- 1School of Medicine, Tehran University of Medical Sciences, Tehran, Iran
- 2Non–Communicable Diseases Research Center, Endocrinology and Metabolism Population Sciences Institute, Tehran University of Medical Sciences, Tehran, Iran
- 3Robert Stempel College of Public Health & Social Work, Florida International University, Miami, FL, United States
- 4Department of Translational Medical Sciences, Federico II University of Naples, Naples, Italy
- 5Department of Translational Medicine, Translational Glycobiology Institute at Florida International University, Herbert Wertheim College of Medicine, Florida International University, Miami, FL, United States
Background: Galectins are an eleven-member class of lectins in humans that function as immune response mediators and aberrancies in their expression are commonly associated with immunological diseases. Several studies have focused on galectins as they may represent an important biomarker and a therapeutic target in the fight against COVID-19. This systematic review and meta-analysis examined the usefulness of clinical assessment of circulating galectin levels in patients with COVID-19.
Methods: International databases including PubMed, Scopus, Web of Science, and Embase were systematically used as data sources for our analyses. The random-effect model was implemented to calculate the standardized mean difference (SMD) and a 95% confidence interval (CI).
Results: A total of 18 studies, comprising 2,765 individuals, were identified and used in our analyses. We found that Gal-3 is the most widely investigated galectin in COVID-19. Three studies reported significantly higher Gal-1 levels in COVID-19 patients. Meta-analysis revealed that patients with COVID-19 had statistically higher levels of Gal-3 compared with healthy controls (SMD 0.53, 95% CI 0.10 to 0.96, P=0.02). However, there was no significant difference between severe and non-severe cases (SMD 0.45, 95% CI -0.17 to 1.07, P=0.15). While one study supports lower levels of Gal-8 in COVID-19, Gal-9 was measured to be higher in patients and more severe cases.
Conclusion: Our study supports Gal-3 as a valuable non-invasive biomarker for the diagnosis and/or prognosis of COVID-19. Moreover, based on the evidence provided here, more studies are needed to confirm a similar diagnostic and prognostic role for Gal-1, -8, and -9.
1 Introduction
In December 2019, the coronavirus (CoV) disease of 2019 (COVID-19) first appeared in Wuhan, Hubei Province (1). There are major diagnostic and assessment challenges associated with the COVID-19 pandemic brought on by severe acute respiratory syndrome (SARS) CoV-2 (SARS-CoV2) as the pathogen responsible for the disease. These include figuring out the importance of signs and symptoms in predicting potential infections, determining whether current biochemical and laboratory tests can detect infections and identify patients needing immediate medical attention, and seeing if new diagnostic tests can enable precise rapid and point-of-care testing (2).
In this regard, biomarkers play a crucial role in the clinical decision since they can anticipate the COVID-19 severity and track its prognosis (3). For this reason, in the last few years, several biomarkers have been investigated and identified. For instance, studies have demonstrated that in patients with a higher severity level of COVID-19 pneumonia, increased serum levels of C-reactive protein, interleukin-10, and interleukin-6/Interferon-γ ratio were frequently found (4–6). In addition, others reported that blood levels of D-dimer, lactate dehydrogenase, and white blood cell count played a role in predicting adverse outcomes in the severe COVID-19 (7).
Galectins are a group of lectins with a phylogenetically conserved structure that share approximately 130 amino acids that make up the consensus amino acid sequence, and the carbohydrate recognition domain is in charge of binding β-galactoside (8, 9). Galectin family members have been identified in circulating cells, main and secondary lymphoid tissues, and other organs (10). To date, fifteen mammalian galectins have been discovered and only eleven are found in humans (11). Among these, galectin (Gal)-1, -3, -8, and -9 have been well-described as key immune response mediators. While Gal-1 generally exhibits suppressive activities on the immune system, Gal-3 and -9 have been shown to suppress or amplify inflammatory responses (12). Likewise, Gal-8 has also been suggested to have disparate roles in immunity, functioning as an enhancer of the expression and secretion of many pro-inflammatory cytokines or as a cytokine-binding partner to sequester and hinder cytokine activity (12). For this reason, galectins have been linked to a variety of inflammatory-driven disorders (e.g., cancer, cardiovascular, and metabolic diseases) (11–16). Moreover, since these molecules are also involved in pro-fibrotic processes (13), their role is particularly relevant because they are involved in the pathogenesis of several disorders affecting several organs including the heart and lungs (16–18).
