- 1Department of Hematology, First Affiliated Hospital of Zhejiang Chinese Medical University, Hangzhou, China
- 2Department of Lymphoma, Cancer Hospital of University of Chinese Academy of Sciences, Hangzhou, China
- 3Department of Hematology, The Second Affiliated Hospital of Zhejiang University School of Medicine, Hangzhou, China
- 4Department of Hematology, Lishui People’s Hospital, Lishui, China
- 5Department of Hematology, Ningbo Yinzhou No.2 Hospital, Ningbo, China
- 6Department of Hematology, Shaoxing People’s Hospital, Shaoxing, China
- 7Department of Hematology, Jinhua People’s Hospital, Jinhua, China
- 8Department of Hematology, The First Hospital Affiliated to Wenzhou Medical University, Weizhou, China
Background/purpose: It has been demonstrated that gut microbes are closely associated with the pathogenesis of lymphoma, but the gut microbe landscape and its association with immune cells in diffuse large B-cell lymphoma (DLBCL) remain largely unknown. In this study, we explored the associations between gut microbiota, clinical features and peripheral blood immune cell subtypes in DLBCL.
Method: A total of 87 newly diagnosed DLBCL adults were enrolled in this study. The peripheral blood samples were collected from all patients and then submitted to immune cell subtyping using full-spectral flow cytometry. Metagenomic sequencing was applied to assess the microbiota landscape of 69 of 87 newly diagnosed DLBCL patients. The microbiotas and peripheral blood immune cell subsets with significant differences between different National Comprehensive Center Network-International Prognostic Indexes (NCCN-IPIs) (low-risk, low-intermediate-risk, intermediate-high-risk, high-risk) groups were screened.
Results: A total of 10 bacterial phyla, 31 orders and 455 bacteria species were identified in 69 patients with newly diagnosed DLBCL. The abundances of 6 bacteria, including Blautia sp.CAG 257, Actinomyces sp.S6 Spd3, Streptococcus parasanguinis, Bacteroides salyersiae, Enterococcus faecalls and Streptococcus salivarius were significantly different between the low-risk, low-intermediate-risk, intermediate-high-risk and high-risk groups, among which Streptococcus parasanguinis and Streptococcus salivarius were markedly accumulated in the high-risk group. The different bacteria species were mostly enriched in the Pyridoxal 5’-phosphate biosynthesis I pathway. In addition, we found that 2 of the 6 bacteria showed close associations with the different immune cell subtypes which were also identified from different NCCN-IPIs. In detail, the abundance of Bacteroides salyersiae was negatively correlated with Treg cells, CD38+ nonrescue exhausted T cells, nature killer 3 cells and CD38+CD8+ effector memory T cells, while the abundance of Streptococcus parasanguinis was negatively correlated with HLA-DR+ NK cells, CD4+ Treg cells, HLA-DR+ NKT cells and HLA-DR+CD94+CD159c+ NKT cells.
Conclusion: This study first reveals the gut microbiota landscape of patients with newly diagnosed DLBCL and highlights the association between the gut microbiota and immunity, which may provide a new idea for the prognosis assessment and treatment of DLBCL.
1 Introduction
Lymphoma, a malignant tumor originating from the lymphohematopoietic system, is one of the most common cancer in both men and women (1). Diffuse large B-cell lymphoma (DLBCL) is the most common subtype of lymphoma worldwide, accounting for 40% of non-Hodgkin lymphomas (2). Despite R-CHOP regimen (rituximab, cyclophosphamide, doxorubicin, vincristine, and prednisone) significantly improves the complete remission rate of DLBCL, one-third patients still do not benefit from it and have a poor prognosis (3). It is urgent to deeply reveal the mechanisms underlying the pathogenesis of DLBCL and develop potent therapeutic strategy.
Microbiome is becoming crucial in maintaining the balance between human health and diseases. Harmful gut microbiotas trigger fatigue, dry skin, headaches, vomiting and other physical discomforts, or accelerate the aging of the intestinal wall, produce carcinogens and eventually lead to gastrointestinal cancers (4, 5). Also, intestinal microecology plays an important role in the development and progression of hematological malignancies (6). Gao et al. (7) found that the dominant intestinal microflora constitutions in children with acute lymphoblastic leukemia were significantly different from healthy controls, with a poorer gut microbial diversity. Cozen et al. (8) using the 16S ribosomal RNA sequencing revealed a lower microbial diversity in patients with Hodgkin’s lymphoma as compared to healthy individuals. Additionally, the gut microbiotas play an indispensable role in the pathogenesis of DLBCL (9). In detail, higher abundance of Proteobacteria was found to be associated with lower immunity and poor survival of DLBCL patients (9). Moreover, the gut microbial components were associated with the response to checkpoint blockade therapies (CBT) and prognosis of cancers (10). For example, the alpha diversity and relative abundance of bacteria of the Ruminococcaceae family were significantly increased in the responders to anti-PD-1 immunotherapy as compared with the nonresponders in melanoma (11). Perturbations of the microbiota composition (B. fragilis and/or B. thetaiotaomicron and Burkholderiales) improved the efficacy of CTLA-4 blockade through promoting T cell-dependent intestinal epithelial cell death (12). However, the profiles and clinical significances of gut microbiota in DLBCL have not been fully understood.
Peripheral immune system, as the first immune barrier of human body, plays an important role in the diagnosis and prognosis of DLBCL (13, 14). For instance, the absolute peripheral monocyte count at diagnosis is an independent risk factor to predict the central nervous system relapse in patients with DLBCL (15). The higher proportion of helper cells is associated with shorter survival in DLBCL patients, especially in younger patients (14). Noticeably, gastrointestinal tract provides a critical interface where crosstalk between the enormous number of microorganisms and the host immune system takes place, allowing the gut microbiome modulates the host immune system both locally and systemically (16). Exploration of the relationship between gut microbial and immune system may provide a new idea for the treatment of DLBCL.
International Prognostic Index (IPI), revised IPI (R-IPI), and National Comprehensive Cancer Network IPI (NCCN-IPI) are the three scoring systems used to predict the prognosis of DLBCL. Among them, NCCN-IPI outperforms the IPI and R-IPI systems (17). To disclose the relationship between gut microbiota and prognosis, we investigated the association between intestinal bacteria and the NCCN-IPI in DLBCL patients. In addition, we studied the associations between intestinal bacteria and immune cell subtypes. This study may provide novel biomarkers used for DLBCL prognostic prediction and new ideas for treatment strategy.
2 Materials and methods
2.1 Sample collection and preparation
A total of 87 newly diagnosed DLBCL patients were recruited from eight hospitals of Zhejiang Province, including the First Affiliated Hospital of Zhejiang Chinese Medical University (n=14), the Second Affiliated Hospital of Zhejiang University School of Medicine (n=16), Cancer Hospital of the University of Chinese Academy of Sciences (n=31), the First Affiliated Hospital of Wenzhou Medical University (n=3), Lishui People’s Hospital (n=6), Shaoxing People’s Hospital (n=7), Jinhua People’s Hospital (n=3) and Ningbo Yinzhou No.2 Hospital (n=7) between 2020 and 2021 (Table 1). The diagnosis of DLBCL was made based on the International Consensus Classification of Mature Lymphoid Neoplasms: a report from the Clinical Advisory Committee and the 2022 revision of the World Health Organization classification of Haematolymphoid Tumours: Lymphoid Neoplasms (18, 19). The inclusion criteria were as follows (1): Age ≥ 18years (2), Tissue biopsy confirmed DLBCL, and the patient had not been treated before (3), No history of other major illnesses. The exclusion criteria for DLBCL patients were as follows (1): Patients with a history of other major diseases (e.g., mental illnesses, hepatitis or severe gastrointestinal diseases) (2), Patients who had used antibiotics within 30 days.
Both blood and feces samples were collected from naïve DLBCL patients before treatment. In detail, 5–10 mL of fresh peripheral blood was collected from 87 patients and stored at the EDTA containing tubes. A total of 69 qualified feces specimens were collected from 69 of the 87 patients, for which the inner middle section was intercepted with a fecal sampler and dispensed into 2 mL EP tubes with 0.5-2 g feces per tube.
Clinicopathological information was collected for each patient, including NCCN-IPI, age, Ann Arbor stage, extranodal involvement number, lactate dehydrogenase (LDH) level, and Eastern Cooperative Oncology Group (ECOG) performance status. Based on NCCN-IPI, the 87 patients were categorized into 4 groups, low-risk (L) group (0-1, n=15), low-intermediate-risk (LM) group (2-3, n=41), high-intermediate-risk (MH) group (4-5, n=23) and high-risk (H) group (6–8, n=8). Each participant provided the written informed consent. Our research was performed in accordance with the ethical standards formulated in the Helsinki Declaration and approved by the Ethics Committee of the First Affiliated Hospital of Zhejiang Chinese Medical University (2020-KL-027-02).
2.2 Metagenomic sequencing and data processing
Genomic DNA from 69 fecal samples was extracted using the EasyPure® Stool Genomic DNA Kit (TransGen Biotech Co., Ltd, Beijing, China), and the metagenomic DNA library was constructed using the Nextera XT DNA Library Preparation Kit (Illumina, California, USA) following the manufacturer’s instructions. The quality of libraries was evaluated using an Agilent bioanalyser with a DNA LabChip 1000 Kit (Agilent, Palo Alto, USA). Samples with genomic DNA concentration less than 10 ng/μL, or abnormal quality (OD260/280>2.5, or OD260/280<1.5) were filtered out. Then, the cBOT was sequenced using the Illumina high-throughput sequencing platform NovaSeq6000 (Illumina, California, USA) according to the patent description provided by the Kangmeihuada Gene Technology Co., Ltd (Shenzhen, China) (20). After the low quality and ambiguous bases of the raw reads were filtered, the remaining reads were aligned to human genome reference (hg37) by KneadData to remove human host DNA contamination (21, 22). The average rate of host contamination was (1.53 ± 2.18) %.
2.3 Gene catalogue construction
Metagenomics of the Human Intestinal Tract (MetaHIT) gene catalogue was constructed according to previous descriptions (23). We performed de novo assembling and gene prediction for the high-quality reads of 69 samples using SOAPdenovo-28 (version 2.04). MetaGeneMark31 (version 3.26) was used to identify open reading frames (ORFs) from the contigs of each sample. Then, the non-redundant gene catalogue was constructed by pairwise comparison of all the predicted ORFs with CD-HIT32 (version 4.5.7). All predicted genes were aligned pairwise using BLAT. Genes that over 90% of their length can be aligned to another one with more than 95% identity (no gaps allowed) were removed as redundancies (24). MetaPhlAn (version 3.0) was used to perform species identification with default parameters. Microbiome constitution and abundance were analyzed using an absolute quantification method provided by the Kangmeihuada Gene Technology Co., Ltd (Shenzhen, China) (20).
