- Diabetes and Metabolism, Bristol Medical School, University of Bristol, Bristol, United Kingdom
Type 1 diabetes (T1D) is an autoimmune condition of children and adults in which immune cells target insulin-producing pancreatic β-cells for destruction. This results in a chronic inability to regulate blood glucose levels. The natural history of T1D is well-characterized in childhood. Evidence of two or more autoantibodies to the islet antigens insulin, GAD, IA-2 or ZnT8 in early childhood is associated with high risk of developing T1D in the future. Prediction of risk is less clear in adults and, overall, the factors controlling the progression rate from multiple islet autoantibody positivity to onset of symptoms are not fully understood. An anti-CD3 antibody, teplizumab, was recently shown to delay clinical progression to T1D in high-risk individuals including adults and older children. This represents an important proof of concept for those at risk of future T1D. Given their role in risk assessment, islet autoantibodies might appear to be the most obvious biomarkers to monitor efficacy. However, monitoring islet autoantibodies in clinical trials has shown only limited effects, although antibodies to the most recently identified autoantigen, tetraspanin-7, have not yet been studied in this context. Measurements of beta cell function remain fundamental to assessing efficacy and different models have been proposed, but improved biomarkers are required for both progression studies before onset of diabetes and in therapeutic monitoring. In this mini-review, we consider some established and emerging predictive and prognostic biomarkers, including markers of pancreatic function that could be integrated with metabolic markers to generate improved strategies to measure outcomes of therapeutic intervention.
1 Introduction
Type 1 diabetes (T1D) results from autoimmune destruction of insulin-producing pancreatic β-cells (1). The condition has a variable incidence rate of between 3.9-57.4/100,000 depending on the country, and annual incidence rates are increasing at approximately 3-4% worldwide (2). Increased incidence was originally reported in those diagnosed under 5 years of age (3) and this was shown to result from a shift to lower age at onset, and not an overall increased incidence across all age groups (4, 5). However, the Centre for Disease Control report from 2002-2015 shows a sharp increase in incidence of T1D in those diagnosed over age 5, most significantly in black, Hispanic, Asian and Pacific Islander populations (6).
The natural history of T1D is increasingly well understood, particularly in children, making it possible to accurately identify individuals “at risk” of future T1D through islet autoantibody screening. This has facilitated clinical trials to delay the onset of T1D, which recently resulted in the regulatory approval in the USA of teplizumab, an anti-CD3 monoclonal antibody. Teplizumab treatment was shown to provide a delay onset of T1D by >2 years on average in "at risk" individuals (7). There is now increased focus on the optimal strategies to:
1. Identify those at risk; children and adults, both relatives of individuals with T1D and those in the general population for additional clinical trials.
2. Monitor the effectiveness of new therapies.
Biomarkers for a disease can be either predictive, prognostic, or both. In T1D, predictive biomarkers, usually islet autoantibodies, are used to assess risk of clinical diagnosis while prognostic biomarkers, for instance measures of beta cell function, are used to monitor disease progression rate. This mini-review provides a brief “snap-shot” of the current status of prediction and highlights the need for improved prognostic biomarkers.
Crucial to monitoring outcomes of immunomodulatory agents is the phase in the natural history when therapeutic intervention occurs. Primary prevention trials rely on identifying those at genetic risk before the autoimmune process has begun. Current examples are trials within the Global Platform for the Prevention of Autoimmune Diabetes (GPPAD) launched in 2015 (8). GPPAD brings together several centres in Europe where neonates are screened for genetic risk of T1D prior to entry into primary prevention trials including POInT (9) and SINT1A (10). In addition, multiple efforts are ongoing in the USA, Australia, Europe, and the UK to screen for risk of ongoing autoimmunity in children and, more recently, in adults.
Much has been learned about risk calculations and screening approaches from studies of first-degree relatives including BABYDIAB (11); DIPP (12); DAISY (13); TrialNet (14); the Belgian Diabetes Registry (15), the Bart’s Oxford (BOX) study (16) and INNODIA (17). It is not clear, however, whether risk assessment in families where one individual already has been diagnosed with T1D will reflect the general population. Here we examine the strategies used to identify individuals “at risk” of future autoimmune diabetes and consider some of the key established predictive biomarkers and those emerging biomarkers which may, in the future, add to predictive and prognostic models (Figure 1).
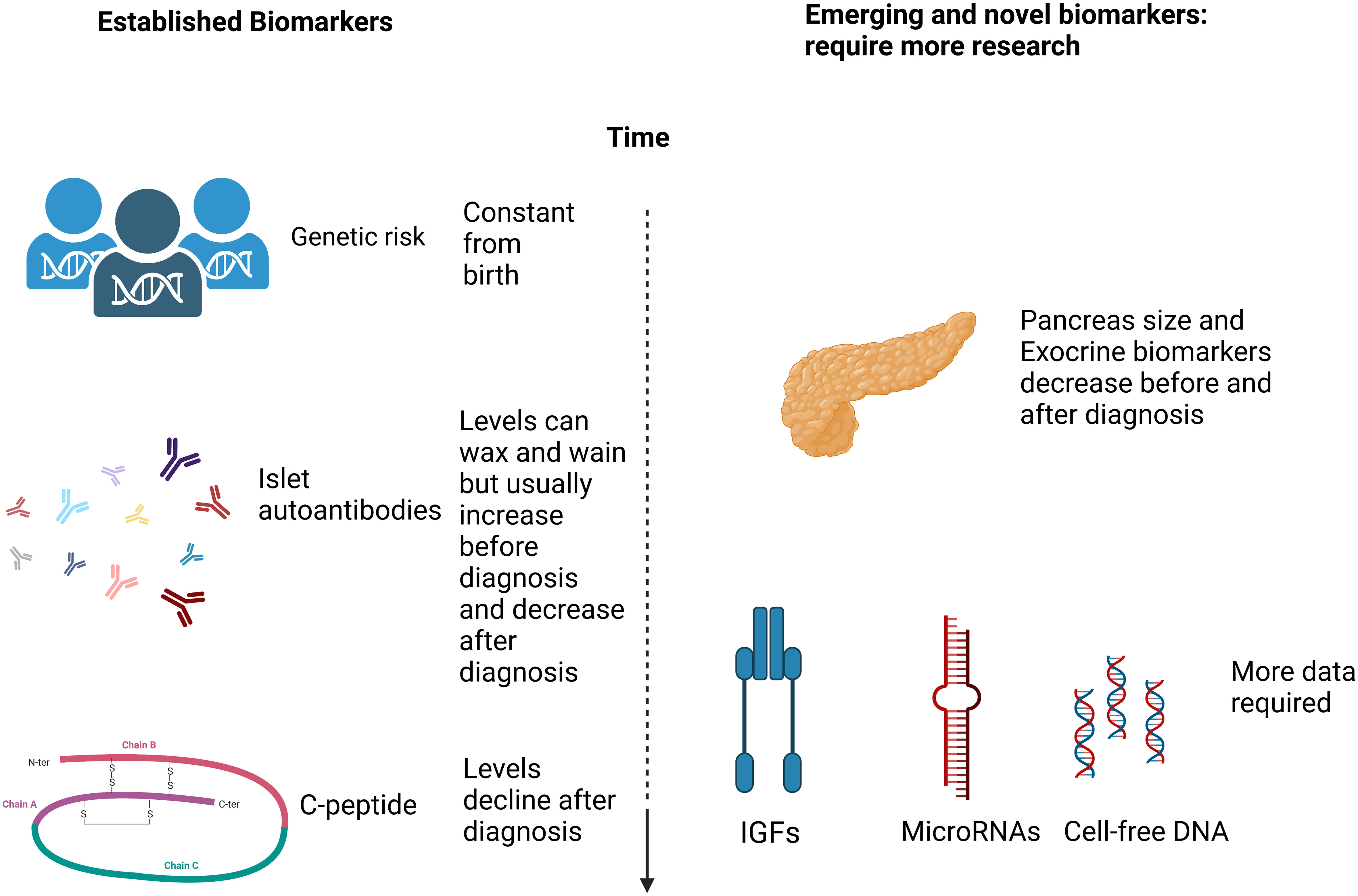
Figure 1 A simplified schematic diagram (created in Biorender.com) of the markers discussed with regard to T1D prediction and prognosis.
2 Identifying risk of future T1D for clinical trial recruitment
