- 1Vrije Universiteit Brussel, Center for Neurosciences (C4N), Jette, Brussels, Belgium
- 2Universiteit Antwerpen, Department of Biomedical Sciences and Institute Born-Bunge, Reference Center for Biological Markers of Dementia (BIODEM), Wilrijk, Antwerp, Belgium
- 3Universitair Ziekenhuis Brussel, Department of Neurology, Jette, Brussels, Belgium
- 4Universitair Ziekenhuis Brussel, Department of Clinical Biology, Laboratory of Clinical Neurochemistry, Jette, Brussels, Belgium
- 5National MS Center (NMSC), Neurology, Melsbroek, Steenokkerzeel, Belgium
Inflammatory processes are involved in the pathophysiology of both Alzheimer’s disease (AD) and multiple sclerosis (MS) but their exact contribution to disease progression remains to be deciphered. Biomarkers are needed to define pathophysiological processes of these disorders, who may increasingly co-exist in the elderly generations of the future, due to the rising prevalence in both and ameliorated treatment options with improved life expectancy in MS. The purpose of this review was to provide a systematic overview of inflammatory biomarkers, as measured in the cerebrospinal fluid (CSF), that are associated with clinical disease progression. International peer-reviewed literature was screened using the PubMed and Web of Science databases. Disease progression had to be measured using clinically validated tests representing baseline functional and/or cognitive status, the evolution of such clinical scores over time and/or the transitioning from one disease stage to a more severe stage. The quality of included studies was systematically evaluated using a set of questions for clinical, neurochemical and statistical characteristics of the study. A total of 84 papers were included (twenty-five for AD and 59 for MS). Elevated CSF levels of chitinase-3-like protein 1 (YKL-40) were associated with disease progression in both AD and MS. Osteopontin and monocyte chemoattractant protein-1 were more specifically related to disease progression in AD, whereas the same was true for interleukin-1 beta, tumor necrosis factor alpha, C-X-C motif ligand 13, glial fibrillary acidic protein and IgG oligoclonal bands in MS. We observed a broad heterogeneity of studies with varying cohort characterization, non-disclosure of quality measures for neurochemical analyses and a lack of adequate longitudinal designs. Most of the retrieved biomarkers are related to innate immune system activity, which seems to be an important mediator of clinical disease progression in AD and MS. Overall study quality was limited and we have framed some recommendations for future biomarker research in this field.
Systematic review registration: https://www.crd.york.ac.uk/prospero/, identifier CRD42021264741.
1 Introduction
Alzheimer’s disease (AD) and multiple sclerosis (MS) are two frequently occurring and debilitating disorders of the central nervous system (CNS). Whereas these conditions exhibit fundamental epidemiological and pathobiological differences, they also share a number of striking commonalities, making them suitable for an integrative approach in translational research. Cognitive decline is the core clinical feature of AD, usually starting with memory loss, language difficulties and visuospatial deficits (1). In MS, physical symptoms are often more visible (2), but impaired cognition (typically affecting information processing speed, episodic memory, attention and executive function), which may be subtle but therefore not necessarily less troublesome, has been reported in up to 70% of patients and can already be present from the early stages of the disease (3, 4). Neurodegeneration is the ultimate pathological mechanism leading to functional decline in both entities, accounting as the main determinant of global long-term prognosis in MS (5), and highly correlating with cognitive decline in individuals with AD (6). Inflammatory responses are involved as well, to varying degrees and as explained more into detail below, but their precise role in the respective neurodegenerative cascades remains to be elucidated (7). Interestingly, the life expectancy of individuals with MS has significantly improved over the past decades, probably due to ameliorated disease modifying treatments (DMT) and/or general medical care. As a consequence, and in combination with a rising prevalence of both disorders (8, 9), there is a growing likelihood of co-existence of MS with classic age-related dementias such as AD (10). Some studies have even reported an increased risk of AD diagnosis in patients with MS, as compared to controls (11–13). In order to enable high-quality precision medicine, it will become increasingly important to unravel the unique and (possibly) shared pathophysiological processes of both disorders. Biomarkers are vital when investigating disease mechanisms as they are the in vivo proxy of neuropathology, used in both medical routine and clinical trials to achieve formal (and early) diagnosis/patient stratification, predict disability progression and/or monitor treatment response (14, 15). In addition, biomarker research may lead to the identification of new druggable targets for disease modification, potentially leading to prevention of functional decline in affected individuals.
AD is classically viewed as a pure neurodegenerative disorder, characterized by the deposition of amyloid beta (Aβ)-containing plaques and neurofibrillary tangles composed of hyperphosphorylated tau protein in the brain. The earliest biomarker sign representing AD pathology is a reduced concentration of Aβ1-42 and a decreased Aβ1-42/Aβ1-40 ratio in the cerebrospinal fluid (CSF), of which the latter is the most specific for plaque pathology (16). Neuropathology may develop up to 20 years before the first manifestation of cognitive symptoms (17) and supports the concept that AD is a continuum with a long preclinical phase, followed by a prodromal phase of mild cognitive impairment (MCI) that eventually leads to dementia (18). Positron emission tomography (PET) studies have demonstrated an increased density of translocator protein (TSPO), a marker for neuroinflammation that is predominantly present in microglia, in patients with AD, as compared to healthy individuals (19). Disease-associated microglia (DAMs), a context-dependent microglia state identified by novel technologies, such as single-cell RNA sequencing (20), have been found in the vicinity of the amyloid plaques (21), while several well-established AD risk genes closely relate to microglial functions and appear to be highly enriched in these cells (22). As such, the innate inflammatory response may be implicated in the early pathological process in AD. However, the heterogenous presentation of AD has previously split the field concerning the role of inflammation and hindered the establishment of inflammatory biomarkers to monitor disease progression. It remains to be determined whether the inflammatory changes are actually a driving force behind the pathology or merely represent a bystander effect, and whether the inflammatory state is beneficial, by clearing pathological aggregates, or detrimental, by acting provocative for the degenerative process (23). Conversely, an inadequate immune response in AD may equally lead to disease progression, as microglia surrounding the plaques apparently fail to take part in phagocytosis (24). Anyhow, current literature does suggest that inflammatory CSF biomarkers are altered in AD (25), but the impact on progression across the disease continuum needs further elaboration.
MS is a neuroinflammatory and -degenerative disorder of the CNS with a causative mechanism that is incompletely understood but presumably autoimmune in origin. Most patients (85%) start with a relapsing-remitting (RR) pattern during which abrupt and at least partially recovering exacerbations of neurological dysfunction (termed relapses) are interchanged with periods of clinical stability. Many will eventually transit into a secondary progressive (SP) phase characterized by a slower but sustained downhill course that may still be accompanied by some relapses, whereas about 15% of patients experience a similar primary progressive (PP) decline from disease onset (2). Focal demyelinating lesions, resulting from acute inflammatory activity with blood-brain barrier (BBB) disruption and perivenular infiltrates of peripheral immune cells (including T-cells, B-cells, plasma cells and macrophages), form the pathological substrate for relapses (2). Recent insights have learned that progressive MS is more likely to rely, among other mechanisms, on innate immune processes behind an intact BBB. Microglia - more specifically microglia inflamed in MS (MIMS; whose transcriptional profile partially overlaps with DAMs) (20, 26) - have been found diffusely throughout the normal-appearing white matter (NAWM) and at the border of a particular subset of chronic slowly expanding lesions (also called chronically active or smoldering lesions), both of which are features increasingly associated with the progressive phase of the disease (27–29). Increased TSPO expression in the brain of patients with MS was found to predict future disability progression and several MS susceptibility genes are enriched in microglia, suggesting an important role of the innate immune response in MS (30, 31). However, similar to the potential double-edged role in AD, microglia also facilitate neuronal repair by clearing debris and stimulating remyelination (32). Indications of MS pathology presumably appear long before clinical diagnosis, as evidenced by various alterations at the clinical, biochemical and/or radiological level (33–37). Some of these prodromal circumstances are well-defined by the terms ‘clinically and radiologically isolated syndrome’ in case of first inflammatory demyelinating episode without proof of dissemination in time (CIS) or when magnetic resonance imaging (MRI) findings are strongly suggestive for MS but without clinical repercussions (RIS), respectively. Despite the identification of the aforementioned inflammatory players in MS pathology, it remains to be seen how they may translate to disease progression biomarkers. Thus far, only oligoclonal bands (OCB), which are the product of intrathecal antibody secretion by activated B-cells, have been included in the official criteria for MS diagnosis (38).
So far, drug development mainly focused on disease-specific characteristics, such as the removal of Aβ plaques in AD and altering the peripheral immune response in MS, but fails to fully cease disease progression (39, 40). Improving our understanding of the complex relationship between neuroinflammation and neurodegeneration in both disorders, which may have more in common than hitherto thought, can be valuable to uncover therapeutic targets that may truly halt disease progression. Notwithstanding the progress that recently has been made in the field of body fluid-based inflammatory biomarker research in AD and MS, its implementation in the real-world clinical practice turns out to be a slow process. With this systematic review, we have listed all CSF inflammatory biomarkers showing an association with clinical disease progression in AD and/or MS patients, in an attempt to identify the most promising candidates for further validation in clinical practice and to gain a deeper understanding in the inflammatory pathways underlying disease progression, which may ultimately culminate in ameliorated clinical care for patients affected by these chronic disorders. Moreover, we have found several methodological and conceptual commonalities in biomarker research for AD and MS, and summarize some general key points to enhance the quality of future efforts studying the relationship with disease progression.
2 Methods
2.1 Research question
We formulated our research question and conceptualized the search terms by using the ‘Patient – Intervention – Comparator – Outcome – Time – Setting (PICOTS) framework’ (41). Our aim was to provide a structured overview of the inflammatory biomarkers in the CSF (I) of patients belonging to the AD and/or MS continuum (P) that have been associated with disease progression (O), as opposed to situations of clinical stability (C), in cross-sectional and/or longitudinal (T) clinical studies (S). Papers were only found suitable for inclusion if disease progression was measured using (a) clinically validated tests representing baseline functional and/or cognitive status, (b) the evolution of such clinical scores over time and/or (c) the transitioning from one (possibly prodromal) disease stage to a more severe stage (i.e., MCI to AD; CIS or RIS to clinically definite MS; RR MS to SP MS). These criteria were also used as the core structure for grouping the retrieved papers, which allows a consistent synthesis of the results. Our review was conducted following the guidelines of the Preferred Reporting Items for Systematic Review and Analyses (PRISMA) (42), we refer to the Supplementary Material for the related checklist (Table S1). The protocol was submitted to the PROSPERO database (Internal Prospective Register of Systematic Reviews; registration number: CRD42021264741), maintained by the Centre for Reviews and Dissemination at the University of York (Heslington, UK), to help avoid duplicate efforts.
2.2 Search strategy
International peer-reviewed literature relevant to our PICOTS research question was screened up to March 24, 2022 using the PubMed and Web of Science databases. To be more inclusive, we developed separate search strategies for AD and MS. Medical Subject Heading search terms were entered in all fields of publication (e.g., abstract, title, keywords); the final search queries are presented in Table 1. Title and abstract from retained papers were subsequently evaluated for eligibility, with additional reading of the entire text in case of non-exclusion. Studies were considered suitable for inclusion and data collection only if they were written in English, were performed in humans and investigated the relationship between CSF inflammatory biomarkers and disease progression in AD and/or MS patients; the latter in accordance with the above described definition. Reviews, case reports and case series were rejected; there were no other exclusion criteria.
