- 1Department of Urology, Tianjin Medical University General Hospital, Tianjin, China
- 2Department of Orthopedics, Tianjin Medical University General Hospital, Tianjin, China
Background: COVID-19, a serious respiratory disease that has the potential to affect numerous organs, is a serious threat to the health of people around the world. The objective of this article is to investigate the potential biological targets and mechanisms by which SARS-CoV-2 affects benign prostatic hyperplasia (BPH) and related symptoms.
Methods: We downloaded the COVID-19 datasets (GSE157103 and GSE166253) and the BPH datasets (GSE7307 and GSE132714) from the Gene Expression Omnibus (GEO) database. In GSE157103 and GSE7307, differentially expressed genes (DEGs) were found using the “Limma” package, and the intersection was utilized to obtain common DEGs. Further analyses followed, including those using Protein-Protein Interaction (PPI), Gene Ontology (GO) function enrichment analysis, and the Kyoto Encyclopedia of Genes and Genomes (KEGG). Potential hub genes were screened using three machine learning methods, and they were later verified using GSE132714 and GSE166253. The CIBERSORT analysis and the identification of transcription factors, miRNAs, and drugs as candidates were among the subsequent analyses.
Results: We identified 97 common DEGs from GSE157103 and GSE7307. According to the GO and KEGG analyses, the primary gene enrichment pathways were immune-related pathways. Machine learning methods were used to identify five hub genes (BIRC5, DNAJC4, DTL, LILRB2, and NDC80). They had good diagnostic properties in the training sets and were validated in the validation sets. According to CIBERSORT analysis, hub genes were closely related to CD4 memory activated of T cells, T cells regulatory and NK cells activated. The top 10 drug candidates (lucanthone, phytoestrogens, etoposide, dasatinib, piroxicam, pyrvinium, rapamycin, niclosamide, genistein, and testosterone) will also be evaluated by the P value, which is expected to be helpful for the treatment of COVID-19-infected patients with BPH.
Conclusion: Our findings reveal common signaling pathways, possible biological targets, and promising small molecule drugs for BPH and COVID-19. This is crucial to understand the potential common pathogenic and susceptibility pathways between them.
1 Introduction
An infectious disease, COVID-19, caused by the SARS Coronavirus 2 (SARS-CoV-2), poses a major danger to worldwide public health (1, 2). The most common symptom of COVID-19 is pneumonia, with severe cases frequently developing life-threatening acute respiratory distress syndrome and respiratory failure, along with fever, sore throat, difficulty breathing and coughing (1, 3). Symptoms can worsen and lead to respiratory failure, which is potentially fatal and affects the heart, liver, neurological system, and kidneys (4–7). The percentage of patients with COVID-19 who develop gastrointestinal symptoms such as nausea, diarrhea, bloating, and bleeding ranges from 3% to 40.7% (8). With the advent of vaccines (9–11) and antiviral drugs (12, 13), the spread and fatality rate of COVID-19 have decreased, but with the advent of new variations such as Delta and Omicron, it continues to pose dangers and difficulties to global health (14, 15).
In older men, benign prostatic hyperplasia (BPH), which causes benign prostate enlargement due to uncontrolled expansion of epithelial and fibromuscular tissue in the migratory zone of the urinary tract and urethral region, is a frequent disorder (16, 17). Lower urinary tract symptoms (LUTS) are common in older men and include frequent urination, inadequate urine flow, delayed urine flow, and nocturia, all of which have a negative influence on quality of life (17). According to a meta-analysis, the lifetime prevalence of BPH is 26.2% (18). Previous research suggests that elderly men may have a higher risk of developing BPH. Older men appear to have more severe cases of COVID-19 and are more likely to infect SARS-CoV-2 (19). Many patients with COVID-19 have experienced serious urinary problems (20, 21). LUTS may be one of the symptoms of COVID-19, and SARS-CoV-2 virus infection may aggravate symptoms in elderly patients with BPH (22, 23). There has not yet been any pertinent research on the possible mode of action between COVID-19 and the symptoms of BPH. To provide novel assistance for the diagnosis and treatment of disorders that have both COVID-19 and BPH, it is necessary to investigate potential hub genes and molecular pathways.
By comparing gene expression across disease groups and healthy tissues, it is now possible to explore the potential pathophysiology of many diseases, thanks to the rapid advance of gene sequencing technologies and bioinformatics analytic techniques. In addition to logistic regression with the least absolute shrinkage and selection operator (LASSO) (24), machine learning techniques such as support vector machine recursive feature elimination (SVM-RFE) (25) and random forest (RF) algorithms (26) are frequently used to accurately identify diagnostic indicators and prediction models. Several studies have been conducted to date to find hub genes and possible biomarkers using different machine learning methods (27, 28).
We attempted to discover common differentially expressed genes (DEGs) in this work by integrating the analysis of the COVID-19 dataset (GSE157103) with the BPH dataset (GSE7307). To identify probable pathways, we used the functional enrichment analysis of gene ontology (GO) and the Kyoto Encyclopedia of Genes and Genomes (KEGG). We also constructed protein-protein interactions (PPI) networks. After that, we used three different machine learning algorithms to find relevant biomarkers and examine their diagnostic value in patients with COVID-19 and BPH. For validation, we additionally used the data sets GSE166253 and GSE132714. The CIBERSORT tool was also applied to calculate the proportion of COVID-19 immune cell infiltration. Finally, we predicted transcription factors (TFs), miRNAs, and small molecule drugs.
2 Materials and methods
2.1 Data acquisition
Four datasets, including two COVID-19 datasets and two BPH datasets, were retrieved from the Gene Expression Omnibus (GEO) database. The training set used GSE157103, which contains 100 samples from COVID-19 patients and 26 samples from controls, as it has a sample size that is significantly larger than GSE166253. The other dataset, GSE166253, which included 10 samples each of COVID-19 patients and healthy individuals, served as a validation set. Because the control sample of GSE132714 is too small, we did not consider it the training set. A BPH dataset, GSE7307, which consists of 7 BPH patients and 12 healthy controls, was used as the training dataset, and another BPH dataset, GSE132714, which consists of 12 BPH patients and 4 healthy controls, was used as a verification set.
2.2 Identification of common DEGs
We applied false discovery rate (FDR) to adjust the P-value. With adj. P < 0.05 and |log2FC| > 0.263 for GSE157103 and adj. P < 0.05 and |log2FC| > 1 for GSE7307, the DEGs were found using the “Limma” R package (29). Heatmaps and volcano plots were created using the “pheatmap” and “ggplot2” tools. Common DEGs of COVID-19 and BPH were obtained through the Venn diagram.
2.3 Functional and pathway enrichment analysis
We analyzed the KEGG and GO enrichment items of common DEGs using the “ClusterProfiler” package (30). GO analysis included three subcategories: molecular function (MF), biological process (BP), and cellular component (CC). Additionally, to select relevant pathways, we employed an adjusted statistical threshold criterion of P < 0.05.
2.4 Construction of PPI networks
We created PPI networks to demonstrate protein interactions, which are critical to understanding the physiology of cellular physiology in health and disease at the protein level. We used STRING (https://www.string-db.org/) (version 11.5) to create PPI networks that hide unconnected nodes (31). Subsequently, Cytoscape (version 3.9.1) was utilized for visual display.
2.5 Using three machine learning algorithms to identify hub genes
The three most common machine learning algorithms used for disease identification and prediction are the RF algorithm, LASSO regression, and SVM-RFE technique. They can help us find the hub genes. The dimensional significance values were determined using the diminishing accuracy approach (Gini coefficient method) using a random forest model (32). The best random forest tree count was 500, and disease-specific genes were identified in the top 15 for significance value. After that, LASSO regression analysis using putative pivotal genes was conducted using the “glmnet” R package to find significant combinations of predicted genes that are consistently connected with COVID-19 (33). In this study, we used the cv.glmnet function here to select the optimal λ value by ten-fold cross-validation. Based on the output, we obtain two λ values: lambda.min=0.01010184 and lambda.1se=0.0212634. We used the value of 0.01010184 to get the coefficients of the final LASSO model, because it makes the cross-validation error minimal. The “e1071” package was applied to perform the SVM-RFE algorithm to find important genes (34). A supervised learning model, called the SVM-RFE, accurately categorizes data points by maximizing the separation between two hyperplanes. The final step was intersecting the possible genes identified by the RF, SVM-RFE, and LASSO algorithms. The overlapping genes were then used as hub genes and displayed by the Venn diagram.
2.6 Evaluation of expression levels and diagnostic value of hub genes
In the COVID-19 training set (GSE157103), analyses of hub genes expression were conducted. The ROC curves were then plotted using GraphPad Prism 9, and to evaluate the prediction effectiveness, the area under the curve (AUC) was best evaluated. The results were then validated using the validation set GSE166253. These genes were strongly predictive for the diagnosis of COVID-19, according to AUC > 0.6 and P < 0.05. ROC curves were developed using the BPH training set (GSE7307) and the validation set (GSE132714) to examine the diagnostic efficacy of hub genes for BPH.
2.7 Immune cell infiltration analysis
To explore the extent of different immune cell infiltration, the CIBERSORT algorithm was utilized to categorize and count the 22 categories of immune cells in the COVID-19 and control groups (35). Ultimately, the link between genes and immune infiltration was discovered using Spearman’s correlation analysis (36).
