- 1Cancer Research Center, Beijing Chest Hospital, Capital Medical University/Beijing Tuberculosis and Thoracic Tumor Research Institute, Beijing, China
- 2Department of Medical Oncology, Beijing Tuberculosis and Thoracic Tumor Research Institute/Beijing Chest Hospital, Capital Medical University, Beijing, China
Background: Remarkably, the anti-cancer efficacy of immunotherapy in lung adenocarcinoma (LUAD) has been demonstrated. However, predicting the beneficiaries of this expensive treatment is still a challenge.
Materials and methods: A group of patients (N = 250) diagnosed with LUAD and receiving immunotherapy were retrospectively studied. They were randomly divided into a training dataset (80%) and a test dataset (20%). The training dataset was utilized to train neural network models to predict patients’ objective response rate (ORR), disease control rate (DCR), responders (progression-free survival time > 6 months), and overall survival (OS) possibility, which were validated by both the training and test datasets and packaged into a tool later.
Results: In the training dataset, the tool scored 0.9016 area under the receiver operating characteristic (AUC) curve on ORR judgment, 0.8570 on DCR, and 0.8395 on responder prediction. In the test dataset, the tool scored 0.8173 AUC on ORR, 0.8244 on DCR, and 0.8214 on responder determination. As for OS prediction, the tool scored 0.6627 AUC in the training dataset and 0.6357 in the test dataset.
Conclusions: This immunotherapy efficacy predictive tool for LUAD patients based on neural networks could predict their ORR, DCR, and responder well.
Highlights
Remarkably, the anti-cancer efficacy of immunotherapy in lung adenocarcinoma has been demonstrated, but predicting the beneficiaries of this expensive treatment is still a challenge.
We developed a clinician-friendly tool to predict lung adenocarcinoma immunotherapy efficacy by only using patients’ demographic features and routine testing to act as predictive variables, without any additional or expensive examination.
This tool might provide some reference for clinicians on the management of lung adenocarcinoma patients’ therapy.
1 Introduction
Lung cancer remains one of the leading causes of cancer-related death worldwide with a 5-year relative survival rate of 23.6%, accounting for approximately one in five (18.0%) deaths (1, 2). Lung adenocarcinoma (LUAD), the most prevalent histological type of lung cancer, comprised virtually 40% of all cases, and the incidence has risen over the last few decades with progressive screening, examinations, and diagnosis (3, 4). Nearly half of LUAD patients harbor activating oncogenes, and identification of oncogenic alteration is routine in clinical practice. Targeted therapy-based precise genotyping has significantly prolonged the survival of LUAD patients with driver gene mutations and profoundly led tumor treatment strategies to a revolutionary era, which emerged and developed rapidly in the past decade (5, 6). However, a majority of patients received an initial response but eventually developed resistance to targeted therapy (6). These cases did not change much until immunotherapy appeared. In addition, patients without driver oncogenes had a poor outcome because of lacking targeted therapy when compared to those with oncogenes, although the combination of chemotherapy with bevacizumab was an optional first-line treatment.
Therapeutic advances in novel immunotherapies or immunotherapy combinations based on the interaction between the human immune system and cancer have emerged rapidly in the past few years. Remarkably, the anti-cancer efficacy and safety of immunotherapy have been demonstrated in numerous ongoing clinical trials (7–11). Among these various types of immunotherapies with different molecular targets, therapeutically targeting immune inhibitory checkpoints through the blockade of programmed cell death 1 (PD-1) or programmed cell death ligand 1 (PD-L1) has unprecedentedly led to durable responses across a broad range of human cancers, which was then widely used in the clinical practice of various solid tumors (12). PD-L1 expression detected by immunohistochemistry (IHC) is widely considered the gold standard in predicting the response for anti-PD1/PDL1 in immunotherapy (13). However, considering the complex and highly regulated nature of the immune system, only a minority of patients experienced durable benefits from these therapies. The role of PD-L1 in predicting efficacy and identifying beneficiary patients is still imperfect. To overcome this dilemma, there has been a growing interest in exploring interior biomarkers or formulating methods or tools based on external clinicopathological characteristics to predict the response of immune checkpoint inhibitors (ICIs) for discovering individualized treatment strategies tailored to patient-specific characteristics to maximize efficacy and achieve personalized medicine.
In order to address this clinical need, 250 LUAD patients with anti-PD1/PDL1 immunotherapy were retrospectively studied, and a predictive tool was developed then based on their real clinical features using a state-of-the-art neural network algorithm. The tool has been uploaded, which might provide some reference for clinicians on the management of LUAD patients.
2 Materials and methods
2.1 Data sources and study design
This research was designed as a retrospective cohort study. Patients diagnosed with LUAD pathologically and received immunotherapy from January 2016 to November 2021 in Beijing Chest Hospital affiliated with Capital Medical University were included. Inclusion criteria were as follows: patients had at least one evaluable lesion, receiving at least two cycles of immunotherapy, and had a response evaluation according to Response Evaluation Criteria in Solid Tumors (RECIST) v1.1. Meanwhile, patients with active autoimmune disease, symptomatic interstitial lung disease, and multiple primary pulmonary carcinomas were excluded. A total of 250 patients met the above criteria and were enrolled finally and were then followed up until lost to follow-up or death. The last follow-up time was 30 April 2022. All data were divided into two groups randomly: the training dataset (80% of the total) and test dataset (20% of the total). The training dataset was utilized to train the model, validated by itself and the test dataset (Figure 1). This study has been approved by the Ethics Committee of Beijing Chest Hospital affiliated with Capital Medical University.
