- 1School of Medicine, Shanghai University, Shanghai, China
- 2Department of Orthopaedics, Shanghai Key Laboratory for Prevention and Treatment of Bone and Joint Diseases, Shanghai Institute of Traumatology and Orthopaedics, Ruijin Hospital, Shanghai Jiao Tong University School of Medicine, Shanghai, China
- 3Department of Orthopedics, BenQ Medical Center, The Affiliated BenQ Hospital of Nanjing Medical University, Nanjing, China
- 4Department of Orthopaedics, Shanghai Changzheng Hospital, Shanghai, China
- 5Department of Neurosurgery, Ruijin Hospital, Shanghai Jiaotong University School of Medicine, Shanghai, China
Background: Intervertebral disc degeneration (IDD) is one of the most common health problems in the elderly and a major causative factor in low back pain (LBP). An increasing number of studies have shown that IDD is closely associated with autophagy and immune dysregulation. Therefore, the aim of this study was to identify autophagy-related biomarkers and gene regulatory networks in IDD and potential therapeutic targets.
Methods: We obtained the gene expression profiles of IDD by downloading the datasets GSE176205 and GSE167931 from the Gene Expression Omnibus (GEO) public database. Subsequently, differentially expressed genes (DEGs) analysis, Kyoto Encyclopedia of Genes and Genomes (KEGG) analysis, gene ontology (GO), and gene set enrichment analysis (GSEA) were performed to explore the biological functions of DEGs. Differentially expressed autophagy-related genes (DE-ARGs) were then crossed with the autophagy gene database. The hub genes were screened using the DE-ARGs protein–protein interaction (PPI) network. The correlation between the hub genes and immune infiltration and the construction of the gene regulatory network of the hub genes were confirmed. Finally, quantitative PCR (qPCR) was used to validate the correlation of hub genes in a rat IDD model.
Results: We obtained 636 DEGs enriched in the autophagy pathway. Our analysis revealed 30 DE-ARGs, of which six hub genes (MAPK8, CTSB, PRKCD, SNCA, CAPN1, and EGFR) were identified using the MCODE plugin. Immune cell infiltration analysis revealed that there was an increased proportion of CD8+ T cells and M0 macrophages in IDD, whereas CD4+ memory T cells, neutrophils, resting dendritic cells, follicular helper T cells, and monocytes were much less abundant. Subsequently, the competitive endogenous RNA (ceRNA) network was constructed using 15 long non-coding RNAs (lncRNAs) and 21 microRNAs (miRNAs). In quantitative PCR (qPCR) validation, two hub genes, MAPK8 and CAPN1, were shown to be consistent with the bioinformatic analysis results.
Conclusion: Our study identified MAPK8 and CAPN1 as key biomarkers of IDD. These key hub genes may be potential therapeutic targets for IDD.
Introduction
Intervertebral disc degeneration (IDD) is a major global public health problem (1) that is frequently observed in middle-aged and elderly people (2) and is the pathological basis of a number of degenerative spinal conditions and diseases, such as low back pain (LBP) (3), disc herniation (4), and cervical spondylosis (5). IDD is a degenerative musculoskeletal disease associated with multiple factors and has a complex and multifaceted pathogenesis that mainly includes excessive mechanical stress (6), excessive apoptosis of nucleus pulposus (NP) cells (7), abnormal degradation of the extracellular matrix (ECM) (8), inflammatory response (9), autophagy disorders (10), oxidative stress injury (11), and genetics (12). The current therapies for symptom management and pain control cannot heal the injured disc or prevent the progression of IDD (13, 14). The primary concern of our study was identifying what molecules are involved and what biological functions are related to the process of IDD, as potential IDD therapeutic targets.
Autophagy is the biological process for the self-degradation and recycling of cellular components; it relies on lysosomes to clear redundant protein polymers and damaged organelles, such as mitochondria and peroxisomes (15–18). Many diseases, notably cancer, diabetes, heart disease, and muscle disease, are related to autophagy disruption (10, 19, 20). An increasing number of studies have shown that there is a close relationship between autophagy and IDD, and it has been reported that autophagy-related apoptosis promotes the progression of IDD (10). In addition, there is a significant correlation between the progression of IDD and immune cell infiltration (21). Moreover, in recent years, particular attention has been given to non-coding RNAs, including microRNAs (miRNAs) and long non-coding RNAs (lncRNAs), which revealed that they play significant roles in the initiation and progression of IDD (22, 23).
Although many studies have provided preliminary evidence for the regulatory role that autophagy dysregulation plays in the development of IDD, most studies have focused only on the biological functions performed by specific genes and have not focused on their integration with the altered immune microenvironment within the intervertebral disc and the regulation of target gene expression by non-coding RNAs. Based on a high-throughput sequencing dataset of IDD patients obtained from public databases, we performed bioinformatics analysis to identify the hub genes associated with immune infiltration and autophagy dysfunction in the development of IDD and performed competing endogenous RNA (ceRNA) network construction for the hub genes. Finally, PCR validation confirmed the relevance of MAPK8 and CAPN1 in the IDD pathogenesis.
Material and methods
Data source and processing
In this study, three IDD-related high-throughput RNA sequencing datasets were obtained from the Gene Expression Omnibus (GEO; https://www.ncbi.nlm.nih.gov/geo/) database, that is, GSE176205, GSE167931, and GSE167199. The detailed contents of three series are listed in Supplementary Table S1. Both the GSE176205 (containing three controls and six IDD samples) and GSE167931 (containing four controls and five IDD samples) datasets were applied for differentially expressed genes (DEGs) analysis and normalized using the normalizeBetweenArrays function via the limma R package. The two preprocessed datasets mentioned above were combined, and batch effects were removed using the sva R package with the ComBat function. After obtaining the combined dataset, the DEGs of the control and IDD samples were analyzed using the limma R package, with |log2FC| > 1.5 and p < 0.05 being used as screening thresholds. The GSE167199 dataset contained three controls and three IDD samples that were applied for microRNAs and lncRNA analysis. The GENCODE database was used for lncRNA annotation. Figure 1 shows the workflow of this study.
Functional enrichment analysis of DEGs
The DAVID database (https://david.ncifcrf.gov/) was used to identify biological terms that were enriched with DEGs, filtering out statistically significant gene ontology (GO) terms and Kyoto Encyclopedia of Genes and Genomes (KEGG) pathways at a threshold of p < 0.05. All results were plotted using the ggplot2 R package.
Gene set enrichment analysis
Gene set enrichment analysis (GSEA) of DEGs obtained from the differential analysis was performed to explore the potential biological processes of DEGs involved in IDD. The hallmark gene set was obtained from the MSigDB database, and GSEA analysis was performed using the ClusterProfiler R package (24). The gene sets of the DEGs were sorted according to log2FC, and the normalized enrichment score (NES) in GSEA was obtained. | NES | > 1 and p < 0.05 were considered to indicate significantly enriched pathways.
Identification and functional enrichment of differentially expressed autophagy-related genes
We selected C5 ontology gene sets in the MsigDB database as the filtering criteria, and the 404 genes from the 11 gene sets that are related to autophagy were obtained. In addition, 232 genes involved in autophagy were obtained from the Human Autophagy database (http://www.autophagy.lu/index.html). The obtained gene sets were combined to create a gene set containing 547 ARGs. The intersection of ARGs and DEGs was used to obtain the differentially expressed autophagy-related genes (DE-ARGs). KEGG and GO analyses of DE-ARGs were performed using the DAVID database, with p < 0.05 as the threshold.
Construction of protein–protein interaction network
The Search Tool for the Retrieval of Interacting Genes/proteins (STRING; https://string-db.org/) database contains both the known and predicted protein interactions; these were used to construct the PPI network. The DE-ARGs list was imported into the STRING online platform, and PPI analysis was performed with the default settings and imported into Cytoscape 3.9.1 software. The hub PPI network was screened and visualized using the MCODE analysis plugin. Genes from the hub PPI network were treated as hub genes for subsequent analysis. Receiver operating characteristic (ROC) curve analysis was used to evaluate the diagnostic performance of the hub genes. ROC analysis was carried out using the pROC package in R.
Immune cell infiltration analysis
The CIBERSORT algorithm, which is used to evaluate changes in immune cells during immune infiltration, was used in this study to investigate changes in the relative proportion of immune cell infiltration in IDD. We performed a correlation analysis of immune cell infiltration and hub gene expression levels using the psych package in R.
Construction of ceRNA network
We utilized the following procedures to construct the ceRNA network: (1) differential analysis of GSE167199 with the limma package in the R environment using | log2FC | > 1 and p < 0.05 as screening thresholds to obtain differentially expressed microRNAs (DE-miRNAs) and differentially expressed lncRNAs (DE-lncRNAs); (2) using the ENCORI database (https://rna.sysu.edu.cn/encori/index.php) to predict the target miRNAs of the hub gene that intersect with DE-miRNAs to construct the final mRNA–miRNA axis; (3) using mirNet (https://www.mirnet.ca/) to predict the lncRNAs that would be targeted by the DE-miRNAs that bind to the hub gene [the predicted lncRNAs were then taken to intersect with the DE-lncRNAs from (1) to construct the miRNA–lncRNA axis]; and (4) combining the multiple mRNA–miRNA and miRNA–lncRNA axes obtained from the above steps into a mRNA–miRNA–lncRNA regulatory axis according to the predicted binding trends. In addition, the ceRNA network was visualized using Cytoscape software.
IDD rat models
Twenty-four 8-week-old adult male Sprague Dawley rats were used in this study. The rats were anesthetized by intraperitoneal injection with 2% (w/v) pentobarbital (40 mg/kg) and randomly assigned to either the control group or the IDD group, with an equal number assigned to each group. The caudal discs C7/8 were identified and selected for the study according to the methods used in the previous study (25). A 27-gauge needle punctured discs C7/8, crossing the nucleus pulposus to the contralateral annulus fibrosus. After complete penetration, the needle was rotated twice 360° and held for 60 s. The resistance of the contralateral annulus fibrosus controlled the depth of needle penetration.
