- 1Liver Transplantation Center, West China Hospital, Sichuan University, Chengdu, China
- 2Department of Liver Surgery, West China Hospital, Sichuan University, Chengdu, China
- 3Department of Hepatopancreatobiliary Minimal Invasive Surgery, Chengdu ShangJin NanFu Hospital, Chengdu, China
- 4Department of Hepatobiliary Pancreatic Tumor Center, Chongqing Key Laboratory of Translational Research for Cancer Metastasis and Individualized Treatment, Chongqing University Cancer Hospital, Chongqing, China
- 5Department of Hepatobiliary Surgery, The People's Hospital of Leshan, Leshan, China
- 6Department of Anesthesiology, The Second Affiliated Hospital, Zhejiang University School of Medicine, Hangzhou, China
- 7Department of Endocrine and Breast Surgery, The First Affiliated Hospital of Chongqing Medical University, Chongqing, China
Background: Immune function, nutrition status, and inflammation influence tumor initiation and progression. This was a retrospective multicenter cohort study that investigated the prognostic value and clinical relevance of immune-, inflammatory-, and nutritional-related biomarkers to develop a novel prognostic immune–inflammatory–nutritional score (PIIN score) for patients with intrahepatic cholangiocarcinoma (ICC).
Methods: The clinical data of 571 patients (406 in the training set and 165 in the validation set) were collected from four large hepato-pancreatico-biliary centers of patients with ICC who underwent surgical resection between January 2011 and September 2017. Twelve blood biomarkers were collected to develop the PIIN score using the LASSO Cox regression model. The predictive value was further assessed using validation datasets. Afterward, nomograms combining the PIIN score and other clinicopathological parameters were developed and validated based on the calibration curve, time-dependent AUC curves, and decision curve analysis (DCA). The primary outcomes evaluated were overall survival (OS) and recurrence-free survival (RFS) from the day of primary resection of ICC.
Results: Based on the albumin–bilirubin (ALBI) grade, neutrophil- to- lymphocyte ratio (NLR), prognostic nutritional index (PNI), and systemic immune- inflammation index (SII) biomarkers, the PIIN score that classified patients into high-risk and low-risk groups could be calculated. Patients with high-risk scores had shorter OS (training set, p < 0.001; validation set, p = 0.003) and RFS (training set, p < 0.001; validation set, p = 0.002) than patients with low-risk scores. The high PIIN score was also associated with larger tumors (≥5 cm), lymph node metastasis (N1 stage), multiple tumors, and high tumor grade or TNM (tumor (T), nodes (N), and metastases (M)) stage. Furthermore, the high PIIN score was a significant independent prognostic factor of OS and RFS in both the training (p < 0.001) and validation (p = 0.003) cohorts, respectively. A PIIN-nomogram for individualized prognostic prediction was constructed by integrating the PIIN score with the clinicopathological variables that yielded better predictive performance than the TNM stage.
Conclusion: The PIIN score, a novel immune–inflammatory–nutritional-related prognostic biomarker, predicts the prognosis in patients with resected ICC and can be a reliable tool for ICC prognosis prediction after surgery. Our study findings provide novel insights into the role of cancer-related immune disorders, inflammation, and malnutrition.
Introduction
Intrahepatic cholangiocarcinoma (ICC) is the second most prevalent primary solid tumor of the liver after hepatocellular carcinoma, with an increasing incidence worldwide over the past few decades (1, 2). Currently, the efficacy of the available treatment options for ICC, including chemotherapy, targeted therapy, and immunotherapy, is limited; surgical resection remains the only curative method (3). Nevertheless, the postoperative recurrence rate is up to 80%, and the 5-year overall survival (OS) of ICC patients is less than 20% (4, 5). Several ordinary clinicopathologic characteristics, such as tumor size, histological grade (6), multifocality (7), lymph node metastasis (LNM) (8, 9), microvascular invasion (MVI) (10), and carbohydrate antigen 19-9 (CA19-9) (11), are used for prognosis evaluation and risk stratification in patients with ICC. However, the value of these conventional factors in predicting ICC prognosis is limited. Novel accurate prognostic indicators in patients with ICC are urgently needed. Recent studies have shown that immune function, nutrition status, and inflammation participate in tumor initiation and progression (12–17). Several immune-, inflammatory-, and nutritional-related biomarkers based on preoperative blood indexes are valuable prognostic markers in many cancer types (18–21). Previous studies have demonstrated that a low controlling nutritional status (CONUT) score and prognostic nutritional index (PNI) score are associated with favorable prognosis, and an elevated systemic immune inflammation index (SII) level worsens OS in patients with ICC (22–24). However, a single blood marker cannot reflect the landscape of a patient’s immune function, nutrition status, and inflammation. It remains unclear whether this combination may help to overcome this limitation. This study analyzed the prognostic role and clinical relevance of immune-, inflammatory-, and nutritional-related factors to develop a novel predictive model termed the prognostic immune–inflammatory–nutritional score (PIIN score). The PIIN score combines immune-, inflammatory-, and nutritional-related biomarkers to evaluate the outcomes of ICC resection. Furthermore, PIIN-nomograms based on the PIIN score and other clinicopathological features were constructed and validated for individualized predictions of the survival probability of the patients.
Materials and methods
Study cohort
Patients with ICC undergoing curative resection between January 2011 and September 2017 at West China Hospital, Chongqing University Cancer Hospital, the People’s Hospital of Leshan, and Chengdu Shang Jin Nan Fu Hospital were screened. The inclusion criteria were as follows: (1) patients with histologically proven ICC; (2) with radical resection; (3) with no extrahepatic metastasis; (4) with complete baseline laboratory test information. The exclusion criteria were as follows: (1) patients who received other treatments before surgery (transarterial chemoembolization, radiofrequency ablation, or systemic therapy); (2) with other malignancy histories; (3) with missing clinical data or follow-up information. The included patients were randomly divided into training (n = 406) and validation sets (n = 165) at a ratio of 7:3.
Data collection and definition of variables
The demographic and tumor-related characteristics comprised the following: age, sex, tumor diameter, tumor number, hepatolithiasis, perineural invasion, biliary invasion, histological grade, MVI, lymph node status, and the 8th edition of the American Joint Committee on Cancer (AJCC)-TNM classification. Preoperative hematological parameters were evaluated; these included fasting blood glucose (FBG); lymphocyte, neutrophil, and platelet (PLT) counts; fibrinogen (FIB); alanine aminotransferase (ALT); aspartate aminotransferase (AST); bilirubin; albumin; globulin; gamma-glutamyl transpeptidase (GGT); cholesterol; alkaline phosphatase (ALP); and carbohydrate antigen 19-9 (CA19-9) levels. This study focused on 12 immune-, inflammatory-, and nutritional-related biomarkers: ALT, AST, albumin–alkaline phosphatase ratio (AAPR), albumin–globulin ratio (AGR), FIB, albumin–bilirubin (ALBI) grade, GGT–albumin ratio (GAR), neutrophil- to- lymphocyte ratio (NLR), platelet- to- lymphocyte ratio (PLR), prognostic nutritional index (PNI), systemic immune inflammation index (SII), and controlling nutritional status (CONUT). The biomarkers were calculated as follows: AAPR = albumin (g/L)/ALP (IU/L), AGR= albumin (g/L)/globulin (g/L), ALBI= log10bilirubin (mol/L) × 0.66–albumin (g/L) ×0.085, GAR= FBG (mmol/L)/lymphocyte, NLR = neutrophil/lymphocyte, PLR = platelet/lymphocyte, PNI = albumin (g/L) + 5 × lymphocyte, and SII = platelet × neutrophil/lymphocyte. The CONUT score was calculated based on the albumin concentration, total lymphocyte count, and total cholesterol concentration (Supplementary Table 1). The maximally selected rank determined the appropriate cut-off values of the continuous parameters. The ALBI grade was classified according to cut-off value ≤-2.60 (ALBI grade 1), >-2.60 to ≤-1.39 (ALBI grade 2), and ≥-1.39 (ALBI grade 3), as previously described. The patients were divided into the low and high CONUT score groups based on the median score. Overall survival (OS) was defined as the interval from the date of curative surgery to death and the last follow-up. Recurrence-free survival (RFS) was defined as the time between curative surgery and disease recurrence and the last follow-up.