2.8 Prediction of transcription factors (RFs), MiRNAs and small-molecule drugs
We searched the ChEA database for transcription factor (TF)-gene interactions using the NetworkAnalyst platform (www.networkanalyst.ca) (37). Similarly, we used this platform to search the Tarbase database (version 8.0) for miRNA-gene interactions (38). The results were then visualized using Cytoscape. DSigDB is a gene set database that is linked to medications/compounds. The Enrichr platform (https://maayanlab.cloud/Enrichr/) was applied to access the DSigDB database (39), and small molecule drugs were predicted by entering the names of hub genes.
2.9 Identification of disease association
The DisGeNet database is one of the most extensive databases of human disease-related genes and variations (40). To discover associated diseases and chronic health conditions, we used the DisGeNET database in the NetworkAnalyst platform.
3 Results
3.1 Identification of common DEGs
In order to demonstrate the entire analysis process, we created a flow chart (Figure 1). In the GSE157103, we identified 4917 DEGs, including 2924 up-regulated genes and 1993 down-regulated genes (Figure 2A). In GSE7307, we identified 827 DEGs, including 419 up-regulated genes and 408 down-regulated genes (Figure 2B). Heatmaps were interpreted for DEGs in COVID-19 and BPH, respectively (Figure S1). According to the Venn diagram, 97 common DEGs were discovered in both the GSE157103 and the GSE7307 (Figure 2C).
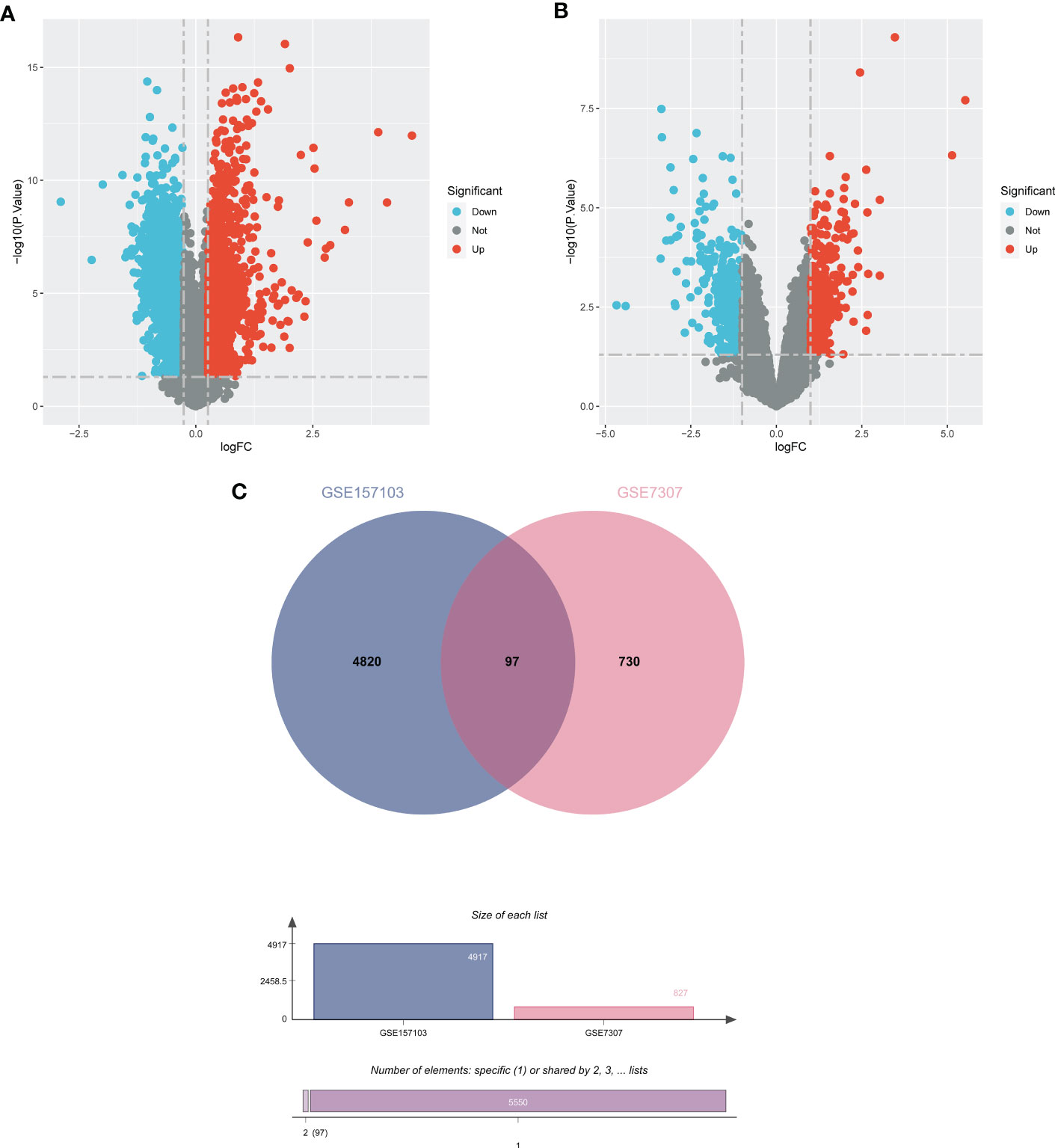
Figure 2 The volcano plots show DEGs of (A) COVID-19 (GSE157103) and (B) BPH (GSE7307) and (C) the Venn diagram of common DEGs.
3.2 Functional and pathway enrichment analysis
GO analysis indicated significantly enriched pathways, including BP, CC, and MF (Figures 3A, B). Significant pathways in the BP category were the immune response-regulating signaling pathway, activation of the immune response, and the immune response regulating cell surface receptor signaling pathway. The main terms in the CC category are the secretory granule lumen, the cytoplasmic vesicle lumen, and the vesicle lumen. Furthermore, in the MF category, the main terms of statistical significance were enzyme activity inhibitor, amide binding, and peptide binding. KEGG analysis revealed the B cell receptor signaling pathway, inflammatory bowel disease, the MAPK signaling pathway, and cytotoxicity mediated by natural killer cells (Figures 3C, D). Our findings showed that these common DEGs are linked to inflammation and immune cells.
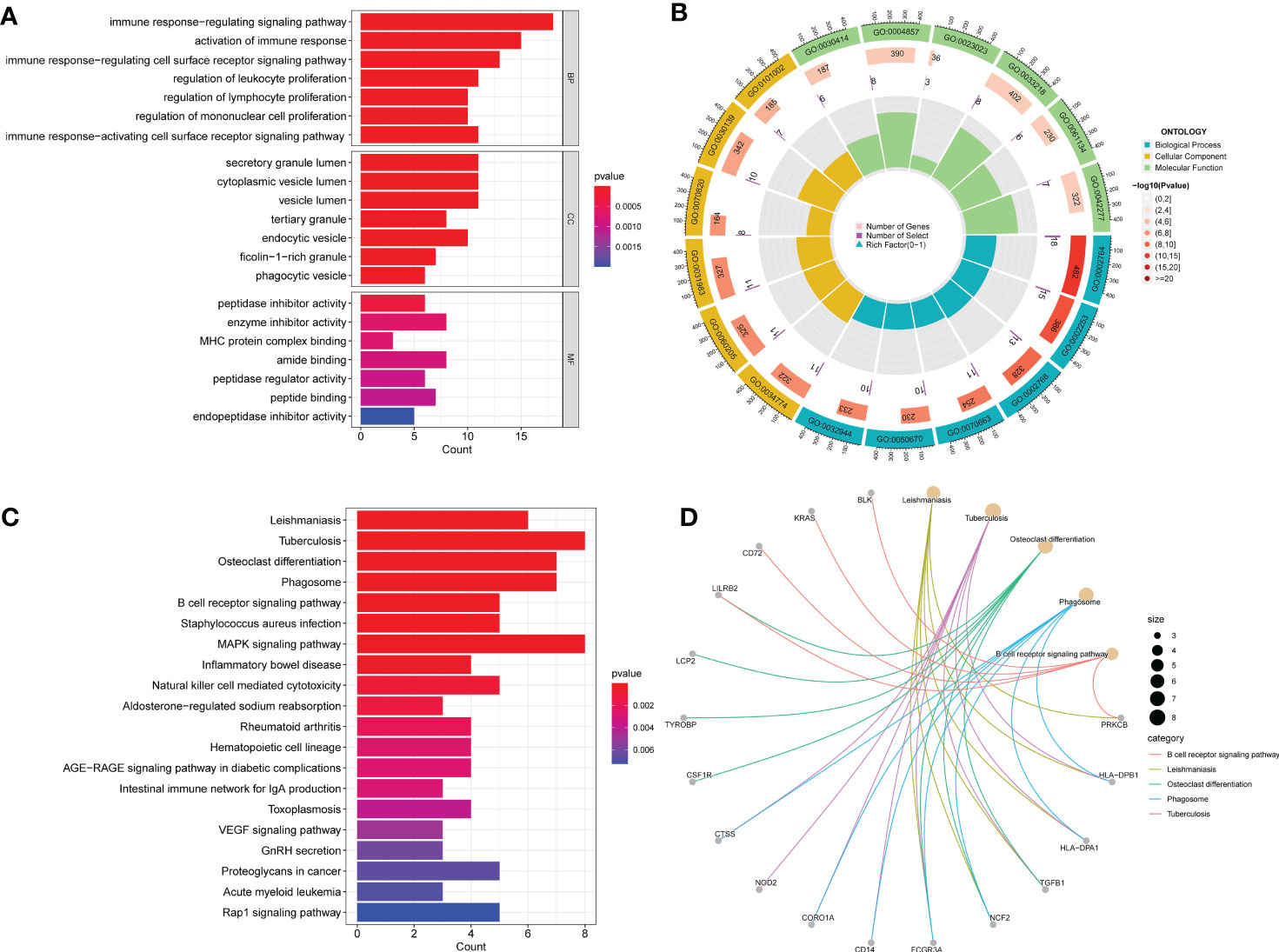
Figure 3 GO and KEGG functional enrichment analysis of the common DEGs between COVID-19 and BPH. (A) The bar plot of GO enrichment analysis. (B) The circle diagram of the GO enrichment analysis. (C) The bar plot of KEGG enrichment analysis. (D) The loop graph of the KEGG enrichment analysis.
