- 1Department of Dermatology, University of California San Diego, La Jolla, CA, United States
- 2Department of Pediatrics, University of California San Diego, La Jolla, CA, United States
- 3Biomedical Sciences Graduate Program, University of California San Diego, La Jolla, CA, United States
- 4Department of Computer Science and Engineering, University of California San Diego, La Jolla, CA, United States
- 5Department of Bioengineering, University of California San Diego, La Jolla, CA, United States
- 6Center for Microbiome Innovation, University of California San Diego, La Jolla, CA, United States
Despite its harsh and dry environment, human skin is home to diverse microbes, including bacteria, fungi, viruses, and microscopic mites. These microbes form communities that may exist at the skin surface, deeper skin layers, and within microhabitats such as the hair follicle and sweat glands, allowing complex interactions with the host immune system. Imbalances in the skin microbiome, known as dysbiosis, have been linked to various inflammatory skin disorders, including atopic dermatitis, acne, and psoriasis. The roles of abundant commensal bacteria belonging to Staphylococcus and Cutibacterium taxa and the fungi Malassezia, where particular species or strains can benefit the host or cause disease, are increasingly appreciated in skin disorders. Furthermore, recent research suggests that the interactions between microorganisms and the host’s immune system on the skin can have distant and systemic effects on the body, such as on the gut and brain, known as the “skin-gut” or “skin-brain” axes. Studies on the microbiome in skin disease have typically relied on 16S rRNA gene sequencing methods, which cannot provide accurate information about species or strains of microorganisms on the skin. However, advancing technologies, including metagenomics and other functional ‘omic’ approaches, have great potential to provide more comprehensive and detailed information about the skin microbiome in health and disease. Additionally, inter-species and multi-kingdom interactions can cause cascading shifts towards dysbiosis and are crucial but yet-to-be-explored aspects of many skin disorders. Better understanding these complex dynamics will require meta-omic studies complemented with experiments and clinical trials to confirm function. Evolving how we profile the skin microbiome alongside technological advances is essential to exploring such relationships. This review presents the current and emerging methods and their findings for profiling skin microbes to advance our understanding of the microbiome in skin disease.
1 Introduction
Human skin acts as a substrate for an ecosystem of diverse life. Serving as our bodies’ physical barrier and largest organ, human skin is home to millions of bacteria, fungi, viruses, and microscopic mites, which collectively compose the “skin microbiome.” Many studies recognize that the combined genomes from the host and microbes, also known as the “hologenome,” determine the complete organism’s health and function. Although human skin is colonized mainly by beneficial microorganisms that share in maintaining skin metabolic processes and act as a frontline defense at our body’s external interface, they and their host all operate in a delicate balance. Microbial dysbiosis, a general term for the disturbed or abnormal distribution, composition, or relative abundance of microorganisms, can influence various local and systemic disease conditions. For example, in dermal layers of the skin, microbes and their gene products directly interact with the host immune system, thereby modulating general skin health (1, 2). Furthermore, growing evidence suggests that microbes from one barrier tissue can alter the activity of distant organ sites between the skin, gut, and brain (3, 4). Thus, skin microbiome studies have the potential to significantly aid our understanding of inflammatory skin disorders (such as atopic dermatitis and psoriasis) and influence wide-ranging pathologies in the same manner that research on the gut microbiome has advanced scientific knowledge of digestive and digestive-related disorders.
Extrinsic and intrinsic differences in skin anatomy and the activity of local cell types drive the variations of both normal and dysbiotic microbial skin communities. As the external barrier from the body, it is constantly bombarded by harsh environmental forces such as water/sun/pollutant exposure, temperature and pH fluctuations, and behavioral influences resulting from hygiene or beauty practices. These constantly changing variables challenge the skin’s ability to adapt and maintain stable and benign/healthy microbiome populations (particularly at the epidermal surface). Additionally, given that skin is our interface, it is important to distinguish the difference between members of the skin microbiome (resident to the skin and holding important functional roles) versus those found on the skin with a transient membership (likely from the environment). Compared to most human microbiomes, the skin’s environment is harsh, arid, and of lower nutrient availability. Thus, by nature, the skin’s ecological habitat limits colonization to a comparatively less diverse subset of organismal taxa than other human microbiome ecosystems, such as the gut. Yet, despite these factors, human skin is host to distinctly different and commonly found microbes belonging to multiple kingdoms (i.e., bacteria, fungi, viruses, etc.) with complex and dynamic community relationships. These resident microorganisms are well-equipped to adapt to the skin, often settling in their preferred niches.
The complexity of human skin and its innate ability to encourage microbial niche specialization can be considered by two major interlinking features. Firstly, skin exhibits a three-dimensional structure akin to a geographical landscape. The average person’s skin surface area is approximately 25 m2, making it the largest epithelial surface of the human body, with great opportunity for host-microbe interactions (5). Furthermore, skin thickness varies depending on the body site (from approximately 0.5 mm on the eyelids to 1.5-2.0 mm on the face and 4.0 mm on the heels of the feet). Unlike the intestine, where microbes are typically separated from direct interaction with the body by an antimicrobial mucus layer (6), host cells (including those of the immune system) are in direct contact with microbes, especially those that inhabit deeper dermal layers. Secondly, beneath the surface, the skin contains a complex topology of hair follicles and eccrine, apocrine, and sebaceous glands. Similar to the villous epithelium of the human gut, the skin has approximately five million hair follicles (pores) and sweat ducts whose concave structure and depth significantly increase the complexity and diversity of the skin. The densities of these micro-organs make for skin microenvironments to be broadly grouped into three categories: sebaceous/oily (forehead, scalp, chest, and back), moist/humid (skin around nose and mouth, underarms, elbow bend, abdominal, lower buttocks, back of the knee, and foot), and dry (forearms, back of the elbow, buttocks, and front of the knee/legs) (7), although far more fine-scale variation exists on human skin (8). While these features are universal to the skin, their ability to influence temporal and interpersonal variation creates even more microscale diversity and, thus, microbial community membership patterns (9).
Since the introduction of sequencing-based genetic analysis techniques for identifying microorganisms, there has been a significant increase in our understanding of microbial life on the skin. As evolving genomic technologies have been refined to produce richer microbial datasets, so have the accompanying analytical methods appropriate for skin microbiome studies. This review will discuss new and improved practices for analyzing complex microbial communities on human skin. First, we will overview the current status of genomic and metagenomic-based tools and highlight the emerging statistical and computational methods for analyzing microbiome data while discussing their application to healthy and diseased skin research. Next, we will complement our discussion of current profiling practices with the challenges in exploring microbial communities on the skin. The following section will focus on our current knowledge of the healthy skin microbiome from present studies, given our available tools and their known limitations. Finally, we provide perspectives on a few inflammatory skin diseases, summarizing what we know about the skin microbiome’s role and suggesting opportunities for future research.
2 Toolbox for surveying microbial skin communities
As recently as twenty years ago, culture-based approaches were the only method of exploring microbial communities on human skin (or in any organ) (Figure 1). Growing microorganisms sampled from the skin provided critical insights into the skin’s microbial ecosystem. These early pioneering studies identified the major bacterial genera on the skin, including Staphylococcus, Cutibacterium (formerly Propionibacterium), Corynebacterium, and fungi such as Malassezia, and built the foundation of skin microbiome explorations today (4). However, this practice had a significant limitation; culturing microorganisms in vitro inevitably provided biased assessments of microbial diversity. Microbes that grow readily in standard growth conditions overwhelmed those with more fastidious growth conditions, which led to a skewed representation of the community. For example, Staphylococcus species are more easily cultured, which led to the underestimation of Cutibacterium and Corynebacterium populations (10). Furthermore, the culture-based approach limited our window to microbes that we knew how to culture or that persistently survived after removal from the skin, meaning that some could be missed and remain functionally invisible. As technologies for DNA sequencing improved and became more accessible, culture-independent methods, including amplicon-based and metagenomic sequencing, have become the conventional practice for profiling the skin microbiome (Figure 1).
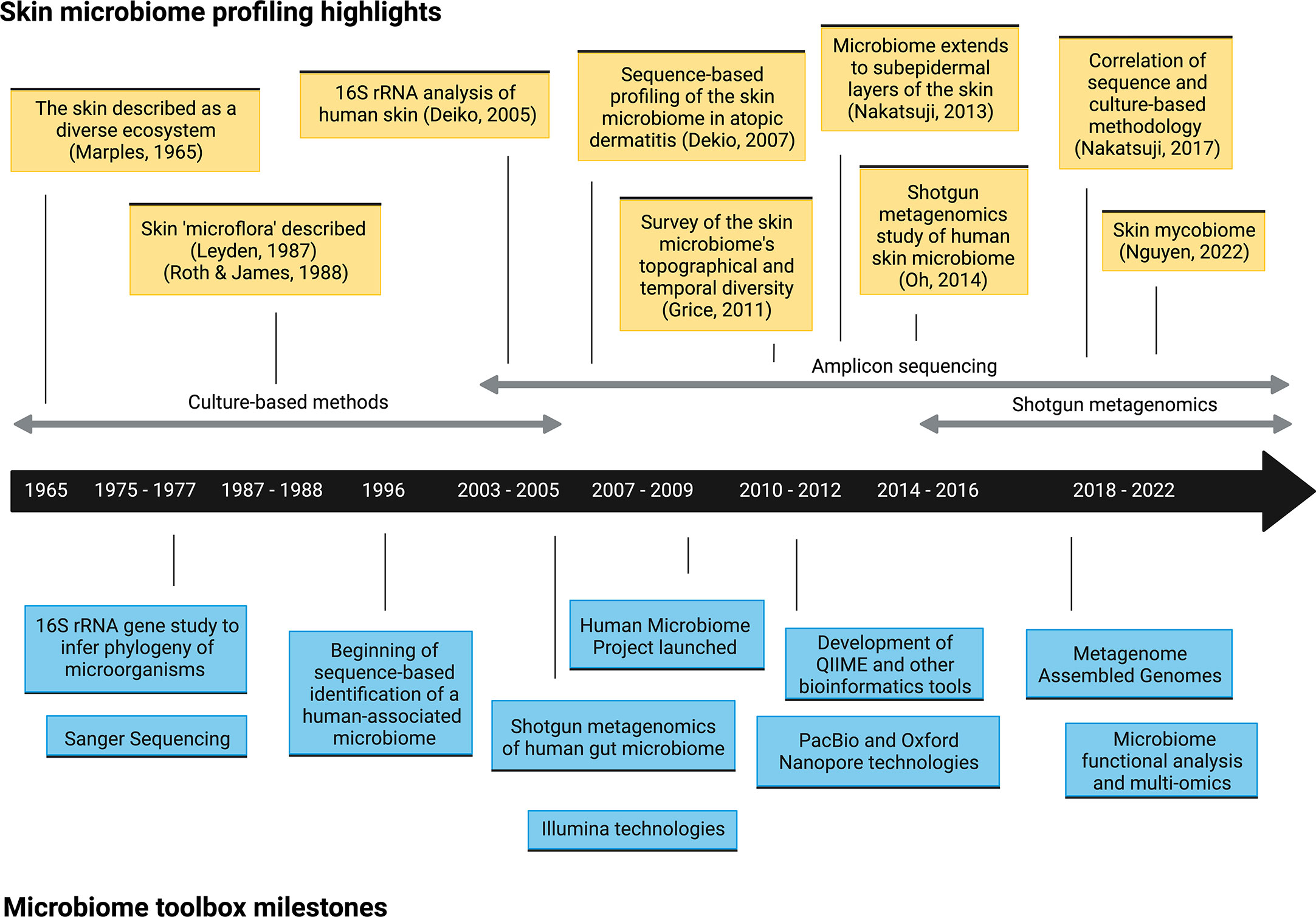
Figure 1 Timeline of selected microbiome toolbox milestones and skin microbiome profiling highlights.
2.1 Amplicon-based sequencing and analysis
Many studies that survey bacterial skin communities in healthy and diseased states continue to employ targeted amplification, sequencing, and analysis of sampled genetic material to identify microorganisms. This method involves amplifying and sequencing conserved taxonomic markers within prokaryotic kingdoms to resolve genetic variation and inform the evolutionary relationships between organisms. Most of this work has focused on the 16S ribosomal RNA gene (16S rRNA) in bacteria, which continues to be the de facto standard for microbial typing, given its ability to identify bacterial taxa. For fungi, the first internal transcribed spacer (ITS1) is the preferred taxonomic marker, whereas 18S rRNA (while still commonly utilized) is far less precise and offers poor discriminatory ability (11, 12). Unlike bacteria and fungi, viruses do not have a common marker gene and are difficult to characterize by amplicon methods.
