- 1Division of Immunology and Allergy, Department of Medicine Solna, Karolinska Institutet, and Karolinska University Hospital, Stockholm, Sweden
- 2Center for Infectious Medicine, Department of Medicine Huddinge, Karolinska Institutet, Stockholm, Sweden
- 3Unit for Experimental Asthma and Allergy Research Centre for Allergy Research, The Institute of Environmental Medicine, Karolinska Institutet, Stockholm, Sweden
- 4National Bioinformatics Infrastructure Sweden, Science for Life Laboratory, Stockholm University, Stockholm, Sweden
- 5Thoracic Surgery, Department of Molecular Medicine and Surgery, Karolinska Institutet, Karolinska University Hospital, Stockholm, Sweden
- 6Department of Medical Sciences, Uppsala University, Uppsala, Sweden
Mast cells are tissue-resident cells playing major roles in homeostasis and disease conditions. Lung mast cells are particularly important in airway inflammatory diseases such as asthma. Human mast cells are classically divided into the subsets MCT and MCTC, where MCT express the mast cell protease tryptase and MCTC in addition express chymase, carboxypeptidase A3 (CPA3) and cathepsin G. Apart from the disctintion of the MCT and MCTC subsets, little is known about the heterogeniety of human lung mast cells and a deep analysis of their heterogeniety has previously not been performed. We therefore performed single cell RNA sequencing on sorted human lung mast cells using SmartSeq2. The mast cells showed high expression of classical mast cell markers. The expression of several individual genes varied considerably among the cells, however, no subpopulations were detected by unbiased clustering. Variable genes included the protease-encoding transcripts CMA1 (chymase) and CTSG (cathepsin G). Human lung mast cells are predominantly of the MCT subset and consistent with this, the expression of CMA1 was only detectable in a small proportion of the cells, and correlated moderately to CTSG. However, in contrast to established data for the protein, CPA3 mRNA was high in all cells and the correlation of CPA3 to CMA1 was weak.
Introduction
Mast cells play important roles in the lung both in homeostasis and in disease and they are particularly recognized for their role in asthma (1). Mast cells are found in all different compartments of the human lung; i.e., in the epithelium, in smooth muscle bundles, around pulmonary vessels, and in the parenchyma (2). Upon activation, mast cells release their preformed granule content, including histamine, proteases, proteoglycans and some cytokines/chemokine. In addition mast cells also start de novo synthesis of lipid mediators and cytokines/chemokines (3). Together, these mediators have multiple effects on the lung causing smooth muscle constriction, mucus production, and edema (1).
Human mast cells are classically divided into the MCT and MCTC subsets depending on the expression of specific proteases. The MCT subset expresses tryptase and MCTC in addition expresses chymase, CPA3 and cathepsin G (4). This distinction has been made with immunohistochemical methods and little is known about the mRNA expression of the proteases at the single cell level. Moreover, the heterogeneity of human lung mast cells appears to be much more diverse, beyond protease expression, including the expression of receptors, enzymes and other markers in different compartments of the lung (5). Recently, using flow cytometry, we demonstrated that human lung mast cell heterogeneity has a continuous nature, rather than distinct populations, both in regard to various cell surface markers but also the classical heterogeneity markers chymase and CPA3 (6). Similarly, a recent scRNAseq study of human mast cells from nasal polyps in patients with chronic rhinosinusitis with nasal polyososis (CRSwNP) also found the heterogeneity of the mast cells to be of a continuous transient nature from MCT to MCTC, but the study also found a separate cluster of proliferative mast cells (7).
Several scRNAseq studies using droplet based techniques have been performed on lung tissue in different human diseases, including asthma (8), COPD (9, 10), COVID-19 (11) and lung cancer (12, 13), and a mast cell cluster has been detected in these studies. The mast cell cluster was shown to be enriched in asthma (8) and in one COPD study (9), but the same was not seen in a separate COPD study (10). However, these studies have not been focused on mast cells, and the heterogeneity of the mast cells have therefore not been examined.
In this study, we set out to investigate the heterogeneity of sorted human lung mast cells by scRNAseq using SmartSeq2, a method that provides deeper sequencing than the previous studies that have used droplet-based techniques.
Materials and methods
Ethical approval
The local ethics committee approved the collection of lung tissue from patients undergoing lobectomy, and all patients provided informed consent (Regionala Etikprövningsnämnden Stockholm, 2010/181-31/2).
Cell preparation
Lung tissue was obtained from patients undergoing lobectomy due to lung cancer (age 57–76 year old, three females, one male). All patients were ex smokers and none of them had preoperative chemo- or radiotherapy. Macroscopically healthy tissue distal to the tumour was used. Single-cell suspensions were obtained by digesting the tissue as previously described (14). Briefly, human lung tissue was cut into small pieces and enzymatically digested for 45 min with DNase I and collagenase. Thereafter, the tissue was mechanically disrupted by shearing with a syringe, debris was removed by 30% Percoll centrifugation and red blood cells lysed with an ACK buffer.
Flow cytometry analysis and cell sorting
For single cell sorting of mast cells the following antibodies were used: V500-CD45 (BD Biosciences, clone HI30), FITC- CD3 (Biolegend, clone SK7), FITC- CD19 (BD Biosciences, clone 4G7), FITC- CD14 (Dako, clone TUK4), FITC- CD1a (Biolegend, clone HI149), FITC- CD123 (Biolegend, clone 6H6), FITC- BDCA2 (Miltenyi Biotech, clone AC144), FITC- TCR α/β (Biolegend, clone IP26), FITC- TCR γ/δ (Biolegend, clone B1), FITC- CD94 (Biolegend, clone DX22), FITC- CD34 (Biolegend, clone 581), PECy5.5-CD117 (Beckman Coulter, clone 104D2D1), and Invitrogen Live/Dead™ Fixable Green Dead Cell Stain kit. FlowJo software was used for flow cytometry data analysis. The mast cells were gated as Live cells, Lineage negative (CD3, CD14, CD19, CD1a, CD123, BDCA2, TCRα/β, TCR γ/δ, CD94, CD34), CD45 positive and CD117 high.