3.3 Construction of PPI network
A PPI network of 97 common DEGs was generated using the STRING online site to find protein interactions and visualized using Cytoscape software (Figure 4).
3.4 Using three machine learning algorithms to identify hub genes
First, we used the RF algorithm to narrow the range to 97 DEGs. Recursive random forest classification was performed for all possible values of 1-97 variables, and the average error rate of the model was assessed for all chosen variables, as shown in Figure 5A. Secondly, we examined the link between model error and the number of decision trees. Finally, we chose the top 15 genes in terms of importance (SLC15A3, DTL, NDC80, NEK2, TBC1D22A, KANSL3, CENPN, RPL18A, DNAJC4, LDLR, KIAA1958, BIRC5, LILRB2, DUSP5, FCGR3A) as the likely genes for further investigation (Figure 5B).
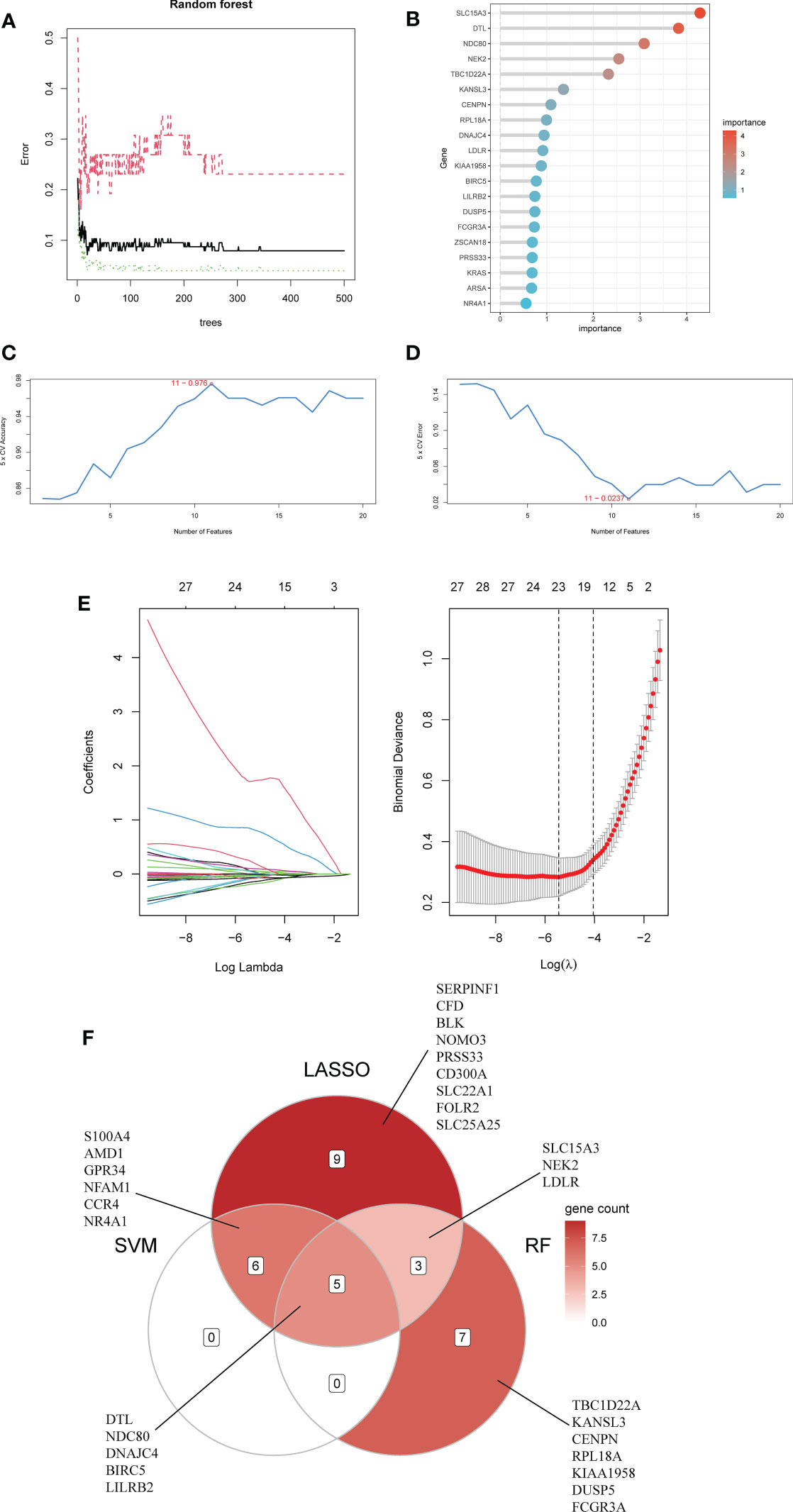
Figure 5 Using Random Forest (RF) to screen characteristic genes from common DEGs: (A) The random forest trees; (B) The importance rankings of features. (C, D) The SVM model with the highest accuracy and lowest error rate was established on 11 characteristic genes. (E) The establishment of LASSO model. (F) The Venn diagram of hub genes identified by three machine algorithms.
The SVM model based on 11 signature genes got the best accuracy (0.976) and the lowest error rate (0.024) (Figures 5C, D). Therefore, 11 genes, including S100A4, DTL, LILRB2, AMD1, BIRC5, NDC80, GPR34, DNAJC4, NFAM1, CCR4, and NR4A1, were potential genes. Using LASSO regression analysis, 23 common specific genes were finally found, including GPR34, DTL, SERPINF1, NEK2, NR4A1, S100A4, CFD, LDLR, NDC80, BLK, NOMO3, SLC15A3, NFAM1, AMD1, PRSS33, BIRC5, LILRB2, CD300A, CCR4, SLC22A1, FOLR2, SLC25A25, and DNAJC4 (Figure 5E). Finally, according to the results of the intersection of the analysis of three machine learning methods, five hub genes (BIRC5, DNAJC4, DTL, LILRB2, and NDC80) were identified using a Venn diagram (Figure 5F).
3.5 Evaluation of expression levels and diagnostic value of hub genes
First, we compared the expression levels of five genes in COVID-19 and controls, finding that the expression of BIRC5, DTL, and NDC80 increased in COVID-19 while DNAJC4 and LILRB2 decreased (Figure 6A). Subsequently, ROC analysis was performed on the BPH and COVID-19 training sets. The area under the curve (AUC) value for all five genes in GSE7307 was greater than 0.714, as was the AUC value for all five genes in GSE157103 (Figures 6B, C). ROC analysis of the COVID-19 validation set (GSE166253) showed that the AUC area of five genes was greater than 0.670 (Figure S2). ROC analysis of the BPH validation set (GSE132714) showed that only four hub genes had AUC areas greater than 0.6, while BIRC5 had an AUC area of 0.542 (Figure S3). The results of the ROC analysis concluded that these hub genes have excellent diagnostic properties for COVID-19 and BPH. In addition, co-expression networks of five hub genes were constructed through gene co-expression network analysis, and gene correlation heatmaps were also built (Figures 6D, E).