Although an important and useful approach, amplicon sequencing techniques require careful methodological choices based on prior assumptions regarding the microorganisms in the sample collection. In order to facilitate sequencing of the full-length 16S gene using shorter-read DNA sequencing technologies, which are often limited in their ability to handle sequences of the gene's full length (~1500 base pairs), most protocols utilize specific locations within the 16S RNA gene known as hypervariable regions (V1-V9). These regions are the most variable between taxa and are situated between conserved regions that can function as PCR primers (13). Because hypervariable areas hold the highest nucleotide variability within 16S, targeting them using specific primers and in different combinations minimizes sequencing cost and effort by capturing the most informative taxon-specific variation in a short sequence (14, 15). Certain specific primer pairs have been more widely employed for different environments, and these primers are most often designed to amplify the V1-V3 or V3-V5 regions. While an “optimal” hypervariable region is not necessarily definitive for different environments, PCR primers designed for different broad-level ecosystems are common, as some regions have been deemed more suitable than others based on microbiome type. For example, the Human Microbiome Project has mainly used primers spanning the V1-V3 and V3-V5 hypervariable regions (16), while the Earth Microbiome Project has traditionally used primers targeting the V4 region (17).
Next, the sequence fragments are read, most popularly on the Illumina platform or using long-read technology via the PacBio or Oxford Nanopore platforms. A crucial subsequent step involves a bioinformatics-based process to remove spurious sequencing errors and merge reads with the same genetic signals. Historically, the most widely employed method was to cluster sequences into Operational Taxonomic Units (OTUs) that share pairwise sequence similarity (often at a 97% probability threshold) into a representative proxy used for downstream analysis (18). However, this method should not be used today. Improved computational methods, known collectively as oligotyping or grouping into Amplicon Sequence Variants (ASVs) or sub-OTUs (sOTUs), provide substantial improvements relative to OTU picking (19). In contrast to OTU clustering, oligotyping includes positional information from sequencing data by making use of the fact that biologically significant variation is more common at specific positions, whereas sequencing errors are randomly distributed. ASVs use a related approach, assuming that rare variants are often sequencing errors of common members in the population. These approaches resolve more biologically relevant groups at higher discriminatory power. Highly cited bioinformatics tools that are OTU-based include QIIME (20) and Mothur (21), while popular ASV-based tools include QIIME2-Deblur (22, 23) and DADA2 (24). Following OTU or ASV-based analysis, a “feature by sample” OTU or ASV table, providing the count of each feature in each sample, is generated and used for taxonomic assignment. QIIME2 and Mothur both support taxonomic profiling, relying on reference databases. Popular taxonomy databases include Silva (25), Greengenes (26), and RDP (27). Finally, functional profiling using PICRUSt (28) or Tax4Fun (29) can link taxonomic data such as that obtained from marker genes to the predicted biological function of taxa within a community. The significant advances in computational methods of 16S and other marker-gene techniques have greatly improved the accuracy of amplicon-based analyses; thus, some of the first conclusions published regarding the identity of bacterial and fungal species on the skin are no longer deemed accurate or of sufficient resolution, underscoring the importance of making the raw data from studies available for periodic re-analyzation with better techniques.
2.2 Shotgun metagenomics and analysis
Shotgun metagenomics, or whole metagenome analysis, attempts to capture and sequence the complete DNA profile of every organism in a sample. This identification method presents an opportunity for a more accurate representation of all microorganisms in a sample and offers multiple advantages over amplicon-based methods regarding the richness of information obtained (30). Multiple systematic comparisons between amplicon sequencing and whole metagenomic sequencing methods have been conducted for the gut microbiome (31–34), although, to the best of our knowledge, none of the skin microbiome. These studies have concluded distinct advantages in using shotgun metagenomics, including identifying a greater diversity of microbial taxa found and enhanced resolution and accuracy of species detection (31, 32), with broad-level biological patterns (such as alpha and beta diversity metrics) being largely consistent (33, 34). Shotgun metagenomics is highly applicable to skin microbiome studies for several reasons. First, this technique can sequence microbes at the whole-genome level, providing a relative abundance of functional genes, not only taxonomic markers. Although marker genes will likely remain an important and efficient way to classify taxa and evaluate microbial communities, shotgun profiling provides a deeper complexity, opening an enhanced window into the microbial dynamics within a skin microbiome sample. Second, because this method is accomplished by randomly fragmenting all DNA within the sample into small pieces, it resolves the sample’s complete DNA profile, including bacteria, viruses, microbial eukaryotes, metagenome elements such as plasmids, and the human host. Thus, whole metagenome analyses in the skin microbiome context can obtain multi-kingdom genomic and metagenomic signatures, extending past the phylogenetically limited views of marker gene analyses. Third, because this method resolves whole genomes, shotgun metagenomics provides higher phylogenetic resolution and is better equipped to differentiate species and strains (provided the sequencing is deep enough and the assembly sufficiently successful) (35). As mentioned previously, human skin is preferentially dominated by many different Staphylococcus species (S. epidermidis, S. hominis, and S. lugdenensis, to name only a few), which makes short-read amplicon-based genus-level taxonomic resolution limiting, and whole metagenome approaches more capable of discriminating this important taxon.
Illumina platforms are the most popularly employed technology for short-read shotgun metagenomics, followed by Ion Torrent. Long-read machines from Oxford Nanopore MinION and PacBio technologies are scaling rapidly and are likely to see more significant usage. Following sequencing and necessary quality filtering and trimming steps, metagenomics workflows require linking fragmented reads to taxonomic and functional information. Two major approaches carry out this process: reference/read-based and assembly/contig-based workflows (30). Reference-based approaches to identifying microorganisms involve mapping sequences and comparing them to reference genomes from relevant databases. Assembly-based approaches attempt to reconstruct fragmented reads representing the community of microorganisms into contigs and then group or “bin” contigs into species, sometimes referred to as Metagenome-assembled genomes (MAGs) (36). The de novo or reference-free approach of MAGs allows for more comprehensive identification of skin microorganisms, including those previously uncharacterized, thus expanding our knowledge and accuracy of the skin’s microbial inhabitants (37).
2.3 Integrating other ‘omics’ technologies for functional studies
For a complete understanding of the composition and function of microbial communities, holistic approaches that go beyond marker gene analysis and metagenomics are necessary to begin inferring the functional roles of different microorganisms. Metatranscriptomics involves sequencing the RNA profile of microorganisms in an environment, thus enabling profiling of which genes are being expressed at a specific time and how they are regulated. Additionally, due to the shorter half-life of RNA, transcriptomic analysis has some ability to distinguish between live or dead microorganisms and can capture a more dynamic community profile. Combining metatranscriptomics with marker gene analysis or shotgun metagenomics can improve understanding of which microorganisms in a community are actively transcribing and their functional role. Likewise, metaproteomics and metabolomic approaches seek to characterize the proteins and small molecules consumed and produced by the microorganisms to validate profiling findings. For example, 16S sequencing combined with mass spectrometry corroborated the hypothesis that C. acnes utilized sebum triglycerides as an energy source by showing their colocalization with fatty acid products (38). A recent study by Roux et al. described the existence of three distinct metabolite-microbe clusters at the skin surface in infants that parallels our current view of the three major environments on human skin; one characterized by C. acnes and sebaceous elements at the epidermal barrier, another microbiome network involving Staphylococcus spp., moisture and pH regulation at the skin surface, and a third niche with a diverse but unique metabolomic profile which was Streptococcus-centered (39). Critically, however, these already complex data sets must be integrated with companion functional data and analysis of the human host to understand how microbes contribute to the health and disease of the “holobiont” (40). Analysis of multi-omics data from the skin microbiome will provide a more complete picture of the behaviors of skin microbes. Furthermore, machine learning and artificial intelligence methods are increasingly being applied to skin microbiome research, including determining changes in abundance or diversity of species and strains, integrating multi-omic microbiome data, and phenotypic prediction (41–43).
2.4 Statistical considerations for microbiome studies
Microbiome data analysis, which typically involves comparing a matrix of features composed of taxa or genes from a simple case to samples of different experimental or phenotypic groups, can be quite complex. Therefore, it is important to use caution when interpreting the results of statistical analyses. Even seemingly basic questions, such as “how does a sample from diseased skin differ from that of healthy skin?” often require complex statistical interpretation. Microbiome data is multi-dimensional, meaning it has a large number of features that can quickly increase with the number of microbes, samples, and time points. Additionally, such data is typically very sparse, with most microbes not present in most of the data, creating a significant skew towards the zeros of the dataset, which represent virtually non-existent microorganisms. Microbiome datasets are also compositional (44), meaning that the sequence counts are heavily influenced by the limitations of the sequencing technology, not just the actual abundance of microbes in the sample (35). These characteristics make it difficult to interpret microbiome data, requiring appropriate and validated techniques.
Microbiome datasets and their broad-level patterns are commonly evaluated using alpha and beta diversity metrics. Alpha diversity measures feature diversity (i.e., taxa or genes) within a single sample and can be compared between different groups of samples. Alpha diversity analyses may describe the diversity of microbial genera or species between skin disease and healthy groups. In contrast, beta diversity measures the difference in composition between two communities. Traditional statistical tests such as Student’s t-test or ANOVA should not be used to compare diversity or the relative abundance of taxa across samples because the data often does not follow a normal statistical distribution and/or violates independence assumptions (18). Instead, non-parametric tests should be used to avoid high false discovery rates. Typically, statistical evaluations use the Mann-Whitney U test or Wilcoxon rank-sum test instead of the t-test, Kruskal-Wallis or PERMANOVA instead of ANOVA, and Spearman rank correlation over Pearson correlation. Newer, compositionally aware tools are especially important for differential abundance comparisons. Effect size calculations provide the magnitude of differences between groups and offer a more quantitative understanding of statistical significance beyond p-values (45, 46). For a more extensive review of the quantitative tools and techniques for microbiome data analysis and visualization, we direct the reader to these reviews (18, 47).
3 Special considerations in the sequencing and analysis of skin microbial communities
3.1 Challenges due to low microbial biomass
Whether pursuing culture-based, amplicon, or shotgun genomics methods, there are many challenges involved with analyzing the skin microbiome due to the skin’s unique features that can lead to poor-quality genomic data and difficult downstream bioinformatics analysis. Standards and best practices for conducting skin microbiome studies have been described primarily for 16S rRNA gene amplicon sequencing (48) but are largely lacking for metagenomics. Compared to the rich environment inhabited by gut microbes, the skin microbiome holds relatively low microbial biomass (especially at the surface) due to its dry and nutrient-poor conditions (49). Thus, it is critical to note that microbial DNA from skin samples is particularly prone to dilution from human and environmental DNA, and 90% of sequencing reads can be from human DNA when applying metagenomics (50). Furthermore, the issue of low microbial biomass makes it difficult to identify rare or naturally low-abundant taxa that may still have clinical relevance and distinguish these from transient skin microorganisms or possible environmental contaminants. Additionally, characterizing non-bacterial DNA, particularly viruses, whose genomes and microbial biomass are magnitudes smaller than bacteria, presents even greater challenges.
Deeper metagenomic sequencing mitigates issues in low microbial biomass; however, this, in conjunction with appropriate DNA extraction and host/free microbial DNA depletion methodology for skin microbiome samples, is likely the most ideal and cost-effective. Reduction in human DNA during the DNA extraction and library preparation process for skin samples is possible and seems to be better performed by some commercial kits than others (50). However, to the best of our knowledge, there are no published studies that apply skin-specific methods for a host, bacterial extracellular DNA, or dead bacterial intracellular DNA depletion for the skin microbiome. Chemical digestion or lysing to deplete excessive host DNA after DNA extraction may be necessary before microbial sequencing proceeds from skin samples, as demonstrated for the oral and nasopharynx microbiomes (51, 52). Likewise, a method for depleting both human and free microbial extracellular DNA for metagenomic sequencing via selective lysis of eukaryotic cells and endonuclease digestion has been proposed for sputum samples from the Cystic Fibrosis respiratory microbiome (53). Here the authors noted significant increases in microbial sequencing depth and detected taxa and genes compared to commercially available kits. Careful storage procedures of microbial DNA from skin samples are also necessary to prevent further degradation (54). Due to the low abundance of microbial DNA on the skin, the environmental contamination risk is high. Thus, it is crucial to use negative/blank controls throughout all processing steps, from DNA extraction to library preparation and sequencing in parallel with experimental samples. Mock communities (artificial mixtures of known microorganisms) and positive/reference samples are also necessary to benchmark results.
3.2 Challenges due to sampling technique
Human skin represents a vast, multi-layered three-dimensional organ that spans the entire bodily surface. Body-site variation and physiological anatomy create distinct environments, allowing for specialized microbial colonization and unique niche microbiomes. Thus, where you sample on the body is considered to heavily influence results. As the skin contains many layers and species are unevenly distributed, the sampling technique will strongly influence the results (55). Most human skin microbiota studies use swabs as the sampling method; however, this practice is limited to collecting surface and epidermal skin microbes (2). In contrast, a pore strip sample captures mostly microbes in the follicular environment, including the hair follicle and sweat duct. Other sampling methodologies, such as skin biopsies, examine the microbial community within follicular and deeper dermal environments; surface swabs do not always reach those areas. An analysis comparing epidermal and dermal sampling methods suggests the dermal compartment has a greater abundance of microbes with less variability between anatomic locations and between individuals, termed the “universal dermal microbiome” (2). While such observations challenge current research assumptions, this finding may not be surprising as environmental influences (including the individual’s hygiene and cosmetic practices) affect the skin microbiome, particularly at the surface. However, so far, fewer studies have performed dermal sampling as it is more invasive. Additionally, performing a skin scrape, biopsy, or other more invasive sampling procedure may capture an even larger proportion of host (human) DNA contamination, posing an additional challenge when choosing this approach.
