- Department of Respiratory and Critical Care Medicine, The First Affiliated Hospital of Chongqing Medical University, Chongqing Medical University, Chongqing, China
Background: A growing number of research strongly suggest that metabolic syndrome and dyslipidemia contribute to the establishment of a pro-inflammatory state in asthma, according to accumulating data. However, the majority of recent research has focused on the association between lipids and asthma in children and adolescents, with contradictory findings. Consequently, we analyzed the relationship between serum lipid and blood eosinophil counts using data from the NHANES in the USA.
Methods: After screening the individuals from the 2011 to 2018 NHANES survey, a total of 2,544 out of 39156 participants were eligible for our study. The potential association was discussed using the linear regression model, XGBoost algorithm model, generalized additive model, and two-piecewise linear regression model. In addition, we ran stratified analysis to identify specific demographics.
Results: After adjusting for covariates, the result indicated that blood eosinophil counts decreased by 45.68 (-68.56, -22.79)/uL for each additional unit of HDL-C (mmol/L). But serum LDL-C, total cholesterol or triglyceride was not correlated with blood eosinophil counts. Furthermore, we used machine learning of the XGBoost model to determine LDL-C, age, BMI, triglyceride, and HDL-C were the five most critical variables in the blood eosinophil counts. The generalized additive model and two-piecewise linear regression model were used to further identify linear relationship between the serum HDL-C and blood eosinophil counts.
Conclusions: Our study elucidated a negative and linear correlation between serum HDL-C and blood eosinophil counts among American asthmatic adults, suggesting that serum HDL-C levels might be associated with the immunological condition of asthmatic adults. There was no correlation between serum LDL-C, total cholesterol, or triglyceride levels and blood eosinophil counts.
1 Introduction
Asthma is a chronic respiratory disease with global prevalence, affecting 1–18% of the populations of different countries (1). Asthma is a complex and heterogeneous disorder resulting from multiple inflammatory mechanisms (2). Eosinophilic airway inflammation has emerged as a defining characteristic of one severe form of asthma, to the point where asthma is routinely classified as eosinophilic or noneosinophilic (2). In the activation of eosinophilic and non-eosinophilic-mediated inflammation pathways, eosinophils are the most important cell type (3). More than 80% of patients in a global real-life severe asthma cohort were likely to have an eosinophilic phenotype (4). Eosinophil (Eos) is the predominant inflammatory effector cell in eosinophilic asthma (EA). EA is characterized by elevated eosinophils in peripheral blood and airway tissue (5, 6). In addition, eosinophil counts in the blood correlate with disease severity in EA (7).
Lipid metabolism issues are known to be linked to inflammation. In recent years, a growing number of studies have strongly suggested that metabolic syndrome and dyslipidemia contribute to the development of an asthmatic pro-inflammatory state (8–10). However, the majority of recent research has focused on the association between lipids and asthma in children and adolescents, with contradictory findings (11). The relationship between lipid profiles and asthma in adults has received less attention, and large-scale studies are lacking.
Consequently, the purpose of this study was to examine the relationship between lipid profiles and peripheral blood eosinophil counts in adults with asthma. The National Health and Nutrition Examination Survey (NHANES) was investigated for secondary data analysis. After controlling for a large number of confounding variables, we attempted to clarify the relationship between serum lipid and blood eosinophil counts among asthmatic patients in the United States and to explain the contradictory findings.
2 Materials and methods
2.1 Data source
Every two years, the National Health and Nutrition Examination Survey (NHANES), conducted by the Centers for Disease Control and Prevention (CDC), collected data on the health and nutritional status of the U.S. population. NHANES utilized a complex, stratified sampling design to select samples of non-institutionalized civilians that are representative of the population. The NCHS Institutional Review Board approved the NHANES database in accordance with the revised Helsinki Declaration. Before the data collection procedures and exhaustive health examinations, the informed consent forms were completed.
2.2 Study population
Our study applied data from four NHANES cycles (2011–2018). For the second analysis, these data included demographic data, examination data, laboratory data, diet data, and questionnaire data. When we initially screened the NHANES database, all participants (n=39156) from 2011 to 2018 were included. We included following individuals: (1) age≥18 years old (N=23825) (2) complete blood eosinophil counts data (N=21678) (3) complete serum lipid data (N=21237). Meanwhile, in the process of further screening, the following individuals were excluded: (1) individuals without asthma (n=18009) (2) missing data about covariates at least one of the following (n=684): education level; marital status; poverty to income ratio (PIR); BMI; smoked status; alcohol intake; hypertension history; diabetes history; COPD history. In the end, a total of 2544 asthmatic American adults were attended in our study. Figure 1 depicts the flowchart for the screening procedure.
2.3 Measurement of serum lipid
The lipid profile was evaluated using samples from subjects who had fasted for at least 8.5 h but less than 24 h. Using enzymatic assays, total cholesterol (TC), triglyceride (TG), high density lipoprotein-cholesterol (HDL-C), and low density lipoprotein-cholesterol (LDL-C) levels were determined. On the NHANES website, you can find thorough instructions for specimen collection and processing.
2.4 Measurement of blood eosinophil counts
Blood differential counts were performed by using the Beckman Coulter HMX (Beckman Coulter, Fullerton, Calif), a quantitative and automated hematologic analyzer and leukocyte differential cell counter for in vitro diagnostic use in clinical laboratories. On the NHANES website, you can find a detailed description of laboratory procedures.
2.5 Covariates and asthma assessment
We chose the following variables as potential covariates in our study due to some confounding factors that may affect the results. Participants’ continuous variables consisted of age (years old), poverty to income ratio (grouped by trisection: low, middle, high), BMI (kg/m2) and alcohol intake (gm). And categorical variables were comprised of race/ethnicity (Mexican American, other Hispanic, non-Hispanic white, non-Hispanic black, others), educational level (less than high school, high school, more than high school), marital status (married, single, living with a partner), smoking status (whether smoked at least 100 cigarettes in life), lipid-lowering drugs use (whether lipid-lowering drugs use in past 30 days), antiallergic drugs use (whether antiallergic drugs use in past 30 days; antiallergic drugs include antihistamines and glucocorticoids), hypertension history (Yes, No) and diabetes history (Yes, No, Borderline), and COPD history (Yes, No). The assessment of asthma was based on the information from the questionnaire section of the US National Health Interview Survey. In order to assess asthma, participants were asked, “Has a doctor or other health professional ever told you that you have asthma?” If the participant responds “yes,” he or she was regarded as asthma patient. The official NHANES website (http://www.cdc.gov/nchs/nhanes/) contains a more comprehensive description of the variables.