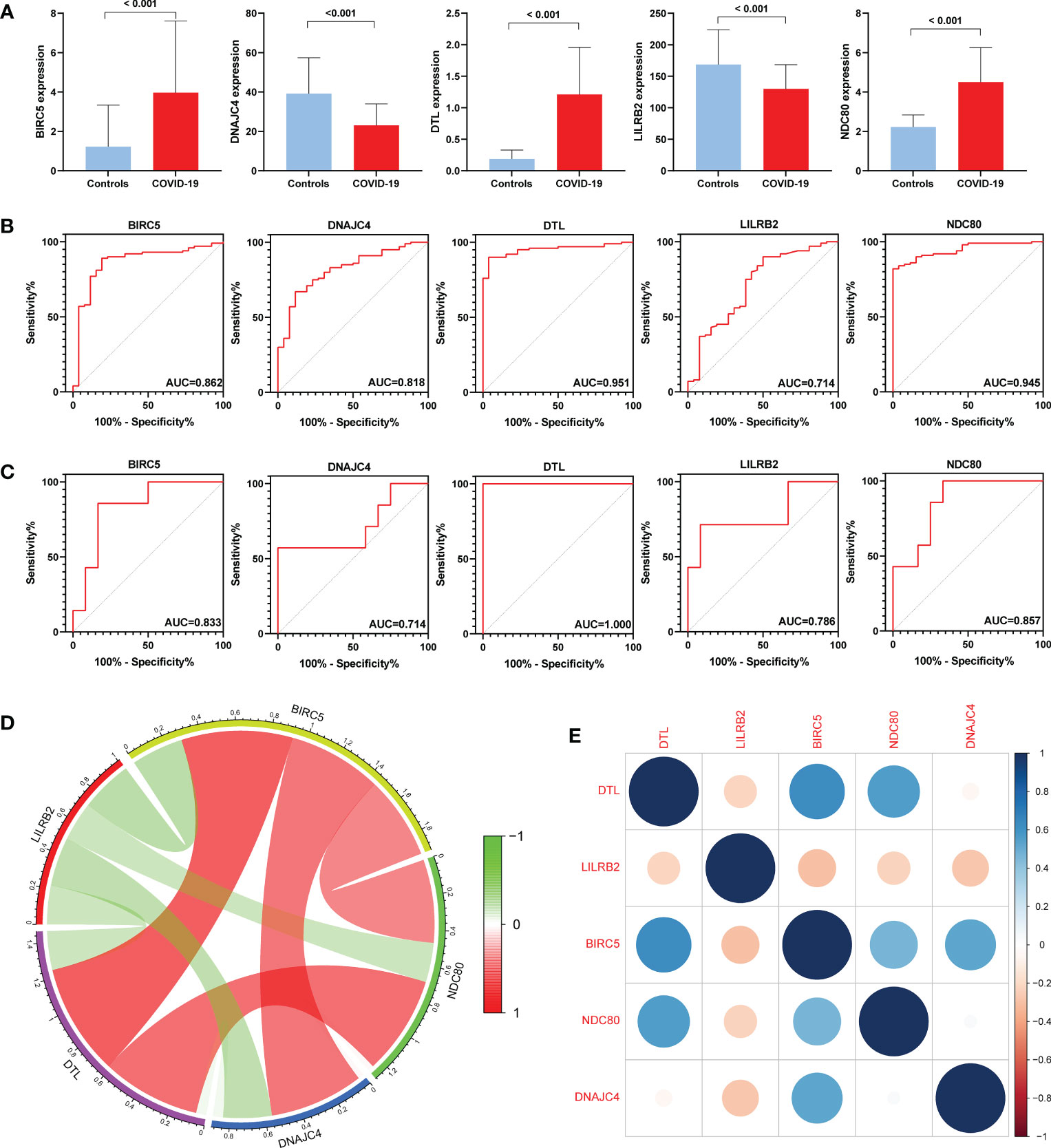
Figure 6 Expression levels and diagnostic significance of hub genes. (A) Expression levels in the COVID-19 set (GSE157103). (B) ROC curves in GSE157103. (C) ROC curves in GSE7307. (D) Constructing co-expression network of hub genes in COVID-19. (E) Heatmap of the hub genes in COVID-19.
3.6 Immune cell infiltration analysis
Ten different types of immune cells were significantly different between COVID-19 and controls according to the CIBERSORT analysis of GSE157103. Five of them are associated with T cells: T cells gamma delta, T cells CD4 naive, T cells CD4 memory activated, T cells follicular helper, and T cells regulatory (Tregs) (Figure 7A). First, BIRC5, DTL, LILRB2, and NDC80 showed positive correlations with CD4 memory activated T cells by the correlation analysis of 5 hub genes and immune cells, but DNAJC4 showed negative correlations with CD4 memory activated T cells. Nevertheless, we found that BIRC5, DTL, LILRB2, and NDC80 had a negative correlation with activated Tregs and NK cells, but DNAJC4 had a positive correlation with them (Figure 7B).
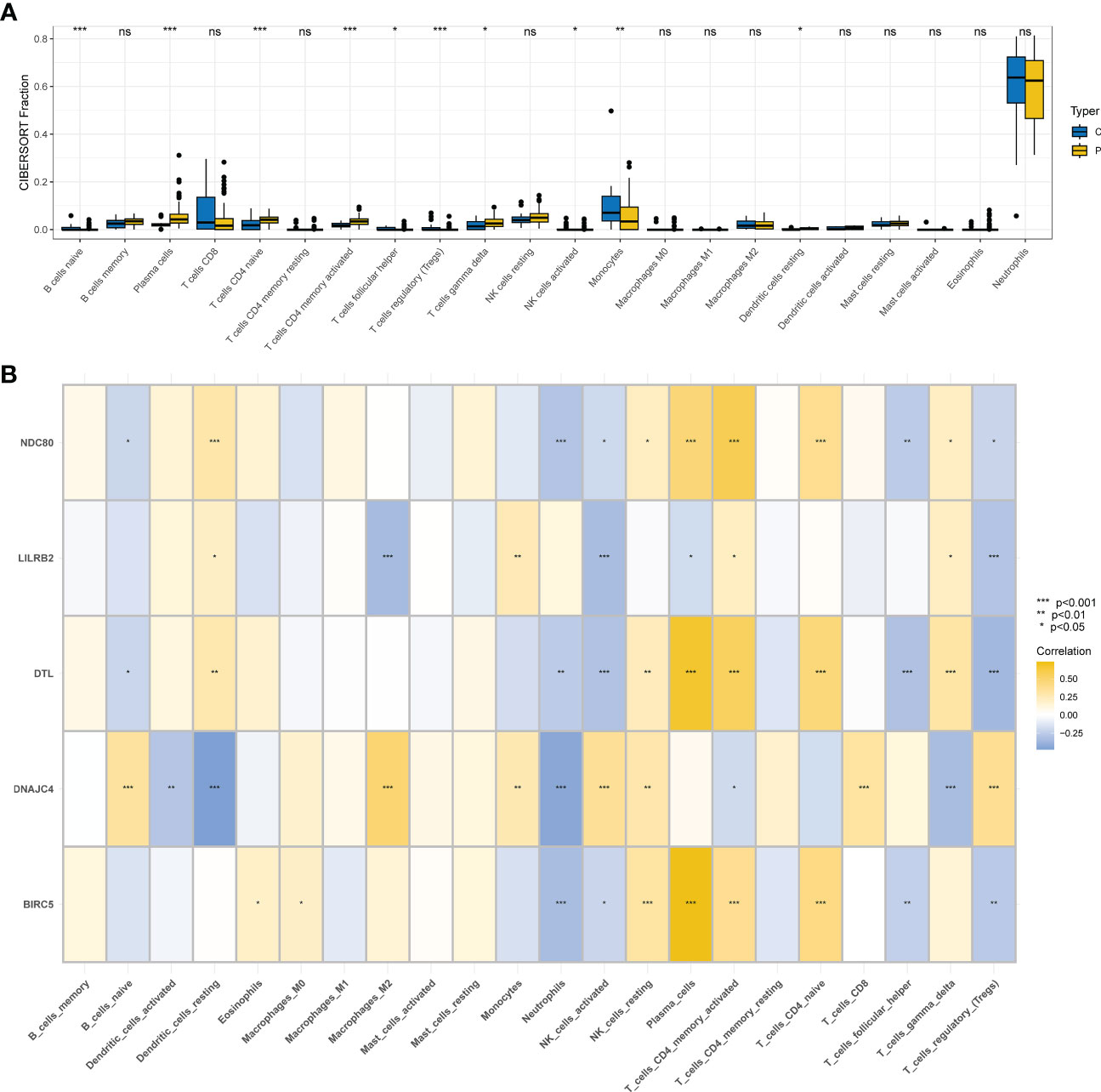
Figure 7 Immunity infiltration analysis based on the CIBERSORT algorithm. (A) Box plot of 22 types of immunity infiltrating cells in the COVID-19 and controls. (B) The correlation between five hub genes and immune cells.
3.7 Prediction of key TFs, MiRNAs, and small-molecule drugs
TFs and miRNAs are two different categories of gene expression regulators. A total of 79 TFs and 5 hub genes were included in the regulatory network of TFs and hub genes that we first examined. The top 10 TFs were ranked according to the betweenes, and the top 10 TFs were SPI1, POU5F1, MYBL2, PDX1, CREB1, CREM, MYC, KDM5B, E2F4, and MYCN (Figure S4). 130 miRNAs and 5 genes in total were engaged in the study of the gene-miRNA regulation network (Figure S5). The above regulatory network suggests a strong correlation between hub genes, TFs and miRNAs.
The DSigDB database was applied to predict small molecule drugs for five hub genes, and the top ten drugs by p-value were lucanthone, phytoestrogens, etoposide, dasatinib, piroxicam, pyrvinium, rapamycin, niclosamide, genistein, and testosterone (Table 1). These identified small molecule compounds may be potential therapeutics for COVID-19 and BPH.
3.8 Identification of disease association
According to previous studies, various diseases are interrelated, and there are common genes (41). We filtered the top ten closely related diseases by importing 97 DEGs into the DisGeNet database and classified them by degree, including liver cirrhosis, schizophrenia, autosomal recessive predisposition, prostatic neoplasms, hypertensive disease, recurrent respiratory infections, diabetes mellitus, asthma, colonic neoplasms and adult T-cell lymphoma/leukemia (Figure 8).
4 Discussion
Many investigations have been conducted since the COVID-19 pandemic to support the theory that many diseases may be correlated with COVID-19 (42–46). As indicated, many male patients were found to have LUTS during COVID-19 clinical therapy of COVID-19, which may be related to BPH. Nevertheless, as of now, we don’t know enough about COVID-19 and BPH. This study sought to identify crucial genes and biological mechanisms that connect COVID-19 to BPH. Using the COVID-19 dataset (GSE157103) and the BPH dataset (GSE7307), we were able to identify 97 common DEGs. We carried out a functional enrichment analysis using KEGG and GO analyses. Five genes (BIRC5, DNAJC4, DTL, LILRB2, and NDC80) were then identified as possible hub genes by three machine learning methods (LASSSO regression, SVM-RFE, and RF).