3.3 Challenges of amplicon methods
Skin microbiome surveys are strongly influenced by the analytical method, including the choice of hypervariable region for 16S-based studies (55). According to Meisel et al., sequencing V1-V3 of skin organisms provides similar results and is more optimal than V4, one of the most commonly employed sequencing targets for investigating the human gut microbiome. Sequencing of the V4 poorly captures Cutibacterium and cannot reliably speciate Staphylococcus (55). Cutibacterium acnes and Staphylococcus species are among the most prevalent commensal bacteria, so this presents a significant limitation in the usability of the V4 region for microbes inhabiting the skin. Given that the V4 hypervariable region is shorter and contains more sequence conservation (56), its difficulty in speciating Staphylococcus was unsurprising. These findings highlight how the optimal choice of the most useful hypervariable region is ecosystem dependent and can affect results. Improved 16S rRNA V4 gene primers have been designed to specifically improve the capture of underrepresented taxa (57); however, specific benchmarking for skin bacterial communities and identification ability towards Cutibacterium and Staphylococcus species has not been validated. Importantly, no single hypervariable region nor the whole 16S gene can distinguish all bacterial species for any human microbiome. Only genus-level resolution is typically reliable when using short 16S regions as a marker, although publications often report species-level resolution without checking whether it is even possible for the relevant 16S region and taxa (resolution varies in different parts of the phylogenetic tree). Consolidating OTUs after sequencing is problematic for two major reasons also. Firstly, selecting one OTU sequence as a proxy tends to ignore minor genetic variants and oversimplify the dataset (58). Second, the canonical 97% clustering threshold is outdated and is now believed to be too low for reliable differentiation for most species (59). Originally proposed in 1994, this metric has been based on multiple linking proxies: short-read sequencing of hypervariable regions to approximate full-length sequence identity and full-length sequence identity to approximate whole genome similarity. Thus, reference libraries created by 16S rRNA gene sequencing approaches require systematic re-evaluation now that whole genome sequencing has produced far richer datasets. Barnes et al. investigated the OTU vs. ASV method in the atopic dermatitis skin microbiome, particularly those assigned to Staphylococcus species (24). Their findings suggested that OTU clustering inflated bacterial richness and concluded the ASV approach with DADA2 managed sequencing errors better. However, the authors stress that when using amplicon sequencing, one should be careful not to overinterpret taxonomic calls at the species level for the Staphylococcus community. Genus-level resolution is possible, but attempting to distinguish between species by sequencing only the 16S gene should be treated carefully.
The next step involves taxonomic assignment based on the homology of sequence reads to reference databases. As 16S rRNA sequencing has been widely used, many well-curated databases are available for taxonomic identification. However, careful considerations regarding taxonomic classification are still required. While it has been suggested that ecosystem-specific databases provide more accurate taxonomic classifications (60), this is likely not true for the skin microbiome. General-purpose microbial taxonomic databases outperform habitat-specific databases in microbiome datasets with significant environmental taxa since these reads may be erroneously assigned if an appropriate reference genome is unavailable (26). Instead, applying environment-specific taxonomic weights within general-purpose databases, such as the Greengenes 16S database with q2-clawback (61), can improve sequence taxonomy classification accuracy from common environments.
3.4 Challenges of whole metagenome methods
Shotgun metagenomics has the potential to yield rich and informative datasets. However, as our technology has advanced, so have the accompanying complexities with data analysis. This technology is still relatively more expensive (though widely becoming more accessible and less costly) than amplicon methods. Producing datasets with sufficiently rich sequencing depths (the number of reads per sample) across the whole genome is crucial relative to other methods, especially when the sample is mostly composed of host DNA reads (50). While shallow- or moderate-depth sequencing has been shown to accurately obtain species-level information, particularly in large-scale studies where deep sequencing is cost-prohibitive, only at deep sequencing depths is the attractive lure of strain-level taxonomic assignment and identification of novel strains and low-abundance taxa with biologically meaningful function within the community possible (35). In skin microbiome studies, the potential to distinguish between Staphylococcus species and other commensal strains on the skin is only legitimate if sufficient sequencing depth is achieved. This remains a critical problem because skin sampling typically provides lower biomass of microbes than other body habitats, and low-depth data is common. Contamination from host-derived DNA is a constant challenge, particularly in areas where host DNA dominates microbial DNA, such as the skin. When conducting shotgun metagenomics studies on the human skin microbiome, it is important to filter out sequences from the host. However, the amount of DNA needed for shotgun studies has steadily decreased as the technology has improved, allowing for more efficient sequencing of skin microbiomes.
In contrast, as a newer technology, shotgun metagenome databases currently lack many full reference genomes of microbes and are currently most well-positioned for gut microbiome studies. With the advent of whole metagenomics technology, previous skin microbiome studies observed significant metagenomic reads that did not match those in reference databases suggesting unmapped prokaryotic diversity (62, 63). Thus, the de novo (reference-free) approach of MAGs and improved assembly algorithms provide avenues to characterize new and unknown skin species from all domains of life. To address this gap, cultivating microbes to provide high-quality whole-genome sequences, which can then be aligned with MAGs, can provide a more comprehensive catalog of microbial diversity on the skin. This integrated approach has been launched by the Skin Microbial Genome Collection and is expanding our understanding of skin microbes to include new bacterial and eukaryotic species and improved assemblies of viruses and jumbo phages (37).
4 Present knowledge of the healthy skin microbiome
Understanding normal microbiota and typical/benign variations within the skin microbiome in health is necessary to establish a point of reference for investigations into dysbiotic disease states. Human skin contains many resident commensal microorganisms, including bacteria, fungi, viruses, and mites, that utilize skin resources while playing a central role in skin homeostasis and health (Figure 2). However, even commensal bacteria can become pathogenic in specific contexts and contribute to inflammatory skin diseases. The following will summarize current information on this topic. However, it is important to recognize that many reports are subject to the limitations of the sequencing technology employed or the sampling methods used. Thus, some revision of current conclusions will likely occur as better data is developed.
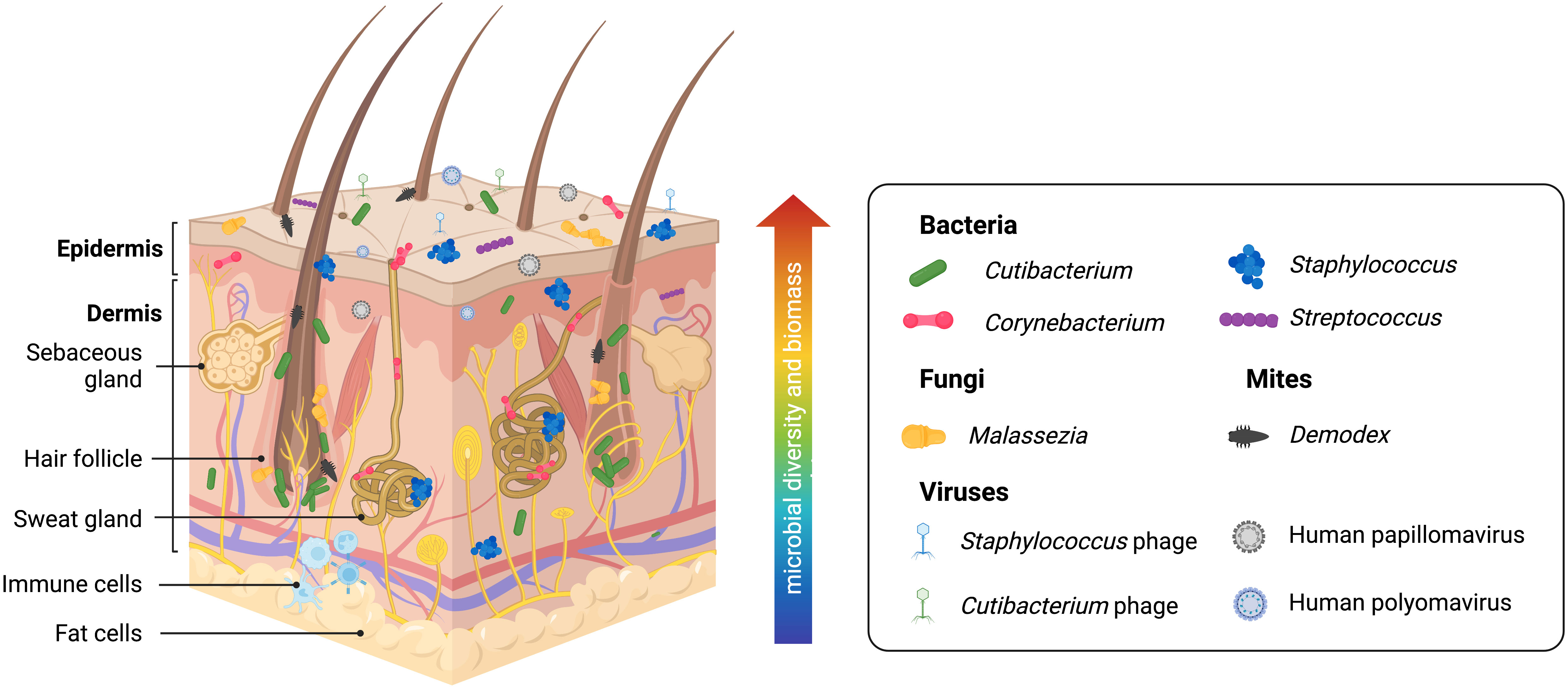
Figure 2 Healthy human skin is host to a diverse range of microbes across multiple skin layers and microenvironments. Skin bacteria, many of which belong to the Cutibacterium, Staphylococcus, Corynebacterium, and Streptococcus genera, are resident members of the skin microbiome. The skin eukaryome commonly includes fungi and microscopic mites belonging to the Malassezia and Demodex genera, respectively. Viruses, most notably including Staphylococcus phages, Cutibacterium phages, human Papillomaviruses, and human Polyomaviruses, are among the dominant members of the skin virome. Archaea (not pictured) has also been identified on the skin microbiome, though likely with a minor or transient presence. Microbial diversity and biomass are typically higher at the skin surface. However, human skin is a three-dimensional space, and microbes form communities that live at the skin surface, deeper skin layers, and within microhabitats such as the hair follicle and sweat glands, allowing complex inter-species relationships and interactions with the host immune system.
Skin microenvironments are broadly grouped into three categories due to the densities of hair follicles and sweat glands: sebaceous/oily, moist/humid, and dry (7) (although far more fine-scale variation exists on human skin) that is preferentially dominated by commensal organisms. Current data suggests sebaceous body sites, particularly within the follicular microenvironments, are the skin sites showing the least species diversity due to being dominated by Cutibacterium species, particularly C. acnes (64, 65). Interestingly, a recent study suggests that skin pores spatially segregate C. acnes genotypes and that each pore on the face is dominated by a population of C. acnes typically differing by < 1 mutation (66). Thus, microenvironments within the skin, such as the hair follicle, represent opportunities for population bottleneck effects. Within most moist areas on the epidermal surface, Staphylococcus and Corynebacterium species are the most abundant (10, 65). Dry sites have the greatest diversity with a mixed population of Cutibacterium, Staphylococcus, Corynebacterium, and Streptococcus species and host more transient microbes (65). Staphylococcus commensal species are highly abundant across all skin sites due to the diversity of this genus and their facultative anaerobic abilities; these most commonly include S. epidermidis, S. capitis, S. hominis, S. lugdengensis, S. haemolyticus, and S. warneri (65). Human skin also contains a diverse fungal microbiome (mycobiome) that has only begun to be explored by sequence-based methods (67). Malassezia species, particularly M. restricta, M. globosa, and M. sympodialis, are the most prevalent fungal microorganisms on human skin, particularly within the sebaceous sites (65, 67). Candida species, including C. albicans and C. auris, are opportunistic fungal pathogens that frequently colonize the skin (67). The human viral microbiome (virome) is also vastly under-characterized for similar reasons as the human skin mycobiome. Bacteriophages (viruses that infect bacteria) are significant members of the microbial ecosystem on the skin. Cutibacterium and Staphylococcus phages are found to be conserved across individuals. However, little is known about the interactions and dynamics between commensal bacteria and bacteriophages on human skin. Eukaryotic viruses, some of which include human papillomaviruses and polyomaviruses (including Merkel cell polyomaviruses associated with skin cancer), have also been found on human skin (62, 65, 68, 69), and they are believed to be more specific to individuals than conserved in certain anatomical sites. Technical limitations with amplicon sequencing and shotgun metagenomics complicate studying the skin virome. Viral genomes lack a taxonomic marker gene like 16S for bacteria or ITS1 for fungi needed for amplicon sequencing and are easily overwhelmed by prokaryotic or eukaryotic genomes (present in populations that are orders of magnitude larger) when shotgun metagenomics is applied (70). Also found on the skin are Demodex mites, microscopic arthropods that are a common (and usually benign) part of the commensal skin microbiome (71–73). These mites prefer sebaceous skin sites as their main food source is sebum. Thus, they have been primarily found in the face and scalp, with greater abundance in the pilosebaceous unit. Two Demodex species have been identified so far on human skin, D. follicularum and D. brevis, with imbalances linked to rosacea (71). Though most overlooked, archaea have also been found to be a part of the skin microbiome (74–77). While there is a general lack of consensus on the abundance and role of archaea on the skin, these 16S gene signatures are reported to belong to the Thermoproteota (formerly Thaumarchaeota), Methanobacteriota, and Halobacteriota phyla (74–77). Ammonia-oxidizing Thaumarchaeota has been suggested to contribute to lowering skin pH and, thus, supporting the skin’s barrier against foreign and pathogenic microorganisms (74). In contrast, a recent study profiling skin archaeal communities shows that archaea comprise less than 1% of mammalian skin samples and are more likely to have a minor and transient presence driven by the environment (76). Commonly touched surfaces that interface with the skin, including computer keyboards, phones, and door handles, contained archaea, highlighting how our built environments contribute to microbial inhabitance on the skin (76).
