- 1SaBio. Instituto de Investigación en Recursos Cinegéticos IREC-CSIC-UCLM-JCCM, Ciudad Real, Spain
- 2Department of Veterinary Pathobiology, Center for Veterinary Health Sciences, Oklahoma State University, Stillwater, OK, United States
The opinion flows from Introduction to the immunological quantum that requires a historical perspective, to Quantum vaccine algorithms supported by a bibliometric analysis, to Quantum vaccinomics describing from our perspective the different vaccinomics and quantum vaccinomics algorithms. Finally, in the Discussion and conclusions we propose novel platforms and algorithms developed to further advance on quantum vaccinomics. In the paper we refer to protective epitopes or immunological quantum for the design of candidate vaccine antigens, which may elicit a protective response through both cellular and antibody mediated mechanisms of the host immune system. Vaccines are key interventions for the prevention and control of infectious diseases affecting humans and animals worldwide. Biophysics led to quantum biology and quantum immunology reflecting quantum dynamics within living systems and their evolution. In analogy to quantum of light, immune protective epitopes were proposed as the immunological quantum. Multiple quantum vaccine algorithms were developed based on omics and other technologies. Quantum vaccinomics is the methodological approach with different platforms used for the identification and combination of immunological quantum for vaccine development. Current quantum vaccinomics platforms include in vitro, in music and in silico algorithms and top trends in biotechnology for the identification, characterization and combination of candidate protective epitopes. These platforms have been applied to different infectious diseases and in the future should target prevalent and emerging infectious diseases with novel algorithms.
Introduction to the immunological quantum
Vaccines are one of the most important achievements in human history. From classical 3Is (isolate-inactivate-inject) to recombinant vaccines and recent vaccinomics approaches, vaccines have prevented millions of deaths worldwide (1–4). However, development of effective vaccines against infectious diseases such as tuberculosis, acquired immunodeficiency syndrome (AIDS), malaria, Lyme disease or Crimean-Congo Hemorrhagic Fever (CCHF) causing millions of deaths annually is still a challenge.
Quantum biology was proposed by Pascual Jordan in 1932 based on the dynamics of biological living systems to maintain the non-equilibrium state (4, 5). Then and based on the double helix structure of DNA, proton tunneling was proposed in 1963 as the quantum mechanics mechanism of DNA point mutations (6). Additionally, stochastic models have been proposed for gene regulation based on gene activation and inactivation by random association and dissociation events (7). These findings support the concept of quantum immunology based on the random processes of electronic structure of molecular interactions behind peptide immunogenicity present in the immune system (4, 8). Then, in allusion to Albert Einstein’s definition of the proton as a quantum of light, immune protective epitopes were proposed as the immunological quantum (9).
Quantum vaccine algorithms
Using a bibliometric analysis by searching “quantum + vaccine + algorithm” in PubMed (https://pubmed.ncbi.nlm.nih.gov; January 16, 2023), the results provided 14 references. The recent origin of these algorithms starts with advances in deep sequencing and structural studies applied to protective candidate antigens to develop safer and more efficacious vaccines (10). Reverse vaccinology approaches such as Vacceed were developed for in silico vaccine candidate discovery (11). Algorithms and methods based on amphipathicity profiles of proteins, sequence motifs, quantitative matrices (QM), artificial neural networks (ANN), support vector machines (SVM), quantitative structure activity relationship (QSAR), T-cell major histocompatibility complex (MHC) class I binding prediction and molecular docking simulations among others were developed to predict T-cell epitopes (12, 13). Quantum chemical calculations to predict biological function were applied to T-cell receptor interaction with a peptide/MHC class I (14). Recently, an algorithm was proposed using semi-empirical quantum mechanical methods for calculating peptide-MHC class I and II molecules binding energy for the rational design of T-cell epitopes with application in vaccinology (15).
