1–40. doi: 10.1515/jdis-2017-0006
14. Aria M, Cuccurullo C. Bibliometrix: an R-tool for comprehensive science mapping analysis. J Informetrics (2022) 11(4):959–75. doi: 10.1016/j.joi.2017.08.007
15. Birkle C, Pendlebury DA, Schnell J, Adams J. Web of Science as a data source for research on scientific and scholarly activity. Quantitative Sci Stud (2021) 1(1):363–76. doi: 10.1162/qss_a_00018
16. Ho Y. Comments on Research trends of macrophage polarization: a bibliometric analysis. Chin Med J (2021) 132(22):2772. doi: 10.1097/CM9.0000000000000499
17. Bertoli-Barsotti L, Lando T. A theoretical model of the relationship between the h-index and other simple citation indicators. Scientometrics (2017) 111(3):1415–48. doi: 10.1007/s11192-017-2351-9
18. Ma N, Guan J, Zhao Y. Bringing PageRank to the citation analysis. Inf Process Manage (2008) 44:800–10. doi: 10.1016/j.ipm.2007.06.006
19. Zhang P, Cheng R. Interdisciplinary influences in headache literature: a network citation analysis of PubMed Central articles. Headache (2021) 61:143–8. doi: 10.1111/head.14022
20. Wang SC, Tian CX, Gao ZZ, Zhang BX, Zhao LH. Research status and trends of the diabetic cardiomyopathy in the past 10 years (2012-2021): A bibliometric analysis. Front Cardiovasc Med (2022) 9:1018841. doi: 10.3389/fcvm.2022.1018841
21. Zhang J, Yu Q, Zheng FS, Long C, Lu ZX, Duan ZG. Comparing keywords plus of WOS and author keywords: A case study of patient adherence research. J Assoc Inf Sci Technol (2016) 67(4):967–72. doi: 10.1002/asi.23437
22. Zhang W, Zhao L, Su SQ, Xu XX, Wu YG. Total glucosides of paeony attenuate renal tubulointerstitial injury in STZ-induced diabetic rats: role of toll-like receptor 2. J Pharmacol Sci (2014) 125(1):59–67. doi: 10.1254/jphs.13173FP
23. Ma R. Epidemiology of diabetes and diabetic complications in China. Diabetologia (2018) 61(6):1249–60. doi: 10.1007/s00125-018-4557-7
24. Cinti S, Mitchell G, Barbatelli G, Murano I, Ceresi E, Faloia E, et al. Adipocyte death defines macrophage localization and function in adipose tissue of obese mice and humans. J Lipid Res (2005) 46(11):2347–55. doi: 10.1194/jlr.M500294-JLR200
25. Esser N, Legrand-Poels S, Piette J, Scheen AJ, Paquot N. Inflammation as a link between obesity, metabolic syndrome and type 2 diabetes. Diabetes Res Clin Pract (2014) 105(2):141–50. doi: 10.1016/j.diabres.2014.04.006
26. Ouchi N, Parker JL, Lugus JJ, Walsh K. Adipokines in inflammation and metabolic disease. Nat Rev Immunol (2011) 11(2):85–97. doi: 10.1038/nri2921
27. Rohm TV, Meier DT, Olefsky JM, Donath MY. Inflammation in obesity, diabetes, and related disorders. Immunity (2021) 55(1):31–55. doi: 10.1016/j.immuni.2021.12.013
28. Ying W, Fu WX, Lee YS, Olefsky JM. The role of macrophages in obesity-associated islet inflammation and beta-cell abnormalities. Nat Rev Endocrinol (2011) 16(2):81–90. doi: 10.1038/s41574-019-0286-3
29. Kojta I, Chacinska M, Blachnio-Zabielska A. Obesity, bioactive lipids, and adipose tissue inflammation in insulin resistance. Nutrients (2020) 12(5):1305. doi: 10.3390/nu12051305
30. Wellen KE, Hotamisligil GS. Obesity-induced inflammatory changes in adipose tissue. J Clin Invest (2003) 112(12):1785–8. doi: 10.1172/JCI200320514
31. Li H, Meng Y, He SW, Tan XC, Zhang YJ, Zhang XL, et al. Macrophages, chronic inflammation, and insulin resistance. Cells (2022) 11(19):3001. doi: 10.3390/cells11193001