5 The skin microbiome in inflammatory skin diseases
Research in the microbiota of other organs has shown that subtle differences in genetic and metabolic diversity can drive otherwise commensal microbes toward pathogenesis, defining these organisms as pathobionts. These alterations in community composition can have significant implications for disease onset and treatment. While progress has been made in identifying the different types of microbes associated with various skin diseases, whether a dysregulated microbiome causes inflammation or if these associations are a byproduct of the inflammatory environment is unclear (to varying degrees) for different skin diseases. Inflammatory skin disorders have multifactorial etiologies where an intimate balance of host determinants, environmental influences, and microbial composition can influence disease development. Most microbiome studies of inflammatory skin disorders have utilized 16S amplicon sequencing to investigate microbial alterations with specific skin diseases, illuminating important findings. However, deciphering only bacterial genera and their relative abundance has limited usefulness for expanding our current understanding of skin diseases toward improved clinical application. Understanding differences in strain behaviors that could cause them to be pathobionts, commensals, or truly beneficial mutuals may become possible as more comprehensive genomic methods are brought to bear. This is becoming apparent as more studies are applying shotgun metagenomics to the microbiome in skin various diseases (Table 1). Likewise, it is becoming clear that inter-species and inter-kingdom interactions between skin microorganisms play a significant role in skin health as well. Understanding how skin-disease associated microbes interact with the immune system allows opportunities for a deeper understanding of skin microbiota. Such insights will yield powerful and actionable insights into skin disease mechanisms that experiments can validate to confirm function and translate to clinical application. Here, we will focus on areas showing the most progress or best evidence regarding the microbiome’s role in inflammatory skin diseases given currently employed methodologies.
5.1 Atopic dermatitis
Atopic dermatitis (AD), also known as eczema, is a chronic inflammatory skin disease characterized by itchy, red, swollen, and cracked skin flares affecting up to 20% of children and 3% of adults (84). Compared to other dermatological disorders, the role of skin microorganisms and dysbiosis in adults with AD is well-documented and exhibits the clearest relationship in which a dysregulated microbiome is causative to skin inflammation. The skin microbiome of adult AD patients typically has reduced biodiversity due to a massive increase in Staphylococcus aureus colonization which precedes lesion onset (85, 86). Likewise, skin colonization by S. aureus has been found to precede AD diagnosis in infants (87). The dominance and overgrowth of S. aureus result in a relative decrease in the proportion of commensal bacteria belonging to the Cutibacterium, Corynebacterium, and Streptococcus genera (85, 88). S. aureus commonly presents as a skin pathogen, and colonization by S. aureus on healthy skin is rare (89). One recent shotgun metagenomics study by Byrd et al. suggests that not all S. aureus strains may be equally pathological (79). This initial work provides insights into S. aureus strains from lesional versus non-lesional skin on AD patients. S. aureus strains from lesional versus non-lesional sites are phylogenetically clustered separately, suggesting that strain differences may be implicated in AD severity. Multiple studies provide functional evidence of S. aureus producing toxins, proteases, phenol-soluble modulins (PSMs), bacteriocins, and other virulence factors exacerbating skin inflammation (90–95).
However, despite a clear link between S. aureus and AD, not all AD lesions are colonized by S. aureus; many AD patients also have greater colonization of S. epidermidis than S. aureus (85, 96, 97). These observations are also noted in studies of the AD microbiome where S. aureus exclusion leads to greater S. epidermidis dominance. Findings from a recent metagenomic study suggest S. epidermidis may be a secondary causative agent of AD disease severity as S. aureus significantly associates with severe disease, while S. epidermidis may be more common with moderate disease (79). Compared to S. aureus, S. epidermidis is universal on human skin, and some strains exhibit many beneficial properties for skin health, like defense against opportunistic pathogens and enhancing epithelial barrier function (98). For example, S. epidermidis induces antimicrobial peptides from keratinocytes and produces phenol-soluble modulins (PSMγ and PSMδ) and other small antimicrobials like lantibiotics to promote colonization by more pathogenic skin bacteria such as Methicillin Resistant Staphylococcus Aureus (MRSA) and Streptococcus pyogenes (98–100). Furthermore, S. epidermidis strain-level diversity and their functional differences under distinct physiological conditions are increasingly being appreciated (79, 98, 101). For example, it has been suggested that disrupted barrier function (such as that seen in AD skin) can be exacerbated by S. aureus and promote the overgrowth of opportunistic S. epidermidis strains (98, 102). This hypothesis has been suggested in mouse studies where S. epidermidis is resistant to S. aureus challenge only in a healthy skin barrier state (103). In AD skin, S. epidermidis can injure the epidermis by producing the cysteine protease EcpA that can damage the skin when the organism achieves sufficient density to activate quorum-sensing mechanisms (104). Similarly, S. epidermidis cysteine protease EcpA is associated with disease in Netherton’s Syndrome and promotes skin damage through a disrupted barrier function (83). Though EcpA is part of the pan-genome of S. epidermidis, it is only expressed by some strains, further exemplifying the strain-level functional differences of S. epidermidis (104).
The characterization of flare vs. non-flare (AD-prone) lesions reveals the potential existence of an AD-susceptible microbiome profile marked by Streptococcus and Gemella enrichment and Dermacoccus depletion (105). Additionally, the function of fungi and viruses and the cross-kingdom interactions of AD microbiomes have yet to be completely investigated, as current studies have mostly concentrated on S. aureus and S. epidermidis. Differences in fungal and viral communities of the AD skin microbiome have also recently been observed and characterized by body site by Bjerre et al. (78). Increased M. osloensis and M. luteus were observed in all AD skin sites except the neck, where it was absent. Perhaps unsurprisingly, AD lesions exhibit a higher abundance of Staphylococcus phages (78). According to these studies, dysbiosis in AD has global and body-site-specific differences involving bacteria, fungi, and viruses, and strain-level functional differences may contribute to disease complexities (Table 1).
5.2 Seborrheic dermatitis
Seborrheic dermatitis (SD) is another chronic skin disorder that causes a red, itchy rash with flaky skin (106). SD commonly occurs on the body’s oily areas like the scalp (where it is known as dandruff), face, and chest. Fungal and bacterial skin microbiome changes are currently implicated in SD, particularly in Malassezia and Staphylococcus species, with Malassezia associated with itchiness and disease severity (106–108). Topical antifungals (ketoconazole) are a common and sometimes effective therapy for SD. However, it is unclear if the mechanism of action occurs on microbes or other effects on the host from this class of drugs (109). To our knowledge, multiple studies have been conducted on the SD scalp microbiome using various 16S regions, and one study employs whole metagenomics. A recent systematic review by Tao et al. of all SD skin microbiome studies (largely conducted on the scalp) suggested an increased M. restrica to M. globosa and Staphylococcus to Cutibacterium ratios in SD skin (106). Only one study by Saxena et al. has performed a whole metagenome approach to study the functional pathways of SD and the skin microbiome. According to this study, bacterial alterations are characterized by increased S. epidermidis, decreased P. nitroreducens, and decreased C. acnes to S. epidermidis ratio (80). Fungal differences included increased M. restricta to M. globosa ratio and a significant portion of the mycobiome composed of uncharacterized Malassezia species and strains highly associated with dandruff presence (Table 1). Thus, SD represents an important example of how our understanding of the skin mycobiome is severely lacking (67) and how metagenomic assemblies of uncharacterized reads may resolve discoveries of new fungal species and strains.
5.3 Acne vulgaris
Acne is the most common inflammatory skin disease affecting approximately 9% of the population worldwide and 85% of teens and young adults (110). The visible nature of active acne and residual scarring in approximately 1 out of 5 disease cases creates psychosocial impacts through their negative effects on affected patients’ physical appearance, social interactions, and self-esteem. The increased incidence of anxiety, depression, and suicide arising from chronic acne, particularly for young adults, is well documented (111). Acne manifests in the pilosebaceous unit, commonly known as the hair follicle or “pore,” a lipid-rich niche on the skin with an acidic environment low in oxygen and nutrients that is hostile to most microorganisms (112). The skin microbiota is thought to play a causal role in acne development. In particular, there has been a focus on the role of the commensal skin bacterium Cutibacterium acnes (formerly named Propionibacterium acnes), an aerotolerant anaerobe that thrives in sebaceous conditions (64, 112). However, the link between C. acnes and acne disease has been challenging to pinpoint, even after decades of research and medical practice. The older model of acne implicated C. acnes skin “infection” as the disease’s cause due to its unique ability to thrive in the same environment where acne develops. This model proposed that increased sebum production in the pores promoted C. acnes overgrowth that physically “plugged” the hair follicle and sebaceous gland, thereby inducing inflammation in surrounding skin cells. Other work has suggested that disease in acne can come from cooperation between C. acnes and other inhabitants of the follicular microbiome, resulting in cell damage from a Christie, Atkins, Munch-Peterson (CAMP) reaction (113). Multiple studies have concluded that C. acnes is the predominant bacterium within most individuals’ skin microbial communities, both in healthy and diseased skin, and can account for nearly 90% of the skin pore microbiota, while its oxygen detoxification abilities allow it also to inhabit the skin surface (67, 79, 86). Nevertheless, topical and systemic antibiotics are a mainstay of acne therapy, and topical bacteriophage therapy has been suggested to have therapeutic potential (112, 114), suggesting that C. acnes has an important role in the pathophysiology of this disease.
As a commensal organism with a ubiquitous presence on the skin, the association of acne lesions with C. acnes is likely strain-dependent (115, 116). As researchers have attempted to understand how C. acnes subgroups are involved in acne disease, several different molecular typing methods have been developed to separate C. acnes into strains, making it difficult to cross-compare between studies (117). Genomic analyses concur specific strains of C. acnes are more commonly associated with acne (112, 116, 118, 119). However, the functional differences between C. acnes strains are generally unknown. It is even less clear if those strains that appear to be associated with acneic skin induce greater skin inflammation (and by what mechanisms). Furthermore, these current strain-typing methods are based on partial amplification and sequencing of conserved genes that likely fail to capture the full extent of C. acnes genetic diversity related to pathobiont behavior. For example, the first whole-genome sequencing analysis of C. acnes revealed the presence of a novel linear plasmid containing genes encoding potential virulence factors (120).
A greater understanding of the community compositional changes between acne and healthy skin is needed. In healthy skin, C. acnes and S. epidermidis are among the most prevalent commensals and have been shown to interact frequently with shifts in one species influencing the other. In acne-affected skin, the strain diversity and relative abundance of S. epidermidis are increased to inhibit the growth of C. acnes via fermentation and other defense mechanisms (112, 117, 121). A deep whole metagenome study by Barnard et al. of acne versus healthy individuals finds a balance of metagenomic elements shapes the skin microbiome in acne and health (81) (Table 1). Compared to healthy individuals, acne patients had a higher diversity of C. acnes populations, more strain types, more virulence-associated factors, and lower metabolic synthesis genes.
Additionally, their analyses suggest the C. acnes to C. granulosum ratio is important in acne disease, with acne patients having a lower relative C. granulosum abundance (81). C. acnes and C. granulosum have been reported to exist in a potentially competitive relationship, with C. granulosum secreting an endogenous extracellular nuclease capable of degrading C. acnes biofilm (122). Recently, a published study by Conwill et al. suggests C. acnes lineages can coexist across an individual’s skin; however, within individual pores, C. acnes is nearly clonal (< 1 mutation apart) (66). However, the C. acnes diversity at the single-pore level in individuals with acne has yet to be explored using per-pore sampling techniques.
