- 1Fujian Provincial Key Laboratory of Brain Aging and Neurodegenerative Diseases, School of Basic Medical Sciences, Fujian Medical University, Fuzhou, Fujian, China
- 2The Chinese Academy of Sciences (CAS) Key Laboratory for Biomedical Effects of Nanomaterials and Nanosafety, The Chinese Academy of Sciences (CAS) Key Laboratory of Standardization and Measurement for Nanotechnology, The Chinese Academy of Sciences (CAS) Center for Excellence in Nanoscience, National Center for Nanoscience and Technology of China, Beijing, China
- 3The First Affiliated Hospital of Fujian Medical University, Fuzhou, Fujian, China
- 4Key Laboratory of Forensic Genetics, Institute of Forensic Sciences, Ministry of Public Security, Beijing, China
- 5Department of Gastroenterology and Hepatology, Beijing You’an Hospital, Capital Medical University, Beijing, China
Background: In the therapeutic process of COVID-19, the majority of indicators that physicians have for assisting treatment have come from clinical tests represented by proteins, metabolites, and immune levels in patients’ blood. Therefore, this study constructs an individualized treatment model based on deep learning methods, aiming to realize timely intervention based on clinical test indicator data of COVID-19 patients and provide an important theoretical basis for optimizing medical resource allocation.
Methods: This study collected clinical data from a total of 1,799 individuals, including 560 controls for non-respiratory infectious diseases (Negative), 681 controls for other respiratory virus infections (Other), and 558 coronavirus infections (Positive) for COVID-19. We first used the Student T-test to screen for statistically significant differences (Pvalue<0.05); we then used the Adaptive-Lasso method stepwise regression to screen the characteristic variables and filter the features with low importance; we then used analysis of covariance to calculate the correlation between variables and filter the highly correlated features; and finally, we analyzed the feature contribution and screened the best combination of features.
Results: Feature engineering reduced the feature set to 13 feature combinations. The correlation coefficient between the projected results of the artificial intelligence-based individualized diagnostic model and the fitted curve of the actual values in the test group was 0.9449 which could be applied to the clinical prognosis of COVID-19. In addition, the depletion of platelets in patients with COVID-19 is an important factor affecting their severe deterioration. With the progression of COVID-19, there is a slight decrease in the total number of platelets in the patient’s body, particularly as the volume of larger platelets sharply decreases. The importance of plateletCV (count*mean platelet volume) in evaluating the severity of COVID-19 patients is higher than the count of platelets and mean platelet volume.
Conclusion: In general, we found that for patients with COVID-19, the increase in mean platelet volume was a predictor for SARS-Cov-2. The rapid decrease of platelet volume and the decrease of total platelet volume are dangerous signals for the aggravation of SARS-Cov-2 infection. The analysis and modeling results of this study provide a new perspective for individualized accurate diagnosis and treatment of clinical COVID-19 patients.
Introduction
Coronavirus disease 2019 (COVID-19) is an extremely contagious disease produced in humans by the severe acute respiratory syndrome coronavirus-2 (SARS-CoV-2) (1). Since the first instance of COVID-19 was found in December 2019, it has quickly spread throughout the entire planet (2–4). The World Health Organization classified COVID-19 as a “Global Pandemic” in March of 2020 (5). 81 percent of COVID-19 patients had cold-like symptoms and moderate pneumonia, 14 percent had severe respiratory syndrome, and 5 percent had critical respiratory failure, septic shock, and/or multiple organ dysfunction or failure; the overall fatality rate was 1%. In terms of severity and duration, COVID-19 has unique characteristics (6). In addition, the incidence of severe and critical cases is considerably greater than that of influenza and tuberculosis (7). Individual differences in gene expression, metabolite levels, and immune cell composition affect the immune response via numerous mechanisms and impact COVID-19 susceptibility, disease severity, and prognosis (8–10). Therefore, it is essential to investigate the pathophysiological characteristics of patients, identify risk factors of disease progression for clinical diagnosis, and create COVID-19 preventive and therapeutic measures (11, 12).
With the continuous optimization and improvement of computer technology, statistics, deep learning and other techniques are widely used for accurate data analysis (13–16). Deep learning-based artificial intelligence algorithms have recently demonstrated distinct advantages in the accurate detection and treatment of diseases (17–21). Using a multi-classification modeling approach with unique risk indices, this method simultaneously predicts numerous disease states and generates a model with a high level of accuracy (22, 23). Using CT images of COVID-19 patients, researchers from the Macau University of Science and Technology presented their findings on deep learning modeling in the journal Cell in June of 2020 (24). Overall, the system differentiated COVID-19 pneumonia patients from the other two groups (other common pneumonia and healthy controls) with a sensitivity of 94.93%, a specificity of 91.13%, and an AUC of 0.89. Active learning is a machine learning method that actively selects the most valuable samples for labeling. The goal is to achieve the best possible performance of the model using the smallest possible number of high-quality samples with annotation (25). Zongwei Zhou et al. significantly reduce the cost of annotating medical images by integrating active learning and migration learning. Compared to state-of-the-art random selection methods, the annotation cost can be reduced by at least half in three medical applications and by over 33% in natural image datasets (26).
However, the majority of convenient features available to clinicians in real-world situations are derived from clinical test findings, such as blood proteins (27), metabolites, immune cell counts, etc (28). This project enrolled patients with varying severity levels, collected individualized clinical testing results from them, and used deep learning multi-model combination technology based on neural networks to obtain an artificial intelligence individualized diagnosis and treatment model for COVID-19 with fewer features, high accuracy, and promoted clinical application by optimizing modeling parameters to achieve convenient and rapid monitoring of COVID-19 (29, 30).
Materials and methods
Data collection and data cleaning
In this research, we collected 1,799 samples, including 560 individuals in the control group of non-respiratory infectious diseases (Negative), 681 patients in the control group of other respiratory viral infections (Other) (table of patients’ viral infections, Supplement Table 1), and 558 patients in the group of coronavirus. To be more in line with the real world and the clinical application, we did not consider comorbidity, sex, and other chronic pulmonary disease from the patient subgroups.
The clinical features are included as follows: 1) Clinical hematology indicators: leukocytes, red blood cells, bone marrow indicators, coagulation factors, platelet functions, blood type, blood rheology indicators, etc. 2) Clinical body fluid indicators: body fluid routine examination, urine chemistry, urine cell types, urine crystalline types, etc. 3) Clinical chemistry indicators: various proteins, sugar and metabolite indicators, lipid and lipoprotein levels, cardiac, hepatobiliary and renal disease indicators, other enzyme indicators, inorganic substance determination and blood gas analysis, vitamins, amino acids, thyroid function indicators, various trace elements, free radicals, sex hormones, other hormones, etc. 4) Clinical immunology indicators: tumor-related antigens, lymphocyte and subpopulation classification, cytokine classes, allergen test results, etc. 5) Other indicators: such as clinical bacteriological test indicators, virology, antibiotic classes, gene mutations, drug resistance genes, etc.