2.4 Full-spectral flow cytometry
Full-spectral flow cytometry was used for immunophenotyping of B lymphocytes, T lymphocytes and NK lymphocytes of the peripheral blood samples (Figure S1). Detailly, the mixed non-bauble violet (BV) antibodies and brilliant staining buffer (Becton, Dickinson and Company, New York, USA) were added to the peripheral blood samples, and incubated for 15 min at room temperature in the dark. Then, lysis buffer (Becton, Dickinson and Company, New York, USA) was added to the samples, and the supernatant was discarded following 10 min of centrifugation. Sediment was washed with fixation/permeabilization buffer (Thermo Fisher Scientific, Massachusetts, USA) followed by centrifugation and incubated with cytoplasmic antibodies for 30 min in the dark. Phosphate buffered saline (Beyotime Biotechnology, Shanghai, China) was used to wash the samples. Finally, the supernatant was removed by centrifugation, and processed in a full-spectrum flow cytometer (Shanghai Xiatai Biotechnology Co., Ltd., Shanghai, China).
2.5 Statistical analysis
Sequencing data was analyzed using the corrplot (version 0.84), vegan (version 2.5-7) and ggplot2 packages of R (version 4.1.0). Phylogenetic tree was plotted by GraPhlAn (version 1.1.4) (25). Heatmap was drawn using the ComplexHeatmap (version 2.2.0) package in R (version 4.1.0) (26). Shannon index and Chao1 index were calculated using QIIME (27). Unifrac distance of principal component analysis (PCA) was generated using the scripts (Metaphlan, version 3.0) (28). PERMANOVA with the adonis function at 999 permutations was used from the vegan package. Dynamic Meta-Storms distance of principal coordinates analysis (PCoA) was generated using dynamic-meta-storms (version 1.1) tools (29).
The top20 most abundant microbes were plotted. RandomForest package (version 4.7-1) was used to identify species associated with NCCN-IPI scores. Differential abundance analysis was performed using Linear discriminant analysis effect size (LEfSe) (version 1.0.7), with the linear discriminant analysis (LDA) score >2 as a threshold for significantly different species. The bacterial abundance among different pathways were compared by Kruskal-Wallis test. Graphpad Prism (version 5.0) was used to plot the graphs and compare the differences of immune cell proportions and bacterial abundances between groups using Two-way ANOVA tests. P value <0.05 was considered statistically significant. * represents P < 0.05, ** represents P < 0.01, and *** represents P < 0.001.
The Simpson’s diversity index corresponding to each pathway was calculated. Pathways were excluded if they had non-zero abundance in DNA in <95% of the samples, or if >25% of the pathway was attributed to unclassified organisms (HUMAnN2) in >25% of the samples (30). The correlations between bacteria species and immune cell subtypes were tested using SPARCC with spearman method (5 inference iterations and 100 bootstrap). Absolute correlation value of ≥0.3 was considered as significant correlation.
3 Results
3.1 Microbial community composition in DLBCL patients and its correlation with clinical features
To investigate the gut microbiome characteristics of DLBCL, we performed metagenomic sequencing in 69 newly diagnosed DLBCL patients. A total of 462.48 Gb of high-quality PE reads were acquired with an average of 6.70 Gb per sample. In total, 10 bacterial phyla, 31 orders and 455 bacteria species were identified. The main bacteria species included Bacteroides vulgatus, Prevotella copri, Eubacterium sp.CAG 180, Bacteroides stercoris, Bacteroides uniformis, Faecalibacterium prausnitzii, Parabacteroides distasonis, Alistipes putredinis, Bacteroides thetaiotaomicron, Bacteroides plebeius, Ruminococcus gnavus, Escherichia coli, Akkermansia muciniphila, Bacteroides fragilis, Bacteroides caccae, Bacteroides dorei, Prevotella sp.CAG 520, Barnesiella intestinihominis, Bacteroides ovatus and Parabacteroides merdae (Figure 1A). The phylogenetic tree clearly demonstrated that there were 5 phyla of intestinal bacteria in 69 DLBCL patients, including Bacteroidetes, Firmicutes, Proteobacteria, Actinobacteria and Fusobacteria. Firmicutes, which showed a continuous evolutionary relationship in the biological classification (Figure 1B).
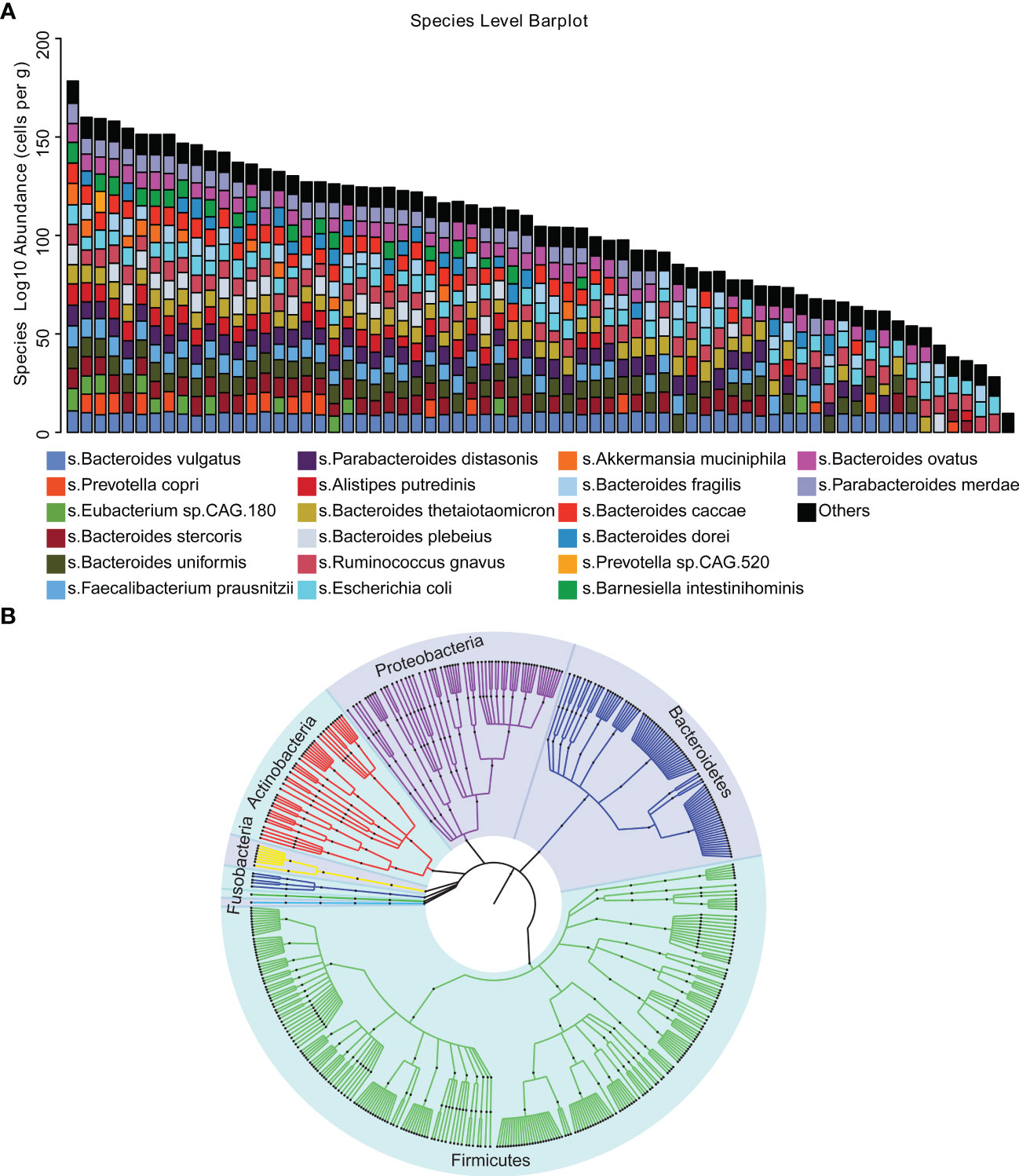
Figure 1 Microbial diversity mapping (A) Analysis of the gut microbiota in stool samples from 69 patients using absolute quantification. (B) Phylogenetic tree demonstrating that the five phylum bacteria of the gut microbiota exhibit continuous evolutionary relationships at six levels, including Bacteroidetes, Firmicutes, Proteobacteria, Actinobacteria, Fusobacteria and Firmicutes.
In addition, we assessed the association between intestinal bacteria constitution and the clinical features of patients with DLBCL, including NCCN-IPI, Ann Arbor stage, age, gender, LDH, extranodal involvement and ECOG performance status. The parallel heatmap demonstrated that Roseburia intestinalis and Phascolarctobacterium faecium were significantly negatively correlated with Ann Arbor stage, while Streptococcus parasanguinis, Streptococcus salivarius, Veillonella parvula and Bifidobacterium dentium were significantly positively correlated with Ann Arbor stage. Notably, Streptococcus parasanguinis, Streptococcus salivarius, Bacteroides fragilis and Bifidobacterium dentium were significantly positively correlated with NCCN-IPI (Figure 2). As NCCN-IPI is a prognostic index of DLBCL, we conjectured that the intestinal bacteria may be linked to the prognosis of DLBCL. In addition, there was no significant difference of clinical factors, such as gender, age, extranodal involvement and LDH, between different NCCN-IPI groups.