5.4 Psoriasis
Psoriasis occurs in approximately 2% of the population worldwide and has characteristic plaques of red, itchy, and dry skin, most often on the elbows, knees, trunk, and scalp (123, 124). Many studies have shown dysbiotic differences in the psoriasis skin microbiome, especially in healthy and psoriatic individuals’ relative bacterial and fungal populations. While these associations are less clear than the previously mentioned diseases, many studies link Streptococcus and Staphylococcus bacterial species and Malassezia and Candida albicans fungi. Additionally, a subtype of psoriasis, known as guttate psoriasis, can be systemically triggered by bacterial infections such as “strep throat” from Group A Streptococcus (125). Psoriasis lesions have also been reported to have proportionately greater populations of Staphylococcus. aureus and Streptococcus pyogenes (126), and decreased incidence of S. epidermidis and C. acnes (82, 127). On psoriatic skin, Malassezia diversity decreases as disease severity increases. M. restricta and M. globosa are predominantly present, and M. furfur is reportedly only found on psoriatic skin. Another fungal microbe, Candida albicans, is also significantly more common in psoriatic patients and is linked to worsening psoriasis skin lesions (126, 127). In the first whole metagenome study of healthy versus psoriasis skin, Tett et al. suggest psoriatic lesions exhibit an increase in Staphylococcus; however, no significant differences were observed at the species level (82). However, their analyses suggest functional differences may lie at the strain level (Table 1). Additionally, similar to findings on the skin mycobiome in seborrheic dermatitis, the psoriasis mycobiome contained uncharacterized Malassezia reads, which the authors attributed to limitations in taxonomic databases.
5.5 Rosacea
Rosacea is a skin condition that causes facial flushing and sometimes small, pus-filled bumps on the face (71). The demographic of people most at risk for rosacea are individuals of Caucasian descent and who have fair skin, particularly women over 30 years of age. The skin microbiome is believed to be involved in rosacea; however, the results are inconsistent, likely due to mixed and limited methodologies (128). To the best of our knowledge, all current rosacea studies have used 16S amplicon sequencing and only used the V3 and V4 regions, which has been reported to be an issue in identifying Staphylococcus and Cutibacterium species (55). Whole metagenomics has not yet been employed to study the rosacea skin microbiome. Multiple studies have reported the skin microbiome of rosacea patients to have increased densities of Demodex mites, especially D. folliculorum and D. brevis (73, 129). (Characterization of Demodex mites is based on mitochondrial 16S rDNA partial sequences). While Demodex species are considered part of the healthy skin microbiome, in rosacea patients, an increased incidence of Demodex is associated with aberrant overactivation of the innate immune system (130). Bacillus oleronius, a bacterium carried by Demodex, can also independently trigger inflammatory pathways and may play a role in rosacea (73). Multiple studies also report a significant increase in the (normally) commensal bacterium S. epidermidis in rosacea patients, particularly within the pus-filled lesions (129, 131). At higher skin temperatures, S. epidermidis is known to produce additional proteins that may act as virulence factors (132). In rosacea, increased blood flow on the face leads to higher temperatures that may allow S. epidermidis to behave more opportunistically (129, 131). A decrease in C. acnes has also been noted in rosacea patients (133). Both C. acnes and Demodex mites live in the pilosebaceous unit and utilize sebum as a primary nutrient. Thus, the decrease in C. acnes may partly be due to the overgrowth of Demodex. However, many of these studies have not seen cross-validation. For a comprehensive review of the skin microbiome studies of rosacea, we direct the reader to the following (128).
5.6 Hidradenitis suppurativa
Also known as acne inversa, hidradenitis suppurativa (HS) is a chronic skin disease with painful nodule-like lesions that presents as abscesses and sinus tracts (tunnel-like wounds) on the skin (134). HS is considered an autoinflammatory disease caused by an aberrant and overactive innate immune system (134, 135). Flares from HS typically occur in body sites with more sweat glands and skin folding, such as the armpits, groin, buttocks, and breasts. Because of its appearance and location, HS can be misdiagnosed as acne, skin infection, herpes, or a sexually transmitted disease (STD). The psychosocial impacts of HS are significant due to its occurrence in private body sites, common misdiagnosis as a contagious STD, and permanent scarring, which is a common outcome. Current therapies are limited for HS, and this disorder is poorly responsive to antibiotics.
Several studies have recently characterized the skin microbiome of HS patients using 16S amplicon sequencing (136–142) (however, none with whole metagenome approaches). Across all studies, an increased abundance of anaerobic bacteria belonging to the Porphyromonas and Prevotella genera is significantly observed (138–142). Some studies also report an increase in Corynebacterium spp., Moraxella spp., Actinomyces spp., Peptoniphilus spp., Mobiluncus spp., and Campylobacter ureolyticus (138, 140, 142). In contrast, the skin of HS individuals has relatively lower populations of commensal bacteria, including Cutibacterium spp., Staphylococcus epidermidis, and Staphylococcus hominis (138, 142). Furthermore, some studies suggest an increased relative abundance of bacteria within lesional dermal tunnels versus non-lesional or non-dermal tunnel skin (142, 143). These findings demonstrate bacterial dysbiosis is associated with HS and varies with disease severity. Extending current findings with shotgun metagenomics will likely provide new insights into the skin microbiome’s role in this disease. Most bacteria currently associated with HS are resolved only at the genus level. An improved genomic analysis of the HS microbiome at the species or strain level will likely elucidate the association between this disease and the skin microbiome. There are currently no whole metagenome studies of the HS skin microbiome. For a comprehensive review of studies investigating the role of the skin microbiome in HS, we recommend the following (144).
5.7 Ichthyoses
Ichthyoses are a family of over 20 genetic skin diseases characterized by widespread, persistent dry, thick, and “fish-scale-like” skin (145). The various types of ichthyoses differ in their genetic inheritance pattern (which may be dominant, recessive, X-linked, or spontaneous), disease symptoms, and onset. Netherton Syndrome (NS) is an autosomal recessive-inherited type of ichthyosis where genetic mutations in the SPINK5 gene results in the loss of function of lymphoepithelial Kazal-type-related serine protease inhibitor (LEKTI-1) (83). Although NS is a disease linked to germline mutation, its severity, and skin inflammation vary. One recent study investigated how the loss of LEKTI-1 influences resident skin bacteria and contributes to NS (83). Shotgun sequencing of the NS lesional skin microbiome demonstrated a dominance of S. aureus and S. epidermidis, both able to induce skin inflammation and barrier damage in mice (83). Due to increased proteolytic activity in the LEKTI-1 deficient state, these microbes promote skin inflammation via S. aureus phenol-soluble modulin α and increased bacterial proteases staphopain A and B from S. aureus or EcpA from S. epidermidis. Thus, NS presents an interesting case where a shotgun metagenomic approach plus experimental validation showed how a monogenic disease could influence microbial community shifts (Table 1).
6 Discussion
Just as culture-based studies helped us to discover microbial life on the skin, amplicon sequencing has greatly expanded our knowledge of previously unknown microorganisms. However, as newer technologies and analytical practices have become more refined, our previous goals of describing microbial skin communities at genus-level resolution are no longer a sufficient research aim, particularly when applied to understanding dermatological disorders. Amplicon-based approaches are still valuable tools, but studies employing them should utilize more resolved primer sets and current bioinformatics approaches to generate the most robust datasets possible. There is increasing awareness that sampling and analysis methods can significantly influence results as the skin exhibits multiple forms of variation by body-site-specific niches and between surface, follicular, and dermal layers. Additionally, improved and validated methods of reducing or depleting host DNA in skin samples will greatly improve skin microbial profiling. Thus, future studies should reassess some older conclusions and include further investigation of the subepidermal microbial distribution of specific skin diseases, which may provide new insights into how microbes may be modulating host physiology and immune responses. Additionally, we stress that the relationship between microorganisms and ourselves is often more nuanced than the commonly employed binary descriptions of commensal versus pathogenic. Many skin disorders are influenced by what we typically consider commensal microorganisms that appear to demonstrate pathological differences at the species or strain level. An improved understanding of higher-order taxa disease associations will likely clarify the involvement of microbes and support explaining the multifactorial nature and diverse manifestation of most skin diseases. Furthermore, an under-surveyed non-bacterial community consisting of fungi, viruses, skin mites, and their inter-species community relationships also appear to play an important role in skin diseases that we are only beginning to uncover.
With the rapid advancement of ‘omics’ technologies and improved accompanying bioinformatics methodologies, designing research studies to address these questions appropriately are now possible. Whole metagenomics profiling (even at shallow sequencing depths) yields richer datasets than amplicon-based sequencing; however, such studies are currently absent or limited in many skin diseases. Larger scale and systematic studies characterizing the healthy human skin microbiome, such as those aimed at understanding the human gut microbiome, will likely expand as the field progresses. Increasing metagenome-assembled genomes from skin-resident microorganisms (though still limited) are seeing greater development. Other methods, such as functional screening using transcriptomics, proteomics, and metabolomics, combined with profiling efforts, will offer a more comprehensive understanding of the functional potential of skin microbes toward disease and present gateways for clinical applications.
The causative relationship between changes in the skin microbiome and inflammatory skin diseases is a complex issue that remains a subject of debate in the field. While some skin diseases, such as atopic dermatitis, show clear links between microbiome dysregulation and disease onset, the degree of causation is less understood in other skin diseases. In most cases, however, it is likely that both dysregulated microbiome and alterations in the skin’s inflammatory environment play a dual role in disease pathogenesis. And whether which comes first may be different for different skin diseases. For example, S. aureus colonization has been shown to disrupt the skin barrier and overall skin health, contributing to enhanced colonization of opportunistic S. epidermidis strains and decreased overall commensal strains, which also negatively affect disease outcomes. Understanding the underlying mechanisms (as we have most clearly elucidated in atopic dermatitis) is critical to developing effective therapeutic interventions for skin diseases.
Future efforts to uncover the functional impact of microbial dysbiosis in the skin may also support an increased understanding of how the effects extend beyond the skin. We will likely continue to think less about microbiome ecosystems as being only confined to their anatomical location, as crosstalk mechanistic studies support such conclusions. There is increasing evidence for the systemic effects of skin microbiome dysbiosis and disease on distant microbial-related pathologies, termed the ‘skin-gut’ or ‘skin-brain’ axes. It is well documented that inflammatory skin disorders are frequently associated with inflammatory bowel disease and that many individuals with a skin disease also have comorbidity with a gut disease. Such relationships between a skin-gut axis have been reported for atopic dermatitis (146), acne (147), psoriasis (148), rosacea (71), and hidradenitis suppurativa (149). Additionally, in psoriasis, a skin-brain axis has been proposed, where skin cells that express neuropeptides and hormones may be directly promoting systemic inflammation and inducing psychological effects in the brain (150). To further explore the role of the skin microbiome in local and systemic health effects, experiments with new and improved clinically applicable models will be necessary to uncover the mechanisms. By continually evolving how we investigate microbes on the skin alongside rapidly advancing technological approaches, new findings push the frontier regarding our scientific knowledge of skin disease and whole-body health, providing opportunities to translate insights into clinical practice.
Author contributions
YC, RK, and RG contributed to researching data and content. YC wrote the manuscript. YC, RK, and RG reviewed and edited the manuscript before submission. All authors contributed to the article and approved the submitted version.
Funding
(NIH) P50AR080594 U01AI152038 R37AI052453 R01DK121760 R01AI153185 R01AR076082.
Acknowledgments
Figures were created using BioRender.com.
Conflict of interest
RG is a co-founder, scientific advisor, consultant, and equity holder in MatriSys Biosciences and is a consultant, receives income, and equity holder in Sente Inc.
The remaining authors declare that the research was conducted in the absence of any commercial or financial relationships that could be construed as a potential conflict of interest.
Publisher’s note
All claims expressed in this article are solely those of the authors and do not necessarily represent those of their affiliated organizations, or those of the publisher, the editors and the reviewers. Any product that may be evaluated in this article, or claim that may be made by its manufacturer, is not guaranteed or endorsed by the publisher.