Feature engineering
In this paper, all statistical learning methods were performed based on R version 4.2.1. Machine learning and deep learning modeling was performed based on python version 3.9.12 and scikit-learn version 1.0.2. The main statistical learning methods include ANOVA (data conform to normal distribution), Wilcox rank sum test (data are discrete variables), and Fisher’s exact test (data are frequency variables). False discovery rate (FDR) correction was performed for all P values using the Benjamini-Hochberg method, and variables were considered to be statistically significantly different when FDR < 0.05. Variables with high significance and statistically significant differences were screened using the adaptive-Lasso regression method. Variables with covariance higher than 0.95 were filtered out using Pearson or Spearman correlation. To explain the impact and contribution of each clinical characteristic on the classification of SARS-CoV-2 positive patients, we screened variables using the Shapley additive explanation method (SHAP).
We serialized patients with different severity levels by numerical simulation. The aim is to use the information of patients with different severity levels as an important reference indicator. Specifically, the transformation of the three subgroups into three continuous variables is based on an intrinsic linear pattern between the three severity levels, with the numbers increasing in severity and increasing in magnitude. The three subgroups belong to the three classification questions. However, the patients in the three subgroups are progressive in severity. The event y that we want to predict is not simply a three-classification problem. Therefore, we use a set of numbers that model the linear relationship between the three y’s internally. and then use the optimal set of features x for artificial neural network-based regression modeling. The hidden association information between the event y to be predicted and between y and the feature set x can be explored and used to the maximum extent to obtain the optimal model. After careful and sufficient debugging, we obtain the optimal numerical simulation of the event to be predicted y. Thus, the three groupings are transformed into three continuous variables, and the numbers become larger as the severity increases step by step. The basis of our grouping is based on the official hospitalization indicators given. Group 1: no hospitalization, patient whose nucleic acid test result is positive and has some clinical symptoms but not be hospitalized. Group 2: regular hospitalization, patient who has some clinical symptoms related to COVID-19 and need hospitalization. Group 3: semi-intensive unit or intensive-care unit, in this group patient need more carefully management which is staffed and equipped to provide a higher level of care than a general ward or patient who has severe and life-threatening medical conditions.
Build the neural network
After screening out the important features by the above steps, the obtained features with significant contribution to different category groups were analyzed by deep learning methods to build disease prediction models. It was optimal to construct deep neural network models for disease prognosis assessment using the optimal set of features. Firstly, the data set was divided into training, validation and test sets (8:1:1). Second, since the initial values of each feature span a large range, this difference will lead to an imbalance of weights among the features introduced during model construction, which will affect the performance of the model. Therefore, we zero-averaged the features of the training, validation, and test sets so that each feature has the same variance. We use SELU as the main activation function of the model in model training to prevent the gradient disappearance/explosion phenomenon; epoch is set to 100, and to prevent overfitting, we set Dropout to 0.5 and early stop, where the patient parameter is 30.
Result
Comparison of the clinical features between COVID-19 and other groups
We collected a total of 1,799 individuals, including 560 controls for non-respiratory infectious diseases (Negative), 681 controls for other respiratory virus infections (Other), and 558 coronavirus infections (Positive) for COVID-19 (Figure 1A, Top). Clinical features, such as hematological indicators, chemical indicators, immunological indicators, and virological indicators, were gathered from each of the three groups to be compared with one another (Supplementary Figure 1). Negative, other, and COVID-19 were differentiated using a total of 32 distinct features (Figure 1B). When compared to other viruses that can cause respiratory viral infections (p = 1.0697e-11, Figure 1C), SARS-CoV-2 appears to preferentially infect older persons.
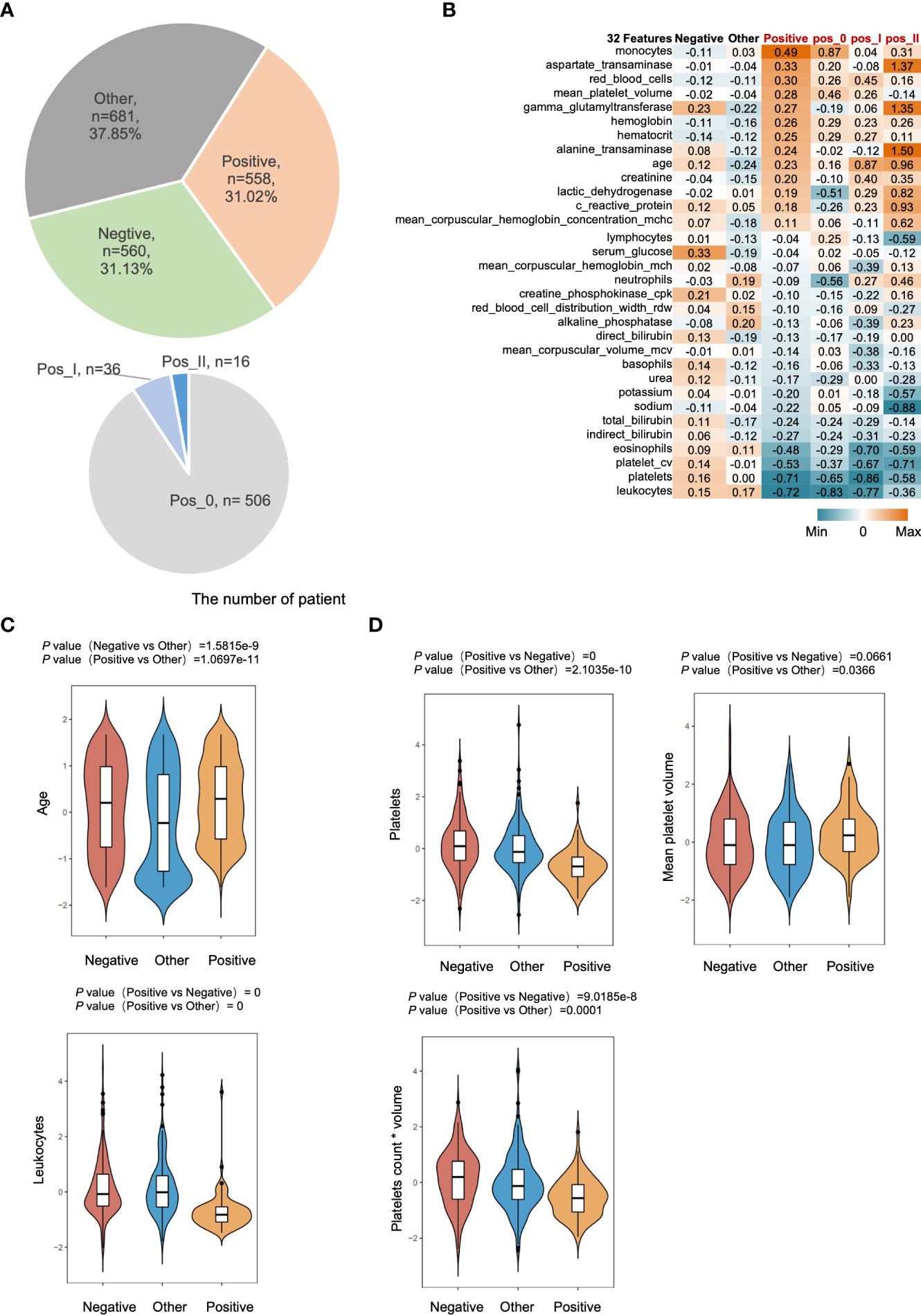
Figure 1 Distinct features of COVID-19. (A) The sample distribution in this study. Positive, represents confirmed COVID-19 infected patients. Negative, represents non-respiratory infectious patients. Other, represents non-COVID-19 infected patients (Top). Pos_0 represents latently infected patients. Pos_1, represents general inpatients. Pos_2, represents serious patients in the intensive care unit (Bottom). (B) The heatmap shows the relative expression level of 32 indicators with a cumulative contribution of 100% among different classification groups the number in heatmap is the average value within the group after zero mean normalization of the original expression value. (C) Box plot of normalize value of age and leukocytes among different classification groups. (D) Box plot of normalize value of platelets, mean platelet volume and platelets count * volume among different classification groups.