Mathematical combinatorial and computational techniques for drug discovery such as topology, combinatorics, graph theory and knot theory could be also applied to vaccinology (16). For example, facing recent challenges associated with severe coronavirus disease (COVID-19), the interactions between SARS-CoV-2 spike glycoprotein (S protein) and human angiotensin-converting enzyme 2 (hACE2) were characterized to identify potential vaccine candidates. Machine learning algorithms were applied to identify changes in infrared spectra associated with variations of the secondary structure of S protein for developing faster than conventional quantum chemistry calculations of real-time spectroscopy of protein dynamics (17). Combining this approach with antibody isotype S epitope mapping in different patient cohorts (18) may facilitate the identification of candidate protective epitopes for vaccine development.
Computational protein design algorithms are important for the combination of immunological quantum in vaccine antigens and have the potential to increase the accuracy and reliability of vaccine chimeric antigens (19). An innovative approach using biocompatible, near-infrared quantum dots (QDs) was recently proposed for the delivery of intradermal QDs with reliable code information together with vaccines for developing tools for vaccine decentralized data storage and biosensing (20).
Quantum vaccinomics
Vaccinomics was defined as the application of immunogenetics and genomics to study the molecular mechanisms in response to vaccines (21). In the vaccinomics platform, systems biology integration of omics datasets combined with Big Data analytics and machine learning allow the identification of candidate vaccine protective antigens (2, 4, 22–28) (Figure 1). Quantum vaccinomics was then proposed as the methodological approach with different platforms for the identification and combination of immunological quantum for vaccine development (4, 29–33). Quantum vaccinomics platforms include in silico prediction and epitope mapping of immunological quantum as well as in vitro, in music and in silico characterization of protein-protein interactions to identify protein interacting domains as candidate protective epitopes (29–33) (Figure 1). These platforms facilitate antigen combination and including probiotics and post-translational modifications such as glycan alpha-gal to boost protective immune response to vaccination (34, 35). In this way, quantum vaccinomics platforms include top trends in biotechnology such as Big Data, gene sequencing and editing, precision medicine, bio manufacturing, and synthetic biology.
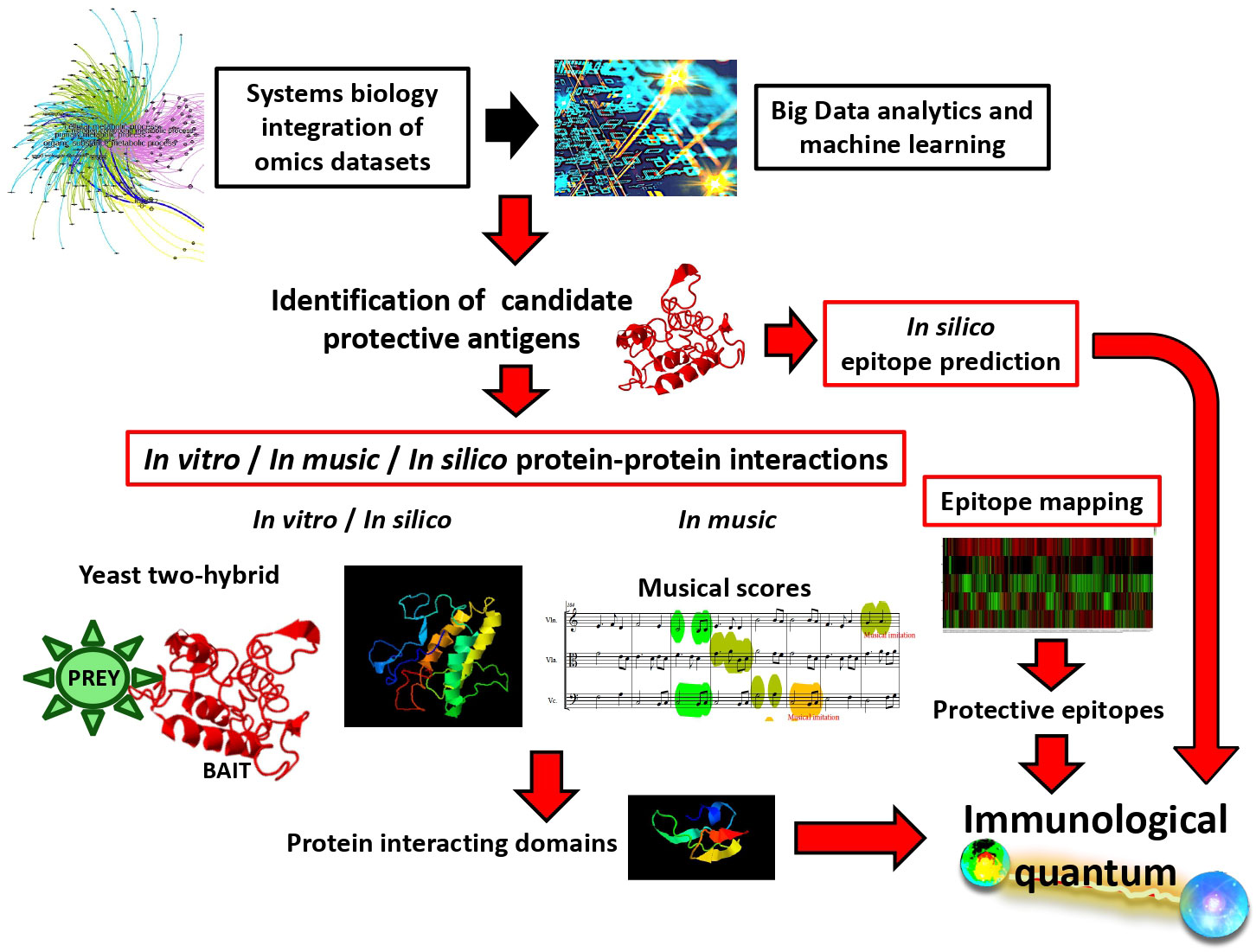
Figure 1 Quantum vaccinomics platforms for the identification of immunological quantum and design of vaccine chimeric protective antigens. Candidate protective antigens are identified using systems biology integration of omics dataset combined Big Data analytics and machine learning. Then, immunological quantum can be identified using in silico algorithms or epitope mapping for predicting protective epitopes or using in vitro, in silico and in music approaches for the identification of domains involved in protein-protein interactions.