PIIN score construction
Briefly, univariate Cox regression analysis was performed to screen prognostic immune-, inflammatory-, and nutritional-related biomarkers. Statistical significance was set at P< 0.05 and maintained for further analysis. The prognostic significance of these prognostic-related biomarkers was evaluated using the least absolute shrinkage and selection operator (LASSO) Cox regression analysis. Finally, the prognostic immune–inflammatory–nutritional score (PIIN score) was calculated based on variables without zero coefficients.
Prognostic value of PIIN score
To investigate whether the PIIN score is associated with adverse clinicopathological characteristics, the PIIN score between the two subgroups of different clinicopathological characteristics was compared using the Wilcoxon test. The predictive value of the PIIN score was evaluated using receiver operating characteristic (ROC) curves for the 1-, 3-, and 5- year OS of the ICC patients in the training and validation sets. The median PIIN score, as a cut-off value, divided patients into high-risk and low-risk groups. Univariate and multivariate Cox regression analyses were performed to identify prognostic factors independently related to ICC prognosis.
Construction and validation of nomograms
The prognostic nomograms for the survival outcomes (OS and RFS) in the training set were constructed based on multivariate Cox regression analyses by backward stepwise selection with the smallest Akaike information criterion (AIC) value. Time-dependent AUC and calibration curves were used to validate the predictive performance of the nomogram in the training and validation sets. The clinical application values between the nomogram and the AJCC-TNM staging system were compared using decision curve analysis (DCA).
Statistical analysis
A t-test was used to compare continuous variables between the two groups. The chi-square test was used to analyze the differences between the groups of categorical data. The Kaplan–Meier (K-M) curves were plotted to compare differences in OS and RFS using the log-rank test. Statistical analysis was performed using R version 4.1.3. P < 0.05 was considered statistically significant.
Results
Baseline characteristics and PIIN score
A total of 571 patients were included in this study, of whom 49.6% were male and 50.4% female. Their median age was 59 years (range 50–65 years), and the median follow-up time was 30 months (range 3–126 months). The general population’s 1-, 3-, and 5-year OS were 74.1%, 37.3%, and 21.8%, respectively. The baseline demographic and clinical characteristics of the training (n = 406) and validation cohorts (n = 165) are presented in Table 1. The distribution of these baseline variables in the two cohorts was well-balanced (p>0.05). The Kaplan–Meier survival analysis revealed low ALT, AST, ALBI grades, low FIB, GAR, NLR, PLR, SII, and CONUT scores, and high AAPR, AGR, and PNI, which were significantly associated with improved OS (Figure 1). Similarly, the Kaplan–Meier analysis for RFS was consistent (P< 0.05) (Figure 2). The correlations of the 12 immune-, inflammatory-, and nutritional-related biomarkers are shown in Figure 3A. Univariate Cox regression analysis showed that these biomarkers were associated with OS (p< 0.05, Figure 3B). To determine the independent prognostic biomarkers, all the variables mentioned above were included in the LASSO Cox regression analysis. The LASSO Cox regression analysis revealed that only four biomarkers, including SII, NLR, PNI, and ALBI, with non-zero coefficients were found to be associated with ICC prognosis after surgery (Figure 3C). The PIIN score was constructed using the formula: Riskscore=NLR*0.876+SII*0.0174+FIB*14.355+ALBI*2.209- PNI*0.386. First, the relationship between the PIIN score and clinicopathological characteristics was assessed. The patients with larger tumors (≥5 cm), lymph node metastasis (N1 stage), multiple tumors, and a higher tumor grade or TNM stage had a higher PIIN score. No association between age, sex, MVI, and PIIN score was found (Figures 3E-L), suggesting that the PIIN score is only associated with adverse clinical and tumoral characteristics. The ROC analysis enhanced the accuracy of the PIIN score, with 1-, 3-, and 5-year OS for AUCs of 0.64,0.66, and 0.69 in the training set (Figure 3M) and 0.66, 0.66, and 0.68 in the validation set, respectively (Figure 3N). The ROC value of the PIIN score was significantly higher than that of a single blood marker in the training and validation cohorts (Supplemental Figure 1). Subsequently, the patients with ICC were classified into low- and high-risk groups based on the medium cut-off value of 3.7. The Kaplan–Meier analysis suggested that patients in the high-risk group had shorter OS (training set, p < 0.001; validation set, p = 0.003) and RFS (training set, p < 0.001; validation set, p = 0.002) than those in the low-risk group (Figures 4A-D). The results of the univariate Cox regression analysis are shown in Table 2. After adjusting for other clinicopathologic factors, multivariate Cox regression analysis of the patients in the training set revealed that the PIIN score was one of the independent prognostic factors of survival outcomes of the ICC patients (OS: HR 0.89, 95% CI 0.84–0.94; RFS: HR 0.88, 95% CI 0.83–0.94) (Figures 4E, F). In the validation cohort, the PIIN score was similar for OS and RFS (HR 0.89, 95% CI 0.84–0.94) (Figures 4G, H). These results demonstrate that the PIIN score is vital and may be an independent predictor of ICC prognosis after resection.
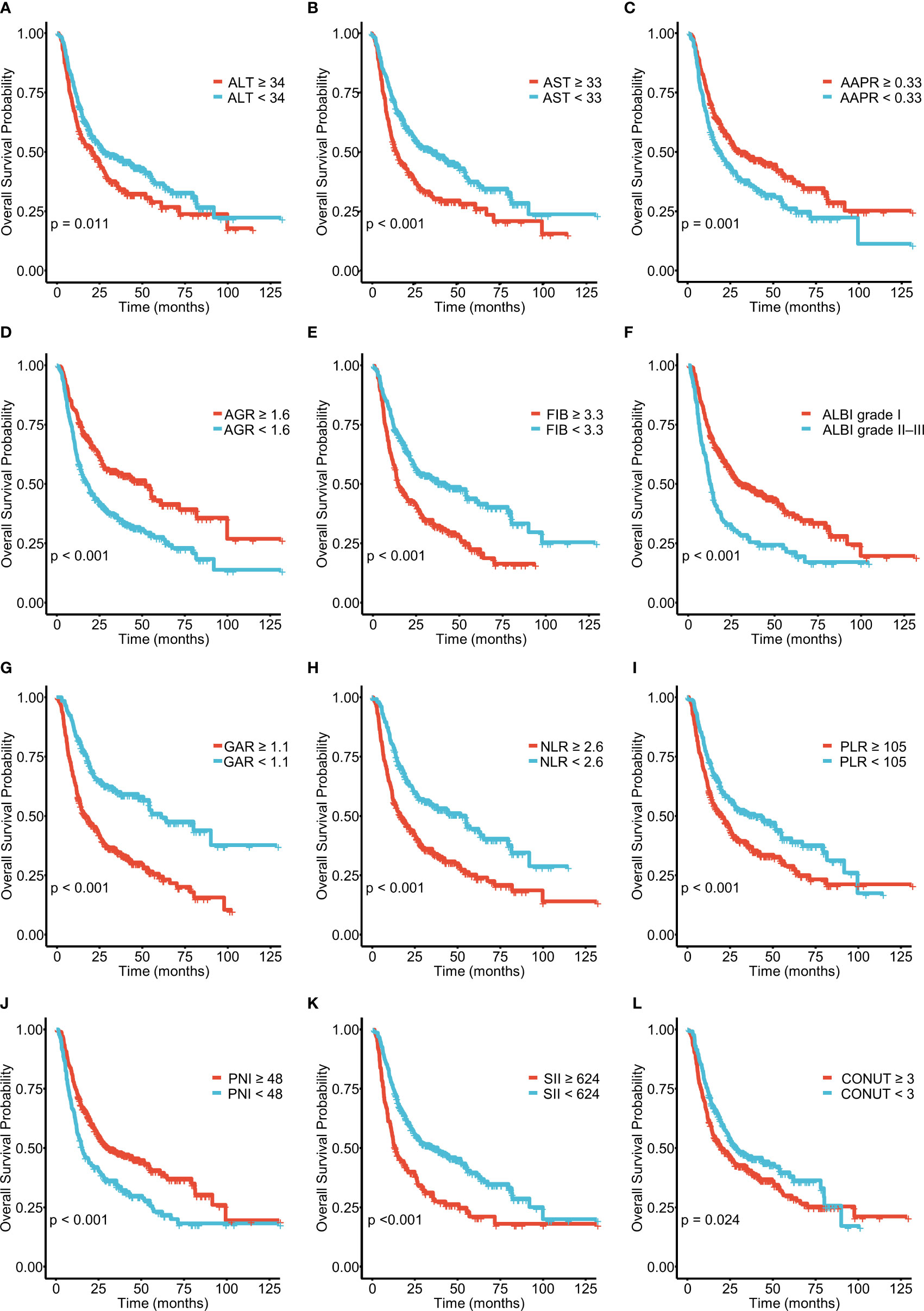
Figure 1 Kaplan–Meier curves for overall survival (OS), stratified by (A) ALT, (B) AST, (C) AAPR, (D) AGR, (E) FIB, and (F) ALBI grades and (G) GAR, (H) NLR, (I) PLR, (J) PNI, (K) SII, and (L) CONUT scores in patients with ICC. ICC, intrahepatic cholangiocarcinoma; ALT, alanine aminotransferase; AST, aspartate aminotransferase; AAPR, albumin–alkaline phosphatase ratio; AGR, albumin–globulin ratio; ALBI, albumin–bilirubin grade; FIB, fibrinogen; GAR, GGT–albumin ratio; NLR, neutrophil- to- lymphocyte ratio; PLR, platelet-to-lymphocyte ratio; PNI, prognostic nutritional index; SII, systemic immune inflammation index; CONUT, controlling nutritional status.