2.1 Genetic Risk
The importance of genetics in susceptibility to T1D has long been recognized; studies of monozygotic twins discordant for diabetes demonstrated that approximately half of risk is attributed to genetic factors and half to unidentified environmental factors (18). Human Leukocyte Antigen (HLA) associations were initially described in the 1970s (19, 20). There are three particularly important haplotypes associated with risk of T1D: DRB1*04-DQB1*03:02 (DR4-DQ8), DRB1*03-DQB1*02:01(DR3-DQ2), and DRB1*15-DQB1*06:02 (DR15-DQ6). DR4-DQ8 and DR3-DQ2 are susceptibility haplotypes, whereas DR15-DQ6 is protective (21) including in adult-onset cases (22). The majority of individuals who develop T1D are positive for one or both susceptibility haplotypes (23) and negative for the protective DR15-DQ6 haplotype (24). However, the high-risk combination of DR3/DR4 has been shown to be decreasing over time (25, 26), which suggests an increase in environmental pressure for developing T1D, but the environmental determinants of T1D remain poorly defined.
Genome-wide association studies (GWAS) have identified more than 60 non-HLA variants associated with T1D (27). These include variants in several genes already identified through case control studies [including INS (28); CTLA-4 (29), and PTPN22 (30, 31)]. Over the last decade there has been a move away from traditional HLA genetic risk assessment to the cheaper, high-throughput strategy of using tagged SNPs to impute HLA risk combining data from HLA and non-HLA variants to generate genetic risk scores (GRS). These scores are proving particularly important in precision medicine approaches to help classify diabetes at diagnosis (32, 33). In terms of identifying risk of future T1D, a GRS has the potential to be used to identify infants in the general population at increased genetic risk of type 1 diabetes through Guthrie spot screening, and the GPAAD platform has paved the way for roll out of this approach (8).
2.2 Humoral risk factors: islet autoantibodies
Despite the heterogeneity of T1D, current consensus classifies the prodrome to T1D as having three stages (34), with Stages 1 and 2 being presymptomatic. Stage 1 is defined by the presence of multiple islet autoantibodies in the blood, without dysglycemia; Stage 2 by the presence of multiple islet autoantibodies in the blood with dysglycemia and Stage 3 represents the onset of symptomatic disease.
The natural history of type 1 diabetes has been studied intensively since the identification of islet autoantibodies with a combined study of three birth cohorts showing that children with two or more islet autoantibodies before the age of 5 years have a >80% risk of developing T1D by the age of 20 (35). The power of islet autoantibodies to predict T1D was first described in the 1970s (36) showing that Islet Cell Antibodies (ICA) could be detected in the blood before the onset of symptoms. This test involves incubating serum on pancreas sections, is operator dependent and lacks specificity. Although still carried out, it has largely been superseded by individual tests for autoantibodies to the four major autoantigens in T1D: insulin (IAA) (37), glutamic acid decarboxylase (GADA) (38), insulinoma-associated protein 2 (IA-2A) (39), zinc transporter 8 (ZnT8A) (40). Tetraspanin-7 (Tspan7A) is a more recently identified autoantigen for T1D (41) although its utility in predicting T1D is not established (42) and initial data suggest that Tspan7A do not provide much added value for T1D prediction (43). More studies are however needed across the age range of T1D to confirm whether or not Tspan7A will be useful as a biomarker for T1D. IAA are often the first islet autoantibody to appear in young children (44), and are more prevalent in this group (10, 45). However, these are often present at lower levels in the blood, which makes them the most difficult islet autoantibody to measure. Autoimmunity to insulin cannot be distinguished from antibodies to exogenous insulin appearing roughly two weeks after the first insulin injection in T1D cases, and therefore samples need to be tested in this window to be useful for diabetes classification or baseline monitoring in trials. Some children develop GADA first (44) while IA-2A and ZnT8A autoantibodies are rarely the first to appear and are usually seen as evidence of epitope spreading of the autoimmune response.
The gold standard islet autoantibody tests are considered to be radiobinding assays (RBAs): liquid phase assays which use a radiolabeled antigen to capture and measure antibodies. They are highly sensitive, and risk data from large international longitudinal research studies such as TEDDY (44) and TrialNet (14) are based on RBAs. However, there are significant cost and safety issues associated with RBA and they are being replaced by other methods including ELISA, LIPS and ADAP (outlined in more detail on Table 1). The performance of these assays is measured through testing of blinded samples in islet autoantibody standardization performance (IASP) workshops associated with the Immunology of Diabetes Society (46). Overall, to facilitate general population screening strategies and future clinical trials in both those “at-risk” and in individuals with diabetes, high throughput and cheap sample collection and islet autoantibody tests are required.