2.6 Statistical analysis
We used complex sample analyses to explore the relationships between serum lipid and blood eosinophil counts among asthmatic adults in the United States, in accordance with CDC guidelines. Continuous and categorical variables were presented separately as means ± SD and percentages. First, eosinophil counts in the blood were transformed into four quartiles. We calculated the p-value of categorical variables using the weighted chi-square test, and the p-value of continuous variables using the Kruskal Wallis rank sum test. If the count variable has a theoretical value less than 10, we calculated the p-value using Fisher’s exact probability test. Second, three weighted univariable and multivariable linear regression models were developed to investigate the associations between serum lipid and blood eosinophil counts. Model I made no adjustments, Model II made adjustments for age, race, and gender, and Model III made adjustments for all variables with the exception of the analyzed variable. In addition, we employed the trend test and the generalized additive model (GAM) to confirm the validity of the regression analysis results. Next, we performed stratified analyses to determine the association between HDL-C and blood eosinophil counts in specific subgroups. To determine whether individual characteristics influenced the association between HDL-C and blood eosinophil counts, interaction tests were conducted. Eventually, the XGBoost algorithm model’s machine learning was intended to determine the relative importance of various variables on the effect of blood eosinophil counts. R software (Version 4.2.0) and the R package were used to conduct all statistical analyses. A p-value 0.05 was considered statistically significant in our study.
3 Results
3.1 Basic characteristics of the analyzed population
Table 1 presents the weighted basic characteristics of individuals based on blood eosinophil counts quartiles (Q1-Q4) for a total of 2544 asthmatic adults aged 20 to 80 years old who participated in our study (2011–2018 NHANES). In our investigation, the mean age of the investigated population was 47.3 years old, and the majority of the population was non-Hispanic White. We observed that the distribution of age, gender, race, BMI, lipid-lowering drugs use, hypertension history, HDL-C and triglyceride was statistically significant (p value < 0.05) across all quartiles of blood eosinophil counts. Nevertheless, no significant differences (p value > 0.05) were observed across different quartiles of blood eosinophil counts for education level, marital status, poverty to income ratio, smoking status, alcohol intake, antiallergic drugs use, diabetes history, COPD history, LDL-C and total cholesterol.
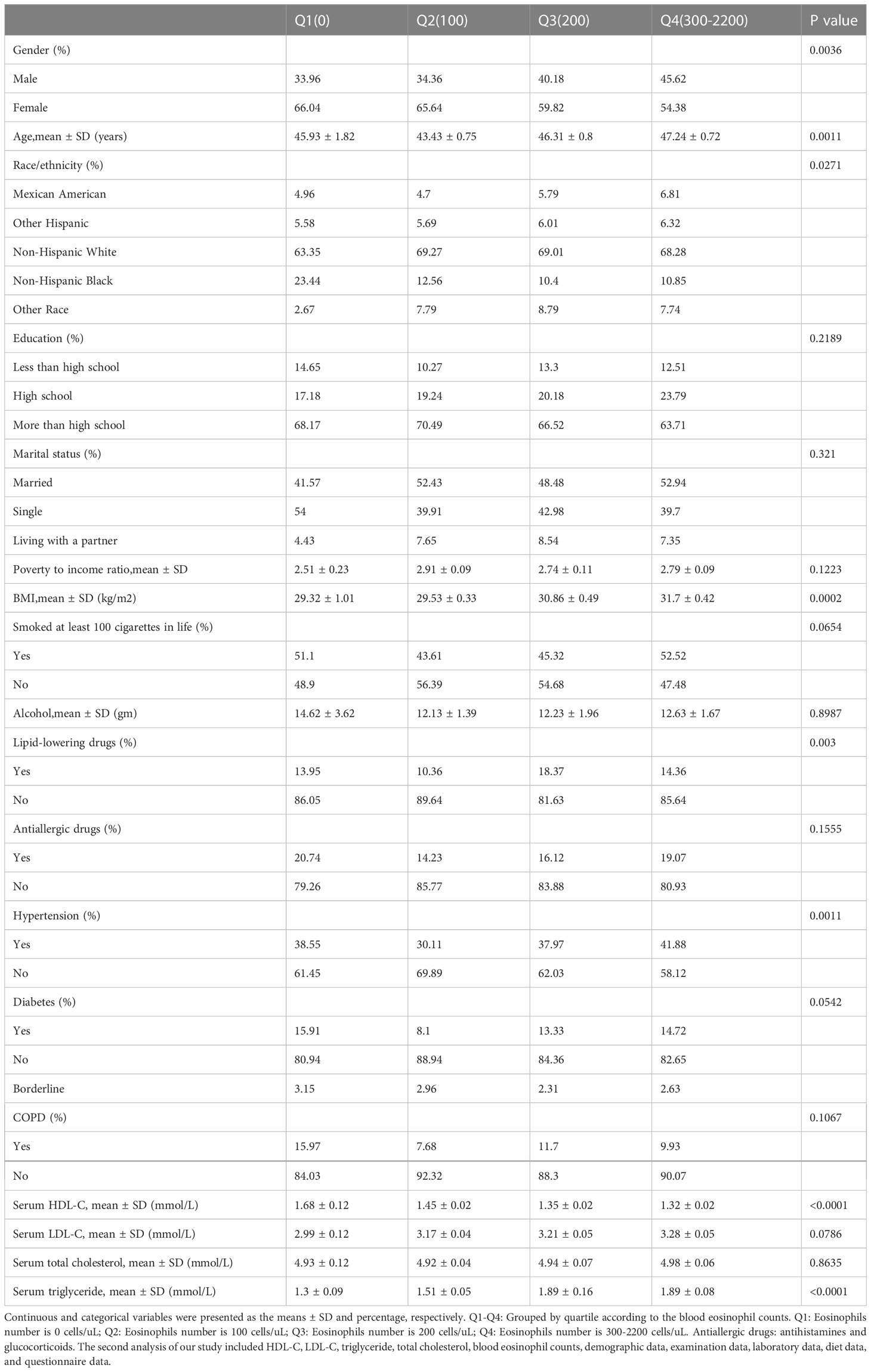
Table 1 By quartiles of blood eosinophil counts, weighted characteristics of study individuals are disaggregated.