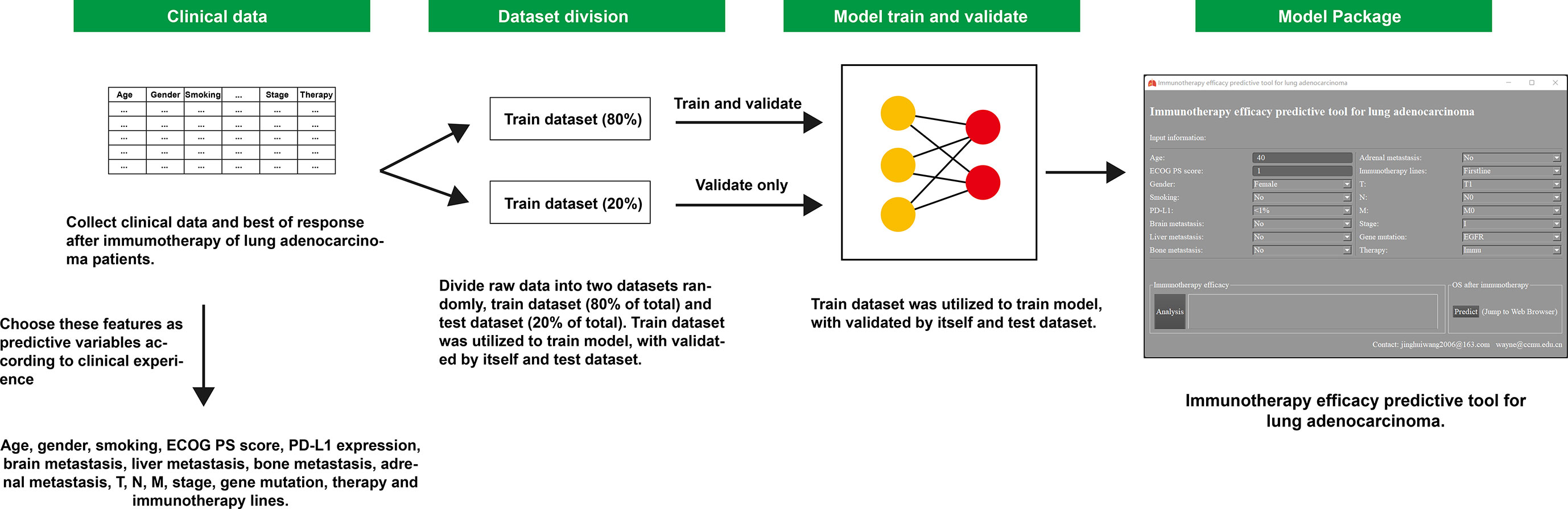
Figure 1 Workflow diagram of this study. ECOG, Eastern Cooperative Oncology Group; PS score, performance status score; PD-L1, programmed cell death ligand 1; OS, overall survival.
2.2 Clinical features and predictive variables
According to clinical experience, we chose and collected these clinical features as predictive variables: age, gender, smoking, Eastern Cooperative Oncology Group (ECOG) performance status score (PS), PD-L1 expression, brain metastasis, liver metastasis, bone metastasis, adrenal metastasis, T, N, M, stage, gene mutation, therapy methods, and immunotherapy lines. TNM stage was evaluated in terms of the 8th American Joint Committee on Cancer (AJCC) stage. Gene mutation was classified into the following categories: EGFR, KRAS, other mutation (TP53), uncommon mutation (ALK, ROS1, RET, MET, BRAF, and HER2), and negative. Therapy methods included immunotherapy (Immu) only, immunotherapy with antiangiogenic (Antiangio), immunotherapy with chemotherapy (Chemo), or a combination of the above three treatments. Immunotherapy medicine was divided into two types: first line or subsequent lines.
There were some missing values (Supplement Figure 1) in certain clinical features, so we used Multivariate Imputation by Chained Equations method to impute them, with the help of R package mice (14).
2.3 Study outcome
The primary outcome was the objective response rate (ORR). According to patients’ best of response (BOR) to immunotherapy, they were assessed as complete response (CR), partial response (PR), stable disease (SD), or progressive disease (PD). Patients showing CR or PR were regarded as ORR. The subordinate outcome was disease control rate (DCR), responder identification, and overall survival (OS). DCR included CR, PR, and SD. Patients whose progression-free survival (PFS) time was more than 6 months after immunotherapy were considered responders. The measurement above complied with RECIST v1.1. The PFS was from the first day of immunotherapy to the date of disease progression or any cause of death. The OS was defined from the first day of immunotherapy to the date of death due to any cause.
2.4 Model training
Before training, numerical variables were standardized, which meant numerical variables subtracted their means and divided by their standard deviations, while categorical variables were converted into dummy variables, such as replacing gender with two dummy variables (female = 0 or male = 1). Age and ECOG PS were standardized, and the other clinical features were transformed into dummy variables.
Given this classification task, we used dense neural networks (DNNs) to predict the outcomes. ORR, DCR, and responder identification were analyzed by three DNNs independently. OS was predicted by a neural network survival model based on Katzman’s DeepSuvr theory (15). Usually, the neural network performed well in classification tasks, but it is not its forte to handle time-dependent data.
To obtain better training effectiveness, we used batch training, dropout layers, and early stopping function during the above process. Batch training meant that models were trained with several samples per training epoch. Dropout layers randomly set input units to 0 at each step during training to prevent overfitting. Early stopping function could end up training immediately as the model’s performance did not get promoted after selected training epochs. These procedures were completed in python 3.9 (https://www.python.org/).
2.5 Model evaluation and packaged into a tool
The receiver operating characteristic (ROC) curve and the area under the receiver operating characteristic curve (AUC) were applied to evaluate the models’ performance. The closer the ROC curve is to the upper left corner of the graph, the higher the accuracy of the model. Similarly, the closer the AUC is to 1, the better the performance of the model. A model with AUC ≥ 0.8 is considered acceptable and performed well (16). The training dataset was used to conduct models, which were then validated by both the training dataset and test dataset.