3 Monitoring efficacy in clinical trials
3.1 Islet autoantibodies vs. Markers of metabolic function for clinical trial monitoring
Different approaches are currently used to measure β-cell function and provide different readouts about the health of insulin-producing cells (47). The methods most commonly used in research studies to monitor progression rate in individuals with multiple islet autoantibodies are stimulated tests; oral glucose tolerance tests (OGTT); intravenous glucose tolerance tests (IVGTT) and mixed-meal tolerance tests (44). These are carried out by skilled staff, usually in a hospital setting and form the basis for primary outcomes in most T1D clinical trials. Modeling metabolic data to inform progression rates is becoming increasingly sophisticated (48, 49).
While islet autoantibodies are crucial to identify individuals “at-risk” of T1D for trials to prevent or delay the onset of the condition, few data suggest that they represent useful biomarkers to monitor efficacy in the way that models of beta cell function, including C-peptide and immune cell compartments, can be used (48–51). Firstly, some tests use a positive/negative readout for islet autoantibodies and only recently has there been a focus on the potential usefulness of islet autoantibody level in studies of type 1 diabetes (52, 53). However interestingly, in a TrialNet study blocking the CD28/CD80/CD86 costimulatory axis with CTLA4Ig (Abatacept) in individuals with diabetes, participants with a poor response (resistance: measured by modeling rate of decline of C peptide) had a transient increase in activated B cell reprogrammed costimulatory ligand gene expression, and reduced inhibition of anti-insulin antibodies (54). Similarly, in the Teplizumab trial (7), the absence of ZnT8A identified individuals most likely to respond to the therapy. This shows that autoantibodies at baseline may be predictive of responses to immunotherapy and substantiates the inclusion of islet autoantibodies in monitoring.
3.2 Emerging biomarkers
3.2.1 The exocrine pancreas
The pancreas performs both endocrine and exocrine functions. Most biomarker studies have focused on the endocrine compartment, but broader pancreas abnormalities have long been detected in T1D; a reduction in pancreatic size after diagnosis is well-described (55, 56) and pancreas weight is reduced in T1D patients compared with healthy controls (57).
In 2012 using Magnetic Resonance Imaging (MRI), Williams and colleagues showed that the pancreas is already reduced in size by 25% at diagnosis (58). An Australian study in very young “at diagnosis” cases (median 5.5 yrs.) confirmed pancreatic shrinkage in early onset T1D (59). This suggests that pancreatic shrinkage is already ongoing in pre-diabetes. In a study of 85 children participating in the ENDIA study, levels of Fecal Elastase-1, another marker of pancreatic function, were shown to decrease over time in 28 progressors compared to non-progressors (60). A study of TrialNet participants at diagnosis and in those with islet autoantibodies showed that lower levels of circulating P-amylase and lipase (both exocrine enzymes) can be detected before the onset of clinical symptoms in at-risk adult individuals, but not in children (61). Further evidence for the importance of pancreatic enzymes comes from a recent Mendelian Randomisation study to identify circulating proteins influencing type 1 diabetes susceptibility, which showed that increased levels of serum chymotrypsinogen was associated with a decreased risk of T1D (62). Such changes in volume are surprising, since beta cells represent only 2-3% of the pancreas, but reduced pancreas size is thought to reflect loss of the trophic effects of insulin. Recent studies have shown that the exocrine compartment may provide an important source of robust and straightforward biomarkers to monitor effects of therapeutic intervention.
3.2.2 Enzymes of the exocrine pancreas as biomarkers in T1D
Trypsinogen is the proenzyme precursor of trypsin and is stored in the pancreas to be released as required for protein digestion. Immunoreactive trypsinogen (IRT) is a term used to describe the two main isoforms of trypsinogen: the cationic trypsinogen-1, and the anionic trypsingoen-2, both of which are produced by pancreatic acinar cells (63). IRT is released into the circulation in small amounts and can therefore be detected in the blood/plasma. Serum IRT is the most studied indirect test of pancreatic function. It was developed to diagnose chronic pancreatitis (64) and is used to aid diagnosis of exocrine atrophy in T1D (65–67). An IRT test is also currently used worldwide in neonatal screening for cystic fibrosis (68).
There is reduced exocrine pancreatic function in T1D; IRT concentrations have been shown to be significantly reduced in T1D patients compared with healthy matched controls (54). In 2017, Li and colleagues showed that serum trypsinogen levels were significantly reduced in T1D patients compared with controls, and that this was also the case for multiple islet autoantibody positive subjects compared to those with single islet autoantibodies and healthy controls (69). Further studies have built on these findings to demonstrate the potential of trypsinogen as a predictive biomarker for T1D. In 2021, the same team of investigators expanded their studies to trypsinogen, lipase, and amylase in a larger cohort. They showed that trypsinogen and lipase are significantly reduced in subjects with established and recent-onset diabetes, and in individuals with multiple islet autoantibodies compared with single islet autoantibody positive and control subjects (70). In contrast, amylase levels were reduced only in patients with established T1D. They concluded that a combination of serum lipase and trypsinogen levels together provide the most sensitive serological biomarker of BMI-normalised relative pancreas volume (RPVBMI), and this could improve disease staging in pre-T1D, although validation in longitudinal samples from “at-risk” individuals is required.
More recently, a proteomics screen of serum from monozygotic twins discordant for T1D unexpectedly identified exocrine proteins as the top five hits compared to co-twins without diabetes (71). Decreased levels were observed for all five proteins and this was subsequently validated for trypsinogen in a large cohort of individuals with T1D where levels were shown to be significantly lower than in healthy control individuals. They also found that trypsinogen levels were lower in recently-diagnosed cases compared with controls across a broad age range, and multiple linear regression in recently-diagnosed participants showed that trypsinogen levels were associated with insulin dose and diabetic ketoacidosis. Age and BMI were important confounders. Trypsinogen levels <15ng/ml were associated with an increased risk of progression in “at-risk” relatives. Together, these results further validate the potential of trypsinogen and possibly other exocrine enzymes as novel and cost-effective biomarkers to monitor efficacy in clinical trials. However, age and BMI need to be incorporated into all models and longitudinal measures will be essential for the outcomes of interventions to be monitored. MRI of the pancreas in combination with measures of exocrine enzymes is also potentially a powerful, if expensive, tool to monitor direct effects of immunomodulation on the pancreas.