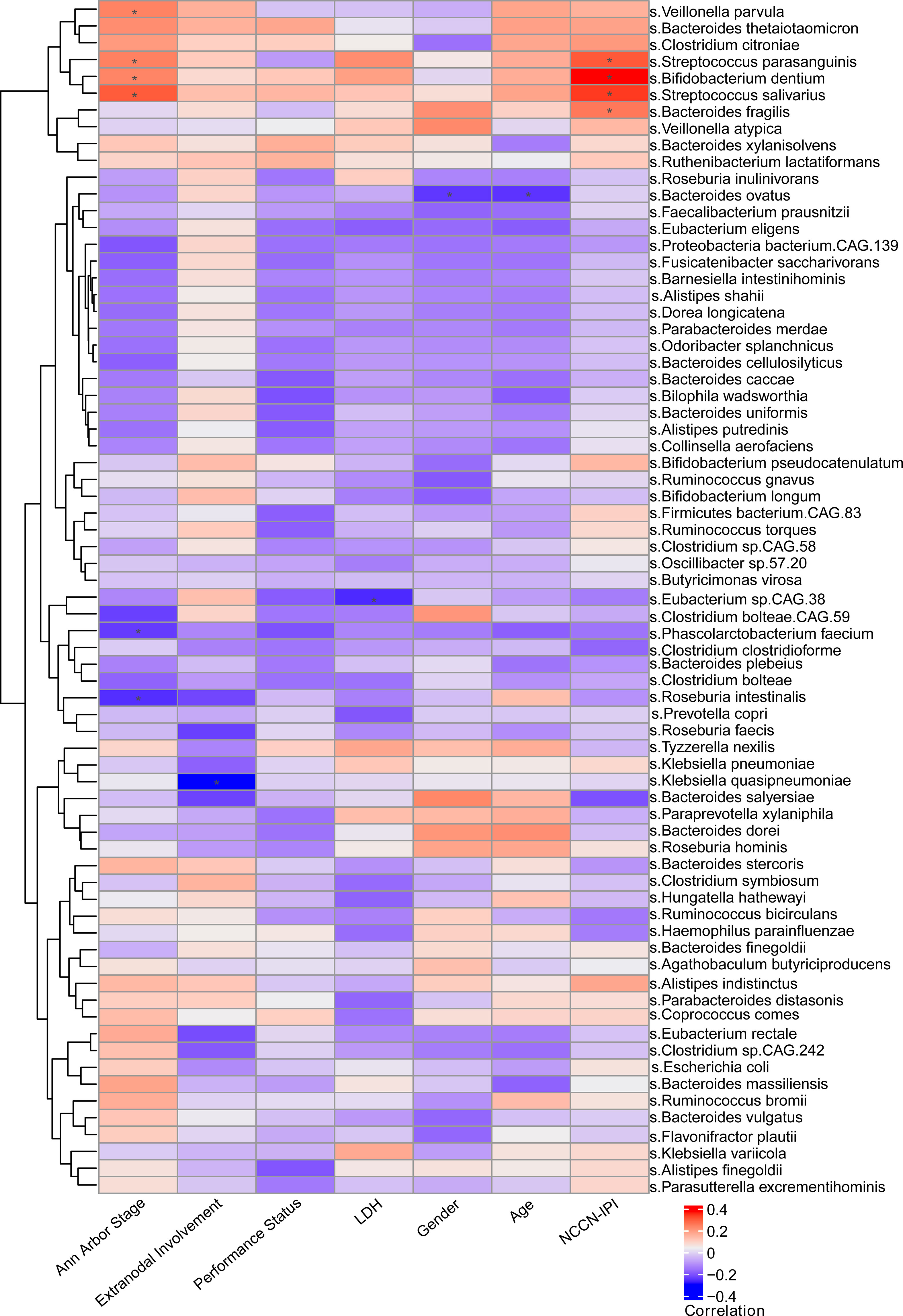
Figure 2 Relationship between clinical characteristics and gut microbiota Through heat map observation, it was found that there was a significant positive correlation between the clinical characteristics (Ann Arbor stage, NCCN-IPI) and gut microbiota in newly diagnosed DLBCL patients.
3.2 Diversity of intestinal bacteria in DLBCL patients with different NCCN-IPIs
To explore the association between intestinal bacteria and the prognosis of DLBCL, we further analyzed the association between intestinal bacteria and NCCN-IPI in patients with newly diagnosed DLBCL. First, we compared the diversity of intestinal bacteria in patients from the L, LM, MH and H groups using the alpha diversity (α-diversity) index. The MH group showed lower biodiversity according to the Chao1 and Shannon indices, but no significant difference was found between the four groups (Figures 3A, B). Also, the PCA and PCoA (β-diversity) were used to further analyze the differences in species diversity among different NCCN-IPI groups. The PCA results revealed no significant separation in bacterial community composition among the four groups using the first two principal component scores of PC1 and PC2, 12.34% and 5.75% of explained variance, respectively (Figure 3C). The PCoA results showed that the four groups of samples were close (Figure 3D). These results revealed a similar constitution of intestinal bacteria among different NCCN-IPI groups in DLBCL patients.
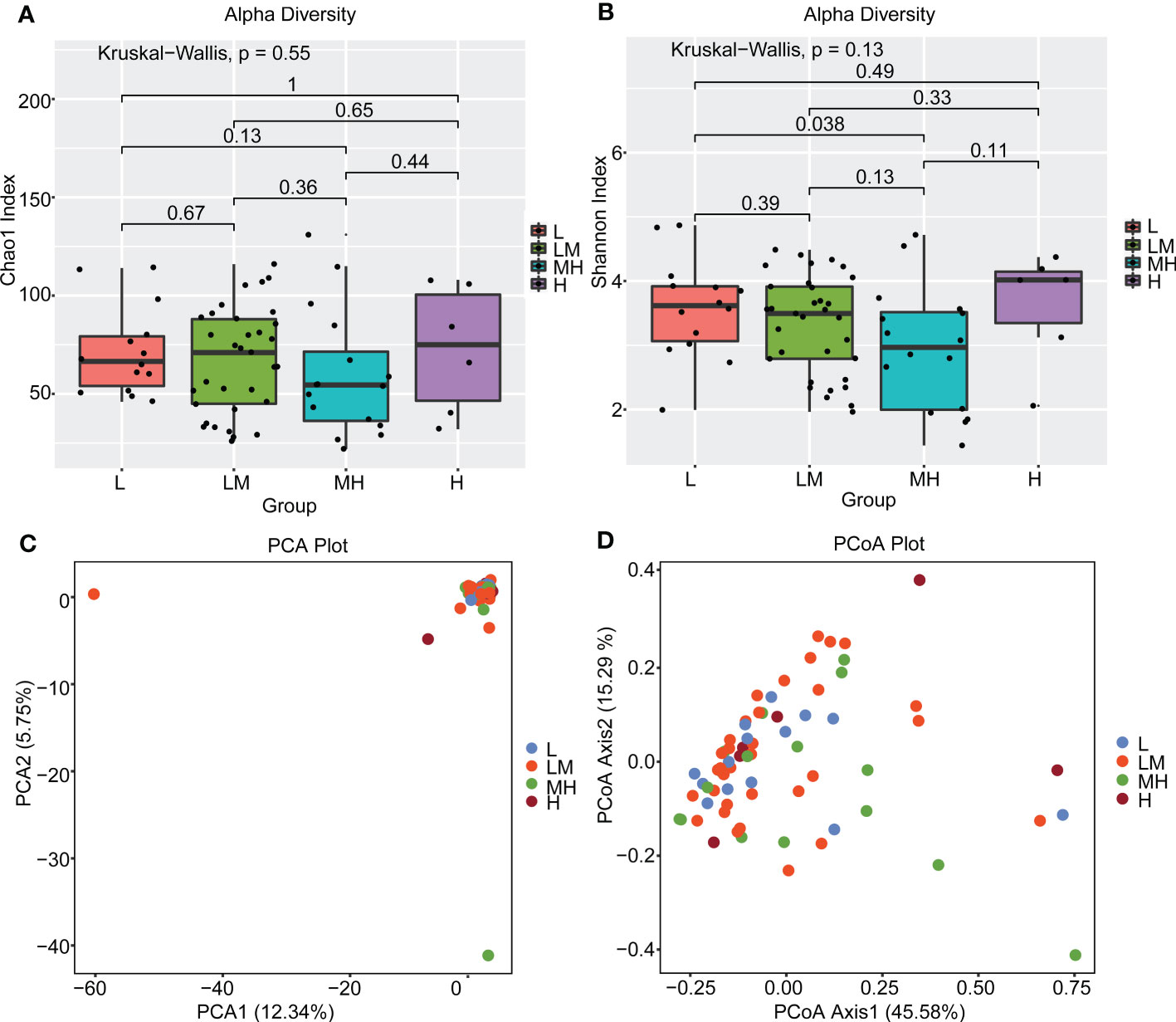
Figure 3 Diversity analysis of gut microbes between different NCCN-IPI groups The gut microbiota of the four groups of patients was analyzed using α diversity analysis based on (A) Chao1 and (B) Shannon index, respectively. β diversity among the four groups of patients was analyzed using (C) PCA and (D) PCoA.
3.3 Different bacterial species identified in DLBCL patients with different NCCN-IPIs
Then, we screened the differently accumulated bacterial species among DLBCL patients with different NCCN-IPIs. The heatmap showed bacteria including Bacteroides_plebeius, Prevotella_copri, and Roseburia_inulinivorans, were mainly enriched in the L and LM groups (Figure 4).
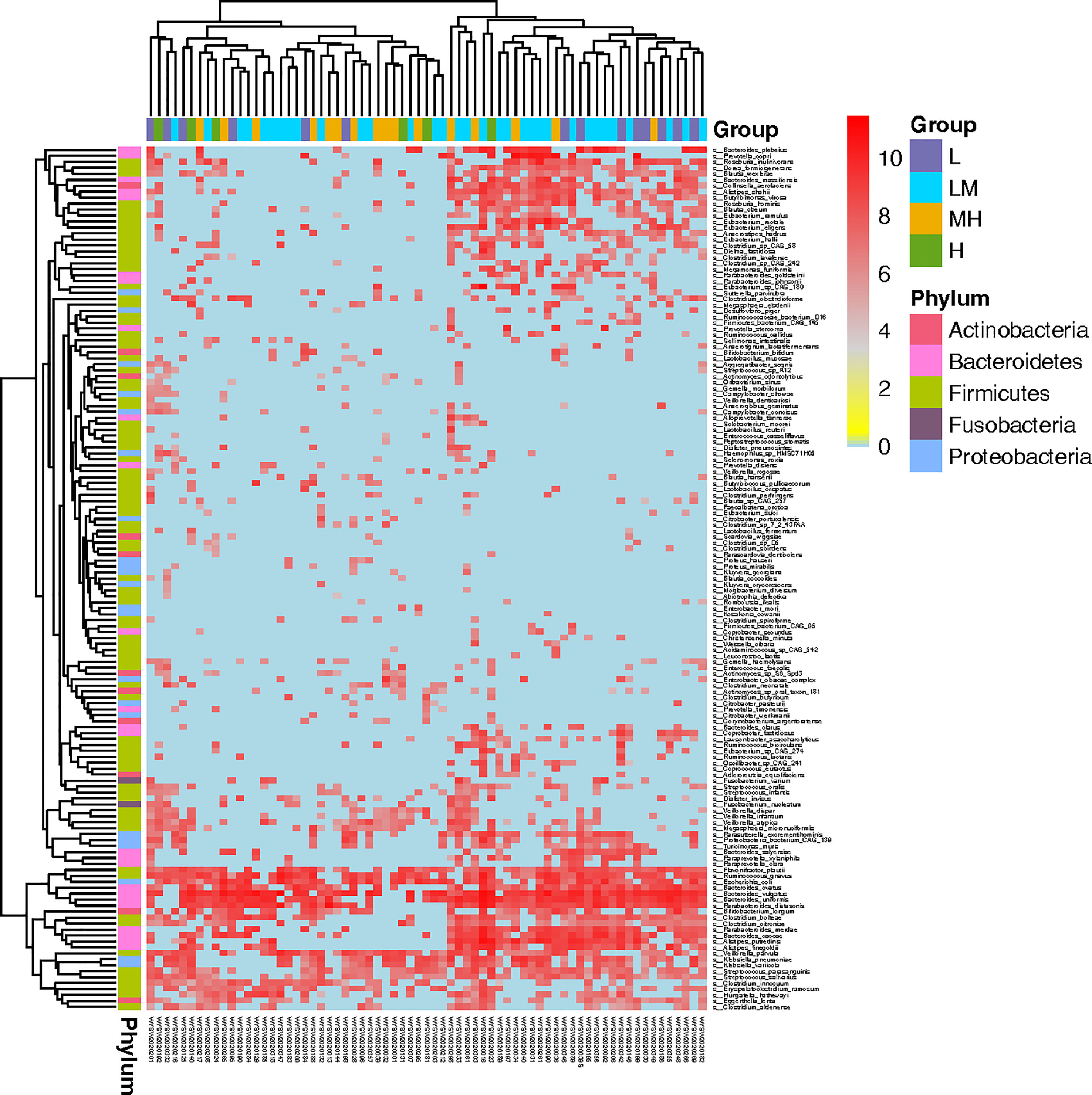
Figure 4 Screening of microbiota associated in DLBCL patients with different NCCN-IPIs The heatmap showed the Top145 differently accumulated bacterial species among DLBCL patients with different NCCN-IPIs.