3.2 The relationships between serum lipid and blood eosinophil counts
Three weighted univariable and multivariable regression models were used to assess whether the relationships between serum lipid and blood eosinophil counts among American asthmatic adults were statistically significant (Table 2). Based on the three models, we observed that only HDL-C was inversely correlated with blood eosinophil counts, with statistical significances. Nevertheless, no significant differences (p value > 0.05) were observed in relationships between LDL-C, total cholesterol or triglyceride with blood eosinophil counts. In the Model I, which didn’t adjust for any variables, the blood eosinophil counts decreased by 49.07 (-65.90, -32.23)/uL for each additional unit of HDL-C (mmol/L). In the Model II, which adjusted for age, gender and race, the blood eosinophil counts decreased by 45.38 (-63.18, -27.58)/uL for each additional unit of HDL-C (mmol/L). In the Model III, which adjusted for age, gender, race, education level, marital status, poverty income ratio, BMI, smoked status, alcohol intake, lipid-lowering drugs use, antiallergic drugs use, hypertension history, diabetes history, COPD history, LDL-C, total cholesterol and triglyceride, blood eosinophil counts decreased by 45.68 (-68.56, -22.79)/uL for each additional unit of HDL-C (mmol/L). Also, we observed the trend test was statistically significant in the Model I and II, whereas not in the Model III (p for trend <0.05), which indicated HDL-C was linear associated with blood eosinophil counts in the Model I and II, but not in in the Model III (Table 3). Therefore, we further employed the generalized additive model (GAM) and threshold effect model to assess the nonlinear or linear relationship between HDL-C and blood eosinophil counts in the Model III. Likewise, we also observed that serum HDL-C was also linearly and inversely linked with blood eosinophil counts among adults without asthma (p for trend <0.05), who met inclusion and exclusion criteria (Supplementary Figure 1, Supplementary Table 1).
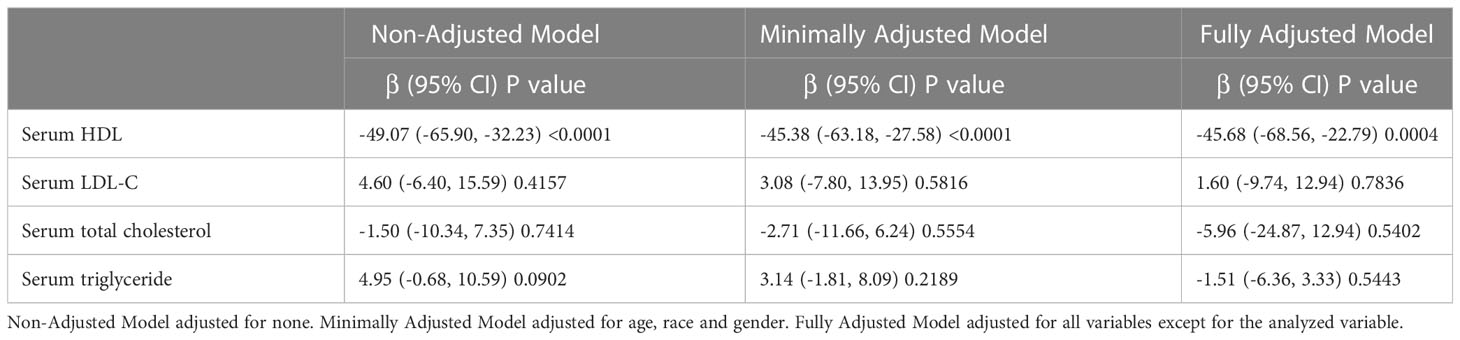
Table 2 The correlation between serum lipid and blood eosinophil counts in three weighted regression models.
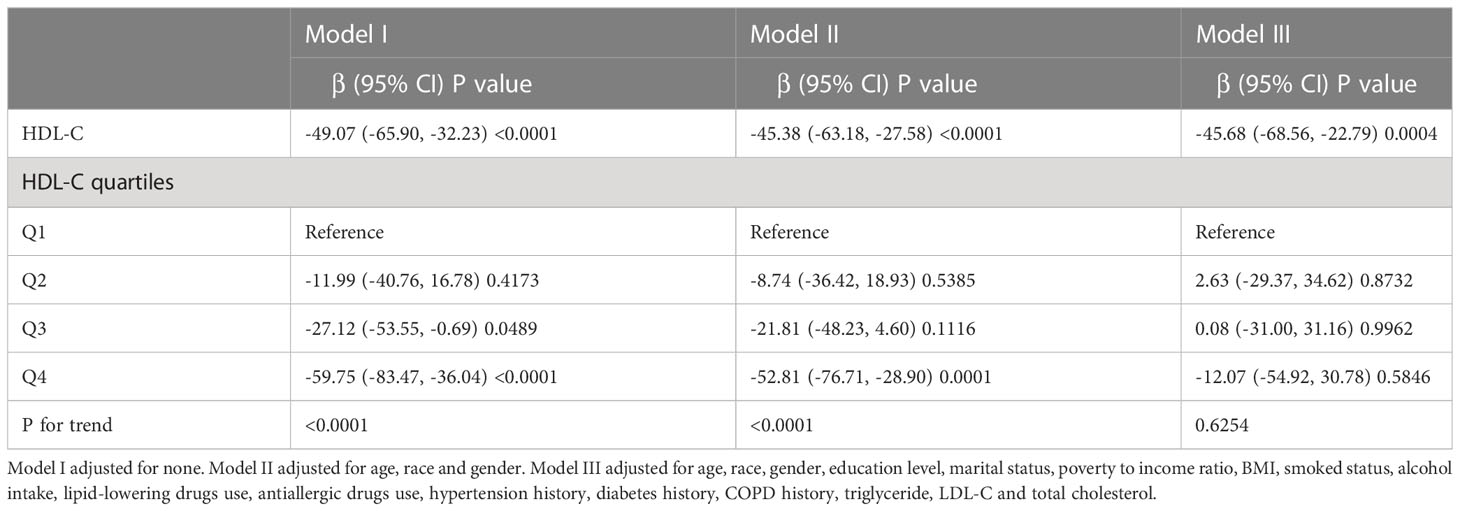
Table 3 Weighted regression models and trend tests elucidate the correlation between HDL-C and blood eosinophil counts.