3.2.3 MicroRNAs
MicroRNAs (miRNAs) are emerging as a potential area deserving further study in T1D research and may prove to be future biomarkers for the disease (72). miRNAs are small, non-coding RNAs approximately 20 nucleotides long (73) which have been identified in biological samples relevant to T1D, with some studies reporting their role in T1D pathogenesis. They act as gene expression regulators, primarily by inhibiting translation or by causing mRNA degradation, which obstructs protein synthesis at the post-transcriptional stage. miRNAs can be isolated from most biological specimens, and are durable, being protected by microsomes and exosomes, which develop a protective outer shell for the miRNAs. However, miRNA testing could be challenging to implement in a clinical trial setting. This is because sample analyses currently need to be carried out within 8 hours post-collection for an accurate assessment of miRNA species in the plasma, but most multi-centre trials will not be able to deliver samples to central laboratories within this time span.
MiRNA-21 is a specific miRNA that has been shown to disrupt β-cell development in animal models of T1D when overexpressed (74). MiRNA-21 also targets bcl-2 gene translation, which results in increased β-cell apoptosis during diabetes development (75, 76). Other specific miRNAs that dysregulate pancreatic function include miRNA-29, which impairs glucose-induced insulin secretion when increased in mouse and human pancreatic islets (77). More recently, plasma levels of five miRNAs were shown to be downregulated in diabetic vs. normoglycaemic mice (78). miR-409-3p was also downregulated in immune islet infiltrates of diabetic mice, and its expression correlated with severity of insulitis. Interestingly, CD8+ central memory T cells were enriched in miR-409-3p. Plasma levels of the microRNA gradually decreased during diabetes development in mice and improved with disease remission after anti-CD3 antibody therapy. However, these results do not necessarily suggest that these miRNAs will be similarly relevant to human T1D, because miRNA data are not fully translatable from rodent to human samples. In human plasma samples, miR-409-3p levels were lower in individuals with recently-diagnosed T1D compared with controls, and levels were inversely correlated with HbA1c levels (78). Studies such as these may suggest the potential of microRNAs to monitor therapeutic intervention in T1D, but they have not yet been studied in individuals at risk for the condition, so much work remains to be carried out to fully validate these molecules as tools for prediction or prognosis.
3.2.4 Insulin-like Growth Factors
Insulin-like growth factors (IGFs) promote glucose metabolism. IGF1 and IGF2 have their availability regulated by IGF-binding proteins (IGFBPs). A recent study by Shapiro et al. found that IGF1 and IGF2 levels were significantly lower in islet autoantibody positive compared with islet autoantibody negative relatives of individuals with T1D, and that IGF1 levels decreased over time in subjects with multiple islet autoantibodies and in those who progressed to T1D, in parallel with decreasing β-cell function (79). This study also found that high-affinity IGFBPs remain unchanged in individuals with pre-T1D, which suggests that total IGF levels may reflect bioactivity. These results indicate that IGF dysregulation occurs both before and after T1D diagnosis, and therefore could be a novel biomarker for disease prediction and monitoring the effects of therapy in secondary prevention trials. Importantly, IGFs could act as metabolic biomarkers in that they reflect metabolic dysregulation and therefore could inform T1D staging.
3.2.5 Cell-Free DNA
Cell-free (cf)DNA refers to β-cell-specific, cell-free DNA fragments that are released into the periphery as β-cells are killed by immune cells. These β-cell-specific cfDNA fragments can be measured, should correlate with β-cell death, and could therefore potentially be the most direct biomarker for β-cell death in T1D. Several years ago, multiple studies focused on methylation-specific cfDNA targets, particularly in the insulin gene, to measure β-cell-specific cell death (80–86). However, the methodology fell out of favour when it was reported that an ultrasensitive assay for detection of a β-cell-specific DNA methylation signature failed to observe increases in β-cell-derived cfDNA in a blinded study of 32 autoantibody-positive subjects at risk for type 1 diabetes, 92 individuals with recent-onset type 1 diabetes, and 38 individuals with long-standing disease (87).
In the meantime however, cfDNA increasingly represents an exciting biomarker in cancer studies; in 2021 the National Health Service in the UK launched a research study to examine cfDNA in 140,000 volunteers, aiming to detect 50 types of cancer before symptoms appear. Sample collection systems for cfDNA have improved significantly with collection of plasma samples in dedicated cfDNA tubes which stabilize the cfDNA fragments as the current standard. Therefore, while cfDNA studies in T1D require further optimization, particularly using multiplex approaches, cfDNA has the potential to become a monitoring biomarker of the future. The exquisite specificity of a biomarker capable of directly measuring beta cell death has to be the ambition when monitoring drug efficacy in T1D.
4 Conclusions
The outcome of the Teplizumab trial in individuals “at-risk” of future T1D has energized researchers to broaden strategies to identify single- and multiple-islet-autoantibody-positive children and adults in the general population. These strategies would aim to help prevent diagnosis in diabetic ketoacidosis and offer participation in intervention trials and monitoring. Here, we have reflected on some existing and possible future biomarkers to determine efficacy of interventions, enroll and stratify individuals and, hopefully, be used to match the right patient to the right drug at the right time.
Author contributions
MF and KG designed the review. MF performed literature searches and wrote the manuscript with oversight by KG. KG is responsible for the integrity of the work as a whole. All authors contributed to the article and approved the submitted version.
Funding
This work was funded by grants to KG from Diabetes UK Grant Reference 20/0006300 and The Helmsley Charitable Trust.
Acknowledgments
The authors are extremely grateful to Dr Claire Williams, Diabetes and Metabolism, University of Bristol for helpful discussions with MF and providing information from her PhD thesis on different islet autoantibody tests.
Conflict of interest
The authors declare that the research was conducted in the absence of any commercial or financial relationships that could be construed as a potential conflict of interest.
Publisher’s note
All claims expressed in this article are solely those of the authors and do not necessarily represent those of their affiliated organizations, or those of the publisher, the editors and the reviewers. Any product that may be evaluated in this article, or claim that may be made by its manufacturer, is not guaranteed or endorsed by the publisher.