Finally, all models were packaged into an immunotherapy efficacy predictive tool for lung adenocarcinoma. This tool was a Windows 11 64-bit executable program and free for doctors and researchers to use.
2.6 Statistical analysis
We conducted Cox proportional hazards regression to explore the prognostic risk factors and protective factors on OS in advanced LUAD patients (III–IV stage) after immunotherapy. All statistical analyses were completed with R software (https://www.r-project.org/). Categorical features were represented by numbers and percentages, compared by chi-square or Fisher’s exact test. Skewed distribution data were represented by the median and interquartile range (IQR) and analyzed by the Wilcoxon test. A two-sided p < 0.05 was considered to be statistically significant.
3 Results
3.1 Clinical features of patients
A total of 250 patients were enrolled. They were classified into two groups—responders (41.6%, 104/250) and non-responders (58.4%, 146/250)—according to the duration of PFS. The PD-L1 expression of responders (PFS > 6 months) was 1%–49% or ≥50% mostly, while non-responders (PFS ≤ 6 months) mainly expressed PD-L1 less than 1%. Responders had less adrenal metastasis and seemed to receive first-line immunotherapy medicine more. All responders showed PR or SD without any PD, but non-responders tended to achieve SD or PD the most. Responders had a longer OS than non-responders. In brief, responders tended to achieve a higher PD-L1 expression and less adrenal metastasis and used first-line immunotherapy medicine more commonly. Otherwise, responders and non-responders had similar features in other aspects, like age, gender, smoking, ECOG PS score, brain metastasis, liver metastasis, bone metastasis, T, N, M, stage, mutation, and therapy (Table 1).
Figure 2 visually illustrates the source of PR, SD, and PD patients. Figure 2A shows the relation between categorical features and BOR, while Figures 2B, C display the numerical features’ distribution (age and ECOG PS score) in different BOR groups.
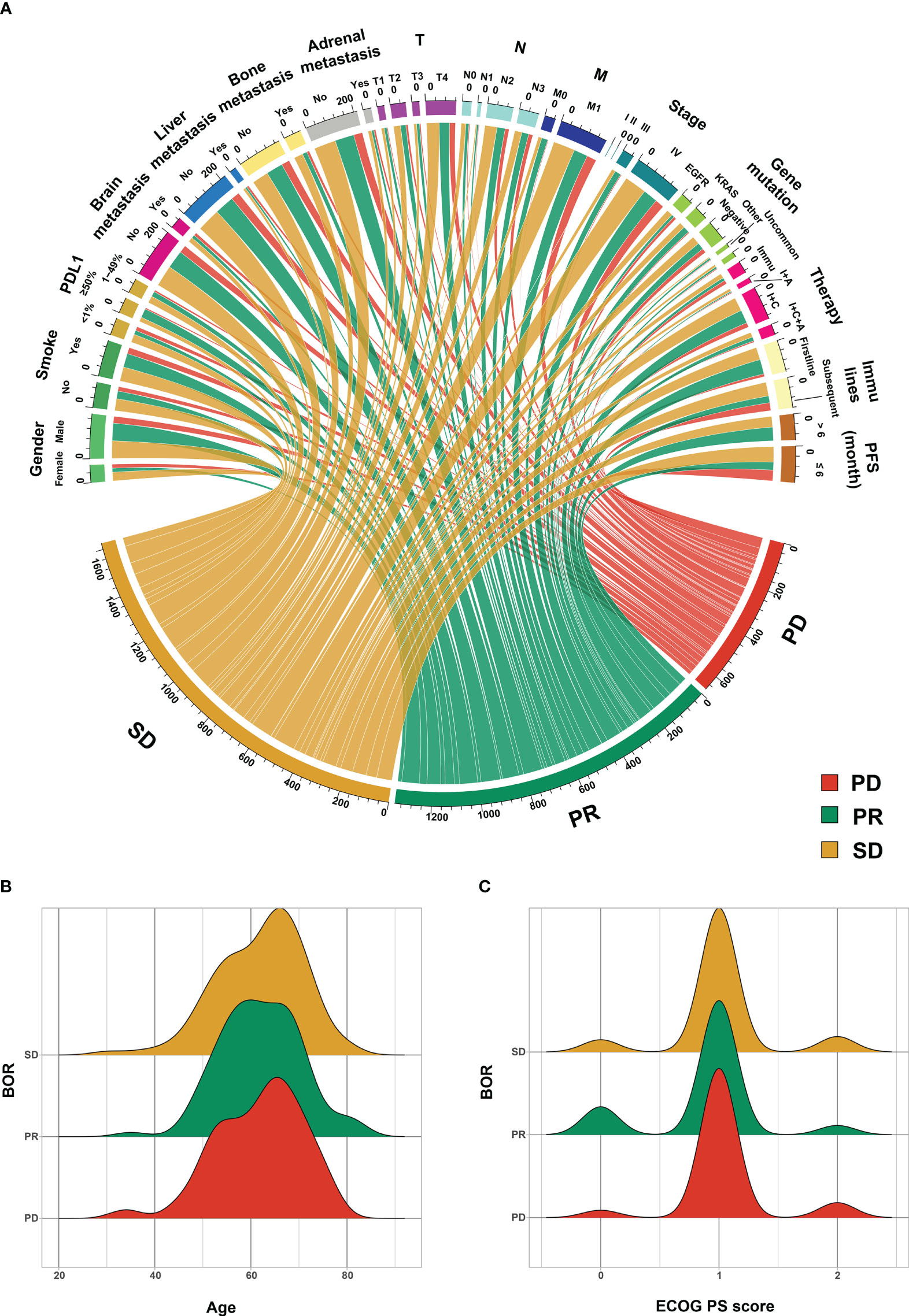
Figure 2 The source of progressive disease (PD) partial response (PR) and stable disease (SD) patients. (A) categorical features, (B) age, and (C) Eastern Cooperative Oncology Group (ECOG) performance status score (PS score). PD-L1, programmed cell death ligand 1; Other gene mutation, TP53; Uncommon gene mutation, ALK, ROS1, RET, MET, BRAF, and HER2; Immu, immunotherapy, I+A, immunotherapy and antiangiogenic therapy; I+C, immunotherapy and chemotherapy therapy; I+C+A, immunotherapy, chemotherapy, and antiangiogenic therapy; PFS, progression-free survival; BOR, best of response.