Search and selection procedures were performed by a single reviewer (J.T.); for flowchart see Figure 1. Title and abstract screening resulted in 165 candidate papers. Following full-text evaluation, 84 papers (all published between 1991 and 2022) complied to our criteria and were included for data extraction and quality assessment. More than double the number of papers were found in the field of MS (n = 59) compared to AD (n = 25). Uncertainties during the search were resolved by consensus after a discussion involving all authors.
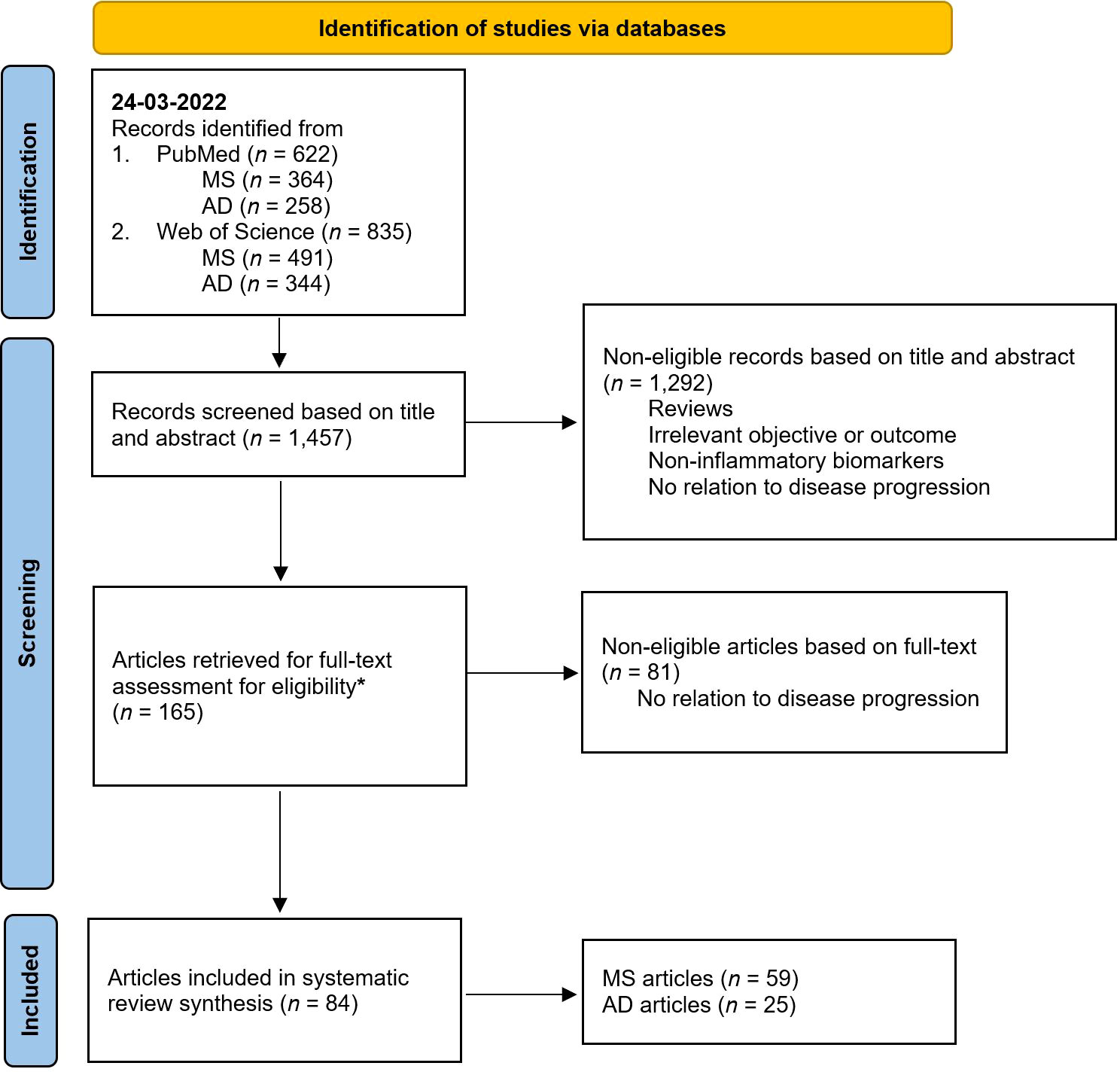
Figure 1 PRISMA 2020 flow chart diagram for systematic reviews. AD, Alzheimer’s disease; MS, multiple sclerosis. * From the 165 papers, 55 raised doubts for inclusion after full-text assessment. These papers were double-checked by all the authors. In the end, 19 papers were included after reaching a consensus, while the remaining 36 were excluded. Illustration adapted from Page et al., 2021 (42).
2.3 Data extraction
Data extraction was performed in a standardized manner by J.T. and M.B. and consisted of the following items: first author, year of publication, journal, study aim, characteristics of the studied cohorts (diagnostic criteria, subgroups, age, clinical status), neurochemical quality measures (assay, intra-assay coefficient of variation, limit of detection, detectability rate, blinding of the researcher), inflammatory biomarkers of interest, observed median and/or mean levels of biomarkers in the studied cohorts, measures of disease progression (with follow-up data in case of longitudinal studies), statistical analyses and main findings. A composite evidentiary Excel spreadsheet (Microsoft Corp, Redmond, WA, USA) compiling all variables was created to ensure reproducibility and completeness of the dataset. The complete overview of the collected data can be found in the Supplementary Material (Table S2).
2.4 Quality assessment
We developed a set of questions to systematically evaluate the methodological quality of each included study, focusing on diagnostic criteria, neurochemical analysis, clinical scoring, cohort size, statistics, validation of findings and, in case of longitudinal studies, duration of follow-up. Separate questions for AD and MS papers were formulated for aspects that were considered disease-specific. The quality assessment was conducted independently by J.T. and M.B., and variations were discussed between both authors until a consensus was reached. Publications with a grade below 50%, from 50% to 80%, and above 80% of the potential maximum score were categorized as “Low”, “Moderate” or “High” in quality, respectively. The quality assessment for all included papers (n = 84) can be found in the Supplementary Material (Table S3). Although most papers were of low quality, none were excluded based on this criterion, in order to be able to provide a complete overview of the literature.
3 Results
3.1 Inflammatory biomarkers related to clinical status
3.1.1 General overview
Of the 84 papers selected for data extraction, 43 focused on the cross-sectional relationship between inflammatory biomarkers and clinically validated scores representing functional status and/or cognition collected at baseline (AD/MS = 14/29). The Mini-Mental State Examination (MMSE) and Expanded Disability Status Scale (EDSS), which are measures of global cognitive impairment and general disability, respectively (43, 44), were the most frequently used scales in AD (MMSE: 85.7%) and MS (EDSS: 96.6%) studies. The vast majority of relationships were assessed using correlation analyses; a detailed overview of these results can be found in the Supplementary Material (Table S4).
3.1.2 AD literature
Cognitive testing in AD studies included MMSE, the Clinical Dementia Rating (CDR), the Alzheimer’s Disease Assessment Scale – Cognitive Subscale (ADAS-Cog), Dementia Rating Scale-2 (DRS-2), the Frontal Assessment Battery (FAB), the Consortium to Establish a Registry for Alzheimer’s Disease (CERAD) neuropsychological battery and the Montreal Cognitive Assessment (MoCA) (45–50). The examined cohorts consisted either of MCI or dementia due to AD patients, with a sample size varying from 19 to 163 individuals.
From the 33 inflammatory biomarkers that were studied, only eight were significantly associated with cognitive impairment: activated helper and cytotoxic T-cells, monocyte chemoattractant protein-1 (MCP-1 or CCL2), C-X-C motif chemokine ligand 8 and 10 (CXCL8 and CXCL10, respectively), interleukin-1 beta (IL-1β), macrophage inflammatory protein-1 alpha (MIP-1α) and osteopontin (OPN) (Table 2A). However, consistent results across multiple studies were only found for OPN (which is a cytokine with regulatory effects on the function of microglia (51)), demonstrating an overall moderate correlation with baseline MMSE scores in subjects with AD (52, 53). These findings were mainly based on cohorts with lower mean MMSE values and older age, likely representative for a more advanced disease stage.
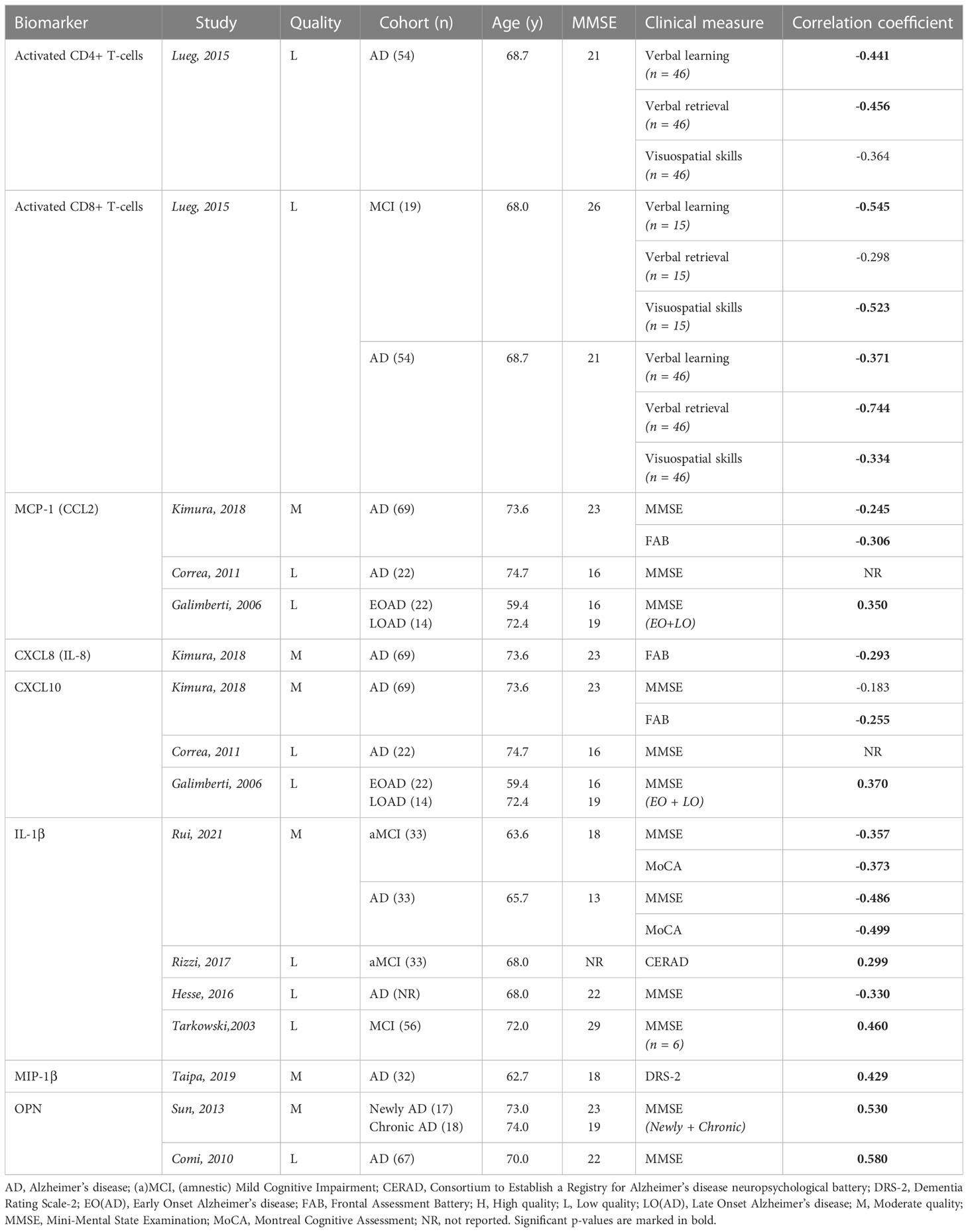
Table 2A Overview inflammatory biomarkers significantly associated with baseline clinical scores in AD publications.