Alterations in patients’ coagulation levels have been found to have a strong correlation with a bad prognosis in COVID-19 patients (31–36). As a result, we analyzed platelet levels and their volumes in three groups and discovered that SARS-CoV-2 infection was linked with a decrease in platelet count but an increase in mean platelet volume. By calculating the plateletCV (count*mean platelet volume), we determined that COVID-19 had a significantly lower total platelet volume than the other two groups (Figure 1D).
Development of three-phase COVID-19 individualized prediction model
To examine the clinical characteristic indicators of diseases with different severity categories, we re-grouped the enrolled patients as control groups (mentioned above) and positive groups, and then classified them into three-phase diseases according to their severity as phase 0 (non-inpatients), phase I (general inpatients), and phase II (ICU inpatients), respectively. A total of 99 testing indexes of clinical features were uploaded as input to screen the variables by modeling importance analysis. The results showed that 13 features had a cumulative contribution of 100% in predicting the three-phase COVID-19 (Figure 2A), among which leukocytes levels, age, neutrophils levels, total platelet volume, mean hemoglobin concentration, and lymphocytes levels were most important. Interestingly, as disease progressed, features such as leukocytes levels, age, neutrophils levels, and mean hemoglobin concentration were obviously elevated (positive correlation), while total platelet volume, lymphocytes levels, and hemoglobin concentration were continuously reduced (negatively correlated) (Figure 2B).
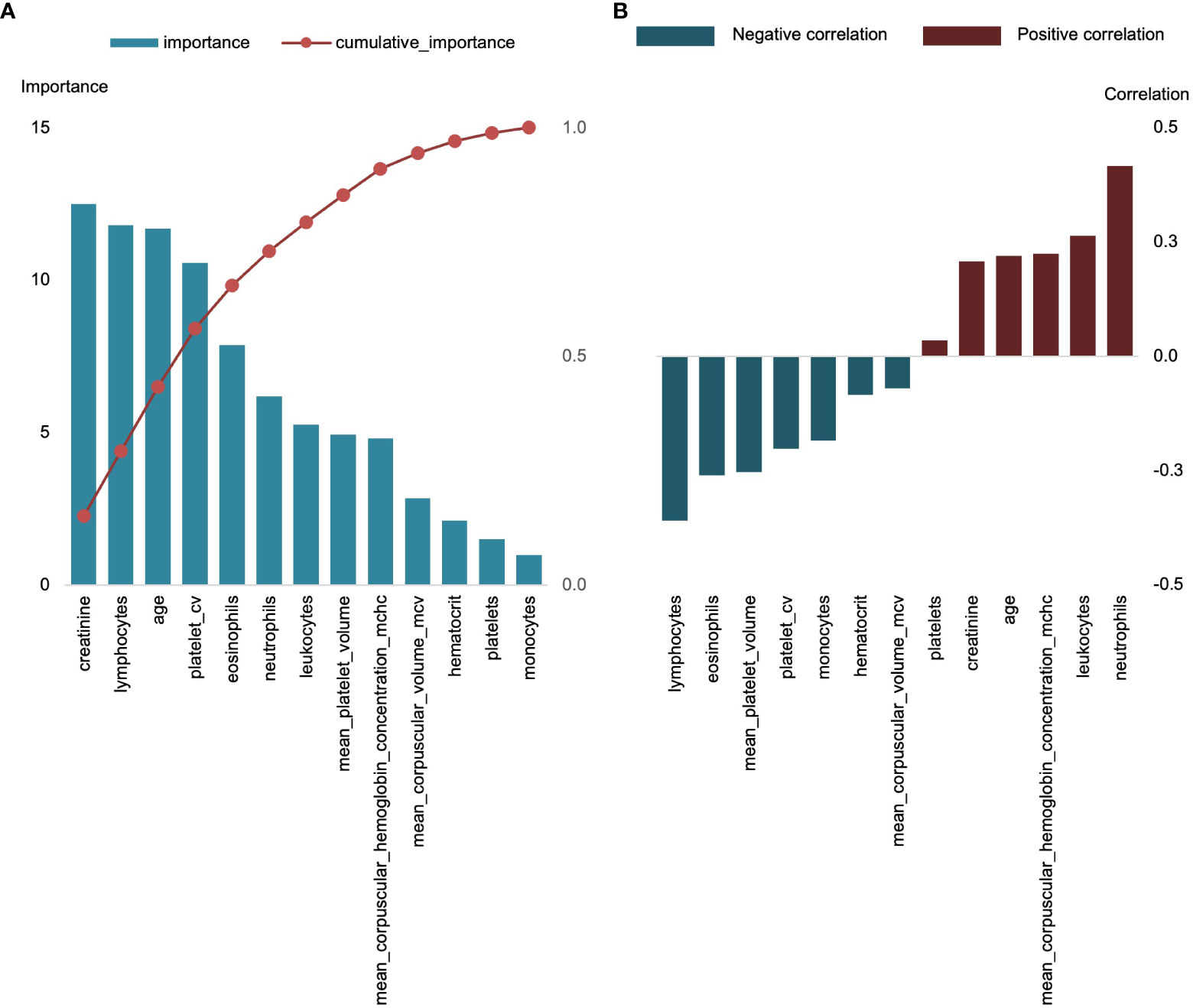
Figure 2 Top 13 features for classification of three-phase COVID-19. (A) The importance distribution and the cumulative importance curve of 13 features, left Y-axis represents importance, right Y-axis represents cumulative importance; X-axis is the name of each feature. (B) Correlation of 13 characteristics with positive group.