In addition, we established a random-forest machine-learning model to further search the different bacterial species among different NCCN-IPI groups. Top30 bacterial species were demonstrated, such as Blautia sp.CAG 257, Streptococcus salivarius, Blautia_wexlers, Veillonella_parvulas and Parabacteroides_distasonis (Figure 5A). LEfSe analysis showed that 9 bacterial species with various abundances were found among the different NCCN-IPI groups. In detail, Bacteroides salyersiae, Eggerthella lenta and Blautia sp.CAG 257 were significantly enriched in the L group, while Clostridium butyricum, Veillonella rogosae, Actinomyces sp.S6 Spd3, Streptococcus parasanguinis, Enterococcus faecalls and Streptococcus salivarius exhibited higher abundances in the H group (Figure 5B). Through integration the results of random-forest and LEfSe, 6 species of bacteria were identified, including Blautia sp.CAG 257, Actinomyces sp.S6 Spd3, Streptococcus parasanguinis, Bacteroides salyersiae, Enterococcus faecalls and Streptococcus salivarius (Figure 5C). Specifically, Streptococcus parasanguinis and Streptococcus salivarius were significantly accumulated in the H group (Figure 5C), while Bacteroides salyersiae was not detected in the H group (Figure 5C). Taken together, these results suggested that Streptococcus parasanguis and Streptococcus salivarius were accumulated in DLBCL patients with higher NCCN-IPI.
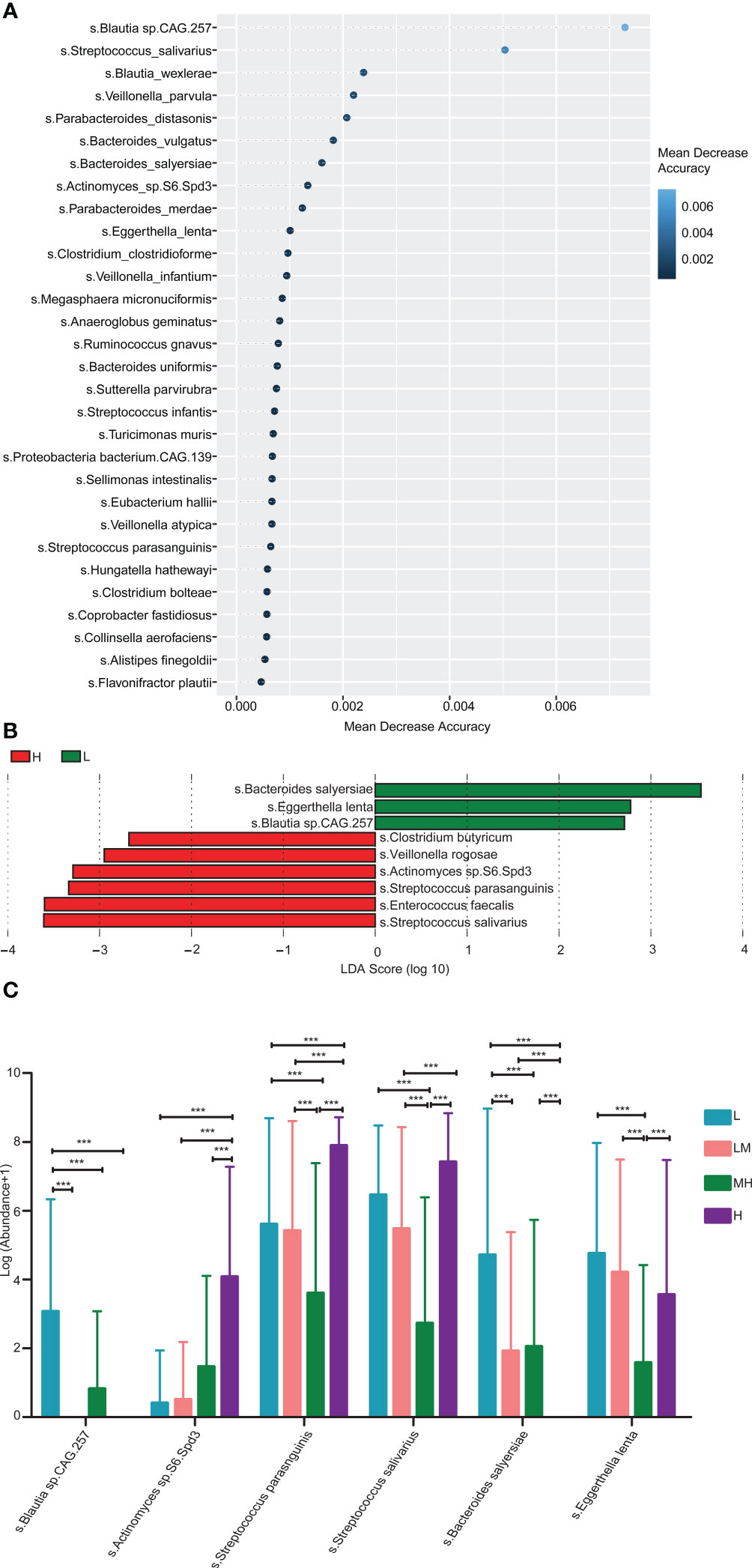
Figure 5 Enrichment pathway analysis of gut microbiota identified in 69 newly diagnosed DLBCL patients (A) The top 30 differential bacterial were identified by applying RandomForest regression of their relative abundances in DLBCL against NCCN-IPI. (B) LEfSe analysis was used to analyze the differences in microbiota between groups. (C) Six differential bacterial groups were screened according to both RandomForest and LEfSe analysis results.
3.4 Enriched pathways of bacterial species identified in DLBCL patients
The Simpson’s diversity index was applied to analyze the enriched pathways of the 455 bacteria species discovered in the 69 newly diagnosed DLBCL patients. The results showed that the 455 bacteria species were mainly enriched in the Pyridoxal 5’-phosphate biosynthesis I pathway with a Simpson diversity index close to 1, indicating that bacteria species in this pathway had the greatest diversity (Figure 6A). Moreover, Bacteroides uniformis, Bacteroides stercoris, Escherichia coli, Klebsiella pneumoniae and Parabacteroides distasonis were involved in Pyridoxal 5’-phosphate biosynthesis I pathway in all of the four groups (Figure 6B). These findings suggested that bacteria species discovered in the newly diagnosed DLBCL patients were mainly enriched in the Pyridoxal 5’-phosphate biosynthesis I pathway.
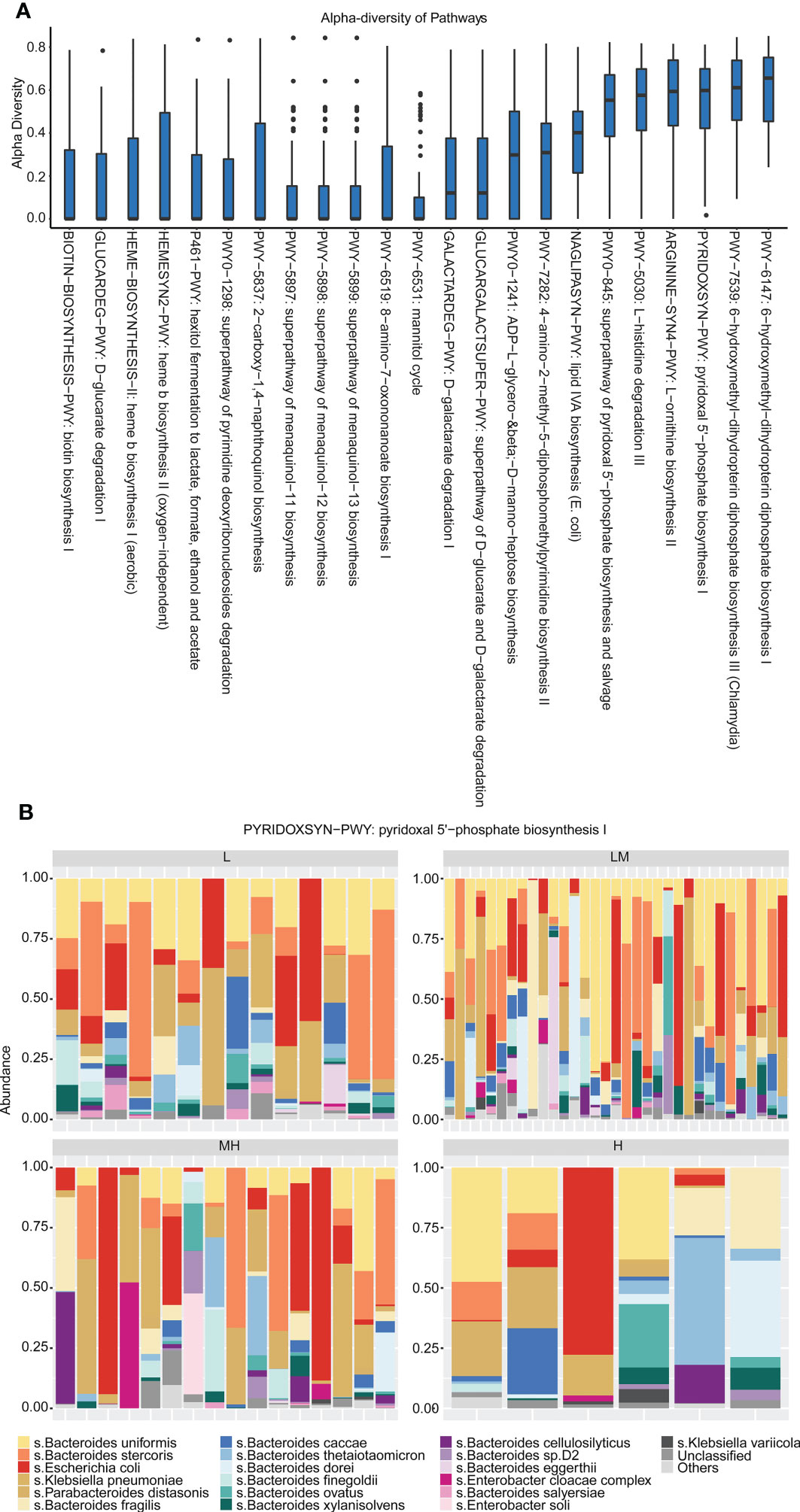
Figure 6 Enrichment pathways of differential gut microbiota (A) The relative abundance of microorganisms in each pathway was analyzed using Simpson’s diversity index. (B) The distribution of gut microbiota in each group in the Pyridoxal 5’-phosphate biosynthesis I pathway.