References
1. Atkinson M, Eisenbarth G, Michels A. Type 1 diabetes. Lancet (2014) 383(9911):69–82. doi: 10.1016/S0140-6736(13)60591-7
2. Maahs DM, West NA, Lawrence JM, Mayer-Davis EJ. Epidemiology of type 1 diabetes. Endocrinol Metab Clin North Am (2010) 39(3):481–97. doi: 10.1016/j.ecl.2010.05.011
3. Gardner SG, Bingley PJ, Sawtell PA, Weeks S, Gale EA. Rising incidence of insulin dependent diabetes in children aged under 5 years in the Oxford region: time trend analysis. the bart's-Oxford study group. BMJ. (1997) 315(7110):713–7. doi: 10.1136/bmj.315.7110.713
4. Patterson CC, Gyürüs E, Rosenbauer J, Cinek O, Neu A, Schober E, et al. Trends in childhood type 1 diabetes incidence in Europe during 1989-2008: evidence of non-uniformity over time in rates of increase. Diabetologia (2012) 55(8):2142–7. doi: 10.1007/s00125-012-2571-8
5. Pundziute-Lycka A, Dahlquist G, Nystrom L, Arnqvist H, Björk E, Blohmé G, et al. The incidence of type I diabetes has not increased but shifted to a younger age at diagnosis in the 34 years group in Sweden 1983–1998. Diabetologia (2002) 45:783–91. doi: 10.1007/s00125-002-0845-2
6. Divers J, Mayer-Davis EJ, Lawrence JM, Isom S, Dabelea D, Dolan L, et al. Trends in incidence of type 1 and type 2 diabetes among youths - selected counties and Indian reservations, united states, 2002-2015. MMWR Morb Mortal Wkly Rep (2020) 69(6):161–5. doi: 10.15585/mmwr.mm6906a3
7. Herold KC, Bundy BN, Long SA, Bluestone JA, DiMeglio LA, Dufort MJ, et al. An anti-CD3 antibody, teplizumab, in relatives at risk for type 1 diabetes. N Engl J Med (2019) 381:603–13. doi: 10.1056/NEJMoa1902226
8. Ziegler AG, Danne T, Dunger DB, Berner R, Puff R, Kiess W, et al. Primary prevention of beta-cell autoimmunity and type 1 diabetes - the global platform for the prevention of autoimmune diabetes (GPPAD) perspectives. Mol Metab (2016) 5:255–62. doi: 10.1016/j.molmet.2016.02.003
9. Ziegler AG, Achenbach P, Berner R, Casteels K, Danne T, Gündert M, et al. Oral insulin therapy for primary prevention of type 1 diabetes in infants with high genetic risk: the GPPAD-POInT (global platform for the prevention of autoimmune diabetes primary oral insulin trial) study protocol. BMJ Open (2019) 9:e028578. doi: 10.1136/bmjopen-2018-028578
10. Ziegler AG, Arnolds S, Kölln A, Achenbach P, Berner R, Bonifacio E, et al. Supplementation with Bifidobacterium longum subspecies infantis EVC001 for mitigation of type 1 diabetes autoimmunity: the GPPAD-SINT1A randomised controlled trial protocol. BMJ Open (2021) 11:e052449. doi: 10.1136/bmjopen-2021-052449
11. Ziegler AG, Bonifacio E, BABYDIAB-BABYDIET Study Group. Age-related islet autoantibody incidence in offspring of patients with type 1 diabetes. Diabetologia (2012) 55:1937–43. doi: 10.1007/s00125-012-2472-x
12. Kuusela S, Keskinen P, Pokka T, Knip M, Ilonen J, Vähäsalo P, et al. Extended family history of type 1 diabetes in HLA-predisposed children with and without islet autoantibodies. Pediatr Diabetes (2020) 21:1447–56. doi: 10.1111/pedi.13122
13. Steck AK, Dong F, Frohnert BI, Waugh K, Hoffman M, Norris JM, et al. Predicting progression to diabetes in islet autoantibody positive children. J Autoimmun (2018) 90:59–63. doi: 10.1016/j.jaut.2018.01.006
14. Skyler JS, Greenbaum CJ, Lachin JM, Leschek E, Rafkin-Mervis L, Savage P, et al. Type 1 diabetes TrialNet–an international collaborative clinical trials network. Ann N Y Acad Sci (2008) 1150:14–24. doi: 10.1196/annals.1447.054
15. Gorus FK, Balti EV, Messaaoui A, Demeester S, Van Dalem A, Costa O, et al. Twenty-year progression rate to clinical onset according to autoantibody profile, age, and HLA-DQ genotype in a registry-based group of children and adults with a first-degree relative with type 1 diabetes. Diabetes Care (2017) 40:1065–72. doi: 10.2337/dc16-2228
16. Gillespie KM, Fareed R, Mortimer GL. Four decades of the bart's Oxford study: Improved tests to predict type 1 diabetes. Diabetes Med (2021) 38:e14717. doi: 10.1111/dme.14717
17. Dunger DB, Bruggraber SFA, Mander AP, Marcovecchio ML, Tree T, Chmura, et al. INNODIA master protocol for the evaluation of investigational medicinal products in children, adolescents and adults with newly diagnosed type 1 diabetes. Trials (2022) 23:414. doi: 10.1186/s13063-022-06259-z
18. Redondo MJ, Fain PR and Eisenbarth GS. Genetics of type 1A diabetes. Recent Prog Horm Res (2001) 56:69–89. doi: 10.1210/rp.56.1.69
19. Cudworth AG, Woodrow JC. HLA system and diabetes mellitus. Diabetes. (1975) 24:345–9. doi: 10.2337/diab.24.4.345
20. Nerup J, Platz P, Andersen OO, Christy M, Lyngsoe J, Poulsen JE, et al. HL-a antigens and diabetes mellitus. Lancet (1974) 2:864–6. doi: 10.1016/s0140-6736(74)91201-x
21. Lambert AP, Gillespie KM, Thomson G, Cordell HJ, Todd JA, Gale EA, et al. Absolute risk of childhood-onset type 1 diabetes defined by human leukocyte antigen class II genotype: a population-based study in the united kingdom. J Clin Endocrinol Metab (2004) 89:4037–43. doi: 10.1210/jc.2003-032084