3.3 Stratified associations between HDL-C and blood eosinophil counts
To verify the stability of multivariable regression analyses results, we further analyzed stratified associations between the serum HDL-C levels and blood eosinophil counts in a specific subgroup by gender, age, race, education level, marital status, poverty to income ratio, BMI, COPD history, lipid-lowering drugs use and antiallergic drugs use (Table 4). According to stratified analysis results, we found an inversely association between HDL-C and blood eosinophil counts in the specific populations with asthma, who were female, age ≥40, Non-Hispanic White people, less or more than high school, single or married status, low or high group of poverty to income ratio, BMI<25 or ≥28, and without lipid-lowering drugs use. Besides, we observed no interactions among all subgroup analyses results (all P values for interaction > 0.05). In addition, we also conducted the stratified analyses of associations between the serum HDL-C levels and blood eosinophil counts in adults with Asthma-COPD Overlap Syndrome (ACOS). We found this inverse association in the specific populations with ACOS, who were female, age ≥60, Non-Hispanic White people, more than high school, single status, low group of poverty to income ratio, and without lipid-lowering drugs use (Supplementary Table 2).
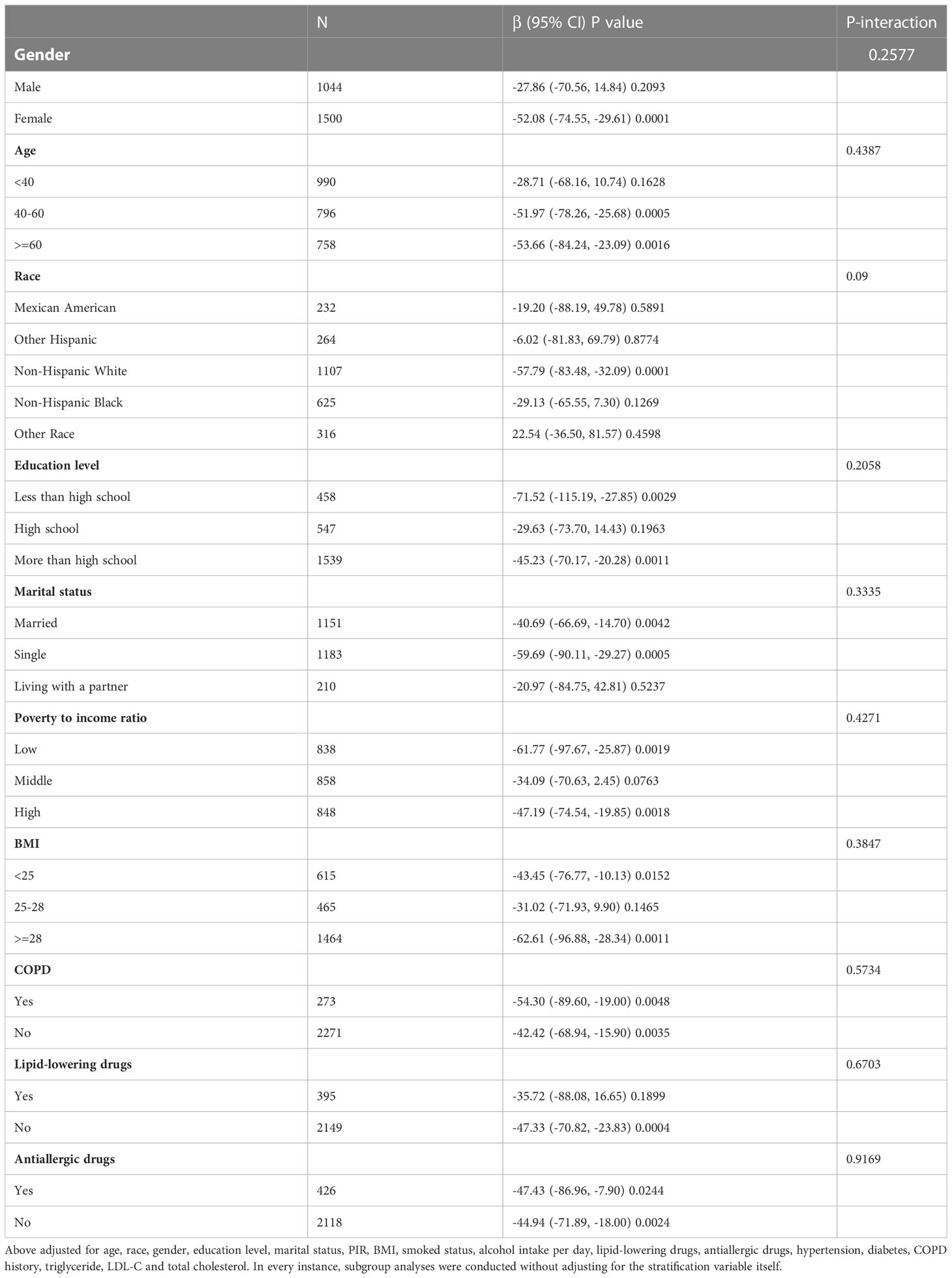
Table 4 Stratified correlation of serum HDL-C on blood eosinophil counts in the predetermined and exploratory subgroups.
3.4 Evaluating chosen variables’ relative significance by XGBoost algorithm model
During the model development and validation phase, we identified the relative importance of chosen variables correlated with blood eosinophil counts using machine learning. Variables included age, poverty to income ratio (PIR), BMI, HDL-C, LDL-C, total cholesterol and triglyceride. According to the results of XGBoost algorithm model, we observed that LDL-C, age, BMI, triglyceride, and HDL-C were the five most critical variables in the blood eosinophil counts (Figure 2). In the end, we selected HDL-C as the relatively critical relative variable to further construct the smooth curve in order to validate the validity of the multivariable regression analyses results.