Using DEGs enrichment analysis, we can better understand the precise mechanisms of action and the regulatory function of genes in the human body. According to KEGG data, these genes seem to be abundant in pathways related to inflammation and infection, including the B-cell receptor signaling pathway, inflammatory bowel disease, and cytotoxicity mediated by natural killer cells. The role of B cells in immunity to SARS-CoV-2 infection and vaccination has been demonstrated in several studies, and the type of SARS-CoV-2 exposure has distinct effects on the formation of B cell receptors (47–50). Russell et al. thought that the spleens of COVID-19 patients had higher levels of some components of the B cell signaling pathway (51). Following SARS-CoV-2 infection, NK cell-mediated cytotoxicity can be reduced as a result of mechanisms that result in a marked reduction in CD16/56+ NK cells and may be related to the severity of the illness or by up-regulation of an inhibitory receptor that regulates NK cell-mediated cytotoxicity (52). Creatinine may boost NK cell-mediated cytotoxic actions to treat individuals with mild to moderate COVID-19, according to recent randomized controlled research (53). Wang et al. confirmed that COVID-19 can cause testicular cell senescence via the MAPK signaling route in addition to inflammation-related pathways and that cellular senescence interacts synergistically with the MAPK pathway to further impair the regular synthesis of cholesterol and androgens (54). It is well recognized that androgens and BPH are closely related (55). High estrogen may cause bladder overactivity by activating the RhoA/ROCK pathway, and altered estrogen/androgen ratios are associated with BPH (56). Estrogen has a pro-inflammatory effect on the prostate, and in men, the combined effects of inflammation, dyslipidemia, and a sex steroid environment can have an impact on the start and progression of BPH (57). A study showed worse prognosis and mortality in SARS-CoV-2 infected men with low testosterone levels (58). This implies that sex steroid hormones represent a significant relationship between COVID-19 and BPH and require more investigation. These common DEGs may also contribute to some chronic inflammatory conditions, like inflammatory bowel disease (IBD), and research points to a potential co-regulatory link between IBD and COVID-19 (59). Altered levels of the enzyme angiotensin converting enzyme 2 (ACE2) may be a co-pathogenic factor in COVID-19 and IBD. If immunotherapy is given to patients with IBD, it may increase the chance of SARS-CoV-2 infection (60). Therefore, further studies are needed for patients with both IBD and COVID-19.
The results of GO analysis showed that the immunological response was the main pathway of the BPs of these common DEGs. The symptoms of SARS-CoV-2 infection are exacerbated by the activation of inflammatory responses, particularly interferon responses (61); when interferon expression is ineffective, SARS-CoV-2 replicates widely, triggering an inflammatory response. This is the case in some people with severe COVID-19 who have delayed or no induction of interferon-I and -III (62). CCs of common DEGs that are primarily concentrated in the secretory granule lumen, the vesicle lumen, and the cytoplasmic vesicle lumen, all of which have been linked to immune cell activity. MFs of common DEGs focus on enzyme inhibitor activity. Recent investigations have discovered that the co-expression of ACE2 and TMPRSS2 in an organ is crucial for viral infection of that organ (63). It is vital to further examine whether the virus affects these organs when ACE2 and TMPRSS2 are co-expressed in other organs such as the testes and prostate (64). One of the essential components of the ACE2/Ang-(1-7)/Mas system, ACE2 is closely related to SARS-CoV-2 infection (64). By reducing Ang-II inflammation and proliferation, it has anti-inflammatory actions. Additionally, Ang (1–7) can reduce inflammation by blocking the NF-B pathway and cytokines (65). Inhibition of ACE2 caused by SARS-CoV-2 infection may activate pro-inflammatory pathways and increase cytokine production, resulting in an inflammatory response in the prostate and worsening of BPH.
In addition, we performed a gene-disease analysis in which there were chronic diseases, including hypertension and diabetes. Angiotensin-converting enzyme inhibitors (ACEI) can reduce excessive inflammation and increase intracellular antiviral responses, while in patients with COVID-19, hypertension inhibits viral clearance and worsens excessive airway inflammation (66). High blood sugar levels may raise the risk of mortality from COVID-19 in diabetics (67). There are also prostatic neoplasms, colonic neoplasms, and T-cell lymphoma/leukemia. A previous study (42) showed that COVID-19 is associated with various tumors, including breast cancer, malignant lymphoma, lymphocytic disorders, and leukemia, which is consistent with our findings. Patients with tumors may be more likely to pass away due to their deteriorating health from the SARS-CoV-2 infection.
We screened five hub genes (BIRC5, DNAJC4, DTL, LILRB2, and NDC80) using three machine learning algorithms, and the other four genes had good diagnostic properties in both the training and validation sets, with the exception of BIRC5, which had an AUC of only 0.542 in the validation set GSE132714 of BPH. We speculated that this may be because the sample size of GSE132714 is too small and further studies are still needed in the future. The apoptosis inhibitory protein family member Survivin can be encoded by BIRC5. According to Beding et al. (68), BIRC5 is highly expressed in 16 different malignancies, including prostate cancer (Pca), and may be used as a diagnostic marker for a number of tumor types. High expression of BIRC5 has been associated with a worse prognosis, tumor stage, and response to therapy in survival and clinicopathology studies. In patients with non-small cell lung cancer with COVID-19, BIRC5, a member of the inhibitor of apoptosis (IAP) gene family, may be a target gene with important predictive significance (69). Chronic inflammation, which has been associated with the formation and progression of Pca, is related to both precancerous and malignant Pca. Myeloid cells, macrophages, and lymphocyte recruitment and growth in the prostate gland can promote DNA double-strand breaks and androgen receptor activation in prostate epithelial cells, accelerating tumor development (70). The proteins encoded by DTL participate in several processes, such as translesion production, control of the G2/M transition of the mitotic cell cycle, and protein ubiquitination. Prior research has shown that DTL may function as a biomarker for COVID-19 (71). LILRB2, a Class I MHC antigen receptor, is implicated in the suppression of immunological responses and the development of tolerance. The decrease of LILRB2 in peripheral blood mononuclear cells (PBMC) suggests that LILRB2 may be a new target to overcome immune evasion and improve vaccination strategies (72). NDC80 is necessary for normal chromosomal segregation, a process closely related to mitosis, and serves to organize and regulate microtubule-kinetochore interactions (73). Aneuploidy development, which is linked to tumors, would result from overexpression of NDC80 because it would interfere with microtubule dynamics and chromosomal segregation in mitosis (74). The cell cycle and cell proliferation in Pca are tightly correlated with the NDC80-related gene Spindle pole body component 25 (SPC25) (75, 76). Previous studies have shown that glucocorticoids can act by modulating DNAJC4. In the treatment of COVID-19, a previous study demonstrated that glucocorticoids can accelerate recovery times and lower hospitalizations (77). Therefore, we hypothesize that DNAJC4 may be an important biological target for glucocorticoids in the treatment of COVID-19 and its complications. The identification of these molecular markers can open up new possibilities for the identification and care of BPH patients who have COVID-19 infections.
It is well known that the development of COVID-19 is highly correlated with immune cell performance. Important components of the adaptive immune system that are crucial in preventing the majority of viral infections are B cells, CD4 T cells, and CD8 T cells (78). Substantial drop in total T cell, CD8 or CD4 T cell counts, especially in the sickest COVID-19 patients (79). Chronic inflammation and immunological dysregulation contribute to the progression of BPH, and in vitro research has shown that the administration of dihydrotestosterone, which inhibits CD4 T cells’ production of pro-inflammatory cytokines, has an immunomodulatory effect (55). In COVID-19, Tregs may have negative consequences by directly promoting inflammation in the most severe phases of the disease and blocking antiviral T-cell responses (80). A new strategy for the treatment and prevention of BPH in clinical practice may be offered by the use of Tregs as cells to reduce inflammation in BPH through CD39 (81). In severe COVID-19, NK cells have impaired antifibrotic function, which may be connected to the development of fibrotic lung disease. NK cells are active against SARS-CoV-2 but perform poorly when COVID-19 is severe (82). Based on the results of our immune infiltration analysis, five hub genes are closely associated with several of the immune cells above and may play key roles in the pathogenesis of BPH and COVID-19. Five hub genes were differentially expressed in COVID-19 patients compared to controls, and they were associated with the activation of regulatory T cells, NK cells, and CD4 memory T cells. Immune infiltration analyses revealed that these three immune cells were differentially expressed in the COVID-19 group and controls. Therefore, we speculate that the five hub genes may influence these three immune cells, which in turn may change the immunological state and inflammatory response.
MiRNAs (83) can regulate target genes and have a significant impact on a variety of biological processes. TFs are proteins that bind to certain DNA sequences to control transcription and gene expression. By binding to particular gene sequences, TFs can perform a crucial function (84). We identified multiple potential drugs that can influence patients with BPH infected with COVID-19. It has been demonstrated that testosterone reduces symptoms through upregulating anti-inflammatory cytokines, downregulating pro-inflammatory cytokines, and changing immunological function (85). Rat prostate weight and testosterone levels can both be decreased by phytoestrogens, according to an animal experiment (86). Phytoestrogens may also have anti-COVID-19 actions and inhibit the adhesion of SARS-CoV-2 to host cells (87). Etoposide causes prostate hyperplasia cells to undergo apoptosis (88). Etoposide, in the meantime, may be used as a salvage therapy to treat the cytokine storm of COVID-19 (89). Rapamycin is a common antifungal drug that may play a role in benign prostatic hyperplasia in rats by affecting autophagy (90). Numerous studies have shown the significance of rapamycin in the prevention and treatment of COVID-19 because it is a mTOR inhibitor and the mTOR pathway plays a significant role in the development and replication of SARS-CoV-2 (91). Genistein may play a role in BPH by inhibiting α1-adrenergic, non-adrenergic, and neurogenic human prostate smooth muscle contraction and stromal cell growth (92). Additionally, genistein may have significant antiviral effects as a strong protease inhibitor of SARS-CoV-2 (93). The predicted drugs mentioned above in COVID-19 with BPH still require more research.