We employed 13 clinical features to construct a deep neural network model to explore the individualized prediction model for three distinct phases of COVID-19 (Figure 3). Using an iterative optimization method, the optimal model was chosen. The loss value for the training set is 31.08 and the validation set is 106.86. (Figure 4A). The severity of COVID-19 was then predicted using a linear model based on deep learning. The correlation coefficient between the projected results of the model and the fitting curve of the actual value is 0.9449 (Figure 4B), which may be applied to the COVID-19 clinical prognosis.
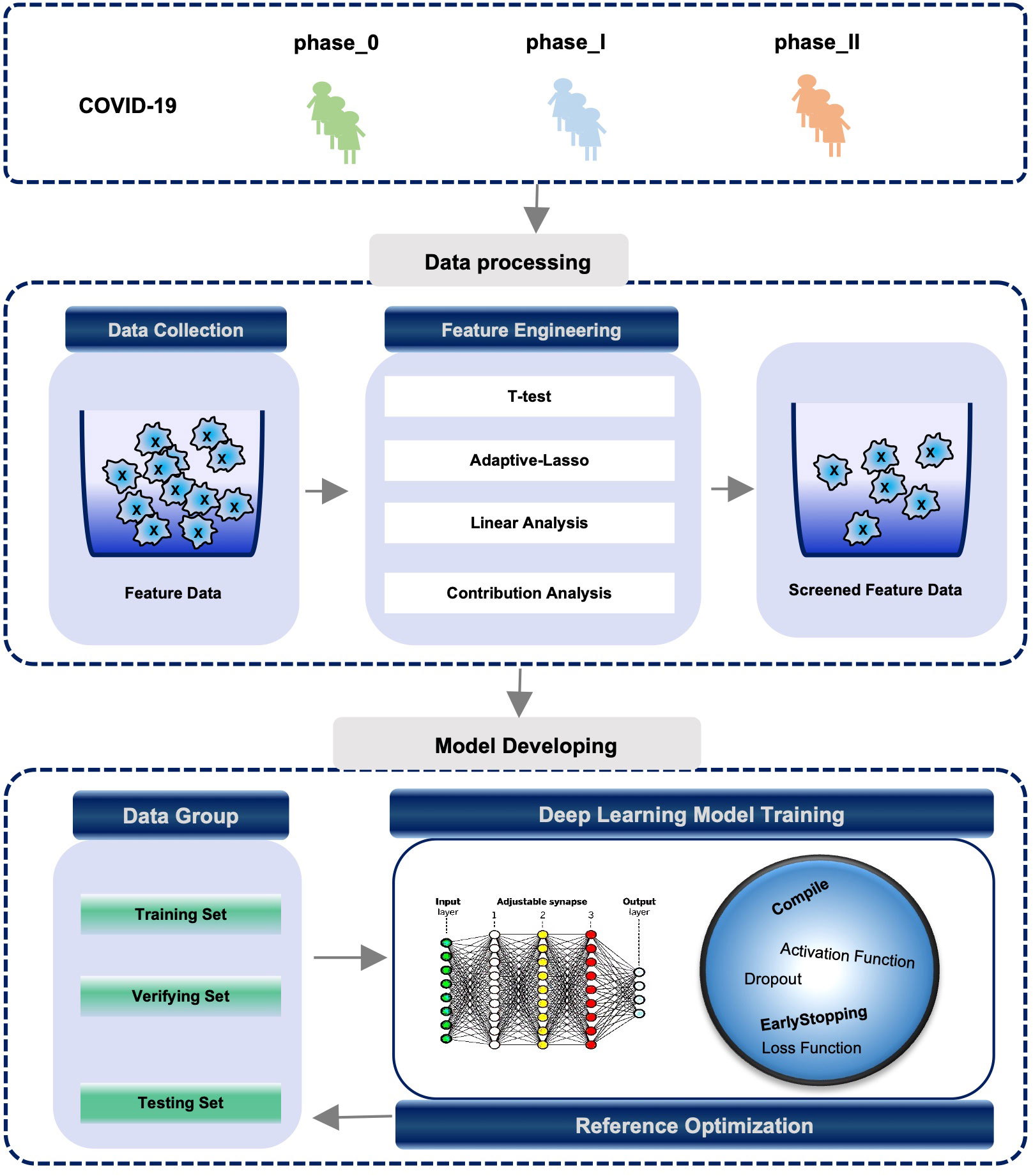
Figure 3 Process of constructing COVID-19 individualized diagnostic model based on active regression learning. Process of constructing a COVID-19 individualized diagnostic model based on active regression learning. (TOP) The samples are grouped into phase_0, phase_I, and phase_II according to different symptoms or disease types. (Middle) The optimal set of features is filtered using feature engineering methods (see Methods). (Bottom) Individualized diagnostic model building logic and model efficacy assessment rules.