Single cell RNA sequencing
The isolated human lung mast cells were single cell sorted into 384 well plate containing lysis buffer using a BD FACS Fusion. In total, 332 single mast cells were sorted from 4 lung tissues (83 mast cells per tissue).The quality of cDNA was assessed by agilent high sensitivity DNA assay as previously described (15). Single cell RNA sequencing (scRNA seq) libraries were prepared and sequenced using Smart-seq2 protocol (16) by the SciLifeLab Eukaryotic Single-cell Genomics Facility, Stockholm.
Data processing and analyses
Data processing and analyses was performed by the National Bioinformatics Infrastructure Sweden (NBIS) and the computations were performed on resources provided by SNIC through Uppsala Multidisciplinary Center for Advanced Computational Science (UPPMAX). Reads were aligned to the reference genome GRCh38 (primary assembly) using star/2.5.3a with default settings (17). Counts per gene (an arbitrary unit of the number or reads that were detected per gene) were calculated for each transcript using featureCounts from subread/1.5.2 using parameters -t exon -g gene_id (18).
The following analysis were performed using R version 3.6.3 (R Core Team 2020). Poor quality cells were excluded. Cells were considered of poor quality if they were outliers (defined using median absolute deviations (MADs)) in at least one of 6 criteria listed (1): cells with percentage of uniquely mapped reads with more than 3 MADs below the median (global median from all plates) (2) cells with percentage of uniquely mapped reads mapping to protein coding regions with more than 3 MADs below the median (global median from all plates) (3) cells with proportions of reads mapped to spike-in transcripts that are more than 3 MADs above the median (global median from all plates) (4) cells with proportions of mitochondrial reads that are more than 3 MADs above the median (global median from all plates) (5) cells with log-library sizes that are more than 3 MADs below the median log-library size of each plate (6) cells which log-transformed number of expressed genes that are more than 3 MADs below the median of each plate.
Genes with no expression (0 count across all mast cells) were removed as well as mitochondrial genes and rRNA genes. Counts were normalized using the logNormCounts function of the scater R/Bioconductor package version 1.14.6 where counts are divided by a cell-specific size factor (19).
Most analyzes were done separately on each plate so no batch correction was performed. The distribution of gene expression of selected marker genes (selected proteases, cell surface receptors and lipid metabolism genes) and the top 20 expressed genes was visualized using violin plots. For each plate, per-gene variance was modeled using spike-ins, highly variable genes across cells were selected using the scran Bioconductor package version 1.14.6 (20). Several dimensionality reduction methods were computed on highly variables genes on the single cell data for each plate separately. Principal Component Analysis (PCA) on the centered normalized log counts (the first 3 principal components were plotted). Uniform Manifold Approximation and Projection (UMAP) on the normalized log counts. t-Distributed Stochastic Neighbor Embedding (tSNE) on the normalized log counts with a perplexity parameter of 100.
The 2-by-2 correlations between CMA1, CPA3 and CTSG was assessed using scatter plots and Spearman’s rank correlation coefficients.
Results
scRNA sequencing of human lung mast cells
Human lung mast cell heterogeneity has been thoroughly examined using immunohistochemistry and flow cytometry (5, 6). However, an unbiased high throughput investigation into human lung mast cell heterogeneity has not been performed. We therefore set out to perform scRNAseq on sorted human lung mast cells. The mast cells were gated as Live Lin- CD45+ CD117high (Figure 1) and scRNAseq was performed using the Smart-seq2 protocol. Among the genes with the highest counts (Figures 2A, B, Supplementary Figure S1) were many known mast cell genes such as the receptor for stem cell factor KIT, that is needed for mast cell maturation and survival, the mast cell proteases tryptase (TPSAB1) and carboxypeptidase A3 (CPA3), the proteoglycan serglycin (SRGN) that is needed for the packing of biogenic amines (histamine, serotonin, dopamine) and proteases in the secretory granules (21–23), and histidine decarboxylase (HDC) that is the rate limiting enzyme in the biosynthesis of histamine. However, we also noted four cells that had low to undetectable level of several mast cell signature genes, including TPSAB1, TPSB2, CPA3, HDC and IL1RL1, indicating that these could be contaminating non-mast cells (data not shown).
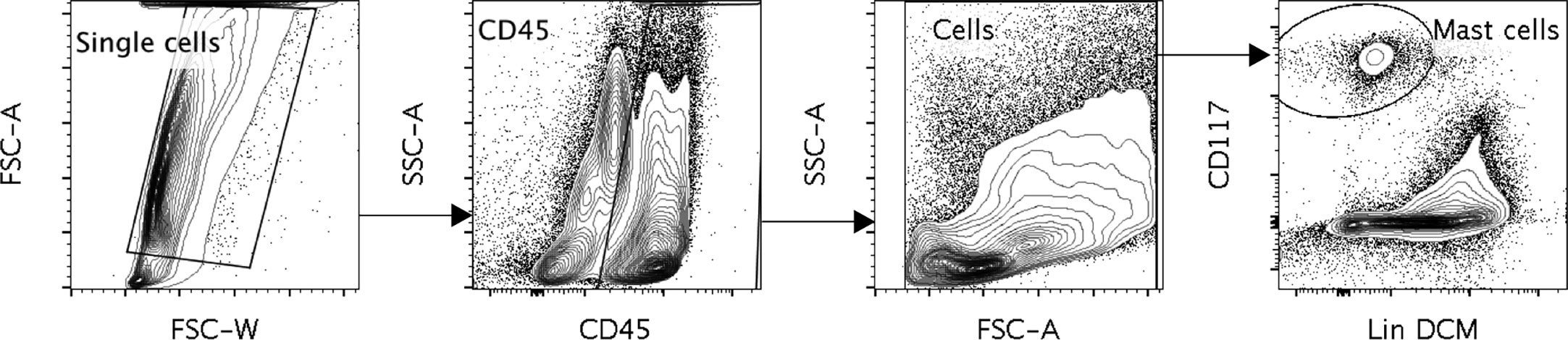
Figure 1 A representative figure of the gating of the human lung mast cells. Cells were gated as Single cells, CD45+, cells selected in the FSC, SSC pattern, CD117 high, dead cell marker (DCM) Low and Lineage (Lin) Low. The Lineage markers consisted of CD3, CD14, CD19, CD1a, CD123, BDCA2, TCRα/β, TCR γ/δ, CD94 and CD34.
