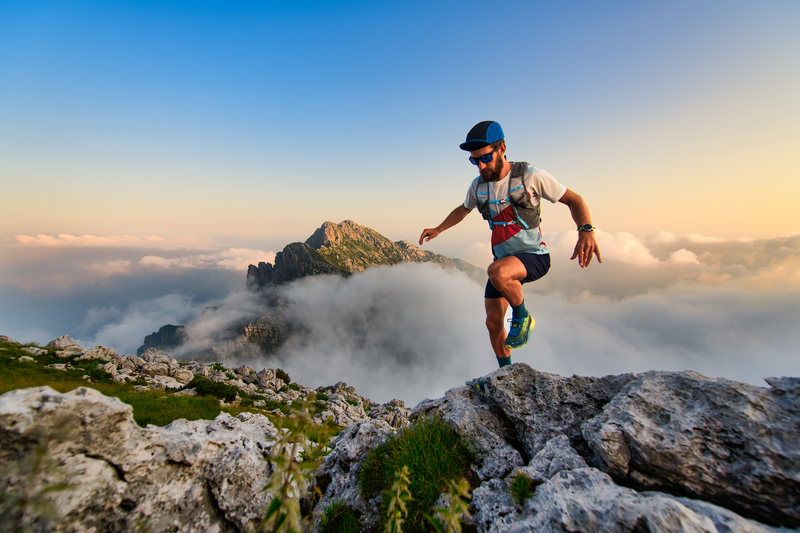
95% of researchers rate our articles as excellent or good
Learn more about the work of our research integrity team to safeguard the quality of each article we publish.
Find out more
SYSTEMATIC REVIEW article
Front. Immunol. , 18 September 2023
Sec. Viral Immunology
Volume 14 - 2023 | https://doi.org/10.3389/fimmu.2023.1184362
This article is part of the Research Topic Immune Correlates of Protection for Emerging Diseases – Lessons from Ebola and COVID-19 View all 21 articles
Background: The virus neutralization assay is a principal method to assess the efficacy of antibodies in blocking viral entry. Due to biosafety handling requirements of viruses classified as hazard group 3 or 4, pseudotyped viruses can be used as a safer alternative. However, it is often queried how well the results derived from pseudotyped viruses correlate with authentic virus. This systematic review and meta-analysis was designed to comprehensively evaluate the correlation between the two assays.
Methods: Using PubMed and Google Scholar, reports that incorporated neutralisation assays with both pseudotyped virus, authentic virus, and the application of a mathematical formula to assess the relationship between the results, were selected for review. Our searches identified 67 reports, of which 22 underwent a three-level meta-analysis.
Results: The three-level meta-analysis revealed a high level of correlation between pseudotyped viruses and authentic viruses when used in an neutralisation assay. Reports that were not included in the meta-analysis also showed a high degree of correlation, with the exception of lentiviral-based pseudotyped Ebola viruses.
Conclusion: Pseudotyped viruses identified in this report can be used as a surrogate for authentic virus, though care must be taken in considering which pseudotype core to use when generating new uncharacterised pseudotyped viruses.
Serological assays are an invaluable tool in detecting exposure of pathogens in organisms and understanding the immune system’s response. The level of insight gained from these assays during a disease outbreak is crucial for the initial medical response, and subsequently understanding the dynamics, strength and longevity of the immune response (1–3). An important protective response requires antibody interaction with the pathogen. Upon infection, the humoral response produces antibodies that bind to the antigens displayed by the pathogen, including those that prevent interaction with the receptors necessary for entry into host cells. Assays for antibody analysis have proved effective during recent viral outbreaks, such as those caused by Ebola virus (4, 5) and Severe Acute Respiratory Coronavirus 2 virus (SARS-CoV-2) (6–8), as they allow for detection and monitoring of viral spread in a population. Such assays are similarly applied to animals, which can also identify intermediary hosts or potential reservoirs and provide information about the potential for zoonotic spillover (9, 10), as well as inform on vaccines and treatment efficacy in preclinical studies.
Some serological assays, such as enzyme-linked immuno-absorbance assays (ELISA), can identify the presence of antigen-binding antibodies within a day of receiving a human or animal blood sample (11, 12). When considering antibodies targeting a viral glycoprotein, typically a proportion of the binding antibodies to a viral glycoprotein successfully impair the virus entry, whilst other antibodies bind to non neutralising epitopes, enabling other antibody-mediated immune functions (13). This highlights a shortcoming of binding assays such as ELISAs which lack the functional component of measuring virus entry into cells. Owing to this, in order to measure functional activity, specifically the ability of antibodies in preventing entry, a neutralisation assay is required. These assays are considered the gold standard for measuring the presence and magnitude of neutralising antibodies and typically require the use of authentic virus (14). As a result, these assays often take several days to allow the virus to grow and are subject to biosafety containment requirements depending on the virus under investigation. This restricts the study of viruses classified as hazard group 3 or 4, such as SARS-CoV-2 or Ebola virus and Nipah virus, due to the paucity of facilities that possess such high level of biocontainment. An approach to circumvent these requirements is to use a pseudotyped virus (PV), which can be handled at containment level 2 or below (Figure 1). These are comparatively easier to produce, typically by plasmid transfections, and, under optimized conditions, can be produced within 3 to 5 days. Many reviews have been published regarding pseudotype production, core composition, and their uses (15–20). These chimeric viruses commonly use a retroviral or VSV nucleocapsid core are surrounded by a lipid envelope bearing viral glycoproteins of a heterologous virus of interest on their surface. Often, PVs do not contain the virus genomic material required for replication. Instead, the modified genome is replaced by a transgene, for example a reporter gene such as green fluorescent protein (GFP) or luciferase enzyme (16). Upon successful entry into target cells, transgene expression allows for quantification of infected cells. Primarily due to their replication deficiency, PVs can be handled in a containment level 2 laboratories, which are common facilities in biological research laboratories (18). Many viruses of high consequence have been pseudotyped successfully and rapidly during the onset of an outbreak, as authentic viruses typically require isolation and stock amplification, whereas PVs require a published sequence of the viral glycoprotein to be cloned into an expression plasmid. Due to their external mimicry of the virus of interest, with reduced risk of acquiring mutations during production in mammalian tissue culture as seen with authentic viruses, PVs are an effective tool to use in neutralisation assays (18, 19). The COVID-19 global pandemic, caused by SARS-CoV-2, caused a significant rise in the use of pseudotype assays for both serology and molecular virology studies (17, 21). When PVs are used in a multi-well plate assay setting they are often referred to as pseudotype virus microneutralisation assays (pMNA). For the purposes of this systematic review, the alternative authentic virus microneutralisation assay will be referred to as vMNA.
Figure 1 Comparison between live virus neutralisation assay and pseudotyped neutralisation assays. Live viruses are commonly used in neutralisation assays though their practicality may depend on the biohazard containment regulations (A). Pseudotyped viruses, despite displaying glycoproteins of highly pathogenic viruses, are designated as a level 2 pathogen (B). The live virus neutralisation assay and the pseudotyped virus neutralisation assay are designed in a similar fashion whereby antibodies are incubated in the presence of virus, followed by addition of a cell line that is susceptible to virus infection (C). In the context of a SARS-CoV-2 neutralisation assay (D), neutralising antibodies bind to the Spike protein of the virus, preventing the virus to bind to the required entry receptor ACE2. Live viruses that enter begin to replicate, whereas pseudotyped viruses only express the desired reporter gene. Plaque assays, fluorescent staining of viral proteins or qPCR are often used to measure neutralisation levels in live virus assays (E), whereas pseudotyped assays typically rely on measuring the intensities of luciferase or fluorescent protein expression (F). The pertinent question of whether the results derived from either assay correlate still remain (G). Figure created with Biorender.com.