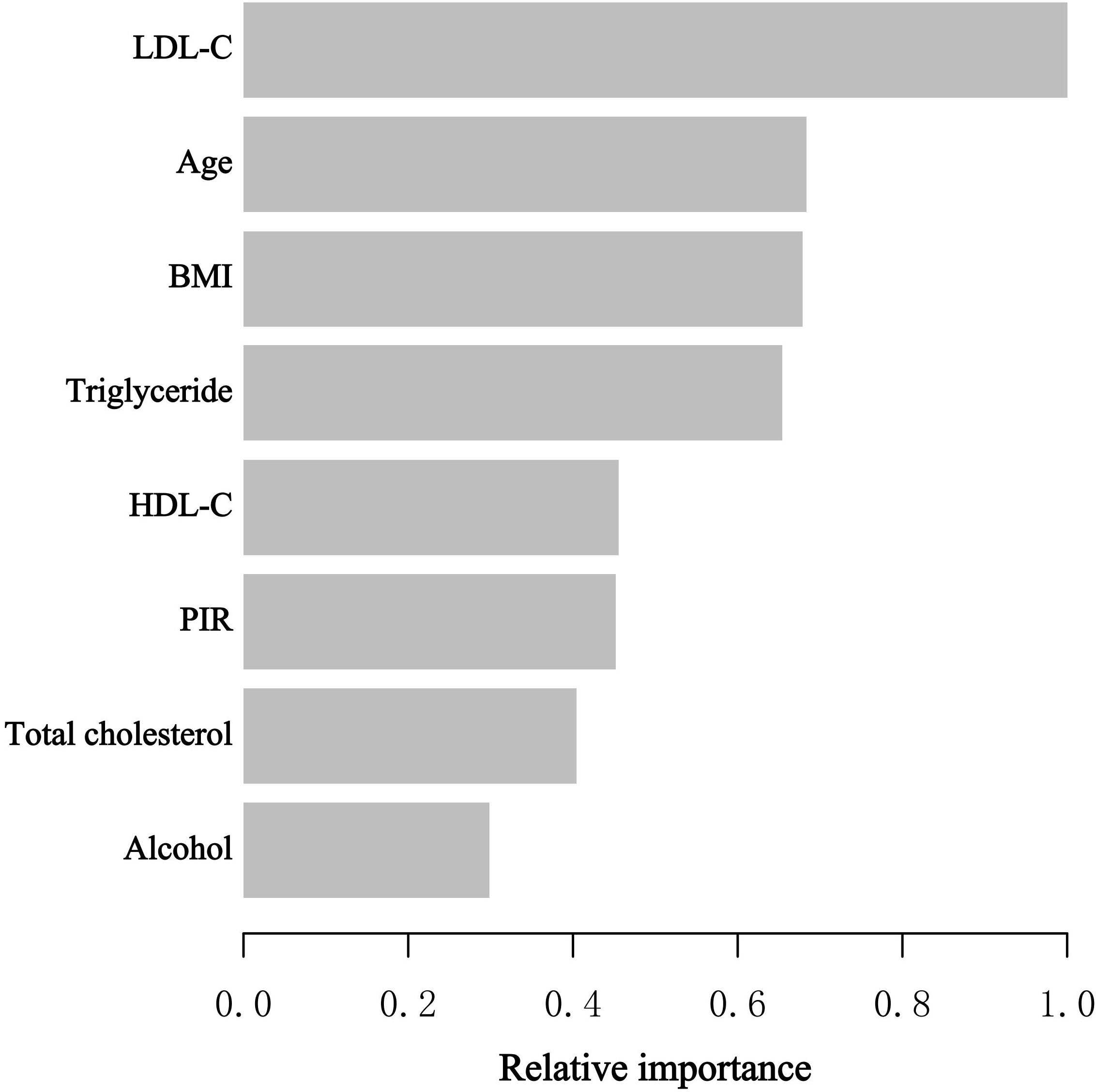
Figure 2 XGBoost algorithm model revealed the relative importance of selected variables on blood eosinophil counts as well as the variable importance score for each variable. The X-axis represented the importance score, the relative number of a variable used to distribute the data, and the Y-axis indicated the selected variables.
3.5 Verifying the linear relationship between HDL-C and blood eosinophil counts
The generalized additive model (GAM) is highly attuned to recognizing linear or nonlinear relationships. To verify the validity of multivariable regression analysis results, we explored the nonlinear or linear relationship between HDL-C levels and blood eosinophil counts using the GAM. Using GAM, we constructed a smooth fit curve to represent the relationship based on Model III (Figure 3). We observed the linear and negative relationship between serum HDL-C with blood eosinophil counts in asthmatic adults after adjusting age, race, gender, education level, marital status, poverty to income ratio, BMI, smoked status, alcohol intake, lipid-lowering drugs use, antiallergic drugs use, hypertension history, diabetes history, COPD history, triglyceride, LDL-C and total cholesterol. Similarly, we also observed the linear and negative relationship in adults with ACOS after adjusting age, race, gender, education level, marital status, poverty to income ratio, BMI, smoked status, alcohol intake, lipid-lowering drugs use, antiallergic drugs use, hypertension history, diabetes history, triglyceride, LDL-C and total cholesterol (Supplementary Figure 2). What’s more, we utilized the threshold effect model to confirm the linear or nonlinear relationship between HDL-C and blood eosinophil counts in asthmatic adults (Table 5). We found that the inflection point was not statistically significant (log-likelihood ratio > 0.05) and that there were no statistically significant differences between the one-line model and the segmented regression model. Therefore, the one-line model was more appropriate to explain the relationship between HDL-C and blood eosinophil counts. All of the aforementioned findings revealed an inverse and linear relationship between HDL-C and blood eosinophil counts among asthmatic adults in the United States.
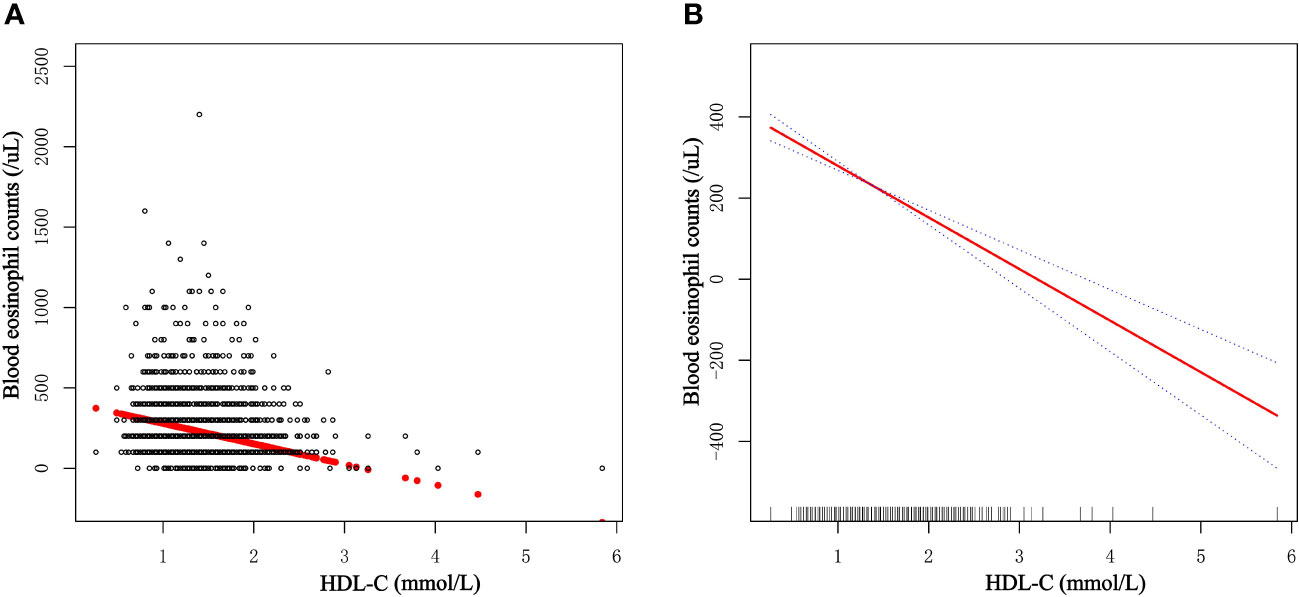
Figure 3 Dose-response relationship of serum HDL-C levels with blood eosinophil counts among asthmatic adults. (A) Each dot represents an individual sample. (B) The solid red line indicates the smooth fitting curve between serum HDL-C and blood eosinophil counts, whereas the dotted blue line represents 95% confidence intervals of the fitting.