3.1.3 MS literature
In total, 93 inflammatory biomarkers were evaluated for cross-sectional associations with clinical scores, including measures of general disability (EDSS), rate/severity of disability (Multiple Sclerosis Severity Score, MSSS; Age-Related Multiple Sclerosis Severity, ARMSS; Progression Index, PI; Multiple Sclerosis Disease Severity Scale, MS-DSS; Bayesian Risk Estimate for Multiple Sclerosis, BREMS) and fatigue (Fatigue Severity Scale, FSS) (54–59). The cohorts used for correlation analyses included between 16 and 244 subjects, but RR, SP and PP MS patients were often grouped together and considered as representative for the entire MS spectrum. Only 15 biomarkers demonstrated significant clinical relevance, with the majority describing a positive yet weak correlation with one or more of the clinical scores (Table 2B). More specifically, anti-neurofilament light chain (NF-L) antibodies, chitinase 3-like protein 1 (CHI3L1 or YKL-40), complement factor 3 (C3), glial fibrillary acidic protein (GFAP) and tumor necrosis factor alpha (TNF-α) were investigated by various independent studies, yet none of these biomarkers consistently showed significant results.
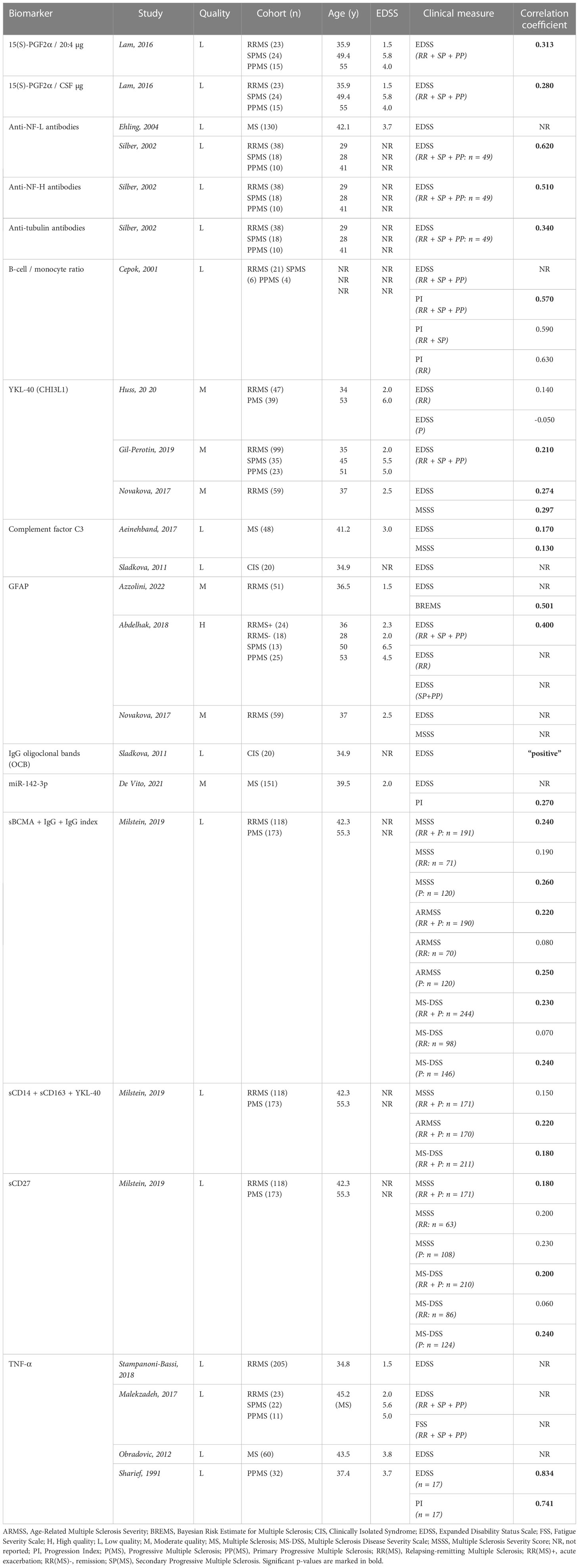
Table 2B Overview inflammatory biomarkers significantly associated with baseline clinical scores in MS publications.
3.2 Inflammatory biomarkers related to changes in clinical status
3.2.1 General overview
In total, 33 papers (AD/MS = 10/23) investigated the relationship between inflammatory biomarkers and disease progression, with the latter based on the longitudinal evolution of validated clinical scores similar to the ones used in the previous section. Statistical methodology mainly relied on correlation analyses and/or regression modelling for both disease entities, but we observed a notable distinction between the AD and MS field for the determination of disease progression. After all, in AD publications, the majority of clinical scores were reported as a change in score over a given follow-up period (e.g., δMMSE defined as the change in MMSE score from baseline to follow-up) with only a few reports of raw scores obtained throughout the study period, whereas for MS the opposite was true with the majority of clinical scores only being cross-sectionally collected at the time of follow-up (e.g., EDSS score at follow-up) and merely a few studies reporting an actual change in scores over time. In case of prediction analyses, a predefined threshold was used when determining the probability of reaching a certain grade of disability with the inflammatory biomarker as a predictor (e.g., the probability to reach an EDSS score of 4.0). Once again, both the MMSE and EDSS were the most frequently used scores for AD (MMSE: 80.0%) and MS (EDSS: 87.0%), respectively. A detailed overview of all the results from the individual papers can be found in the Supplementary Material (Table S5).
3.2.2 AD literature
AD literature described 41 distinct inflammatory biomarkers that were investigated for their relationship with disease progression, represented by various clinical scores including the MMSE, DRS-2, ADAS-Cog and Clinical Dementia Rating – Sum of Boxes (CDR-SB), as well as composites of memory and/or executive function (60). Cohorts consisted of patients with MCI, MCI due to AD (based on having an AD biomarker profile and/or clinical AD diagnosis at follow-up; MCI-AD), and dementia due to AD. Sample sizes varied from 32 up to 174 participants and follow-up periods ranged from 9 months to 5 years. Similar to what we observed in cross-sectional analyses, cohorts were mainly representative of the later stages of AD, characterized by lower MMSE scores and higher age. Twenty-two biomarkers were significantly associated with cognitive decline, many of which were also studied in the previous section describing cross-sectional relationships (Table 3A). Only MCP-1 (a crucial mediator of the innate immune response that has been implicated in the pathology of several neurodegenerative diseases (61)) showed promising results in more than one study (62, 63).
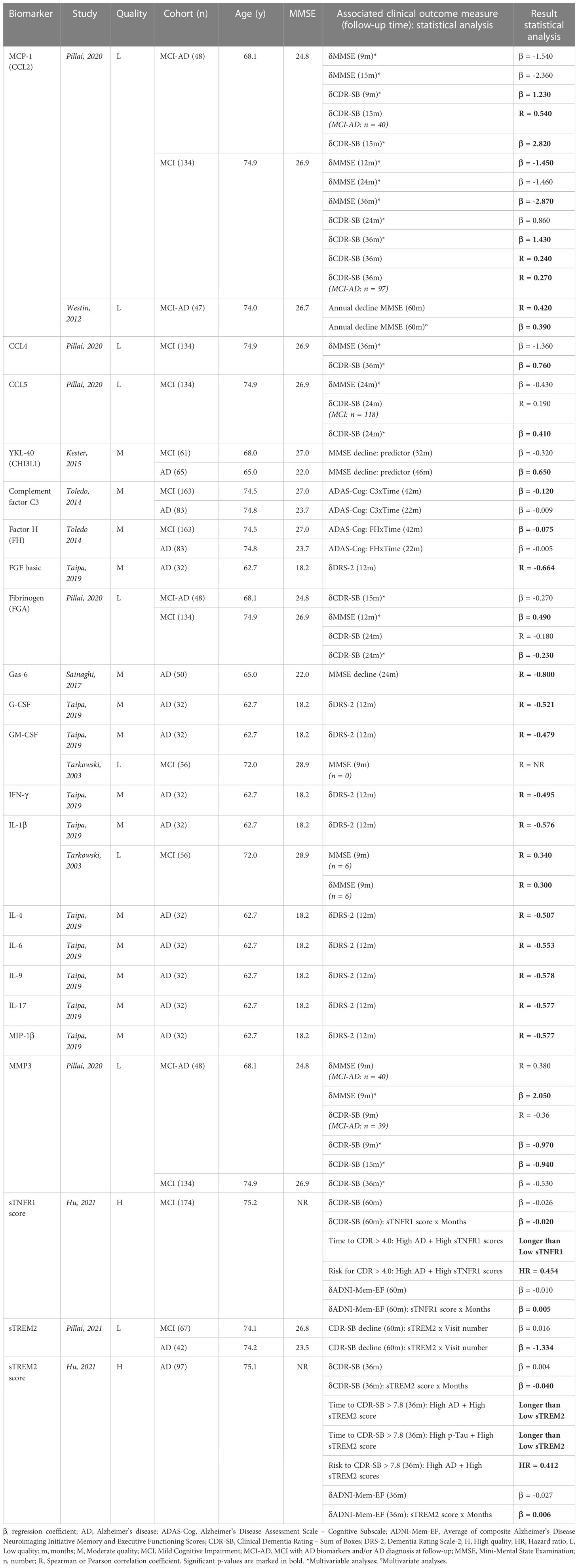
Table 3A Overview inflammatory biomarkers associated with longitudinal clinical measures in AD publications.
3.2.3 MS literature
MS papers presented longitudinal associations for 29 inflammatory biomarkers with functional disability measured by clinical scoring instruments such as the EDSS, MSSS, PI and the Multiple Sclerosis Functional Composite Scale (MSFC), but also with cognitive performance, based on the Brief Repeatable Battery of Neuropsychological Tests (BRB) or Symbol Digit Modalities Test (SDMT) (64–66). Cohorts were once again mainly representing MS in general and included individuals with CIS, CIS that converted to clinically definite MS during the study, RR and/or progressive MS, with a sample size ranging from 15 to 6,398 participants and a follow-up period between 1 and 10 years. The majority of biomarkers (n = 20) showed significant results, though most were evaluated in single studies. OCB, YKL-40 (a glycoprotein secreted by glial cells (67)) and IL-1β (a cytokine involved in the regulation of the innate immune response via microglia and astrocytes (68)) were significantly associated with longitudinal measures of clinical status based on multiple publications and may reflect underlying pathways driving disease progression (Table 3B).