Given that neutralising antibodies are one of the principle components measured to determine correlates or surrogates of protection against disease or infection (22–24), the neutralisation test remains a critical assay. An important aspect when determining a correlate or surrogate of protection is to be able to draw comparisons between data and bridge between studies. By calibrating assays to a common reference reagent, often a pooled sera sample, assay readouts can be standardised across laboratories worldwide as these relative results are reported in a standard unitage (25–27). It is important that such common reagent is used correctly to calibrate in house standards, but in some cases, this is still not enough and the reduction of inter-laboratory variation can only be achieved by sharing common protocols and critical reagents similar to the approach used by the CEPI Centralised Laboratories network. Such reference reagents have been produced for several viruses, including many of high consequence which are applicable to pseudotyping (28–30). Whilst reporting results relative to a reference reagent reduces inter-laboratory variations and allows comparisons between assays, it is fundamentally important to investigate whether surrogate assays, designed to mimic and replace vMNAs which employ highly pathogenic viruses, correlate. If there is a correlation between a pMNA and a vMNA, then the results from either assay could be applied within clinical trials and investigations aimed at identifying the correlates for protection against a virus.
However, it is commonly queried how well the results from a pMNA correlate with those from a vMNA. The question is particularly relevant with the increasing uptake of pMNAs as a consequence of the recent COVID-19 pandemic and their increasing application to clinical trials as focus turns to vaccine development for other high consequence pathogens (31, 32). The studies to-date use a mixture of correlation formulae, most of which are Pearson’s R and/or Spearman’s Rho (33, 34). Other studies have instead fitted linear regressions to understand the relationship between the two variables, with the R2 value providing an equivalent measure to the square of Pearson’s R in the case of a positive relationship (35). Several reviews on PVs or neutralisation assays have included some of these studies which sought to correlate results from both assays, yet only a handful are cited (17–19). Despite several studies directly comparing PV and authentic virus neutralization assays, correlation information tends to be buried in the mass of data or supplementary material in these reports. It is likely that for these reasons, the question as to whether the two assays correlate is still frequently posed.
To the best of our knowledge, there is no systematic review nor meta-analysis that has condensed the literature that has correlated pMNA and vMNA. Therefore, the purpose of this systematic review and meta-analysis is to collect the available information on the comparison between the two tests, analyse the strength of correlations, and present the results in a clear and coherent manner. Overall, we aim to inform the wider community whether pseudotyped viruses can be used as surrogates for authentic virus for the purposes of a neutralisation assay and subsequently to determine the correlates of protection against a virus. Despite the findings within this report, it remains critical that PV-based assays continue to be assayed and correlated with authentic virus wherever possible, particularly if a new PV has been designed for use. Given that correlation coefficient values have different classifications of strength based on the field of study, we included a table based on the definitions that are often cited in the field of medicine (34, 36, 37) (Table 1).
Google Scholar and PubMed were used to identify published research articles which reported data on correlation between pMNA and vMNAs. The following Boolean search terms were employed to filter studies indexed in Google Scholar and PubMed: “pseudotype|pseudotyped|pseudoparticle” “correlate|correlated|correlation” “live” “virus” “neutralisation|neutralization”.
The criteria for inclusion were reports that contained neutralisation assays with both pseudotype virus and authentic virus, as well as application of a mathematical formula to assess the relationship between the results, either by linear regression, Pearson’s correlation, Spearman’s rank, or a combination of the three. Studies that did not present any form of analysis of correlation were excluded.
We extracted the following data from reports that satisfied our selection criteria: report author name and year, virus used, pseudotype core used, neutralisation assay readout (both for pMNA and vMNA), correlation method, p value of the correlation coefficients, number of samples, and sample types. In total, we identified 67 reports that satisfied our selection criteria and were used for comparative data analysis.
For our meta-analysis, we considered data for the relationships between SARS-CoV-2 PVs and authentic virus. There was insufficient data to consider other viruses in separate meta-analyses and we decided not to analyse the results from multiple viruses together. We instead present the data for other viruses in a table in the supplementary materials (Suppl. Table 1). For the studies reporting a linear regression (R2), we opted to convert the value by its square-root, so that it may be combined with the Pearson’s R values derived from other studies and therefore included in the analysis. We checked that all regressions reported only included the PVs and authentic virus and that the relationships were all positive. We did not have sufficient Spearman’s Rho values to analyse and these cannot be directly combined with the Pearson’s R values, as they do not measure the same characteristic. Therefore, we did not attempt to carry out a meta-analysis of Spearman’s Rho coefficients. These values are reported in the supplementary materials (Suppl. Table 1). We therefore used a dataset of 50 Pearson’s R coefficients from 22 papers. Since studies on SARS-CoV-2 used different PV cores (HIV and VSV), PV assays (eGFP, GFP, Luciferase, PRNT and SEAP) and sample types (hamster sera, human mAbs, human plasma and human sera), we checked for differences in the Pearson’s correlations between studies using t-tests with a null hypothesis of no difference in the mean Pearson’s correlations between the groups containing at least 10 results (Suppl. Figure 1). Since we failed to reject the null hypothesis for any comparison, we decided to carry out our meta-analysis on the full dataset. We had only very limited results reported for different SARS-CoV-2 variants, so that investigating differences in results for each variant alone is left for future work. The analysed datasets used identical variants for PV and authentic viruses.
We conducted a three-level meta-analysis of Fisher’s z-transformed Pearson’s correlations, using the inverse-variance method, accounting for the dependence between multiple results from the same study (38, 39). We assigned data to “clusters” based on their dependence on other data. All coefficients calculated using the same dataset were considered dependent and were assigned to the same cluster, resulting in 26 clusters in total. Taking the example of Wang et al, 2020 (40), a correlation coefficient was calculated for each of two independent datasets, so that these two coefficients were assigned to separate clusters, while Sholukh et al, 2021 (41) presented four correlation coefficients that were calculated using the same datasets, so that these coefficients were all assigned to the same cluster. Clusters with higher estimated sampling variance of their correlation coefficients, e.g., due to lower sample sizes, are given lower weights in the calculation of the pooled correlation, while clusters are given higher weights if there is less dependence among their correlation coefficients (39). The heterogeneity variance, τ2, was calculated using the restricted maximum likelihood estimator, with confidence interval estimates calculated using the profile likelihood method. We assessed heterogeneity using the I2 and H statistics (42) and we calculated prediction intervals (using the t-distribution) for the pooled correlation estimate. While confidence intervals provide measures of uncertainty around the true mean values of correlation, the prediction interval provides a measure of uncertainty around the likely values of correlation to be seen in future studies (38). We checked for influential outliers by removing correlations in turn and recalculating all estimates. We plotted Fisher’s z-transformed correlation against standard error (a “funnel plot”) to assess possible publication bias. All calculations were carried out in R version 4.3.1 (R Core Team, 2022) using the packages meta (43), metafor (44) and dmetar (45).