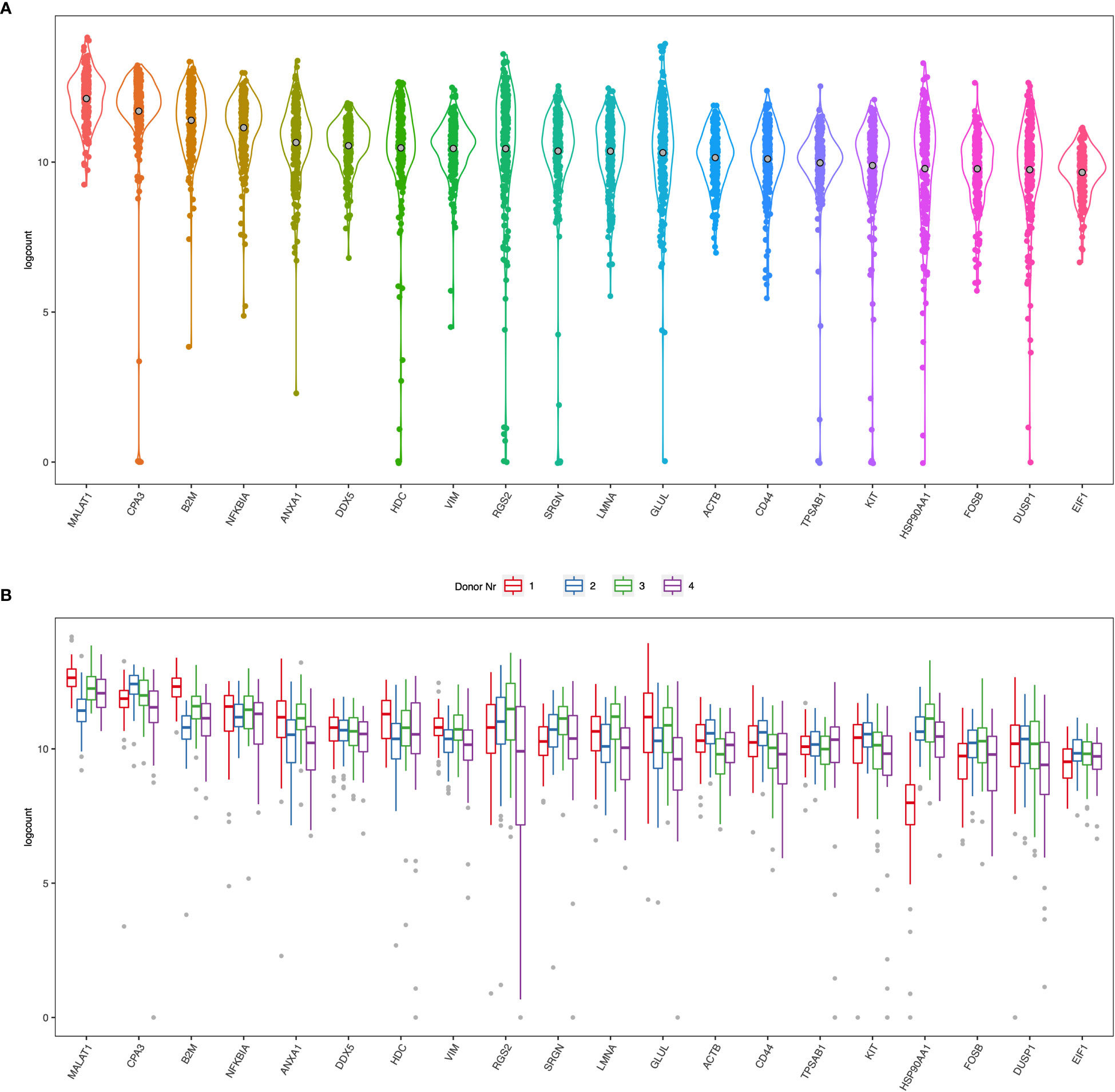
Figure 2 (A) Violin plots of the top 20 ranked genes in the dataset from all four donors (B) Boxplots of the top 20 ranked genes separated by each donor.
Many of the highest expressed genes were also involved in basic functions of cells and therefore highly expressed in most cells such as β2 microglobulin (B2M), β-actin (ACTB), vimentin (VIM), lamin (LMNA), glutamine synthase (GLUL). MALAT1 is a long non-coding RNA that is frequently high in poly-A captured RNA sequencing data (24, 25). There are also several genes involved in transcription and translation, such as transcription factors NFKBIA and FOSB, regulator of transcription and splicing DDX5, and regulator of translation initiation EIF1, regulator of the transcription complex and chaperone HSP90AA1. Annexin A1 (ANX1) is a protein that binds phospholipids and inhibits phospholipase A2. Synthesis and secretion of annexin A1 is enhanced by glucocorticoids (26, 27) and mast cell activation is inhibited by annexin A1 (28). Another highly expressed gene was CD44, a ubiquitously expressed adhesion molecule with several ligands, including hyaluronic acid, that previously has been shown to be expressed by human mast cells (29, 30).
Heterogenous expression of mast cell genes
Next, we specifically examined the heterogeneity of expression of selected mast-cell related genes. The cell surface receptors KIT and the IL-33 receptor ST2 (IL1RL1) were as expected high in most cells, while the two of the subunits of the FcεRI receptor (FCER1A and MS4A2) and CD203c (ENPP3) had a more diverse expression pattern (Figure 3). Of the enzymes involved in lipid metabolism, arachidonate 5-lipoxygenase (ALOX5, also known as 5-LOX) was highly detectable in most cells (Figure 3). 5-LOX converts arachidonic acid to leukotriene A4 (LTA4), that is further metabolized to LTB4 by LTA4 hydrolase (LTA4H) or to LTC4 by leukotriene C4 synthase (LTC4S). In the human lung mast cells LTA4H was only detectable in a small proportion of the cells, while LTC4S was detectable in a higher proportion of the cells and had a variable expression pattern (Figure 3). Both cyclooxygenases that converts arachidonic acid to prostaglandin endoperoxide H2 (a precursor for the prostaglandins D2, E2 and F2), prostaglandin-endoperoxide synthase 1 (PTGS1- also known as cyclooxygenase 1 (COX-1)) and PTGS2 (also known as COX-2) were variably expressed (Figure 3). Of the proteases, the tryptases (TPSAB1 and TPSB2) and CPA3 were high in most cells whereas chymase (CMA1) and Cathepsin G (CTSG) had a variable expression pattern (Figure 3).