Based on this evidence, recent studies suggest the clinical utility of evaluating Gal-1, -3, -8, and -9 circulating levels as novel potential diagnostic and prognostic biomarkers of COVID-19 either alone or in conjunction with other known biomarkers (19–22). Hence, here we conducted a systematic review and a meta-analysis to combine the findings from previous studies assessing Gal-1, -3, -8, and -9 as putative, non-invasive biomarkers of COVID-19 severity and COVID-19-related complications (e.g., cardiovascular). The conclusions obtained by this study can pave the way for future research on these biomarkers and further enlighten their role in clinical settings.
2 Methods
2.1 Search strategy
International online databases including PubMed, SCOPUS, Web of Science, and SCOPUS were searched from inception to October 2022 without any filters or limitations. Search queries consisted of keywords related to COVID-19 and galectins. Details of the search strategy are depicted in Supplementary Table 1.
2.2 Inclusion and exclusion criteria
Inclusion criteria were clinical studies that (1): compared galectin levels between COVID-19 patients and healthy controls (2), compared galectin levels between different severities of COVID-19 (3), compared galectin levels between patients with comorbidity and without comorbidity related to COVID-19, or (4) used galectin levels to discriminate COVID-19 patients from healthy controls or differentiate different severities of COVID-19. We excluded animal studies, case reports, reviews, and conference abstracts. Finally, non-English abstracts were also excluded.
2.3 Screening, data extraction, and quality assessment
Required data were extracted by two reviewers (AHB and SYA) in a pre-designed sheet by the third reviewer (AKh). We extracted these for each study (1): study characteristics including publication year, locations(s), and first author’s name (2), population characteristics including total population number, mean age, and female percentage, and (3) main findings.
The Newcastle-Ottawa Scale (NOS) for non-randomized studies was used to assess the risk of bias in included studies (23). NOS evaluates studies according to pre-specified items including selection, comparability, and exposure. Studies with a total NOS ≥ 8 are considered high quality.
2.4 Statistical analysis and data synthesis
Data synthesis was performed from the mean and standard deviations (SDs) of galectin levels in COVID-19 cases or different severities of the disease. As there were sufficient studies for Gal-3, we conducted meta-analyses for comparison of this biomarker between COVID-19 cases vs controls, and severe vs. non-severe forms of the disease. Random-effect meta-analysis was performed to calculate the pooled effect size using standardized mean difference (SMD) and 95% confidence interval (CI). To find the source of heterogeneity, meta-regression was conducted with variables including sample size, mean age, and female percentage. The associated bubble plots were also shown. Moreover, publication bias was evaluated using the visual symmetry of funnel plots and statistical tests of Begg’s and Egger’s (24, 25). Finally, to find the possible outliers of analyses, the Galbraith plots were designed, and sensitivity analysis was performed with the removal of each study in analyses.
3 Results
3.1 Literature search and baseline characteristics of included studies
Our search resulted in 841 records: 197 from PubMed, 168 from SCOPUS, 104 from Web of Science, and 372 from Embase. After removing duplicates, 524 records were screened by titles and abstracts. Following this step, 63 studies were evaluated by full texts, resulting in 18 final included studies (7, 19–22, 26–38). The PRISMA (Preferred Reporting Items for Systematic reviews and Meta-Analyses) flow chart summarizing the selection process is available in Figure 1.
A total of 2,765 individuals were included between 2020 and 2022. Gal-1 (20, 22, 35), Gal-3 (7, 20–22, 27, 29–34, 36–38), Gal-8 (19), and Gal-9 (19–21, 26, 28) were evaluated in three, 14, one, and five studies, respectively. Twelve studies evaluated serum galectin levels (7, 22, 27, 29–37), while six others evaluated galectins in plasma (19–21, 26, 28, 38). Table 1 summarizes baseline characteristics and main findings of included studies. All studies had high quality based on NOS (Supplementary Table 2).
3.2 Gal-1 and COVID-19
3.2.1 Gal-1 levels comparison between COVID-19 patients and controls
The association between Gal-1 levels and COVID-19 was evaluated by three studies (20, 22, 35). In a case-control study by De Biasi et al. (20), Gal-1 levels were compared between 39 COVID-19 patients and 25 age- and sex-matched healthy controls. They found significantly higher levels of Gal-1 in COVID-19 patients compared to healthy controls. In line with this study, Kazancioglu et al. (22) found higher serum levels of Gal-1 in COVID-19 patients compared to healthy controls (P < 0.001).