X-rays and MRI
After we anesthetized the rats with isoflurane (3% for induction and 1% for maintenance), we placed them in an induction box in which the air flow rate was adjusted to 1 L/min. Once the rats were fully anesthetized, we removed them from the induction box and placed respiratory masks on them, through which they inhaled isoflurane gas at an adjusted concentration of 1%. The rats were placed in prone positions under the X-ray equipment (Toshiba China, E7252) and in a 7.0 T small-animal MRI system (CG NOVILA 7.0 T, Chenuang, China), and images were collected.
Hematoxylin and eosin staining
After 4 weeks of feeding, all rats were euthanized, and their caudal intervertebral discs were collected. Half of the samples from each group were fixed in 10% buffered formalin for 48 h, and the other half were frozen and stored at –20°C. Subsequently, the caudal intervertebral disc samples were decalcified in 10% etheylenediaminetetraacetic acid (EDTA) for 14 days and embedded in paraffin. The samples were cut into 4-μm-thick coronal-oriented sections, which were then processed for hematoxylin and eosin (H&E) staining (26, 27).
Real-time quantitative polymerasechain reaction
Total RNA was extracted from the frozen NP tissue of caudal vertebra using the TRIzol reagent (Invitrogen, CA, USA). PrimeScriptTM RT Master Mix #RR036 A (Takara, Beijing, China) was used to synthesize complementary DNA (cDNA) according to information on the quantity and quality of the RNA. TB Green® Premix Ex Taq™ #RR420B (Takara) was used to perform quantitative PCR (qPCR) on the 7500 Real-Time PCR System (Applied Biosystems, CA, USA). The primer sequences are listed in Supplementary Table S2, and the primers were created by Sangon Biotech (Shanghai, China). The relative expression levels of the genes were calculated utilizing the 2–(ΔΔCT) method, with GAPDH serving as the internal reference gene for the PCR data.
Statistical analysis
The data processing, statistical analysis, and graphs were performed or generated via R 4.2.1 software and GraphPad Prism 9 software. In all analyses, p < 0.05 was regarded as statistically significant.
Results
Identification of DEGs
Two high-throughput sequencing datasets, GSE176205 and GSE167931, were normalized and merged into one dataset for this study. Batch effect elimination was then performed on the merged dataset before data analysis (Figures 2A, B). A total of 636 DEGs, containing 468 upregulated genes and 168 downregulated genes, were obtained from the combined dataset using the limma package for differential gene analysis in R (Figures 3A, B). All DEGs are shown in Supplementary Table S3.
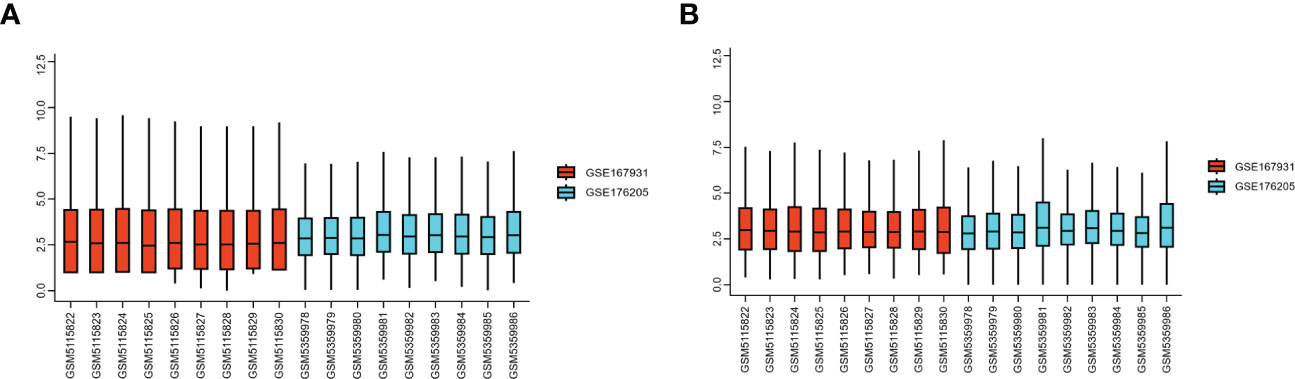
Figure 2 GEO dataset preprocessing. (A) Gene expression level of the dataset before preprocessing. (B) Gene expression level of the dataset after preprocessing. GEO, Gene Expression Omnibus.
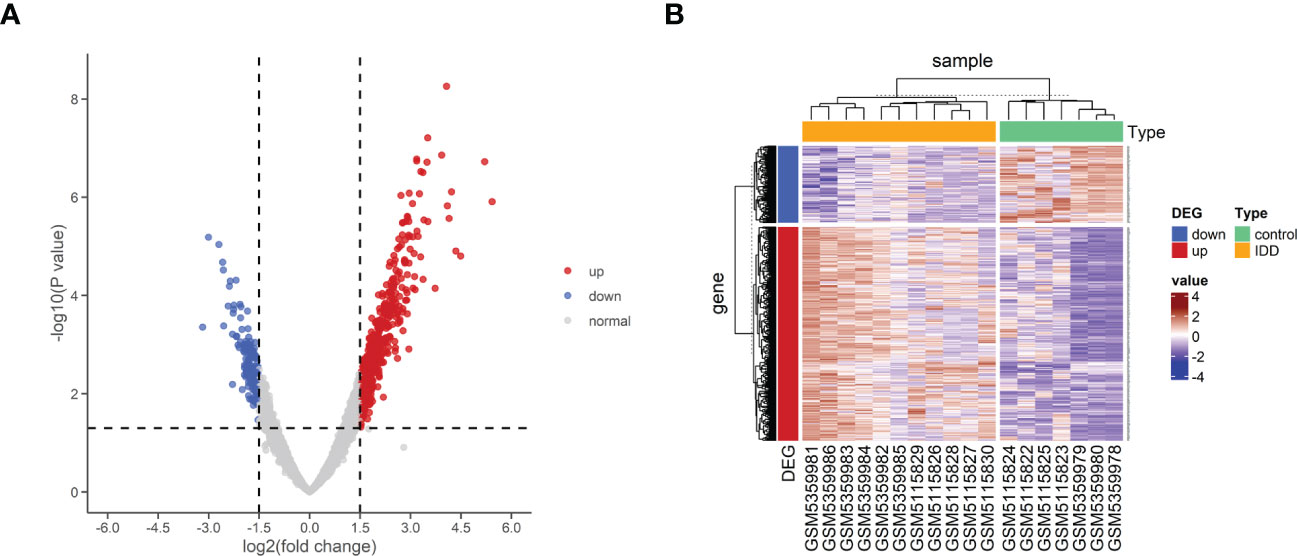
Figure 3 Differential expression of mRNA associated with IDD. (A) Volcano map of differentially expressed genes between control and IDD samples. (B) Heatmap of differentially expressed genes between control and IDD samples. The scale() function was used to normalize the expressions of the DEGs in R. DEG, differentially expressed genes; IDD, intervertebral disc degeneration; mRNA, messenger RNA.
Enrichment analysis of DEGs
To further explore the potential biological changes of these screened DEGs, KEGG and GO enrichment analyses were performed using the DAVID online database and exported into the R environment for visualization. The KEGG results showed that the DEGs were mainly enriched in amyotrophic lateral sclerosis, coronavirus disease (COVID-19), shigellosis, Salmonella infection, and protein processing in the endoplasmic reticulum. Most of the top 15 KEGG pathways were in immune-related diseases (Figures 4A, B). The results of GO BP (biological process) annotation showed that the DEGs were mainly enriched in chromatin organization, protein autophosphorylation, and autophagy. The top 15 GO biological processes revealed that the DEGs were closely associated with autophagy (Figures 4C, D).
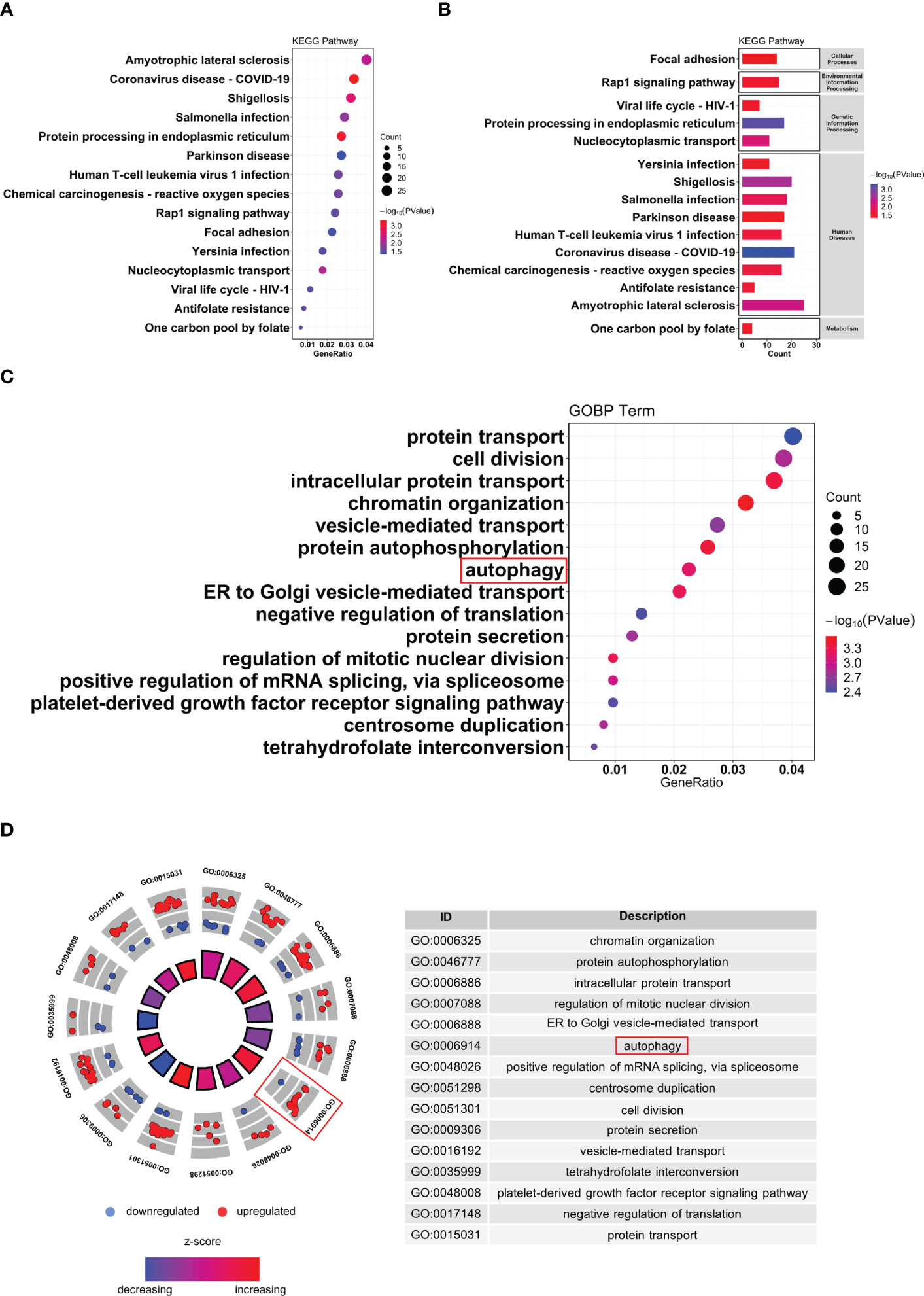
Figure 4 Functional enrichment of analysis of DEGs. (A, B) KEGG pathway analysis of DEGs. (C, D) GO biological process enrichment results of DEGs. DEG, differentially expressed genes; GO, Gene Ontology; KEGG, Kyoto Encyclopedia of Genes and Genomes.