As recently reported (33), vaccines may elicit protective response in some species but in others prevalent antibodies may recognize non-protective epitopes. Using sera from both vaccinated protected and non-protected species it is possible to conduct a microarray epitope mapping to identify immunological quantum for the design of a chimeric protective antigen (33). Epitope mapping can also be used to identify immunological quantum recognized by immunized hosts and reactive to different pathogen and vector species. For example, using sera from tick Subolesin-immunized cattle it was possible to identify B-cell reactive epitopes in different tick species (32). This information could then be used to combine protective epitopes and design a vaccine chimeric antigen with improve protection against multiple tick species parasitizing on the same host.
The collaboration between science and art has shown an impact on approaching scientific and social challenges (e.g., (29, 36–38)). In this context, musical algorithms to translate gene/protein sequences into music provide insights into biomolecule evolution and interactome (30, 39). Protein interactome involves functional sequences that are not highly exposed to the immune system but play a key function and thus constitute candidate protective epitopes. The information obtained from in music approaches translates into the identification of protein-protein interacting motifs that in combination with in vitro (e.g., yeast two-hybrid) and in silico methods provide candidate immunological quantum for vaccine antigen design (29, 30) (Figure 1). In silico computational algorithms applied to vaccine discovery transforms digital abstractions of this complex interdisciplinary and interdependent system into candidate protective antigens (31, 40, 41).
Discussion and conclusions
Despite the advances represented by quantum vaccine algorithms, limitations of these algorithms were approached using quantum vaccinomics. For example, Van Regenmortel (42, 43) discussed that peptide antigenicity can be chemically and structurally modified to improve antibody-peptide interactions, but it does not necessarily improve immunogenicity mediated by multiple factors of the host immune system. Using human immunodeficiency virus (HIV) model, Van Regenmortel (44) illustrates the limitations of reductionist methods, systems biology and structure-based reverse vaccinology to address the complexity of the human immune system for a rational design of anti-HIV vaccines for the prevention of acquired immunodeficiency syndrome (AIDS). However, quantum vaccinomics combines different platforms including not only in vitro, in music and in silico characterization of protein-protein interactions but also mapping of B-cell reactive protective epitopes and characterization of cellular immune mechanisms associated with protection in response to vaccine using integration of omics datasets (18, 29, 32, 33). For example, quantum vaccinomics was successfully applied to the tick protective antigen Subolesin. Subolesin was discovered by expression library immunization in the mouse model of Ixodes ricinus tick infestations (45). Protective linear B-cell and conformational epitopes were mapped and in silico modeling of protein structure was then used to identify and combine candidate protective epitopes in the chimeric antigen Q38 (31, 46). The Q38 antigen was validated using other quantum vaccinomics algorithms (29, 32) and elicited a protective immune response against tick infestations (47).