3.5 Peripheral immune cell subtypes associate with NCCN-IPI in DLBCL
In addition, we explored the relationship between peripheral immune cell subtypes and NCCN-IPI in DLBCL patients. B lymphocytes, T lymphocytes and NK lymphocytes in the L, LM, MH and H groups were identified and quantified using Full-spectral flow cytometry. The screening process of T, B and NK lymphocytes was shown in Figure S1. The markers of immune cells were summarized in Figure S2. Among the B lymphocytes, H group showed a higher population of naïve B cells while a lower population of pre-naïve B cells as compared with other groups (Figure 7A). The populations of CD69+ naïve B cells, memory B cells and regulatory B cells were increased in H group (Figure 7B). A declined trend of HLA-DR+ B cells, transfer B cells and plasmablast B cells was observed in the H group (Figure 7C), as well as the CD86+ plasmablast B cells, naïve B cells, pre-naïve B cells and regulatory B cells (Figure 7D). Compared with LM group, L group showed a higher population of CD83+ B cells and naïve B cells (Figure 7E). In addition, an increased trend of CD80+ B cells, transfer B cells and plasmablast B cells was observed in the H group (Figure 7F), and the populations of CD25+ or CD38+ B cells were increased in MH group (Figures 7G, H).
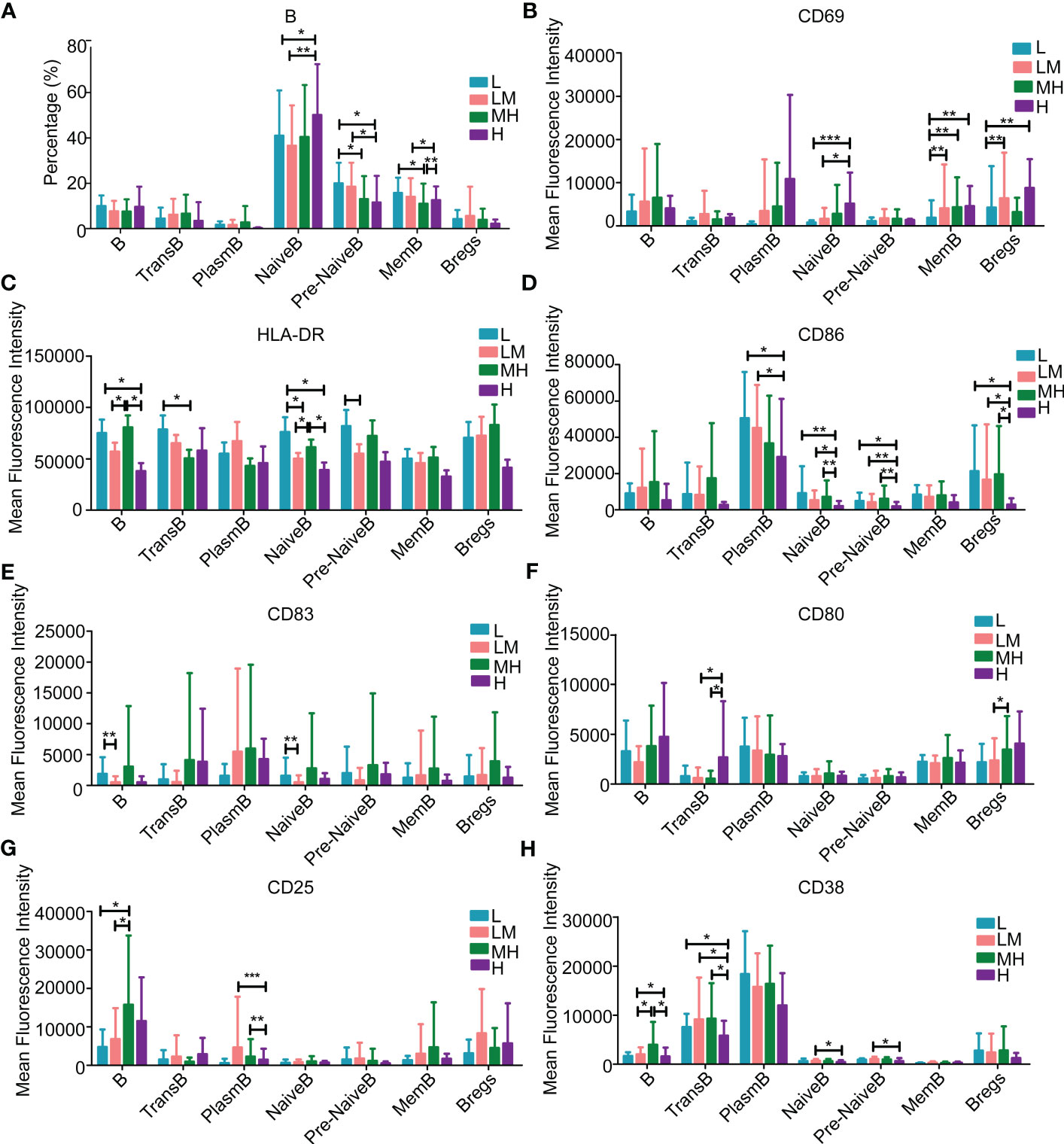
Figure 7 Populations of B lymphocytes in the peripheral blood samples of patients with different NCCN-IPIs The content of B lymphocytes (A) in each period. The mean fluorescence intensity of (B) CD69, (C) HLA-DR, (D) CD86, (E) CD83, (F) CD80, (G) CD25, (H) CD38 in each period of B lymphocytes. TransB: Transfer B cell; PlasmB: Plasmablast B cell; MemB: Memory B cell; Bregs: Regulatory B cell.
Among the T lymphocytes, the H group showed higher populations of stem cell-like memory (SCM) CD4+T cells and T-helper 17 (TH17) cells, while lower populations of T-helper 2 (TH2) cells and regulatory T (Treg) cells as compared with other groups (Figure 8A). The populations of SCM CD8+ T cells and nonrescue exhausted T (NREXT) cells were increased in H group (Figure 8B). Compared with other groups, HM group showed a higher population of all kinds of PD-1+ or PD-1+CD8+ T cells, except for CD4/CD8 double-positive (DP) T cells (Figures 8C, D). L group showed a higher population of TIM3+CD4+ T cells, TH2 cells and Treg cells while a lower population of TIM3+ central memory (CM) CD8+ T cells, NREXT cells and T cell receptor δγ+ (TCRδγ) cells as compared with other groups (Figure 8E). An increased trend of CD38+CD8+ T cells, NREXT cells and rescue exhausted T (REXT) cells were observed in H group (Figure 8F), as well as CD69+ T-helper 1 cells (Figure 8G). Compared with other group, HM group showed a higher population of CD152+CD8+ T cells, effector memory (EM) CD8+ T cells and CD4/CD8 DPT cells (Figure 8H).
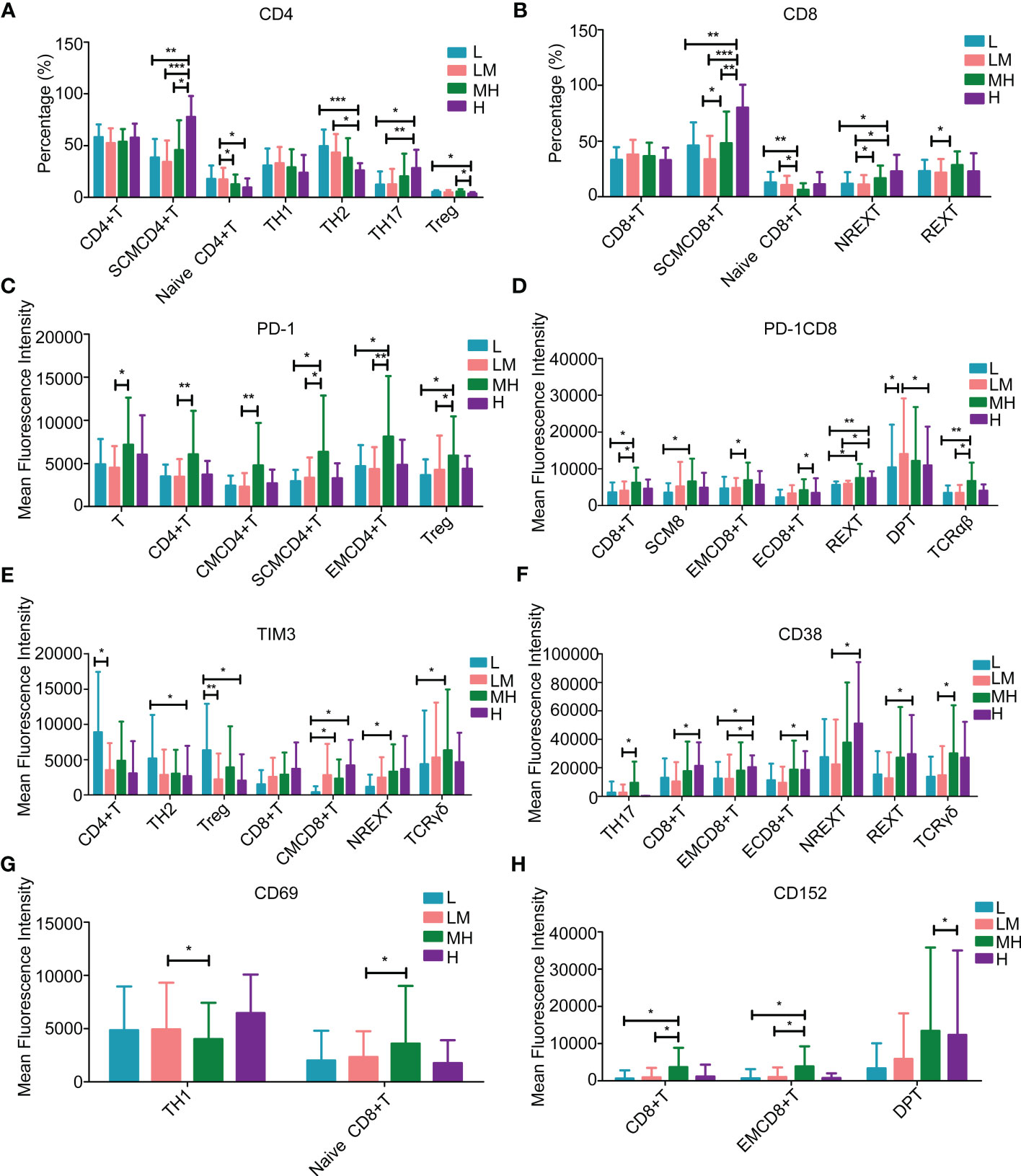
Figure 8 Populations of T lymphocytes in peripheral blood samples of patients with different NCCN-IPIs The content of (A) CD4 and (B) CD8 in T lymphocytes at each period. The mean fluorescence intensity of (C) PD-1, (D) PD-1CD8, (E) TIM3, (F) CD38, (G) CD69, (H) CD152 in each period of T lymphocytes. SCMCD4+ T: Stem cell-like memory CD4+T cell; SCMCD8+T: Stem cell-like memory CD8+ T cell; Treg: Regulatory T cell; REXT: Rescue exhausted T cells; NREXT: Unrescue exhausted T cells; EMCD4+ T: Effector memory CD4+T cells; EMCD8+ T: Effector memory CD8+ T cells; CMCD4+T: Central memory CD4+ T cell; CMCD8+ T: Central memory CD8+ T cell; DPT: CD4/CD8 double-positive T.