Our search terms returned a total of 33 reports in PubMed and 5,880 reports in Google Scholar. After manually screening abstracts and titles, we identified 80 studies that met our selection criteria and ultimately included 67 reports in this systematic review (Suppl. Table 1). The primary reason for exclusion were reports that either did not include both pMNA and vMNA, or reported neutralisation titres in both the pMNA and vMNA, but did not carry out a correlation analysis between the two methods. Briefly, the total number of reports found for each virus were; SARS-CoV-2 (n=32) (40, 41, 46–75), SARS-CoV-1 (n=2) (76, 77), Canine distemper virus (CDV, n=1) (78), Chikungunya virus (CHIKV, n=1) (79), European bat lyssavirus 1 (EBLV-1, n=1) (80), EBLV-2 (n=1) (80), Ebola virus (EBOV, n=3) (81–83), Hepatitis C virus (HCV, n=3) (84–86), Human immunodeficiency virus (HIV, n=1) (87), Hantaan orthohantavirus (HTNV, n=2) (88, 89), Influenza A virus H5N1 (IAV H5N1, n=5) (90–94), IAV H7N9 (n=1) (95), Japanese encephalitis virus (JEV, n=1) (96), Lagos bat virus (LBV, n=1) (97), Middle East respiratory syndrome virus (MERS, n=4) (98–101), Newcastle disease virus (NDV, n=1) (102), Nipah virus (NIV, n=1) (103), Peste des petite ruminants virus (PPRV, n=1) (104), Puumala virus (PUUV, n=1) (105), Rift Valley fever virus (RVF, n=1) (106), Rabies virus (RABV, n=2) (107, 108), and Seoul orthohantavirus (SEOV, n=2) (88, 89). A summary of the findings from these reports can be viewed in Table 2, whereas a more detailed breakdown for each report can be viewed in the supplementary file (Suppl. Table 1).
Table 2 Summary of reported correlation coefficients. The bounds represent the minimum and maximum point values across the studies.
Aside from SARS-CoV-2 which will be analysed in the following sections of this study, we found that in general, most of the pseudotypes correlated well with the vMNA, irrespective of pseudotype cores and the readout techniques used to measure the assay results (Suppl. Table 1.). We found some studies that did not clarify the correlation test used, and were therefore omitted from Table 2, though relevant information including the r value is still included in the Supplementary Table 1. Interestingly, a study analysing the EBOV PVs reported that the choice of the PV core had a substantial impact on correlation with authentic virus (82, 83). When the negative control samples were omitted from the neutralisation assays, the correlation coefficients dropped from 0.68, 0.77 to -0.03 and 0.18, effectively showing no correlation, whereas the samples assayed with the VSV core PVs retained correlation coefficients of 0.84 and 0.96 (Suppl. Table 1.). This study highlights the need to consistently verify whether cores of pseudotypes can affect correlations with vMNAs.
From 22 SARS-CoV-2 studies we analysed 50 Pearson’s correlation coefficients, which were derived from a combined total of 1238 data points by pMNA and vMNA (Figure 2). As stated in the methods, we verified that there were no significant differences in the mean Pearson’s correlation values between studies that used different PV cores, neutralising reagents and assay readout types (Suppl. Figure 1). We calculated a pooled correlation of 0.86 (95% CI; 0.82-0.89, p < 0.01). These results suggest that there is a strong correlation between the results derived by pMNA and vMNA.
The results indicated the presence of low to moderate between-cluster heterogeneity [I2 = 37.1% (CI: 11.2%-55.5%); H=1.26 (CI: 1.06 to 1.50); τ2 = 0.05 (CI: 0.02-0.12)]. This means that there is some weak evidence of differences in the true effect sizes in the study. A 95% prediction interval (PI) for the pooled correlation is 0.69-0.94, which means that it is highly likely that the true correlation between pMNA and vMNA in a future study will lie between 0.69 and 0.94. Since this is entirely greater than 0.5, this provides us with evidence of a positive relationship between pMNA and vMNA for SARS-CoV-2, appropriately accounting for the distribution of effects amongst the studies. Removing results in turn did not lead to substantial reductions in heterogeneity. Our “funnel plot” (Suppl. Figure 2) shows that most points lie within the funnel shape in a symmetrical pattern, providing no evidence of publication bias.
Our “forest plot” (Figure 3) shows the calculated interval estimates for each study. We note that the majority of the interval estimates include our pooled estimate and that all studies except Mykytyn et al. (61), which has very small reported sample sizes, have entirely positive interval estimates.
Figure 3 Forest Plot of the three-level meta-analysis results. The endpoints of the black or white horizontal lines represent the endpoints of the 95% CIs for the Pearson’s correlation coefficients for each study. The grey boxes represent the sample sizes of each study. The vertical dotted line represents the pooled Pearson’s correlation coefficient estimate and the grey diamond represents the 95% CI for the pooled Pearson’s correlation coefficient estimate. The 95% prediction interval is shown by the red line. The table columns are, respectively, study name, cluster indicator, sample size (n) from which Pearson’s correlation coefficient was calculated, correlation as described above, Pearson’s correlation coefficients, 95% CI of Pearson’s correlation coefficients, and weighting assigned to each coefficient.
Since Pearson’s or Spearman’s correlation coefficients are used for understanding correlation between two variables, they may not determine whether different assays are strictly in agreement with each other. The Bland-Altman method (109) is a frequently applied analysis which is often used to determine agreement between two methods that aim to measure the same variable, in this case, antibody neutralising capability. Within our literature search, several studies have used the Bland-Altman method of analysis. Therefore, we also refined the literature search used for this study by adding the search terms; “Bland-Altman”. All four resulting papers identified were already included from the main literature search. Due to the power of this statistical method, we opted to present the results by the Bland-Altman method within the reports in a separate table (Table 3). All studies that reported results from the Bland-Altman method showed high levels of agreement between pMNA and vMNA.
Given the interest in the results derived by pMNA compared to vMNA, our systematic review and meta-analysis sought to consolidate the data to inform the wider community on whether there is a correlation and subsequently, agreement between the two assays. The results of the meta-analysis would confirm that for SARS-CoV-2 there is a strong degree of correlation between pMNA and vMNA. Despite the limited number of studies, the Bland-Altman results presented in this manuscript also indicate a high level of agreement between the two assays. This data support the use of pMNA as a surrogate to the vMNA, though more correlation studies by Bland-Altman would be very valuable to perform in future reports.
Moreover, since multiple viral cores can be used for pseudotyping, it is important to assess whether this could impact the pMNA vs vMNA correlation. It would appear that in the case of the Ebola virus, there is a lower concordance, if a lentiviral core is used in the pMNA compared with a VSV core (82, 83). Whilst the precise reason for influence of the core remains unknown, though speculated to be due to the morphological difference between a VSV capsid and a filamentous EBOV particle (82) or the target cells, which is the same for the authentic virus and EBOV-VSV but differ for the lenti-based pMNA. It will be important to determine whether these differences exist in the case of other filoviruses and indeed other viruses, as there may be a high risk of reporting erroneous results. Therefore, it is important to optimize all aspects of the pMNA and different pseudotype cores combined with identical envelope glycoproteins should always be assessed in parallel with the authentic virus in neutralization tests, if possible. Critically, the two EBOV studies observed the reduced correlation of the lentiviral cores when negative control sera were excluded from their analyses. Therefore, we advise future correlation studies to consider not only including negative control samples within their analyses, but also consider deriving correlations with and without the negative control samples, especially if the number of samples is low and multiple cores are under assessment.
Interestingly, multiple studies have mentioned that one of the benefits of using PVs is that they are more sensitive in discriminating samples containing weaker or a low concentration of neutralising antibodies (92, 100, 104). In fact, one report provided evidence of the vMN assay reporting false negative results on samples that contained neutralising antibodies, successfully detected by the pMN (102). Whilst this would highlight the benefits of using PVs for detecting positive samples within a human or animal population, it is may also bring into question whether the results derived from the weaker samples could protect the individual or animal from subsequent infection, given that the authentic virus was not neutralised. However, it is essential to consider that lower limits of detections can change based on assay design, virus species, the titre of the virus used, and the volume of serum sample used. This highlights reporting of results relative to a reference reagent can add value by enabling comparisons between data produced by different methods. Whilst use of a reference material will not ultimately improve assay performance, it helps to highlight differences. In any case, having a more sensitive assay such as the pMNA would prove to be very useful for epidemiological studies that are aiming to determine whether a virus exists or existed in a particular human or animal population, as opposed to correlating neutralising titres towards disease severity or protection.