32. Desai HR, Sivasubramaniyam T, Revelo XS, Schroer SA, Luk CT, Rikkala PR, et al. Macrophage JAK2 deficiency protects against high-fat diet induced inflammation. Sci Rep (2017) 7:7653. doi: 10.1038/s41598-017-07923-0
33. Lee YS, Olefsky J. Chronic tissue inflammation and metabolic disease. Genes Dev (2021) 35(5-6):307–28. doi: 10.1101/gad.346312
34. Hotamisligil G, Shargill N, Spiegelman B. Adipose expression of tumor necrosis factor-alpha: Direct role in obesity-linked insulin resistance. Science (1993) 259(5091):87–91. doi: 10.1126/science.7678183
35. Weisberg SP, McCann D, Desai M, Rosenbaum M, Leibel RL, Ferrante AW. Obesity is associated with macrophage accumulation in adipose tissue. J Clin Invest (2003) 112(12):1796–808. doi: 10.1172/JCI200319246
36. De Taeye BM, Novitskaya T, McGuinness OP, Gleaves L, Medda M, Covington JW. Macrophage TNF-alpha contributes to insulin resistance and hepatic steatosis in diet-induced obesity. Am J Physiology-Endocrinology Metab (2007) 293(3):E713–25. doi: 10.1152/ajpendo.00194.2007
37. Hotamisligil GS, Spiegelman BM. Tumor necrosis factor alpha: a key component of the obesity-diabetes link. Diabetes (1994) 43(11):1271–8. doi: 10.2337/diabetes.43.11.1271
38. Najafian B, Alpers EA, Fogo AB. Pathology of human diabetic nephropathy. Contributions to Nephrol (2011) 170:36–47. doi: 10.1159/000324942
39. Barrera-Chimal J, Jaisser F. Pathophysiologic mechanisms in diabetic kidney disease: A focus on current and future therapeutic targets. Diabetes Obes Metab (2020) Suppl 1:16–31. doi: 10.1111/dom.13969
40. Tang PMK, Nikolic-Paterson DJ, Lan HY. Macrophages: versatile players in renal inflammation and fibrosis. Nat Rev Nephrol (2019) 15(3):144–58. doi: 10.1038/s41581-019-0110-2
41. Li HD, You YK, Shao BY, Wu WF, Wang YF, Guo JB, et al. Roles and crosstalks of macrophages in diabetic nephropathy. Front Immunol (2022) 13:1015142. doi: 10.3389/fimmu.2022.1015142
42. Yang Y, Feng XJ, Liu XY, Wang Y, Hu M, Cao Q, et al. alteration of bone marrow-derived macrophages ameliorates kidney fibrosis in murine model of unilateral ureteral obstruction. Nephrol Dialysis Transplant (2019) 34(10):1657–68. doi: 10.1093/ndt/gfy381
43. Chow FY, Nikolic-Paterson DJ, Ozols E, Atkins RC, Rollin BJ, Tesch GH. Monocyte chemoattractant protein-1 promotes the development of diabetic renal injury in streptozotocin-treated mice. Kidney Int (2006) 69(1):73–80. doi: 10.1038/sj.ki.5000014
44. Nguyen D, Ping F, Mu W, Hill P, Atkins RC, Chadban SJ. Macrophage accumulation in human progressive diabetic nephropathy. Nephrology (2006) 11(3):226–31. doi: 10.1111/j.1440-1797.2006.00576.x
45. Jha JC, Gray SP, Barit D, Okabe J, El-Osta A, Namikoshi T. Genetic Targeting or Pharmacologic Inhibition of NADPH Oxidase Nox4 Provides Renoprotection in Long-Term Diabetic Nephropathy. J Am Soc Nephrol (2014) 25(6):1237–54. doi: 10.1681/ASN.2013070810
46. Libby P, Okamoto Y, Rocha VZ, Folco E. Inflammation in atherosclerosis: Transition from theory to practice. Circ J (2003) 74(2):213–20. doi: 10.1253/circj.CJ-09-0706
47. Watanabe R, Hilhorst M, Zhang H, Zeisbrich M, Berry GJ, Wallis BB, et al. Glucose metabolism controls disease-specific signatures of macrophage effector functions. JCI Insight (2003) 3(20):e123047. doi: 10.1172/jci.insight.123047