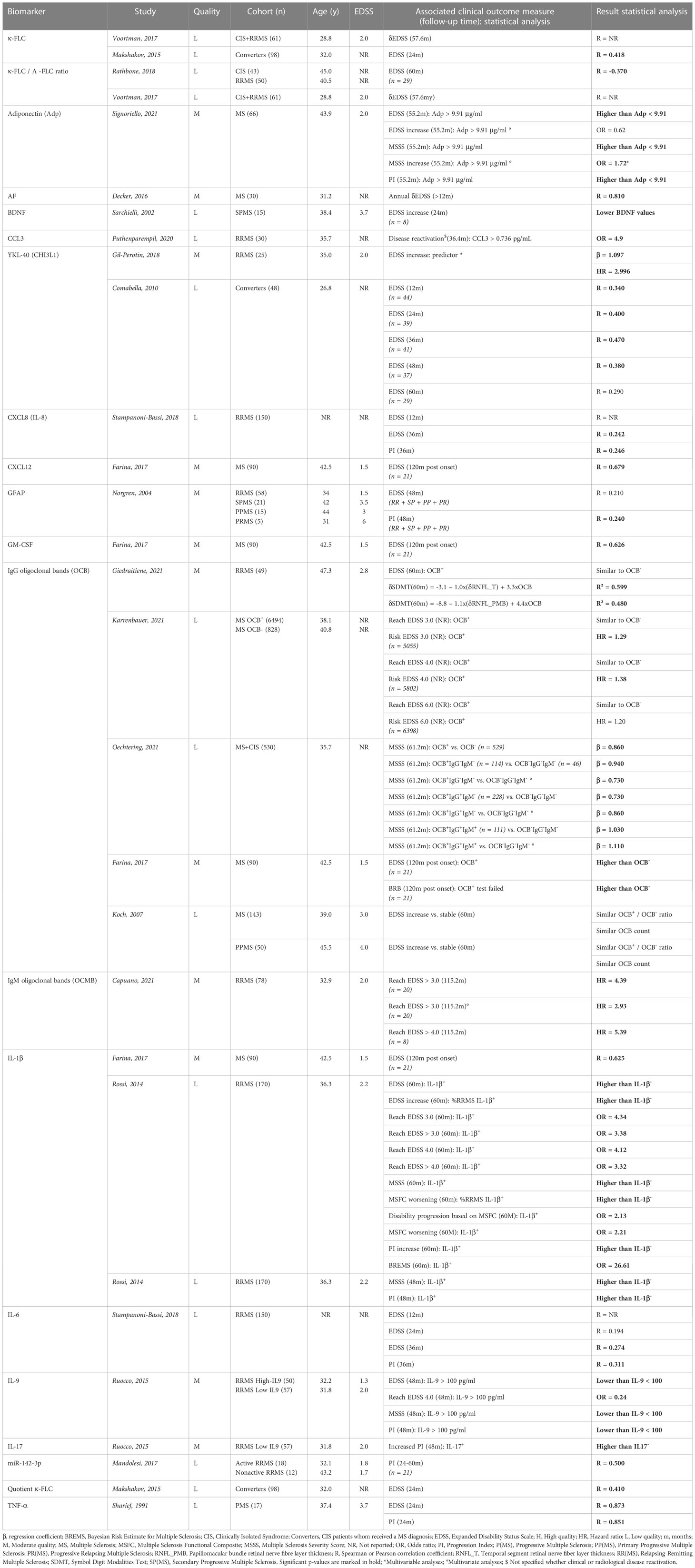
Table 3B Overview inflammatory biomarkers associated with longitudinal clinical measures in MS publications.
3.3 Inflammatory biomarkers related to transition to a more severe disease stage
3.3.1 General overview
Thirty (AD/MS = 8/22) of the 84 distinct publications investigated the clinical relevance of inflammatory biomarkers with regard to transitioning from one (possibly prodromal) disease stage to a more severe disease stage. The progression rate (i.e., the ratio of participants progressing over those remaining stable) varied from 35 to 68% in AD publications and from 28 to 93% in MS reports. Numerous statistical methods were used for the subsequent data analyses, including between-group comparisons, prediction (such as Cox proportional hazard and regression modelling) and discrimination analyses with receiver operating characteristic measures. A comprehensive overview of all statistical analyses and the corresponding results is provided in the Supplementary Material (Table S6).
3.3.2 AD literature
Cohorts consisted of MCI subjects, with a sample size varying from 31 to 174 individuals and an observation period ranging from 9 months to 5 years. Sixteen inflammatory biomarkers were investigated for their clinical relevance with regard to progression in the AD continuum; however, MCI cohorts showed characteristics suggestive for late-onset AD, based on a relatively older age and higher MMSE value. Eight biomarkers demonstrated significant relevance for patients that progressed from MCI to dementia due to AD, including MCP-1 (63), neuronal pentraxin 2 (NPTX2), OPN, YKL-40 and several TNF receptors (with related proteins that were also part of a composite score) (Table 4A). Only YKL-40 was assessed in two independent studies but conflicting results were reported (69, 70). Both of them showed similar cohort characteristics, but the study with the highest progression rate (i.e., higher number of MCI patients that developed dementia due to AD), what in itself could be due to the longer follow-up period (32.4 versus 24 months), favored YKL-40 as a predictive biomarker (70). Although OPN was only investigated in one study, it was the sole biomarker evaluated at two distinct time points within the same cohort. With this approach, authors could measure the intra-individual change of the CSF levels throughout the disease course. Results demonstrated increased levels in MCI patients that progressed to AD at follow-up, compared with those who remained stable (53).
3.3.3 MS literature
The vast majority of publications collected from the MS literature investigated the transition from CIS to clinical definite MS, with cohort sizes varying from 18 to 139 participants and a follow-up duration between 10 months and 10 years. The clinical relevance of 62 distinct inflammatory biomarkers was assessed. Half of the studies presented significant results, yet only ten biomarkers were evaluated in multiple studies (Table 4B). YKL-40 and CXCL13 (a chemokine implicated in B cell aggregates that develop in the inflamed meninges of PMS patients (71)) showed the most consistent results, with increased CSF levels at baseline in CIS subjects who received a MS diagnosis during follow-up, as compared with those who did not. These studies highlight the importance of both glial cells as well as the innate immune response in the early stages of the disease.
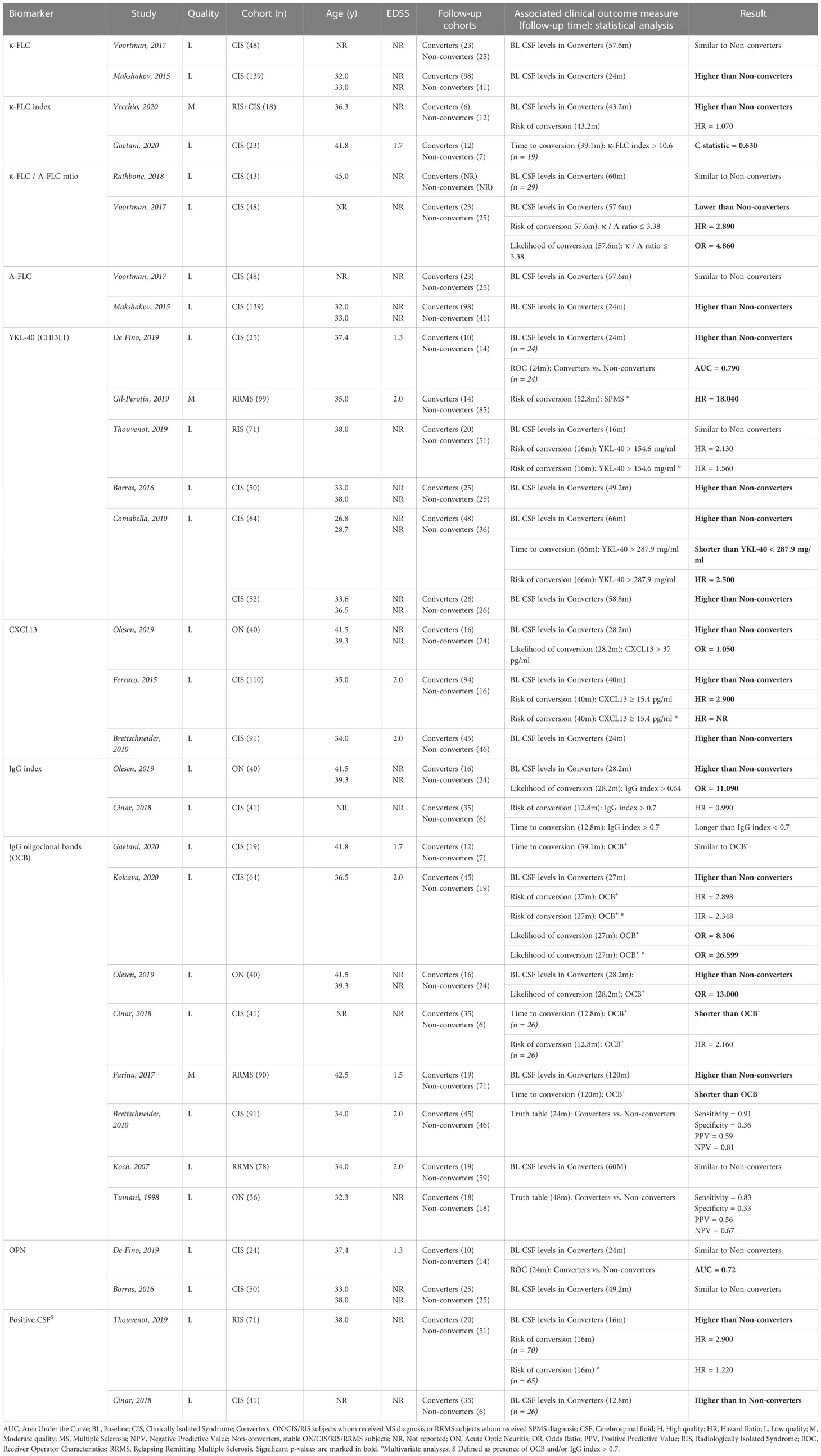
Table 4B Overview inflammatory biomarkers investigated in multiple studies and found to be associated with progression across the MS spectrum.
4 Discussion
With this review, we provide an overview of the inflammatory CSF biomarkers that are related to disease progression in AD and/or MS. YKL-40 was the only biomarker showing relevance for both disorders. In addition, alterations in MCP-1 and OPN were associated with clinical deterioration in patients with AD exclusively, while the same was true for IL-1β, TNF-α, CXCL13, GFAP and OCB in subjects affected by MS. These combined results point towards a prominent role for innate immune response in the CNS, in which microglia are considered to be key mediators (72), in the progression of both disorders. However, we must acknowledge that the studies retrieved by our search were highly heterogenous in study design and methodology, largely complicating the interpretation of their findings. The ‘Standard for Reporting of Diagnostic Accuracy’ initiative states: “To comprehend the results of diagnostic accuracy studies, readers must understand the study design, conduct, analysis, and results of such studies. That goal can be achieved only through complete transparency from authors” (73). This statement nicely summarizes the main difficulties encountered when reviewing the literature. In the following paragraphs, we sum up the most important findings for both disorders, as well as some recommendations that, in our vision, may contribute to an enhanced clinical relevance and improved quality of result reporting when incorporated in future biomarker studies in this field (Table 5).