22. Thomas NJ, Dennis JM, Sharp SA, Kaur A, Misra S, Walkey HC, et al. DR15-DQ6 remains dominantly protective against type 1 diabetes throughout the first five decades of life. Diabetologia (2021) 64:2258–65. doi: 10.1007/s00125-021-05599-w
23. Devendra D, Eisenbarth GS. Immunologic endocrine disorders. J Allergy Clin Immunol (2003) 111(2 Suppl):S624–36. doi: 10.1067/mai.2003.81
24. Eisenbarth GS, Gottlieb PA. Autoimmune polyendocrine syndromes. N Engl J Med (2004) 350:2068–79. doi: 10.1056/NEJMra030158
25. Gillespie KM, Bain SC, Barnett AH, Bingley PJ, Christie MR, Gill GV, et al. The rising incidence of childhood type 1 diabetes and reduced contribution of high-risk HLA haplotypes. Lancet (2004) 364:1699–700. doi: 10.1016/S0140-6736(04)17357-1
26. Fourlanos S, Varney MD, Tait BD, Morahan G, Honeyman MC, Colman PG, et al. The rising incidence of type 1 diabetes is accounted for by cases with lower-risk human leukocyte antigen genotypes. Diabetes Care (2008) 31:1546–9. doi: 10.2337/dc08-0239
27. Robertson CC, Inshaw JRJ, Onengut-Gumuscu S, Chen WM, Santa Cruz DF, Yang H, et al. Fine-mapping, trans-ancestral and genomic analyses identify causal variants, cells, genes and drug targets for type 1 diabetes. Nat Genet (2021) 53:962–71. doi: 10.1038/s41588-021-00880-5
28. Bell GI, Horita S and Karam JH. A polymorphic locus near the human insulin gene is associated with insulin-dependent diabetes mellitus. Diabetes (1984) 33:176–83. doi: 10.2337/diab.33.2.176
29. Ueda H, Howson JM, Esposito L, Heward J, Snook H, Chamberlain G, et al. Association of the T-cell regulatory gene CTLA4 with susceptibility to autoimmune disease. Nature (2003) 423:506–11. doi: 10.1038/nature01621
30. Bottini N, Musumeci L, Alonso A, Rahmouni S, Nika K, Rostamkhani, et al. A functional variant of lymphoid tyrosine phosphatase is associated with type I diabetes. Nat Genet (2004) 36:337–8. doi: 10.1038/ng1323
31. Smyth D, Cooper JD, Collins JE, Heward JM, Franklyn JA, Howson JMM, et al. Replication of an association between the lymphoid tyrosine phosphatase locus (LYP/PTPN22) with type 1 diabetes, and evidence for its role as a general autoimmunity locus. Diabetes (2004) 53:3020–3. doi: 10.2337/diabetes.53.11.3020
32. Oram RA, Patel K, Hill A, Shields B, McDonald TJ, Jones AG, et al. A type 1 diabetes genetic risk score can aid discrimination between type 1 and type 2 diabetes in young adults. Diabetes Care (2016) 39:337–44. doi: 10.2337/dc15-1111
33. Carr ALJ, Evans-Molina C and Oram RA. Precision medicine in type 1 diabetes. Diabetologia (2022) 65:1854–66. doi: 10.1007/s00125-022-05778-3
34. Insel RA, Dunne JL, Atkinson MA, Chiang JL, Dabelea D, Gottlieb PA, et al. Staging presymptomatic type 1 diabetes: a scientific statement of JDRF, the endocrine society, and the American diabetes association. Diabetes Care (2015) 38:1964–74. doi: 10.2337/dc15-1419
35. Ziegler AG, Rewers M, Simell O, Simell T, Lempainen J, Steck A, et al. Seroconversion to multiple islet autoantibodies and risk of progression to diabetes in children. JAMA (2013) 309:2473–9. doi: 10.1001/jama.2013.6285
36. Bottazzo GF, Mann JI, Thorogood M, Baum JD, Doniach D. Autoimmunity in juvenile diabetics and their families. Br Med J (1978) 2:165–8. doi: 10.1136/bmj.2.6131.165
37. Palmer JP, Asplin CM, Clemons P, Lyen K, Tatpati O, Raghu PK, et al. Insulin antibodies in insulin-dependent diabetics before insulin treatment. Science (1983) 222:1337–9. doi: 10.1126/science.6362005
38. Baekkeskov S, Aanstoot HJ, Christgau S, Reetz A, Solimena M, Cascalho M, et al. Identification of the 64K autoantigen in insulin-dependent diabetes as the GABA-synthesizing enzyme glutamic acid decarboxylase. Nature (1990) 347:151–6. doi: 10.1038/347151a0
39. Payton MA, Hawkes CJ, Christie MR. Relationship of the 37,000- and 40,000-m(r) tryptic fragments of islet antigens in insulin-dependent diabetes to the protein tyrosine phosphatase-like molecule IA-2 (ICA512). J Clin Invest (1995) 96:1506–11. doi: 10.1172/JCI118188
40. Wenzlau JM, Juhl K, Yu L, Moua O, Sarkar SA, Gottlieb P, et al. The cation efflux transporter ZnT8 (Slc30A8) is a major autoantigen in human type 1 diabetes. Proc Natl Acad Sci USA (2007) 104:17040–5. doi: 10.1073/pnas.0705894104
41. McLaughlin KA, Richardso CC, Ravishankar A, Brigatti C, Liberati D, Lampasona V, et al. Identification of tetraspanin-7 as a target of autoantibodies in type 1 diabetes. Diabetes (2016) 65:1690–8. doi: 10.2337/db15-1058
42. McLaughlin KA, Tombs MA, Christie MR. Autoimmunity to tetraspanin-7 in type 1 diabetes. Med Microbiol Immunol (2020) 209(4):437–45. doi: 10.1007/s00430-020-00674-2
43. Walther D, Eugster A, Jergens S, Weinzierl C, Telieps T, Winkler C, et al. Tetraspanin 7 autoantibodies in type 1 diabetes. Diabetologia (2016) 59(9):1973–6. doi: 10.1007/s00125-016-3997-1
44. Vehik K, Bonifacio E, Lernmark Å, Yu L, Williams A, Schatz D, et al. Hierarchical order of distinct autoantibody spreading and progression to type 1 diabetes in the TEDDY study. Diabetes Care (2020) 43:2066–73. doi: 10.2337/dc19-2547
45. Williams CL, Aitken RJ, Wilson IV, Mortimer GLM, Long AE, Williams AJK, et al. The measurement of autoantibodies to insulin informs diagnosis of diabetes in a childhood population negative for other autoantibodies. Diabetes Med (2022) 39:e14979. doi: 10.1111/dme.14979