The gene set h.all.v2022.1.Hs.symbols was used for GSEA analysis. After excluding the results without statistical significance, MITOTIC_SPINDLE, IL2_STAT5_ SIGNALING, TNFA_SIGNALING_VIA_NFKB, and UV_RESPONSE_DN were significantly activated in IDD (Figure 5), suggesting that these biological processes may be closely related to IDD.
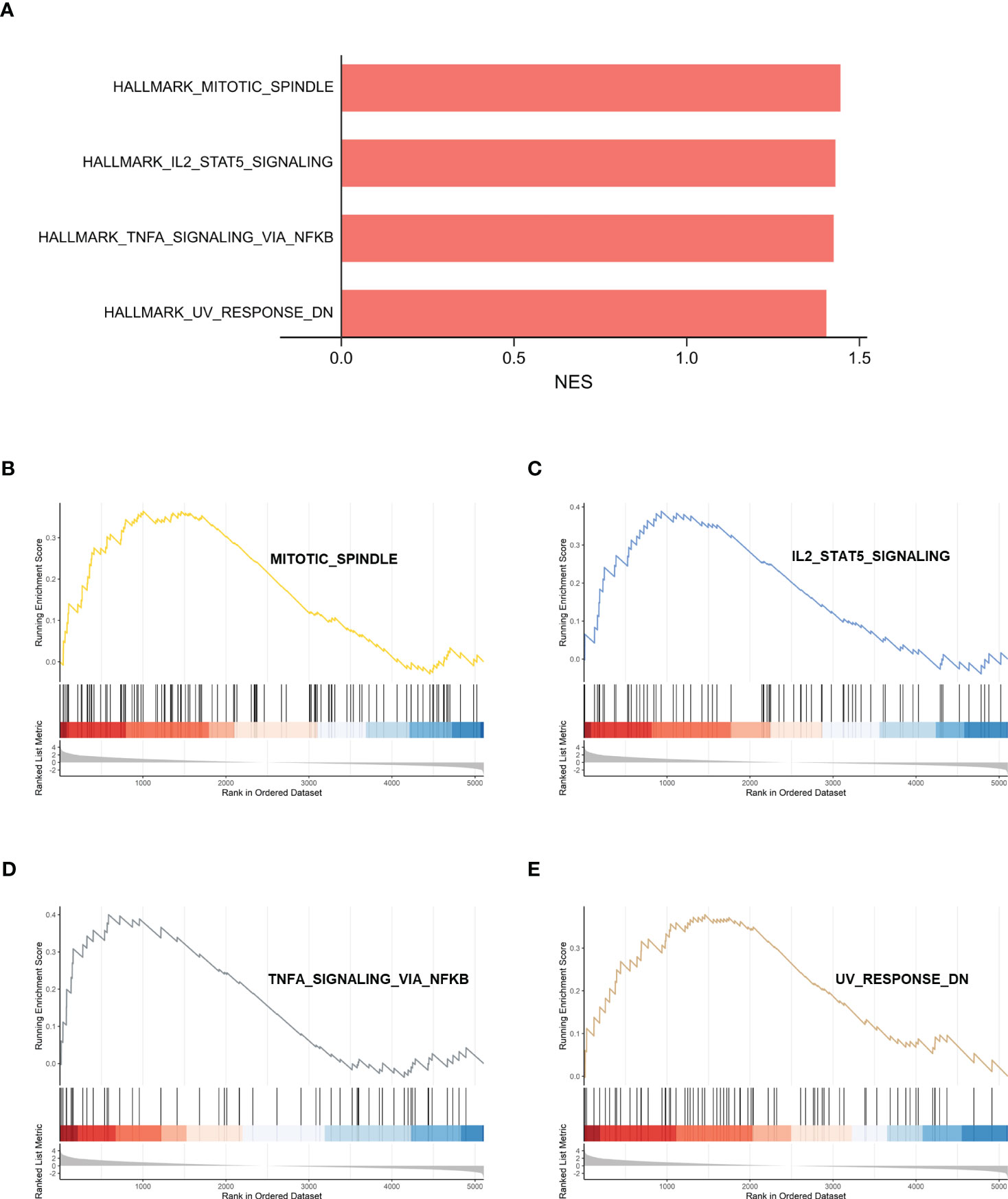
Figure 5 GSEA analysis of HALLMARK between control samples and IDD samples. (A) Normalized enrichment scores (NES) for HALLMARK gene sets representing the combined dataset. (B–E) Results of GSEA analysis after using threshold screening. GSEA, gene set enrichment analysis; IDD, intervertebral disc degeneration.
Identification of DE-ARGs
To further explore autophagy-related genes (ARGs) in IDD, we combined the 404 ARGs obtained from the MsigDB database with the 232 ARGs obtained from the Human Autophagy database to obtain a total of 547 ARGs. The ARGs were then intersected with the DEGs to obtain the 30 DE-ARGs (Figure 6A). KEGG and GO analyses of the DE-ARGs were performed using the DAVID database with p < 0.05 as the threshold, and the enrichment results were visualized in R software (Figures 6B, C).
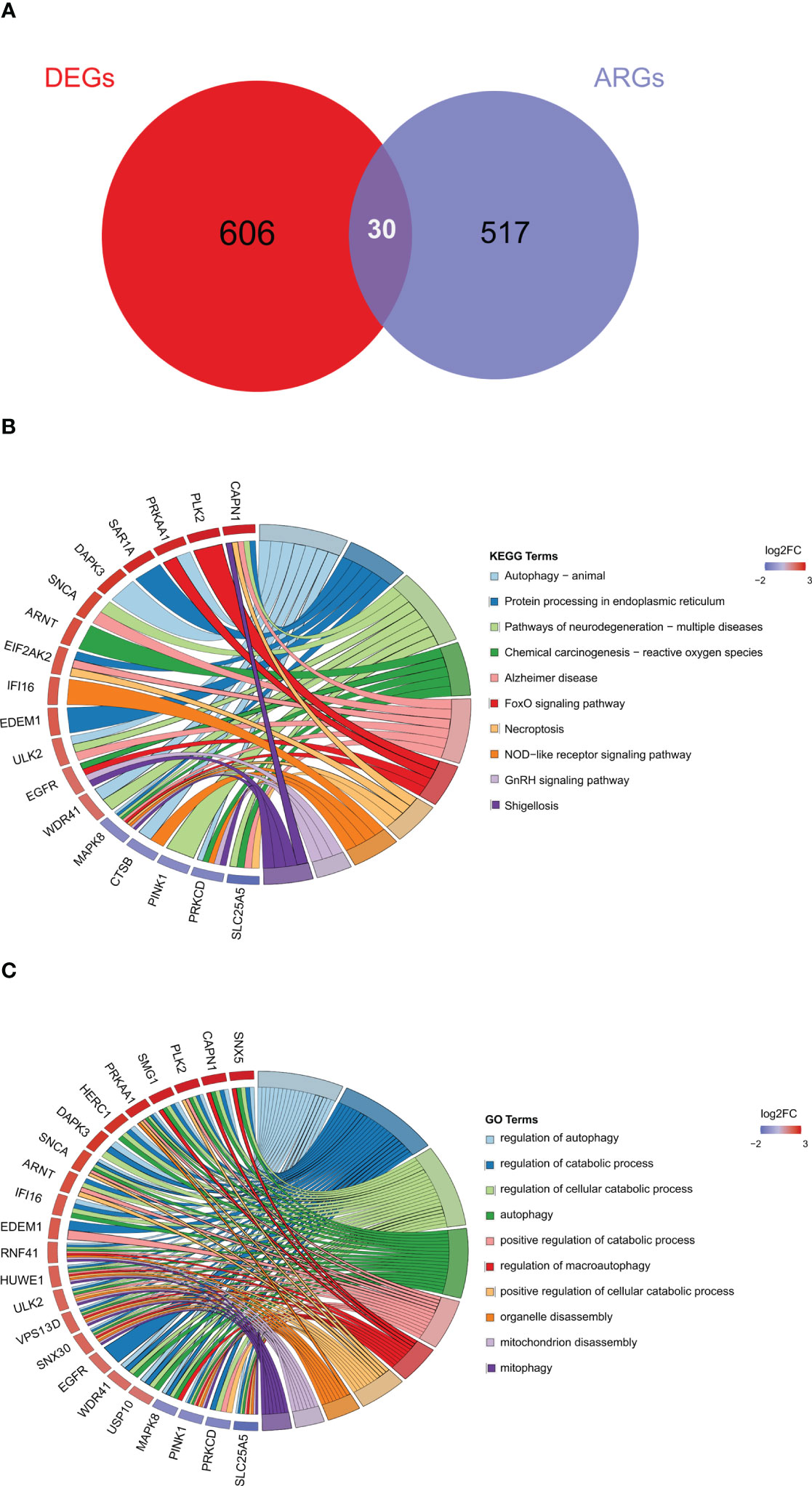
Figure 6 Identification of autophagy-related differentially expressed genes. (A) Venn diagram of DE-ARGs between DEGs and ARGs. (B, C) KEGG and GO biological process enrichment results of DE-ARGs. ARGS, autophagy-related genes; DE-ARGs, differentially expressed autophagy-related genes; DEGs, differentially expressed genes; GO, Gene Ontology; KEGG, Kyoto Encyclopedia of Genes and Genomes.