No distinct mast cell subsets were detected
To examine possible subsets of human lung mast cells we used three different methods to cluster the cells based on the scRNAseq data: PCA, UMAP and tSNE plots. None of these three methods provided any obvious sub-clusters (one representative donor in Figure 4 and the additional three donor in Supplementary Figure S2). We noted that there were a few cells that positioned apart from the main cell cluster in two donors, these cells were mainly the suspected non- mast cells that had low to undectectable levels of the mast cell signature genes (Supplementary Figures S2A, C). To specifically examine the classical mast cell subsets MCT and MCTC, we examined the correlation in expression between the proteases that are present in the MCTC subset, that is chymase, CPA3 and cathepsin G. To the contrary of the protein (6), the CPA3 mRNA was highly detectable in all cells, and the correlation to CMA1 and CTSG was weak (Figure 5). In contrast, the chymase, CMA1 expression was only detected in a small fraction of the cells that also had relatively high CTSG expression, but on the other hand there where cells with high CTSG that did not have detectable CMA1 expression. Thus, CMA1 correlated moderately with that of CTSG with a Spearman’s rank correlation coefficient of 0.5 (Figure 5). CTSG and CMA1 did not show correlation (moderate or high, rs>0.4) to any other genes in the dataset (Supplementary Tables S1, S2).
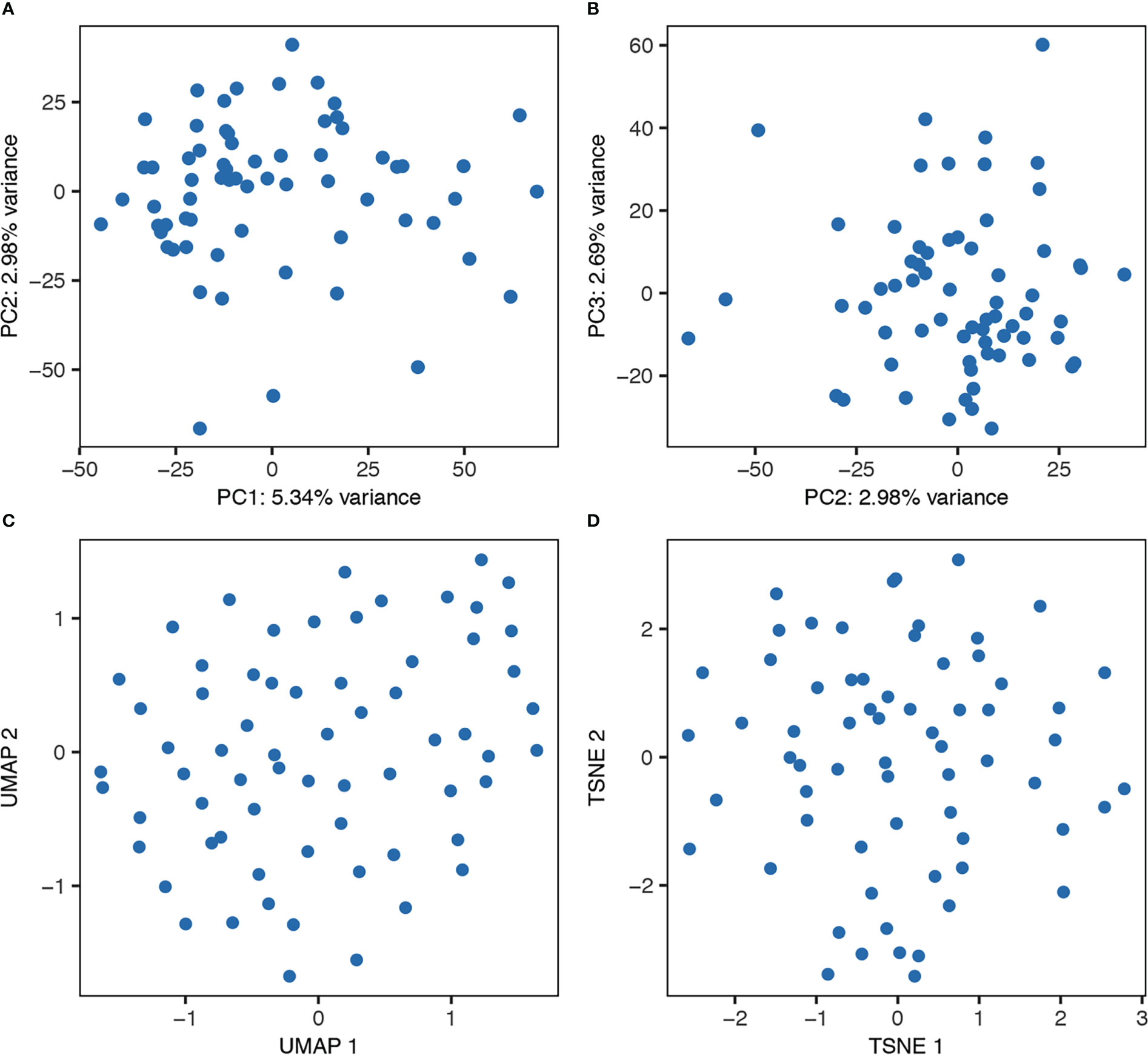
Figure 4 Unbiased clustering of the cells from one representative donor using PCA (A, B), UMAP (C), and tSNE (D).