46. Marzinotto I, Pittman DL, Williams AJK, Long AE, Achenbach P, Schlosser M, et al. Islet autoantibody standardization program: interlaboratory comparison of insulin autoantibody assay performance in 2018 and 2020 workshops. Diabetologia (2023) 66:897–912. doi: 10.1007/s00125-023-05877-9
47. Robertson RP. Estimation of beta-cell mass by metabolic tests: necessary, but how sufficient? Diabetes (2007) 56:2420–4. doi: 10.2337/db07-0742
48. Xu P, Wu Y, Zhu Y, Dagne G, Johnson G, Cuthbertson D, et al. Diabetes prevention trial-type 1 (DPT-1) study group. prognostic performance of metabolic indexes in predicting onset of type 1 diabetes. Diabetes Care (2010) 33:2508–13. doi: 10.2337/dc10-0802
49. Sims EK, Cuthbertson D, Felton JL, Ismail HM, Nathan BM, Jacobsen LM, et al. Persistence of β-cell responsiveness for over two years in autoantibody-positive children with marked metabolic impairment at screening. Diabetes Care (2022) 45:2982–90. doi: 10.2337/dc22-1362
50. Wentworth JM, Bediaga NG, Giles LC, Ehlers M, Gitelman SE, Geyer S, et al. Beta cell function in type 1 diabetes determined from clinical and fasting biochemical variables. Diabetologia (2019) 62:33–40. doi: 10.1007/s00125-018-4722-z
51. Naushad N, Perdigoto AL, Rui J, Herold KC. Have we pushed the needle for treatment of type 1 diabetes? Curr Opin Immunol (2017) 49:44–50. doi: 10.1016/j.coi.2017.09.004
52. Kwon BC, Achenbach P, Anand V, Frohnert BI, Hagopian W, Hu J, et al. Islet autoantibody levels differentiate progression trajectories in individuals with presymptomatic type 1 diabetes. Diabetes (2022) 71:2632–41. doi: 10.2337/db22-0360
53. Grace SL, Bowden J, Walkey HC, Kaur A, Misra S, Shields BM, et al. Islet autoantibody level distribution in type 1 diabetes and their association with genetic and clinical characteristics. J Clin Endocrinol Metab (2022) 107:e4341–9. doi: 10.1210/clinem/dgac507
54. Linsley PS, Greenbaum CJ, Speake C, Long SA and Dufort MJ. B lymphocyte alterations accompany abatacept resistance in new-onset type 1 diabetes. JCI Insight (2019) 4:e126136. doi: 10.1172/jci.insight.126136
55. Gepts W. Pathologic anatomy of the pancreas in juvenile diabetes mellitus. Diabetes (1965) 14:619–33. doi: 10.2337/diab.14.10.619
56. Williams AJ, Chau W, Callaway MP, Dayan CM. Magnetic resonance imaging: a reliable method for measuring pancreatic volume in type 1 diabetes. Diabetes Med (2007) 24:35–40. doi: 10.1111/j.1464-5491.2007.02027.x
57. Campbell-Thompson M, Wasserfall C, Montgomery EL, Atkinson MA, Kaddis JS. Pancreas organ weight in individuals with disease-associated autoantibodies at risk for type 1 diabetes. JAMA (2012) 308:2337–9. doi: 10.1001/jama.2012.15008
58. Williams AJ, Thrower SL, Sequeiros IM, Ward A, Bickerton AS, Triay J, et al. Pancreatic volume is reduced in adult patients with recently diagnosed type 1 diabetes. J Clin Endocrinol Metab (2012) 97:E2109–13. doi: 10.1210/jc.2012-1815
59. Augustine P, Gent R, Louise J, Taranto M, Penno M, Linke R, et al. Pancreas size and exocrine function is decreased in young children with recent-onset type 1 diabetes. Diabetes Med (2020) 37:1340–3. doi: 10.1111/dme.13987
60. Penno MAS, Oakey H, Augustine P, Taranto M, Barry SC, Colman PG, et al. Changes in pancreatic exocrine function in young at-risk children followed to islet autoimmunity and type 1 diabetes in the ENDIA study. Pediatr Diabetes (2020) 21:945–9. doi: 10.1111/pedi.13056
61. Giovenzana A, Vecchio F, Cugnata F, Nonis A, Mandelli A, Stabilini A, et al. Exocrine pancreas function is impaired in adult relatives of patients with type 1 diabetes. Acta Diabetol (2022) 59:473–9. doi: 10.1007/s00592-021-01819-2
62. Yazdanpanah N, Yazdanpanah M, Wan Y, Forgetta V, Pollak M, Polychronakos C, et al. Clinically relevant circulating protein biomarkers for type 1 diabetes: Evidence from a two-sample mendelian randomization study. Diabetes Care (2022) 45:169–77. doi: 10.2337/dc21-1049
63. Lippi G, Valentino M, Cervellin G. Laboratory diagnosis of acute pancreatitis: in search of the holy grail. Crit Rev Clin Lab Sci (2012) 49:18–31. doi: 10.3109/10408363.2012.658354
64. Basnayake C, Ratnam D. Blood tests for acute pancreatitis. Aust Prescr (2015) 38:128–30. doi: 10.18773/austprescr.2015.043
65. Moffat A, Marks V, Gamble DR. Serum immunoreactive trypsin concentrations in diabetic children. J Clin Pathol (1980) 33(9):871–5. doi: 10.1136/jcp.33.9.871
66. Masoero G, Andriulli A, Bianco A, Benitti V, Marchetto M, de la Pierre M. Diagnostic accuracy of serum cationic trypsinogen estimation for pancreatic diseases. Dig Dis Sci (1982) 27:1089–94. doi: 10.1007/BF01391446
68. Baker MW, Atkins AE, Cordovado SK, Hendrix M, Earley MC, Farrell PM. Improving newborn screening for cystic fibrosis using next-generation sequencing technology: a technical feasibility study. Genet Med (2016) 18:231–8. doi: 10.1038/gim.2014.209
69. Li X, Campbell-Thompson M, Wasserfall CH, McGrail K, Posgai A, Schultz AR, et al. Serum trypsinogen levels in type 1 diabetes. Diabetes Care (2017) 40:577–82. doi: 10.2337/dc16-1774