The combination of the different quantum vaccine and quantum vaccinomics approaches is important to provide reliable information on the proposed immunological quantum. These results need to be validated ex vivo and in vivo to advance in vaccine development. Our research in this area is mainly focused on ticks and tick-borne diseases (29–33), but quantum vaccinomics approaches have been applied to other diseases such as COVID-19 (17), tuberculosis (48), AIDS (41) and neosporosis (40). Future directions using quantum vaccinomics approaches should target highly prevalent and emerging infectious diseases.
Novel platforms and algorithms will be developed to further advance in quantum vaccinomics for the development of vaccines and other control interventions. These novel platforms include (a) use of commensal bacteria to produce and secrete protective antigens to interfere with pathogen infection or serve for vaccine delivery (49), (b) combination of vaccines with probiotics (e.g., with high alpha-gal content) and heat inactivated mycobacteria to serve as adjuvants/immunostimulants (50, 51), (c) new vaccine delivery platforms (e.g., nanoparticle (NP)-based formulations, lipid NP-mRNA, viral vectors, virus-like particles) to stimulate innate and trained immunity and boost protective immune response (52), (d) oral vaccine formulations to improve safety and access to developing countries (50), and (e) stimulating trained immunity mechanisms in response to vaccination (51).
Author contributions
Both authors listed have made substantial, direct and intellectual contributions to the work and approved it for publication.
Funding
This work was supported in part by Ministerio de Ciencia e Innovación/Agencia Estatal de Investigación MCIN/AEI/10.13039/501100011033, Spain and EU-FEDER (Grant BIOGAL PID2020-116761GB-I00). MC is funded by the Ministerio de Ciencia, Innovación y Universidades, Spain (Grant IJC2020-042710-I).
Acknowledgments
We thank members of our group for their contribution to research on quantum vaccinomics.
Conflict of interest
The authors declare that the research was conducted in the absence of any commercial or financial relationships that could be construed as a potential conflict of interest.
Publisher’s note
All claims expressed in this article are solely those of the authors and do not necessarily represent those of their affiliated organizations, or those of the publisher, the editors and the reviewers. Any product that may be evaluated in this article, or claim that may be made by its manufacturer, is not guaranteed or endorsed by the publisher.
References
1. World Health Organization (WHO). Vaccines and immunization. Available at: https://www.who.int/health-topics/vaccines-and-immunization (Accessed February 2023).
2. Bragazzi NL, Gianfredi V, Villarini M, Rosselli R, Nasr A, Hussein A, et al. Vaccines meet big data: state-of-the-art and future prospects. from the classical 3Is (“Isolate-Inactivate-Inject”) vaccinology 1.0 to vaccinology 3.0, vaccinomics, and beyond: a historical overview. Front Public Health (2018) 6:62. doi: 10.3389/fpubh.2018.00062
3. Andreano E, D’Oro U, Rappuoli R, Finco O. Vaccine evolution and its application to fight modern threats. Front Immunol (2019) 10:1722. doi: 10.3389/fimmu.2019.01722
4. de la Fuente J, Contreras M. Vaccinomics: a future avenue for vaccine development against emerging pathogens. Expert Rev Vaccines (2021) 20:1561–9. doi: 10.1080/14760584.2021.1987222
5. McFadden J, Al-Khalili J. The origins of quantum biology. Proc Math Phys Eng Sci (2018) 474:20180674. doi: 10.1098/rspa.2018.0674
6. Löwdin P-O. Proton tunneling in DNA and its biological implications. Rev Mod Phys (1963) 35:724–32. doi: 10.1103/RevModPhys.35.724
7. de la Fuente J. A stochastic model for type I interferon gene expression. J Biochem Mol Biol Biophys JBMBB (2000) 4:305–11.