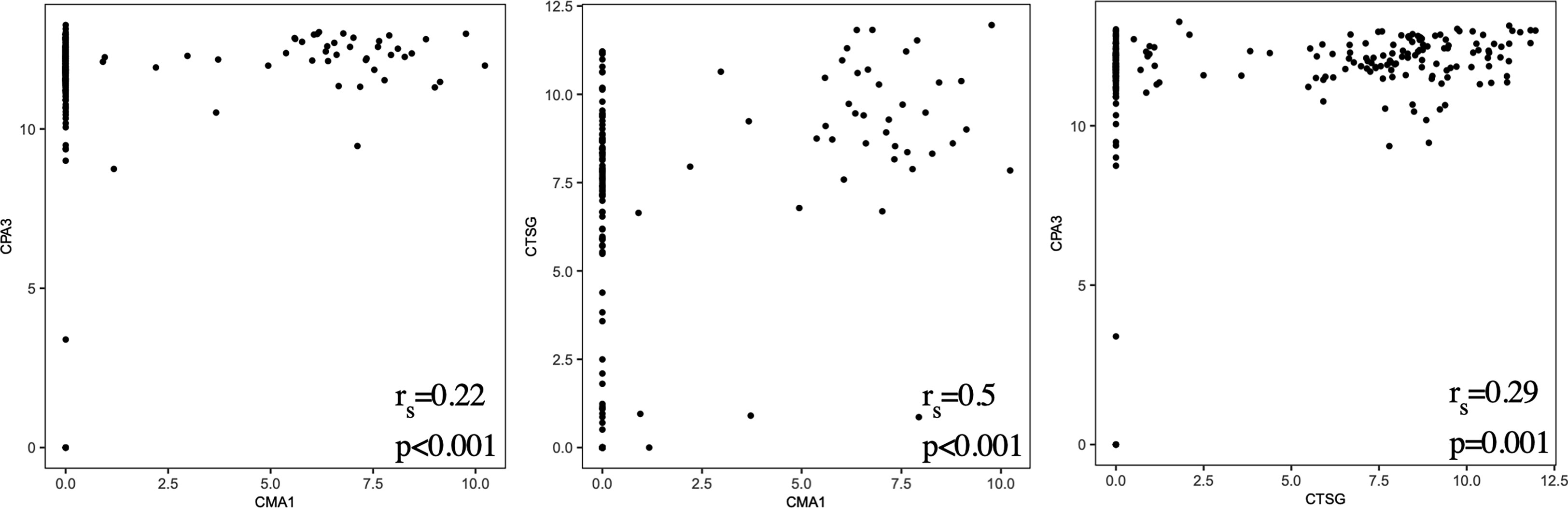
Figure 5 Scatter plots of CPA3/CMA1/CTSG expression from all four donors and Spearman’s rank correlation coefficients (rs).
Discussion
We have investigated the heterogeneity of human lung mast cells using SmartSeq2, a method that provides deep sequencing of single cells. Among the genes with the highest counts were several mast cell genes, including KIT, tryptase (TPSAB1), carboxypeptidase A3 (CPA3), serglycin (SRGN), histidine decarboxylase (HDC), verifying the identity of the cells as mast cells.
The expression of two subunits of the FcεRI receptor was shown to be variable (Figure 3), they did not however correlate to each other (data not shown). Variable surface expression of FcεRI has previously been reported on human lung mast cells as well as for human skin mast cells (6, 31). The expression of the FcεRI protein on mast cells has also been shown to vary between different lung compartments, i.e. central airways, small airways, parenchyma, and pulmonary vessels, with particularly low expression on the parenchymal mast cells (5). The low parenchymal expression of FcεRI in healthy subjects is, however, increased in patients with atopic uncontrolled asthma (32). The underlying mechanism for the varying FcεRI expression is not completely known. The FcεRI mRNA expression has been shown to be regulated by IL-4 (33) and IL-33 (34, 35), and the receptor is stabilized on the cell-surface by the presence of IgE (36), but other mechanisms might also be involved in the regulation.
The main prostanoid released from human mast cells upon activation is PGD2 (37–39), which have potent biological effects in the lung, such as broncoconstriction (40), vasodilation (41), and activation and recruitment of group 2 innate lymphoid cells (42). The first step in the biosynthesis of PGD2, is catalyzed by two enzymes COX-1 (PTGS1) and COX-2 (PTGS2). In our study both genes encoding for COX-1 and -2, PTGS1 and PTGS2, were variably expressed (Figure 3). However, the expression of the two genes did not correlate to each other (data not shown). Additionally, in nasal polyps, PTGS2 is enriched in the MCTC cluster (7), which we could not observe in HLMC. In human mast cells, PGD2 biosynthesis have been shown to be entirely dependent on COX-1 (37, 43), so, why PTGS2 (COX-2) is transcribed in these cells and under what conditions it is enzymatically active remains to be elucidated.
Human mast cells have been shown to be heterogeneous when it comes to the presence of proteases and thus divided into MCT and MCTC depending on their expression of chymase (CMA1). The MCTC subset has in addition also been shown to contain CPA3 and cathepsin G (44, 45). Human lung mast cells are predominantly of the MCT subtype, and consistent with this CMA1 was only detectable in a small proportion of the cells (Figures 3, 5). We found high expression of CPA3 mRNA in all lung mast cells and the correlation of this expression to CMA1 was weak (Figures 3, 5). In contrast, CPA3 and chymase proteins strongly correlate in human lung mast cells as judged by flow cytometry (6). This discrepancy of CPA3 mRNA and protein has also been shown in human lung mast cells using RNAscope and immunohistochemistry (46). When the gene for the equivalent to chymase in mice (based on sequence alignment), mouse mast cells protease- 5 (mMCP-5), is deleted the granule storage of CPA3 is lost, despite the CPA3 mRNA expression being unaffected (47). Similarly, if the CPA3 gene is deleted, the granule storage of the mMCP-5 protein is lost (48). However, when CPA3 is mutated to render it inactive without deletion of the CPA3 protein, the storage of mMCP-5 is unaffective (49). Thus, in mice mMCP-5 and CPA3 protein are interdependent on each other for their granule storage in mast cells. One can speculate that the same situation could be true for human CPA3, that chymase needs to be present for the CPA3 protease to be stored in the granules. Supporting this hypothesis is the finding that when comparing lung and skin mast cells, skin mast cells have higher expression of chymase and store more CPA3 protein in spite of expressing lower level of CPA3 mRNA. This theory does however raise the question; why do the cells express high levels of the mRNA if the protein is not stored? Is there a high CPA3 turnover or perhaps a continuous release of CPA3 in the MCT subtype? Further studies are needed to answer these questions.
The mast cells in this study comes from lobectomies performed on cancer patients. The tissue used was macroscopically healthy distal to the tumour, nevertheless it is of course possible that the disease has affected the included mast cells. In this context it is worth noting that CPA3 counts were high (Figure 3) and CPA3 expression has been shown to correlate to decreased lung function in patients with severe chronic obstructive pulmonary disease (COPD) and idiopathic lung fibrosis (IPF) (50).