Among the NK lymphocytes, MH group showed a higher population of NK2 cells while a lower population of NK1 cells as compared with other groups (Figure 9A). The populations of CD69+ NKT cells were increased in MH group (Figure 9B). Compared with the LM group, the L group showed a lower population of CD159a+ NK cells (Figure 9C) and an increased trend of CD159c+ NK/NK1/NK2/NK3/CD94+CD159+ NKT cells (Figure 9D). These results revealed various immune microenvironments between newly diagnosed DLBCL patients with different NCCN-IPIs.
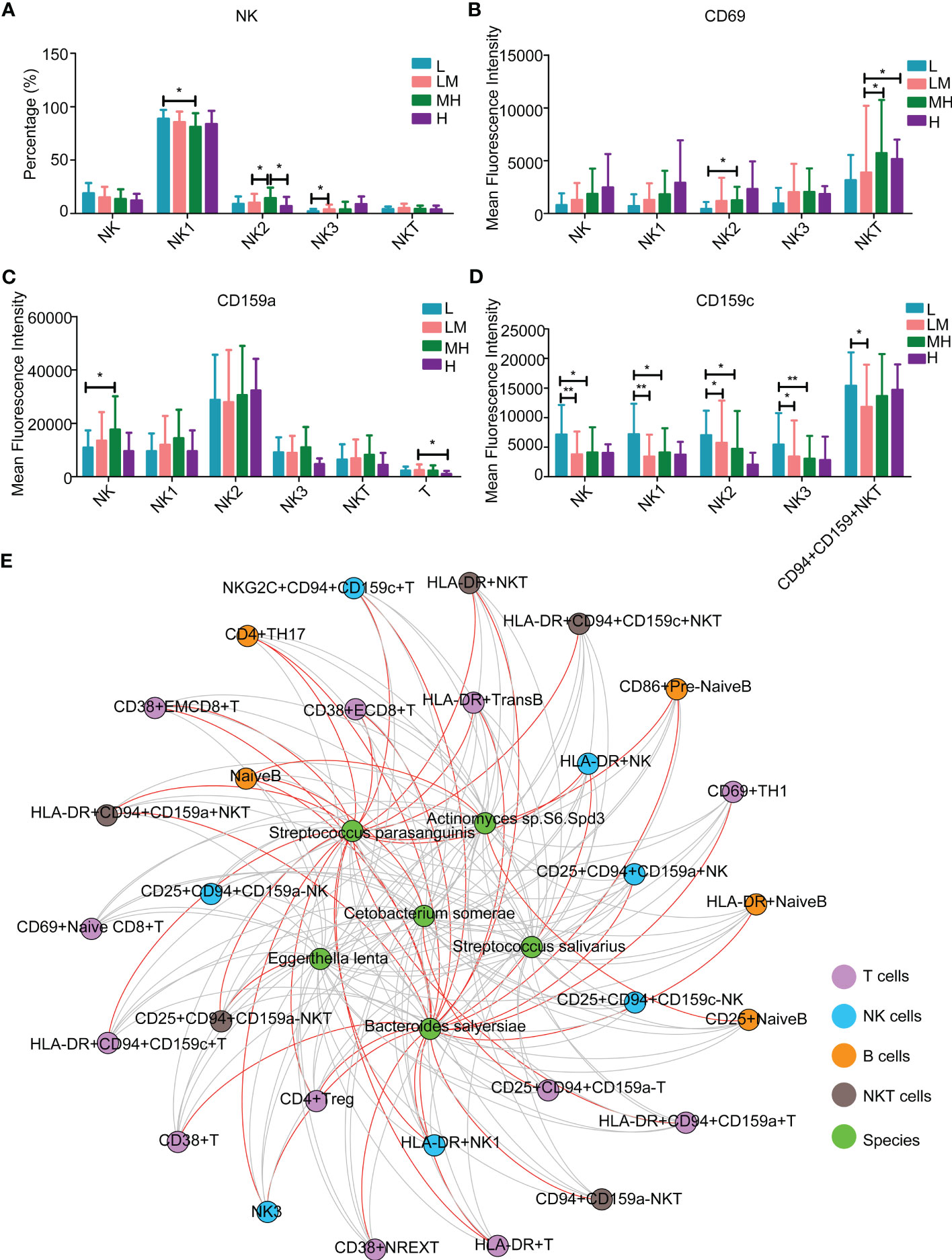
Figure 9 The relationship between peripheral immune cells and microbiotaThe content of NK lymphocytes (A) in each period. The mean fluorescence intensity of (B) CD69, (C) CD159a, (D) CD159c in each period of NK lymphocytes. (E) Interaction network diagram between 6 differential flora and differential immune markers.
3.6 Bacterial species interact with peripheral immune cell subtypes
Moreover, we investigated the association between the above mentioned 6 different bacteria species and 32 detected blood immune cell subpopulations. Streptococcus parasanguinis and Bacteroides salyersiae showed a stronger correlation with immune cells compared to other four bacteria species (Figure 9E and Table 2). In detail, Bacteroides salyersiae was mainly negatively associated with NK lymphocytes and T lymphocytes, including CD4+ Treg, CD38+ NREXT, NK3 and CD38+CD8+ EMT cells. Streptococcus parasanguinis was mainly negatively associated with NK lymphocytes and T lymphocytes, including HLA-DR+CD94+CD159c+ NKT, HLA-DR+ NK, HLA-DR+ NK1 and CD4+ Treg cells (Table 2). These results revealed a close association between intestinal bacteria and peripheral immune cells in DLBCL patients.
4 Discussion
In this study, we first described the pyridoxal of DLBCL and explored its association with immune microenvironment and NCCN-IPI, which was widely used for the prognosis assessment of patients with DLBCL (17). A total of 10 bacterial phyla, 31 orders and 455 species were identified in 69 patients with newly diagnosed DLBCL.
The diversity of bacteria showed no obvious difference between DLBCL patients with different NCCN-IPIs, which may be related to the perturbed gut microbe prior to treatment (31). Noticeably, we found the abundance of Streptococcus parasanguinis and Streptococcus salivarius were significantly increased in patients of the H group through integrating the association, Lefse and RandomForest results. Streptococcus parasanguinis is a dominant oral commensal and opportunistic pathogen for subacute endocarditis (32), and links to the prognosis of cancers. For instance, a significantly longer overall survival (OS) was observed in cutaneous melanoma patients carrying Streptococcus parasanguinis as compared to the non-carriers (33). Streptococcus salivarius consists of two clusters of strains with genomic and functional specificities (34). In 2018, Gavazza et al. (35) analyzed the gut microbiome of dogs with multicenter lymphoma and found that the abundance of Streptococcus was significantly higher in dogs with lymphoma as compared to that in the healthy dogs. In addition, Streptococcus pneumoniae predisposes to sepsis in patients with leukemia or lymphoma (36). Our study demonstrated for the first time that the abundance of Streptococcus salivarius was significantly elevated in patients with high NCCN-IPI, which may be a new direction for finding prognostic biomarkers for DLBCL patients.
Furthermore, we found that the bacteria species detected in the newly diagnosed DLBCL patients were enriched in the Pyridoxal 5’-phosphate biosynthesis I pathway, which had the highest diversity. Pyridoxal 5’-phosphate is the main component of vitamin B6, which is involved in the one-carbon metabolism of DLBCL, and thereafter affects the prognosis of DLBCL patients (37). Pyridoxal phosphates are produced by PDXK kinase from vitamin B6, and have been identified as an acute myeloid leukemia-selective dependency (38). Through inhibiting PDXK, the vitamin B6 pathway can be attenuated, thereby hindering the reproduction of leukemia cells (38). Thus, we hypothesize that intestinal microbiota may affect the progression of DLBCL through Pyridoxal 5’-phosphate biosynthesis I pathway, or more boldly vitamin B6, which needs to be verified in future studies.
In addition, our results revealed that the proportions of some kinds of immune cells were statistically different between different NCCN-IPI groups. For example, the populations of CD86+ plasmablasts cells, naïve B cells and pre-naïve B cells were decreased in DLBCL patients with higher NCCN-IPI. It has been reported that the loss expression of CD86, which was implicated in controlling tumor growth, was associated with decreased tumor-infiltrating T lymphocytes (T-TILs) in DLBCL patients (39). Besides, Xu et al. (40) found that DLBCL patients with a higher percentage of TIL-T always had a better survival. Taken together, these reports suggest that the high expression of CD86 predict a good outcome of DLBCL patients (39, 40). Consistently, our results showed that CD86+ B cells population was decreased in DLBCL patients with higher NCCN-IPI, suggesting that higher CD86+ might be a good prognosis marker for DLBCL. In addition, Dehghani et al. (41) demonstrated that higher peripheral IFN-γ/IL-4 T-helper 2 lymphocytes were associated with a favorable prognosis in DLBCL, which is consistent with our results that the proportion of T-helper 2 cells was higher in DLBCL patients with low NCCN-IPI. Moreover, we found that the proportion of CD8+ T cells was significantly increased in H group when compared with low NCCN-IPI, which was also reported in a previous report (42). In terms of TH17, it has been reported that TH17 cell number was increase DLBCL patients with high risk (43, 44). Similarly, our results showed that the percentage of TH17 cells was positively correlated with clinical stage, which suggested that increased TH17 cells may be associated with poor prognosis in DLBCL patients. These findings suggest that the immune cells were associated with the NCCN-IPI/prognosis of DLBCL patients, and may act as potential markers to evaluate the prognosis of DLBCL patients. In fact, many researchers have built prognostic evaluation models for DLBCL based on immune cells (42, 45, 46). Our results may provide further supporting clues for future relevant researches.