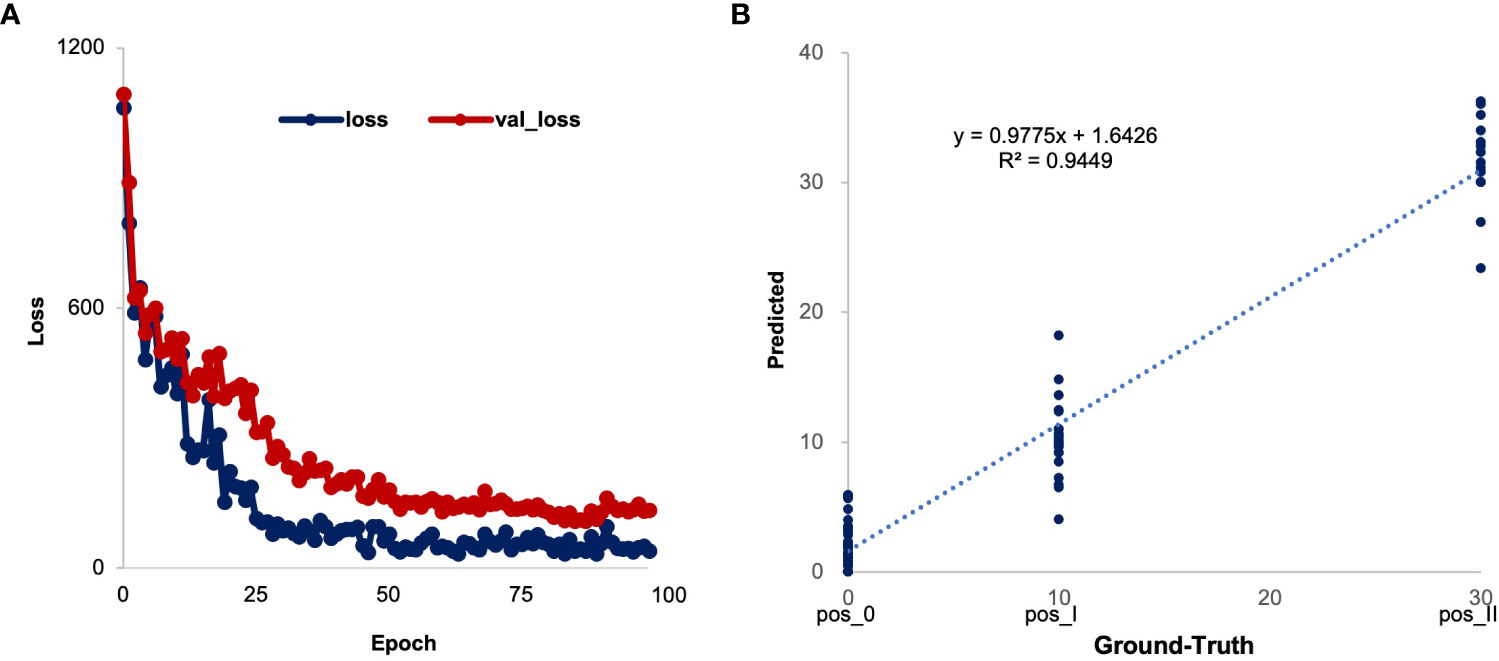
Figure 4 Process of constructing COVID-19 individualized diagnostic model based on active regression learning. (A) The change curve of the loss value of the training and validation sets during epoch iterations. The value of the loss function of the model is calculated from the mean squared error (MSE). (B) The test set results are used to evaluate the performance of model.
Risk factors of severe COVID-19
To determine the correlation between coagulation levels and the severity of COVID-19, we classified SARS-CoV-2 positive patients into three phases: phase 0 patients were latently infected; phase I patients were general inpatients; and phase II patients were serious patients in the intensive care unit (ICU). As COVID-19 deteriorated, levels of total platelets in the patient’s blood declined somewhat, while the number of large platelets decreased substantially and the volume of total platelets in the blood continued to decrease (Figure 5). Differential analysis indicated that the overall age of the positive group was significantly higher compared to the control group. Severe COVID-19 (phase_II) were predominantly of advanced age, while other diseases (control group) generally lead to severe states in younger patients (Figure 5A). Leukocyte levels were slightly elevated in the severe COVID-19 (phase_II) compared to other less sever phases, but overall levels of leukocytes were lower than in the control group. (Figure 5B). However, leukocytes remained at a low level in patients after SARS-Cov-2 infection.
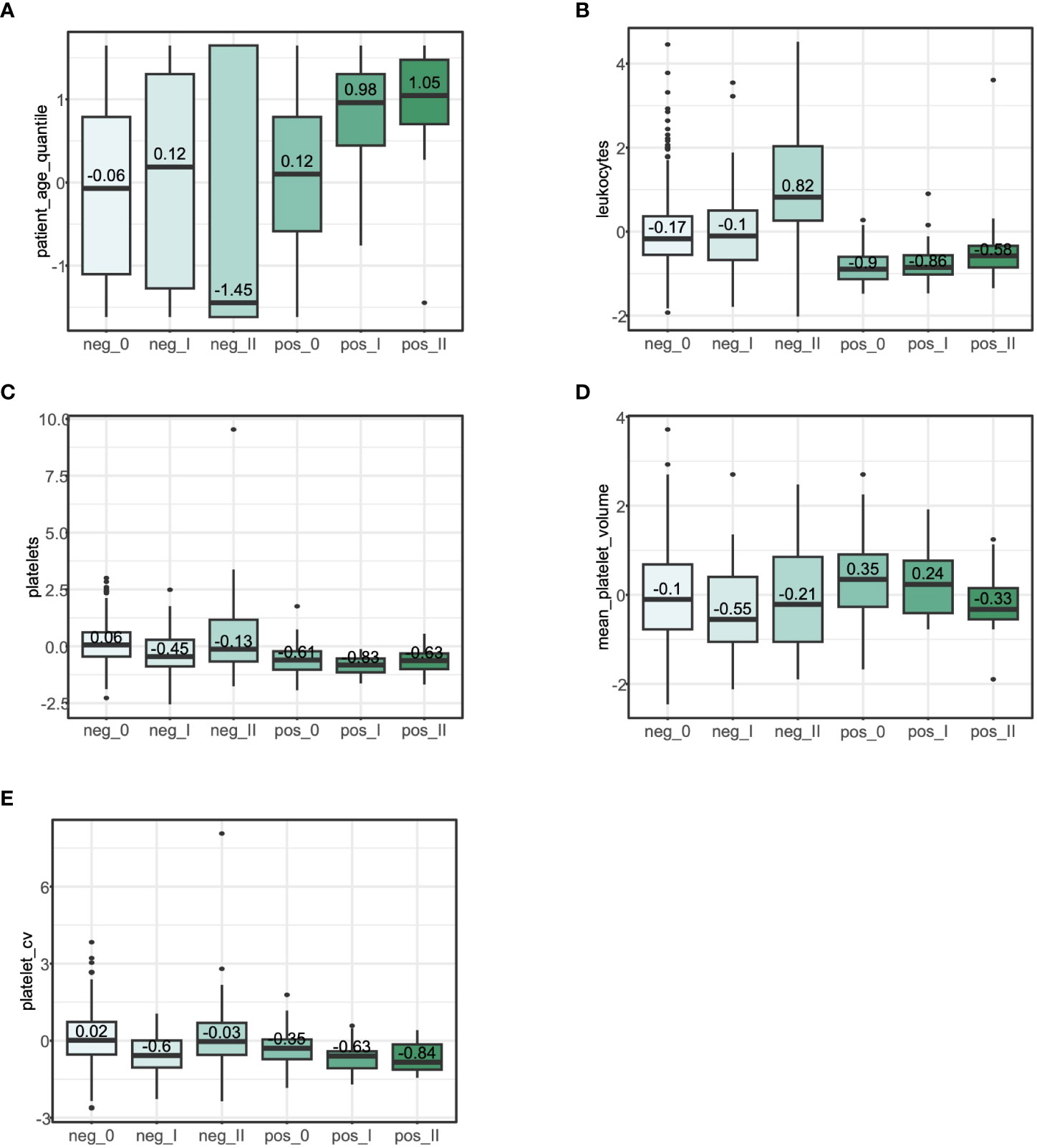
Figure 5 Risk factor analysis for three groups of COVID-19. pahse_0, latently infected; pahse_I, general inpatients; pahse_II, serious patients in ICU. Box plots show the differences in characteristics between different severity levels within different infection groups. Numbers are within-group means after zero-mean normalization of the original expression values. (A), Box plot of the distribution of the age among different groups. (B), Box plot of the distribution of the leukocyte among different groups. (C), Box plot of the distribution of the number of total platelets among different groups. (D), Box plot of the distribution of the mean platelet volume of platelets among different groups. (E), Box plot of the distribution of the count*mean platelet volume among different groups.