48. Mishra P, Ying W, Nandi SS, Bandyopadhyay G, Patel K, Mahata S. Diabetic cardiomyopathy: an immunometabolic perspective. Front Endocrinol (2017) 8:72. doi: 10.3389/fendo.2017.00072
49. Mouton AJ, Li X, Hall ME, Hall JE. Obesity, hypertension, and cardiac dysfunction novel roles of immunometabolism in macrophage activation and inflammation. Circ Res (2020) 126(6):789–806. doi: 10.1161/CIRCRESAHA.119.312321
50. Kuznetsova T, Prange KHM, Glass CK, de Winther MPJ. Transcriptional and epigenetic regulation of macrophages in atherosclerosis. Nat Rev Cardiol (2020) 17(4):216–28. doi: 10.1038/s41569-019-0265-3
51. Davis FM, Gallagher KA. Epigenetic Mechanisms in Monocytes/Macrophages Regulate Inflammation in Cardiometabolic and Vascular Disease. Arterioscler Thromb Vasc Biol (2019) 39(4):623–34. doi: 10.1161/ATVBAHA.118.312135
52. Saccani A, Schioppa T, Porta C, Biswas SK, Nebuloni M, Vago L, et al. P50 nuclear factor-kappa B overexpression in tumor-associated macrophages inhibits M1 inflammatory responses and antitumor resistance. Cancer Res (2006) 66(23):11432–40. doi: 10.1158/0008-5472.CAN-06-1867
53. Wynn T, Vannella K. Macrophages in Tissue Repair, Regeneration, and Fibrosis. Immunity (2016) 44(3):450–62. doi: 10.1016/j.immuni.2016.02.015
54. Maruyama K, Asai J, Li M, Thorne T, Losordo DW, D'Amore PA. Decreased macrophage number and activation lead to reduced lymphatic vessel formation and contribute to impaired diabetic wound healing. Am J Pathol (2007) 170(4):1178–91. doi: 10.2353/ajpath.2007.060018
55. Willenborg S, Lucas T, van Loo G, Knipper JA, Krieg T, Haase I, et al. CCR2 recruits an inflammatory macrophage subpopulation critical for angiogenesis in tissue repair. Blood (2012) 120(3):613–25. doi: 10.1182/blood-2012-01-403386
56. Boniakowski AE, Kimball AS, Jacobs BN, Kunkel SL, Gallagher KA. Macrophage-Mediated Inflammation in Normal and Diabetic Wound Healing. J Immunol (2017) 199(1):17–24. doi: 10.4049/jimmunol.1700223
57. Murray PJ, Allen JE, Biswas SK, Fisher EA, Gilroy DW, Goerdt S, et al. Macrophage activation and polarization: nomenclature and experimental guidelines. Immunity (2014) 41(1):14–20. doi: 10.1016/j.immuni.2014.06.008
58. Den Dekker A, Davis FM, Kunkel SL, Gallagher KA. Targeting epigenetic mechanisms in diabetic wound healing. Trans Res (2019) 204:39–50. doi: 10.1016/j.trsl.2018.10.001
59. Kimball A, Schaller M, Joshi A, Davis FM, denDekker A, Boniakowski A, et al. Ly6C(Hi) Blood Monocyte/Macrophage Drive Chronic Inflammation and Impair Wound Healing in Diabetes Mellitus. Arterioscler Thromb Vasc Biol (2018) 38(5):1102–14. doi: 10.1161/ATVBAHA.118.310703
60. Davis FM, Tsoi LC, Wasikowski R, denDekker A, Joshi A, Wilke C, et al. Epigenetic regulation of the PGE(2) pathway modulates macrophage phenotype in normal and pathologic wound repair. JCI Insight (2020) 51(7):e138443. doi: 10.1172/jci.insight.138443
61. Kalantarinia K, Awad A, Siragy H. Urinary and renal interstitial concentrations of TNF-alpha increase prior to the rise in albuminuria in diabetic rats. Kidney Int (2003) 64(4):1208–13. doi: 10.1046/j.1523-1755.2003.00237.x
62. Awad AS, You HN, Gao T, Cooper TK, Nedospasov SA, Vacher J, et al. Macrophage-derived tumor necrosis factor-alpha mediates diabetic renal injury. Kidney Int (2015) 88(4):722–33. doi: 10.1038/ki.2015.162