8. Germenis A, Manoussakis M, Antipas G. The dawn of quantum immunology. SRL Immunol Immunother (2016) 1:3–6.
10. Prachi P, Donati C, Masciopinto F, Rappuoli R, Bagnoli F. Deep sequencing in pre- and clinical vaccine research. Public Health Genomics (2013) 16:62–8. doi: 10.1159/000345611
11. Goodswen SJ, Kennedy PJ, Ellis JT. Vacceed: a high-throughput in silico vaccine candidate discovery pipeline for eukaryotic pathogens based on reverse vaccinology. Bioinformatics (2014) 30:2381–3. doi: 10.1093/bioinformatics/btu300
12. Desai DV, Kulkarni-Kale U. T-Cell epitope prediction methods: an overview. Methods Mol Biol (2014) 1184:333–64. doi: 10.1007/978-1-4939-1115-8_19
13. Goodswen SJ, Kennedy PJ, Ellis JT. Enhancing in silico protein-based vaccine discovery for eukaryotic pathogens using predicted peptide-mhc binding and peptide conservation scores. PloS One (2014) 9:e115745. doi: 10.1371/journal.pone.0115745
14. Antipas GSE, Germenis AE. Quantum chemical calculations predict biological function: the case of T cell receptor interaction with a peptide/MHC class I. Front Chem (2015) 3:9. doi: 10.3389/fchem.2015.00009
15. Ortiz-Mahecha CA, Agudelo WA, Patarroyo MA, Patarroyo ME, Suárez CF. MHCBI: a pipeline for calculating peptide-mhc binding energy using semi-empirical quantum mechanical methods with explicit/implicit solvent models. Brief Bioinform (2021) 22:bbab171. doi: 10.1093/bib/bbab171
16. Balasubramanian K. Mathematical and computational techniques for drug discovery: promises and developments. Curr Top Med Chem (2018) 18:2774–99. doi: 10.2174/1568026619666190208164005
17. Ye S, Zhang G, Jiang J. AI-Based spectroscopic monitoring of real-time interactions between SARS-CoV-2 and human ACE2. Proc Natl Acad Sci USA (2021) 118:e2025879118. doi: 10.1073/pnas.2025879118
18. Contreras M, Vicente J, Cerón JJ, Martinez Subiela S, Urra JM, Rodríguez-Del-Río FJ, et al. Antibody isotype epitope mapping of SARS-CoV-2 spike RBD protein: targets for COVID-19 symptomatology and disease control. Eur J Immunol (2023) 53(4):e2250206. doi: 10.1002/eji.202250206
19. Talluri S. Algorithms for protein design. Adv Protein Chem Struct Biol (2022) 130:1–38. doi: 10.1016/bs.apcsb.2022.01.003
20. McHugh KJ, Jing L, Severt SY, Cruz M, Sarmadi M, Jayawardena HSN, et al. Biocompatible near-infrared quantum dots delivered to the skin by microneedle patches record vaccination. Sci Transl Med (2019) 11:eaay7162. doi: 10.1126/scitranslmed.aay7162
21. Poland GA. Pharmacology, vaccinomics, and the second golden age of vaccinology. Clin Pharmacol Ther (2007) 82:623–6. doi: 10.1038/sj.clpt.6100379
22. Oberg AL, Kennedy RB, Li P, Ovsyannikova IG, Poland GA. Systems biology approaches to new vaccine development. Curr Opin Immunol (2011) 23:436–43. doi: 10.1016/j.coi.2011.04.005
23. Poland GA, Kennedy RB, Ovsyannikova IG. Vaccinomics and personalized vaccinology: is science leading us toward a new path of directed vaccine development and discovery? PloS Pathog (2011) 7:e1002344. doi: 10.1371/journal.ppat.1002344
24. Ovsyannikova IG, Poland GA. Vaccinomics: current findings, challenges and novel approaches for vaccine development. AAPS J (2011) 13:438–44. doi: 10.1208/s12248-011-9281-x
25. de la Fuente J, Merino O. Vaccinomics, the new road to tick vaccines. Vaccine (2013) 31:5923–9. doi: 10.1016/j.vaccine.2013.10.049