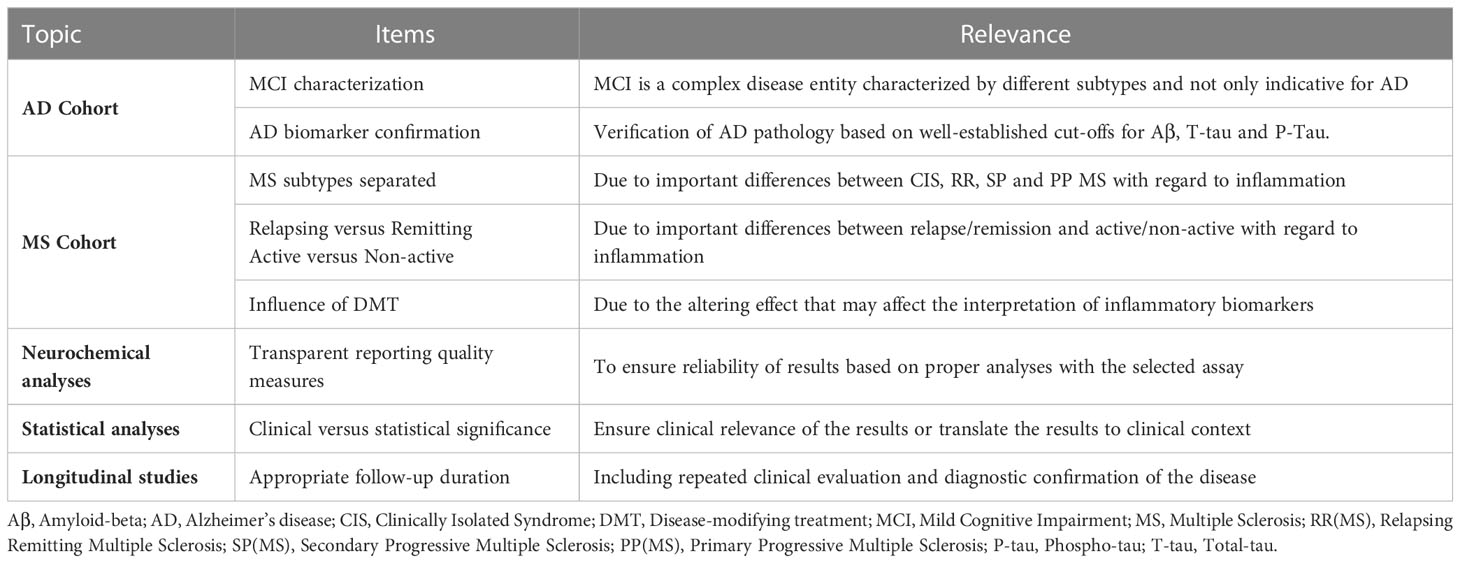
Table 5 Recommendations for future research, based on the 'Standard for Reporting of Diagnostic Accuracy’ initiative for diagnostic accuracy tests.
Is neuroinflammation beneficial or detrimental in AD? To answer this question, we need to take a closer look at the complex biology and temporal behavior of microglia in this disorder. These residential CNS macrophages are responsible for maintaining local homeostasis and can elicit an inflammatory response against potentially harmful agents, both endo- and exogenous. Microglia are highly dynamic cells that were historically described as being activated into a pro- (M1) or anti-inflammatory phenotype (M2). In fact, these cells exists in various states depending on the specific context (age, spatial location, environment) and are active in both health and disease. In line with this, neuroinflammation cannot be generalized as being either detrimental or beneficial, but will, also depending on the context, exert adaptive or maladaptive effects (20, 74). A recent PET imaging study described increased TSPO expression in MCI and dementia due to AD patients, as compared with healthy controls. However, at follow-up, a decreased expression in MCI and increased expression in dementia due to AD was observed. These results prompted the two-peak hypothesis, stating that across the AD spectrum microglia may undergo two major morphological changes, related with changes in microglia function (75). This hypothesis may explain the distinction in microglia states between MCI and dementia due to AD observed at follow-up, despite both of them showing increased TSPO expression when compared with healthy controls. However, increased TSPO expression in the diseased brain is considered a neuroinflammatory marker, not a microglia specific marker (19). Furthermore, equating microglia morphology with function is considered wrong, as different morphologies can execute similar functions (20). We found promising results for YKL-40, MCP-1 and OPN in reflecting disease progression, particularly at the time of transition from the MCI to the dementia stage in the AD continuum (53, 63, 70). These proteins are mainly produced by microglia (51, 76, 77), and have shown increased expression near amyloid plaques or in the cytoplasm of AD brains (78, 79). MCP-1 (which is the most potent activator of the CCR2 receptor) and OPN have been implicated in several neurodegenerative diseases through their respective role as chemoattractant and regulator of microglia and DAMs (77, 80). In vivo studies using AD mouse models suggest that the MCP-1/CCR2 axis has varying effects on AD pathogenesis across the stage of the disease. In the early stages, it may enhance accumulation of microglia and clearance of Aβ, whereas in the late stage, it may cause exaggerated inflammatory responses and the development of insoluble Aβ within microglia (81). Perivascular macrophages (PVMs) are the main source of increased OPN in AD mouse models and postmortem AD brains, and seem to regulate phagocytic activity of microglia. Moreover, the notion that the timing of microglial phagocytosis across the disease stages may be crucial to determine whether the outcome will be beneficial or harmful has been lifted forward recently (82). As the CNS immune response seemingly changes depending on the stage of the disease, this may in part explain the inconsistency we found in our results as biomarker findings would then highly depend on the selected cohort and microglia state at the time of sampling. Moreover, worsening of AD pathology may not necessarily follow a linear course (83), further complicating the interpretation of biomarker results. Nonetheless, together these observations point towards a complex and dynamic role of the innate immune response and microglia across the AD spectrum and motivate a continued effort in studying the earliest stages of AD.
In MS, there seems to be a notable distinction between the types of inflammation predominantly underlying the relapsing versus progressive phase of the disease. Whereas the former is mostly driven by recurrent invasion of peripheral immune cells into the CNS with formation of classic active demyelinating lesions, the latter has been associated with seemingly more subtle yet chronically perseverating and compartmentalized immune-related phenomena, such as (a) meningeal collections of lymphoid cells, some of which are organized into follicle-like structures, with underlying subpial cortical demyelination and atrophy, (b) smoldering lesions characterized by a border zone of iron-rich MIMS and (c) widespread microglial inflammation and astrogliosis throughout the NAWM, sometimes also referred to as ‘dirty white matter’ (84, 85). Smoldering lesions can be visualized in vivo by susceptibility-weighted MRI as paramagnetic rim lesions and were found to be more abundant in patients with progressive MS (86), correlating with concurrent and forthcoming clinical disability and brain volume loss (87–90). In addition, such lesions have been linked to (presumably intracellular) sodium accumulation and neurofilament release (91, 92), which are considered as very early pathobiological features of the neurodegenerative cascade in MS (93). Recent data have demonstrated that ongoing inflammation in smoldering lesions is mediated by a microglial-astrocytic axis in which complement component C1q seems to be crucial for MIMS activation (26). In general, T- and B-cells do not seem to be directly causing neuronal damage in MS but interact with local microglia, hereby mediating tissue injury via a complex cascade that is still not fully understood (94, 95). At the histological level, microglia account for approximately 40% of the phagocytic cell population in classic active lesions (96), while slowly expanding chronic lesions demonstrate a preferential accumulation of MIMS at the lesion’s edge (97). In fact, it is hypothesized that “true MS” is best represented by such chronic inflammatory state, since relapses (representative of focal inflammation) do not predict disease outcome in untreated patients (98), and confirmed disability worsening occurring independently from relapse and/or MRI activity has frequently been described in cohorts of RR MS (99–101). Microglia seem to be characterized by a similar expression pattern in active and chronic lesions (95), suggesting a parallel function in both types. Since there are less active lesions during progressive MS, microglia become more prominent compared with peripheral immune cell infiltration (102), as the disease evolves. Beside the association between increased TSPO expression in the NAWM and an enhanced likelihood of experiencing progression independent of relapse activity, TSPO is also associated with higher EDSS and MSSS scores and found to be more common in SP compared with RR MS (30, 103). Interestingly, recent work using this technique showed that TSPO expression was most intense in the periventricular region, suggesting a diffusion of inflammatory CSF-derived factors into surrounding tissues (104), while the thalamic activation pattern appeared to be more sensitive than conventional grey matter atrophy measures in predicting future clinical disability worsening (105). These findings, together with our results, support the hypothesis of microglia driving disease progression across the entire MS spectrum.
Due to the role microglia seem to be playing in disease progression in AD and MS, it is not surprising that they are considered as interesting targets for future treatments. One possible approach could be to change the microglia state in one that clears debris and drives regeneration. However, as already mentioned, microglia are highly versatile depending on the specific context and it is likely that the environment, not the state, is the determining factor in deciding how microglia act in disease (20)Nonetheless, attempts are being made to alter the innate immune response in AD and MS through microglia. Bruton’s tyrosine kinase (BTK) is a non-receptor cytosolic signal transducing enzyme predominantly expressed by B-lymphocytes and myeloid cells. Its downstream signaling pathways are involved in multiple processes, including cellular growth, metabolism, proliferation and differentiation (106). Increased activity patterns have been observed post-mortem in AD brain and focal lesions of patients with progressive MS (107, 108). BTK inhibition has been found to reduce phagocytic activity of microglia in preclinical AD models but did not have a major impact on cytokine release (107). Further research is required to truly determine its potential within the AD continuum. On the other hand, BTK inhibition is a booming business in the MS field with several oral agents currently tested in clinical trials (109). For example, a recent phase IIb study has demonstrated a significantly decreased lesional accumulation on conventional brain MRI after 12 weeks of treatment with tolebrutinib, a selective BTK inhibitor for which evidence of BBB penetration exists in animals and humans, as compared with placebo (110). Multiple phase III trials with this agent are ongoing for the moment in relapsing, non-active SP and PP MS, and their results will be eagerly anticipated by the scientific community. Interestingly, glatiramere acetate and dimethyl fumarate, which are commonly used as DMT for MS, have shown therapeutic promise for AD and other models of neurocognitive decline, at least partially attributed to effects on CNS microglia (111–114).
We were unable to perform a meta-analysis due to the heterogeneous nature of the included studies. The observed diversity in AD and MS study designs is in agreement with other reviews (15, 115, 116) and long known to be questionable for evaluating candidate biomarkers. Cohort discrepancies were one of the major limiting factors for the interpretability and reproducibility of the findings yielded by our search. In AD studies, we observed a high variety in age and disease stage of participants at the time of inclusion. MCI cohorts, for example, were rarely described by subtype (e.g., amnestic versus non-amnestic, single- versus multiple-domain, etc.), by follow-up diagnosis (or even lacking follow-up) or by verification of AD pathology via CSF biomarkers (117). In MS papers, individuals were clearly subdivided based on established diagnostic criteria but eventually often grouped together as “general MS” for analytical purposes. Inflammation is highly fluctuating and/or situational in MS, even in the RR group alone as, for instance, increased levels of GFAP were found in a cohort of MS patients (compared with controls) but once you start to depict the subgroups you may find that the results are due to an increase at relapse in RR MS, as compared with stable or even progressive MS (118). The effects of DMT (119) may also influence the interpretation of CSF inflammatory biomarkers with regards to pathophysiological mechanisms such as relation to disease progression. We generally observed a lack of longitudinal studies with appropriate time of observation and periodic clinical evaluation, as well as diagnostic confirmation after extended follow-up in both AD and MS papers. Moreover, the majority of included studies did not report on the analytical quality, including the intra- and inter-assay coefficient of variance, upper and lower limit of quantification and detectability rate. Similarly, statistical analyses generally lacked reports of model assumptions (e.g., visual inspection of QQ-plots and residuals for linear regression models) and approaches for regression analyses were highly heterogenous. Some biomarkers were based on computations that showed statistically significant relevance concerning disease progression but might be too complex for the routine clinical setting. It will be important to make a distinction between statistical and clinical significance when looking for clinically relevant biomarkers and to translate outcomes into interpretable results for clinical use. The abovementioned aspects contributed to the limited quality we observed in a substantial number of papers based on our quality assessment tool (see Table S3). Additionally, there is also an important caveat with regard to our own approach for this systematic review. Disease progression was defined only by validated clinical scores, not by other measures such as imaging parameters (e.g., MRI brain volume changes) which will have influenced the selection of papers. However, we wanted to ensure the findings from this manuscript were relevant for the clinical practice, therefore we only included those measures that were examined in this setting. The EDSS score was confirmed as the most frequently used measure of clinical disability (appearing in 96.6% of the MS papers) but is also known to be a rather insensitive outcome, especially in older patients with a higher grade of disability (e.g., on average merely showing an increase of 1 point over 10 years in the natural history of MS) (120), which may be a reason why most of the biomarkers failed to demonstrate clinical relevance.