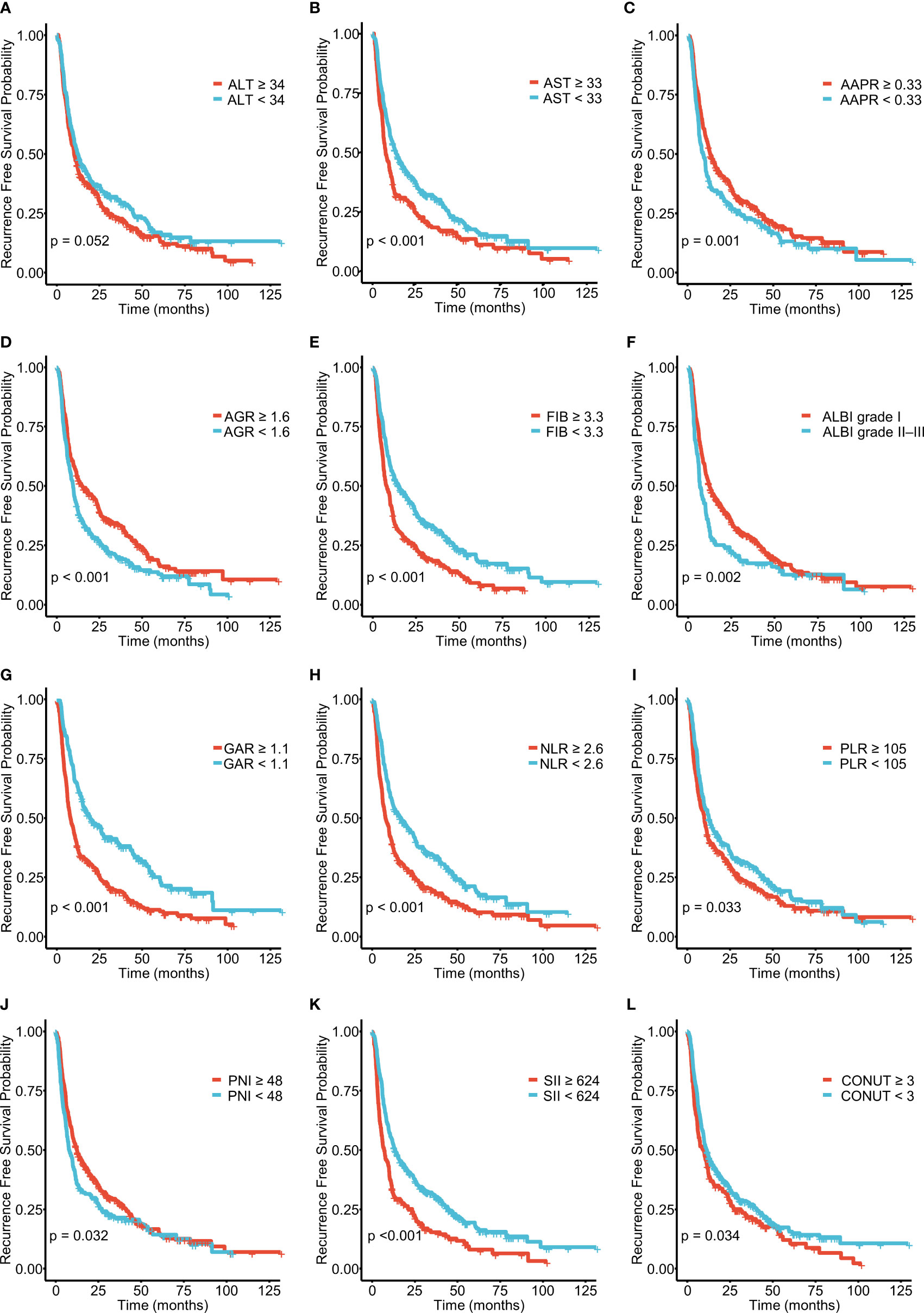
Figure 2 Kaplan–Meier curves for recurrence-free survival (RFS), stratified by (A) ALT, (B) AST, (C) AAPR, (D) AGR, (E) FIB, and (F) ALBI grades and (G) GAR, (H) NLR, (I) PLR, (J) PNI, (K) SII, and (L) PLR in patients with ICC.
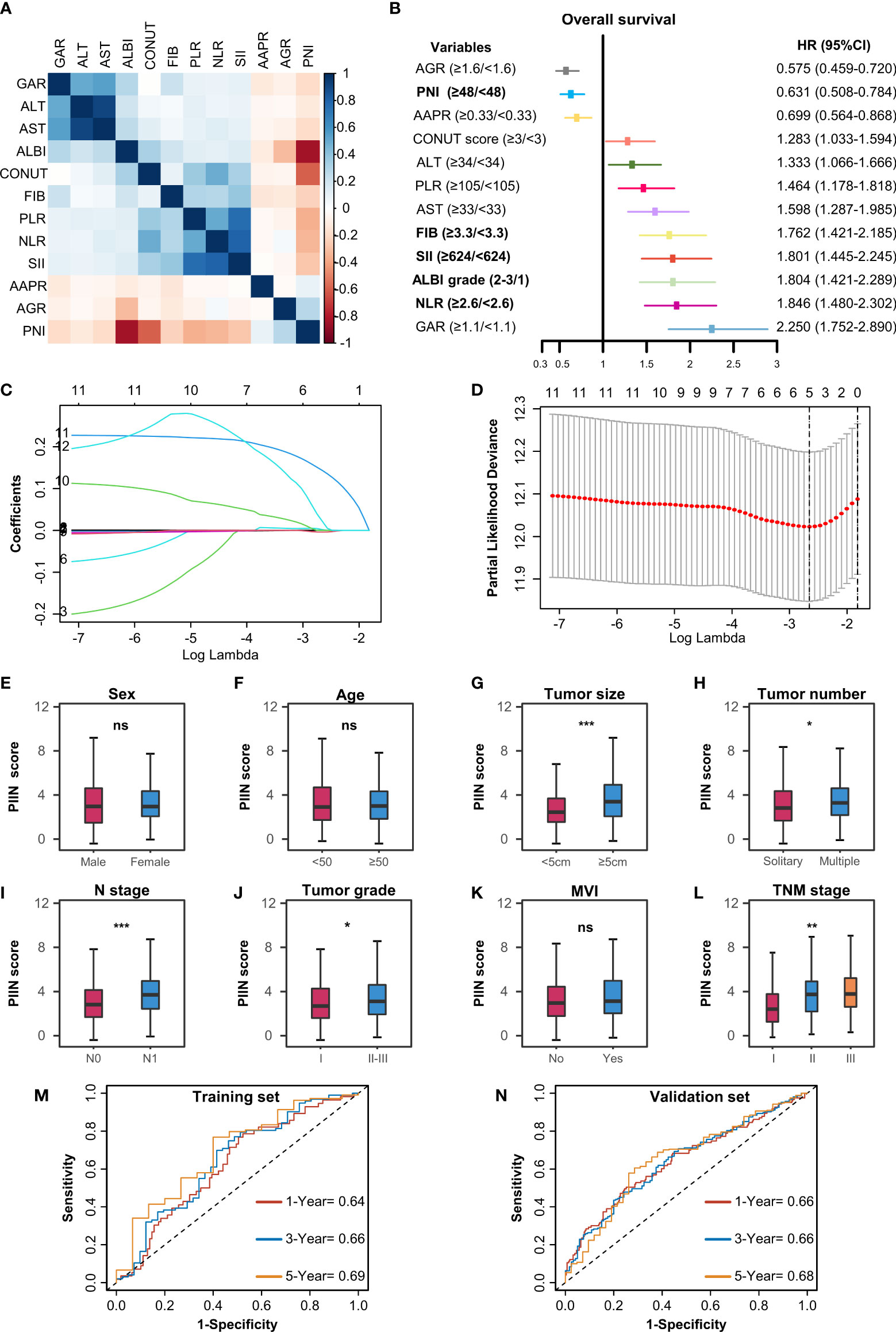
Figure 3 Construction of the PIIN score using the LASSO Cox regression model. (A) Heatmap of the correlations of the immune–inflammatory–nutritional- related biomarkers. (B) Forest plot of the univariate Cox regression analysis for OS. (C) Partial likelihood deviance for LASSO coefficient profiles. The red dots represent the partial likelihood values, the grey lines represent the standard error (SE), and the vertical dotted line shows the optimal values by 1-s.e. (D) Least absolute shrinkage and selection operator (LASSO) coefficient profiles of 12 immune–inflammatory–nutritional- related biomarkers. (E-L) Differential analysis of the distribution of the PIIN scores in different clinicopathologic features. A comparison between the two groups was performed using the Wilcoxon test. Three group comparisons were performed using the Kruskal–Wallis test. *P< 0.05; **P< 0.01; ***P< 0.001; ns not significant. The ROC curves for predicting OS at 1-, 3-, and 5 years in the training set (M) and the validation set (N). ROC, receiver operating characteristic.