Lastly, it is very important to distinguish the type of interpretation derived from either Pearson’s R or Spearman’s rank correlation analyses and the Bland-Altman plot. Neither the Pearson’s R, which is a measure of the linear relationship between two variables, nor the Spearman’s rank, that informs on correlation from measurements taken on an ordinal scale, provide information on the agreement between two different assays. In this case, the Bland-Altman method is required (109). Our literature search has shown for multiple viruses that the pMNA and vMNA have high agreement for multiple viruses in several families.
The main limitation of our systematic review is that it was biased towards SARS-CoV-2, due to the sheer number of publications dedicated to this virus in the past three years, providing enough correlation values that allowed for the meta-analysis. Whilst it would have been useful to carry out the same analysis for other viruses, unfortunately there were not enough correlation values. We did not use the Spearman’s Rho coefficients in our analyses, but the strong positive values of these, for both SARS-CoV-2 and other viruses (Suppl. Table 1) do not disagree with our main conclusions that PVs and authentic virus showed strong positive relationships. Some of the studies used very small sample sizes, which was accounted for through giving lower weights to these studies. We opted to include studies that used PVs that are non-replicative, single cycle of infection, therefore excluding studies that used replicon infection systems, despite some of these reports showing high correlation and high level of agreement between single-round replicons and authentic virus in a neutralisation assay (110, 111). Lastly, new virus and cell-free assays have now been developed for SARS-CoV-2 that measure the capability of antibodies blocking the spike protein from interacting with its receptor ACE-2, effectively becoming a surrogate neutralisation assay, have shown to have strong correlations with both pMNAs and vMNAs (51, 69, 112–114). Whilst these assays do not fit the scope of this study, we believe it is worth mentioning and monitoring for follow up meta-analyses.
In summary, our systematic review and meta-analysis shows that the pMNA designed for use towards SARS-CoV-2 serological studies demonstrated a high degree of correlation with assays performed using the authentic virus. In addition, many other viruses that have been pseudotyped also show a high degree of correlation. We recommend, where possible, that future studies on methods agreement should continue to investigate the use of multiple PV cores, to determine whether there could be differences in neutralisation titres, such as that exemplified with Ebola virus PVs. It is also essential that future studies incorporate the Bland-Altman analysis to determine the agreement between the two assays as well as this is substantially more informative, especially when both assay results are to be applied to clinical trials and assessed for determining correlates of protection. Ultimately, we would encourage laboratories to calibrate assays to reference materials, if one is available and relevant for the isolate under study, which will support these future comparisons and critically provide traceability to a correlate of protection once derived.
The original contributions presented in the study are included in the article/Supplementary Material. Further inquiries can be directed to the corresponding author.
DC and NT conceptualised the study. DC, CW, EB, MM-N, EW, SS, SR, JC-O, JH, GM assisted in the literature search and proof-reading of the manuscript. CW and SR carried out the statistical analysis. GM, JC-O, JH and NT provided critical evaluation of the manuscript. All authors contributed to the article and approved the submitted version.
Funding was provided by UKRI (MC_PC_20016) and NIHR (COV0170 – HICC: Hummoral Immune Correlates for COVID-19), SARS-CoV-2 Immunity and Reinfection Evaluation (SIREN) study (UKRI: MR/W02067X/1) and Wellcome Trust (360G-Wellcome-220981/Z/20/Z). DC is supported by core funding the MRC-University of Glasgow Centre for Virus Research (MC_UU_00034/1).
We would like to acknowledge the Hummoral Immune Correlates of COVID-19 (HICC) consortium and the SARS-CoV-2 Immunity and reinfection evaluation (SIREN) consortium.
JH is a founder and a shareholder in DIOSynVax Ltd.
The remaining authors declare that the research was conducted in the absence of any commercial or financial relationships that could be construed as a potential conflict of interest.
All claims expressed in this article are solely those of the authors and do not necessarily represent those of their affiliated organizations, or those of the publisher, the editors and the reviewers. Any product that may be evaluated in this article, or claim that may be made by its manufacturer, is not guaranteed or endorsed by the publisher.
The Supplementary Material for this article can be found online at: https://www.frontiersin.org/articles/10.3389/fimmu.2023.1184362/full#supplementary-material
1. Katz JM, Hancock K, Xu X. Serologic assays for influenza surveillance, diagnosis and vaccine evaluation. Expert Rev Anti-infective Ther (2011) 9:669–83. doi: 10.1586/eri.11.51
2. Haselbeck AH, Im J, Prifti K, Marks F, Holm M, Zellweger RM. Serology as a tool to assess infectious disease landscapes and guide public health policy. Pathogens (2022) 11:732. doi: 10.3390/pathogens11070732
3. Krammer F, Simon V. Serology assays to manage COVID-19. Science (2020) 368:1060–1. doi: 10.1126/science.abc1227
4. Mather S, Scott S, Temperton N, Wright E, King B, Daly J. Current progress with serological assays for exotic emerging/re-emerging viruses. Future Virol (2013) 8:745–55. doi: 10.2217/fvl.13.60
5. MacNeil A, Reed Z, Rollin PE. Serologic cross-reactivity of human igM and igG antibodies to five species of ebola virus. PloS Negl Trop Dis (2011) 5:e1175. doi: 10.1371/journal.pntd.0001175
6. Lou B, Li T-D, Zheng S-F, Su Y-Y, Li Z-Y, Liu W, et al. Serology characteristics of SARS-CoV-2 infection after exposure and post-symptom onset. Eur Respir J (2020) 56(2):2000763. doi: 10.1183/13993003.00763-2020
7. Theel ES, Slev P, Wheeler S, Couturier MR, Wong SJ, Kadkhoda K. The role of antibody testing for SARS-coV-2: is there one? J Clin Microbiol (2020) 58:e00797–20. doi: 10.1128/JCM.00797-20
8. Amanat F, Stadlbauer D, Strohmeier S, Nguyen THO, Chromikova V, McMahon M, et al. A serological assay to detect SARS-CoV-2 seroconversion in humans. Nat Med (2020) 26:1033–6. doi: 10.1038/s41591-020-0913-5