The aim of the present study was to investigate human lung mast cell heterogeneity in more depth using SmartSeq2 single-cell RNA sequencing. However, we could not detect any obvious transcriptional different subpopulations of the human lung mast cells (Figure 4). There could be several explanations for this. The human lung mast cells could be homogenous in nature but it could also be that the number of mast cells analyzed are too low to distinguish small subsets. The facts that the mRNA of one of the proteases present in the MCTC subtype, CPA3, does not correlate to the protein, and that no other genes then CTSG show a correlation (rs>0.4) to CMA1, likely contribute to the inability to distinguish the classical mast cell subsets based on unbiased clustering. Furthermore, our study covered mainly mast cells in the parenchyma and it might be that mast cells in different compartments of the lung show greater heterogeneity. Thus, additional analyses including higher number of single mast cells from different lung compartments will be instrumental to decipher further the heterogeneity of human lung mast cells.
Data availability statement
The data presented in the study are deposited in the Gene Expression Omnibus (GEO) repository, accession number GSE227712.
Ethics statement
The studies involving human participants were reviewed and approved by Regionala Etikprövningsnämden i Stockholm. The patients/participants provided their written informed consent to participate in this study.
Author contributions
GN, JM, AR, ER, and JD, conceived and designed the studies. AR, and LM, performed the experiments. JL, OD, YG, ER, and JD analyzed the data. JS, A-CO, MA-A, MA and S-ED provided samples. ER, AR and GN wrote the manuscript draft. All authors contributed to the article and approved the submitted version.
Funding
This study was supported by grants from the Swedish Research Council (2018-02070, 2020-01693, and 2022-00558); the Heart-Lung Foundation; The Swedish Cancer Society; the Ollie and Elof Ericssons Foundation, the Ellen, Walter and Lennart Hesselman Foundation; the Tore Nilsson Foundation; the Lars Hierta Memorial Foundation; the Konsul Th C Bergh Foundation; the Tornspiran Foundation; the O. E. and Edla Johanssons Foundation; the Swedish Society for Medical Research; The Magnus Bergvall Foundation; The Åke Wiberg Foundation; The Centre for Allergy Research Highlights Asthma Markers of Phenotype (ChAMP) consortium funded by the Swedish Foundation for Strategic Research; the AstraZeneca & Science for Life Laboratory Joint Research Collaboration; the Vårdal Foundation and the Karolinska Institutet. The authors declare that this study received funding from AstraZeneca. The funder was not involved in the study design, collection, analysis, interpretation of data, the writing of this article, or the decision to submit it for publication.
Acknowledgments
We would like to acknowledge support from National Bioinformatics Infrastructure Sweden (NBIS), Science for Life Laboratory, the National Genomics Infrastructure (NGI), Swedish National Infrastructure for Computing (SNIC) and Uppsala Multidisciplinary Center for Advanced Computational Science (UPPMAX) for providing assistance in bioinformatics, massively parallel sequencing analysis and computational infrastructure.
Conflict of interest
S-ED reports personal fees from AstraZeneca, Cayman Chemicals, GSK, Novartis, Regeneron, Sanofi, and Teva, for consultancies outside the submitted work.
The remaining authors declare that the research was conducted in the absence of any commercial or financial relationships that could be construed as a potential conflict of interest.
Publisher’s note
All claims expressed in this article are solely those of the authors and do not necessarily represent those of their affiliated organizations, or those of the publisher, the editors and the reviewers. Any product that may be evaluated in this article, or claim that may be made by its manufacturer, is not guaranteed or endorsed by the publisher.
Supplementary material
The Supplementary Material for this article can be found online at: https://www.frontiersin.org/articles/10.3389/fimmu.2023.1151754/full#supplementary-material
References
1. Bradding P, Arthur G. Mast cells in asthma–state of the art. Clin Exp Allergy (2016) 46(2):194–263. doi: 10.1111/cea.12675
2. Erjefalt JS. Mast cells in human airways: The culprit? Eur Respir Rev (2014) 23(133):299–307. doi: 10.1183/09059180.00005014
3. Dahlin JS, Maurer M, Metcalfe DD, Pejler G, Sagi-Eisenberg R, Nilsson G. The ingenious mast cell: Contemporary insights into mast cell behavior and function. Allergy (2021) 77(1):83–99. doi: 10.1111/all.14881
4. Irani AA, Schechter NM, Craig SS, DeBlois G, Schwartz LB. Two types of human mast cells that have distinct neutral protease compositions. Proc Natl Acad Sci U.S.A. (1986) 83(12):4464–8. doi: 10.1073/pnas.83.12.4464
5. Andersson CK, Mori M, Bjermer L, Lofdahl CG, Erjefalt JS. Novel site-specific mast cell subpopulations in the human lung. Thorax (2009) 64(4):297–305. doi: 10.1136/thx.2008.101683
6. Ronnberg E, Boey DZH, Ravindran A, Safholm J, Orre AC, Al-Ameri M, et al. Immunoprofiling reveals novel mast cell receptors and the continuous nature of human lung mast cell heterogeneity. Front Immunol (2021) 12:804812. doi: 10.3389/fimmu.2021.804812