70. Ross JJ, Wasserfall CH, Bacher R, Perry DJ, McGrail K, Posgai AL, et al. Exocrine pancreatic enzymes are a serological biomarker for type 1 diabetes staging and pancreas size. Diabetes (2021) 70:944–54. doi: 10.2337/db20-0995
71. Bakinowska L, Vartak T, Phuthego T, Taylor M, Chandler K, Jerram ST, et al. Exocrine proteins including trypsin(ogen) as a key biomarker in type 1 diabetes. Diabetes Care (2023) 46:714–21. doi: 10.2337/dc22-1317
72. Dotta F, Ventriglia G, Snowhite IV, Pugliese A. MicroRNAs: markers of β-cell stress and autoimmunity. Curr Opin Endocrinol Diabetes Obes (2018) 25:237–45. doi: 10.1097/MED.0000000000000420
73. Filipowicz W, Bhattacharyya SN, Sonenberg N. Mechanisms of post-transcriptional regulation by microRNAs: are the answers in sight? Nat Rev Genet (2008) 9:102–14. doi: 10.1038/nrg2290
74. Miao C, Chang J, Zhang G, Fang Y. MicroRNAs in type 1 diabetes: New research progress and potential directions. Biochem Cell Biol (2018) 96:498–506. doi: 10.1139/bcb-2018-0027
75. Lakhter AJ, Pratt RE, Moore RE, Doucette KK, Maier BF, DiMeglio LA, et al. Beta cell extracellular vesicle miR-21-5p cargo is increased in response to inflammatory cytokines and serves as a biomarker of type 1 diabetes. Diabetologia (2018) 61:1124–34. doi: 10.1007/s00125-018-4559-5
76. Grieco GE, Cataldo D, Ceccarelli E, Nigi L, Catalano G, Brusco, et al. Serum levels of miR-148a and miR-21-5p are increased in type 1 diabetic patients and correlated with markers of bone strength and metabolism. Noncoding RNA (2018) 4:37. doi: 10.3390/ncrna4040037
77. Roggli E, Gattesco S, Caille D, Briet C, Boitard C, Meda P, et al. Changes in microRNA expression contribute to pancreatic β-cell dysfunction in prediabetic NOD mice. Diabetes (2012) 61:1742–51. doi: 10.2337/db11-1086
78. Ventriglia G, Mancarella F, Sebastiani G, Cook DP, Mallone R, Mathieu C, et al. miR-409-3p is reduced in plasma and islet immune infiltrates of NOD diabetic mice and is differentially expressed in people with type 1 diabetes. Diabetologia (2020) 63:124–36. doi: 10.1007/s00125-019-05026-1
79. Shapiro MR, Wasserfall CH, McGrail SM, Posgai AL, Bacher R, Muir AH, et al. Insulin-like growth factor dysregulation both preceding and following type 1 diabetes diagnosis. Diabetes (2020) 69:413–23. doi: 10.2337/db19-0942
80. Akirav EM, Lebastchi J, Galvan EM, Henegariu O, Akirav M, Ablamunits V, et al. Detection of β cell death in diabetes using differentially methylated circulating DNA. Proc Natl Acad Sci USA (2011) 108:19018–23. doi: 10.1073/pnas.1111008108
81. Lebastchi J, Deng S, Lebastchi AH, Beshar I, Gitelman S, Willi S, et al. Immune therapy and β-cell death in type 1 diabetes. Diabetes (2013) 62:1676–80. doi: 10.2337/db12-1207
82. Usmani-Brown S, Lebastchi J, Steck AK, Beam C, Herold KC, Ledizet M. Analysis of β-cell death in type 1 diabetes by droplet digital PCR. Endocrinology (2014) 155:3694–8. doi: 10.1210/en.2014-1150
83. Husseiny MI, Kaye A, Zebadua E, Kandeel F and Ferreri K. Tissue-specific methylation of human insulin gene and PCR assay for monitoring beta cell death. PLoS One (2014) 9:e94591. doi: 10.1371/journal.pone.0094591
84. Fisher MM, Watkins RA, Blum J, Evans-Molina C, Chalasani N, DiMeglio LA, et al. Elevations in circulating methylated and unmethylated preproinsulin DNA in new-onset type 1 diabetes. Diabetes (2015) 64:3867–72. doi: 10.2337/db15-0430
85. Herold KC, Usmani-Brown S, Ghazi T, Lebastchi J, Beam CA, Bellin MD, et al. β cell death and dysfunction during type 1 diabetes development in at-risk individuals. J Clin Invest (2015) 125:1163–73. doi: 10.1172/JCI78142
86. Lehmann-Werman R, Neiman D, Zemmour H, Moss J, Magenheim J, Vaknin-Dembinsky A, et al. Identification of tissue-specific cell death using methylation patterns of circulating DNA. Proc Natl Acad Sci USA (2016) 113:E1826–34. doi: 10.1073/pnas.1519286113
Keywords: islet autoantibodies, genetics, exocrine, endocrine, trypsinogen, cfDNA (cell-free DNA), pancreatic enzymes, microRNAs
Citation: Fyvie MJ and Gillespie KM (2023) The importance of biomarker development for monitoring type 1 diabetes progression rate and therapeutic responsiveness. Front. Immunol. 14:1158278. doi: 10.3389/fimmu.2023.1158278
Received: 03 February 2023; Accepted: 28 March 2023;
Published: 15 May 2023.
Edited by:
Bernard Khor, Benaroya Research Institute, United StatesReviewed by:
Johnna D. Wesley, Novo Nordisk, United StatesSusanne Cabrera, Medical College of Wisconsin, United States
Copyright © 2023 Fyvie and Gillespie. This is an open-access article distributed under the terms of the Creative Commons Attribution License (CC BY). The use, distribution or reproduction in other forums is permitted, provided the original author(s) and the copyright owner(s) are credited and that the original publication in this journal is cited, in accordance with accepted academic practice. No use, distribution or reproduction is permitted which does not comply with these terms.
*Correspondence: Kathleen M. Gillespie, k.m.gillespie@bristol.ac.uk