9. Ogawa H, Miyamoto H, Nakayama E, Yoshida R, Nakamura I, Sawa H, et al. Seroepidemiological prevalence of multiple species of filoviruses in fruit bats (Eidolon helvum) migrating in Africa. J Infect Dis (2015) 212:S101–8. doi: 10.1093/infdis/jiv063
10. Spengler JR, Bergeron É, Rollin PE. Seroepidemiological studies of crimean-congo hemorrhagic fever virus in domestic and wild animals. PloS Negl Trop Dis (2016) 10:e0004210. doi: 10.1371/journal.pntd.0004210
11. Lequin RM. Enzyme immunoassay (EIA)/enzyme-linked immunosorbent assay (ELISA). Clin Chem (2005) 51:2415–8. doi: 10.1373/clinchem.2005.051532
12. Crowther JR. The ELISA Guidebook. Totowa, NJ: Humana Press (2009). doi: 10.1007/978-1-60327-254-4
13. Lu LL, Suscovich TJ, Fortune SM, Alter G. Beyond binding: antibody effector functions in infectious diseases. Nat Rev Immunol (2018) 18:46–61. doi: 10.1038/nri.2017.106
14. Liu K-T, Han Y-J, Wu G-H, Huang K-YA, Huang P-N. Overview of neutralization assays and international standard for detecting SARS-CoV-2 neutralizing antibody. Viruses (2022) 14:1560. doi: 10.3390/v14071560
15. Li Q, Liu Q, Huang W, Li X, Wang Y. Current status on the development of pseudoviruses for enveloped viruses. Rev Med Virol (2018) 28:e1963. doi: 10.1002/rmv.1963
16. Joglekar AV, Sandoval S. Pseudotyped lentiviral vectors: one vector, many guises. Hum Gene Ther Methods (2017) 28:291–301. doi: 10.1089/hgtb.2017.084
17. Cantoni D, Mayora-Neto M, Temperton N. The role of pseudotype neutralization assays in understanding SARS CoV-2. Oxford Open Immunol (2021) 2(1):iqab005. doi: 10.1093/oxfimm/iqab005
18. Bentley EM, Mather ST, Temperton NJ. The use of pseudotypes to study viruses, virus sero-epidemiology and vaccination. Vaccine (2015) 33:2955–62. doi: 10.1016/j.vaccine.2015.04.071
19. Toon K, Bentley EM, Mattiuzzo G. More than just gene therapy vectors: lentiviral vector pseudotypes for serological investigation. Viruses (2021) 13:217. doi: 10.3390/v13020217
20. Sanders DA. No false start for novel pseudotyped vectors. Curr Opin Biotechnol (2002) 13:437–42. doi: 10.1016/S0958-1669(02)00374-9
21. Lamikanra A, Nguyen D, Simmonds P, Williams S, Bentley EM, Rowe C, et al. Comparability of six different immunoassays measuring SARS-CoV-2 antibodies with neutralizing antibody levels in convalescent plasma: From utility to prediction. Transfusion (2021) 61:2837–43. doi: 10.1111/trf.16600
22. Plotkin SA. Correlates of protection induced by vaccination. Clin Vaccine Immunol (2010) 17:1055–65. doi: 10.1128/CVI.00131-10
23. Huang AT, Garcia-Carreras B, Hitchings MDT, Yang B, Katzelnick LC, Rattigan SM, et al. A systematic review of antibody mediated immunity to coronaviruses: kinetics, correlates of protection, and association with severity. Nat Commun (2020) 11:4704. doi: 10.1038/s41467-020-18450-4
24. Castillo-Olivares J, Wells DA, Ferrari M, Chan ACY, Smith P, Nadesalingam A, et al. Towards Internationally standardised humoral Immune Correlates of Protection from SARS CoV 2 infection and COVID-19 disease. Front Immunol (2021) 12:748291. doi: 10.1101/2021.05.21.21257572
25. Rampling T, Page M, Horby P. International biological reference preparations for epidemic infectious diseases. Emerg Infect Dis (2019) 25:205–11. doi: 10.3201/eid2502.180798
26. WHO. WHO Expert Committee on Biological Standardization: Sixtieth Report. Geneva, Switzerland: World Health Organization (2013). p. 241.
27. Page M, Wilkinson DE, Mattiuzzo G, Efstathiou S, Minor P. Developing biological standards for vaccine evaluation. Future Virol (2017) 12:431–7. doi: 10.2217/fvl-2017-0003
28. Mattiuzzo G, Bentley EM, Page M. The role of reference materials in the research and development of diagnostic tools and treatments for haemorrhagic fever viruses. Viruses (2019) 11:781. doi: 10.3390/v11090781
29. Kristiansen PA, Page M, Bernasconi V, Mattiuzzo G, Dull P, Makar K, et al. WHO International Standard for anti-SARS-CoV-2 immunoglobulin. Lancet (2021) 397:1347–8. doi: 10.1016/S0140-6736(21)00527-4
30. Dowall SD, Kempster S, Findlay-Wilson S, Mattiuzzo G, Graham VA, Page M, et al. Towards quantification of protective antibody responses by passive transfer of the 1st WHO International Standard for Ebola virus antibody in a Guinea pig model. Vaccine (2020) 38:345–9. doi: 10.1016/j.vaccine.2019.10.009
31. Background to the WHO R&D blueprint pathogens . Available at: https://www.who.int/observatories/global-observatory-on-health-research-and-development/analyses-and-syntheses/who-r-d-blueprint/background (Accessed February 28, 2023).
32. CEPI. New Vaccines For A Safer World . CEPI. Available at: https://cepi.net/ (Accessed February 28, 2023).
33. Benesty J, Chen J, Huang Y, Cohen I. Pearson Correlation Coefficient. In: Noise Reduction in Speech Processing. Springer Topics in Signal Processing. Berlin, Heidelberg: Springer Berlin Heidelberg (2009). p. 1–4. doi: 10.1007/978-3-642-00296-0_5
34. Akoglu H. User’s guide to correlation coefficients. Turkish J Emergency Med (2018) 18:91–3. doi: 10.1016/j.tjem.2018.08.001
35. Montgomery DC, Peck EA, Vining GG. Introduction to Linear Regression Analysis. Hoboken, N.J.: John Wiley & Sons (2021). p. 706.
37. Mukaka MM. A guide to appropriate use of Correlation coefficient in medical research. Malawi Med J (2012) 24:69–71. doi: 10.4314/mmj.v24i3
38. Harrer M, Cuijpers P, Furukawa T, Ebert D. Doing Meta-Analysis with R: A Hands-On Guide. New York: Chapman and Hall/CRC (2021). p. 500. doi: 10.1201/9781003107347
39. Van den Noortgate W, López-López JA, Marín-Martínez F, Sánchez-Meca J. Three-level meta-analysis of dependent effect sizes. Behav Res (2013) 45:576–94. doi: 10.3758/s13428-012-0261-6
40. Wang P, Liu L, Nair MS, Yin MT, Luo Y, Wang Q, et al. SARS-CoV-2 neutralizing antibody responses are more robust in patients with severe disease. Emerging Microbes Infections (2020) 9:2091–3. doi: 10.1080/22221751.2020.1823890
41. Sholukh AM, Fiore-Gartland A, Ford ES, Miner MD, Hou YJ, Tse LV, et al. Evaluation of Cell-based and surrogate SARS-CoV-2 neutralization assays. J Clin Microbiol (2021) 59:e0052721. doi: 10.1128/JCM.00527-21
42. Higgins JPT, Thompson SG. Quantifying heterogeneity in a meta-analysis. Stat Med (2002) 21:1539–58. doi: 10.1002/sim.1186
43. Balduzzi S, Rücker G, Schwarzer G. How to perform a meta-analysis with R: a practical tutorial. Evid Based Ment Health (2019) 22:153–60. doi: 10.1136/ebmental-2019-300117
44. Viechtbauer W. Conducting meta-analyses in R with the metafor package. J Stat Software (2010) 36:1–48. doi: 10.18637/jss.v036.i03
45. Companion R Package for the Guide Doing Meta-Analysis in R . Available at: https://dmetar.protectlab.org/ (Accessed February 27, 2023).