Construction of PPI and identification of hub genes
We explored the interactions among the 30 DE-ARGs using the STRING database with medium confidence and obtained a PPI network containing 30 nodes and 24 edges (Figure 7A). Using Cytoscape’s internal analysis plug-in, MCODE, the PPI network of the DE-ARGs was filtered to gain the subnetworks with the highest clustering scores for visualization (Figure 7B). As shown in the figure, we hypothesized that MAPK8, CTSB, PRKCD, SNCA, CAPN1, and EGFR are hub genes.
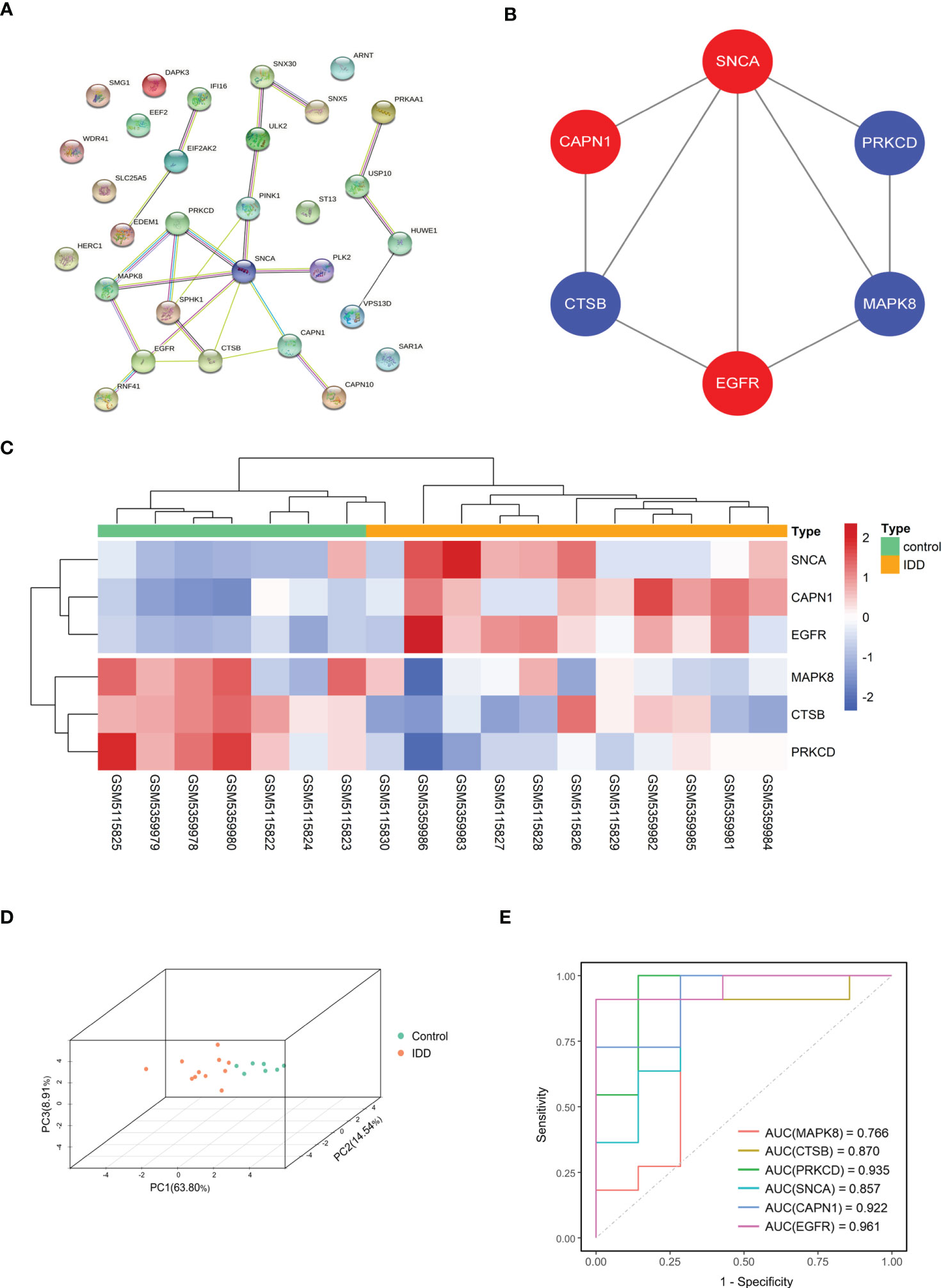
Figure 7 Screening of hub gene in DE-ARGs. (A) The protein–protein interaction network visualized by STRING. (B) Six hub genes were screened by the MCODE plug-in. Red nodes represent upregulated expressed genes, blue nodes represent downregulated expressed genes. (C, D) Gene expression heat map and 3D PCA map of the six hub genes. (E) ROC curve of six hub genes in GSE176205 and GSE167931. DE-ARGs, differentially expressed autophagy-related genes; PCA, principal component analysis; ROC, receiver operating characteristic; STRING, Search Tool for the Retrieval of Interacting Genes/Proteins.
The heat map and 3D PCA map of these six hub genes in the combined dataset revealed that the control group was significantly different from the IDD group (Figures 7C, D). In both the GSE176205 and GSE167931 datasets, the AUC values of the six hub genes were above 0.75, indicating that the six hub genes have excellent diagnostic performance and can be used as promising biomarkers in the diagnosis of IDD (Figure 7E).
The changes in immune cell infiltration in IDD
We evaluated the relative abundance of infiltrating immune cell subtypes in normal and IDD samples using the CIBERSORT algorithm. The bar graph and heat map show the infiltration of multiple immune cell subtypes in each sample (Figure 8A). The violin plot shows the difference in the percentage of immune cells between the two sample groups (Figure 8B). Compared with the normal samples, the IDD samples showed increased infiltration of CD8+T cells and M0 macrophages, whereas CD4 memory resting T cells, neutrophils, resting dendritic cells, follicular helper T cells, and monocytes showed decreased infiltration (Figure 8C).
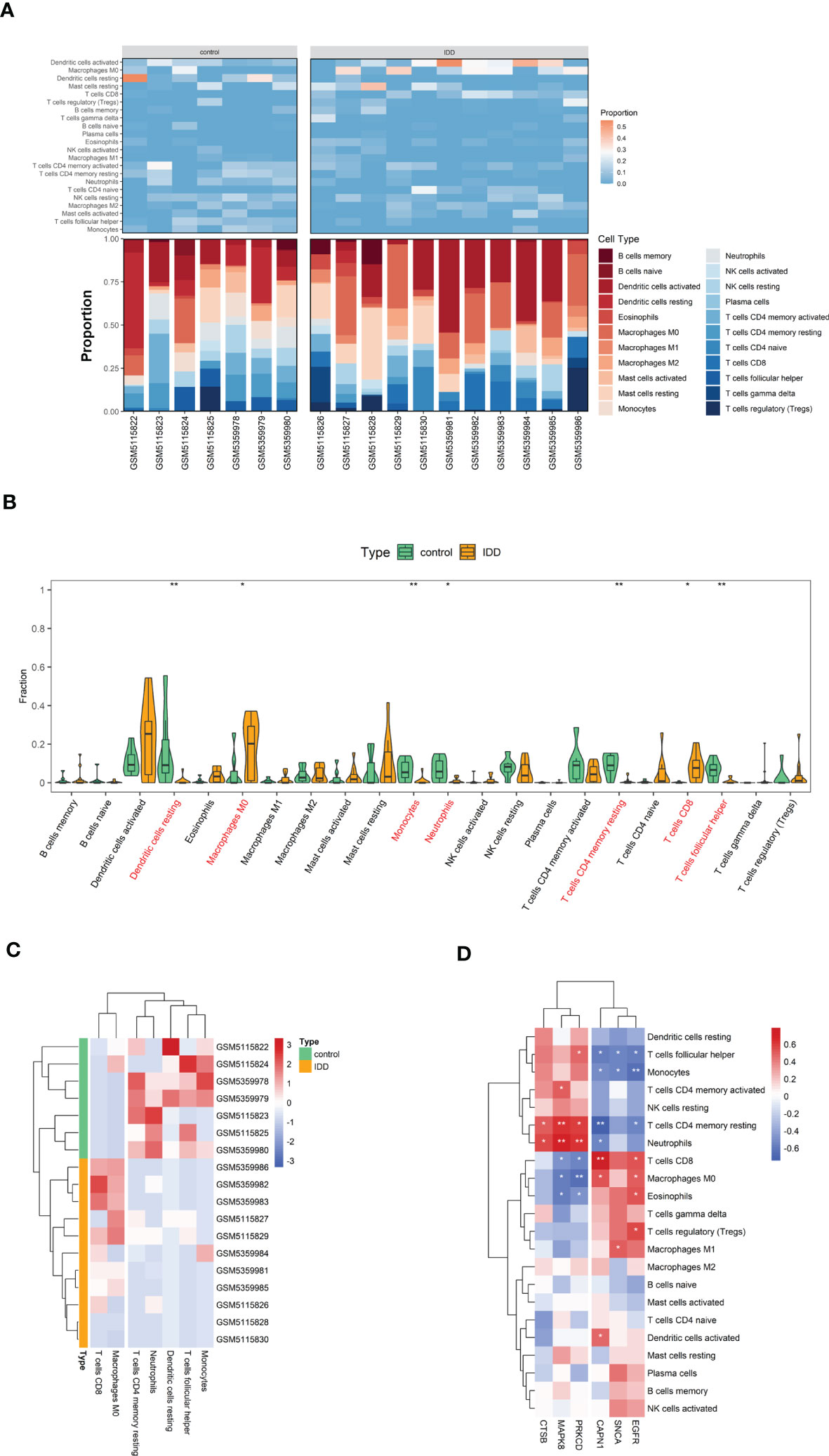
Figure 8 The visualization of cell infiltration between the IDD and control groups. (A) The stacked bar chart and heat map indicates the relative proportions of 22 immune cells. (B) A violin plot of the immune cell proportions in two groups. (C) Significantly different immune cell infiltration in the two sample groups. (D) Heat map of the correlation between the expression of the six hub genes and the infiltration of 22 immune cell type proportions. (* p < 0.05, ** p < 0.01) .