3.2 Model performance
The immunotherapy efficacy predictive models showed satisfactory performance. In the training dataset, models scored 0.9016 (95% confidence interval (CI): 0.8592–0.9441) on ORR judgment, 0.8570 (95% CI, 0.7923–0.9218) on DCR, and 0.8395 (95% CI, 0.7829–0.8960) on responder prediction. In the test dataset, the models scored 0.8173 (95% CI, 0.6959–0.9388) on ORR, 0.8244 (95% CI, 0.7000–0.9488) on DCR, and 0.8214 (95% CI, 0.6903–0.9526) on responder determination (Table 2 and Figure 3). As for OS prediction, model performance was very ordinary, scoring 0.6627 (95% CI, 0.6613–0.6640) in the training dataset and 0.6357 (95% CI, 0.6331–0.6384) in the test dataset (Table 2).
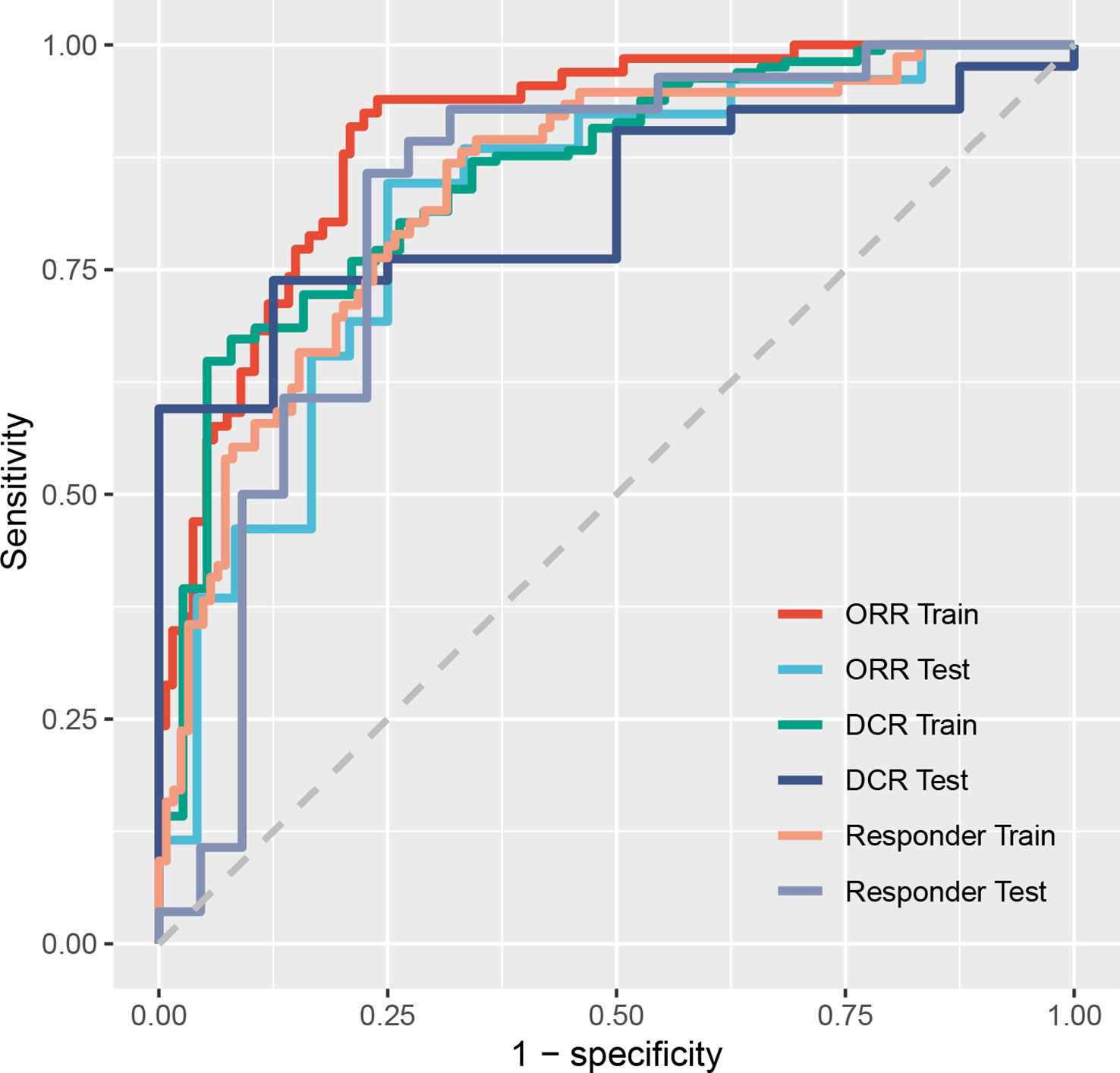
Figure 3 Receiver operating characteristic curves of models on predicting ORR, DCR, and responder. ORR, objective response rate; DCR, disease control rate; Responder, progression-free survival time was more than 6 months.