26. Contreras M, Alberdi P, Fernández De Mera IG, Krull C, Nijhof A, Villar M, et al. Vaccinomics approach to the identification of candidate protective antigens for the control of tick vector infestations and Anaplasma phagocytophilum infection. Front Cell Infect Microbiol (2017) 7:360. doi: 10.3389/fcimb.2017.00360
27. de la Fuente J, Villar M, Estrada-Peña A, Olivas JA. High throughput discovery and characterization of tick and pathogen vaccine protective antigens using vaccinomics with intelligent big data analytic techniques. Expert Rev Vaccines (2018) 17:569–76. doi: 10.1080/14760584.2018.1493928
28. Kennedy RB, Ovsyannikova IG, Palese P, Poland GA. Current challenges in vaccinology. Front Immunol (2020) 11:1181. doi: 10.3389/fimmu.2020.01181
29. Artigas-Jerónimo S, Comín JJP, Villar M, Contreras M, Alberdi P, Viera IL, et al. A novel combined scientific and artistic approach for the advanced characterization of interactomes: the Akirin/Subolesin model. Vaccines (2020) 8:77. doi: 10.3390/vaccines8010077
30. Contreras M, Artigas-Jerónimo S, Pastor Comín JJ, de la Fuente J. A quantum vaccinomics approach based on protein-protein interactions. Methods Mol Biol (2022) 2411:287–305. doi: 10.1007/978-1-0716-1888-2_17
31. Moreno-Cid JA, de la Lastra Pérez JM, Villar M, Jiménez M, Pinal R, Estrada-Peña A, et al. Control of multiple arthropod vector infestations with Subolesin/Akirin vaccines. Vaccine (2013) 31:1187–96. doi: 10.1016/j.vaccine.2012.12.073
32. Contreras M, Kasaija PD, Kabi F, Mugerwa S, de la Fuente J. The correlation between subolesin-reactive epitopes and vaccine efficacy. Vaccines (2022) 10:1327. doi: 10.3390/vaccines10081327
33. de la Fuente J, Moraga-Fernández A, Alberdi P, Díaz-Sánchez S, García-Álvarez O, Fernández-Melgar R, et al. A quantum vaccinomics approach for the design and production of MSP4 chimeric antigen for the control of Anaplasma phagocytophilum infections. Vaccines (2022) 10:1995. doi: 10.3390/vaccines10121995
34. Galili U. Increasing efficacy of enveloped whole-virus vaccines by in situ immune-complexing with the natural anti-gal antibody. Med Res Arch (2021) 9:2481. doi: 10.18103/mra.v9i7.2481
35. Cabezas Cruz A, Valdés JJ, de la Fuente J. Control of vector-borne infectious diseases by human immunity against α-gal. Expert Rev Vaccines (2016) 15:953–5. doi: 10.1080/14760584.2016.1181547
36. de la Fuente J. Art-science multidisciplinary collaborations to address the scientific challenges posed by COVID-19. Ann Med (2022) 54:2535–48. doi: 10.1080/07853890.2022.2123557
37. de la Fuente J. The exquisite corpse for the advance of science. Un cadavre exquis pour le progrès scientifique. Art Sci (2020) 4:43. doi: 10.21494/ISTE.OP.2020.0506
38. Veis N. Evolution: four takes on the evolution of art. Nature (2017) 543:490. doi: 10.1038/543490a
39. de la Fuente J, Pastor Comín JJ, Gortázar C. The sound of host-SARS-CoV-2 molecular interactions. Innovation (Camb) (2021) 2:100126. doi: 10.1016/j.xinn.2021.100126
40. Goodswen SJ, Kennedy PJ, Ellis JT. Discovering a vaccine against neosporosis using computers: is it feasible? Trends Parasitol (2014) 30:401–11. doi: 10.1016/j.pt.2014.06.004
41. Diwan B, Saxena R, Tiwari A. HIV-2 and its role in conglutinated approach towards acquired immunodeficiency syndrome (AIDS) vaccine development. Springerplus (2013) 2:7. doi: 10.1186/2193-1801-2-7
42. Van Regenmortel MH. Antigenicity and immunogenicity of synthetic peptides. Biologicals (2001) 29:209–13. doi: 10.1006/biol.2001.0308