By comparing the levels of platelet-related features, we found that the level of platelets with large volume was dramatically reduced and the total platelet volume in blood was continuously decreased (Figures 5C–E) within progressing three-phase COVID-19, which was in accordance with our three-phase categories of COVID-19 in Figure 1. It has been reported that myocardial infarction patients with elevated mean platelet volumes had a significantly increased incidence of death and heart failure (37, 38).
Discussion
Since the outbreak of SARS-Cov-2 virus in 2019, it has caused a huge impact on global health security and economic security. There has been an ongoing effort to develop artificial intelligence models for automated diagnosis and real-time surveillance of COVID-19 (39–43). In this study, we developed a simple, efficient, and clinically accessible AI model based on a large cohort population. The model uses fewer and easily accessible clinical features (N=13) to achieve high accuracy in patient prognosis assessment and monitoring (independent test set R2 = 0.9449). Feature filtering and extraction of large-scale clinical multi-omics data using a feature engineering approach provided us with a clearer understanding of changes in clinical indicators in patients infected with SARS-CoV-2, such as significantly lower serum leukocyte counts in COVID-19 patients than in other groups; impaired coagulation-related function was an important risk factor for COVID-19 patients, especially the mean platelet volume of rapid reduction. It is noteworthy that the leukocyte levels in COVID-19 were significantly lower than those in the Negative and Other groups, which was consistent with Xiaoqing Liu et al.’s (44) claim that a viral infection could cause a decrease in the number of leukocytes, whereas bacteria typically produce an increase in leukocyte numbers.
The interpretability of deep learning models has been one of the main reasons why the performance of the models has been questioned. In the present study, our model was transparent, and the individual metrics were clinically meaningful and valuable, with leukocyte levels, age, neutrophil levels, total platelet volume, mean hemoglobin concentration and lymphocyte levels being the most important. In this study, we found that a decrease in platelet levels and a decrease in mean platelet volume were strongly correlated with the severity of the patients. In the study of Nishikawa M et al. showed a strong association between the concentration of platelet aggregates and the severity, mortality, respiratory status and level of vascular endothelial dysfunction in patients with COVID-19 (45). Thrombocytopenia has been reported in patients hospitalized with COVID-19, and lower platelet counts are associated with poorer clinical outcomes. A meta-analysis of 7,613 participants from 31 studies noted that lower platelet counts in severe COVID-19 infections were associated with a 3-fold increased risk of developing severe COVID-19 (46). In addition, individuals such as the elderly, men, those with a history of underlying disease, and those with blood type A have been reported to be at greater risk for SARS-CoV-2 or severe COVID-19 disease (47, 48). In addition, severe COVID-19 is characterized by lymphopenia, which may be caused by direct infection of lymphocytes or suppression of bone marrow by antiviral responses (49, 50).
Overall, our study presents 13 clinical indicators that are closely related to the course of disease progression in patients with COVID-19. In actual clinical monitoring, it is recommended that physicians in the emergency or intensive care unit pay closer attention to these indicators to be able to detect adverse conditions in patients in a timely manner. Nevertheless, there are still some problems in this study. For example, we lacked serial blood macroscopic measurements from the same COVID-19 patients to further confirm the causal relationship between platelet volume and disease severity. As well as, in this study, the cases of severe patients was limited, and the absence of predictors of mortality.
Data availability statement
The original contributions presented in the study are included in the article/Supplementary Material. Further inquiries can be directed to the corresponding authors.
Author contributions
XZ and YS participated in the development of the project and manuscript preparation. YS, JD, ZC and LL and MY performed data collection and analyses and interpreted the results. YS, JD, XL and XZ wrote the manuscript. XZ conducted this study. All authors contributed to the article and approved the submitted version.
Funding
This work was supported by the China Postdoctoral Science Foundation (2021M690806). Beijing Natural Science Foundation Haidian original innovation joint fund (L202023).
Conflict of interest
The authors declare that the research was conducted in the absence of any commercial or financial relationships that could be construed as a potential conflict of interest.
Publisher’s note
All claims expressed in this article are solely those of the authors and do not necessarily represent those of their affiliated organizations, or those of the publisher, the editors and the reviewers. Any product that may be evaluated in this article, or claim that may be made by its manufacturer, is not guaranteed or endorsed by the publisher.
Supplementary material
The Supplementary Material for this article can be found online at: https://www.frontiersin.org/articles/10.3389/fimmu.2023.1141996/full#supplementary-material
Supplementary Figure 1 | Statistical table of sample information. (A), Distribution of the number of patients in different age groups and the number of patients with COVID-19 positive, COVID-19 negative, or other pneumonia. (B), Distribution of total platelet volume and mean platelet volume in patients of different ages. (C), Statistical bar chart of pathogens in patients with non-COVID-19 infections.