3.3 Predictive tool
Immunotherapy efficacy predictive models for LUAD based on the neural network were packaged into a predictive tool. As Figure 4 shows, after related clinical information is inputted, models will calculate and report this patient’s possibility of ORR, DCR, and responder. The tool can also predict this patient’s OS after immunotherapy when the Predict button is clicked.
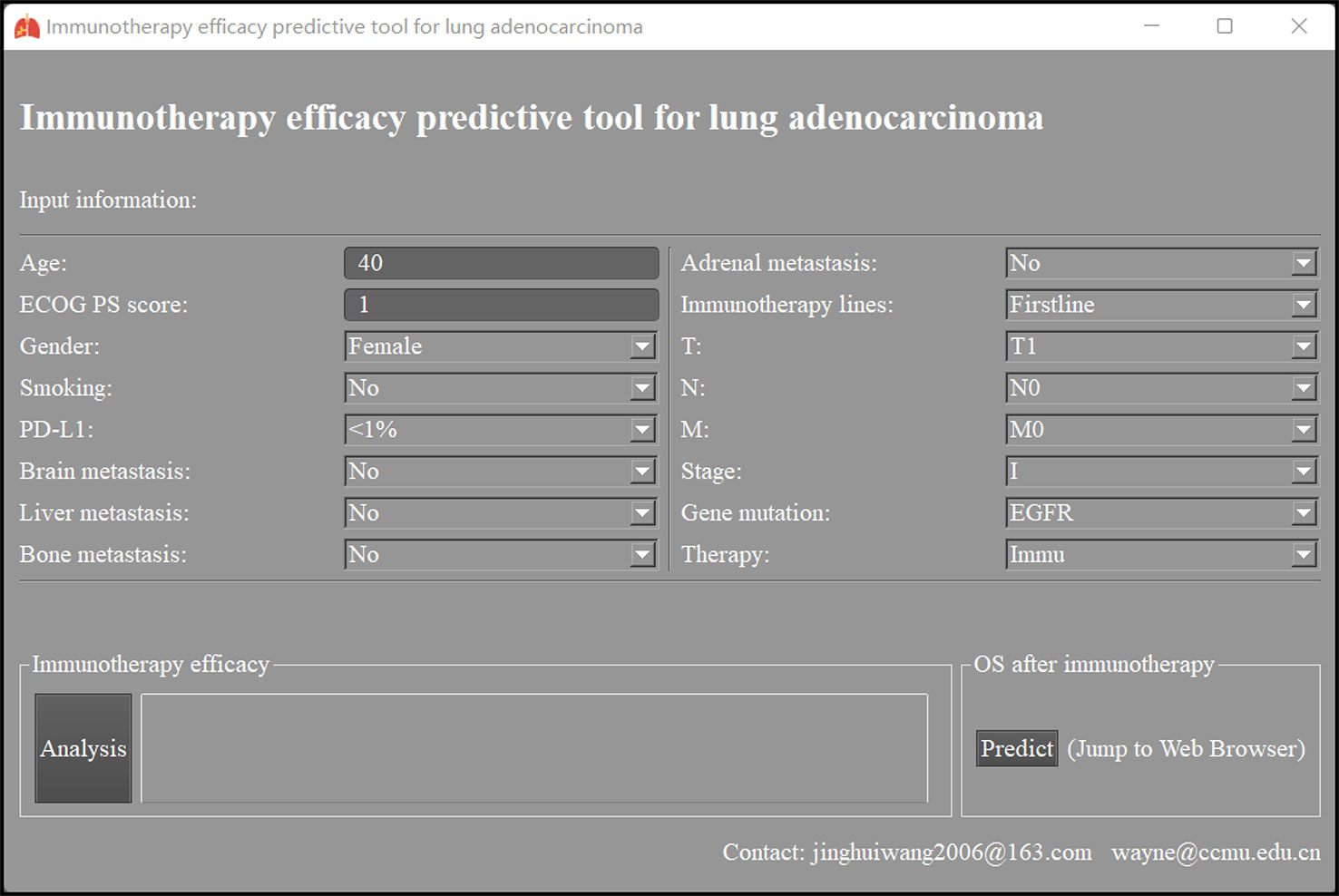
Figure 4 The interface of immunotherapy efficacy predictive tool for lung adenocarcinoma based on neural network. ECOG PS score, Eastern Cooperative Oncology Group performance status score; PD-L1, programmed cell death ligand 1; Immu, immunotherapy; OS, overall survival.
3.4 Survival analysis
In our clinical practice, the majority of LUAD patients receiving immunotherapy were primarily advanced ones (stage III–IV). Therefore, advanced LUAD patients’ data were utilized to orient risk and protective factors on their OS after missing values were omitted. As Supplement Figure 2 illustrates, patients with bone metastasis had shorter survival, while patients with PR and SD had better prognoses than those with PD.
4 Discussion
In recent years, numerous clinical trials have strongly supported immunotherapy for routine clinical use (7–11). According to KEYNOTE-024, pembrolizumab has shown a durable, long-term survival benefit with a 5-year OS rate of 31.9%, further providing meaningful evidence of immunotherapy’s clinical application (17). Currently, whether monotherapy or combination with chemotherapy plus antiangiogenic therapy or not, immunotherapy has been commonly used in clinical practice as the first-line or subsequent treatment for LUAD patients without targetable mutations.