References
1. Nakatsuji T, Chiang HI, Jiang SB, Nagarajan H, Zengler K, Gallo RL. The microbiome extends to subepidermal compartments of normal skin. Nat Commun (2013) 4(1):1431. doi: 10.1038/ncomms2441
2. Bay L, Barnes CJ, Fritz BG, Thorsen J, Restrup MEM, Rasmussen L, et al. Universal dermal microbiome in human skin. mBio (2020) 11(1):e02945–19. doi: 10.1128/mBio.02945-19
3. Arck P, Handjiski B, Hagen E, Pincus M, Bruenahl C, Bienenstock J, et al. Is there a ‘gut-brain-skin axis’? Exp Dermatol (2010) 19(5):401–5. doi: 10.1111/j.1600-0625.2009.01060.x
4. De Pessemier B, Grine L, Debaere M, Maes A, Paetzold B, Callewaert C. Gut–skin axis: Current knowledge of the interrelationship between microbial dysbiosis and skin conditions. Microorganisms (2021) 9(2):353. doi: 10.3390/microorganisms9020353
5. Gallo RL. Human skin is the largest epithelial surface for interaction with microbes. J Invest Dermatol (2017) 137(6):1213–4. doi: 10.1016/j.jid.2016.11.045
6. Schroeder BO. Fight them or feed them: How the intestinal mucus layer manages the gut microbiota. Gastroenterol Rep (2019) 7(1):3–12. doi: 10.1093/gastro/goy052
7. Cundell AM. Microbial ecology of the human skin. Microb Ecol (2018) 76(1):113–20. doi: 10.1007/s00248-016-0789-6
8. Wei Q, Li Z, Gu Z, Liu X, Krutmann J, Wang J, et al. Shotgun metagenomic sequencing reveals skin microbial variability from different facial sites. Front Microbiol (2022) 13:933189. doi: 10.3389/fmicb.2022.933189
9. Grice EA, Segre JA. The skin microbiome. Nat Rev Microbiol (2011) 9(4):244–53. doi: 10.1038/nrmicro2537
10. Grice EA, Kong HH, Conlan S, Deming CB, Davis J, Young AC, et al. Topographical and temporal diversity of the human skin microbiome. Science (2009) 324(5931):1190–2. doi: 10.1126/science.1171700
11. Schoch CL, Seifert KA, Huhndorf S, Robert V, Spouge JL, Levesque CA, et al. Nuclear ribosomal internal transcribed spacer (ITS) region as a universal DNA barcode marker for Fungi. Proc Natl Acad Sci (2012) 109(16):6241–6. doi: 10.1073/pnas.1117018109
12. Banos S, Lentendu G, Kopf A, Wubet T, Glöckner FO, Reich M. A comprehensive fungi-specific 18S rRNA gene sequence primer toolkit suited for diverse research issues and sequencing platforms. BMC Microbiol (2018) 18(1):190. doi: 10.1186/s12866-018-1331-4
13. Kim M, Morrison M, Yu Z. Evaluation of different partial 16S rRNA gene sequence regions for phylogenetic analysis of microbiomes. J Microbiol Methods (2011) 84(1):81–7. doi: 10.1016/j.mimet.2010.10.020
14. Liu Z, Lozupone C, Hamady M, Bushman FD, Knight R. Short pyrosequencing reads suffice for accurate microbial community analysis. Nucleic Acids Res (2007) 35(18):e120–0. doi: 10.1093/nar/gkm541
15. Soergel DAW, Dey N, Knight R, Brenner SE. Selection of primers for optimal taxonomic classification of environmental 16S rRNA gene sequences. ISME J (2012) 6(7):1440–4. doi: 10.1038/ismej.2011.208
16. The Human Microbiome Project Consortium. A framework for human microbiome research. Nature (2012) 486(7402):215–21. doi: 10.1038/nature11209
17. Thompson LR, Sanders JG, McDonald D, Amir A, Ladau J, Locey KJ, et al. A communal catalogue reveals earth’s multiscale microbial diversity. Nature (2017) 551(7681):457–63. doi: 10.1038/nature24621
18. Knight R, Vrbanac A, Taylor BC, Aksenov A, Callewaert C, Debelius J, et al. Best practices for analysing microbiomes. Nat Rev Microbiol (2018) 16(7):410–22. doi: 10.1038/s41579-018-0029-9
19. Eren AM, Borisy GG, Huse SM, Mark Welch JL. Oligotyping analysis of the human oral microbiome(2014) (Accessed 2022 Dec 29).
20. Caporaso JG, Kuczynski J, Stombaugh J, Bittinger K, Bushman FD, Costello EK, et al. QIIME allows analysis of high-throughput community sequencing data. Nat Methods (2010) 7(5):335–6. doi: 10.1038/nmeth.f.303
21. Schloss PD, Westcott SL, Ryabin T, Hall JR, Hartmann M, Hollister EB, et al. Introducing mothur: Open-source, platform-independent, community-supported software for describing and comparing microbial communities. Appl Environ Microbiol (2009) 75(23):7537–41. doi: 10.1128/AEM.01541-09
22. Bolyen E, Rideout JR, Dillon MR, Bokulich NA, Abnet CC, Al-Ghalith GA, et al. Reproducible, interactive, scalable and extensible microbiome data science using QIIME 2. Nat Biotechnol (2019) 37(8):852–7. doi: 10.1038/s41587-019-0209-9
23. Amir A, McDonald D, Navas-Molina JA, Kopylova E, Morton JT, Zech Xu Z, et al. Deblur rapidly resolves single-nucleotide community sequence patterns. Gilbert JA editor. mSystems (2017) 2(2):e00191–16. doi: 10.1128/mSystems.00191-16
24. Barnes CJ, Rasmussen L, Asplund M, Knudsen SW, Clausen ML, Agner T, et al. Comparing DADA2 and OTU clustering approaches in studying the bacterial communities of atopic dermatitis. J Med Microbiol (2020) 69(11):1293–302. doi: 10.1099/jmm.0.001256
25. Silva C, Puente JL, Calva E. Salmonella virulence plasmid: pathogenesis and ecology(2017) (Accessed 2022 Nov 9).
26. McDonald D, Price MN, Goodrich J, Nawrocki EP, DeSantis TZ, Probst A, et al. An improved greengenes taxonomy with explicit ranks for ecological and evolutionary analyses of bacteria and archaea. ISME J (2012) 6(3):610–8. doi: 10.1038/ismej.2011.139
27. Cole JR, Wang Q, Cardenas E, Fish J, Chai B, Farris RJ, et al. The ribosomal database project: improved alignments and new tools for rRNA analysis. Nucleic Acids Res (2009) 37(Database):D141–5. doi: 10.1093/nar/gkn879
28. Langille MGI, Zaneveld J, Caporaso JG, McDonald D, Knights D, Reyes JA, et al. Predictive functional profiling of microbial communities using 16S rRNA marker gene sequences. Nat Biotechnol (2013) 31(9):814–21. doi: 10.1038/nbt.2676
29. Aßhauer KP, Wemheuer B, Daniel R, Meinicke P. Tax4Fun: predicting functional profiles from metagenomic 16S rRNA data: Fig. 1. Bioinf (2015) 31(17):2882–4. doi: 10.1093/bioinformatics/btv287
30. Quince C, Walker AW, Simpson JT, Loman NJ, Segata N. Shotgun metagenomics, from sampling to analysis. Nat Biotechnol (2017) 35(9):833–44. doi: 10.1038/nbt.3935
31. Ranjan R, Rani A, Metwally A, McGee HS, Perkins DL. Analysis of the microbiome: Advantages of whole genome shotgun versus 16S amplicon sequencing. Biochem Biophys Res Commun (2016) 469(4):967–77. doi: 10.1016/j.bbrc.2015.12.083
32. Durazzi F, Sala C, Castellani G, Manfreda G, Remondini D, De Cesare A. Comparison between 16S rRNA and shotgun sequencing data for the taxonomic characterization of the gut microbiota. Sci Rep (2021) 11(1):3030. doi: 10.1038/s41598-021-82726-y
33. Rausch P, Rühlemann M, Hermes BM, Doms S, Dagan T, Dierking K, et al. Comparative analysis of amplicon and metagenomic sequencing methods reveals key features in the evolution of animal metaorganisms. Microbiome (2019) 7(1):133. doi: 10.1186/s40168-019-0743-1
34. Peterson D, Bonham KS, Rowland S, Pattanayak CW, Consortium RESONANCE, Klepac-Ceraj V. Comparative analysis of 16S rRNA gene and metagenome sequencing in pediatric gut microbiomes. Front Microbiol (2021) 512:670336. doi: 10.3389/fmicb.2021.670336
35. Knights D, Beckman KB, Gohl DM, Zhu Q, Shields-Cutler RR, Al-Ghalith GA, et al. Evaluating the information content of shallow shotgun metagenomics. mSystem (2018) 3(6):12. doi: 10.1128/mSystems.00069-18
36. Yang C, Chowdhury D, Zhang Z, Cheung WK, Lu A, Bian Z, et al. A review of computational tools for generating metagenome-assembled genomes from metagenomic sequencing data. Comput Struct Biotechnol J (2021) 19:6301–14. doi: 10.1016/j.csbj.2021.11.028
37. Saheb Kashaf S, Proctor DM, Deming C, Saary P, Hölzer M, Program NISCCS, et al. Integrating cultivation and metagenomics for a multi-kingdom view of skin microbiome diversity and functions. Nat Microbiol (2021) 7(1):169–79. doi: 10.1038/s41564-021-01011-w
38. Bouslimani A, Porto C, Rath CM, Wang M, Guo Y, Gonzalez A, et al. Molecular cartography of the human skin surface in 3D(2015) (Accessed 2023 Jan 16).
39. Roux PF, Oddos T, Stamatas G. Deciphering the role of skin surface microbiome in skin health: An integrative multiomics approach reveals three distinct Metabolite−Microbe clusters. J Invest Dermatol (2022) 142(2):469–79. doi: 10.1016/j.jid.2021.07.159
40. Simon JC, Marchesi JR, Mougel C, Selosse MA. Host-microbiota interactions: from holobiont theory to analysis. Microbiome (2019) 7(1):5. doi: 10.1186/s40168-019-0619-4
41. Hernández Medina R, Kutuzova S, Nielsen KN, Johansen J, Hansen LH, Nielsen M, et al. Machine learning and deep learning applications in microbiome research. ISME Commun (2022) 2(1):98. doi: 10.1038/s43705-022-00182-9
42. Carrieri AP, Haiminen N, Maudsley-Barton S, Gardiner LJ, Murphy B, Mayes AE, et al. Explainable AI reveals changes in skin microbiome composition linked to phenotypic differences. Sci Rep (2021) 11(1):4565. doi: 10.1038/s41598-021-83922-6
43. Jaiswal SK, Agarwal SM, Thodum P, Sharma VK. SkinBug: an artificial intelligence approach to predict human skin microbiome-mediated metabolism of biotics and xenobiotics. iScience (2021) 24(1):101925. doi: 10.1016/j.isci.2020.101925
44. Gloor GB, Macklaim JM, Pawlowsky-Glahn V, Egozcue JJ. Microbiome datasets are compositional: And this is not optional. Front Microbiol (2017) 8:2224. doi: 10.3389/fmicb.2017.02224
45. Sullivan GM, Feinn R. Using effect size–or why the P value is not enough. J Grad Med Educ (2012) 4(3):279–82. doi: 10.4300/JGME-D-12-00156.1
46. Ferdous T, Jiang L, Dinu I, Groizeleau J, Kozyrskyj AL, Greenwood CMT, et al. The rise to power of the microbiome: Power and sample size calculation for microbiome studies. Mucosal Immunol (2022) 15(6):1060–70. doi: 10.1038/s41385-022-00548-1
47. Peeters J, Thas O, Shkedy Z, Kodalci L, Musisi C, Owokotomo OE, et al. Exploring the microbiome analysis and visualization landscape. Front Bioinforma (2021) 1:774631. doi: 10.3389/fbinf.2021.774631
48. Kong HH, Andersson B, Clavel T, Common JE, Jackson SA, Olson ND, et al. Performing skin microbiome research: A method to the madness. J Invest Dermatol (2017) 137(3):561–8. doi: 10.1016/j.jid.2016.10.033
49. Chen YE, Fischbach MA, Belkaid Y. Skin microbiota–host interactions. Nature (2018) 553(7689):427–36. doi: 10.1038/nature25177
50. Bjerre RD, Hugerth LW, Boulund F, Seifert M, Johansen JD, Engstrand L. Effects of sampling strategy and DNA extraction on human skin microbiome investigations. Sci Rep (2019) 9(1):17287. doi: 10.1038/s41598-019-53599-z