Finally, the heat map revealed the correlation between the hub genes and immune cell infiltration (Figure 8D). As shown, the expression of hub genes was significantly correlated with immune cell infiltration across the subtypes. The reduced CD4 memory resting T cells in IDD samples are negatively correlated with highly expressed CAPN1 and EGFR and positively correlated with MAPK8, CTSB, and PRKCD expression.
Potential mRNA–miRNA–lncRNA (ceRNA) network of hub genes
To reveal the potential post-transcriptional regulatory mechanisms of these six hub genes, we screened differentially expressed miRNAs and lncRNAs during IDD development and constructed a ceRNA network. DE-miRNAs and DE-lncRNAs of GSE167199 were identified and screened in R software using the limma package, with |log2FC| > 1 and p < 0.05 set as significance thresholds. A total of 139 DE-lncRNAs (Supplementary Table S4) and 65 DE-miRNAs (Supplementary Table S5) were identified (Figures 9A, B).
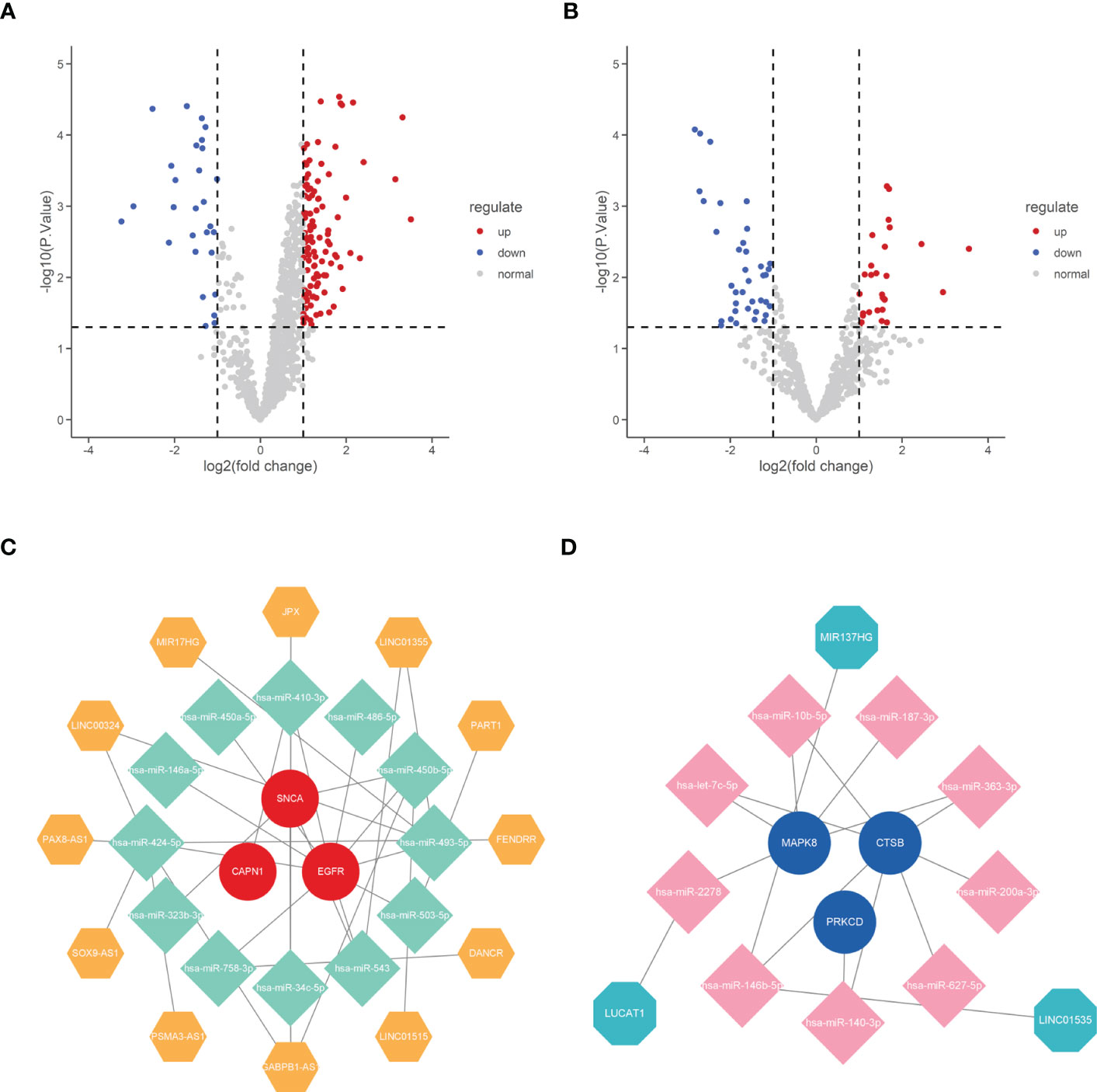
Figure 9 Construction of ceRNA network of the six hub genes. (A) Volcano plot of differentially expressed lncRNAs between normal and IDD samples. (B) Volcano plot of differentially expressed miRNAs between normal and IDD samples. (C) The ceRNA network of upregulated expression of hub genes. (D) The ceRNA network of downregulated expression of hub genes. IDD, intervertebral disc degeneration; ceRNA, competing endogenous RNA; lncRNA, long non-coding RNA; miRNA, microRNA.
Subsequently, the six hub genes targeted miRNAs were predicted using the ENCORI online database and intersected with DE-miRNAs to obtain mRNA–miRNA binding pairs (Supplementary Table S6). Moreover, the mirNet database was used to predict the possible binding lncRNAs of DE-miRNAs screened in the previous step, and the predicted lncRNAs were intersected with DE-lncRNAs to construct miRNA–lncRNA binding pairs (Supplementary Table S7). The mRNA–miRNA and miRNA–lncRNA binding pairs were then integrated to construct the ceRNA network of the six hub genes (Figures 9C, D).
Validation of hub genes
According to the results, X-ray, MRI, and H&E staining of NP tissue sections revealed a severely damaged NP in IDD rat models (Figure 10A). Reverse transcription qPCR (RT-qPCR) analysis showed that the mRNA levels of aggregated proteoglycan (aggrecan) and type II collagen (COL-2) were decreased in IDD models (Figure 10B). We examined the mRNA expression of the hub genes in the IDD model. As the results showed, unlike other hub genes, only the expressions of CAPN1 and MAPK8 were consistent with our DEGs analysis results (Figure 10C). In addition, we also performed correlation analysis of CAPN1 and MAPK8 with immune cell subtype infiltration using p < 0.05 as screening threshold (Figure 10D). Therefore, CAPN1 and MAPK8 were validated as the final hub genes in the progression of IDD.