In conclusion, our study has several advantages. First, we are the first to screen DEGs and investigate common biological functions using the COVID-19 and BPH datasets from open databases. Second, we used three machine learning methods to search for hub genes, and two datasets were used to confirm the diagnosis accuracy of hub genes. Also, we investigated the association between hub genes and immune cells using the CIBERSORT approach. Finally, we also predicted how gene transcription levels would be regulated and potential small-molecule medicines. Despite the fact that our study is convincing, it has several limitations. Our work did not use in vivo or in vitro validation experiments; instead, it merely used data from public databases to conduct investigations to find prospective biomarkers. Second, more research needs to be done on the molecular mechanism by which COVID-19 is connected to BPH. In the future, we will conduct more studies to demonstrate the potential role of these hub genes in COVID-19 and BPH.
5 Conclusion
Bioinformatics research of the COVID-19 and BPH databases revealed the biological relationship between COVID-19 and BPH. This study also provided some information on the pathogenesis of COVID-19 and BPH, which confirms the function of inflammation-related pathways and immune cells. Additionally, this study provides prospective small-molecule drugs for therapeutic use. This is crucial to understand the potential common pathogenic and susceptibility pathways between them.
Data availability statement
The original contributions presented in the study are included in the article/Supplementary Material. Further inquiries can be directed to the corresponding author.
Ethics statement
Our data on human participants were obtained from the GEO database, accession numbers GSE157103, GSE166253, GSE7307, and GSE132714.
Author contributions
HZ and MX designed the study and wrote the manuscript; PH and YL performed bioinformatic analysis of the data; CR, ML, and YP collated the data. SW and XL reviewed and revised the final manuscript of the article. All authors contributed to the article and approved the submitted version.
Funding
This work was supported by the National Natural Science Foundation of China (No. 82171594).
Acknowledgments
The authors would like to appreciate the GEO database and the people who contributed to those datasets.
Conflict of interest
The authors declare that the research was conducted in the absence of any commercial or financial relationships that could be construed as a potential conflict of interest.
Publisher’s note
All claims expressed in this article are solely those of the authors and do not necessarily represent those of their affiliated organizations, or those of the publisher, the editors and the reviewers. Any product that may be evaluated in this article, or claim that may be made by its manufacturer, is not guaranteed or endorsed by the publisher.
Supplementary material
The Supplementary Material for this article can be found online at: https://www.frontiersin.org/articles/10.3389/fimmu.2023.1172724/full#supplementary-material
References
1. Wang D, Hu B, Hu C, Zhu F, Liu X, Zhang J, et al. Clinical characteristics of 138 hospitalized patients with 2019 novel coronavirus-infected pneumonia in wuhan, China. Jama (2020) 323:1061–69. doi: 10.1001/jama.2020.1585
2. Finkel Y, Mizrahi O, Nachshon A, Weingarten-Gabbay S, Morgenstern D, Yahalom-Ronen Y, et al. The coding capacity of SARS-CoV-2. Nature (2021) 589:125–30. doi: 10.1038/s41586-020-2739-1
3. Eckermann M, Frohn J, Reichardt M, Osterhoff M, Sprung M, Westermeier F, et al. 3D virtual pathohistology of lung tissue from covid-19 patients based on phase contrast X-ray tomography. eLife (2020) 9:e60408. doi: 10.7554/eLife.60408
4. Xie Y, Xu E, Bowe B, Al-Aly Z. Long-term cardiovascular outcomes of COVID-19. Nat Med (2022) 28:583–90. doi: 10.1038/s41591-022-01689-3
5. Russo FP, Burra P, Zanetto A. COVID-19 and liver disease: where are we now? nature reviews. Gastroenterol Hepatol (2022) 19:277–78. doi: 10.1038/s41575-022-00607-9
6. Hingorani KS, Bhadola S, Cervantes-Arslanian AM. COVID-19 and the brain. Trends Cardiovasc Med (2022) 32:323–30. doi: 10.1016/j.tcm.2022.04.004
7. Copur S, Berkkan M, Basile C, Tuttle K, Kanbay M. Post-acute COVID-19 syndrome and kidney diseases: what do we know? J Nephrol (2022) 35:795–805. doi: 10.1007/s40620-022-01296-y
8. Ye L, Yang Z, Liu J, Liao L, Wang F. Digestive system manifestations and clinical significance of coronavirus disease 2019: a systematic literature review. J Gastroenterol Hepatol (2021) 36:1414–22. doi: 10.1111/jgh.15323
9. Vitiello A, Ferrara F, Troiano V, La Porta R. COVID-19 vaccines and decreased transmission of SARS-CoV-2. Inflammopharmacology (2021) 29:1357–60. doi: 10.1007/s10787-021-00847-2
10. Polack FP, Thomas SJ, Kitchin N, Absalon J, Gurtman A, Lockhart S, et al. Safety and efficacy of the BNT162b2 mRNA covid-19 vaccine. New Engl J Med (2020) 383:2603–15. doi: 10.1056/NEJMoa2034577
11. Knoll MD, Wonodi C. Oxford-AstraZeneca COVID-19 vaccine efficacy. Lancet (London England) (2021) 397:72–4. doi: 10.1016/s0140-6736(20)32623-4
12. Saravolatz LD, Depcinski S, Sharma M. Molnupiravir and nirmatrelvir-ritonavir: oral coronavirus disease 2019 antiviral drugs. Clin Infect Dis (2023) 76:165–71. doi: 10.1093/cid/ciac180
13. McKee DL, Sternberg A, Stange U, Laufer S, Naujokat C. Candidate drugs against SARS-CoV-2 and COVID-19. Pharmacol Res (2020) 157:104859. doi: 10.1016/j.phrs.2020.104859
14. Andrews N, Stowe J, Kirsebom F, Toffa S, Rickeard T, Gallagher E, et al. Covid-19 vaccine effectiveness against the omicron (B.1.1.529) variant. New Engl J Med (2022) 386:1532–46. doi: 10.1056/NEJMoa2119451
15. Lauring AS, Tenforde MW, Chappell JD, Gaglani M, Ginde AA, McNeal T, et al. Clinical severity of, and effectiveness of mRNA vaccines against, covid-19 from omicron, delta, and alpha SARS-CoV-2 variants in the united states: prospective observational study. BMJ (Clinical Res ed.) (2022) 376:e069761. doi: 10.1136/bmj-2021-069761
16. Roehrborn CG. Pathology of benign prostatic hyperplasia. Int J impotence Res (2008) 2(Suppl 3):S11–8. doi: 10.1038/ijir.2008.55
17. Devlin CM, Simms MS, Maitland NJ. Benign prostatic hyperplasia - what do we know? BJU Int (2021) 127:389–99. doi: 10.1111/bju.15229
18. Lee SWH, Chan EMC, Lai YK. The global burden of lower urinary tract symptoms suggestive of benign prostatic hyperplasia: a systematic review and meta-analysis. Sci Rep (2017) 7:7984. doi: 10.1038/s41598-017-06628-8
19. Li LQ, Huang T, Wang YQ, Wang ZP, Liang Y, Huang TB, et al. COVID-19 patients' clinical characteristics, discharge rate, and fatality rate of meta-analysis. J Med Virol (2020) 92:577–83. doi: 10.1002/jmv.25757
20. Balawender K, Pliszka A, Krowiak A, Sito M, Grabarek BO, Boroń D. Does SARS-CoV-2 affect Male urogenital system? Curr Pharm Biotechnol (2022) 23:1792–99. doi: 10.2174/1389201023666220307102147
21. Sighinolfi MC, Rocco B, Mussini C. COVID-19: importance of the awareness of the clinical syndrome by urologists. Eur Urol (2020) 78:e40–1. doi: 10.1016/j.eururo.2020.03.029
22. Can O, Erkoç M, Ozer M, Karakanli MU, Otunctemur A. The effect of COVID-19 on lower urinary tract symptoms in elderly men. Int J Clin Pract (2021) 75:e14110. doi: 10.1111/ijcp.14110
23. Creta M, Sagnelli C, Celentano G, Napolitano L, La Rocca R, Capece M, et al. SARS-CoV-2 infection affects the lower urinary tract and male genital system: a systematic review. J Med Virol (2021) 93:3133–42. doi: 10.1002/jmv.26883
24. Mullah MAS, Hanley JA, Benedetti A. LASSO type penalized spline regression for binary data. BMC Med Res Method (2021) 21:83. doi: 10.1186/s12874-021-01234-9
25. Tian XP, Su N, Wang L, Huang WJ, Liu YH, Zhang X, et al. A CpG methylation classifier to predict relapse in adults with T-cell lymphoblastic lymphoma. Clin Cancer Res (2020) 26:3760–70. doi: 10.1158/1078-0432.Ccr-19-4207