51. Marotz CA, Sanders JG, Zuniga C, Zaramela LS, Knight R, Zengler K. Improving saliva shotgun metagenomics by chemical host DNA depletion. Microbiome (2018) 6(1):42. doi: 10.1186/s40168-018-0426-3
52. Rajar P, Dhariwal A, Salvadori G, Junges R, Åmdal HA, Berild D, et al. Microbial DNA extraction of high-host content and low biomass samples: Optimized protocol for nasopharynx metagenomic studies. Front Microbiol (2022) 13:1038120. doi: 10.3389/fmicb.2022.1038120
53. Nelson MT, Pope CE, Marsh RL, Wolter DJ, Weiss EJ, Hager KR, et al. Human and extracellular DNA depletion for metagenomic analysis of complex clinical infection samples yields optimized viable microbiome profiles. Cell Rep (2019) 26(8):2227–2240.e5. doi: 10.1016/j.celrep.2019.01.091
54. Marotz C, Cavagnero KJ, Song SJ, McDonald D, Wandro S, Humphrey G, et al. Evaluation of the effect of storage methods on fecal, saliva, and skin microbiome composition. Beiko RG editor. mSystems (2021) 6(2):e01329–20. doi: 10.1128/mSystems.01329-20
55. Meisel JS, Hannigan GD, Tyldsley AS, SanMiguel AJ, Hodkinson BP, Zheng Q, et al. Skin microbiome surveys are strongly influenced by experimental design. J Invest Dermatol (2016) 136(5):947–56. doi: 10.1016/j.jid.2016.01.016
56. Chakravorty S, Helb D, Burday M, Connell N, Alland D. A detailed analysis of 16S ribosomal RNA gene segments for the diagnosis of pathogenic bacteria. J Microbiol Methods (2007) 69(2):330–9. doi: 10.1016/j.mimet.2007.02.005
57. Walters W, Hyde ER, Berg-Lyons D, Ackermann G, Humphrey G, Parada A, et al. Improved bacterial 16S rRNA gene (V4 and V4-5) and fungal internal transcribed spacer marker gene primers for microbial community surveys. Bik H editor. mSystems (2016) 1(1):e00009–15. doi: 10.1128/mSystems.00009-15
58. Callahan BJ, McMurdie PJ, Rosen MJ, Han AW, Johnson AJA, Holmes SP. DADA2: High-resolution sample inference from illumina amplicon data. Nat Methods (2016) 13(7):581–3. doi: 10.1038/nmeth.3869
59. Edgar RC. Updating the 97% identity threshold for 16S ribosomal RNA OTUs. Bioinformatics (2018) 34(14):2371–5. doi: 10.1093/bioinformatics/bty113
60. Lobanov V, Gobet A, Joyce A. Ecosystem-specific microbiota and microbiome databases in the era of big data. Environ Microbiome (2022) 17(1):37. doi: 10.1186/s40793-022-00433-1
61. Kaehler BD, Bokulich NA, McDonald D, Knight R, Caporaso JG, Huttley GA. Species abundance information improves sequence taxonomy classification accuracy. Nat Commun (2019) 10(1):4643. doi: 10.1038/s41467-019-12669-6
62. Comparative Sequencing Program NISC, Oh J, Byrd AL, Deming C, Conlan S, Kong HH, et al. Biogeography and individuality shape function in the human skin metagenome. Nature (2014) 514(7520):59–64. doi: 10.1038/nature13786
63. Oh J, Byrd AL, Park M, Kong HH, Segre JA. Temporal stability of the human skin microbiome. Cell (2016) 165(4):854–66. doi: 10.1016/j.cell.2016.04.008
64. Scholz CFP, Kilian M. The natural history of cutaneous propionibacteria, and reclassification of selected species within the genus propionibacterium to the proposed novel genera acidipropionibacterium gen. nov., cutibacterium gen. nov. and pseudopropionibacterium gen. nov. Int J Syst Evol Microbiol (2016) 66(11):4422–32. doi: 10.1099/ijsem.0.001367
65. Byrd AL, Belkaid Y, Segre JA. The human skin microbiome. Nat Rev Microbiol (2018) 16(3):143–55. doi: 10.1038/nrmicro.2017.157
66. Conwill A, Kuan AC, Damerla R, Poret AJ, Baker JS, Tripp AD, et al. Anatomy promotes neutral coexistence of strains in the human skin microbiome. Cell Host Microbe (2022) 30(2):171–82.e7. doi: 10.1016/j.chom.2021.12.007
67. Nguyen UT, Kalan LR. Forgotten fungi: the importance of the skin mycobiome. Curr Opin Microbiol (2022) 70:102235. doi: 10.1016/j.mib.2022.102235
68. Foulongne V, Sauvage V, Hebert C, Dereure O, Cheval J, Gouilh MA, et al. Human skin microbiota: High diversity of DNA viruses identified on the human skin by high throughput sequencing. PloS One (2012) 7(6):e38499. doi: 10.1371/journal.pone.0038499
69. Wylie KM, Mihindukulasuriya KA, Zhou Y, Sodergren E, Storch GA, Weinstock GM. Metagenomic analysis of double-stranded DNA viruses in healthy adults. BMC Biol (2014) 12:71. doi: 10.1186/s12915-014-0071-7
70. Grice EA. The intersection of microbiome and host at the skin interface: Genomic- and metagenomic-based insights. Genome Res (2015) 25(10):1514–20. doi: 10.1101/gr.191320.115
71. Daou H, Paradiso M, Hennessy K, Seminario-Vidal L. Rosacea and the microbiome: A systematic review. Dermatol Ther (2021) 11(1):1–12. doi: 10.1007/s13555-020-00460-1
72. Chen W, Plewig G. Human demodicosis: revisit and a proposed classification. Br J Dermatol (2014) 170(6):1219–25. doi: 10.1111/bjd.12850
73. O’Reilly N, Menezes N, Kavanagh K. Positive correlation between serum immunoreactivity to Demodex -associated Bacillus proteins and erythematotelangiectatic rosacea: Correlation between Bacillus proteins from Demodex and erythematotelangiectatic rosacea. Br J Dermatol (2012) 167(5):1032–6. doi: 10.1111/j.1365-2133.2012.11114.x
74. Moissl-Eichinger C, Probst AJ, Birarda G, Auerbach A, Koskinen K, Wolf P, et al. Human age and skin physiology shape diversity and abundance of archaea on skin. Sci Rep (2017) 7(1):4039. doi: 10.1038/s41598-017-04197-4
75. Probst AJ, Auerbach AK, Moissl-Eichinger C. Archaea on human skin. PloS One (2013) 8(6):e65388. doi: 10.1371/journal.pone.0065388
76. Umbach AK, Stegelmeier AA, Neufeld JD. Archaea are rare and uncommon members of the mammalian skin microbiome. mSystems (2021) 6(4):e00642–21. doi: 10.1128/mSystems.00642-21
77. Koskinen K, Pausan MR, Perras AK, Beck M, Bang C, Mora M, et al. First insights into the diverse human archaeome: Specific detection of archaea in the gastrointestinal tract, lung, and nose and on skin. mBio (2017) 8(6):e00824–17. doi: 10.1128/mBio.00824-17
78. Bjerre RD, Holm JB, Palleja A, Sølberg J, Skov L, Johansen JD. Skin dysbiosis in the microbiome in atopic dermatitis is site-specific and involves bacteria, fungus and virus. BMC Microbiol (2021) 21(1):256. doi: 10.1186/s12866-021-02302-2
79. Byrd AL, Deming C, Cassidy SKB, Harrison OJ, Ng WI, Conlan S, et al. Staphylococcus aureus and Staphylococcus epidermidis strain diversity underlying pediatric atopic dermatitis. Sci Transl Med (2017) 9(397):eaal4651. doi: 10.1126/scitranslmed.aal4651
80. Saxena R, Mittal P, Clavaud C, Dhakan DB, Hegde P, Veeranagaiah MM, et al. Comparison of healthy and dandruff scalp microbiome reveals the role of commensals in scalp health. Front Cell Infect Microbiol (2018) 8:346. doi: 10.3389/fcimb.2018.00346
81. Barnard E, Shi B, Kang D, Craft N, Li H. The balance of metagenomic elements shapes the skin microbiome in acne and health. Sci Rep (2016) 6(1):39491. doi: 10.1038/srep39491
82. Tett A, Pasolli E, Farina S, Truong DT, Asnicar F, Zolfo M, et al. Unexplored diversity and strain-level structure of the skin microbiome associated with psoriasis. NPJ Biofilms Microbiomes (2017) 3(1):14. doi: 10.1038/s41522-017-0022-5
83. Williams MR, Cau L, Wang Y, Kaul D, Sanford JA, Zaramela LS, et al. Interplay of staphylococcal and host proteases promotes skin barrier disruption in netherton syndrome. Cell Rep (2020) 30(9):2923–2933.e7. doi: 10.1016/j.celrep.2020.02.021
84. Nutten S. Atopic dermatitis: Global epidemiology and risk factors. Ann Nutr Metab (2015) 66(Suppl. 1):8–16. doi: 10.1159/000370220
85. Kong HH, Oh J, Deming C, Conlan S, Grice EA, Beatson MA, et al. Temporal shifts in the skin microbiome associated with disease flares and treatment in children with atopic dermatitis. Genome Res (2012) 22(5):850–9. doi: 10.1101/gr.131029.111
86. Leyden JJ, Marples RR, Kligman AM. Staphylococcus aureus in the lesions of atopic dermatitis. Br J Dermatol (1974) 90(5):525–5. doi: 10.1111/j.1365-2133.1974.tb06447.x
87. Meylan P, Lang C, Mermoud S, Johannsen A, Norrenberg S, Hohl D, et al. Skin colonization by staphylococcus aureus precedes the clinical diagnosis of atopic dermatitis in infancy. J Invest Dermatol (2017) 137(12):2497–504. doi: 10.1016/j.jid.2017.07.834
88. Shi B, Bangayan NJ, Curd E, Taylor PA, Gallo RL, Leung DYM, et al. The skin microbiome is different in pediatric versus adult atopic dermatitis. J Allergy Clin Immunol (2016) 138(4):1233–6. doi: 10.1016/j.jaci.2016.04.053
89. Di Domenico EG, Cavallo I, Capitanio B, Ascenzioni F, Pimpinelli F, Morrone A, et al. Staphylococcus aureus and the cutaneous microbiota biofilms in the pathogenesis of atopic dermatitis. Microorganisms (2019) 7(9):301. doi: 10.3390/microorganisms7090301
90. Bekeredjian-Ding I, Inamura S, Giese T, Moll H, Endres S, Sing A, et al. Staphylococcus aureus protein a triggers T cell-independent b cell proliferation by sensitizing b cells for TLR2 ligands. J Immunol (2007) 178(5):2803–12. doi: 10.4049/jimmunol.178.5.2803
91. Spaulding AR, Salgado-Pabón W, Kohler PL, Horswill AR, Leung DYM, Schlievert PM. Staphylococcal and streptococcal superantigen exotoxins. Clin Microbiol Rev (2013) 26(3):422–47. doi: 10.1128/CMR.00104-12
92. Nakagawa S, Matsumoto M, Katayama Y, Oguma R, Wakabayashi S, Nygaard T, et al. Staphylococcus aureus virulent PSMα peptides induce keratinocyte alarmin release to orchestrate IL-17-Dependent skin inflammation. Cell Host Microbe (2017) 22(5):667–677.e5. doi: 10.1016/j.chom.2017.10.008
93. Vu AT, Baba T, Chen X, Le TA, Kinoshita H, Xie Y, et al. Staphylococcus aureus membrane and diacylated lipopeptide induce thymic stromal lymphopoietin in keratinocytes through the toll-like receptor 2–toll-like receptor 6 pathway. J Allergy Clin Immunol (2010) 126(5):985–93.e3. doi: 10.1016/j.jaci.2010.09.002
94. Nakamura Y, Oscherwitz J, Cease KB, Chan SM, Muñoz-Planillo R, Hasegawa M, et al. Staphylococcus δ-toxin induces allergic skin disease by activating mast cells. Nature (2013) 503(7476):397–401. doi: 10.1038/nature12655
95. Shaw L, Golonka E, Potempa J, Foster SJ. The role and regulation of the extracellular proteases of staphylococcus aureus. Microbiology (2004) 150(1):217–28. doi: 10.1099/mic.0.26634-0
96. Seite S, Flores G, Henley J, Martin R, Zelenkova H, Luc A. Microbiome of affected and unaffected skin of patients with atopic dermatitis before and after emollient treatment. J Drugs Dermatol (2014) 13(11):1365–72.