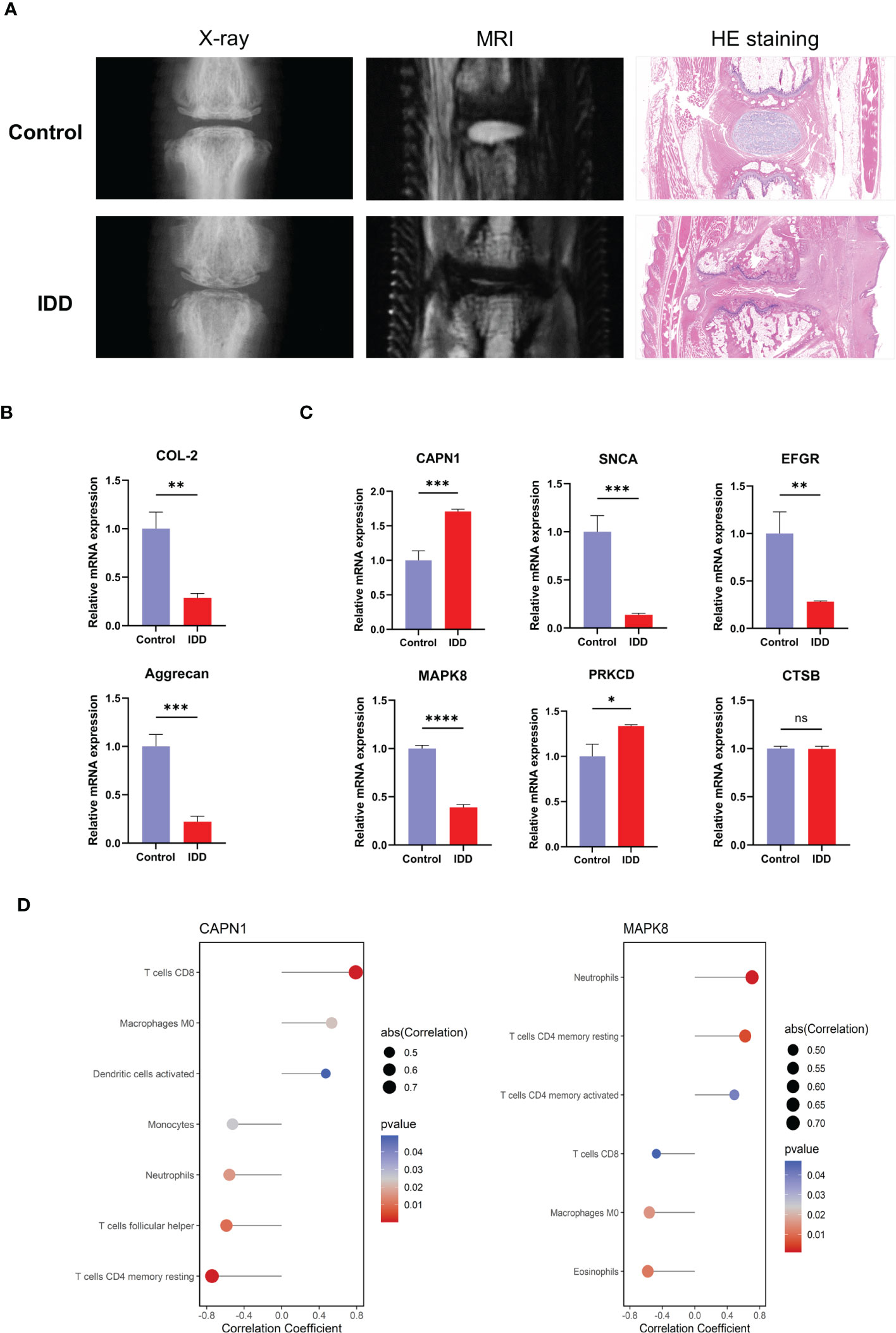
Figure 10 Expression levels of the hub genes in normal rat and IDD models. (A) Representative images of X-ray, MRI and H&E staining after needle puncture (B) The mRNA expression level of marker genes of NP. (C) The mRNA expression of the six hub genes in normal and IDD models. (D) CAPN1 and MAPK8 were analyzed for correlation with immune infiltration, and the analysis results of p < 0.05 were retained. H&E, hematoxylin and eosin; IDD, intervertebral disc degeneration; mRNA, messenger RNA. (* p < 0.05, ** p < 0.01, *** p < 0.001, **** p < 0.0001; ns, not significant).
Discussion
LBP is one of the most common health problems and represents a significant economic and lifestyle burden to human society, affecting approximately 60%–80% of the global population (28–30). IDD is one of the most important factors in the pathogenesis of LBP, and the application of high-throughput sequencing technology combined with bioinformatics analysis could help us to identify the biomarkers or key biological functions during IDD development and provide new ideas and options for IDD treatment (31, 32).
Autophagy, a highly conserved self-phagocytosis process in eukaryotic cells, prevents excessive apoptosis and promotes the secretion of the ECM in NP cells, and therefore plays a protective role in disc degeneration (20). Several compounds, such as autophagy agonists, can attenuate IDD by promoting autophagy to reduce oxidative stress, apoptosis, and ECM degradation in NP cells (33).
The NP tissue of the disc is surrounded by annulus fibrosus and cartilage endplate, a unique structure that sets NP cells and ECM in this tissue apart from the immune cells. However, when degeneration occurs, the NP outflow is recognized as a “foreign antigen” when it comes into contact with the external immune system, and an autoimmune response is generated to initial immune response. Once the NP tissue is damaged, the granulation tissue is formed, allowing the external blood vessels to extend and causing the NP tissue to be exposed to external immune cells. At this point, the ECMs secreted by NP cells, such as collagen and proteoglycan, are recognized as autoantigens and trigger a secondary immune response mediated by cytotoxic T cells (34, 35). Therefore, identifying the molecules related to inflammatory environment and immune cell infiltration in degenerating discs is important for revealing the underlying mechanisms of IDD pathogenesis.
In this study, we performed KEGG and GO enrichment analyses with the 636 obtained DEGs from the IDD database. The KEGG results showed that many immune-related disease pathways were highly activated in the IDD group, such as shigellosis, Salmonella infection, and Yersinia infection. Previous studies have shown that bacterial infections, such as Staphylococcus aureus and Cutibacterium acnes, create an inflammatory environment in the intervertebral disc and promote IDD progression (36–38).
Moreover, GO results demonstrate that autophagy plays a central role during IDD development. Thus, based on above results, we next screened for only autophagy-related genes in DEGs and explored the possible core DEGs. Using the MCODE plug-in of Cytoscape software, we successfully screened the core gene clusters of 30 DE-ARGs. Combined with PPI network identification, we selected six genes as the hub genes, that is MAPK8, CTSB, PRKCD, SNCA, CAPN1, and EGFR. The expression of these six hub genes in the original raw data was significantly different between the control and IDD samples.
CIBERSORT is an inverse convolution analysis algorithm based on linear support vector regression that estimates the relative abundance of immune cells in a mixed cell population by analyzing gene expression data (39). Using this algorithm, we found that there was increased infiltration of CD8+ T cells and M0 macrophages in IDD samples, and decreased infiltration of CD4+ memory T cells, neutrophils, resting dendritic cells, follicular helper T cells, and monocytes. Correlation analysis of the expression of six hub genes with the relative abundance of 22 immune cell types revealed that the hub genes were significantly correlated with several immune cell types that were increased or decreased in the IDD group. The increased infiltration of CD4+ and CD8+ T cells has been reported in spontaneous disc herniation in the human tumor necrosis factor alpha (TNF-α)-overexpressing transgenic mouse model (Tg197) (40). Thus, we speculate that the efflux of NP from the degenerating disc and exposure to the external immune environment generates an inflammatory environment. Released pro-inflammatory cytokines promote the infiltration of other immune cells, regulate hub gene expression and the biological behavior of NP cells, maintain the inflammatory microenvironment, and ultimately exacerbate disc herniation.
Non-coding RNAs, such as lncRNAs and miRNAs, have also attracted widespread attention for their role in mediating autophagy in IDD (41–43). Non-coding RNAs can regulate the degree of activation of autophagy in immune cells by directly or indirectly targeting autophagy-related genes and the associated signaling pathways. However, relatively little is known about the co-regulation mechanisms of non-coding RNAs. LncRNAs, microRNAs, and mRNAs can regulate the development and progression of IDD by forming ceRNA networks (21, 44, 45). The GSE167199 dataset used in this study was also used previously for the study of ceRNA networks and identified two ceRNA axes, lncRNA XIST-hsa-miR-4775-PLA2G7 and lncRNA XIST-hsa-miR-424–5p-AMOT/TGFBR3, that may be involved in the progression of IDD (46). However, the experimental results were mainly based on one RNA sequencing dataset. They did not involve online databases for target prediction, so multiple samples and online databases need to be combined for comprehensive analysis. The ceRNA networks regulating IDD’s molecular mechanisms remain to be further investigated and explored.
Through differential analysis of gene expression profiles downloaded from the GEO database and online database prediction, we identified several pairs of ceRNA axis and constructed ceRNA regulatory networks for the six hub genes. Among the ceRNA networks, several miRNAs and lncRNAs have been reported in the literature to be involved in disease regulation. In NP cells, the overexpression of LINC00324 increases Fas ligand (FasL) expression and promotes disc degeneration (47). The miR-140–3p affects bone marrow stromal cells (BMSCs) in degenerative intervertebral disc disease (IVD) by directly targeting KLF5 and interacting with the N–cadherin/MDM2/Slug axis, thereby regulating regenerative effects in degenerative IVD (48). LINC01535 affects clear cell renal cell carcinoma progression by mediating PI3K/Akt signaling through the LINC01535/miR–146b-5p/TRIM2 axis (49), corresponding to the LINC01535/miR-146b-5p binding pair predicted in this study. Although there are no studies on the relationship between LINC01535 and IDD, based on the results of previous studies and bioinformatic analysis, it is reasonable to believe that the ceRNA axis, composed of LINC01535 and several other non-coding RNAs, is involved in the regulatory network on disc degeneration.
Finally, we designed in vitro experiments to verify whether or not the hub genes were differentially expressed in the NP of the IDD rat model. Based on the RT-qPCR results, we found that two hub genes, MAPK8 and CAPN1, were differentially expressed in the IDD and control groups, and that these expression trends were consistent with the results of previous bioinformatics analysis.
MAPK8, also known as c-JUN N-terminal kinase (JNK), is a member of the MAPK family that regulates a variety of physiological responses, including inflammatory responses, cell differentiation, cell proliferation, and cell death. The dysregulation of MAPK8 has also been implicated in several diseases, including diabetes, cancer, autoimmune diseases, cardiac hypertrophy, and asthma (50). Previous studies have shown that MAPK8 also has some relevance to the physiological state of NP cells. In human NP cells, the use of JNK pathway inhibitors can counteract interleukin 17 (IL-17)-induced COX2/PGE2 production and IVD inflammation, which may be a potential therapeutic target for alleviating IDD (51). At high osmotic pressure, the JNK pathway can regulate cell generation, proliferation, and apoptosis in rabbit NP cells, which helps to elucidate the pathological mechanisms involved in intervertebral discs under elevated osmotic pressure and load (52). In another study, the development of disc degeneration in rabbits was prevented by regulating the JNK signaling pathway and the downstream p53 pathway, in which JNK/p53 plays an important role (53). Therefore, MAPK8 could be further investigated as a potential biomarker for IDD.