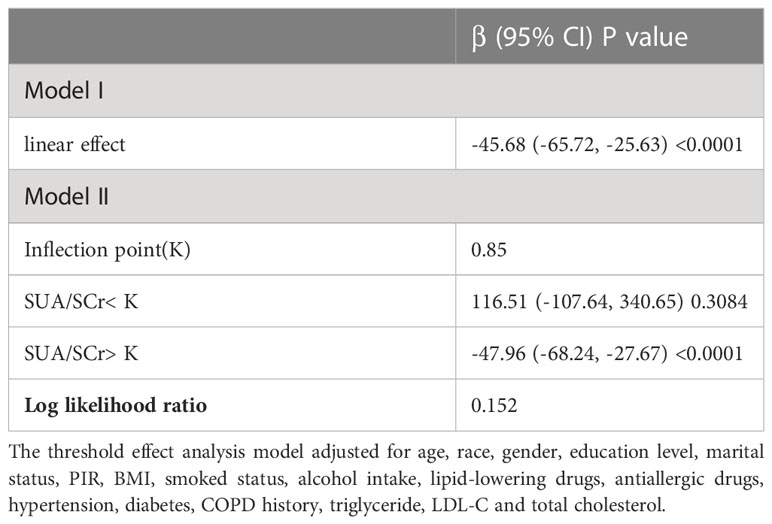
Table 5 Threshold effect analysis of between HDL-C on blood eosinophil counts in asthmatic adults using the two-piecewise linear regression model.
4 Discussion
According to our knowledge, this study is one of the largest cross-sectional studies to explore the relationship between serum lipid levels and blood eosinophil counts in asthmatic individuals to date. The study population consisted of 2,544 National Health and Nutrition Examination Survey participants (2011–2018). In this investigation, HDL-C levels were adversely linked with blood eosinophil counts both before and after adjustment for sociodemographic, laboratory, personal life history, comorbidities, and medication use data, but serum LDL-C, total cholesterol or triglyceride was not correlated with blood eosinophil counts. LDL-C, age, BMI, triglyceride, and HDL-C were determined to be the five most important factors in blood eosinophil counts by machine learning of the XGBoost model. After controlling for confounders, the blood eosinophil counts fell by 45.68/uL for each increased unit of HDL-C (mmol/L), demonstrating a statistically significant difference. As a relatively essential variable, HDL-C was incorporated into generalized additive model and two-piecewise linear regression model to validate the robustness of the outcome.
Previous studies had shown the association between eosinophilia and dyslipidemia in general populations (12–14). A cross-sectional analysis included 417132 participants and demonstrated that a higher eosinophil count was associated with lower HDL cholesterol and apolipoproteinA-I (ApoA-I) concentration, as well as that higher triglyceride levels were associated with higher total leukocyte, basophil, eosinophil, monocyte, and neutrophil counts (12). Moreover, there are increasing studies finding an association between asthma and serum lipids (10, 15, 16). In 2015, Barochia et al. investigated the differences in lipid profiles between asthmatics and normal, healthy individuals. According to the findings of that study, atopic asthmatic patients had significantly higher HDL-C levels than nonatopic nonasthmatic patients (15). In 2017, Barochia and colleagues further indicated a positive association of triglycerides and a negative association of HDL-C with eosinophil count in atopic asthmatics (17), which was partially consistent with our results. However, their study solely focused on the connection and had a limited sample size. Our research indicates that HDL-C particles are inversely related to blood eosinophil counts in asthma. The durability of the results among asthmatics after correcting for many potential confounders, including the use of lipid-lowering medicines or antiallergic drugs, suggests that the relationship may be biologically significant (including antihistamines and glucocorticoids). In addition, we employed GAM to confirm the linear and negative association between HDL-C levels and blood eosinophil counts in asthmatic adults.
ACOS is a condition characterized by overlapping clinical features of both diseases. A meta-analysis of 19 studies indicated the prevalence of ACOS across studies to be even higher with an average of 26.5% (18). Previous studies had showed that airway and systemic eosinophilia are important treatable traits in both severe asthma and COPD (19). Luo et al. found that eosinophil levels were highly expressed in patients with asthma, patients with COPD and patients with ACOS (20). Moreover, Fricker’s study showed that molecular markers of type 2 airway inflammation do not differ between eosinophilic asthma and eosinophilic COPD (21). Therefore, we confer that blood eosinophil counts could also reflect the severity of ACOS which was consistent with asthma. There have been few reports regarding the relationship between serum lipid levels and blood eosinophil counts in ACOS. Gu et al. developed a model for predicting the severity of COPD. The study found that both eosinophil count and HDL were the factors that could distinguish between mild/moderate and severe (22). Our study was also the first study to explore the association between serum lipid levels and blood eosinophil counts in patients with ACOS. And we found the linear and negative relationship between HDL-C and blood eosinophil counts in adults with ACOS.
The findings that HDL-C particles are negatively associated with blood eosinophil counts in asthma, which are similar to the associations of HDL-C with lower cardiovascular risk (23), HDL not only mediates reverse cholesterol transport but also has anti-oxidant, anti-thrombotic, and anti-inflammatory functions (24). Endothelial lipase expressed in the lung could hydrolyze phospholipids, promote the catabolism of HDL particles, and regulate eosinophilic inflammation. In addition, the researchers found that endothelial lipase knockout mice had higher serum HDL levels after stimulation by allergens, and eosinophilic lung inflammation was alleviated (9). It has been proven that HDL and ApoA-I can inhibit antigen presentation-mediated T cell activation by disrupting lipid rafts in antigen-presenting cells (25). ApoA-I, the main apolipoprotein in HDL-C, has known functions including anti-inflammation and cholesterol efflux (26). Yao’s experiment discovered that apolipoprotein E or apolipoprotein A-I mimetic peptides could attenuate experimental asthma in the murine model, possibly by reducing pulmonary eosinophil infiltration (27, 28). These suggest that the anti-inflammatory properties of HDL and ApoA-I might be negatively correlated with blood eosinophil counts in asthmatics. And in our study, no significant differences were observed in relationships between LDL-C, total cholesterol, or triglyceride with blood eosinophil counts. This suggests that HDL-C might be related to the immune status of asthmatic adults.