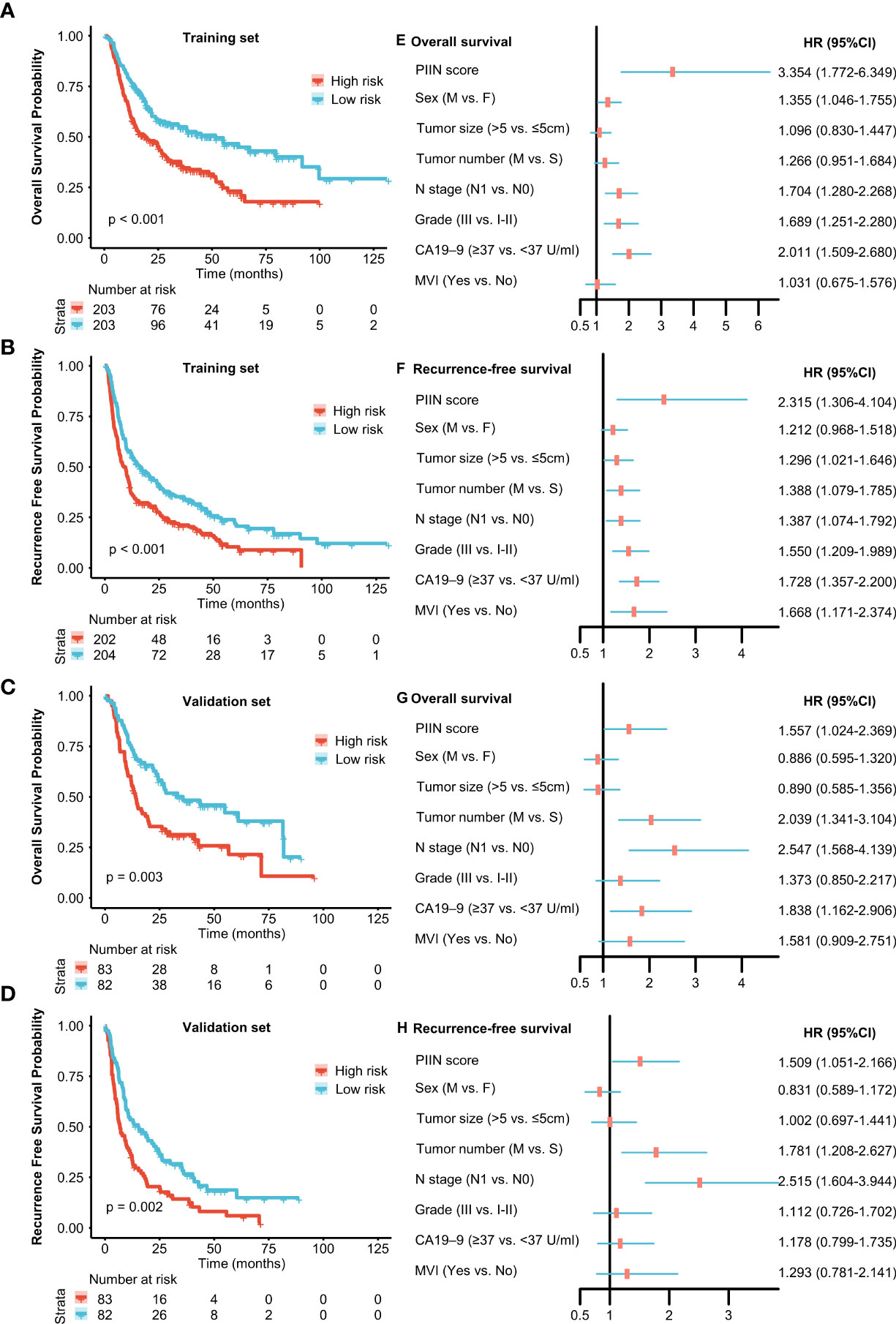
Figure 4 Prognostic implications of the PIIN score. Kaplan–Meier curves of OS (A) and RFS (B) for patients in the low- and high-risk groups according to the PIIN score in the training set. Kaplan–Meier curves of OS (C) and RFS (D) for patients in the low- and high-risk groups according to the PIIN score in the validation set. Forest plot of multivariable Cox regression analysis of OS (E) and RFS (F) in the training set. Forest plot of multivariable Cox regression analysis of OS (G) and RFS (H) in the validation set.
Development and validation of PIIN-nomograms
According to the multivariate Cox regression analysis by backward stepwise selection with the smallest AIC value, the PIIN score, sex, grade, CA19-9, N stage, and tumor number were used in the final nomogram for OS (Figure 5A). The PIIN score, sex, grade, CA19-9, N stage, MVI, tumor number, and tumor size were included in the nomogram for RFS (Figure 5B). Based on their predictive ability, time-dependent AUC curves were plotted to compare the predictive accuracy of the PIIN-nomograms with the AJCC-TNM staging system. The AUC values for OS and RFS nomograms were significantly higher than those of the AJCC-TNM staging system in the training and validation sets (Figures 5C, D). The calibration curves also showed consistency between nomogram prediction and observed survival outcomes (Figure 6). Collectively, the two PIIN-nomograms were particularly discriminative and calibrative. Furthermore, DCA was performed to evaluate the clinical application of the nomogram by quantifying the net benefits at different threshold probabilities (Figure 7). DCA showed that the PIIN-nomograms were superior to the AJCC-TNM staging system in predicting ICC prognosis.