26. Wang H, Yang F, Luo Z. An experimental study of the intrinsic stability of random forest variable importance measures. BMC Bioinf (2016) 17:60. doi: 10.1186/s12859-016-0900-5
27. Zhang J, Huang C, Liu Z, Ren S, Shen Z, Han K, et al. Screening of potential biomarkers in the peripheral serum for steroid-induced osteonecrosis of the femoral head based on WGCNA and machine learning algorithms. Dis Markers (2022) 2022:2639470. doi: 10.1155/2022/2639470
28. Qu LH, Luo WJ, Yan ZG, Liu WP. FAM171B as a novel biomarker mediates tissue immune microenvironment in pulmonary arterial hypertension. Mediators Inflammation (2022) 2022:1878766. doi: 10.1155/2022/1878766
29. Ritchie ME, Phipson B, Wu D, Hu Y, Law CW, Shi W, et al. Limma powers differential expression analyses for RNA-sequencing and microarray studies. Nucleic Acids Res (2015) 43:e47. doi: 10.1093/nar/gkv007
30. Yu G, Wang LG, Han Y, He QY. clusterProfiler: an r package for comparing biological themes among gene clusters. Omics (2012) 16:284–7. doi: 10.1089/omi.2011.0118
31. Szklarczyk D, Gable AL, Nastou KC, Lyon D, Kirsch R, Pyysalo S, et al. The STRING database in 2021: customizable protein-protein networks, and functional characterization of user-uploaded gene/measurement sets. Nucleic Acids Res (2021) 49:D605–d12. doi: 10.1093/nar/gkaa1074
32. Ishwaran H, Lu M. Standard errors and confidence intervals for variable importance in random forest regression, classification, and survival. Stat Med (2019) 38:558–82. doi: 10.1002/sim.7803
33. Friedman J, Hastie T, Tibshirani R. Regularization paths for generalized linear models via coordinate descent. J Stat software (2010) 33:1–22. doi: 10.18637/jss.v033.i01
34. Huang ML, Hung YH, Lee WM, Li RK, Jiang BR. SVM-RFE based feature selection and taguchi parameters optimization for multiclass SVM classifier. TheScientificWorldJournal (2014) 2014:795624. doi: 10.1155/2014/795624
35. Newman AM, Liu CL, Green MR, Gentles AJ, Feng W, Xu Y, et al. Robust enumeration of cell subsets from tissue expression profiles. Nat Methods (2015) 12:453–7. doi: 10.1038/nmeth.3337
36. Finotello F, Trajanoski Z. Quantifying tumor-infiltrating immune cells from transcriptomics data. Cancer immunol immunother CII (2018) 67:1031–40. doi: 10.1007/s00262-018-2150-z
37. Lachmann A, Xu H, Krishnan J, Berger SI, Mazloom AR, Ma'ayan A. ChEA: transcription factor regulation inferred from integrating genome-wide ChIP-X experiments. Bioinf (Oxford England) (2010) 26:2438–44. doi: 10.1093/bioinformatics/btq466
38. Papadopoulos GL, Reczko M, Simossis VA, Sethupathy P, Hatzigeorgiou AG. The database of experimentally supported targets: a functional update of TarBase. Nucleic Acids Res (2009) 37:D155–8. doi: 10.1093/nar/gkn809
39. Hu H, Tang N, Zhang F, Li L, Li L. Bioinformatics and system biology approach to identify the influences of COVID-19 on rheumatoid arthritis. Front Immunol (2022) 13:860676. doi: 10.3389/fimmu.2022.860676
40. Piñero J, Ramírez-Anguita JM, Saüch-Pitarch J, Ronzano F, Centeno E, Sanz F, et al. The DisGeNET knowledge platform for disease genomics: 2019 update. Nucleic Acids Res (2020) 48:D845–d55. doi: 10.1093/nar/gkz1021
41. Mahmud SMH, Al-Mustanjid M, Akter F, Rahman MS, Ahmed K, Rahman MH, et al. Bioinformatics and system biology approach to identify the influences of SARS-CoV-2 infections to idiopathic pulmonary fibrosis and chronic obstructive pulmonary disease patients. Briefings Bioinf (2021) 22(5):bbab115. doi: 10.1093/bib/bbab115
42. Yan C, Niu Y, Wang X. Blood transcriptome analysis revealed the crosstalk between COVID-19 and HIV. Front Immunol (2022) 13:1008653. doi: 10.3389/fimmu.2022.1008653
43. Lu L, Liu LP, Gui R, Dong H, Su YR, Zhou XH, et al. Discovering common pathogenetic processes between COVID-19 and sepsis by bioinformatics and system biology approach. Front Immunol (2022) 13:975848. doi: 10.3389/fimmu.2022.975848
44. Rahman MR, Islam T, Shahjaman M, Islam MR, Lombardo SD, Bramanti P, et al. Discovering common pathogenetic processes between COVID-19 and diabetes mellitus by differential gene expression pattern analysis. Briefings Bioinf (2021) 22(6):bbab262. doi: 10.1093/bib/bbab262
45. Zhang F, Yu C, Xu W, Li X, Feng J, Shi H, et al. Identification of critical genes and molecular pathways in COVID-19 myocarditis and constructing gene regulatory networks by bioinformatic analysis. PloS One (2022) 17:e0269386. doi: 10.1371/journal.pone.0269386
46. Chen Q, Xia S, Sui H, Shi X, Huang B, Wang T. Identification of hub genes associated with COVID-19 and idiopathic pulmonary fibrosis by integrated bioinformatics analysis. PloS One (2022) 17:e0262737. doi: 10.1371/journal.pone.0262737
47. Kotagiri P, Mescia F, Rae WM, Bergamaschi L, Tuong ZK, Turner L, et al. B cell receptor repertoire kinetics after SARS-CoV-2 infection and vaccination. Cell Rep (2022) 38:110393. doi: 10.1016/j.celrep.2022.110393
48. Lin K, Zhou Y, Ai J, Wang YA, Zhang S, Qiu C, et al. B cell receptor signatures associated with strong and poor SARS-CoV-2 vaccine responses. Emerg Microbes infect (2022) 11:452–64. doi: 10.1080/22221751.2022.2030197
49. Wen W, Su W, Tang H, Le W, Zhang X, Zheng Y, et al. Immune cell profiling of COVID-19 patients in the recovery stage by single-cell sequencing. Cell Discovery (2020) 6:31. doi: 10.1038/s41421-020-0168-9
50. Funakoshi Y, Ohji G, Yakushijin K, Ebisawa K, Arakawa Y, Saegusa J, et al. Massive surge of mRNA expression of clonal b-cell receptor in patients with COVID-19. Heliyon (2021) 7:e07748. doi: 10.1016/j.heliyon.2021.e07748
51. Russell CD, Valanciute A, Gachanja NN, Stephen J, Penrice-Randal R, Armstrong SD, et al. Tissue proteomic analysis identifies mechanisms and stages of immunopathology in fatal COVID-19. Am J Respir Cell Mol Biol (2022) 66:196–205. doi: 10.1165/rcmb.2021-0358OC
52. Bouayad A. Innate immune evasion by SARS-CoV-2: comparison with SARS-CoV. Rev Med Virol (2020) 30:1–9. doi: 10.1002/rmv.2135
53. Jayanthi CR, Swain AK, Ganga RT, Halnor D, Avhad A, Khan MS, et al. Efficacy and safety of inosine pranobex in COVID-19 patients: a multicenter phase 3 randomized double-blind, placebo-controlled trial. Advanced Ther (2022) 2200159. doi: 10.1002/adtp.202200159
54. Wang Z, Ma Y, Chen Z, Yang R, Liu Q, Pan J, et al. COVID-19 inhibits spermatogenesis in the testes by inducing cellular senescence. Front Genet (2022) 13:981471. doi: 10.3389/fgene.2022.981471
55. Vignozzi L, Cellai I, Santi R, Lombardelli L, Morelli A, Comeglio P, et al. Antiinflammatory effect of androgen receptor activation in human benign prostatic hyperplasia cells. J Endocrinol (2012) 214:31–43. doi: 10.1530/joe-12-0142
56. Chavalmane AK, Comeglio P, Morelli A, Filippi S, Fibbi B, Vignozzi L, et al. Sex steroid receptors in male human bladder: expression and biological function. J sexual Med (2010) 7:2698–713. doi: 10.1111/j.1743-6109.2010.01811.x
57. Vignozzi L, Rastrelli G, Corona G, Gacci M, Forti G, Maggi M. Benign prostatic hyperplasia: a new metabolic disease? J endocrinological Invest (2014) 37:313–22. doi: 10.1007/s40618-014-0051-3
58. Rastrelli G, Di Stasi V, Inglese F, Beccaria M, Garuti M, Di Costanzo D, et al. Low testosterone levels predict clinical adverse outcomes in SARS-CoV-2 pneumonia patients. Andrology (2021) 9:88–98. doi: 10.1111/andr.12821
59. Tao SS, Wang XY, Yang XK, Liu YC, Fu ZY, Zhang LZ, et al. COVID-19 and inflammatory bowel disease crosstalk: from emerging association to clinical proposal. J Med Virol (2022) 94:5640–52. doi: 10.1002/jmv.28067
60. Neurath MF. COVID-19 and immunomodulation in IBD. Gut (2020) 69:1335–42. doi: 10.1136/gutjnl-2020-321269
61. Lowery SA, Sariol A, Perlman S. Innate immune and inflammatory responses to SARS-CoV-2: implications for COVID-19. Cell Host Microbe (2021) 29:1052–62. doi: 10.1016/j.chom.2021.05.004
62. Galani IE, Rovina N, Lampropoulou V, Triantafyllia V, Manioudaki M, Pavlos E, et al. Untuned antiviral immunity in COVID-19 revealed by temporal type I/III interferon patterns and flu comparison. Nat Immunol (2021) 22:32–40. doi: 10.1038/s41590-020-00840-x