Our study has several advantages over earlier research. First, our research provides a nationally representative, somewhat large sample of asthmatic adults with information on a number of potential confounding variables. Secondly, because confounders may influence the results, we use stratified analysis to identify the potentially protected group with higher HDL-C values. Then, we employ the machine learning of the XGBoost algorithm model to determine the relative importance of selected variables correlated with blood eosinophil counts. Using the generalized additive model, we observed the linear association between serum HDL levels and blood eosinophil counts. Although the precise relationship between HDL-C and eosinophil count remains unknown, the linkage has the potential to inspire the development of innovative asthma treatments. To elucidate the relationship between eosinophil count and serum lipid and to identify the underlying mechanism, additional research is required.
Nonetheless, we accept that our study has some limitations. Although the scope of our study is nationwide, the majority of the data is derived from the American population. As a result of unequal national development, dietary models may vary between countries. Due to the limitations of the cross-sectional study design, we cannot establish a causal association between serum lipid levels and blood eosinophil counts. Recent research has revealed, however, that free fatty acids directly impact myelopoiesis in the bone marrow via their release from bone marrow adipocytes (29), which then stimulates an increase in peripheral eosinophil counts. Next, we included asthmatic individuals based on questionnaire data rather than the lung function test. And the drugs included in our study that affected blood eosinophils were mainly cortisol drugs and other anti-allergy drugs, but no biologics. Therefore, additional prospective studies will be required in the future to shed light on the potential involvement of serum lipid in the control, progression, or treatment of asthma and to identify potential mechanisms of action.
5 Conclusion
This nationally representative study demonstrated that serum HDL-C was independently and inversely connected with blood eosinophil counts among American asthmatic adults, suggesting that serum HDL-C might be associated with the immunological status of asthmatic adults. However, there was no correlation between serum LDL-C, total cholesterol, or triglyceride levels and blood eosinophil counts. We hope that more individuals will recognize the significance lipids play in asthma, in the future.
Data availability statement
Publicly available datasets were analyzed in this study. This data can be found here: http://www.cdc.gov/nchs/nhanes/.
Ethics statement
The studies involving human participants were reviewed and approved by NCHS Research Ethics Review Board. The patients/participants provided their written informed consent to participate in this study.
Author contributions
JW carried out the study design, data extraction and statistical analysis, drafted and revised the manuscript. MG conducted data extraction, statistical analysis, and revision of the paper. RZ carried out statistical analysis, drafted and revised the manuscript. CH conducted data extraction, drafted and revised the manuscript. SG participated in the study design, management of study and revision of the paper. All authors contributed to the article and approved the submitted version.
Funding
Chongqing Talents, Teachers and Masters (SG).
Acknowledgments
The protocol for the National Health and Nutrition Examination Survey was approved by the NCHS Research Ethics Review Board.
Conflict of interest
The authors declare that the research was conducted in the absence of any commercial or financial relationships that could be construed as a potential conflict of interest.
Publisher’s note
All claims expressed in this article are solely those of the authors and do not necessarily represent those of their affiliated organizations, or those of the publisher, the editors and the reviewers. Any product that may be evaluated in this article, or claim that may be made by its manufacturer, is not guaranteed or endorsed by the publisher.
Supplementary material
The Supplementary Material for this article can be found online at: https://www.frontiersin.org/articles/10.3389/fimmu.2023.1166406/full#supplementary-material
References
1. Wenzel S. Severe asthma: from characteristics to phenotypes to endotypes. Clin Exp Allergy J Br Soc Allergy Clin Immunol (2012) 42(5):650–8. doi: 10.1111/j.1365-2222.2011.03929.x
2. Kuruvilla ME, Lee FE, Lee GB. Understanding asthma phenotypes, endotypes, and mechanisms of disease. Clin Rev Allergy Immunol (2019) 56(2):219–33. doi: 10.1007/s12016-018-8712-1
3. Enilari O, Sinha S. The global impact of asthma in adult populations. Ann Glob Health (2019) 85(1):2. doi: 10.5334/aogh.241
4. Heaney LG, Perez de Llano L, Al-Ahmad M, Backer V, Busby J, Canonica GW, et al. Eosinophilic and noneosinophilic asthma: An expert consensus framework to characterize phenotypes in a global real-life severe asthma cohort. Chest (2021) 160(3):814–30. doi: 10.1016/j.chest.2021.04.013
5. Van Hulst G, Bureau F, Desmet CJ. Eosinophils as drivers of severe eosinophilic asthma: Endotypes or plasticity? Int J Mol Sci (2021) 22(18):10150. doi: 10.3390/ijms221810150
6. Kaur R, Chupp G. Phenotypes and endotypes of adult asthma: Moving toward precision medicine. J Allergy Clin Immunol (2019) 144(1):1–12. doi: 10.1016/j.jaci.2019.05.031
7. Szefler SJ, Wenzel S, Brown R, Erzurum SC, Fahy JV, Hamilton RG, et al. Asthma outcomes: Biomarkers. J Allergy Clin Immunol (2012) 129(3 Suppl):S9–23. doi: 10.1016/j.jaci.2011.12.979
8. Otera H, Ishida T, Nishiuma T, Kobayashi K, Kotani Y, Yasuda T, et al. Targeted inactivation of endothelial lipase attenuates lung allergic inflammation through raising plasma HDL level and inhibiting eosinophil infiltration. Am J Physiol Lung Cell Mol Physiol (2009) 296(4):L594–602. doi: 10.1152/ajplung.90530.2008