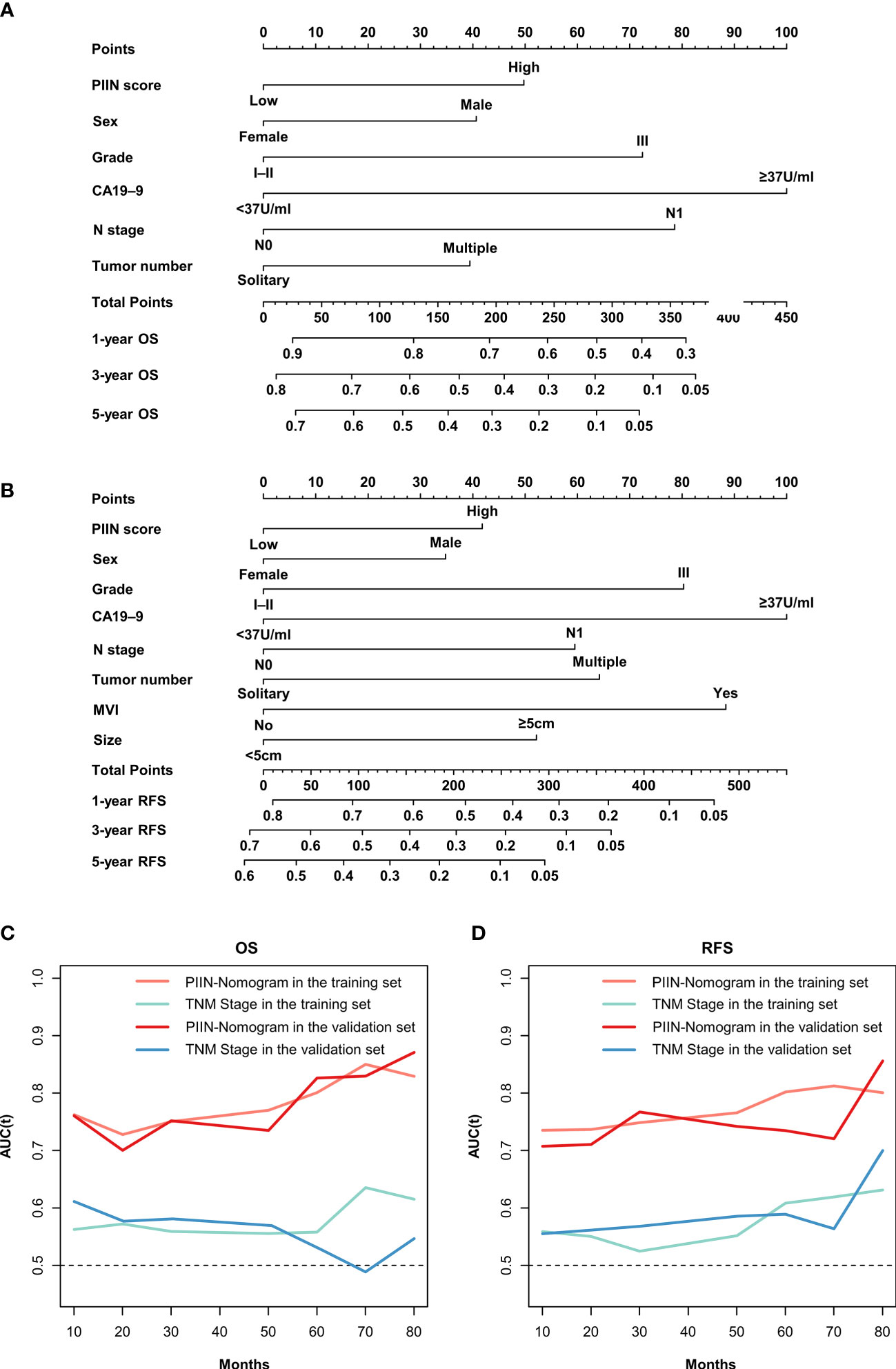
Figure 5 Construction and validation of the nomograms. Nomograms incorporating the PIIN score and other clinicopathological parameters for OS (A) and RFS (B) prediction in the training cohort. (C) Time-dependent AUC curves of the PIIN-nomogram and the AJCC-TNM staging system for the prediction of OS in the training and validation sets. (D) Time-dependent AUC curves of the PIIN-nomogram and the AJCC-TNM staging system for predicting RFS in the training and validation sets. AUC, area under the curve; AJCC-TNM, American Joint Committee on Cancer tumor–node–metastasis.
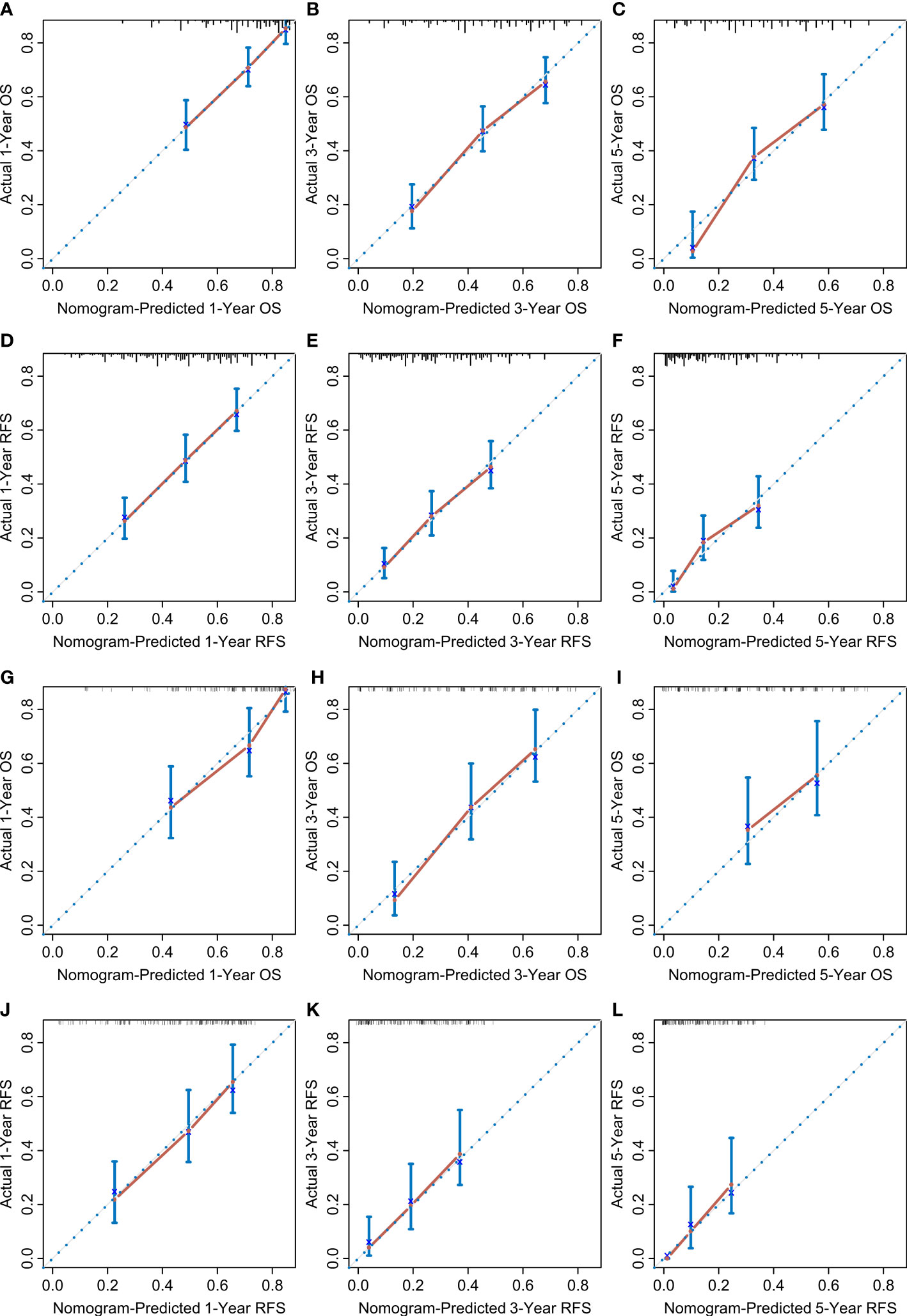
Figure 6 Calibration curves. The calibration curves of the nomograms between predicted and observed 1-, 3-, and 5-year OS of patients in the training set (A–C) and the validation set (G–I). The calibration curves of the nomograms between predicted and observed 1-, 3-, and 5-year RFS in the training set (D–F) and the validation set (J–L). The dashed line of 45° represents the perfect prediction of the nomogram.