With the widely applied and remarkable achievement of immunotherapy in LUAD, there is an increasingly great concern for how to accurately select the best candidates (10, 18). The current standards for selection still have certain limitations. First, although PD-L1 expression in tumor tissue has been advocated as a standard for anti-PD1/PD-L1 immunotherapy and moderate concordance has been shown by previous studies in PD-L1 assay results, the results are still influenced by multiple factors such as intra-tumoral heterogeneity of PD-L1 expression, cellularity, and more three-dimensional cell clusters in cytology samples (19). Second, researchers were committed to discovering new biomarkers, such as tumor mutational burden (TMB). TMB, which is defined as the total number of non-synonymous somatic mutations in tumor cells, has been found to be related to tumor antigenicity, and might be an independent biomarker of immunotherapy outcome (20). Patients with high TMB and receiving immunotherapy were significantly correlated with longer PFS and higher ORR, compared to those receiving traditional chemotherapy (21, 22). Based on KEYNOTE-158 (NCT02628067), TMB was approved as a reference index for the treatment of solid tumors using pembrolizumab (23). However, TMB has not been widely endorsed because of its inability to predict OS and its inconsistent predictive efficacy in all cancer types (21, 24). Furthermore, the accuracy of TMB evaluation could be affected by different methods of specimen handling (25). Third, researchers began to use more sophisticated and better algorithms to handle medical issues, like predicting immunotherapy efficacy. The combination of chemotherapy and immunotherapy is more popular because of a better outcome, but still, there is a lack of predictive markers. Predictive tools or models based on artificial intelligence have been increasingly emerging in recent years. T. Araujo and colleagues used a convolutional neural network to diagnose breast cancer based on hematoxylin and eosin-stained breast biopsy images, and the model achieved 83.3% accuracy and 95.6% sensitivity (26). C. Du and colleagues applied a neural network to classify imbalanced electrocardiosignal data, achieving 98.45% accuracy and 97.03% sensitivity (27). T. Dratsch developed and validated a neural network to identify the 30 most common categories of plain radiographs, and the model showed 90.3% overall accuracy (28). Deep learning is gradually recognized by clinicians. Therefore, we chose a neural network to predict LUAD patients’ immunotherapy efficacy.
In this study, we aimed to develop a predictive tool to help identify the ideal candidates for immunotherapy in LUAD, which was packaged into a Windows file later and convenient to use. We collected the clinical features of 250 patients included, dividing them into the training dataset and the test dataset randomly. Then, we utilized the training dataset to conduct models and validated them with both the training and test datasets. The predictive performance of our tool is satisfactory, with AUC > 0.8 on most evaluated items (ORR, DCR, and responder possibility), except OS. Notably, our model scored 0.9016 and 0.8173 AUC in ORR classification, 0.8570 and 0.8244 in DCR prediction, and 0.8395 and 0.8214 in responder identification. These showed that our tool had good efficacy in predicting LUAD patients’ immunotherapy benefits.
More and more researchers have devoted themselves to developing a model to predict the benefit of immunotherapy for cancer patients. Some investigators focused on building a model on the database of gene mutation. Jie Peng et al. developed a predictive model associated with the durable clinical benefit of ICIs to LUAD patients, using deep neural networks based on somatic mutations tested by whole-exome sequencing and targeted next-generation sequencing (29). The model exhibited 0.884 AUC in the training set and 0.914 AUC in the two validation sets. Regretfully, the authors did not make the neural network available as a clinician-friendly website or software. Meanwhile, other researchers were dedicated to the information on the tumor microenvironment. Jinteng Feng et al. constructed a nomogram associated with CD8+ T cells to predict survival rates and immunotherapy benefits of stage III LUAD patients (30). A moderate performance showed in an online database that their prediction model had approximately 0.649–0.709 AUC, but a survival difference between the two groups (high- and low-risk groups) was not observed (30). A similar study used the next-generation sequencing method mainly; these predictive models might be limited to being widely applied for lack of gene sequencing data, which might be too expensive for most patients. Compared to those studies, our study provided novel insights into predicting the clinical benefit of ICIs for LUAD patients. We only used patients’ demographic features and routine testing to act as predictive variables, without any additional or expensive examination. Moreover, to facilitate clinicians’ use, we have packaged the model into available Windows software.
In spite of the advantages, our tool still had some limitations. Regrettably, the efficacy of our tool to predict the patients’ OS remained unsatisfactory. Increasing the sample size may improve the predictive efficacy of our tool. Moreover, it is better to use prospective clinical data to validate our tool again.
In summary, we developed a neural network model to predict immunotherapy efficacy for LUAD patients using general clinical features and packaged it into Windows software. This tool showed satisfactory performance and has been uploaded in Supplement File 1, which might help optimize immunotherapy management for LUAD patients. More biomarkers may further enhance the model’s predictive accuracy.
Data availability statement
The original contributions presented in the study are included in the article/Supplementary Materials. Further inquiries can be directed to the corresponding authors.
Ethics statement
This research has been approved by the Ethics Committee of Beijing Chest Hospital affiliated with Capital Medical University.
Author contributions
JW, WYL, WL, SF, and XG came up with the idea for the study. SF, XG, ZL, RJ, NQ, XZ, and YW collected the data and conducted the follow-up. WL carried out the statistical analysis. WL, SF, and XG wrote the manuscript. JW and WYL edited and reviewed the manuscript. All authors discussed the results and commented on the manuscript. All the authors contributed to the article and approved the submitted version.
Conflict of interest
The authors declare that the research was conducted in the absence of any commercial or financial relationships that could be construed as a potential conflict of interest.
Publisher’s note
All claims expressed in this article are solely those of the authors and do not necessarily represent those of their affiliated organizations, or those of the publisher, the editors and the reviewers. Any product that may be evaluated in this article, or claim that may be made by its manufacturer, is not guaranteed or endorsed by the publisher.
Supplementary material
The Supplementary Material for this article can be found online at: https://www.frontiersin.org/articles/10.3389/fimmu.2023.1141408/full#supplementary-material
Supplementary Figure 1 | The distribution of missing values. ECOG PS score, Eastern Cooperative Oncology Group performance status score. PD-L1, programmed cell death ligand 1. BOR, best of response. PFS, progression free survival. OS, overall survival.