9. Hara T, Ishida T, Kojima Y, Tanaka H, Yasuda T, Shinohara M, et al. Targeted deletion of endothelial lipase increases HDL particles with anti-inflammatory properties both in vitro and in vivo. J Lipid Res (2011) 52(1):57–67. doi: 10.1194/jlr.M008417
10. Fenger RV, Gonzalez-Quintela A, Linneberg A, Husemoen LL, Thuesen BH, Aadahl M, et al. The relationship of serum triglycerides, serum HDL, and obesity to the risk of wheezing in 85,555 adults. Respir Med (2013) 107(6):816–24. doi: 10.1016/j.rmed.2013.02.001
11. Ko SH, Jeong J, Baeg MK, Han KD, Kim HS, Yoon JS, et al. Lipid profiles in adolescents with and without asthma: Korea national health and nutrition examination survey data. Lipids Health Dis (2018) 17(1):158. doi: 10.1186/s12944-018-0807-4
12. Tucker B, Sawant S, McDonald H, Rye KA, Patel S, Ong KL, et al. The association of serum lipid and lipoprotein levels with total and differential leukocyte counts: Results of a cross-sectional and longitudinal analysis of the UK biobank. Atherosclerosis (2021) 319:1–9. doi: 10.1016/j.atherosclerosis.2020.12.016
13. Kim DJ, Noh JH, Lee BW, Choi YH, Chung JH, Min YK, et al. The associations of total and differential white blood cell counts with obesity, hypertension, dyslipidemia and glucose intolerance in a Korean population. J Korean Med Sci (2008) 23(2):193–8. doi: 10.3346/jkms.2008.23.2.193
14. Amini M, Bashirova D, Prins BP, Corpeleijn E, Bruinenberg M, Franke L, et al. Eosinophil count is a common factor for complex metabolic and pulmonary traits and diseases: The LifeLines cohort study. PloS One (2016) 11(12):e0168480. doi: 10.1371/journal.pone.0168480
15. Barochia AV, Kaler M, Cuento RA, Gordon EM, Weir NA, Sampson M, et al. Serum apolipoprotein a-I and large high-density lipoprotein particles are positively correlated with FEV1 in atopic asthma. Am J Respir Crit Care Med (2015) 191(9):990–1000. doi: 10.1164/rccm.201411-1990OC
16. Chanachon PN, Jotikasthira W, Kiewngam P, Sawatchai A, Kanchongkittiphon W, Manuyakorn W. Association of dyslipidemia and respiratory resistance assessed by the forced oscillation technique in asthmatic children. Lung (2022) 200(1):73–82. doi: 10.1007/s00408-021-00502-1
17. Barochia AV, Gordon EM, Kaler M, Cuento RA, Theard P, Figueroa DM, et al. High density lipoproteins and type 2 inflammatory biomarkers are negatively correlated in atopic asthmatics. J Lipid Res (2017) 58(8):1713–21. doi: 10.1194/jlr.P077776
18. Hosseini M, Almasi-Hashiani A, Sepidarkish M, Maroufizadeh S. Global prevalence of asthma-COPD overlap (ACO) in the general population: A systematic review and meta-analysis. Respir Res (2019) 20(1):229. doi: 10.1186/s12931-019-1198-4
19. Garudadri S, Woodruff PG. Targeting chronic obstructive pulmonary disease phenotypes, endotypes, and biomarkers. Ann Am Thorac Soc (2018) 15(Suppl 4):S234–s8. doi: 10.1513/AnnalsATS.201808-533MG
20. Luo JY, Chen HA, Feng YY, Chen YP, Lei XC, Guo SL, et al. Blood eosinophil endotypes across asthma and chronic obstructive pulmonary disease (COPD). Can Respir J (2022) 2022:9656278. doi: 10.1155/2022/9656278
21. Fricker M, McDonald VM, Winter NA, Baines KJ, Wark PAB, Simpson JL, et al. Molecular markers of type 2 airway inflammation are similar between eosinophilic severe asthma and eosinophilic chronic obstructive pulmonary disease. Allergy (2021) 76(7):2079–89. doi: 10.1111/all.14741
22. Gu YF, Chen L, Qiu R, Wang SH, Chen P. Development of a model for predicting the severity of chronic obstructive pulmonary disease. Front Med (Lausanne) (2022) 9:1073536. doi: 10.3389/fmed.2022.1073536
23. Nicholls SJ, Nelson AJ. HDL and cardiovascular disease. Pathology (2019) 51(2):142–7. doi: 10.1016/j.pathol.2018.10.017
24. Barter PJ, Nicholls S, Rye KA, Anantharamaiah GM, Navab M, Fogelman AM. Antiinflammatory properties of HDL. Circ Res (2004) 95(8):764–72. doi: 10.1161/01.RES.0000146094.59640.13
25. Wang SH, Yuan SG, Peng DQ, Zhao SP. HDL and ApoA-I inhibit antigen presentation-mediated T cell activation by disrupting lipid rafts in antigen presenting cells. Atherosclerosis (2012) 225(1):105–14. doi: 10.1016/j.atherosclerosis.2012.07.029
26. Rye KA. High density lipoprotein structure, function, and metabolism: a new thematic series. J Lipid Res (2013) 54(8):2031–3. doi: 10.1194/jlr.E041350
27. Yao X, Vitek MP, Remaley AT, Levine SJ. Apolipoprotein mimetic peptides: A new approach for the treatment of asthma. Front Pharmacol (2012) 3:37. doi: 10.3389/fphar.2012.00037
28. Yao X, Gordon EM, Figueroa DM, Barochia AV, Levine SJ. Emerging roles of apolipoprotein e and apolipoprotein a-I in the pathogenesis and treatment of lung disease. Am J Respir Cell Mol Biol (2016) 55(2):159–69. doi: 10.1165/rcmb.2016-0060TR
Keywords: serum lipid, high density lipoprotein-cholesterol (HDL-C), eosinophil, asthma, National Health and Nutrition Examination Survey (NHANES), machine learning
Citation: Wen J, Zhuang R, He C, Giri M and Guo S (2023) High density lipoprotein-cholesterol is inversely associated with blood eosinophil counts among asthmatic adults in the USA: NHANES 2011-2018. Front. Immunol. 14:1166406. doi: 10.3389/fimmu.2023.1166406
Received: 15 February 2023; Accepted: 11 April 2023;
Published: 24 April 2023.
Edited by:
Sara Manti, University of Messina, ItalyReviewed by:
Qian Jiang, Hunan Agricultural University, ChinaStewart J. Levine, National Heart, Lung, and Blood Institute (NIH), United States
Copyright © 2023 Wen, Zhuang, He, Giri and Guo. This is an open-access article distributed under the terms of the Creative Commons Attribution License (CC BY). The use, distribution or reproduction in other forums is permitted, provided the original author(s) and the copyright owner(s) are credited and that the original publication in this journal is cited, in accordance with accepted academic practice. No use, distribution or reproduction is permitted which does not comply with these terms.
*Correspondence: Shuliang Guo, Z3Vvc2w5OTlAc2luYS5jb20=
†These authors have contributed equally to this work