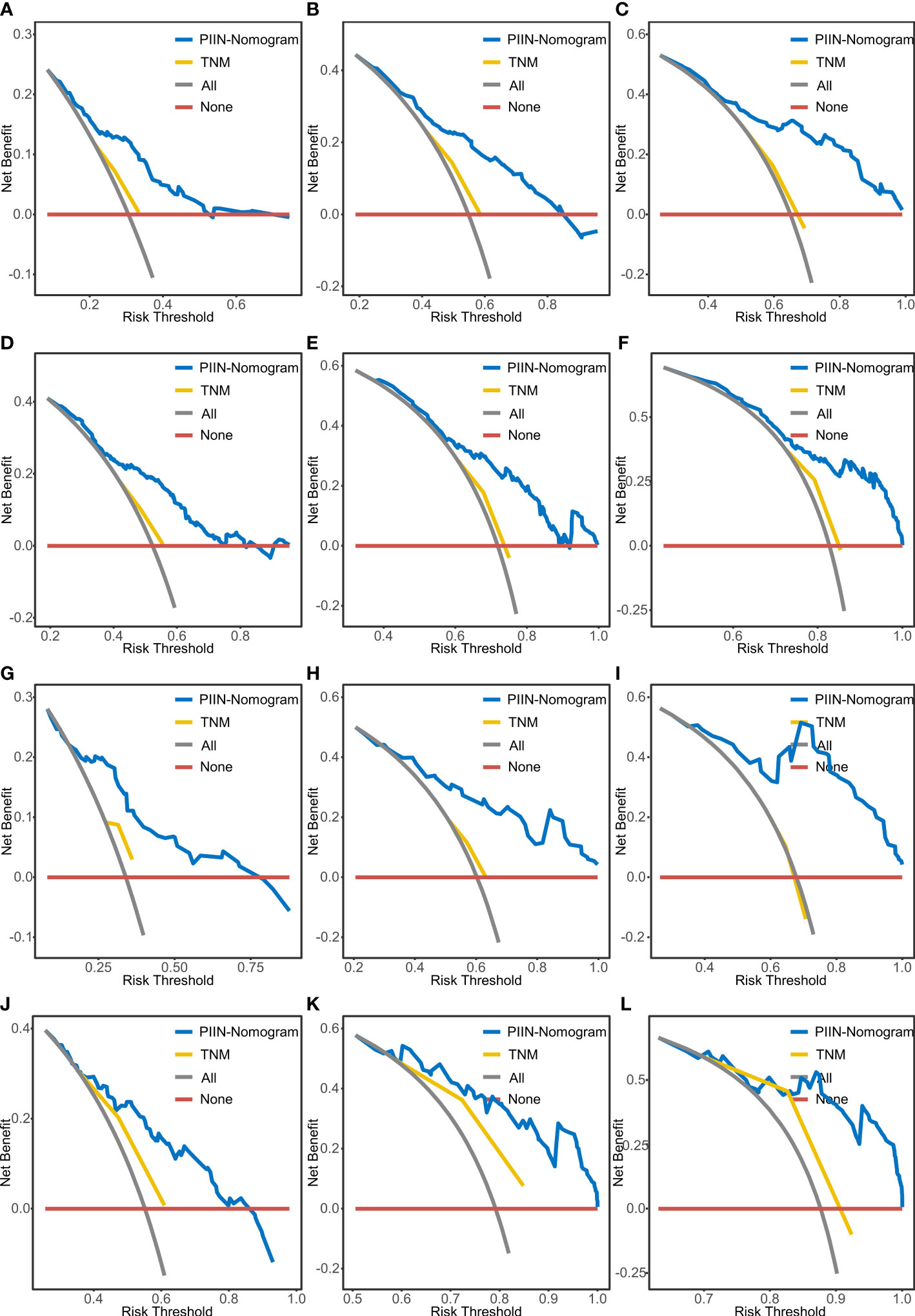
Figure 7 DCA of OS and RFS prediction by the nomograms. The DCA of the nomogram and AJCC-TNM stage for 1-year OS (A), 3-year OS (B), and 5-year OS (C) and for 1-year RFS (D), 3-year RFS (E), and 5-year RFS (F) in the training set. DCA of the nomogram and AJCC-TNM stage for 1-year OS (G), 3-year OS (H), and 5-year OS (I) and for 1-year RFS (J), 3-year RFS (K), and 5-year RFS (L) in the validation set. DCA, decision curve analysis; AJCC-TNM, American Joint Committee on Cancer tumor–node–metastasis.
Discussion
A specific connection exists between immunity, inflammation, nutrition, and cancer (15, 25, 26). Several studies have demonstrated the core role of cancer-related inflammation in cancer development and progression (14, 27). Previous studies revealed that the relevant biomarkers, such as the systemic inflammation score (SIS) and inflammatory marker C-reactive protein (CRP), can predict the prognosis of cancer patients (28, 29). Several nutrition indexes, such as the BMI, PNI, and CONUT scores, have also been reported as potential prognostic cancer predictors (30, 31). Immunity-related biomarkers derived from peripheral blood are significantly associated with survival outcomes in multiple human cancers, including ICC (21, 32–34).
A single indicator is insufficient for prognosis risk stratification, highlighting the urgent need to integrate these markers. Immune-, inflammatory-, and nutritional-related biomarkers were extensively collected in this study based on the peripheral blood testing of patients with ICC. To our knowledge, this is the first study to evaluate the prognostic value and clinical relevance of immune-, inflammatory-, and nutritional-related factors and to establish the PIIN score based on these biomarkers. Our study findings showed that the ALT, AST, and ALBI grades, the AAPR, AGR, FIB, GAR, NLR, PLR, SII, and CONUT scores, and PNI are significantly related to the survival of patients with ICC. The PIIN score was a valuable marker for prognostic stratification and was predictive for patients in the training and validation sets. Moreover, compared with the TNM staging system, nomograms used in this study combined the PIIN score and clinical factors, improving the survival prediction of patients with ICC.
The PIIN score consists of four biomarkers (NLR, SII, FIB, and ALBI grade) reflecting the immune function, inflammation, and nutrition status. The predictive capacity of these markers has been confirmed in several types of cancer. The ALBI grade, proposed by Japanese scholars in 2014 (35), is an independent prognostic predictor after hepatic resection in the early stage of ICC (36), because it is calculated from albumin and bilirubin. A high ALBI grade causes high levels of malnutrition (37) and was associated with worse outcomes in patients with ICC. Consistent with previous reports (38, 39), serum NLR was an independent prognostic factor for the survival of patients with ICC. The increase in serum NLR levels reflected lymphopenia, neutrophilia, or both and represented an inability of the immune system to suppress cancer progression, thus contributing to poor prognosis. SII comprises peripheral blood, lymphocyte, platelet counts, and neutrophil, which can comprehensively estimate the host’s immune response and inflammatory status (18, 23). Hu et al. (40) found that SII is significantly associated with worse survival in hepatocellular carcinoma. Our study findings demonstrate that SII could also serve as a valuable independent prognostic factor for patients with ICC. Moreover, the complex cross-talk between the activation of coagulation and inflammation in cancer has received much attention. Abnormal hypercoagulable condition is often observed in most cancer patients (41). FIB is a glycoprotein in coagulation and is synthesized by the hepatocytes. It promotes tumor development, angiogenesis, and metastasis (42, 43). In this study, FIB was a useful prognostic marker for evaluating clinical outcomes in patients with ICC. The biochemical parameters were routinely examined for preoperative blood tests. Therefore, the PIIN score can be easily obtained in a clinical setting. The favorable predictive performance of the PIIN score implies that it is associated with malnutrition, immunosuppression, active inflammatory reaction, and tumor progression. As validated in the multicenter dataset, the PIIN score was expected to have a considerable predictive capacity for ICC. A nomogram model was constructed to accurately predict the survival of patients with ICC by incorporating the PIIN score and other clinicopathological variables with the time-dependent AUC reaching about 0.70~0.88. This study had several limitations. First, given that all the participants were Chinese patients, the PIIN score in other cohorts worldwide was not evaluated using the nomogram model. Secondly, none of the patients included in this study received adjuvant immunotherapies. Thus, the possible application value of the PIIN score in predicting response to immunotherapy was not evaluated. Finally, genomic, transcriptomic, and proteomic level investigations are needed to reveal prognosis differences in patients with ICC (44, 45).