63. Hoffmann M, Kleine-Weber H, Schroeder S, Krüger N, Herrler T, Erichsen S, et al. SARS-CoV-2 cell entry depends on ACE2 and TMPRSS2 and is blocked by a clinically proven protease inhibitor. Cell (2020) 181:271–80.e8. doi: 10.1016/j.cell.2020.02.052
64. Haghpanah A, Masjedi F, Salehipour M, Hosseinpour A, Roozbeh J, Dehghani A. Is COVID-19 a risk factor for progression of benign prostatic hyperplasia and exacerbation of its related symptoms?: a systematic review. Prostate Cancer prostatic Dis (2022) 25:27–38. doi: 10.1038/s41391-021-00388-3
65. Mahmudpour M, Roozbeh J, Keshavarz M, Farrokhi S, Nabipour I. COVID-19 cytokine storm: the anger of inflammation. Cytokine (2020) 133:155151. doi: 10.1016/j.cyto.2020.155151
66. Trump S, Lukassen S, Anker MS, Chua RL, Liebig J, Thürmann L, et al. Hypertension delays viral clearance and exacerbates airway hyperinflammation in patients with COVID-19. Nat Biotechnol (2021) 39:705–16. doi: 10.1038/s41587-020-00796-1
67. Hartmann-Boyce J, Rees K, Perring JC, Kerneis SA, Morris EM, Goyder C, et al. Risks of and from SARS-CoV-2 infection and COVID-19 in people with diabetes: a systematic review of reviews. Diabetes Care (2021) 44:2790–811. doi: 10.2337/dc21-0930
68. Fäldt Beding A, Larsson P, Helou K, Einbeigi Z, Parris TZ. Pan-cancer analysis identifies BIRC5 as a prognostic biomarker. BMC Cancer (2022) 22:322. doi: 10.1186/s12885-022-09371-0
69. Zhuang Z, Chen Q, Zhong X, Chen H, Yu R, Tang Y. Ginsenoside Rg3, a promising agent for NSCLC patients in the pandemic: a large-scale data mining and systemic biological analysis. J ginseng Res (2022) 47(2):291–301. doi: 10.1016/j.jgr.2022.09.006
70. de Bono JS, Guo C, Gurel B, De Marzo AM, Sfanos KS, Mani RS, et al. Prostate carcinogenesis: inflammatory storms. Nat Rev Cancer (2020) 20:455–69. doi: 10.1038/s41568-020-0267-9
71. Auwul MR, Rahman MR, Gov E, Shahjaman M, Moni MA. Bioinformatics and machine learning approach identifies potential drug targets and pathways in COVID-19. Briefings Bioinf (2021) 22(5):bbab120. doi: 10.1093/bib/bbab120
72. He Y, Sun M, Xu Y, Hu C, Wang Y, Zhang Y, et al. Weighted gene co-expression network-based identification of genetic effect of mRNA vaccination and previous infection on SARS-CoV-2 infection. Cell Immunol (2023) 385:104689. doi: 10.1016/j.cellimm.2023.104689
73. Powers AF, Franck AD, Gestaut DR, Cooper J, Gracyzk B, Wei RR, et al. The Ndc80 kinetochore complex forms load-bearing attachments to dynamic microtubule tips via biased diffusion. Cell (2009) 136:865–75. doi: 10.1016/j.cell.2008.12.045
74. Tang NH, Toda T. MAPping the Ndc80 loop in cancer: a possible link between Ndc80/Hec1 overproduction and cancer formation. BioEssays (2015) 37:248–56. doi: 10.1002/bies.201400175
75. Cui F, Tang H, Tan J, Hu J. Spindle pole body component 25 regulates stemness of prostate cancer cells. Aging (2018) 10:3273–82. doi: 10.18632/aging.101631
76. Cui F, Hu J, Fan Y, Tan J, Tang H. Knockdown of spindle pole body component 25 homolog inhibits cell proliferation and cycle progression in prostate cancer. Oncol Lett (2018) 15:5712–20. doi: 10.3892/ol.2018.8003
77. Yu LM, Bafadhel M, Dorward J, Hayward G, Saville BR, Gbinigie O, et al. Inhaled budesonide for COVID-19 in people at high risk of complications in the community in the UK (PRINCIPLE): a randomised, controlled, open-label, adaptive platform trial. Lancet (London England) (2021) 398:843–55. doi: 10.1016/s0140-6736(21)01744-x
78. Sette A, Crotty S. Adaptive immunity to SARS-CoV-2 and COVID-19. Cell (2021) 184:861–80. doi: 10.1016/j.cell.2021.01.007
79. Diao B, Wang C, Tan Y, Chen X, Liu Y, Ning L, et al. Reduction and functional exhaustion of T cells in patients with coronavirus disease 2019 (COVID-19). Front Immunol (2020) 11:827. doi: 10.3389/fimmu.2020.00827
80. Galván-Peña S, Leon J, Chowdhary K, Michelson DA, Vijaykumar B, Yang L, et al. Profound treg perturbations correlate with COVID-19 severity. Proc Natl Acad Sci United States America (2021) 118(37):e2111315118. doi: 10.1073/pnas.2111315118
81. Jin X, Lin T, Yang G, Cai H, Tang B, Liao X, et al. Use of tregs as a cell-based therapy via CD39 for benign prostate hyperplasia with inflammation. J Cell Mol Med (2020) 24:5082–96. doi: 10.1111/jcmm.15137
82. Krämer B, Knoll R, Bonaguro L, ToVinh M, Raabe J, Astaburuaga-García R, et al. Early IFN-α signatures and persistent dysfunction are distinguishing features of NK cells in severe COVID-19. Immunity (2021) 54:2650–69.e14. doi: 10.1016/j.immuni.2021.09.002
83. O'Brien J, Hayder H, Zayed Y, Peng C. Overview of MicroRNA biogenesis, mechanisms of actions, and circulation. Front Endocrinol (2018) 9:402. doi: 10.3389/fendo.2018.00402
84. Chen K, Rajewsky N. The evolution of gene regulation by transcription factors and microRNAs. Nat Rev Genet (2007) 8:93–103. doi: 10.1038/nrg1990
85. Al-Kuraishy HM, Al-Gareeb AI, Faidah H, Alexiou A, Batiha GE. Testosterone in COVID-19: an adversary bane or comrade boon. Front Cell infect Microbiol (2021) 11:666987. doi: 10.3389/fcimb.2021.666987
86. Weber KS, Setchell KD, Stocco DM, Lephart ED. Dietary soy-phytoestrogens decrease testosterone levels and prostate weight without altering LH, prostate 5alpha-reductase or testicular steroidogenic acute regulatory peptide levels in adult male sprague-dawley rats. J Endocrinol (2001) 170:591–9. doi: 10.1677/joe.0.1700591
87. Elfiky AA. Natural products may interfere with SARS-CoV-2 attachment to the host cell. J biomol struct dyn (2021) 39:3194–203. doi: 10.1080/07391102.2020.1761881
88. Cheng L, Wang Y, Wang J, Qin H, Zhu G, Tao L. Cytotoxic action of palladium-based compound on prostate stem cells, primary prostate epithelial cells, prostate epithelial cells, and prostate cell lines. J healthc Eng (2022) 2022:4993405. doi: 10.1155/2022/4993405
89. Patel M, Dominguez E, Sacher D, Desai P, Chandar A, Bromberg M, et al. Etoposide as salvage therapy for cytokine storm due to coronavirus disease 2019. Chest (2021) 159:e7–e11. doi: 10.1016/j.chest.2020.09.077
90. Liu RF, Fu G, Li J, Yang YF, Wang XG, Bai PD, et al. Roles of autophagy in androgen-induced benign prostatic hyperplasia in castrated rats. Exp Ther Med (2018) 15:2703–10. doi: 10.3892/etm.2018.5772
91. Karam BS, Morris RS, Bramante CT, Puskarich M, Zolfaghari EJ, Lotfi-Emran S, et al. mTOR inhibition in COVID-19: a commentary and review of efficacy in RNA viruses. J Med Virol (2021) 93:1843–46. doi: 10.1002/jmv.26728
92. Huang R, Liu Y, Hu S, Tamalunas A, Waidelich R, Strittmatter F, et al. Inhibition of α(1)-adrenergic, non-adrenergic and neurogenic human prostate smooth muscle contraction and of stromal cell growth by the isoflavones genistein and daidzein. Nutrients (2022) 14(23):4943. doi: 10.3390/nu14234943
Keywords: COVID-19, benign prostatic hyperplasia, functional enrichment, hub genes, machine learning algorithms
Citation: Zhou H, Xu M, Hu P, Li Y, Ren C, Li M, Pan Y, Wang S and Liu X (2023) Identifying hub genes and common biological pathways between COVID-19 and benign prostatic hyperplasia by machine learning algorithms. Front. Immunol. 14:1172724. doi: 10.3389/fimmu.2023.1172724
Received: 23 February 2023; Accepted: 07 June 2023;
Published: 23 June 2023.
Edited by:
William Tolbert, Henry M Jackson Foundation for the Advancement of Military Medicine (HJF), United StatesReviewed by:
Linda Vignozzi, University of Florence, ItalyYupeng Wu, First Affiliated Hospital of Fujian Medical University, China
Yuxuan Song, Peking University People’s Hospital, China
Copyright © 2023 Zhou, Xu, Hu, Li, Ren, Li, Pan, Wang and Liu. This is an open-access article distributed under the terms of the Creative Commons Attribution License (CC BY). The use, distribution or reproduction in other forums is permitted, provided the original author(s) and the copyright owner(s) are credited and that the original publication in this journal is cited, in accordance with accepted academic practice. No use, distribution or reproduction is permitted which does not comply with these terms.
*Correspondence: Xiaoqiang Liu, xiaoqiangliu1@163.com
†These authors have contributed equally to this work