46. Atti A, Insalata F, Carr EJ, Otter AD, Castillo-Olivares J, Wu M, et al. Antibody correlates of protection from SARS-CoV-2 reinfection prior to vaccination: A nested case-control within the SIREN study. J Infect (2022) 85:545–56. doi: 10.1016/j.jinf.2022.09.004
47. Bewley KR, Coombes NS, Gagnon L, McInroy L, Baker N, Shaik I, et al. Quantification of SARS-CoV-2 neutralizing antibody by wild-type plaque reduction neutralization, microneutralization and pseudotyped virus neutralization assays. Nat Protoc (2021) 16:3114–40. doi: 10.1038/s41596-021-00536-y
48. Chi X, Yan R, Zhang J, Zhang G, Zhang Y, Hao M, et al. A neutralizing human antibody binds to the N-terminal domain of the Spike protein of SARS-CoV-2. Science (2020) 369:650–5. doi: 10.1126/science.abc6952
49. Collier DA, Ferreira IATM, Kotagiri P, Datir RP, Lim EY, Touizer E, et al. Age-related immune response heterogeneity to SARS-CoV-2 vaccine BNT162b2. Nature (2021) 596:417–22. doi: 10.1038/s41586-021-03739-1
50. D’Apice L, Trovato M, Gramigna G, Colavita F, Francalancia M, Matusali G, et al. Comparative analysis of the neutralizing activity against SARS-CoV-2 Wuhan-Hu-1 strain and variants of concern: Performance evaluation of a pseudovirus-based neutralization assay. Front Immunol (2022) 13:981693. doi: 10.3389/fimmu.2022.981693
51. Fenwick C, Turelli P, Pellaton C, Farina A, Campos J, Raclot C, et al. A high-throughput cell- and virus-free assay shows reduced neutralization of SARS-CoV-2 variants by COVID-19 convalescent plasma. Sci Transl Med (2021) 13:eabi8452. doi: 10.1126/scitranslmed.abi8452
52. Grzelak L, Temmam S, Planchais C, Demeret C, Tondeur L, Huon C, et al. A comparison of four serological assays for detecting anti–SARS-CoV-2 antibodies in human serum samples from different populations. Sci Trans Med (2020) 12:eabc3103. doi: 10.1126/scitranslmed.abc3103
53. Harvala H, Robb ML, Watkins N, Ijaz S, Dicks S, Patel M, et al. Convalescent plasma therapy for the treatment of patients with COVID-19: Assessment of methods available for antibody detection and their correlation with neutralising antibody levels. Transfusion Med (2021) 31:167–75. doi: 10.1111/tme.12746
54. Hyseni I, Molesti E, Benincasa L, Piu P, Casa E, Temperton NJ, et al. Characterisation of SARS-coV-2 lentiviral pseudotypes and correlation between pseudotype-based neutralisation assays and live virus-based micro neutralisation assays. Viruses (2020) 12:1011. doi: 10.3390/v12091011
55. James J, Rhodes S, Ross CS, Skinner P, Smith SP, Shipley R, et al. Comparison of serological assays for the detection of SARS-CoV-2 antibodies. Viruses (2021) 13:713. doi: 10.3390/v13040713
56. Legros V, Denolly S, Vogrig M, Boson B, Siret E, Rigaill J, et al. A longitudinal study of SARS-CoV-2-infected patients reveals a high correlation between neutralizing antibodies and COVID-19 severity. Cell Mol Immunol (2021) 18:318–27. doi: 10.1038/s41423-020-00588-2
57. Li H, Zhao C, Zhang Y, Yuan F, Zhang Q, Shi X, et al. Establishment of replication-competent vesicular stomatitis virus-based recombinant viruses suitable for SARS-CoV-2 entry and neutralization assays. Emerging Microbes Infections (2020) 9:2269–77. doi: 10.1080/22221751.2020.1830715
58. Liu K-T, Gong Y-N, Huang C-G, Huang P-N, Yu K-Y, Lee H-C, et al. Quantifying neutralizing antibodies in patients with COVID-19 by a two-variable generalized additive model. mSphere (2022) 7:e00883–21. doi: 10.1128/msphere.00883-21
59. Maciola AK, La Raja M, Pacenti M, Salata C, De Silvestro G, Rosato A, et al. Neutralizing antibody responses to SARS-CoV-2 in recovered COVID-19 patients are variable and correlate with disease severity and receptor-binding domain recognition. Front Immunol (2022) 13. doi: 10.3389/fimmu.2022.830710
60. Merluza J, Ung J, Makowski K, Robinson A, Manguiat K, Mueller N, et al. Validation and establishment of the SARS-CoV-2 lentivirus surrogate neutralization assay as a prescreening tool for the plaque reduction neutralization test. Microbiol Spectr (2023) 11:e03789–22. doi: 10.1128/spectrum.03789-22
61. Mykytyn AZ, Rissmann M, Kok A, Rosu ME, Schipper D, Breugem TI, et al. Antigenic cartography of SARS-CoV-2 reveals that Omicron BA.1 and BA.2 are antigenically distinct. Sci Immunol (2022) 7:eabq4450. doi: 10.1126/sciimmunol.abq4450
62. Neerukonda SN, Vassell R, Herrup R, Liu S, Wang T, Takeda K, et al. Establishment of a well-characterized SARS-CoV-2 lentiviral pseudovirus neutralization assay using 293T cells with stable expression of ACE2 and TMPRSS2. PloS One (2021) 16:e0248348. doi: 10.1371/journal.pone.0248348
63. Newman J, Thakur N, Peacock TP, Bialy D, Elrefaey AME, Bogaardt C, et al. Neutralizing antibody activity against 21 SARS-CoV-2 variants in older adults vaccinated with BNT162b2. Nat Microbiol (2022) 7:1180–8. doi: 10.1038/s41564-022-01163-3
64. Nguyen D, Xiao J, Simmonds P, Lamikanra A, Odon V, Ratcliff J, et al. Effects of severe acute respiratory syndrome coronavirus 2 strain variation on virus neutralization titers: therapeutic use of convalescent plasma. J Infect Dis (2022) 225:971–6. doi: 10.1093/infdis/jiab563
65. Ni L, Ye F, Cheng M-L, Feng Y, Deng Y-Q, Zhao H, et al. Detection of SARS-CoV-2-specific humoral and cellular immunity in COVID-19 convalescent individuals. Immunity (2020) 52:971–977.e3. doi: 10.1016/j.immuni.2020.04.023
66. Noval MG, Kaczmarek ME, Koide A, Rodriguez-Rodriguez BA, Louie P, Tada T, et al. Antibody isotype diversity against SARS-CoV-2 is associated with differential serum neutralization capacities. Sci Rep (2021) 11:5538. doi: 10.1038/s41598-021-84913-3
67. Oguntuyo KY, Stevens CS, Hung CT, Ikegame S, Acklin JA, Kowdle SS, et al. Quantifying absolute neutralization titers against SARS-CoV-2 by a standardized virus neutralization assay allows for cross-cohort comparisons of COVID-19 sera. mBio (2021) 12:e02492–20. doi: 10.1128/mBio.02492-20
68. Schmidt F, Weisblum Y, Muecksch F, Hoffmann H-H, Michailidis E, Lorenzi JCC, et al. Measuring SARS-CoV-2 neutralizing antibody activity using pseudotyped and chimeric viruses. J Exp Med (2020) 217(11):e20201181. doi: 10.1084/jem.20201181
69. Tan CW, Chia WN, Qin X, Liu P, Chen MI-C, Tiu C, et al. A SARS-CoV-2 surrogate virus neutralization test based on antibody-mediated blockage of ACE2–spike protein–protein interaction. Nat Biotechnol (2020) 38:1073–8. doi: 10.1038/s41587-020-0631-z