Conclusion
The PIIN score, as a promising immune-, inflammatory-, and nutritional-related prognostic biomarker, provides insights into the biological and clinical evaluation of patients with ICC. Moreover, by integrating the PIIN scores and other clinical risk factors, the PIIN- nomograms facilitate individualized prognostic assessments for patients with ICC.
Data availability statement
The raw data supporting the conclusions of this article will be made available by the authors, without undue reservation.
Ethics statement
The studies involving human participants were reviewed and approved by The local Ethics Committee of West China Hospital of Sichuan University. The patients/participants provided their written informed consent to participate in this study.
Author contributions
Conception and design: JZ, HW. Development of methodology: JZ, RH. Acquisition of data: CL, FG, TL, HL, HW. Analysis and interpretation of data: JZ, RH, DW, XF, HL. Writing, review, and/or revision of the manuscript: JZ, TL, DW, XF, HW. Administrative, technical, or material support: RH, FG, HL. Study supervision: HW. All authors read and approved the final manuscript.
Funding
This work was supported by grants from the National Natural Science Foundation of China (82173124, 82103533, 81972747, and 82203823), the Natural Science Foundation of Sichuan Province (2023NSFSC1877), the Natural Science Foundation of Chongqing (CSTB2022NSCQ-MSX0477), and the China Postdoctoral Science Foundation (2022TQ0393).
Conflict of interest
The authors declare that the research was conducted in the absence of any commercial or financial relationships that could be construed as a potential conflict of interest.
Publisher’s note
All claims expressed in this article are solely those of the authors and do not necessarily represent those of their affiliated organizations, or those of the publisher, the editors and the reviewers. Any product that may be evaluated in this article, or claim that may be made by its manufacturer, is not guaranteed or endorsed by the publisher.
Supplementary material
The Supplementary Material for this article can be found online at: https://www.frontiersin.org/articles/10.3389/fimmu.2023.1165510/full#supplementary-material
Supplementary Figure 1 | ROC curves showing the predictive power of the PIIN score compared to a single blood marker in the training and validation sets.
References
1. Bertuccio P, Malvezzi M, Carioli G, Hashim D, Boffetta P, El-Serag HB, et al. Global trends in mortality from intrahepatic and extrahepatic cholangiocarcinoma. J Hepatol (2019) 71(1):104–14. doi: 10.1016/j.jhep.2019.03.013
2. Zhang H, Yang T, Wu M, Shen F. Intrahepatic cholangiocarcinoma: Epidemiology, risk factors, diagnosis and surgical management. Cancer Lett (2016) 379(2):198–205. doi: 10.1016/j.canlet.2015.09.008
3. Cillo U, Fondevila C, Donadon M, Gringeri E, Mocchegiani F, Schlitt HJ, et al. Surgery for cholangiocarcinoma. Liver Int (2019) 39(Suppl Suppl 1):143–55. doi: 10.1111/liv.14089
4. Li Q, Chen C, Su J, Qiu Y, Wu H, Song T, et al. Recurrence and prognosis in intrahepatic cholangiocarcinoma patients with different etiology after radical resection: A multi-institutional study. BMC Cancer (2022) 22(1):329. doi: 10.1186/s12885-022-09448-w
5. Zhou SN, Lu SS, Ju DW, Yu LX, Liang XX, Xiang X, et al. A new prognostic model covering all stages of intrahepatic cholangiocarcinoma. J Clin Trans Hepatol (2022) 10(2):254–62. doi: 10.14218/jcth.2021.00099
6. Nassar A, Tzedakis S, Sindayigaya R, Hobeika C, Marchese U, Veziant J, et al. Factors of early recurrence after resection for intrahepatic cholangiocarcinoma. World J Surg (2022) 46(10):2459–67. doi: 10.1007/s00268-022-06655-1
7. Turner KM, Delman AM, Kharofa J, Olowokure O, Sohal D, Quillin RC, et al. A national assessment of T2 staging for intrahepatic cholangiocarcinoma and the poor prognosis associated with multifocality. Ann Surg Oncol (2022) 29(8):5094–102. doi: 10.1245/s10434-022-11762-3
8. Zhu J, Liu C, Li H, Ren H, Cai Y, Lan T, et al. Adequate lymph node dissection is essential for accurate nodal staging in intrahepatic cholangiocarcinoma: A population-based study. Cancer Med (2023) 00:1–15. doi: 10.1002/cam4.5620
9. Zhang XF, Xue F, Dong DH, Weiss M, Popescu I, Marques HP, et al. Number and station of lymph node metastasis after curative-intent resection of intrahepatic cholangiocarcinoma impact prognosis. Ann Surg (2021) 274(6):e1187–e95. doi: 10.1097/SLA.0000000000003788
10. Xiang F, Wei S, Liu X, Liang X, Yang L, Yan S. Radiomics analysis of contrast-enhanced ct for the preoperative prediction of microvascular invasion in mass-forming intrahepatic cholangiocarcinoma. Front Oncol (2021) 11:774117. doi: 10.3389/fonc.2021.774117
11. Moro A, Mehta R, Sahara K, Tsilimigras DI, Paredes AZ, Farooq A, et al. The impact of preoperative Ca19-9 and cea on outcomes of patients with intrahepatic cholangiocarcinoma. Ann Surg Oncol (2020) 27(8):2888–901. doi: 10.1245/s10434-020-08350-8
12. Wiseman MJ. Nutrition and cancer: Prevention and survival. Br J Nutr (2019) 122(5):481–7. doi: 10.1017/S0007114518002222
13. Engelhard VH, Rodriguez AB, Mauldin IS, Woods AN, Peske JD, Slingluff CL Jr. Immune cell infiltration and tertiary lymphoid structures as determinants of antitumor immunity. J Immunol (2018) 200(2):432–42. doi: 10.4049/jimmunol.1701269
14. Kiely M, Lord B, Ambs S. Immune response and inflammation in cancer health disparities. Trends Cancer (2022) 8(4):316–27. doi: 10.1016/j.trecan.2021.11.010
15. Zitvogel L, Pietrocola F, Kroemer G. Nutrition, inflammation and cancer. Nat Immunol (2017) 18(8):843–50. doi: 10.1038/ni.3754
16. Demaria O, Cornen S, Daëron M, Morel Y, Medzhitov R, Vivier E. Harnessing innate immunity in cancer therapy. Nature (2019) 574(7776):45–56. doi: 10.1038/s41586-019-1593-5
17. Mirza AH, Thomas G, Ottensmeier CH, King EV. Importance of the immune system in head and neck cancer. Head Neck (2019) 41(8):2789–800. doi: 10.1002/hed.25716
18. Nost TH, Alcala K, Urbarova I, Byrne KS, Guida F, Sandanger TM, et al. Systemic inflammation markers and cancer incidence in the uk biobank. Eur J Epidemiol (2021) 36(8):841–8. doi: 10.1007/s10654-021-00752-6
19. Xie H, Ruan G, Ge Y, Zhang Q, Zhang H, Lin S, et al. Inflammatory burden as a prognostic biomarker for cancer. Clin Nutr (Edinburgh Scotland) (2022) 41(6):1236–43. doi: 10.1016/j.clnu.2022.04.019
20. Jung S-H, Hao J, Shivakumar M, Nam Y, Kim J, Kim MJ, et al. Development and validation of a novel strong prognostic index for colon cancer through a robust combination of laboratory features for systemic inflammation: A prognostic immune nutritional index. Br J Cancer (2022) 126(11):1539–47. doi: 10.1038/s41416-022-01767-w
21. Li WZ, Hua X, Lv SH, Liang H, Liu GY, Lu N, et al. A scoring system based on nutritional and inflammatory parameters to predict the efficacy of first-line chemotherapy and survival outcomes for De novo metastatic nasopharyngeal carcinoma. J Inflammation Res (2021) 14:817–28. doi: 10.2147/JIR.S296710