63. Fu J, Sun ZG, Wang X, Zhang T, Yuan WJ, Salem F, et al. The single-cell landscape of kidney immune cells reveals transcriptional heterogeneity in early diabetic kidney disease. Kidney Int (2022) 106(6):1291–304. doi: 10.1016/j.kint.2022.08.026
64. Wang X, Yao B, Wang YQ, Fan XF, Wang SW, Niu AL, et al. Macrophage cyclooxygenase-2 protects against development of diabetic nephropathy. Diabetes (2017) 66(2):494–504. doi: 10.2337/db16-0773
65. Pan BX, Liu GH, Jiang ZP, Zheng DW. Regulation of Renal Fibrosis by Macrophage Polarization. Cell Physiol Biochem (2015) 35(3):1062–9. doi: 10.1159/000373932
66. Mohamed R, Jayakumar C, Chen F, Fulton D, Stepp D, Gansevoort RT, et al. Low-dose IL-17 therapy prevents and reverses diabetic nephropathy, metabolic syndrome, and associated organ fibrosis. J Am Soc Nephrol (2022) 27(3):745–65. doi: 10.1681/ASN.2014111136
67. Ndisang J, Jadhav A. Hemin therapy improves kidney function in male streptozotocin-induced diabetic rats: Role of the heme oxygenase/atrial natriuretic peptide/adiponectin axis. Endocrinology (2014) 155(1):215–29. doi: 10.1210/en.2013-1050
68. Ji LQ, Chen YF, Wang HQ, Zhang W, He LX, Wu JM, et al. Overexpression of Sirt6 promotes M2 macrophage transformation, alleviating renal injury in diabetic nephropathy. Int J Oncol (2019) 55(1):103–15. doi: 10.3892/ijo.2019.4800
69. Calle P, Hotter G. Macrophage phenotype and fibrosis in diabetic nephropathy. Int J Mol Sci (2020) 21(8):2806. doi: 10.3390/ijms21082806
70. Xing YJ, Pan S, Zhu L, Cui QW, Tang ZG, Liu ZW, et al. Advanced glycation end products induce atherosclerosis via RAGE/TLR4 signaling mediated-M1 macrophage polarization-dependent vascular smooth muscle cell phenotypic conversion. Oxid Med Cell Longevity (2022) 2022:9763377. doi: 10.1155/2022/9763377
71. Ross R. Mechanisms of disease- Atherosclerosis- an inflammatory disease. New Engl J Med (1999) 340(2):115–26. doi: 10.1056/NEJM199901143400207
72. Moore KJ, Sheedy FJ, Fisher EA. Macrophages in atherosclerosis: a dynamic balance. Nat Rev Immunol (2013) 13(10):709–21. doi: 10.1038/nri3520
73. Tabas I, Bornfeldt KE. Macrophage phenotype and function in different stages of atherosclerosis. Circ Res (2022) 108(8):985–95. doi: 10.1161/CIRCRESAHA.115.306256
74. Yahagi K, Kolodgie FD, Lutter C, Mori H, Romero ME, Finn AV, et al. Pathology of human coronary and carotid artery atherosclerosis and vascular calcification in diabetes mellitus. Arterioscler Thromb Vasc Biol (2017) 37(2):191–204. doi: 10.1161/ATVBAHA.116.306256
75. Liu ZW, Ma YP, Cui QW, Xu J, Tang ZG, Wang Y, et al. Toll-like receptor 4 plays a key role in advanced glycation end products- induced M1 macrophage polarization. Biochem Biophys Res Commun (2020) 531(4):602–8. doi: 10.1016/j.bbrc.2020.08.014
76. Bi CL, Fu YL, Zhang ZQ, Li B. Prostaglandin E2 confers protection against diabetic coronary atherosclerosis by stimulating M2 macrophage polarization via the activation of the CREB/BDNF/TrkB signaling pathway. FASEB J (2020) 34(6):7360–71. doi: 10.1096/fj.201902055R
77. Hong JH, Park HM, Byun KH, Lee BH, Kang WC, Jeong GB. BDNF expression of macrophages and angiogenesis after myocardial infarction. Int J Cardiol (2014) 176(3):1405–8. doi: 10.1016/j.ijcard.2014.08.019