7. Dwyer DF, Ordovas-Montanes J, Allon SJ, Buchheit KM, Vukovic M, Derakhshan T, et al. Human airway mast cells proliferate and acquire distinct inflammation-driven phenotypes during type 2 inflammation. Sci Immunol (2021) 6(56):eabb7221. doi: 10.1126/sciimmunol.abb7221
8. Vieira Braga FA, Kar G, Berg M, Carpaij OA, Polanski K, Simon LM, et al. A cellular census of human lungs identifies novel cell states in health and in asthma. Nat Med (2019) 25(7):1153–63. doi: 10.1038/s41591-019-0468-5
9. Sauler M, McDonough JE, Adams TS, Kothapalli N, Barnthaler T, Werder RB, et al. Characterization of the copd alveolar niche using single-cell rna sequencing. Nat Commun (2022) 13(1):494. doi: 10.1038/s41467-022-28062-9
10. Li X, Noell G, Tabib T, Gregory AD, Trejo Bittar HE, Vats R, et al. Single cell rna sequencing identifies Igfbp5 and qki as ciliated epithelial cell genes associated with severe copd. Respir Res (2021) 22(1):100. doi: 10.1186/s12931-021-01675-2
11. Delorey TM, Ziegler CGK, Heimberg G, Normand R, Yang YM, Segerstolpe A, et al. Covid-19 tissue atlases reveal sars-Cov-2 pathology and cellular targets. Nature (2021) 595(7865):107. doi: 10.1038/s41586-021-03570-8
12. Wu F, Fan J, He Y, Xiong A, Yu J, Li Y, et al. Single-cell profiling of tumor heterogeneity and the microenvironment in advanced non-small cell lung cancer. Nat Commun (2021) 12(1):2540. doi: 10.1038/s41467-021-22801-0
13. Lu T, Yang X, Shi Y, Zhao M, Bi G, Liang J, et al. Single-cell transcriptome atlas of lung adenocarcinoma featured with ground glass nodules. Cell Discovery (2020) 6:69. doi: 10.1038/s41421-020-00200-x
14. Ravindran A, Ronnberg E, Dahlin JS, Mazzurana L, Safholm J, Orre AC, et al. An optimized protocol for the isolation and functional analysis of human lung mast cells. Front Immunol (2018) 9:2193. doi: 10.3389/fimmu.2018.02193
15. Bjorklund AK, Forkel M, Picelli S, Konya V, Theorell J, Friberg D, et al. The heterogeneity of human Cd127(+) innate lymphoid cells revealed by single-cell rna sequencing. Nat Immunol (2016) 17(4):451–60. doi: 10.1038/ni.3368
16. Picelli S, Faridani OR, Bjorklund AK, Winberg G, Sagasser S, Sandberg R. Full-length rna-seq from single cells using smart-Seq2. Nat Protoc (2014) 9(1):171–81. doi: 10.1038/nprot.2014.006
17. Dobin A, Davis CA, Schlesinger F, Drenkow J, Zaleski C, Jha S, et al. Star: Ultrafast universal rna-seq aligner. Bioinformatics (2013) 29(1):15–21. doi: 10.1093/bioinformatics/bts635
18. Liao Y, Smyth GK, Shi W. The subread aligner: Fast, accurate and scalable read mapping by seed-and-Vote. Nucleic Acids Res (2013) 41(10):e108. doi: 10.1093/nar/gkt214
19. McCarthy DJ, Campbell KR, Lun AT, Wills QF. Scater: Pre-processing, quality control, normalization and visualization of single-cell rna-seq data in r. Bioinformatics (2017) 33(8):1179–86. doi: 10.1093/bioinformatics/btw777
20. Lun AT, McCarthy DJ, Marioni JC. A step-by-Step workflow for low-level analysis of single-cell rna-seq data with bioconductor. F1000Res (2016) 5:2122. doi: 10.12688/f1000research.9501.2
21. Ronnberg E, Calounova G, Pejler G. Mast cells express tyrosine hydroxylase and store dopamine in a serglycin-dependent manner. Biol Chem (2012) 393(1-2):107–12. doi: 10.1515/BC-2011-220
22. Ringvall M, Ronnberg E, Wernersson S, Duelli A, Henningsson F, Abrink M, et al. Serotonin and histamine storage in mast cell secretory granules is dependent on serglycin proteoglycan. J Allergy Clin Immunol (2008) 121(4):1020–6. doi: 10.1016/j.jaci.2007.11.031
23. Abrink M, Grujic M, Pejler G. Serglycin is essential for maturation of mast cell secretory granule. J Biol Chem (2004) 279(39):40897–905. doi: 10.1074/jbc.M405856200
24. Aissa AF, Islam A, Ariss MM, Go CC, Rader AE, Conrardy RD, et al. Single-cell transcriptional changes associated with drug tolerance and response to combination therapies in cancer. Nat Commun (2021) 12(1):1628. doi: 10.1038/s41467-021-21884-z
25. Zhang B, Arun G, Mao YS, Lazar Z, Hung G, Bhattacharjee G, et al. The lncrna Malat1 is dispensable for mouse development but its transcription plays a cis-regulatory role in the adult. Cell Rep (2012) 2(1):111–23. doi: 10.1016/j.celrep.2012.06.003
26. Hannon R, Croxtall JD, Getting SJ, Roviezzo F, Yona S, Paul-Clark MJ, et al. Aberrant inflammation and resistance to glucocorticoids in annexin 1-/- mouse. FASEB J (2003) 17(2):253–5. doi: 10.1096/fj.02-0239fje
27. Peers SH, Smillie F, Elderfield AJ, Flower RJ. Glucocorticoid-and non-glucocorticoid induction of lipocortins (Annexins) 1 and 2 in rat peritoneal leucocytes in vivo. Br J Pharmacol (1993) 108(1):66–72. doi: 10.1111/j.1476-5381.1993.tb13441.x
28. Sinniah A, Yazid S, Bena S, Oliani SM, Perretti M, Flower RJ. Endogenous annexin-A1 negatively regulates mast cell-mediated allergic reactions. Front Pharmacol (2019) 10:1313. doi: 10.3389/fphar.2019.01313
29. Valent P, Majdic O, Maurer D, Bodger M, Muhm M, Bettelheim P. Further characterization of surface membrane structures expressed on human basophils and mast cells. Int Arch Allergy Appl Immunol (1990) 91(2):198–203. doi: 10.1159/000235115
30. Girodet PO, Ozier A, Trian T, Begueret H, Ousova O, Vernejoux JM, et al. Mast cell adhesion to bronchial smooth muscle in asthma specifically depends on Cd51 and Cd44 variant 6. Allergy (2010) 65(8):1004–12. doi: 10.1111/j.1398-9995.2009.02308.x
31. Babina M, Guhl S, Artuc M, Trivedi NN, Zuberbier T. Phenotypic variability in human skin mast cells. Exp Dermatol (2016) 25(6):434–9. doi: 10.1111/exd.12924