Calpain 1 (CAPN1), an intracellular cysteine protease, is ubiquitously expressed in mammals, and is involved in the cleavage of cytoskeletal, mitochondrial, and lysosomal membrane proteins and mediated impairment of autophagic flux to neurons (54). Virus-infected cardiomyocytes induce inflammation in an immune-mediated manner via NLRP3 inflammasome. CAPN1 inhibitors can inhibit NLRP3 inflammasome release and alleviate myocardial injury (55). CAPN1 has been well studied in autophagy-related diseases, but whether or not it impacts the progress of IDD, or whether the signal pathway related to CAPN1 regulates autophagy activity and finally acts on the degenerated NP, has not been reported on in detail in published studies and needs further research.
Of course, this study inevitably has some limitations. First, the sample size of gene expression profiles downloaded from public databases is slightly inadequate, and individual differences in the samples may affect the analysis results’ generalizability. In addition, only the mRNA levels and not the protein levels of the hub gene were validated by RT-qPCR. More relevant in vivo and in vitro experiments are needed to demonstrate the role of these hub genes and their potential mechanisms in IDD. Finally, some of the lncRNAs and miRNAs obtained from the analysis have not been reported in IDD-related studies, and further experiments are needed for validation.
In summary, we analyzed the DEGs of IDD using bioinformatics methods, including the functional enrichment analysis of DEGs, acquisition of autophagy-related DEGs, PPI network analysis, and in vitro qPCR validation. In addition, we performed immune cell infiltration analysis and lncRNA–miRNA–mRNA network construction, and investigated the correlation between immune cell infiltration and ceRNA network in IDD. In animal experiments, we validated the expression of two hub genes (MAPK8 and CAPN1) at the mRNA level, which was consistent with the results of bioinformatics analysis. Our study provides new insights into the pathogenesis of IDD and helps to identify new potential therapeutic targets in the pathogenesis of IDD.
Data availability statement
The datasets presented in this study can be found in online repositories. The names of the repository/repositories and accession number(s) can be found in the article/Supplementary Material.
Ethics statement
The animal study was reviewed and approved by Vital River Laboratory Animal Care and Use Committee.
Author contributions
YXZ, JZ, and ZS contributed to the design of the experiments, analysis of the data, and writing of the article. HW and RN contributed to the validation of the experimental results. LX and YCZ contributed to the collection of the experimental data. KY, XX, and JT supervised the entire experimental process and helped revise the manuscript. All authors contributed to the article and approved the submitted version.
Funding
This study was funded by the Natural Science Foundation of Jiangsu Province (BK20201130), the Nanjing Medical Key Science and Technology Development Project (ZKX21062), the National Natural Science Foundation of China (82070902), the Shanghai Municipal Commission of Health and Family Planning (NO.ZY(2021–2023)-0208), the Shanghai key clinical specialty construction project (SHSLCZDZK04802), and the Key Project in Medical and Industrial Intersection of Shanghai Jiaotong University (YG2019ZDA16).
Conflict of interest
The authors declare that the research was conducted in the absence of any commercial or financial relationships that could be construed as a potential conflict of interest.
Publisher’s note
All claims expressed in this article are solely those of the authors and do not necessarily represent those of their affiliated organizations, or those of the publisher, the editors and the reviewers. Any product that may be evaluated in this article, or claim that may be made by its manufacturer, is not guaranteed or endorsed by the publisher.
Supplementary material
The Supplementary Material for this article can be found online at: https://www.frontiersin.org/articles/10.3389/fimmu.2023.1188774/full#supplementary-material
References
1. Hartvigsen J, Hancock MJ, Kongsted A, Louw Q, Ferreira ML, Genevay S, et al. What low back pain is and why we need to pay attention. Lancet (2018) 391(10137):2356–67. doi: 10.1016/S0140-6736(18)30480-X
2. Buckwalter JA. Aging and degeneration of the human intervertebral disc. Spine (Phila Pa 1976) (1995) 20(11):1307–14. doi: 10.1097/00007632-199506000-00022
3. Liao Z, Wu X, Song Y, Luo R, Yin H, Zhan S, et al. Angiopoietin-like protein 8 expression and association with extracellular matrix metabolism and inflammation during intervertebral disc degeneration. J Cell Mol Med (2019) 23(8):5737–50. doi: 10.1111/jcmm.14488
4. Cassinelli EH, Hall RA, Kang JD. Biochemistry of intervertebral disc degeneration and the potential for gene therapy applications. Spine J (2001) 1(3):205–14. doi: 10.1016/S1529-9430(01)00021-3
5. Theodore N. Degenerative cervical spondylosis. N Engl J Med (2020) 383(2):159–68. doi: 10.1056/NEJMra2003558
6. Zhao R, Liu W, Xia T, Yang L. Disordered mechanical stress and tissue engineering therapies in intervertebral disc degeneration. Polymers (Basel) (2019) 11(7)1151. doi: 10.3390/polym11071151
7. Liao Z, Luo R, Li G, Song Y, Zhan S, Zhao K, et al. Exosomes from mesenchymal stem cells modulate endoplasmic reticulum stress to protect against nucleus pulposus cell death and ameliorate intervertebral disc degeneration in vivo. Theranostics (2019) 9(14):4084–100. doi: 10.7150/thno.33638
8. Wu X, Song Y, Liu W, Wang K, Gao Y, Li S, et al. IAPP modulates cellular autophagy, apoptosis, and extracellular matrix metabolism in human intervertebral disc cells. Cell Death Discovery (2017) 3:16107. doi: 10.1038/cddiscovery.2016.107
9. Cunha C, Silva AJ, Pereira P, Vaz R, Gonçalves RM, Barbosa MA. The inflammatory response in the regression of lumbar disc herniation. Arthritis Res Ther (2018) 20(1):251. doi: 10.1186/s13075-018-1743-4
10. Gong CY, Zhang HH. Autophagy as a potential therapeutic target in intervertebral disc degeneration. Life Sci (2021) 273:119266. doi: 10.1016/j.lfs.2021.119266
11. Cao G, Yang S, Cao J, Tan Z, Wu L, Dong F, et al. The role of oxidative stress in intervertebral disc degeneration. Oxid Med Cell Longev (2022) 2022:2166817. doi: 10.1155/2022/2166817
12. Feng Y, Egan B, Wang J. Genetic factors in intervertebral disc degeneration. Genes Dis (2016) 3(3):178–85. doi: 10.1016/j.gendis.2016.04.005
13. Zhou X, Hong Y, Zhan Y. Karacoline, identified by network pharmacology, reduces degradation of the extracellular matrix in intervertebral disc degeneration via the NF-κB signaling pathway. J Pharm Anal (2020) 10(1):13–22. doi: 10.1016/j.jpha.2019.07.002
14. Kamali A, Ziadlou R, Lang G, Pfannkuche J, Cui S, Li Z, et al. Small molecule-based treatment approaches for intervertebral disc degeneration: current options and future directions. Theranostics (2021) 11(1):27–47. doi: 10.7150/thno.48987
15. Liu M, Zeng T, Zhang X, Liu C, Wu Z, Yao L, et al. ATR/Chk1 signaling induces autophagy through sumoylated RhoB-mediated lysosomal translocation of TSC2 after DNA damage. Nat Commun (2018) 9(1):4139. doi: 10.1038/s41467-018-06556-9
16. Maiuri MC, Zalckvar E, Kimchi A, Kroemer G. Self-eating and self-killing: crosstalk between autophagy and apoptosis. Nat Rev Mol Cell Biol (2007) 8(9):741–52. doi: 10.1038/nrm2239
17. Mizushima N, Komatsu M. Autophagy: renovation of cells and tissues. Cell (2011) 147(4):728–41. doi: 10.1016/j.cell.2011.10.026
18. Levine B, Kroemer G. Biological functions of autophagy genes: a disease perspective. Cell (2019) 176(1-2):11–42. doi: 10.1016/j.cell.2018.09.048
19. Qi Z, He Q, Ji L, Ding S. Antioxidant supplement inhibits skeletal muscle constitutive autophagy rather than fasting-induced autophagy in mice. Oxid Med Cell Longev (2014) 2014:315896. doi: 10.1155/2014/315896
20. Madhu V, Guntur AR, Risbud MV. Role of autophagy in intervertebral disc and cartilage function: implications in health and disease. Matrix Biol (2021) 100-101:207–20. doi: 10.1016/j.matbio.2020.12.002
21. Cao S, Liu H, Fan J, Yang K, Yang B, Wang J, et al. An oxidative stress-related gene pair (CCNB1/PKD1), competitive endogenous RNAs, and immune-infiltration patterns potentially regulate intervertebral disc degeneration development. Front Immunol (2021) 12:765382. doi: 10.3389/fimmu.2021.765382
22. Guo W, Mu K, Zhang B, Sun C, Zhao L, Dong ZY, et al. The circular RNA FAM169A functions as a competitive endogenous RNA and regulates intervertebral disc degeneration by targeting miR-583 and BTRC. Cell Death Dis (2020) 11(5):315. doi: 10.1038/s41419-020-2543-8