5 Conclusion
YKL-40 was identified as the only CSF-based biomarker related to clinical disease progression in both AD and MS. MCP-1 and OPN showed similar relevance in patients with AD, while the same accounts for IL-1β, TNF-α, CXCL13, GFAP and OCB in subjects affected by MS. Our findings support the increasingly recognized role of the innate immune system, including the microglia, in the pathology of both disorders. However, the retrieved biomarker studies for AD and MS were generally heterogeneous in nature, challenging the interpretational reliability of their results. We have framed some suggestions to tackle this problem in future research (see Table 5), focusing on better cohort characterization (e.g., the use of highly qualitative cohorts with biomarker confirmation for AD studies, particularly for the MCI stage, and clear separation of MS subtypes, as well as a distinct grouping of remitting and relapsing MS patients), transparency in neurochemical quality measures, interpretable statistical analyses and longitudinal studies with repeated clinical assessments and diagnostic confirmation during follow-up.
Author contributions
JT, SE, MB and MD contributed to conception and design of the study. JT performed the systematic search and organized the database. JT and MB performed the quality assessment and analyses. JT wrote the first draft of the manuscript. MB and MD wrote sections of the manuscript. MB, SE and MD supervised the project. All authors contributed to the article and approved the submitted version.
Acknowledgments
We would like to thank Odile Chevalley, Carl Verduyn and Melissa Wittens for assisting us throughout this review.
Conflict of interest
The authors declare that the research was conducted in the absence of any commercial or financial relationships that could be construed as a potential conflict of interest.
Publisher’s note
All claims expressed in this article are solely those of the authors and do not necessarily represent those of their affiliated organizations, or those of the publisher, the editors and the reviewers. Any product that may be evaluated in this article, or claim that may be made by its manufacturer, is not guaranteed or endorsed by the publisher.
Supplementary material
The Supplementary Material for this article can be found online at: https://www.frontiersin.org/articles/10.3389/fimmu.2023.1162340/full#supplementary-material
References
1. Lane CA, Hardy J, Schott JM. Alzheimer’s disease. Eur J Neurol (2018) 25(1):59–70. doi: 10.1111/ene.13439
2. Dobson R, Giovannoni G. Multiple sclerosis - a review. Eur J Neurol (2019) 26(1):27–40. doi: 10.1111/ene.13819
3. Chiaravalloti ND, Deluca J. Cognitive impairment in multiple sclerosis. Lancet Neurology (2008) 7:1139–51. doi: 10.1016/S1474-4422(08)70259-X
4. Deloire MSA, Salort E, Bonnet M, Arimone Y, Boudineau M, Amieva H, et al. Cognitive impairment as marker of diffuse brain abnormalities in early relapsing remitting multiple sclerosis. J Neurol Neurosurg Psychiatry (2005) 76:519–26. doi: 10.1136/jnnp.2004.045872
5. Compston A, Coles A. Multiple sclerosis. Lancet (2008) 372(9648):1502–17. doi: 10.1016/S0140-6736(08)61620-7
6. Timmers M, Tesseur I, Bogert J, Zetterberg H, Blennow K, Börjesson-Hanson A, et al. Relevance of the interplay between amyloid and tau for cognitive impairment in early alzheimer’s disease. Neurobiol Aging (2019) 79:131–41. doi: 10.1016/j.neurobiolaging.2019.03.016
7. Lassmann H. Mechanisms of neurodegeneration shared between multiple sclerosis and alzheimer’s disease. J Neural Transm (2011) 118:747–52. doi: 10.1007/s00702-011-0607-8
8. Nichols E, Steinmetz JD, Vollset SE, Fukutaki K, Chalek J, Abd-Allah F, et al. Estimation of the global prevalence of dementia in 2019 and forecasted prevalence in 2050: an analysis for the global burden of disease study 2019. Lancet Public Health (2022) 7(2):e105–25. doi: 10.1016/S2468-2667(21)00249-8
9. Walton C, King R, Rechtman L, Kaye W, Leray E, Marrie RA, et al. Rising prevalence of multiple sclerosis worldwide: insights from the atlas of MS, third edition. Mult Scler (2020) 26(14):1816–21. doi: 10.1177/1352458520970841
10. Luczynski P, Laule C, Hsiung GYR, Moore GRW, Tremlett H. Coexistence of multiple sclerosis and alzheimer’s disease: a review. Mult Scler Relat Disord (2019) 27:232–8. doi: 10.1016/j.msard.2018.10.109
11. Huang J, Su B, Karhunen V, Gill D, Zuber V, Ahola-Olli A, et al. Inflammatory diseases, inflammatory biomarkers, and Alzheimer disease: an observational analysis and mendelian randomization. Neurology (2023) 100(6):568–81. doi: 10.1212/WNL.0000000000201489
12. Mahmoudi E, Sadaghiyani S, Lin P, Kamdar N, Norcott A, Peterson MD, et al. Diagnosis of alzheimer’s disease and related dementia among people with multiple sclerosis: Large cohort study, USA. Mult Scler Relat Disord (2022) 57:103351. doi: 10.1016/j.msard.2021.103351
13. Goldacre MJ, Wotton CJ. Associations between specific autoimmune diseases and subsequent dementia: retrospective record-linkage cohort study, UK. J Epidemiol Community Health (2017) 71(6):576–83. doi: 10.1136/jech-2016-207809
14. Califf RM. Biomarker definitions and their applications. Exp Biol Med (2018) 243(3):213–21. doi: 10.1177/1535370217750088
15. Ziemssen T, Akgün K, Brück W. Molecular biomarkers in multiple sclerosis. J Neuroinflamm (2019) 16(1):1–11. doi: 10.1186/s12974-019-1674-2
16. Niemantsverdriet E, Ottoy J, Somers C, De Roeck E, Struyfs H, Soetewey F, et al. The cerebrospinal fluid Aβ1-42/Aβ1-40 ratio improves concordance with amyloid-PET for diagnosing alzheimer’s disease in a clinical setting. J Alzheimers Dis (2017) 60(2):561–76. doi: 10.3233/JAD-170327
17. Jack CR, Knopman DS, Jagust WJ, Petersen RC, Weiner MW, Aisen PS, et al. Tracking pathophysiological processes in alzheimer’s disease: an updated hypothetical model of dynamic biomarkers. Lancet Neurol (2013) 12(2):207–16. doi: 10.1016/S1474-4422(12)70291-0
18. Sperling RA, Aisen PS, Beckett LA, Bennett DA, Craft S, Fagan AM, et al. Toward defining the preclinical stages of alzheimer’s disease: recommendations from the national institute on aging-alzheimer’s association workgroups on diagnostic guidelines for alzheimer’s disease. Alzheimers Dement (2011) 7(3):280–92. doi: 10.1016/j.jalz.2011.03.003
19. Kreisl WC, Kim MJ, Coughlin JM, Henter ID, Owen DR, Innis RB. PET imaging of neuroinflammation in neurological disorders. Lancet Neurol (2020) 19(11):940–50. doi: 10.1016/S1474-4422(20)30346-X
20. Paolicelli RC, Sierra A, Stevens B, Tremblay ME, Aguzzi A, Ajami B, et al. Microglia states and nomenclature: a field at its crossroads. Neuron (2022) 110(21):3458–83. doi: 10.1016/j.neuron.2022.10.020
21. Kinney JW, Bemiller SM, Murtishaw AS, Leisgang AM, Salazar AM, Lamb BT. Inflammation as a central mechanism in alzheimer’s disease. Alzheimer’s Dementia: Trans Res Clin Interventions (2018) 4(1):575–90. doi: 10.1016/j.trci.2018.06.014
22. Gao C, Shen X, Tan Y, Chen S. Pathogenesis, therapeutic strategies and biomarker development based on “omics” analysis related to microglia in alzheimer’s disease. J Neuroinflamm (2022) 19(1):1–23. doi: 10.1186/s12974-022-02580-1
23. Frost GR, Jonas LA, Li YM. Friend, foe or both? immune activity in alzheimer’s disease. Front Aging Neurosci (2019) 11:337. doi: 10.3389/fnagi.2019.00337
24. Prokop S, Lee VMY, Trojanowski JQ. Neuroimmune interactions in alzheimer’s disease–new frontier with old challenges? Prog Mol Biol Transl Sci (2019) 168:183–201. doi: 10.1016/bs.pmbts.2019.10.002
25. Bellaver B, Ferrari-Souza JP, da Ros LU, Carter SF, Rodriguez-Vieitez E, Nordberg A, et al. Astrocyte biomarkers in Alzheimer disease: a systematic review and meta-analysis. Neurology (2021) 96(24):E2944–55. doi: 10.1212/WNL.0000000000012109
26. Absinta M, Maric D, Gharagozloo M, Garton T, Smith MD, Jin J, et al. A lymphocyte-microglia-astrocyte axis in chronic active multiple sclerosis. Nature (2021) 597(7878):709–14. doi: 10.1038/s41586-021-03892-7
27. Dendrou CA, Fugger L, Friese MA. Immunopathology of multiple sclerosis. Nat Rev Immunol (2015) 15:545–58. doi: 10.1038/nri3871
28. Correale J, Gaitán MI, Ysrraelit MC, Fiol MP. Progressive multiple sclerosis: from pathogenic mechanisms to treatment. Brain (2017) 140(3):527–46. doi: 10.1093/brain/aww258
29. Faissner S, Plemel JR, Gold R, Yong VW. Progressive multiple sclerosis: from pathophysiology to therapeutic strategies. Nat Rev Drug Discov (2019) 18(12):905–22. doi: 10.1038/s41573-019-0035-2
30. Sucksdorff M, Matilainen M, Tuisku J, Polvinen E, Vuorimaa A, Rokka J, et al. Brain TSPO-PET predicts later disease progression independent of relapses in multiple sclerosis. Brain (2020) 143(11):3318–30. doi: 10.1093/brain/awaa275
31. Patsopoulos NA, Baranzini SE, Santaniello A, Shoostari P, Cotsapas C, Wong G, et al. Multiple sclerosis genomic map implicates peripheral immune cells and microglia in susceptibility. Science (2019) 365(6460):eaav7188. doi: 10.1126/science.aav7188
32. McNamara NB, Munro DAD, Bestard-Cuche N, Uyeda A, Bogie JFJ, Hoffmann A, et al. Microglia regulate central nervous system myelin growth and integrity. Nature (2022) 17:1–10. doi: 10.1038/s41586-022-05534-y
33. Allen CM, Mowry EM, Tintoré M, Dphil NE. Prognostication and contemporary management of clinically isolated syndrome. Neurol Neurosurg Psychiatry (2021) 92:391–7. doi: 10.1136/jnnp-2020-323087
34. Makhani N, Tremlett H. The multiple sclerosis prodrome. Nat Rev Neurol (2021) 17(8):515–21. doi: 10.1038/s41582-021-00519-3
35. Disanto G, Adiutori R, Dobson R, Martinelli V, Costa GD, Runia T, et al. Serum neurofilament light chain levels are increased in patients with a clinically isolated syndrome. J Neurol Neurosurg Psychiatry (2016) 87(2):126–9. doi: 10.1136/jnnp-2014-309690
36. Bjornevik K, Munger KL, Cortese M, Barro C, Healy BC, Niebuhr DW, et al. Serum neurofilament light chain levels in patients with presymptomatic multiple sclerosis. JAMA Neurol (2020) 77(1):58–64. doi: 10.1001/jamaneurol.2019.3238
37. Hosseiny M, Newsome SD, Yousem DM. Radiologically isolated syndrome: a review for neuroradiologists. Am J Neuroradiol (2020) 41(9):1542–9. doi: 10.3174/ajnr.A6649
38. Thompson AJ, Banwell BL, Barkhof F, Carroll WM, Coetzee T, Comi G, et al. Diagnosis of multiple sclerosis: 2017 revisions of the McDonald criteria. Lancet Neurol (2018) 17(2):162–73. doi: 10.1016/S1474-4422(17)30470-2
39. van Bokhoven P, de Wilde A, Vermunt L, Leferink PS, Heetveld S, Cummings J, et al. The alzheimer’s disease drug development landscape. Alzheimers Res Ther (2021) 13(1):1–9. doi: 10.1186/s13195-021-00927-z
40. Piehl F. Current and emerging disease-modulatory therapies and treatment targets for multiple sclerosis. J Intern Med (2021) 289(6):771–91. doi: 10.1111/joim.13215
41. Samson D, Schoelles KM. Chapter 2: medical tests guidance (2) developing the topic and structuring systematic reviews of medical tests: utility of PICOTS, analytic frameworks, decision trees, and other frameworks. J Gen Intern Med (2012) 27(SUPPL.1):11–9. doi: 10.1007/s11606-012-2007-7
42. Page MJ, Mckenzie JE, Bossuyt PM, Boutron I, Hoffmann TC, Mulrow CD, et al. The PRISMA 2020 statement: an updated guideline for reporting systematic reviews. BMJ (2021) 372:n71. doi: 10.1136/bmj.n71
43. Folstein MF, Folstein SE, McHugh PR. “Mini-mental state”: a practical method for grading the cognitive state of patients for the clinician. J Psychiatr Res (1975) 12(3):189–98. doi: 10.1016/0022-3956(75)90026-6
44. Kurtzke JF. Rating neurologic impairment in multiple sclerosis: an expanded disability status scale (EDSS). Neurology (1983) 33(11):1444–52. doi: 10.1212/WNL.33.11.1444
45. Berg L. Clinical dementia rating. Br J Psychiatry (1984) 145(3):339–9. doi: 10.1192/S0007125000118082
46. Rosen WG, Mohs RC, Davis KL. A new rating scale for alzheimer’s disease. Am J Psychiatry (1984) 141(11):1356–64. doi: 10.1176/ajp.141.11.1356
47. Griffiths S, Sherman EMS, Strauss E. Dementia rating scale-2. In: Encyclopedia of clinical neuropsychology (2011) (New York: Springer New York). p. 810–1.