32. Andersson CK, Bergqvist A, Mori M, Mauad T, Bjermer L, Erjefalt JS. Mast cell-associated alveolar inflammation in patients with atopic uncontrolled asthma. J Allergy Clin Immunol (2011) 127(4):905–12 e1-7. doi: 10.1016/j.jaci.2011.01.022
33. Toru H, Ra C, Nonoyama S, Suzuki K, Yata J, Nakahata T. Induction of the high-affinity ige receptor (Fc epsilon ri) on human mast cells by il-4. Int Immunol (1996) 8(9):1367–73. doi: 10.1093/intimm/8.9.1367
34. Ronnberg E, Ghaib A, Ceriol C, Enoksson M, Arock M, Safholm J, et al. Divergent effects of acute and prolonged interleukin 33 exposure on mast cell ige-mediated functions. Front Immunol (2019) 10:1361. doi: 10.3389/fimmu.2019.01361
35. Babina M, Wang Z, Franke K, Guhl S, Artuc M, Zuberbier T. Yin-yang of il-33 in human skin mast cells: Reduced degranulation, but augmented histamine synthesis through P38 activation. J Invest Dermatol (2019) 139(7):1516–25.e3. doi: 10.1016/j.jid.2019.01.013
36. Kraft S, Kinet JP. New developments in fcepsilonri regulation, function and inhibition. Nat Rev Immunol (2007) 7(5):365–78. doi: 10.1038/nri2072
37. Johnsson AK, Choi JH, Ronnberg E, Fuchs D, Kolmert J, Hamberg M, et al. Selective inhibition of prostaglandin D2 biosynthesis in human mast cells to overcome need for multiple receptor antagonists: Biochemical consequences. Clin Exp Allergy (2021) 51(4):594–603. doi: 10.1111/cea.13831
38. Lewis RA, Soter NA, Diamond PT, Austen KF, Oates JA, Roberts LJ 2nd. Prostaglandin D2 generation after activation of rat and human mast cells with anti-ige. J Immunol (1982) 129(4):1627–31. doi: 10.4049/jimmunol.129.4.1627
39. Peters SP, MacGlashan DW Jr., Schulman ES, Schleimer RP, Hayes EC, Rokach J, et al. Arachidonic acid metabolism in purified human lung mast cells. J Immunol (1984) 132(4):1972–9. doi: 10.4049/jimmunol.132.4.1972
40. Hardy CC, Robinson C, Tattersfield AE, Holgate ST. The bronchoconstrictor effect of inhaled prostaglandin D2 in normal and asthmatic men. N Engl J Med (1984) 311(4):209–13. doi: 10.1056/NEJM198407263110401
41. Alving K, Matran R, Lundberg JM. The possible role of prostaglandin D2 in the long-lasting airways vasodilatation induced by allergen in the sensitized pig. Acta Physiol Scand (1991) 143(1):93–103. doi: 10.1111/j.1748-1716.1991.tb09204.x
42. Xue L, Salimi M, Panse I, Mjosberg JM, McKenzie AN, Spits H, et al. Prostaglandin D2 activates group 2 innate lymphoid cells through chemoattractant receptor-homologous molecule expressed on Th2 cells. J Allergy Clin Immunol (2014) 133(4):1184–94. doi: 10.1016/j.jaci.2013.10.056
43. Baothman BK, Smith J, Kay LJ, Suvarna SK, Peachell PT. Prostaglandin D2 generation from human lung mast cells is catalysed exclusively by cyclooxygenase-1. Eur J Pharmacol (2018) 819:225–32. doi: 10.1016/j.ejphar.2017.12.005
44. Schechter NM, Irani AM, Sprows JL, Abernethy J, Wintroub B, Schwartz LB. Identification of a cathepsin G-like proteinase in the mctc type of human mast cell. J Immunol (1990) 145(8):2652–61. doi: 10.4049/jimmunol.145.8.2652
45. Irani AM, Goldstein SM, Wintroub BU, Bradford T, Schwartz LB. Human mast cell carboxypeptidase. Selective Localization to Mctc Cells J Immunol (1991) 147(1):247–53.
46. Siddhuraj P, Clausson CM, Sanden C, Alyamani M, Kadivar M, Marsal J, et al. Lung mast cells have a high constitutive expression of carboxypeptidase A3 mrna that is independent from granule-stored Cpa3. Cells (2021) 10(2):309. doi: 10.3390/cells10020309
47. Stevens RL, McNeil HP, Wensing LA, Shin K, Wong GW, Hansbro PM, et al. Experimental arthritis is dependent on mouse mast cell protease-5. J Biol Chem (2017) 292(13):5392–404. doi: 10.1074/jbc.M116.773416
48. Feyerabend TB, Hausser H, Tietz A, Blum C, Hellman L, Straus AH, et al. Loss of histochemical identity in mast cells lacking carboxypeptidase a. Mol Cell Biol (2005) 25(14):6199–210. doi: 10.1128/MCB.25.14.6199-6210.2005
49. Schneider LA, Schlenner SM, Feyerabend TB, Wunderlin M, Rodewald HR. Molecular mechanism of mast cell mediated innate defense against endothelin and snake venom sarafotoxin. J Exp Med (2007) 204(11):2629–39. doi: 10.1084/jem.20071262
Keywords: mast cells (MC), single cell RNA sequencing, lung, heterogeniety, chymase (CMA1), cathepsin G (CTSG), carboxypeptidase A3 (CPA3)
Citation: Rönnberg E, Ravindran A, Mazzurana L, Gong Y, Säfholm J, Lorent J, Dethlefsen O, Orre A-C, Al-Ameri M, Adner M, Dahlén S-E, Dahlin JS, Mjösberg J and Nilsson G (2023) Analysis of human lung mast cells by single cell RNA sequencing. Front. Immunol. 14:1151754. doi: 10.3389/fimmu.2023.1151754
Received: 26 January 2023; Accepted: 15 March 2023;
Published: 30 March 2023.
Edited by:
Ulrich Blank, Institut National de la Santé et de la Recherche Médicale (INSERM), FranceReviewed by:
Margarita Martin, University of Barcelona, SpainNadine Varin-Blank, Institut National de la Santé et de la Recherche Médicale (INSERM), France
Copyright © 2023 Rönnberg, Ravindran, Mazzurana, Gong, Säfholm, Lorent, Dethlefsen, Orre, Al-Ameri, Adner, Dahlén, Dahlin, Mjösberg and Nilsson. This is an open-access article distributed under the terms of the Creative Commons Attribution License (CC BY). The use, distribution or reproduction in other forums is permitted, provided the original author(s) and the copyright owner(s) are credited and that the original publication in this journal is cited, in accordance with accepted academic practice. No use, distribution or reproduction is permitted which does not comply with these terms.
*Correspondence: Elin Rönnberg, elin.ronnberg.hockerlind@ki.se; Gunnar Nilsson, gunnar.p.nilsson@ki.se