It has been proved that the gut microbiota is closely implicated in innate and adaptive immunity, and the clinical response to immunotherapy (47, 48). However, the association between gut microbe and immune cell subtypes in DLBCL patients has not been studied. Streptococcus parasanguinis was reported to be associated with the prognosis and immune checkpoint inhibitor therapy in cutaneous melanoma patients (33). Bacteroides salyersiae was involved in the regulation of immunotherapy resistance in renal cell carcinoma (49). However, their roles in regulating the curative effect of immunotherapy in DLBCL remain unclear. HLA-DR, a major histocompatibility complex class II, is mainly expressed in B lymphocytes, monocytes, macrophages, activated T lymphocytes, activated NK lymphocytes and progenitor cells (50). Low HLA-DR expression was associated with the poor prognosis of DLBCL patients receiving intensified upfront therapy (51). Higashi et al. (50) discovered that loss of HLA-DR expression in DLBCL led to a reduction of helper T-cells in the tumor microenvironment and was related to adverse outcome in DLBCL patients. Furthermore, it has been demonstrated that HLA-DR-positive DLBCL patients had longer OS when compared with HLA-DR-negative patients (52). Consistently, our results showed that HLA-DR+ cells showed a negative correlation with Streptococcus parasanguinis, which was more abundant in H group and was considered as a marker of poor prognosis in DLBCL, indicating HLA-DR is linked to good prognosis of DLBCL. The association between Tregs and DLBCL prognosis is controversial, some researchers found that the low number of Tregs was correlated with poor prognosis (53–55), while other researchers hold the opposite view or found no close relationship (56–58). Carreras J. et al. (59) found that low infiltration of FOXP3+ Tregs were associated with a poor prognosis in DLBCL. Similarly, in our study, we also discovered that the proportion of Tregs was decreased in the H group as compared with the L and MH group, suggesting that the higher Treg proportion may be associated with a better prognosis of DLBCL. In addition, our results showed that Bacteroides salyersiae was accumulated in the L group and Streptococcus parasanguinis was abundant in the H group. However, both of them were negatively correlated with the number of Tregs, suggesting a confusing role of Tregs in DLBCL patients.
5 Conclusion
This study reveals that Streptococcus parasanguinis and Streptococcus salivarius are enriched DLBCL patients with high NCCN-IPI score, and also show negative correlations with the proportions of peripheral immune cells (Tregs, CD38+ NREXT, NK3, HLA-DR+ NK, HLA-DR+ NKT, HLA-DR+CD94+CD159c+ NKT and CD38+EMCD8+ T cells). Our results indicate that the aberrant accumulation of Streptococcus parasanguinis and Bacteroides salyersiae may be the trigger of DLBCL and lead to peripheral immune system dysregulation. Collectively, this study first describes the gut microbiota landscape of DLBCL, and highlights the association between gut microbiota and NCCN-IPI, as well as the immune microenvironment, which may provide a new idea for the prognosis assessment and treatment of DLBCL.
Data availability statement
The datasets generated for this study can be found in the National Genomics Data Center: https://ngdc.cncb.ac.cn/search/?dbId=&q=CRA009581.
Ethics statement
The studies involving human participants were reviewed and approved by the Ethics Committee of the First Affiliated Hospital of Zhejiang Chinese Medical University. The patients/participants provided their written informed consent to participate in this study.
Author contributions
Design of the work: JS, WQ, HY, YZ. Acquisition and analysis: YZ, SH, XX, LZ. Interpretation of data: YC, ZZ, XG, SZ, KY, LH, JF, YH, JJ. Drafted the manuscript: YZ. All authors contributed to the article and approved the submitted version.
Funding
This study was supported by the Special Project for the Modernization of Traditional Chinese Medicine in Zhejiang Province (No.2020ZX007), Zhejiang Provincial Natural Science Foundation (No. LTGY23H270004, LTGY23H290001), Zhejiang Traditional Medicine and Technology Program for Young Scholar (No.2021ZQ030, 2022ZQ034) and the National TCM Clinical Research Base Construction Project (No.2015H0105).
Acknowledgments
We thank Shanghai Rightongene Biotechnology Co., Ltd. (Shanghai, China) for performance and analysis of the full-spectral flow cytometry.
Conflict of interest
The authors declare that the research was conducted in the absence of any commercial or financial relationships that could be construed as a potential conflict of interest.
Publisher’s note
All claims expressed in this article are solely those of the authors and do not necessarily represent those of their affiliated organizations, or those of the publisher, the editors and the reviewers. Any product that may be evaluated in this article, or claim that may be made by its manufacturer, is not guaranteed or endorsed by the publisher.
Supplementary material
The Supplementary Material for this article can be found online at: https://www.frontiersin.org/articles/10.3389/fimmu.2023.1146861/full#supplementary-material
References
1. Ennishi D, Hsi ED, Steidl C, Scott DW. Toward a new molecular taxonomy of diffuse Large b-cell lymphoma. Cancer Discov (2020) 10(9):1267–81. doi: 10.1158/2159-8290.CD-20-0174
2. Arber DA, Orazi A, Hasserjian R, Thiele J, Borowitz MJ, Le Beau MM, et al. The 2016 revision to the world health organization classification of myeloid neoplasms and acute leukemia. Blood (2016) 127(20):2391–405. doi: 10.1182/blood-2016-03-643544
3. Roschewski M, Staudt LM, Wilson WH. Diffuse large b-cell lymphoma-treatment approaches in the molecular era. Nat Rev Clin Oncol (2014) 11(1):12–23. doi: 10.1038/nrclinonc.2013.197
4. Tong Y, Gao H, Qi Q, Liu X, Li J, Gao J, et al. High fat diet, gut microbiome and gastrointestinal cancer. Theranostics (2021) 11(12):5889–910. doi: 10.7150/thno.56157
5. Bishehsari F, Voigt RM, Keshavarzian A. Circadian rhythms and the gut microbiota: from the metabolic syndrome to cancer. Nat Rev Endocrinol (2020) 16(12):731–9. doi: 10.1038/s41574-020-00427-4
6. Severyn CJ, Brewster R, Andermann TM. Microbiota modification in hematology: still at the bench or ready for the bedside? Blood Adv (2019) 3(21):3461–72. doi: 10.1182/bloodadvances.2019000365
7. Gao X, Miao R, Zhu Y, Lin C, Yang X, Jia R, et al. A new insight into acute lymphoblastic leukemia in children: influences of changed intestinal microfloras. BMC Pediatr (2020) 20(1):290. doi: 10.1186/s12887-020-02192-9
8. Cozen W, Yu G, Gail MH, Ridaura VK, Nathwani BN, Hwang AE, et al. Fecal microbiota diversity in survivors of adolescent/young adult Hodgkin lymphoma: a study of twins. Br J Cancer. (2013) 108(5):1163–7. doi: 10.1038/bjc.2013.60
9. Yuan L, Wang W, Zhang W, Zhang Y, Wei C, Li J, et al. Gut microbiota in untreated diffuse Large b cell lymphoma patients. Front Microbiol (2021) 12:646361. doi: 10.3389/fmicb.2021.646361
10. Mager LF, Burkhard R, Pett N, Cooke NCA, Brown K, Ramay H, et al. Microbiome-derived inosine modulates response to checkpoint inhibitor immunotherapy. Science (2020) 369(6510):1481–9. doi: 10.1126/science.abc3421
11. Gopalakrishnan V, Spencer CN, Nezi L, Reuben A, Andrews MC, Karpinets TV, et al. Gut microbiome modulates response to anti-PD-1 immunotherapy in melanoma patients. Science (2018) 359(6371):97–103. doi: 10.1126/science.aan4236
12. Vetizou M, Pitt JM, Daillere R, Lepage P, Waldschmitt N, Flament C, et al. Anticancer immunotherapy by CTLA-4 blockade relies on the gut microbiota. Science (2015) 350(6264):1079–84. doi: 10.1126/science.aad1329
13. Chang C, Lin CH, Cheng AL, Medeiros LJ, Chang KC. Primary central nervous system diffuse large b-cell lymphoma has poorer immune cell infiltration and prognosis than its peripheral counterpart. Histopathology (2015) 67(5):625–35. doi: 10.1111/his.12706
14. Shin HJ, Kim DY, Chung J, Shin KH, Lee H. Prognostic impact of peripheral blood T-cell subsets at the time of diagnosis on survival in patients with diffuse Large b-cell lymphoma. Acta Haematol (2021) 144(4):427–37. doi: 10.1159/000510912
15. Nitta H, Terui Y, Yokoyama M, Mishima Y, Nishimura N, Ueda K, et al. Absolute peripheral monocyte count at diagnosis predicts central nervous system relapse in diffuse large b-cell lymphoma. Haematologica (2015) 100(1):87–90. doi: 10.3324/haematol.2014.114934
16. Round JL, Mazmanian SK. The gut microbiota shapes intestinal immune responses during health and disease. Nat Rev Immunol (2009) 9(5):313–23. doi: 10.1038/nri2515
17. Ruppert AS, Dixon JG, Salles G, Wall A, Cunningham D, Poeschel V, et al. International prognostic indices in diffuse large b-cell lymphoma: a comparison of IPI, r-IPI, and NCCN-IPI. Blood (2020) 135(23):2041–8. doi: 10.1182/blood.2019002729
18. Campo E, Jaffe ES, Cook JR, Quintanilla-Martinez L, Swerdlow SH, Anderson KC, et al. The international consensus classification of mature lymphoid neoplasms: a report from the clinical advisory committee. Blood (2022) 140(11):1229–53. doi: 10.1182/blood.2022015851
19. Alaggio R, Amador C, Anagnostopoulos I, Attygalle AD, Araujo IBO, Berti E, et al. The 5th edition of the world health organization classification of haematolymphoid tumours: lymphoid neoplasms. Leukemia (2022) 36(7):1720–48. doi: 10.1038/s41375-022-01620-2
21. Bolger AM, Lohse M, Usadel B. Trimmomatic: a flexible trimmer for illumina sequence data. Bioinformatics (2014) 30(15):2114–20. doi: 10.1093/bioinformatics/btu170
22. Beghini F, McIver LJ, Blanco-Miguez A, Dubois L, Asnicar F, Maharjan S, et al. Integrating taxonomic, functional, and strain-level profiling of diverse microbial communities with bioBakery 3. Elife (2021) 10. doi: 10.7554/eLife.65088