48. Dubois B, Slachevsky A, Litvan I, Pillon B. The FAB: a frontal assessment battery at bedside. Neurology (2000) 55(11):1621–6. doi: 10.1212/WNL.55.11.1621
49. Morris JC, Heyman A, Mohs RC, Hughes JP, van Belle G, Fillenbaum G, et al. The consortium to establish a registry for alzheimer’s disease (CERAD). part i. clinical and neuropsychological assesment of alzheimer’s disease. Neurology (1989) 39(9):1159–9. doi: 10.1212/wnl.39.9.1159
50. Nasreddine ZS, Phillips NA, Bédirian V, Charbonneau S, Whitehead V, Collin I, et al. The Montreal cognitive assessment, MoCA: a brief screening tool for mild cognitive impairment. J Am Geriatr Soc (2005) 53(4):695–9. doi: 10.1111/j.1532-5415.2005.53221.x
51. Yu H, Liu X, Zhong Y. The effect of osteopontin on microglia. BioMed Res Int (2017) 2017:1879437. doi: 10.1155/2017/1879437
52. Comi C, Carecchio M, Chiocchetti A, Galimberti D. Osteopontin is increased in the cerebrospinal fluid of patients with alzheimer’s disease and its levels correlate with cognitive decline. J Alzheimer’s Dis (2010) 19:1143–8. doi: 10.3233/JAD-2010-1309
53. Sun Y, Yin XS, Guo H, Han RK, Dong He R, Chi LJ. Elevated osteopontin levels in mild cognitive impairment and alzheimer’s disease. Mediators Inflamm (2013) 2013:615745. doi: 10.1155/2013/615745
54. Roxburgh RHSR, Seaman SR, Masterman T, Hensiek AE, Sawcer SJ, Vukusic S, et al. Multiple sclerosis severity score. Neurology (2005) 64(7):1144–51. doi: 10.1212/01.WNL.0000156155.19270.F8
55. Manouchehrinia A, Westerlind H, Kingwell E, Zhu F, Carruthers R, Ramanujam R, et al. Age related multiple sclerosis severity score: disability ranked by age. Mult Scler (2017) 23(14):1938–46. doi: 10.1177/1352458517690618
56. Poser S, Bauer HJ, Poser W. Prognosis of multiple sclerosis. results from an epidemiological area in Germany. Acta Neurol Scand (1982) 65(4):347–54. doi: 10.1111/j.1600-0404.1982.tb03091.x
57. Tackenberg B, Ramanujam R, Institute K, Trygve Holmøy S, Marie Weideman A, Barbour C, et al. New multiple sclerosis disease severity scale predicts future accumulation of disability. Front Neurol (2017) 8:598. doi: 10.3389/fneur.2017.00598
58. Bergamaschi R, Quaglini S, Trojano M, Amato MP, Tavazzi E, Paolicelli D, et al. Early prediction of the long term evolution of multiple sclerosis: the Bayesian risk estimate for multiple sclerosis (BREMS) score. J Neurol Neurosurg Psychiatry (2007) 78(7):757–9. doi: 10.1136/jnnp.2006.107052
59. Krupp LB, Larocca NG, Muir Nash J, Steinberg AD. The fatigue severity scale: application to patients with multiple sclerosis and systemic lupus erythematosus. Arch Neurol (1989) 46(10):1121–3. doi: 10.1001/archneur.1989.00520460115022
60. Hughes CP, Berg L, Danziger WL, Coben LA, Martin RL. A new clinical scale for the staging of dementia. Br J Psychiatry (1982) 140(6):566–72. doi: 10.1192/bjp.140.6.566
61. Sokolova A, Hill MD, Rahimi F, Warden LA, Halliday GM, Shepherd CE. Monocyte chemoattractant protein-1 plays a dominant role in the chronic inflammation observed in alzheimer’s disease. Brain Pathol (2009) 19(3):392–8. doi: 10.1111/j.1750-3639.2008.00188.x
62. Pillai JA, Bena J, Bebek G, Bekris LM, Bonner-Jackson A, Kou L, et al. Inflammatory pathway analytes predicting rapid cognitive decline in MCI stage of alzheimer’s disease. Ann Clin Transl Neurol (2020) 7(7):1225–39. doi: 10.1002/acn3.51109
63. Westin K, Buchhave P, Nielsen H, Minthon L, Janciauskiene S, Hansson O. CCL2 is associated with a faster rate of cognitive decline during early stages of alzheimer’s disease. PloS One (2012) 7(1):e30525. doi: 10.1371/journal.pone.0030525
64. Fischer JS, Rudick RA, Cutter GR, Reingold SC. The multiple sclerosis functional composite measure (MSFC): an integrated approach to MS clinical outcome assessment. Multiple Sclerosis J (1999) 5(4):244–50. doi: 10.1177/135245859900500409
65. Bever CT, Grattan L, Panitch HS, Johnson KP. The brief repeatable battery of neuropsychological tests for multiple sclerosis: a preliminary serial study. Multiple Sclerosis (1995) 1(3):165–9. doi: 10.1177/135245859500100306
66. Parmenter BA, Weinstock-Guttman B, Garg N, Munschauer F, Benedict RHB. Screening for cognitive impairment in multiple sclerosis using the symbol digit modalities test. Mult Scler (2007) 13(1):52–7. doi: 10.1177/1352458506070750
67. Dichev V, Kazakova M, Sarafian V. YKL-40 and neuron-specific enolase in neurodegeneration and neuroinflammation. Rev Neurosci (2020) 31(5):539–53. doi: 10.1515/revneuro-2019-0100
68. Mendiola AS, Cardona AE. The IL-1β phenomena in neuroinflammatory diseases. J Neural Transm (2018) 125(5):781–95. doi: 10.1007/s00702-017-1732-9
69. Swanson A, Willette AA, Initia ADN. Neuronal pentraxin 2 predicts medial temporal atrophy and memory decline across the alzheimer’s disease spectrum. Brain Behav Immun (2016) 58:201–8. doi: 10.1016/j.bbi.2016.07.148
70. Kester MI, Teunissen CE, Sutphen C, Herries EM, Ladenson JH, Xiong C, et al. Cerebrospinal fluid VILIP-1 and YKL-40, candidate biomarkers to diagnose, predict and monitor alzheimer’s disease in a memory clinic cohort. Alzheimers Res Ther (2015) 7(1):59. doi: 10.1186/s13195-015-0142-1
71. Huber AK, Irani DN. Targeting CXCL13 during neuroinflammation. Adv Neuroimmune Biol (2015) 6(1):1. doi: 10.3233/NIB-150101
72. Lehnardt S. Innate immunity and neuroinflammation in the CNS: the role of microglia in toll-like receptor-mediated neuronal injury. Glia (2010) 58(3):253–63. doi: 10.1002/glia.20928
73. Bossuyt PM, Reitsma JB, Bruns DE, Gatsonis CA, Glasziou PP, Irwig LM, et al. Towards complete and accurate reporting of studies of diagnostic accuracy: the STARD initiative. BMJ (2003) 326(7379):41–4. doi: 10.1136/bmj.326.7379.41
74. Colonna M, Butovsky O. Microglia function in the central nervous system during health and neurodegeneration. Annu Rev Immunol (2017) 35:441–68. doi: 10.1146/annurev-immunol-051116-052358
75. Fan Z, Brooks DJ, Okello A, Edison P. An early and late peak in microglial activation in alzheimer’s disease trajectory. Brain (2017) 140(3):792–803. doi: 10.1093/brain/aww34
76. Rosén C, Hansson O, Blennow K, Zetterberg H. Fluid biomarkers in alzheimer’s disease - current concepts. Mol Neurodegener (2013) 8(1):1–11. doi: 10.1186/1750-1326-8-20
77. Bose S, Cho J. Role of chemokine CCL2 and its receptor CCR2 in neurodegenerative diseases. Arch Pharm Res (2013) 36(9):1039–50. doi: 10.1007/s12272-013-0161-z
78. Molinuevo JL, Ayton S, Batrla R, Bednar MM, Bittner T, Cummings J, et al. Current state of alzheimer’s fluid biomarkers. Acta Neuropathol (2018) 136(6):23. doi: 10.1007/s00401-018-1932-x
79. McGrowder DA, Miller F, Vaz K, Nwokocha C, Wilson-Clarke C, Anderson-Cross M, et al. Cerebrospinal fluid biomarkers of alzheimer’s disease: current evidence and future perspectives. Brain Sci (2021) 11(2):1–56. doi: 10.3390/brainsci11020215
80. Rosmus DD, Lange C, Ludwig F, Ajami B, Wieghofer P, Cappellano G, et al. The role of osteopontin in microglia biology: current concepts and future perspectives. Biomedicines (2022) 10(4):840. doi: 10.3390/biomedicines10040840
81. Wojcieszak J, Kuczyńska K, Zawilska JB. Role of chemokines in the development and progression of alzheimer’s disease. J Mol Neurosci (2022) 72(9):1929–51. doi: 10.1007/s12031-022-02047-1
82. De Schepper S, Ge JZ, Crowley G, Ferreira LSS, Garceau D, Toomey CE, et al. Perivascular cells induce microglial phagocytic states and synaptic engulfment via SPP1 in mouse models of alzheimer’s disease. Nat Neurosci (2023) 26(3):406–15. doi: 10.1038/s41593-023-01257-z
83. Mcfarland KN, Chakrabarty P. Microglia in alzheimer’s disease: a key player in the transition between homeostasis and pathogenesis. Neurotherapeutics (2022) 19:186–208. doi: 10.1007/s13311-021-01179-3
84. Goldman MD, Ward M, Goldman MD. Epidemiology and pathophysiology of multiple sclerosis. CONTINUUM Lifelong Learn Neurol (2022) 28(4):988–1005. doi: 10.1212/CON.0000000000001136
85. Hauser SL, Cree BAC. Treatment of multiple sclerosis: a review. Am J Med (2020) 133:1380–90.e2. doi: 10.1016/j.amjmed.2020.05.049
86. Lassmann H, Van Horssen J, Mahad D. Progressive multiple sclerosis: pathology and pathogenesis. Nat Rev Neurol (2012) 8(11):647–56. doi: 10.1038/nrneurol.2012.168
87. Preziosa P, Pagani E, Meani A, Moiola L, Rodegher M, Filippi M, et al. Slowly expanding lesions predict 9-year multiple sclerosis disease progression. Neurol Neuroimmunol Neuroinflamm (2022) 9:e1139. doi: 10.1212/NXI.0000000000001139
88. Calvi A, Carrasco FP, Tur C, Chard DT, Stutters J, De Angelis F, et al. Association of slowly expanding lesions on MRI with disability in people with secondary progressive multiple sclerosis. Neurology (2022) 98(17):E1783–93. doi: 10.1212/WNL.0000000000200144