70. Wohlgemuth N, Whitt K, Cherry S, Kirkpatrick Roubidoux E, Lin C-Y, Allison KJ, et al. An assessment of serological assays for SARS-coV-2 as surrogates for authentic virus neutralization. Microbiol Spectr (2021) 9:e01059–21. doi: 10.1128/Spectrum.01059-21
71. Xiong H-L, Wu Y-T, Cao J-L, Yang R, Liu Y-X, Ma J, et al. Robust neutralization assay based on SARS-CoV-2 S-protein-bearing vesicular stomatitis virus (VSV) pseudovirus and ACE2-overexpressing BHK21 cells. Emerging Microbes Infections (2020) 9:2105–13. doi: 10.1080/22221751.2020.1815589
72. Yang R, Huang B AR, Li W, Wang W, Deng Y, Tan W. Development and effectiveness of pseudotyped SARS-CoV-2 system as determined by neutralizing efficiency and entry inhibition test in vitro. Biosaf Health (2020) 2:226–31. doi: 10.1016/j.bsheal.2020.08.004
73. Yu J, Li Z, He X, Gebre MS, Bondzie EA, Wan H, et al. Deletion of the SARS-coV-2 spike cytoplasmic tail increases infectivity in pseudovirus neutralization assays. J Virol (2021) 95:e00044–21. doi: 10.1128/JVI.00044-21
74. Zettl F, Meister TL, Vollmer T, Fischer B, Steinmann J, Krawczyk A, et al. Rapid quantification of SARS-CoV-2-neutralizing antibodies using propagation-defective vesicular stomatitis virus pseudotypes. Vaccines (2020) 8:386. doi: 10.3390/vaccines8030386
75. von Rhein C, Scholz T, Henss L, Kronstein-Wiedemann R, Schwarz T, Rodionov RN, et al. Comparison of potency assays to assess SARS-CoV-2 neutralizing antibody capacity in COVID-19 convalescent plasma. J Virol Methods (2021) 288:114031. doi: 10.1016/j.jviromet.2020.114031
76. Temperton NJ, Chan PK, Simmons G, Zambon MC, Tedder RS, Takeuchi Y, et al. Longitudinally profiling neutralizing antibody response to SARS coronavirus with pseudotypes. Emerg Infect Dis (2005) 11:411–6. doi: 10.3201/eid1103.040906
77. Fukushi S, Mizutani T, Saijo M, Kurane I, Taguchi F, Tashiro M, et al. Evaluation of a novel vesicular stomatitis virus pseudotype-based assay for detection of neutralizing antibody responses to SARS-CoV. J Med Virol (2006) 78:1509–12. doi: 10.1002/jmv.20732
78. Logan N, McMonagle E, Drew AA, Takahashi E, McDonald M, Baron MD, et al. Efficient generation of vesicular stomatitis virus (VSV)-pseudotypes bearing morbilliviral glycoproteins and their use in quantifying virus neutralising antibodies. Vaccine (2016) 34:814–22. doi: 10.1016/j.vaccine.2015.12.006
79. Kishishita N, Takeda N, Anuegoonpipat A, Anantapreecha S. Development of a pseudotyped-lentiviral-vector-based neutralization assay for chikungunya virus infection. J Clin Microbiol (2013) 51:1389–95. doi: 10.1128/JCM.03109-12
80. Wright E, Temperton NJ, Marston DA, McElhinney LM, Fooks AR, Weiss RA. Investigating antibody neutralization of lyssaviruses using lentiviral pseudotypes: a cross-species comparison. J Gen Virol (2008) 89:2204–13. doi: 10.1099/vir.0.2008/000349-0
81. Konduru K, Shurtleff AC, Bavari S, Kaplan G. High degree of correlation between Ebola virus BSL-4 neutralization assays and pseudotyped VSV BSL-2 fluorescence reduction neutralization test. J Virological Methods (2018) 254:1–7. doi: 10.1016/j.jviromet.2018.01.003
82. Steeds K, Hall Y, Slack GS, Longet S, Strecker T, Fehling SK, et al. Pseudotyping of VSV with Ebola virus glycoprotein is superior to HIV-1 for the assessment of neutralising antibodies. Sci Rep (2020) 10:14289. doi: 10.1038/s41598-020-71225-1
83. Wilkinson DE, Page M, Mattiuzzo G, Hassall M, Dougall T, Rigsby P, et al. Comparison of platform technologies for assaying antibody to Ebola virus. Vaccine (2017) 35:1347–52. doi: 10.1016/j.vaccine.2016.11.083
84. Wasilewski LN, Ray SC, Bailey JR. Hepatitis C virus resistance to broadly neutralizing antibodies measured using replication-competent virus and pseudoparticles. J Gen Virol (2016) 97:2883–93. doi: 10.1099/jgv.0.000608
85. Bailey JR, Wasilewski LN, Snider AE, El-Diwany R, Osburn WO, Keck Z, et al. Naturally selected hepatitis C virus polymorphisms confer broad neutralizing antibody resistance. J Clin Invest (2015) 125:437–47. doi: 10.1172/JCI78794
86. Urbanowicz RA, McClure CP, Brown RJP, Tsoleridis T, Persson MAA, Krey T, et al. A diverse panel of hepatitis C virus glycoproteins for use in vaccine research reveals extremes of monoclonal antibody neutralization resistance. J Virol (2016) 90:3288–301. doi: 10.1128/JVI.02700-15
87. Chan E, Heilek-Snyder G, Cammack N, Sankuratri S, Ji C. Development of a moloney murine leukemia virus-based pseudotype anti-HIV assay suitable for accurate and rapid evaluation of HIV entry inhibitors. J Biomol Screen (2006) 11:652–63. doi: 10.1177/1087057106288881
88. Li W, Cao S, Zhang Q, Li J, Zhang S, Wu W, et al. Comparison of serological assays to titrate Hantaan and Seoul hantavirus-specific antibodies. Virol J (2017) 14:133. doi: 10.1186/s12985-017-0799-0
89. Ning T, Wang L, Liu S, Ma J, Nie J, Huang W, et al. Monitoring neutralization property change of evolving hantaan and seoul viruses with a novel pseudovirus-based assay. Virol Sin (2021) 36:104–12. doi: 10.1007/s12250-020-00237-y
90. Alberini I, Del Tordello E, Fasolo A, Temperton NJ, Galli G, Gentile C, et al. Pseudoparticle neutralization is a reliable assay to measure immunity and cross-reactivity to H5N1 influenza viruses. Vaccine (2009) 27:5998–6003. doi: 10.1016/j.vaccine.2009.07.079
91. Buchy P, Vong S, Chu S, Garcia J-M, Hien TT, Hien VM, et al. Kinetics of neutralizing antibodies in patients naturally infected by H5N1 virus. PloS One (2010) 5:e10864. doi: 10.1371/journal.pone.0010864
92. Garcia J-M, Pepin S, Lagarde N, Ma ESK, Vogel FR, Chan KH, et al. Heterosubtype neutralizing responses to influenza A (H5N1) viruses are mediated by antibodies to virus haemagglutinin. PloS One (2009) 4:e7918. doi: 10.1371/journal.pone.0007918
93. Temperton NJ, Hoschler K, Major D, Nicolson C, Manvell R, Hien VM, et al. A sensitive retroviral pseudotype assay for influenza H5N1-neutralizing antibodies. Influenza Other Respir Viruses (2007) 1:105–12. doi: 10.1111/j.1750-2659.2007.00016.x
94. Wang W, Xie H, Ye Z, Vassell R, Weiss CD. Characterization of lentiviral pseudotypes with influenza H5N1 hemagglutinin and their performance in neutralization assays. J Virological Methods (2010) 165:305–10. doi: 10.1016/j.jviromet.2010.02.009
95. Tian Y, Zhao H, Liu Q, Zhang C, Nie J, Huang W, et al. Development of in vitro and in vivo neutralization assays based on the pseudotyped H7N9 virus. Sci Rep (2018) 8:8484. doi: 10.1038/s41598-018-26822-6