78. Krabbe KS, Nielsen AR, Krogh-Madsen R, Plomgaard P, Rasmussen P, Erikstrup C, et al. Brain-derived neurotrophic factor (BDNF) and type 2 diabetes,”. Diabetologia (2007) 50(2):431–8. doi: 10.1007/s00125-006-0537-4
79. Manni L, Nikolova V, Vyagova D, Chaldakov GN, Aloe L. Reduced plasma levels of NGF and BDNF in patients with acute coronary syndromes. Int J Cardiol (2005) 102(1):169–71. doi: 10.1016/j.ijcard.2004.10.041
80. Console L, Scalise M, Indiveri C. Exosomes in inflammation and role as biomarkers. Clinica Chimica Acta (2019) 488:165–71. doi: 10.1016/j.cca.2018.11.009
81. Zhao H, Shang QW, Pan ZZ, Bai Y, Li ZQ, Zhang HY, et al. Exosomes from adipose-derived stem cells attenuate adipose inflammation and obesity through polarizing M2 macrophages and beiging in white adipose tissue. Diabetes (2018) 67(2):235–47. doi: 10.2337/db17-0356
82. Yin ZY, Han ZL, Hu TP, Zhang SS, Ge XT, Huang S, et al. Neuron-derived exosomes with high miR-21-5p expression promoted polarization of M1 microglia in culture. Brain Behav Immun (2020) 83:270–82. doi: 10.1016/j.bbi.2019.11.004
83. Lv LL, Feng Y, Wu M, Wang B, Li ZL, Zhong X, et al. Exosomal miRNA-19b-3p of tubular epithelial cells promotes M1 macrophage activation in kidney injury. Cell Death Differentiation (2018) 27(1):210–26. doi: 10.1038/s41418-019-0349-y
84. Jia YJ, Zheng ZJ, Xue M, Zhang ST, Hu F, Li Y, et al. Extracellular vesicles from albumin−induced tubular epithelial cells promote the M1 macrophage phenotype by targeting klotho. Mol Ther (2019) 27(8):1452–66. doi: 10.1016/j.ymthe.2019.05.019
Keywords: macrophage, diabetes mellitus, macrophage polarization, bibliometric analysis, visualization
Citation: Wang S, Zhang L, Jin Z, Wang Y, Zhang B and Zhao L (2023) Visualizing temporal dynamics and research trends of macrophage-related diabetes studies between 2000 and 2022: a bibliometric analysis. Front. Immunol. 14:1194738. doi: 10.3389/fimmu.2023.1194738
Received: 27 March 2023; Accepted: 10 July 2023;
Published: 26 July 2023.
Edited by:
Xiangwei Xiao, University of Pittsburgh, United StatesReviewed by:
Ioanna Galani, Biomedical Research Foundation of the Academy of Athens (BRFAA), GreeceCopyright © 2023 Wang, Zhang, Jin, Wang, Zhang and Zhao. This is an open-access article distributed under the terms of the Creative Commons Attribution License (CC BY). The use, distribution or reproduction in other forums is permitted, provided the original author(s) and the copyright owner(s) are credited and that the original publication in this journal is cited, in accordance with accepted academic practice. No use, distribution or reproduction is permitted which does not comply with these terms.
*Correspondence: Boxun Zhang, MTI0Mzg3NjU2MEBxcS5jb20=; Linhua Zhao, bWVsb256aGFvQDE2My5jb20=
†These authors have contributed equally to this work
Disclaimer: All claims expressed in this article are solely those of the authors and do not necessarily represent those of their affiliated organizations, or those of the publisher, the editors and the reviewers. Any product that may be evaluated in this article or claim that may be made by its manufacturer is not guaranteed or endorsed by the publisher.
Research integrity at Frontiers
Learn more about the work of our research integrity team to safeguard the quality of each article we publish.