Supplementary Figure 2 | Risk factors and protective factors on overall survival in III-IV stage lung adenocarcinoma patients after immunotherapy. PS score, performance status score. PD-L1, programmed death-ligand 1. Other mutation, TP53. Uncommon mutation, ALK, ROS1, RET, MET, BRAF, HER2. Immu, immunotherapy. Antiangio, antiangiogenic. Chemo, chemotherapy. BOR, best of response. PD, progressive disease. PR, partial response. SD, stable disease. *P<0.05, **P<0.01, ***P<0.001.
Supplement File 1 | The installation program of immunotherapy efficacy predictive tool.
Supplement File 2 | The raw clinical data.
Abbreviations
LUAD, lung adenocarcinoma; PD-1, programmed cell death 1; PD-L1, programmed cell death ligand 1; IHC, immunohistochemistry; ICIs, immune checkpoint inhibitors; RECIST, Response Evaluation Criteria in Solid Tumors; ECOG, Eastern Cooperative Oncology Group; PS score, performance status score; AJCC, American Joint Committee on Cancer; Immu, immunotherapy; Antiangio, antiangiogenic; Chemo, chemotherapy; ORR, objective response rate; BOR, best of response; CR, complete response; PR, partial response; SD, stable disease; PD, progressive disease; DCR, disease control rate; OS, overall survival; PFS, progression-free survival; DNNs, dense neural networks; ROC, receiver operating characteristic; AUC, area under the receiver operating characteristic curve; IQR, interquartile range; CI, confidence interval; TMB, tumor mutational burden.
References
1. Nasim F, Sabath BF, Eapen GA. Lung cancer. Med Clin North Am (2019) 103(3):463–73. doi: 10.1016/j.mcna.2018.12.006
2. Sung H, Ferlay J, Siegel RL, Laversanne M, Soerjomataram I, Jemal A, et al. Global cancer statistics 2020: Globocan estimates of incidence and mortality worldwide for 36 cancers in 185 countries. CA Cancer J Clin (2021) 71(3):209–49. doi: 10.3322/caac.21660
3. Zappa C, Mousa SA. Non-small cell lung cancer: Current treatment and future advances. Transl Lung Cancer Res (2016) 5(3):288–300. doi: 10.21037/tlcr.2016.06.07
4. Cardona AF, Ruiz-Patino A, Arrieta O, Ricaurte L, Zatarain-Barron ZL, Rodriguez J, et al. Genotyping squamous cell lung carcinoma in Colombia (Geno1.1-clicap). Front Oncol (2020) 10:588932. doi: 10.3389/fonc.2020.588932
5. Martinez-Jimenez F, Muinos F, Sentis I, Deu-Pons J, Reyes-Salazar I, Arnedo-Pac C, et al. A compendium of mutational cancer driver genes. Nat Rev Cancer (2020) 20(10):555–72. doi: 10.1038/s41568-020-0290-x
6. Herbst RS, Morgensztern D, Boshoff C. The biology and management of non-small cell lung cancer. Nature (2018) 553(7689):446–54. doi: 10.1038/nature25183
7. Borghaei H, Paz-Ares L, Horn L, Spigel DR, Steins M, Ready NE, et al. Nivolumab versus docetaxel in advanced nonsquamous non-Small-Cell lung cancer. N Engl J Med (2015) 373(17):1627–39. doi: 10.1056/NEJMoa1507643
8. Reck M, Rodriguez-Abreu D, Robinson AG, Hui R, Csoszi T, Fulop A, et al. Pembrolizumab versus chemotherapy for pd-L1-Positive non-Small-Cell lung cancer. N Engl J Med (2016) 375(19):1823–33. doi: 10.1056/NEJMoa1606774
9. Gandhi L, Rodriguez-Abreu D, Gadgeel S, Esteban E, Felip E, De Angelis F, et al. Pembrolizumab plus chemotherapy in metastatic non-Small-Cell lung cancer. N Engl J Med (2018) 378(22):2078–92. doi: 10.1056/NEJMoa1801005
10. Socinski MA, Jotte RM, Cappuzzo F, Orlandi F, Stroyakovskiy D, Nogami N, et al. Atezolizumab for first-line treatment of metastatic nonsquamous nsclc. N Engl J Med (2018) 378(24):2288–301. doi: 10.1056/NEJMoa1716948
11. Antonia SJ, Villegas A, Daniel D, Vicente D, Murakami S, Hui R, et al. Durvalumab after chemoradiotherapy in stage iii non-Small-Cell lung cancer. N Engl J Med (2017) 377(20):1919–29. doi: 10.1056/NEJMoa1709937
12. Liu B, Song Y, Liu D. Recent development in clinical applications of pd-1 and pd-L1 antibodies for cancer immunotherapy. J Hematol Oncol (2017) 10(1):174. doi: 10.1186/s13045-017-0541-9
13. Schoenfeld AJ, Rizvi H, Bandlamudi C, Sauter JL, Travis WD, Rekhtman N, et al. Clinical and molecular correlates of pd-L1 expression in patients with lung adenocarcinomas. Ann Oncol (2020) 31(5):599–608. doi: 10.1016/j.annonc.2020.01.065
14. Van Buuren S, Groothuis-Oudshoorn K. Mice: Multivariate imputation by chained equations in r. J Stat Softw (2011) 45:1–67. doi: 10.18637/jss.v045.i03
15. Katzman JL, Shaham U, Cloninger A, Bates J, Jiang T, Kluger Y. Deepsurv: Personalized treatment recommender system using a cox proportional hazards deep neural network. BMC Med Res Methodol (2018) 18(1):24. doi: 10.1186/s12874-018-0482-1
16. Nahm FS. Receiver operating characteristic curve: Overview and practical use for clinicians. Korean J Anesthesiol (2022) 75(1):25–36. doi: 10.4097/kja.21209