96. Lee H-J, Min K-I, Park KH, Choi HJ, Kim M-K, Ahn C-Y, et al. Comparison of JEV neutralization assay using pseudotyped JEV with the conventional plaque-reduction neutralization test. J Microbiol (2014) 52:435–40. doi: 10.1007/s12275-014-3529-y
97. Wright E, Hayman DTS, Vaughan A, Temperton NJ, Wood JLN, Cunningham AA, et al. Virus neutralising activity of African fruit bat (Eidolon helvum) sera against emerging lyssaviruses. Virology (2010) 408:183–9. doi: 10.1016/j.virol.2010.09.014
98. Perera RA, Wang P, Gomaa MR, El-Shesheny R, Kandeil A, Bagato O, et al. Seroepidemiology for MERS coronavirus using microneutralisation and pseudoparticle virus neutralisation assays reveal a high prevalence of antibody in dromedary camels in Egypt, June 2013. Eurosurveillance (2013) 18:20574. doi: 10.2807/1560-7917.ES2013.18.36.20574
99. Park SW, Perera RAPM, Choe PG, Lau EHY, Choi SJ, Chun JY, et al. Comparison of serological assays in human Middle East respiratory syndrome (MERS)-coronavirus infection. Eurosurveillance (2015) 20:30042. doi: 10.2807/1560-7917.ES.2015.20.41.30042
100. Lester S, Harcourt J, Whitt M, Al-Abdely HM, Midgley CM, Alkhamis AM, et al. Middle East respiratory coronavirus (MERS-CoV) spike (S) protein vesicular stomatitis virus pseudoparticle neutralization assays offer a reliable alternative to the conventional neutralization assay in human seroepidemiological studies. Access Microbiol (2019) 1:e000057. doi: 10.1099/acmi.0.000057
101. Alharbi NK, Qasim I, Almasoud A, Aljami HA, Alenazi MW, Alhafufi A, et al. Humoral immunogenicity and efficacy of a single dose of ChAdOx1 MERS vaccine candidate in dromedary camels. Sci Rep (2019) 9:16292. doi: 10.1038/s41598-019-52730-4
102. Wang B, Liu P, Li T, Si W, Xiu J, Liu H. Package of NDV-pseudotyped HIV-luc virus and its application in the neutralization assay for NDV infection. PloS One (2014) 9:e99905. doi: 10.1371/journal.pone.0099905
103. Tamin A, Harcourt BH, Lo MK, Roth JA, Wolf MC, Lee B, et al. Development of a neutralization assay for Nipah virus using pseudotype particles. J Virological Methods (2009) 160:1–6. doi: 10.1016/j.jviromet.2009.02.025
104. Logan N, Dundon WG, Diallo A, Baron MD, James Nyarobi M, Cleaveland S, et al. Enhanced immunosurveillance for animal morbilliviruses using vesicular stomatitis virus (VSV) pseudotypes. Vaccine (2016) 34:5736–43. doi: 10.1016/j.vaccine.2016.10.010
105. Paneth Iheozor-Ejiofor R, Levanov L, Hepojoki J, Strandin T, Lundkvist Å, Plyusnin A, et al. Vaccinia virus-free rescue of fluorescent replication-defective vesicular stomatitis virus and pseudotyping with Puumala virus glycoproteins for use in neutralization tests. J Gen Virol (2016) 97:1052–9. doi: 10.1099/jgv.0.000437
106. Bukbuk DN, Fukushi S, Tani H, Yoshikawa T, Taniguchi S, Iha K, et al. Development and validation of serological assays for viral hemorrhagic fevers and determination of the prevalence of Rift Valley fever in Borno State, Nigeria. Trans R Soc Trop Med Hygiene (2014) 108:768–73. doi: 10.1093/trstmh/tru163
107. Nie J, Wu X, Ma J, Cao S, Huang W, Liu Q, et al. Development of in vitro and in vivo rabies virus neutralization assays based on a high-titer pseudovirus system. Sci Rep (2017) 7:42769. doi: 10.1038/srep42769
108. Wright E, McNabb S, Goddard T, Horton DL, Lembo T, Nel LH, et al. A robust lentiviral pseudotype neutralisation assay for in-field serosurveillance of rabies and lyssaviruses in Africa. Vaccine (2009) 27:7178–86. doi: 10.1016/j.vaccine.2009.09.024
109. Bland JM, Altman DG. Statistical methods for assessing agreement between two methods of clinical measurement. Lancet (1986) 1:307–10. doi: 10.1016/S0140-6736(86)90837-8
110. Li K, Dong F, Cui B, Cui L, Liu P, Ma C, et al. Development of a pseudovirus-based assay for measuring neutralizing antibodies against Coxsackievirus A10. Hum Vaccines Immunotherapeutics (2020) 16:1434–40. doi: 10.1080/21645515.2019.1691404
111. Wu X, Mao Q, Yao X, Chen P, Chen X, Shao J, et al. Development and evaluation of a pseudovirus-luciferase assay for rapid and quantitative detection of neutralizing antibodies against enterovirus 71. PloS One (2013) 8:e64116. doi: 10.1371/journal.pone.0064116
112. Valcourt EJ, Manguiat K, Robinson A, Chen JC-Y, Dimitrova K, Philipson C, et al. Evaluation of a commercially-available surrogate virus neutralization test for severe acute respiratory syndrome coronavirus-2 (SARS-CoV-2). Diagn Microbiol Infect Dis (2021) 99:115294. doi: 10.1016/j.diagmicrobio.2020.115294
113. Abe KT, Li Z, Samson R, Samavarchi-Tehrani P, Valcourt EJ, Wood H, et al. A simple protein-based surrogate neutralization assay for SARS-CoV-2. JCI Insight (2020) 5:e142362. doi: 10.1172/jci.insight.142362
Keywords: pseudotype, neutralisation, correlation, virus, live virus correlates of protection
Citation: Cantoni D, Wilkie C, Bentley EM, Mayora-Neto M, Wright E, Scott S, Ray S, Castillo-Olivares J, Heeney JL, Mattiuzzo G and Temperton NJ (2023) Correlation between pseudotyped virus and authentic virus neutralisation assays, a systematic review and meta-analysis of the literature. Front. Immunol. 14:1184362. doi: 10.3389/fimmu.2023.1184362
Received: 11 March 2023; Accepted: 28 August 2023;
Published: 18 September 2023.
Edited by:
Heribert Stoiber, Innsbruck Medical University, AustriaCopyright © 2023 Cantoni, Wilkie, Bentley, Mayora-Neto, Wright, Scott, Ray, Castillo-Olivares, Heeney, Mattiuzzo and Temperton. This is an open-access article distributed under the terms of the Creative Commons Attribution License (CC BY). The use, distribution or reproduction in other forums is permitted, provided the original author(s) and the copyright owner(s) are credited and that the original publication in this journal is cited, in accordance with accepted academic practice. No use, distribution or reproduction is permitted which does not comply with these terms.
*Correspondence: Nigel James Temperton, bi50ZW1wZXJ0b25Aa2VudC5hYy51aw==
†These authors have contributed equally to this work
Disclaimer: All claims expressed in this article are solely those of the authors and do not necessarily represent those of their affiliated organizations, or those of the publisher, the editors and the reviewers. Any product that may be evaluated in this article or claim that may be made by its manufacturer is not guaranteed or endorsed by the publisher.
Research integrity at Frontiers
Learn more about the work of our research integrity team to safeguard the quality of each article we publish.