References
1. Sadeghi Dousari A, Taati Moghadam M, Satarzadeh N. COVID-19 (Coronavirus disease 2019): A new coronavirus disease. Infect Drug Resist (2020) 13:2819–28. doi: 10.2147/IDR.S259279
2. Lu H, Stratton CW, Tang Y-W. Outbreak of pneumonia of unknown etiology in wuhan, China: The mystery and the miracle. J Med Virol (2020) 92:401–2. doi: 10.1002/jmv.25678
3. From the American Association of Neurological Surgeons (AANS), American Society of Neuroradiology (ASNR), Cardiovascular and Interventional Radiology Society of Europe (CIRSE), Canadian Interventional Radiology Association (CIRA), Congress of Neurological Surgeons (CNS), European Society of Minimally Invasive Neurological Therapy (ESMINT), et al. Multisociety consensus quality improvement revised consensus statement for endovascular therapy of acute ischemic stroke. Int J Stroke (2018) 13:612–32. doi: 10.1177/1747493018778713
4. Huang C, Wang Y, Li X, Ren L, Zhao J, Hu Y, et al. Clinical features of patients infected with 2019 novel coronavirus in wuhan, China. Lancet (2020) 395:497–506. doi: 10.1016/S0140-6736(20)30183-5
5. Cucinotta D, Vanelli M. WHO declares COVID-19 a pandemic. Acta BioMed (2020) 91:157–60. doi: 10.23750/abm.v91i1.9397
6. Wu Z, McGoogan JM. Characteristics of and important lessons from the coronavirus disease 2019 (COVID-19) outbreak in China: Summary of a report of 72 314 cases from the Chinese center for disease control and prevention. JAMA (2020) 323:1239–42. doi: 10.1001/jama.2020.2648
7. Robba C, Battaglini D, Pelosi P, Rocco PRM. Multiple organ dysfunction in SARS-CoV-2: MODS-CoV-2. Expert Rev Respir Med (2020) 14:865–8. doi: 10.1080/17476348.2020.1778470
8. Schultze JL, Aschenbrenner AC. COVID-19 and the human innate immune system. Cell (2021) 184:1671–92. doi: 10.1016/j.cell.2021.02.029
9. Kloc M, Ghobrial RM, Kubiak JZ. The role of genetic sex and mitochondria in response to COVID-19 infection. Int Arch Allergy Immunol (2020) 181:629–34. doi: 10.1159/000508560
10. Su Y, Yuan D, Chen DG, Ng RH, Wang K, Choi J, et al. Multiple early factors anticipate post-acute COVID-19 sequelae. Cell (2022) 185:881–95.e20. doi: 10.1016/j.cell.2022.01.014
11. Salton F, Confalonieri P, Campisciano G, Cifaldi R, Rizzardi C, Generali D, et al. Cytokine profiles as potential prognostic and therapeutic markers in SARS-CoV-2-Induced ARDS. J Clin Med (2022) 11:2951. doi: 10.3390/jcm11112951
12. Kołtan S, Ziętkiewicz M, Grześk E, Becht R, Berdej-Szczot E, Cienkusz M, et al. COVID-19 in unvaccinated patients with inborn errors of immunity-polish experience. Front Immunol (2022) 13:953700. doi: 10.3389/fimmu.2022.953700
13. Alrashed FA, Alsubiheen AM, Alshammari H, Mazi SI, Al-Saud SA, Alayoubi S, et al. Stress, anxiety, and depression in pre-clinical medical students: Prevalence and association with sleep disorders. Sustainability (2022) 14:11320. doi: 10.3390/su141811320
14. Ahmad F, Shahid M, Alam M, Ashraf Z, Sajid M, Kotecha K, et al. Levelized multiple workflow allocation strategy under precedence constraints with task merging in IaaS cloud environment. IEEE Access (2022) 10:92809–27. doi: 10.1109/ACCESS.2022.3202651
15. Singamaneni KK, Dhiman G, Juneja S, Muhammad G, AlQahtani SA, Zaki J. A novel QKD approach to enhance IIOT privacy and computational knacks. Sensors (Basel) (2022) 22:6741. doi: 10.3390/s22186741
16. Guan S, Loew M. Breast cancer detection using transfer learning in convolutional neural networks. In: 2017 IEEE applied imagery pattern recognition workshop (AIPR). Washington, DC: IEEE (2017). p. 1–8. doi: 10.1109/AIPR.2017.8457948
17. Jiang F, Jiang Y, Zhi H, Dong Y, Li H, Ma S, et al. Artificial intelligence in healthcare: past, present and future. Stroke Vasc Neurol (2017) 2:230–43. doi: 10.1136/svn-2017-000101
18. Zhou Y, Wang F, Tang J, Nussinov R, Cheng F. Artificial intelligence in COVID-19 drug repurposing. Lancet Digit Health (2020) 2:e667–76. doi: 10.1016/S2589-7500(20)30192-8
19. Taleghani N, Taghipour F. Diagnosis of COVID-19 for controlling the pandemic: A review of the state-of-the-art. Biosens Bioelectron (2021) 174:112830. doi: 10.1016/j.bios.2020.112830
20. Shi F, Wang J, Shi J, Wu Z, Wang Q, Tang Z, et al. Review of artificial intelligence techniques in imaging data acquisition, segmentation, and diagnosis for COVID-19. IEEE Rev BioMed Eng (2021) 14:4–15. doi: 10.1109/RBME.2020.2987975
21. Alsharif W, Qurashi A. Effectiveness of COVID-19 diagnosis and management tools: A review. Radiogr (Lond) (2021) 27:682–7. doi: 10.1016/j.radi.2020.09.010
22. Sh Y, Liu B, Zhang J, Zhou Y, Hu Z, Zhang X. Application of artificial intelligence modeling technology based on fluid biopsy to diagnose alzheimer’s disease. Front Aging Neurosci (2021) 13:768229. doi: 10.3389/fnagi.2021.768229
23. Sh Y, Zhang X, Yang Z, Dong J, Wang Y, Zhou Y, et al. CaSee: A lightning transfer-learning model directly used to discriminate cancer/normal cells from scRNA-seq. Oncogene (2022) 41:4866–76. doi: 10.1038/s41388-022-02478-5
24. Zhang K, Liu X, Shen J, Li Z, Sang Y, Wu X, et al. Clinically applicable AI system for accurate diagnosis, quantitative measurements, and prognosis of COVID-19 pneumonia using computed tomography. Cell (2020) 181:1423–33.e11. doi: 10.1016/j.cell.2020.04.045
25. Ren P, Xiao Y, Chang X, Huang P-Y, Li Z, Gupta BB, et al. A survey of deep active learning. ACM Comput Surv (2022) 54:1–40. doi: 10.1145/3472291
26. Zhou Z, Shin JY, Gurudu SR, Gotway MB, Liang J. Active, continual fine tuning of convolutional neural networks for reducing annotation efforts. Med Image Anal (2021) 71:101997. doi: 10.1016/j.media.2021.101997
27. Bellan M, Azzolina D, Hayden E, Gaidano G, Pirisi M, Acquaviva A, et al. Simple parameters from complete blood count predict in-hospital mortality in COVID-19. Dis Markers (2021) 2021:8863053. doi: 10.1155/2021/8863053