89. Klistorner S, Barnett MH, Graham SL, Wang C, Klistorner A. The expansion and severity of chronic MS lesions follows a periventricular gradient. Multiple Sclerosis J (2022) 28(10):1504–14. doi: 10.1177/13524585221080667
90. Absinta M, Sati P, Masuzzo F, Nair G, Sethi V, Kolb H, et al. Association of chronic active multiple sclerosis lesions with disability in vivo. JAMA Neurol (2019) 76(12):1474–83. doi: 10.1001/jamaneurol.2019.2399
91. Eisele P, Kraemer M, Dabringhaus A, Weber CE, Ebert A, Platten M, et al. Characterization of chronic active multiple sclerosis lesions with sodium ( 23 Na) magnetic resonance imaging-preliminary observations. Eur J Neurol (2021) 28:2392–5. doi: 10.1111/ene.14873
92. Maggi P, Kuhle J, Schädelin S, van der Meer F, Weigel M, Galbusera R, et al. Chronic white matter inflammation and serum neurofilament levels in multiple sclerosis. Neurology (2021) 97(6):e543–53. doi: 10.1212/WNL.0000000000012326
93. Van Schependom J, Guldolf K, D’Hooghe MB, Nagels G, D’Haeseleer M. Detecting neurodegenerative pathology in multiple sclerosis before irreversible brain tissue loss sets in. Transl Neurodegener (2019) 8(1):1–17. doi: 10.1186/s40035-019-0178-4
94. Lassmann H. Pathogenic mechanisms associated with different clinical courses of multiple sclerosis. Front Immunol (2019) 9:3116. doi: 10.3389/FIMMU.2018.03116/PDF
95. Voet S, Prinz M, van Loo G. Microglia in central nervous system inflammation and multiple sclerosis pathology. Trends Mol Med (2019) 25(2):112–23. doi: 10.1016/j.molmed.2018.11.005
96. Zrzavy T, Hametner S, Wimmer I, Butovsky O, Weiner HL, Lassmann H. Loss of “homeostatic” microglia and patterns of their activation in active multiple sclerosis. Brain (2017) 140(7):1900–13. doi: 10.1093/brain/awx113
97. Jäckle K, Zeis T, Schaeren-Wiemers N, Junker A, van der Meer F, Kramann N, et al. Molecular signature of slowly expanding lesions in progressive multiple sclerosis. Brain (2020) 143(7):2073–88. doi: 10.1093/brain/awaa158
98. Giovannoni G, Popescu V, Wuerfel J, Hellwig K, Iacobeus E, Jensen MB, et al. Smouldering multiple sclerosis: the “real MS. Ther Adv Neurol Disord (2022) 15:17562864211066751. doi: 10.1177/17562864211066751
99. Cree BAC, Hollenbach JA, Bove R, Kirkish G, Sacco S, Caverzasi E, et al. Silent progression in disease activity-free relapsing multiple sclerosis. Ann Neurol (2019) 85(5):653–66. doi: 10.1002/ana.25463
100. Kappos L, Butzkueven H, Wiendl H, Spelman T, Pellegrini F, Chen Y, et al. Greater sensitivity to multiple sclerosis disability worsening and progression events using a roving versus a fixed reference value in a prospective cohort study. Mult Scler (2018) 24(7):963–73. doi: 10.1177/1352458517709619
101. Kappos L, Wolinsky JS, Giovannoni G, Arnold DL, Wang Q, Bernasconi C, et al. Contribution of relapse-independent progression vs relapse-associated worsening to overall confirmed disability accumulation in typical relapsing multiple sclerosis in a pooled analysis of 2 randomized clinical trials. JAMA Neurol (2020) 77(9):1132–40. doi: 10.1001/jamaneurol.2020.1568
102. Baecher-Allan C, Kaskow BJ, Weiner HL. Multiple sclerosis: mechanisms and immunotherapy. Neuron (2018) 97(4):742–68. doi: 10.1016/j.neuron.2018.01.021
103. Rissanen E, Tuisku J, Vahlberg T, Sucksdorff M, Paavilainen T, Parkkola R, et al. Microglial activation, white matter tract damage, and disability in MS. Neurol Neuroimmunol Neuroinflamm (2018) 5(3):e443. doi: 10.1212/NXI.0000000000000443
104. Poirion E, Tonietto M, Lejeune FX, Ricigliano VAG, Boudot de la Motte M, Benoit C, et al. Structural and clinical correlates of a periventricular gradient of neuroinflammation in multiple sclerosis. Neurology (2021) 96(14):e1865–75. doi: 10.1212/WNL.0000000000011700
105. Misin O, Matilainen M, Nylund M, Honkonen E, Rissanen E, Sucksdorff M, et al. Innate immune cell–related pathology in the thalamus signals a risk for disability progression in multiple sclerosis. Neurol Neuroimmunol Neuroinflamm (2022) 9(4):e1182. doi: 10.1212/NXI.0000000000001182
106. Carnero Contentti E, Correale J. Bruton’s tyrosine kinase inhibitors: a promising emerging treatment option for multiple sclerosis. Expert Opin Emerg Drugs (2020) 25(4):377–81. doi: 10.1080/14728214.2020.1822817
107. Keaney J, Gasser J, Gillet G, Scholz D, Kadiu I. Inhibition of bruton’s tyrosine kinase modulates microglial phagocytosis: therapeutic implications for alzheimer’s disease. J Neuroimmune Pharmacol (2019) 14(3):448–61. doi: 10.1007/s11481-019-09839-0
108. Gruber RC, Dufault MR, Chretien N, Proto JD, Zhang M, Havari E, et al. Establishing a role for the bruton’s tyrosine kinase inhibitor tolebrutinib in modulating neuroinflammation and disease progression in MS (1974). Neurology (2021) 96(15 Supplement).
109. Oh J, Bar-Or A. Emerging therapies to target CNS pathophysiology in multiple sclerosis. Nat Rev Neurol (2022) 18(8):466–75. doi: 10.1038/s41582-022-00675-0
110. Reich DS, Arnold DL, Vermersch P, Bar-Or A, Fox RJ, Matta A, et al. Safety and efficacy of tolebrutinib, an oral brain-penetrant BTK inhibitor, in relapsing multiple sclerosis: a phase 2b, randomised, double-blind, placebo-controlled trial. Lancet Neurol (2021) 20(9):729–38. doi: 10.1016/S1474-4422(21)00237-4
111. Butovsky O, Koronyo-Hamaoui M, Kunis G, Ophir E, Landa G, Cohen H, et al. Glatiramer acetate fights against alzheimer’s disease by inducing dendritic-like microglia expressing insulin-like growth factor 1. Proc Natl Acad Sci USA (2006) 103(31):11784–9. doi: 10.1073/pnas.0604681103
112. Paraiso HC, Kuo PC, Curfman ET, Moon HJ, Sweazey RD, Yen JH, et al. Dimethyl fumarate attenuates reactive microglia and long-term memory deficits following systemic immune challenge. J Neuroinflamm (2018) 15(1):1–14. doi: 10.1186/s12974-018-1125-5
113. Rosito M, Testi C, Parisi G, Cortese B, Baiocco P, Di Angelantonio S. Exploring the use of dimethyl fumarate as microglia modulator for neurodegenerative diseases treatment. Antioxidants (2020) 9(8):700. doi: 10.3390/antiox9080700
114. Sun X, Suo X, Xia X, Yu C, Dou Y. Dimethyl fumarate is a potential therapeutic option for alzheimer’s disease. J Alzheimers Dis (2022) 85(1):443–56. doi: 10.3233/JAD-215074
115. McGhee DJM, Ritchie CW, Thompson PA, Wright DE, Zajicek JP, Counsell CE. A systematic review of biomarkers for disease progression in alzheimer’s disease. PloS One (2014) 9(2):e88854. doi: 10.1371/JOURNAL.PONE.0088854
116. Brosseron F, Krauthausen M, Kummer M, Heneka MT. Body fluid cytokine levels in mild cognitive impairment and alzheimer’s disease: a comparative overview. Mol Neurobiol (2014) 50(2):534–44. doi: 10.1007/s12035-014-8657-1
117. Park JE, Choi KY, Kim BC, Choi SM, Song MK, Lee JJ, et al. Cerebrospinal fluid biomarkers for the diagnosis of prodromal alzheimer’s disease in amnestic mild cognitive impairment. Dement Geriatr Cognit Dis Extra (2019) 9(1):100–13. doi: 10.1159/000496920
118. Sun MJ, Liu N, Xie QF, Li X, Sun J, Wang H, et al. A candidate biomarker of glial fibrillary acidic protein in CSF and blood in differentiating multiple sclerosis and its subtypes: a systematic review and meta-analysis. Multiple Sclerosis Related Disord (2021) 51:102870. doi: 10.1016/j.msard.2021.102870
119. Bross M, Hackett M, Bernitsas E. Approved and emerging disease modifying therapies on neurodegeneration in multiple sclerosis. Int J Mol Sci (2020) 21(12):4312. doi: 10.3390/ijms21124312
Keywords: inflammation, biomarkers, Alzheimer’s disease (AD), multiple scleorsis (MS), disease progression
Citation: Temmerman J, Engelborghs S, Bjerke M and D’haeseleer M (2023) Cerebrospinal fluid inflammatory biomarkers for disease progression in Alzheimer’s disease and multiple sclerosis: a systematic review. Front. Immunol. 14:1162340. doi: 10.3389/fimmu.2023.1162340
Received: 09 February 2023; Accepted: 12 June 2023;
Published: 13 July 2023.
Edited by:
Rudolph Tanzi, Harvard Medical School, United StatesReviewed by:
Peter Kosa, National Institutes of Health (NIH), United StatesGiovanni Di Liberto, Centre Hospitalier Universitaire Vaudois (CHUV), Switzerland
Copyright © 2023 Temmerman, Engelborghs, Bjerke and D’haeseleer. This is an open-access article distributed under the terms of the Creative Commons Attribution License (CC BY). The use, distribution or reproduction in other forums is permitted, provided the original author(s) and the copyright owner(s) are credited and that the original publication in this journal is cited, in accordance with accepted academic practice. No use, distribution or reproduction is permitted which does not comply with these terms.
*Correspondence: Miguel D’haeseleer, miguel.dhaeseleer@uzbrussel.be
†These authors have contributed equally to this work and share senior authorship