97. Hon KL, Tsang YCK, Pong NH, Leung TF, Ip M. Exploring staphylococcus epidermidis in atopic eczema: Friend or foe? Clin Exp Dermatol (2016) 41(6):659–63. doi: 10.1111/ced.12866
98. Brown MM, Horswill AR. Staphylococcus epidermidis–skin friend or foe? PloS Pathog (2020) 16(11):e1009026. doi: 10.1371/journal.ppat.1009026
99. Wanke I, Steffen H, Christ C, Krismer B, Götz F, Peschel A, et al. Skin commensals amplify the innate immune response to pathogens by activation of distinct signaling pathways. J Invest Dermatol (2011) 131(2):382–90. doi: 10.1038/jid.2010.328
100. Cogen AL, Yamasaki K, Muto J, Sanchez KM, Crotty Alexander L, Tanios J, et al. Staphylococcus epidermidis antimicrobial δ-toxin (Phenol-soluble modulin-γ) cooperates with host antimicrobial peptides to kill group a streptococcus. PloS One (2010) 55(1):e8557. doi: 10.1371/journal.pone.0008557
101. Conlan S, Mijares LA NISC, Comparative Sequencing Program, Becker J, RW B, GG B, et al. Staphylococcus epidermidis pan-genome sequence analysis reveals diversity of skin commensal and hospital infection-associated isolates. Genome Biol (2012) 13(7):R64. doi: 10.1186/gb-2012-13-7-r64
102. Ochlich D, Rademacher F, Drerup KA, Gläser R, Harder J. The influence of the commensal skin bacterium staphylococcus epidermidis on the epidermal barrier and inflammation: Implications for atopic dermatitis. Exp Dermatol (2022) 20:exd.14727. doi: 10.1111/exd.14727
103. Burian M, Bitschar K, Dylus B, Peschel A, Schittek B. The protective effect of microbiota on s. aureus skin colonization depends on the integrity of the epithelial barrier. J Invest Dermatol (2017) 137(4):976–9. doi: 10.1016/j.jid.2016.11.024
104. Cau L, Williams MR, Butcher AM, Nakatsuji T, Kavanaugh JS, Cheng JY, et al. Staphylococcus epidermidis protease EcpA can be a deleterious component of the skin microbiome in atopic dermatitis. J Allergy Clin Immunol (2021) 147(3):955–966.e16. doi: 10.1016/j.jaci.2020.06.024
105. Chng KR, Tay ASL, Li C, Ng AHQ, Wang J, Suri BK, et al. Whole metagenome profiling reveals skin microbiome-dependent susceptibility to atopic dermatitis flare. Nat Microbiol (2016) 1(9):16106. doi: 10.1038/nmicrobiol.2016.106
106. Tao R, Li R, Wang R. Skin microbiome alterations in seborrheic dermatitis and dandruff: A systematic review. Exp Dermatol (2021) 30(10):1546–53. doi: 10.1111/exd.14450
107. Lin Q, Panchamukhi A, Li P, Shan W, Zhou H, Hou L, et al. Malassezia and staphylococcus dominate scalp microbiome for seborrheic dermatitis. Bioprocess Biosyst Eng (2021) 44(5):965–75. doi: 10.1007/s00449-020-02333-5
108. Carmona-Cruz S, Orozco-Covarrubias L, Sáez-de-Ocariz M. The human skin microbiome in selected cutaneous diseases. Front Cell Infect Microbiol (2022) 12:834135. doi: 10.3389/fcimb.2022.834135
109. Goularte-Silva V, Paulino LC. Ketoconazole beyond antifungal activity: Bioinformatics-based hypothesis on lipid metabolism in dandruff and seborrheic dermatitis. Exp Dermatol (2022) 31(5):821–2. doi: 10.1111/exd.14505
110. Tan JKL, Bhate K. A global perspective on the epidemiology of acne. Br J Dermatol (2015) 172:3–12. doi: 10.1111/bjd.13462
111. Vallerand IA, Lewinson RT, Parsons LM, Lowerison MW, Frolkis AD, Kaplan GG, et al. Risk of depression among patients with acne in the U.K.: A population-based cohort study. Br J Dermatol (2018) 178(3):e194–5. doi: 10.1111/bjd.16099
112. O’Neill AM, Gallo RL. Host-microbiome interactions and recent progress into understanding the biology of acne vulgaris. Microbiome (2018) 6(1):177. doi: 10.1186/s40168-018-0558-5
113. Nakatsuji T, Tang D chu C, Zhang L, Gallo RL, Huang CM. Propionibacterium acnes CAMP factor and host acid sphingomyelinase contribute to bacterial virulence: Potential targets for inflammatory acne treatment. Horsburgh MJ editor. PloS One (2011) 6(4):e14797. doi: 10.1371/journal.pone.0014797
114. Rimon A, Rakov C, Lerer V, Sheffer-Levi S, Oren SA, Shlomov T, et al. Topical phage therapy in a mouse model of cutibacterium acnes-induced acne-like lesions. Nat Commun (2023) 14(1):1005. doi: 10.1038/s41467-023-36694-8
115. Jahns AC, Lundskog B, Ganceviciene R, Palmer RH, Golovleva I, Zouboulis CC, et al. An increased incidence of propionibacterium acnes biofilms in acne vulgaris: a case-control study: Increased incidence of p. acnes biofilms in acne vulgaris. Br J Dermatol (2012) 167(1):50–8. doi: 10.1111/j.1365-2133.2012.10897.x
116. Fitz-Gibbon S, Tomida S, Chiu BH, Nguyen L, Du C, Liu M, et al. Propionibacterium acnes strain populations in the human skin microbiome associated with acne. J Investigate Derma (2013) 133:2152–60. doi: 10.1038/jid.2013.21
117. Dagnelie MA, Khammari A, Dréno B, Corvec S. Cutibacterium acnes molecular typing: time to standardize the method. Clin Microbiol Infect (2018) 24(11):1149–55. doi: 10.1016/j.cmi.2018.03.010
118. Salar-Vidal L, Achermann Y, Aguilera-Correa JJ, Poehlein A, Esteban J, Brüggemann H, et al. Genomic analysis of cutibacterium acnes strains isolated from prosthetic joint infections. Microorganisms (2021) 9(7):1500. doi: 10.3390/microorganisms9071500
119. Spittaels KJ, Coenye T. Developing an in vitro artificial sebum model to study propionibacterium acnes biofilms. Anaerobe (2018) 49:21–9. doi: 10.1016/j.anaerobe.2017.11.002
120. Kasimatis G, Fitz-Gibbon S, Tomida S, Wong M, Li H. Analysis of complete genomes of Propionibacterium acnes reveals a novel plasmid and increased pseudogenes in an acne associated strain. BioMed Res Int (2013) 2013:1–11. doi: 10.1155/2013/918320
121. Dreno B, Martin R, Moyal D, Henley JB, Khammari A, Seité S. Skin microbiome and acne vulgaris Staphylococcus, a new actor in acne. Exp Dermatol (2017) 26(9):798–803. doi: 10.1111/exd.13296
122. Bronnec V, Eilers H, Jahns AC, Omer H, Alexeyev OA. Propionibacterium (Cutibacterium) granulosum extracellular DNase BmdE targeting propionibacterium (Cutibacterium) acnes biofilm matrix, a novel inter-species competition mechanism. Front Cell Infect Microbiol (2022) 11:809792. doi: 10.3389/fcimb.2021.809792
123. Greb JE, Goldminz AM, Elder JT, Lebwohl MG, Gladman DD, Wu JJ, et al. Psoriasis. Nat Rev Dis Primer (2016) 2(1):16082. doi: 10.1038/nrdp.2016.82
125. Weisenseel P. Streptococcal infection distinguishes different types of psoriasis. J Med Genet (2002) 39(10):767–8. doi: 10.1136/jmg.39.10.767
126. Lewis DJ, Chan WH, Hinojosa T, Hsu S, Feldman SR. Mechanisms of microbial pathogenesis and the role of the skin microbiome in psoriasis: A review. Clin Dermatol (2019) 37(2):160–6. doi: 10.1016/j.clindermatol.2019.01.011
127. Chang HW, Yan D, Singh R, Liu J, Lu X, Ucmak D, et al. Alteration of the cutaneous microbiome in psoriasis and potential role in Th17 polarization. Microbiome (2018) 6(1):154. doi: 10.1186/s40168-018-0533-1
128. Tutka K, Żychowska M, Reich A. Diversity and composition of the skin, blood and gut microbiome in rosacea–a systematic review of the literature. Microorganisms (2020) 8(11):1756. doi: 10.3390/microorganisms8111756
129. Holmes AD. Potential role of microorganisms in the pathogenesis of rosacea. J Am Acad Dermatol (2013) 69(6):1025–32. doi: 10.1016/j.jaad.2013.08.006
130. Casas C, Paul C, Lahfa M, Livideanu B, Lejeune O, Alvarez-Georges S, et al. Quantification of Demodex folliculorum by PCR in rosacea and its relationship to skin innate immune activation. Exp Dermatol (2012) 21(12):906–10. doi: 10.1111/exd.12030
131. Whitfeld M, Gunasingam N, Leow LJ, Shirato K, Preda V. Staphylococcus epidermidis: A possible role in the pustules of rosacea. J Am Acad Dermatol (2011) 64(1):49–52. doi: 10.1016/j.jaad.2009.12.036
132. Dahl MV, Ross AJ, Schlievert PM. Temperature regulates bacterial protein production: possible role in rosacea. J Am Acad Dermatol (2004) 50(2):266–72. doi: 10.1016/j.jaad.2003.05.005
133. Wang R, Farhat M, Na J, Li R, Wu Y. Bacterial and fungal microbiome characterization in patients with rosacea and healthy controls. Br J Dermatol (2020) 183(6):1112–4. doi: 10.1111/bjd.19315
134. Chopra D, Arens RA, Amornpairoj W, Lowes MA, Tomic-Canic M, Strbo N, et al. Innate immunity and microbial dysbiosis in hidradenitis suppurativa – vicious cycle of chronic inflammation. Front Immunol (2022) 13:960488. doi: 10.3389/fimmu.2022.960488
135. Nomura T. Hidradenitis suppurativa as a potential subtype of autoinflammatory keratinization disease. Front Immunol (2020) 11:847. doi: 10.3389/fimmu.2020.00847
136. Goldburg SR, Strober BE, Payette MJ. Hidradenitis suppurativa. J Am Acad Dermatol (2020) 82(5):1045–58. doi: 10.1016/j.jaad.2019.08.090
137. Mintoff D, Borg I, Pace NP. The clinical relevance of the microbiome in hidradenitis suppurativa: A systematic review. Vaccines (2021) 9(10):1076. doi: 10.3390/vaccines9101076
138. Riverain-Gillet É, Guet-Revillet H, Jais JP, Ungeheuer MN, Duchatelet S, Delage M, et al. The surface microbiome of clinically unaffected skinfolds in hidradenitis suppurativa: A cross-sectional culture-based and 16S rRNA gene amplicon sequencing study in 60 patients. J Invest Dermatol (2020) 140(9):1847–1855.e6. doi: 10.1016/j.jid.2020.02.046
139. Ring HC, Sigsgaard V, Thorsen J, Fuursted K, Fabricius S, Saunte DM, et al. The microbiome of tunnels in hidradenitis suppurativa patients. J Eur Acad Dermatol Venereol (2019) 33(9):1775–80. doi: 10.1111/jdv.15597
140. Ring HC, Thorsen J, Saunte DM, Lilje B, Bay L, Riis PT, et al. The follicular skin microbiome in patients with hidradenitis suppurativa and healthy controls. JAMA Dermatol (2017) 153(9):897. doi: 10.1001/jamadermatol.2017.0904
141. Guet-Revillet H, Jais JP, Ungeheuer MN, Coignard-Biehler H, Duchatelet S, Delage M, et al. The microbiological landscape of anaerobic infections in hidradenitis suppurativa: A prospective metagenomic study. Clin Infect Dis (2017) 65(2):282–91. doi: 10.1093/cid/cix285
142. Naik HB, Jo JH, Paul M, Kong HH. Skin microbiota perturbations are distinct and disease severity–dependent in hidradenitis suppurativa. J Invest Dermatol (2020) 140(4):922–5.e3. doi: 10.1016/j.jid.2019.08.445
143. Ring HC, Thorsen J, Jørgensen AH, Bay L, Bjarnsholt T, Fuursted K, et al. Predictive metagenomic analysis reveals a role of cutaneous dysbiosis in the development of hidradenitis suppurativa. J Invest Dermatol (2020) 140(7):1473–6. doi: 10.1016/j.jid.2019.11.011
144. Świerczewska Z, Lewandowski M, Surowiecka A, Barańska-Rybak W. Microbiome in hidradenitis suppurativa–what we know and where we are heading. Int J Mol Sci (2022) 23(19):11280. doi: 10.3390/ijms231911280
145. Gutiérrez-Cerrajero C, Sprecher E, Paller AS, Akiyama M, Mazereeuw-Hautier J, Hernández-Martín A, et al. Ichthyosis. Nat Rev Dis Primers (2023) 9:2. doi: 10.1038/s41572-022-00412-3
146. Lee SY, Lee E, Park YM, Hong SJ. Microbiome in the gut-skin axis in atopic dermatitis. Allergy Asthma Immunol Res (2018) 10(4):354. doi: 10.4168/aair.2018.10.4.354
147. Siddiqui R, Makhlouf Z, Khan NA. The increasing importance of the gut microbiome in acne vulgaris. Folia Microbiol (Praha) (2022) 67(6):825–35. doi: 10.1007/s12223-022-00982-5
148. Chen L, Li J, Zhu W, Kuang Y, Liu T, Zhang W, et al. Skin and gut microbiome in psoriasis: Gaining insight into the pathophysiology of it and finding novel therapeutic strategies. Front Microbiol (2020) 11:589726. doi: 10.3389/fmicb.2020.589726
149. Luck ME, Tao J, Lake EP. The skin and gut microbiome in hidradenitis suppurativa: Current understanding and future considerations for research and treatment. Am J Clin Dermatol (2022) 23(6):841–52. doi: 10.1007/s40257-022-00724-w
Keywords: microbiome and dysbiosis, genomics, metagenomics, next-generation sequencing, atopic dermatitis (AD), acne (acne vulgaris), psoriasis
Citation: Chen Y, Knight R and Gallo RL (2023) Evolving approaches to profiling the microbiome in skin disease. Front. Immunol. 14:1151527. doi: 10.3389/fimmu.2023.1151527
Received: 26 January 2023; Accepted: 14 March 2023;
Published: 04 April 2023.
Edited by:
Tej Pratap Singh, University of Pennsylvania, United StatesReviewed by:
Jürgen Harder, University of Kiel, GermanyChao Yuan, Shanghai Dermatology Hospital, China
Copyright © 2023 Chen, Knight and Gallo. This is an open-access article distributed under the terms of the Creative Commons Attribution License (CC BY). The use, distribution or reproduction in other forums is permitted, provided the original author(s) and the copyright owner(s) are credited and that the original publication in this journal is cited, in accordance with accepted academic practice. No use, distribution or reproduction is permitted which does not comply with these terms.
*Correspondence: Richard L. Gallo, cmdhbGxvQGhlYWx0aC51Y3NkLmVkdQ==