43. Van Regenmortel MH. Structure-based reverse vaccinology failed in the case of HIV because it disregarded accepted immunological theory. Int J Mol Sci (2016) 17:1591. doi: 10.3390/ijms17091591
44. Van Regenmortel MHV. Development of a preventive HIV vaccine requires solving inverse problems which is unattainable by rational vaccine design. Front Immunol (2018) 8:2009. doi: 10.3389/fimmu.2017.02009
45. Almazán C, Kocan KM, Bergman DK, Garcia-Garcia JC, Blouin EF, de la Fuente J. Identification of protective antigens for the control of Ixodes scapularis infestations using cDNA expression library immunization. Vaccine (2003) 21:1492–501. doi: 10.1016/s0264-410x(02)00683-7
46. Prudencio CR, Pérez de la Lastra JM, Canales M, Villar M, de la Fuente J. Mapping protective epitopes in the tick and mosquito subolesin ortholog proteins. Vaccine (2010) 28:5398–406. doi: 10.1016/j.vaccine.2010.06.021
47. Merino O, Antunes S, Mosqueda J, Moreno-Cid JA, Pérez de la Lastra JM, Rosario-Cruz R, et al. Vaccination with proteins involved in tick-pathogen interactions reduces vector infestations and pathogen infection. Vaccine (2013) 31:5889–96. doi: 10.1016/j.vaccine.2013.09.037
48. Merchant SA, Shaikh MJS, Nadkarni P. Tuberculosis conundrum - current and future scenarios: a proposed comprehensive approach combining laboratory, imaging, and computing advances. World J Radiol (2022) 14:114–36. doi: 10.4329/wjr.v14.i6.114
49. Mazuecos L, Alberdi P, Hernández-Jarguín A, Contreras M, Villar M, Cabezas-Cruz A, et al. Frankenbacteriosis targeting interactions between pathogen and symbiont to control infection in the tick vector. iScience (2023) 26:106697. doi: 10.1016/j.isci.2023.106697
50. Kasaija PD, Contreras M, Kabi F, Mugerwa S, Garrido JM, Gortazar C, et al. Oral vaccine formulation combining tick subolesin with heat inactivated mycobacteria provides control of cross-species cattle tick infestations. Vaccine (2022) 40:4564–73. doi: 10.1016/j.vaccine.2022.06.036
51. Juste RA, Ferreras-Colino E, de la Fuente J, Domínguez M, Risalde MA, Domínguez L, et al. Heat inactivated mycobacteria, alpha-gal and zebrafish: insights gained from experiences with two promising trained immunity inductors and a validated animal model. Immunology (2022) 167:139–53. doi: 10.1111/imm.13529
Keywords: vaccine, vaccinomics, vaccinology, immunology, quantum
Citation: de la Fuente J and Contreras M (2023) Quantum vaccinomics platforms to advance in vaccinology. Front. Immunol. 14:1172734. doi: 10.3389/fimmu.2023.1172734
Received: 23 February 2023; Accepted: 05 June 2023;
Published: 15 June 2023.
Edited by:
Pere-Joan Cardona, Hospital Universitari Germans Trias i Pujol, SpainReviewed by:
Ariel L. Rivas, University of New Mexico, United StatesCopyright © 2023 de la Fuente and Contreras. This is an open-access article distributed under the terms of the Creative Commons Attribution License (CC BY). The use, distribution or reproduction in other forums is permitted, provided the original author(s) and the copyright owner(s) are credited and that the original publication in this journal is cited, in accordance with accepted academic practice. No use, distribution or reproduction is permitted which does not comply with these terms.
*Correspondence: José de la Fuente, jose_delafuente@yahoo.com; Marinela Contreras, marinelacr@hotmail.com