17. Reck M, Rodriguez-Abreu D, Robinson AG, Hui R, Csoszi T, Fulop A, et al. Five-year outcomes with pembrolizumab versus chemotherapy for metastatic non-Small-Cell lung cancer with pd-L1 tumor proportion score >/= 50. J Clin Oncol (2021) 39(21):2339–49. doi: 10.1200/JCO.21.00174
18. Borghaei H, Gettinger S, Vokes EE, Chow LQM, Burgio MA, de Castro Carpeno J, et al. Five-year outcomes from the randomized, phase iii trials checkmate 017 and 057: Nivolumab versus docetaxel in previously treated non-Small-Cell lung cancer. J Clin Oncol (2021) 39(7):723–33. doi: 10.1200/JCO.20.01605
19. Satturwar S, Girolami I, Munari E, Ciompi F, Eccher A, Pantanowitz L. Program death ligand-1 immunocytochemistry in lung cancer cytological samples: A systematic review. Diagn Cytopathol (2022) 50(6):313–23. doi: 10.1002/dc.24955
20. Yarchoan M, Albacker LA, Hopkins AC, Montesion M, Murugesan K, Vithayathil TT, et al. Pd-L1 expression and tumor mutational burden are independent biomarkers in most cancers. JCI Insight (2019) 4(6):e126908. doi: 10.1172/jci.insight.126908
21. Carbone DP, Reck M, Paz-Ares L, Creelan B, Horn L, Steins M, et al. First-line nivolumab in stage iv or recurrent non-Small-Cell lung cancer. N Engl J Med (2017) 376(25):2415–26. doi: 10.1056/NEJMoa1613493
22. Hellmann MD, Ciuleanu TE, Pluzanski A, Lee JS, Otterson GA, Audigier-Valette C, et al. Nivolumab plus ipilimumab in lung cancer with a high tumor mutational burden. N Engl J Med (2018) 378(22):2093–104. doi: 10.1056/NEJMoa1801946
23. Marabelle A, Fakih M, Lopez J, Shah M, Shapira-Frommer R, Nakagawa K, et al. Association of tumour mutational burden with outcomes in patients with advanced solid tumours treated with pembrolizumab: Prospective biomarker analysis of the multicohort, open-label, phase 2 keynote-158 study. Lancet Oncol (2020) 21(10):1353–65. doi: 10.1016/S1470-2045(20)30445-9
24. McGrail DJ, Pilie PG, Rashid NU, Voorwerk L, Slagter M, Kok M, et al. High tumor mutation burden fails to predict immune checkpoint blockade response across all cancer types. Ann Oncol (2021) 32(5):661–72. doi: 10.1016/j.annonc.2021.02.006
25. Pepe F, Pisapia P, Gristina V, Rocco D, Micheli M, Micheli P, et al. Tumor mutational burden on cytological samples: A pilot study. Cancer Cytopathol (2021) 129(6):460–7. doi: 10.1002/cncy.22400
26. Araujo T, Aresta G, Castro E, Rouco J, Aguiar P, Eloy C, et al. Classification of breast cancer histology images using convolutional neural networks. PloS One (2017) 12(6):e0177544. doi: 10.1371/journal.pone.0177544
27. Du C, Liu PX, Zheng M. Classification of imbalanced electrocardiosignal data using convolutional neural network. Comput Methods Programs BioMed (2022) 214:106483. doi: 10.1016/j.cmpb.2021.106483
28. Dratsch T, Korenkov M, Zopfs D, Brodehl S, Baessler B, Giese D, et al. Practical applications of deep learning: Classifying the most common categories of plain radiographs in a pacs using a neural network. Eur Radiol (2021) 31(4):1812–8. doi: 10.1007/s00330-020-07241-6
29. Peng J, Zou D, Gong W, Kang S, Han L. Deep neural network classification based on somatic mutations potentially predicts clinical benefit of immune checkpoint blockade in lung adenocarcinoma. Oncoimmunology (2020) 9(1):1734156. doi: 10.1080/2162402X.2020.1734156
Keywords: immunotherapy, lung adenocarcinoma, neural network, deep learning, predictive model
Citation: Li W, Fu S, Gao X, Lu Z, Jin R, Qin N, Zhang X, Wu Y, Li W and Wang J (2023) Immunotherapy efficacy predictive tool for lung adenocarcinoma based on neural network. Front. Immunol. 14:1141408. doi: 10.3389/fimmu.2023.1141408
Received: 10 January 2023; Accepted: 15 March 2023;
Published: 28 March 2023.
Edited by:
Yutian Zou, Sun Yat-sen University Cancer Center (SYSUCC), ChinaReviewed by:
Albino Eccher, Integrated University Hospital Verona, ItalyWalid Shalata, Soroka Medical Center, Israel
Copyright © 2023 Li, Fu, Gao, Lu, Jin, Qin, Zhang, Wu, Li and Wang. This is an open-access article distributed under the terms of the Creative Commons Attribution License (CC BY). The use, distribution or reproduction in other forums is permitted, provided the original author(s) and the copyright owner(s) are credited and that the original publication in this journal is cited, in accordance with accepted academic practice. No use, distribution or reproduction is permitted which does not comply with these terms.
*Correspondence: Jinghui Wang, amluZ2h1aXdhbmcyMDA2QDE2My5jb20=; Weiying Li, bGlfd2VpeWluZzQxMkBhbGl5dW4uY29t
†These authors have contributed equally to this work and share the first authorship
‡ORCID: Weiying Li, orcid.org/0000-0003-1507-5104
Jinghui Wang, orcid.org/0000-0002-4999-2106