23. Qin J, Li R, Raes J, Arumugam M, Burgdorf KS, Manichanh C, et al. A human gut microbial gene catalogue established by metagenomic sequencing. Nature (2010) 464(7285):59–65. doi: 10.1038/nature08821
24. Qin J, Li Y, Cai Z, Li S, Zhu J, Zhang F, et al. A metagenome-wide association study of gut microbiota in type 2 diabetes. Nature (2012) 490(7418):55–60. doi: 10.1038/nature11450
25. Asnicar F, Weingart G, Tickle TL, Huttenhower C, Segata N. Compact graphical representation of phylogenetic data and metadata with GraPhlAn. PeerJ (2015) 3:e1029. doi: 10.7717/peerj.1029
26. Gu Z, Eils R, Schlesner M. Complex heatmaps reveal patterns and correlations in multidimensional genomic data. Bioinformatics (2016) 32(18):2847–9. doi: 10.1093/bioinformatics/btw313
27. Caporaso JG, Kuczynski J, Stombaugh J, Bittinger K, Bushman FD, Costello EK, et al. QIIME allows analysis of high-throughput community sequencing data. Nat Methods (2010) 7(5):335–6. doi: 10.1038/nmeth.f.303
28. McDonald D, Vazquez-Baeza Y, Koslicki D, McClelland J, Reeve N, Xu Z, et al. Striped UniFrac: enabling microbiome analysis at unprecedented scale. Nat Methods (2018) 15(11):847–8. doi: 10.1038/s41592-018-0187-8
29. Jing G, Zhang Y, Yang M, Liu L, Xu J, Su X. Dynamic meta-storms enables comprehensive taxonomic and phylogenetic comparison of shotgun metagenomes at the species level. Bioinformatics (2020) 36(7):2308–10. doi: 10.1093/bioinformatics/btz910
30. Schirmer M, Franzosa EA, Lloyd-Price J, McIver LJ, Schwager R, Poon TW, et al. Dynamics of metatranscription in the inflammatory bowel disease gut microbiome. Nat Microbiol (2018) 3(3):337–46. doi: 10.1038/s41564-017-0089-z
31. Diefenbach CS, Peters BA, Li H, Raphael B, Moskovits T, Hymes K, et al. Microbial dysbiosis is associated with aggressive histology and adverse clinical outcome in b-cell non-Hodgkin lymphoma. Blood Adv (2021) 5(5):1194–8. doi: 10.1182/bloodadvances.2020003129
32. Chen YM, Tsai PH, Ye ZS, Huang YW, Shieh HR, Wu CH, et al. Functional analysis of the collagen binding proteins of streptococcus parasanguinis FW213. mSphere (2020) 5(5). doi: 10.1128/mSphere.00863-20
33. Wind TT, Gacesa R, Vich Vila A, de Haan JJ, Jalving M, Weersma RK, et al. Gut microbial species and metabolic pathways associated with response to treatment with immune checkpoint inhibitors in metastatic melanoma. Melanoma Res (2020) 30(3):235–46. doi: 10.1097/CMR.0000000000000656
34. Delorme C, Abraham AL, Renault P, Guedon E. Genomics of streptococcus salivarius, a major human commensal. Infect Genet Evol (2015) 33:381–92. doi: 10.1016/j.meegid.2014.10.001
35. Gavazza A, Rossi G, Lubas G, Cerquetella M, Minamoto Y, Suchodolski JS. Faecal microbiota in dogs with multicentric lymphoma. Vet Comp Oncol (2018) 16(1):E169–E75. doi: 10.1111/vco.12367
36. Allen JB, Weiner LB. Pneumococcal sepsis in childhood leukemia and lymphoma. Pediatrics (1981) 67(2):292–5. doi: 10.1542/peds.67.2.292
37. Cao Y, Chen P, Cai M, Shi Q, Xu P, Wang L, et al. Prognostic impact of b-vitamins involved in one-carbon metabolism in patients with diffuse large b-cell lymphoma. Hematol Oncol (2020) 38(4):456–66. doi: 10.1002/hon.2752
38. Chen CC, Li B, Millman SE, Chen C, Li X, JPt M, et al. Vitamin B6 addiction in acute myeloid leukemia. Cancer Cell (2020) 37(1):71–84 e7. doi: 10.1016/j.ccell.2019.12.002
39. Stopeck AT, Gessner A, Miller TP, Hersh EM, Johnson CS, Cui H, et al. Loss of B7.2 (CD86) and intracellular adhesion molecule 1 (CD54) expression is associated with decreased tumor-infiltrating T lymphocytes in diffuse b-cell large-cell lymphoma. Clin Cancer Res (2000) 6(10):3904–9.
40. Xu Y, Kroft SH, McKenna RW, Aquino DB. Prognostic significance of tumour-infiltrating T lymphocytes and T-cell subsets in de novo diffuse large b-cell lymphoma: a multiparameter flow cytometry study. Br J Haematol (2001) 112(4):945–9. doi: 10.1046/j.1365-2141.2001.02649.x
41. Dehghani M, Ramzi M, Kalani M, Golmoghaddam H, Arandi N. Higher peripheral blood IFN-gamma-/IL-4+ Th2 lymphocytes are associated with lower rate of relapse in patients with lymphoma. Immunol Invest (2022) 51(2):452–63. doi: 10.1080/08820139.2020.1840583
42. Gong Y, Chen R, Zhang X, Zou ZM, Chen XH. Role of immune microenvironmental factors for improving the IPI-related risk stratification of aggressive b cell lymphoma. BioMed Environ Sci (2017) 30(7):492–500. doi: 10.3967/bes2017.065
43. Cha Z, Guo H, Tu X, Zang Y, Gu H, Song H, et al. Alterations of circulating follicular helper T cells and interleukin 21 in diffuse large b-cell lymphoma. Tumour Biol (2014) 35(8):7541–6. doi: 10.1007/s13277-014-1999-5
44. Sun R, Zheng Z, Wang L, Cheng S, Shi Q, Qu B, et al. A novel prognostic model based on four circulating miRNA in diffuse large b-cell lymphoma: implications for the roles of MDSC and Th17 cells in lymphoma progression. Mol Oncol (2021) 15(1):246–61. doi: 10.1002/1878-0261.12834
45. Ma SY, Tian XP, Cai J, Su N, Fang Y, Zhang YC, et al. A prognostic immune risk score for diffuse large b-cell lymphoma. Br J Haematol (2021) 194(1):111–9. doi: 10.1111/bjh.17478
46. Yu H, Fu D, Xu PP, Cheng S, Wang L, Zhang YZ, et al. Implication of immune cell signature of tumor microenvironment in diffuse large b-cell lymphoma. Hematol Oncol (2021) 39(5):616–24. doi: 10.1002/hon.2905
47. Gopalakrishnan V, Helmink BA, Spencer CN, Reuben A, Wargo JA. The influence of the gut microbiome on cancer, immunity, and cancer immunotherapy. Cancer Cell (2018) 33(4):570–80. doi: 10.1016/j.ccell.2018.03.015
48. Matson V, Fessler J, Bao R, Chongsuwat T, Zha Y, Alegre ML, et al. The commensal microbiome is associated with anti-PD-1 efficacy in metastatic melanoma patients. Science (2018) 359(6371):104–8. doi: 10.1126/science.aao3290
49. Derosa L, Routy B, Fidelle M, Iebba V, Alla L, Pasolli E, et al. Gut bacteria composition drives primary resistance to cancer immunotherapy in renal cell carcinoma patients. Eur Urol (2020) 78(2):195–206. doi: 10.1016/j.eururo.2020.04.044
50. Higashi M, Tokuhira M, Fujino S, Yamashita T, Abe K, Arai E, et al. Loss of HLA-DR expression is related to tumor microenvironment and predicts adverse outcome in diffuse large b-cell lymphoma. Leuk Lymphoma. (2016) 57(1):161–6. doi: 10.3109/10428194.2015.1038708
51. Veelken H, Vik Dannheim S, Schulte Moenting J, Martens UM, Finke J, Schmitt-Graeff A. Immunophenotype as prognostic factor for diffuse large b-cell lymphoma in patients undergoing clinical risk-adapted therapy. Ann Oncol (2007) 18(5):931–9. doi: 10.1093/annonc/mdm012
52. Rimsza LM, Farinha P, Fuchs DA, Masoudi H, Connors JM, Gascoyne RD. HLA-DR protein status predicts survival in patients with diffuse large b-cell lymphoma treated on the MACOP-b chemotherapy regimen. Leuk Lymphoma (2007) 48(3):542–6. doi: 10.1080/10428190601078605
53. Cha Z, Gu H, Zang Y, Wang Z, Li J, Huang W, et al. The prevalence and function of CD4(+)CXCR5(+)Foxp3(+) follicular regulatory T cells in diffuse large b cell lymphoma. Int Immunopharmacol (2018) 61:132–9. doi: 10.1016/j.intimp.2018.05.025
54. Rusak M, Bolkun L, Chociej-Stypulkowska J, Pawlus J, Kloczko J, Dabrowska M. Flow-cytometry-based evaluation of peripheral blood lymphocytes in prognostication of newly diagnosed DLBCL patients. Blood Cells Mol Dis (2016) 59:92–6. doi: 10.1016/j.bcmd.2016.04.004
55. Glowala-Kosinska M, Chwieduk A, Nieckula J, Sadus-Wojciechowska M, Grosicki S, Rusin A, et al. Association of circulating regulatory T cell number with the incidence and prognosis of diffuse large b-cell lymphoma. Eur J Haematol (2013) 91(2):122–8. doi: 10.1111/ejh.12144
56. Nam SJ, Kim S, Kwon D, Kim H, Kim S, Lee E, et al. Prognostic implications of tumor-infiltrating macrophages, M2 macrophages, regulatory T-cells, and indoleamine 2,3-dioxygenase-positive cells in primary diffuse large b-cell lymphoma of the central nervous system. Oncoimmunology (2018) 7(7):e1442164. doi: 10.1080/2162402X.2018.1442164
57. Zhong W, Xu X, Zhu Z, Yang L, Du H, Xia Z, et al. Increased interleukin-17A levels promote rituximab resistance by suppressing p53 expression and predict an unfavorable prognosis in patients with diffuse large b cell lymphoma. Int J Oncol (2018) 52(5):1528–38. doi: 10.3892/ijo.2018.4299
58. Nakayama S, Yokote T, Akioka T, Hiraoka N, Nishiwaki U, Miyoshi T, et al. Infiltration of effector regulatory T cells predicts poor prognosis of diffuse large b-cell lymphoma, not otherwise specified. Blood Adv (2017) 1(8):486–93. doi: 10.1182/bloodadvances.2016000885
Keywords: diffuse large B-cell lymphoma, gut microbiota, National Comprehensive Center Network-International Prognostic Index, metagenomic sequencing, immunity
Citation: Zhang Y, Han S, Xiao X, Zheng L, Chen Y, Zhang Z, Gao X, Zhou S, Yu K, Huang L, Fu J, Hong Y, Jiang J, Qian W, Yang H and Shen J (2023) Integration analysis of tumor metagenome and peripheral immunity data of diffuse large-B cell lymphoma. Front. Immunol. 14:1146861. doi: 10.3389/fimmu.2023.1146861
Received: 18 January 2023; Accepted: 24 April 2023;
Published: 09 May 2023.
Edited by:
Laurel L. Lenz, University of Colorado Anschutz Medical Campus, United StatesReviewed by:
Guichuan Lai, Chongqing Medical University, ChinaHuilai Zhang, Tianjin Medical University, China
Joaquim Carreras, Tokai University, Japan
Copyright © 2023 Zhang, Han, Xiao, Zheng, Chen, Zhang, Gao, Zhou, Yu, Huang, Fu, Hong, Jiang, Qian, Yang and Shen. This is an open-access article distributed under the terms of the Creative Commons Attribution License (CC BY). The use, distribution or reproduction in other forums is permitted, provided the original author(s) and the copyright owner(s) are credited and that the original publication in this journal is cited, in accordance with accepted academic practice. No use, distribution or reproduction is permitted which does not comply with these terms.
*Correspondence: Jianping Shen, sjping88@163.com; Wenbin Qian, Qianwb@zju.edu.cn; Haiyan Yang, haiyanyang1125@163.com