22. Sun L, Su S, Xiong J, Hu W, Liu L, Xu H, et al. Controlling nutritional status score as a prognostic marker to predict overall survival in resected biliary tract cancers. Ann Transl Med (2021) 9(8):644. doi: 10.21037/atm-20-6770
23. Tsilimigras DI, Moris D, Mehta R, Paredes AZ, Sahara K, Guglielmi A, et al. The systemic immune-inflammation index predicts prognosis in intrahepatic cholangiocarcinoma: An international multi-institutional analysis. HPB (Oxford) (2020) 22(12):1667–74. doi: 10.1016/j.hpb.2020.03.011
24. Yang Z, Zhang D, Zeng H, Fu Y, Hu Z, Pan Y, et al. Inflammation-based scores predict responses to pd-1 inhibitor treatment in intrahepatic cholangiocarcinoma. J Inflammation Res (2022) 15:5721–31. doi: 10.2147/JIR.S385921
25. Shaul ME, Fridlender ZG. Tumour-associated neutrophils in patients with cancer. Nat Rev Clin Oncol (2019) 16(10):601–20. doi: 10.1038/s41571-019-0222-4
26. Maiorino L, Daßler-Plenker J, Sun L, Egeblad M. Innate immunity and cancer pathophysiology. Annu Rev Pathol (2022) 17:425–57. doi: 10.1146/annurev-pathmechdis-032221-115501
27. Karin M, Shalapour S. Regulation of antitumor immunity by inflammation-induced epigenetic alterations. Cell Mol Immunol (2022) 19(1):59–66. doi: 10.1038/s41423-021-00756-y
28. Zhang HY, Xie HL, Ruan GT, Zhang Q, Ge YZ, Liu XY, et al. Lymphocyte to c-reactive protein ratio could better predict the prognosis of patients with stage iv cancer. BMC Cancer (2022) 22(1):1080. doi: 10.1186/s12885-022-10145-x
29. Ruan GT, Xie HL, Deng L, Ge YZ, Zhang Q, Wang ZW, et al. A novel systemic inflammation prognostic score to stratify survival in elderly patients with cancer. Front Nutr (2022) 9:893753. doi: 10.3389/fnut.2022.893753
30. Petrelli F, Cortellini A, Indini A, Tomasello G, Ghidini M, Nigro O, et al. Association of obesity with survival outcomes in patients with cancer: A systematic review and meta-analysis. JAMA Netw Open (2021) 4(3):e213520. doi: 10.1001/jamanetworkopen.2021.3520
31. Lu C, Chen Q, Fei L, Wang J, Wang C, Yu L. Prognostic impact of the controlling nutritional status score in patients with hematologic malignancies: A systematic review and meta-analysis. Front Immunol (2022) 13:952802. doi: 10.3389/fimmu.2022.952802
32. Wang N, Xi W, Lu S, Jiang J, Wang C, Zhu Z, et al. A novel inflammatory-nutritional prognostic scoring system for stage iii gastric cancer patients with radical gastrectomy followed by adjuvant chemotherapy. Front Oncol (2021) 11:650562. doi: 10.3389/fonc.2021.650562
33. Zhou Z, Wang P, Sun R, Li J, Hu Z, Xin H, et al. Tumor-associated neutrophils and macrophages interaction contributes to intrahepatic cholangiocarcinoma progression by activating Stat3. J immunother Cancer (2021) 9(3):e001946. doi: 10.1136/jitc-2020-001946
34. Li J, Cao D, Huang Y, Xiong Q, Tan D, Liu L, et al. The prognostic and clinicopathological significance of systemic immune-inflammation index in bladder cancer. Front Immunol (2022) 13:865643. doi: 10.3389/fimmu.2022.865643
35. Johnson PJ, Berhane S, Kagebayashi C, Satomura S, Teng M, Reeves HL, et al. Assessment of liver function in patients with hepatocellular carcinoma: A new evidence-based approach-the albi grade. J Clin Oncol (2015) 33(6):550–8. doi: 10.1200/JCO.2014.57.9151
36. Pinato DJ, Sharma R, Allara E, Yen C, Arizumi T, Kubota K, et al. The albi grade provides objective hepatic reserve estimation across each bclc stage of hepatocellular carcinoma. J Hepatol (2017) 66(2):338–46. doi: 10.1016/j.jhep.2016.09.008
37. Kaneko S, Kurosaki M, Tsuchiya K, Yasui Y, Inada K, Kirino S, et al. Prognosis of intrahepatic cholangiocarcinoma stratified by albumin-bilirubin grade. Hepatol Res (2021) 51(8):902–8. doi: 10.1111/hepr.13673
38. Maeda T, Hiura A, Uehara J, Toyoshima R, Nakagawa T, Yoshino K. Neutrophil-to-Lymphocyte ratio is associated with survival and sentinel lymph node positivity in invasive cutaneous squamous cell carcinoma: A retrospective study. J Am Acad Dermatol (2022) 86(3):615–20. doi: 10.1016/j.jaad.2021.10.033
39. Buettner S, Spolverato G, Kimbrough CW, Alexandrescu S, Marques HP, Lamelas J, et al. The impact of neutrophil-to-Lymphocyte ratio and platelet-to-Lymphocyte ratio among patients with intrahepatic cholangiocarcinoma. Surgery (2018) 164(3):411–8. doi: 10.1016/j.surg.2018.05.002
40. Hu B, Yang X-R, Xu Y, Sun Y-F, Sun C, Guo W, et al. Systemic immune-inflammation index predicts prognosis of patients after curative resection for hepatocellular carcinoma. Clin Cancer Res (2014) 20(23):6212–22. doi: 10.1158/1078-0432.Ccr-14-0442
41. Parisi R, Panzera T, Russo L, Gamba S, De Curtis A, Di Castelnuovo A, et al. Fibrinogen levels in relation to colorectal cancer onset: A nested case-cohort study from the moli-sani cohort. Front Cardiovasc Med (2022) 9:1009926. doi: 10.3389/fcvm.2022.1009926
42. Liu H, Qiu G, Hu F, Wu H. Fibrinogen/Albumin ratio index is an independent predictor of recurrence-free survival in patients with intrahepatic cholangiocarcinoma following surgical resection. World J Surg Oncol (2021) 19(1):218. doi: 10.1186/s12957-021-02330-2
43. Zhang F, Wang Y, Sun P, Wang Z-q, Wang D-s, Zhang D-s, et al. Fibrinogen promotes malignant biological tumor behavior involving epithelial–mesenchymal transition Via the p-Akt/P-Mtor pathway in esophageal squamous cell carcinoma. J Cancer Res Clin Oncol (2017) 143(12):2413–24. doi: 10.1007/s00432-017-2493-4
44. Chen S, Xie Y, Cai Y, Hu H, He M, Liu L, et al. Multiomic analysis reveals comprehensive tumor heterogeneity and distinct immune subtypes in multifocal intrahepatic cholangiocarcinoma. Clin Cancer Res (2022) 28(9):1896–910. doi: 10.1158/1078-0432.Ccr-21-1157
Keywords: intrahepatic cholangiocarcinoma, immunity, inflammation, nutrition, prognosis, nomogram
Citation: Zhu J, Wang D, Liu C, Huang R, Gao F, Feng X, Lan T, Li H and Wu H (2023) Development and validation of a new prognostic immune–inflammatory–nutritional score for predicting outcomes after curative resection for intrahepatic cholangiocarcinoma: A multicenter study. Front. Immunol. 14:1165510. doi: 10.3389/fimmu.2023.1165510
Received: 14 February 2023; Accepted: 21 March 2023;
Published: 31 March 2023.
Edited by:
Xuefeng Li, Guangzhou Medical University, ChinaReviewed by:
Yi Han, University of Texas Southwestern Medical Center, United StatesXin Long, University of Texas Southwestern Medical Center, United States
Wen Li, Guangzhou Medical University, China
Copyright © 2023 Zhu, Wang, Liu, Huang, Gao, Feng, Lan, Li and Wu. This is an open-access article distributed under the terms of the Creative Commons Attribution License (CC BY). The use, distribution or reproduction in other forums is permitted, provided the original author(s) and the copyright owner(s) are credited and that the original publication in this journal is cited, in accordance with accepted academic practice. No use, distribution or reproduction is permitted which does not comply with these terms.
*Correspondence: Hong Wu, d3Vob25nQHNjdS5lZHUuY24=; Hui Li, NDQ1NjEzNDg3QHFxLmNvbQ==; Tian Lan, Ymx1ZV9za3lfbGFuZEAxNjMuY29t
†These authors have contributed equally to this work
‡ORCID: Jiang Zhu, orcid.org/0000-0003-4194-2355