23. Wang K, Song Y, Liu W, Wu X, Zhang Y, Li S, et al. The noncoding RNA linc-ADAMTS5 cooperates with RREB1 to protect from intervertebral disc degeneration through inhibiting ADAMTS5 expression. Clin Sci (Lond) (2017) 131(10):965–79. doi: 10.1042/CS20160918
24. Wu TZ, Hu EQ, Xu SB, Chen MJ, Guo PF, Dai ZH, et al. clusterProfiler 4.0: a universal enrichment tool for interpreting omics data. Innovation (2021) 2(3)100141. doi: 10.1016/j.xinn.2021.100141
25. Issy AC, Castania V, Castania M, Salmon CEG, Nogueira-Barbosa MH, Bel ED, et al. Experimental model of intervertebral disc degeneration by needle puncture in wistar rats. Braz J Med Biol Res (2013) 46(3):235–44. doi: 10.1590/1414-431X20122429
26. He R, Wang Z, Cui M, Liu S, Wu W, Chen M, et al. HIF1A alleviates compression-induced apoptosis of nucleus pulposus derived stem cells via upregulating autophagy. Autophagy (2021) 17(11):3338–60. doi: 10.1080/15548627.2021.1872227
27. Ni S, Ling Z, Wang X, Cao Y, Wu T, Deng R, et al. Sensory innervation in porous endplates by netrin-1 from osteoclasts mediates PGE2-induced spinal hypersensitivity in mice. Nat Commun (2019) 10(1):5643. doi: 10.1038/s41467-019-13476-9
28. Shvartzman L, Weingarten E, Sherry H, Levin S, Persaud A. Cost-effectiveness analysis of extended conservative therapy versus surgical intervention in the management of herniated lumbar intervertebral disc. Spine (Phila Pa 1976) (1992) 17(2):176–82. doi: 10.1097/00007632-199202000-00010
29. Buchbinder R, van Tulder M, Oberg B, Costa LM, Woolf A, Schoene M, et al. Low back pain: a call for action. Lancet (2018) 391(10137):2384–8. doi: 10.1016/S0140-6736(18)30488-4
31. Bydon M, Moinuddin FM, Yolcu YU, Wahood W, Alvi MA, Goyal A, et al. Lumbar intervertebral disc mRNA sequencing identifies the regulatory pathway in patients with disc herniation and spondylolisthesis. Gene (2020) 750:144634. doi: 10.1016/j.gene.2020.144634
32. Cui S, Zhou Z, Chen X, Wei F, Richards RG, Alini M, et al. Transcriptional profiling of intervertebral disc in a post-traumatic early degeneration organ culture model. JOR Spine (2021) 4(3):e1146. doi: 10.1002/jsp2.1146
33. Liu J, Yu P, Dai F, Jiang H, Ma Z. Tetrandrine reduces oxidative stress, apoptosis, and extracellular matrix degradation and improves intervertebral disc degeneration by inducing autophagy. Bioengineered (2022) 13(2):3944–57. doi: 10.1080/21655979.2022.2031396
34. Ye F, Lyu FJ, Wang H, Zheng Z. The involvement of immune system in intervertebral disc herniation and degeneration. JOR Spine (2022) 5(1):e1196. doi: 10.1002/jsp2.1196
35. Sun Z, Liu B, Luo ZJ. The immune privilege of the intervertebral disc: implications for intervertebral disc degeneration treatment. Int J Med Sci (2020) 17(5):685–92. doi: 10.7150/ijms.42238
36. Ganko R, Rao PJ, Phan K, Mobbs RJ. Can bacterial infection by low virulent organisms be a plausible cause for symptomatic disc degeneration? a systematic review. Spine (Phila Pa 1976) (2015) 40(10):E587–92. doi: 10.1097/BRS.0000000000000832
37. Lan W, Wang X, Tu X, Hu X, Lu H. Different phylotypes of cutibacterium acnes cause different modic changes in intervertebral disc degeneration. PloS One (2022) 17(7):e0270982. doi: 10.1371/journal.pone.0270982
38. Schweizer TA, Andreoni F, Acevedo C, Scheier TC, Heggli I, Maggio EM, et al. Intervertebral disc cell chondroptosis elicits neutrophil response in staphylococcus aureus spondylodiscitis. Front Immunol (2022) 13:908211. doi: 10.3389/fimmu.2022.908211
39. Newman AM, Liu CL, Green MR, Gentles AJ, Feng W, Xu Y, et al. Robust enumeration of cell subsets from tissue expression profiles. Nat Methods (2015) 12(5):453–7. doi: 10.1038/nmeth.3337
40. Gorth DJ, Shapiro IM, Risbud MV. Transgenic mice overexpressing human TNF-alpha experience early onset spontaneous intervertebral disc herniation in the absence of overt degeneration. Cell Death Dis (2018) 10(1):7. doi: 10.1038/s41419-018-1246-x
41. Mei L, Zheng Y, Gao X, Ma T, Xia B, Hao Y, et al. Hsa-let-7f-1-3p targeting the circadian gene Bmal1 mediates intervertebral disc degeneration by regulating autophagy. Pharmacol Res (2022) 186:106537. doi: 10.1016/j.phrs.2022.106537
42. Wu T, Jia X, Zhu Z, Guo K, Wang Q, Gao Z, et al. Inhibition of miR-130b-3p restores autophagy and attenuates intervertebral disc degeneration through mediating ATG14 and PRKAA1. Apoptosis (2022) 27(5-6):409–25. doi: 10.1007/s10495-022-01725-0
43. Wang XB, Wang H, Long HQ, Li DY, Zheng X. LINC00641 regulates autophagy and intervertebral disc degeneration by acting as a competitive endogenous RNA of miR-153-3p under nutrition deprivation stress. J Cell Physiol (2019) 234(5):7115–27. doi: 10.1002/jcp.27466
44. Xiang Q, Kang L, Wang J, Liao Z, Song Y, Zhao K, et al. CircRNA-CIDN mitigated compression loading-induced damage in human nucleus pulposus cells via miR-34a-5p/SIRT1 axis. EBioMedicine (2020) 53:102679. doi: 10.1016/j.ebiom.2020.102679
45. Song J, Wang HL, Song KH, Ding ZW, Wang HL, Ma XS, et al. CircularRNA_104670 plays a critical role in intervertebral disc degeneration by functioning as a ceRNA. Exp Mol Med (2018) 50(8):1–12. doi: 10.1038/s12276-018-0125-y
46. Li Z, Sun Y, He M, Liu J. Differentially-expressed mRNAs, microRNAs and long noncoding RNAs in intervertebral disc degeneration identified by RNA-sequencing. Bioengineered (2021) 12(1):1026–39. doi: 10.1080/21655979.2021.1899533
47. Chen Y, Wu Y, Chen R, Xu C, Chen Q. LncRNA LINC00324 is upregulated in intervertebral disk degeneration and upregulates FasL in nucleus pulposus cells. Mol Cell Biochem (2021) 476(5):1995–2000. doi: 10.1007/s11010-021-04058-9
48. Wang Z, Zhang S, Zhao Y, Qu Z, Zhuang X, Song Q, et al. MicroRNA-140-3p alleviates intervertebral disc degeneration via KLF5/N-cadherin/MDM2/Slug axis. RNA Biol (2021) 18(12):2247–60. doi: 10.1080/15476286.2021.1898176
49. Zhang Z, Fu X, Gao Y, Nie Z. LINC01535 attenuates ccRCC progression through regulation of the miR-146b-5p/TRIM2 axis and inactivation of the PI3K/Akt pathway. J Oncol (2022) 2022:2153337. doi: 10.1155/2022/2153337
50. Kumar A, Singh UK, Kini SG, Garg V, Agrawal S, Tomar PK, et al. JNK pathway signaling: a novel and smarter therapeutic targets for various biological diseases. Future Med Chem (2015) 7(15):2065–86. doi: 10.4155/fmc.15.132
51. Li JK, Nie L, Zhao YP, Zhang YQ, Wang X, Wang SS, et al. IL-17 mediates inflammatory reactions via p38/c-fos and JNK/c-jun activation in an AP-1-dependent manner in human nucleus pulposus cells. J Transl Med (2016) 14:77. doi: 10.1186/s12967-016-0833-9
52. Dong ZH, Wang DC, Liu TT, Li FH, Liu RL, Wei JW, et al. The roles of MAPKs in rabbit nucleus pulposus cell apoptosis induced by high osmolality. Eur Rev For Med Pharmacol Sci (2014) 18(19):2835–45. doi: 10.1055/s-0034-1376584
53. Ge J, Cheng X, Yuan C, Qian J, Wu C, Cao C, et al. Syndecan-4 is a novel therapeutic target for intervertebral disc degeneration via suppressing JNK/p53 pathway. Int J Biol Sci (2020) 16(5):766–76. doi: 10.7150/ijbs.40189
54. Liu Y, Che X, Zhang H, Fu X, Yao Y, Luo J, et al. CAPN1 (Calpain1)-mediated impairment of autophagic flux contributes to cerebral ischemia-induced neuronal damage. Stroke (2021) 52(5):1809–21. doi: 10.1161/STROKEAHA.120.032749
Keywords: intervertebral disc degeneration (IDD), immune infiltration, bioinformatic analysis, autophagy, MAPK8, CAPN1
Citation: Zhang Y, Zhang J, Sun Z, Wang H, Ning R, Xu L, Zhao Y, Yang K, Xi X and Tian J (2023) MAPK8 and CAPN1 as potential biomarkers of intervertebral disc degeneration overlapping immune infiltration, autophagy, and ceRNA. Front. Immunol. 14:1188774. doi: 10.3389/fimmu.2023.1188774
Received: 17 March 2023; Accepted: 16 May 2023;
Published: 30 May 2023.
Edited by:
Jin Wang, Houston Methodist Research Institute, United StatesReviewed by:
Margaret A. Jordan, James Cook University, AustraliaBohua Chen, The Affiliated Hospital of Qingdao University, China
Yu Song, Huazhong University of Science and Technology, China
Copyright © 2023 Zhang, Zhang, Sun, Wang, Ning, Xu, Zhao, Yang, Xi and Tian. This is an open-access article distributed under the terms of the Creative Commons Attribution License (CC BY). The use, distribution or reproduction in other forums is permitted, provided the original author(s) and the copyright owner(s) are credited and that the original publication in this journal is cited, in accordance with accepted academic practice. No use, distribution or reproduction is permitted which does not comply with these terms.
*Correspondence: Jiwei Tian, tjw609@163.com; Xiaobing Xi, skxixiaobing@163.com; Kai Yang, cyan.kai@shsmu.edu.cn
†These authors have contributed equally to this work and share first authorship