28. Pelosi P, Tonelli R, Torregiani C, Baratella E, Confalonieri M, Battaglini D, et al. Different methods to improve the monitoring of noninvasive respiratory support of patients with severe Pneumonia/ARDS due to COVID-19: An update. JCM (2022) 11:1704. doi: 10.3390/jcm11061704
29. Orlandi M, Landini N, Sambataro G, Nardi C, Tofani L, Bruni C, et al. The role of chest CT in deciphering interstitial lung involvement: systemic sclerosis versus COVID-19. Rheumatology (2022) 61:1600–9. doi: 10.1093/rheumatology/keab615
30. Bonnet B, Chabrolles H, Archimbaud C, Brebion A, Cosme J, Dutheil F, et al. Decline of humoral and cellular immune responses against SARS-CoV-2 6 months after full BNT162b2 vaccination in hospital healthcare workers. Front Immunol (2022) 13:842912. doi: 10.3389/fimmu.2022.842912
31. Luo H-C, You C-Y, Lu S-W, Fu Y-Q. Characteristics of coagulation alteration in patients with COVID-19. Ann Hematol (2021) 100:45–52. doi: 10.1007/s00277-020-04305-x
32. Corrêa TD, Cordioli RL, Campos Guerra JC, Caldin da Silva B, Dos Reis Rodrigues R, de Souza GM, et al. Coagulation profile of COVID-19 patients admitted to the ICU: An exploratory study. PloS One (2020) 15:e0243604. doi: 10.1371/journal.pone.0243604
33. Teimury A, Khameneh MT, Khaledi EM. Major coagulation disorders and parameters in COVID-19 patients. Eur J Med Res (2022) 27:25. doi: 10.1186/s40001-022-00655-6
34. Araya S, Mamo MA, Tsegay YG, Atlaw A, Aytenew A, Hordofa A, et al. Blood coagulation parameter abnormalities in hospitalized patients with confirmed COVID-19 in Ethiopia. PloS One (2021) 16:e0252939. doi: 10.1371/journal.pone.0252939
35. Asakura H, Ogawa H. COVID-19-associated coagulopathy and disseminated intravascular coagulation. Int J Hematol (2021) 113:45–57. doi: 10.1007/s12185-020-03029-y
36. Iba T, Connors JM, Levy JH. The coagulopathy, endotheliopathy, and vasculitis of COVID-19. Inflammation Res (2020) 69:1181–9. doi: 10.1007/s00011-020-01401-6
37. Chu SG, Becker RC, Berger PB, Bhatt DL, Eikelboom JW, Konkle B, et al. Mean platelet volume as a predictor of cardiovascular risk: a systematic review and meta-analysis. J Thromb Haemost (2010) 8:148–56. doi: 10.1111/j.1538-7836.2009.03584.x
38. Khattab MH, Prodan CI, Vincent AS, Xu C, Jones KR, Thind S, et al. Increased procoagulant platelet levels are predictive of death in COVID-19. Geroscience (2021) 43:2055–65. doi: 10.1007/s11357-021-00385-3
39. Alimadadi A, Aryal S, Manandhar I, Munroe PB, Joe B, Cheng X. Artificial intelligence and machine learning to fight COVID-19. Physiol Genomics (2020) 52:200–2. doi: 10.1152/physiolgenomics.00029.2020
40. Sarker IH. AI-Based modeling: Techniques, applications and research issues towards automation, intelligent and smart systems. SN Comput Sci (2022) 3:158. doi: 10.1007/s42979-022-01043-x
41. Sodhi GK, Kaur S, Gaba GS, Kansal L, Sharma A, Dhiman G. COVID-19: Role of robotics, artificial intelligence and machine learning during the pandemic. Curr Med Imaging (2022) 18:124–34. doi: 10.2174/1573405617666210224115722
42. Zoabi Y, Deri-Rozov S, Shomron N. Machine learning-based prediction of COVID-19 diagnosis based on symptoms. NPJ Digit Med (2021) 4:3. doi: 10.1038/s41746-020-00372-6
43. Walter W, Haferlach C, Nadarajah N, Schmidts I, Kühn C, Kern W, et al. How artificial intelligence might disrupt diagnostics in hematology in the near future. Oncogene (2021) 40:4271–80. doi: 10.1038/s41388-021-01861-y
44. Liu X, Zhang R, He G. Hematological findings in coronavirus disease 2019: indications of progression of disease. Ann Hematol (2020) 99:1421–8. doi: 10.1007/s00277-020-04103-5
45. Nishikawa M, Kanno H, Zhou Y, Xiao T-H, Suzuki T, Ibayashi Y, et al. Massive image-based single-cell profiling reveals high levels of circulating platelet aggregates in patients with COVID-19. Nat Commun (2021) 12:7135. doi: 10.1038/s41467-021-27378-2
46. Barrett TJ, Bilaloglu S, Cornwell M, Burgess HM, Virginio VW, Drenkova K, et al. Platelets contribute to disease severity in COVID-19. J Thromb Haemost (2021) 19:3139–53. doi: 10.1111/jth.15534
47. Jin J-M, Bai P, He W, Wu F, Liu X-F, Han D-M, et al. Gender differences in patients with COVID-19: Focus on severity and mortality. Front Public Health (2020) 8:152. doi: 10.3389/fpubh.2020.00152
48. Zhao J, Yang Y, Huang H, Li D, Gu D, Lu X, et al. Relationship between the ABO blood group and the coronavirus disease 2019 (COVID-19) susceptibility. Clin Infect Dis (2021) 73:328–31. doi: 10.1093/cid/ciaa1150
49. Prompetchara E, Ketloy C, Palaga T. Immune responses in COVID-19 and potential vaccines: Lessons learned from SARS and MERS epidemic. Asian Pac J Allergy Immunol (2020) 38:1–9. doi: 10.12932/AP-200220-0772
Keywords: COVID-19, deep learning, active regression, feature engineering, clinical data
Citation: Sh Y, Dong J, Chen Z, Yuan M, Lyu L and Zhang X (2023) Active regression model for clinical grading of COVID-19. Front. Immunol. 14:1141996. doi: 10.3389/fimmu.2023.1141996
Received: 11 January 2023; Accepted: 13 March 2023;
Published: 21 March 2023.
Edited by:
Jagadeesh Bayry, Indian Institute of Technology Palakkad, IndiaReviewed by:
Chiara Bozzi, University of Trieste, ItalyMattia Bellan, University of Eastern Piedmont, Italy
Copyright © 2023 Sh, Dong, Chen, Yuan, Lyu and Zhang. This is an open-access article distributed under the terms of the Creative Commons Attribution License (CC BY). The use, distribution or reproduction in other forums is permitted, provided the original author(s) and the copyright owner(s) are credited and that the original publication in this journal is cited, in accordance with accepted academic practice. No use, distribution or reproduction is permitted which does not comply with these terms.
*Correspondence: Xiuli Zhang, zhxiuli@gmail.com; Lingna Lyu, lvlingna003@163.com; Meiqing Yuan, sequence17@163.com
†These authors have contributed equally to this work