3.2.2 Gal-1 levels in different severities of COVID-19
The study by Kazancioglu et al. (22) found no significant difference between severe (n = 29) and non-severe (n = 55) COVID-19 patients (median = 9.86 vs. 6.35 ng/mL). In contrast, Markovic et al. (35) found higher levels in stage III of COVID-19 compared to stages I and II (P = 0.001). Detection of stage III COVID-19 was possible with a cut-off of 9359 pg/mL (specificity: 25.9% and sensitivity: 72.2%). In addition, stage II was also associated with higher levels of Gal-1 compared to stage I (P < 0.05) and detection of stage II COVID-19 was possible with a cut-off of 3959 pg/mL (specificity: 23.4% and sensitivity: 89.5%).
3.2.3 Gal-1 levels and COVID-19 complications
Markovic et al. (35) was the only study that investigated Gal-1 levels with COVID-19 complications. The correlation between Gal-1 levels and dry cough (r = 0.242; P = 0.001), headache (r = 0.158; P = 0.034), and chest radiographic findings (r = 0.352; P = 0.001) was positive. However, a negative correlation between normal breathing sound and Gal-1 levels was also seen (r = 0.151; P = 0.043).
3.3 Gal-3 and COVID-19
3.3.1 Meta-analysis of Gal-3 levels in COVID-19 vs. controls
Random-effects meta-analysis of seven studies, comprised of 1,123 cases (728 COVID-19 and 395 controls), showed that Gal-3 levels were significantly higher in COVID-19 cases compared to healthy controls (SMD 0.53, 95% CI 0.10 to 0.96, P = 0.02, Figure 2). This was associated with high heterogeneity (I2 = 89.55%). The outliers for this analysis were recognized using the Galbraith plot, which is shown in Supplementary Figure 1. After removing Karsli et al. (33), no change was observed in the significance of the results (SMD 0.69, 95% CI 0.44 to 0.94, P < 0.01, Figure 3), although heterogeneity was reduced to 60.42%. Removing five of the studies made the overall result insignificant (21, 22, 27, 31, 32).
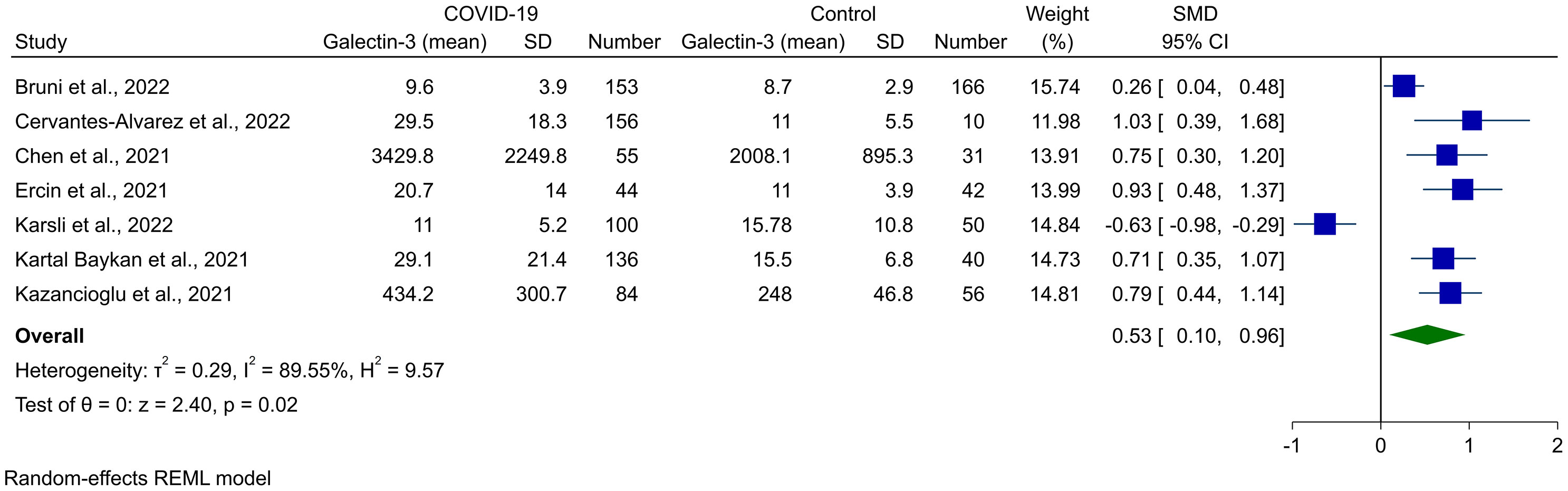
Figure 2 Forest plot for the meta-analysis of Gal-3 levels in COVID-19 patients vs. healthy controls.
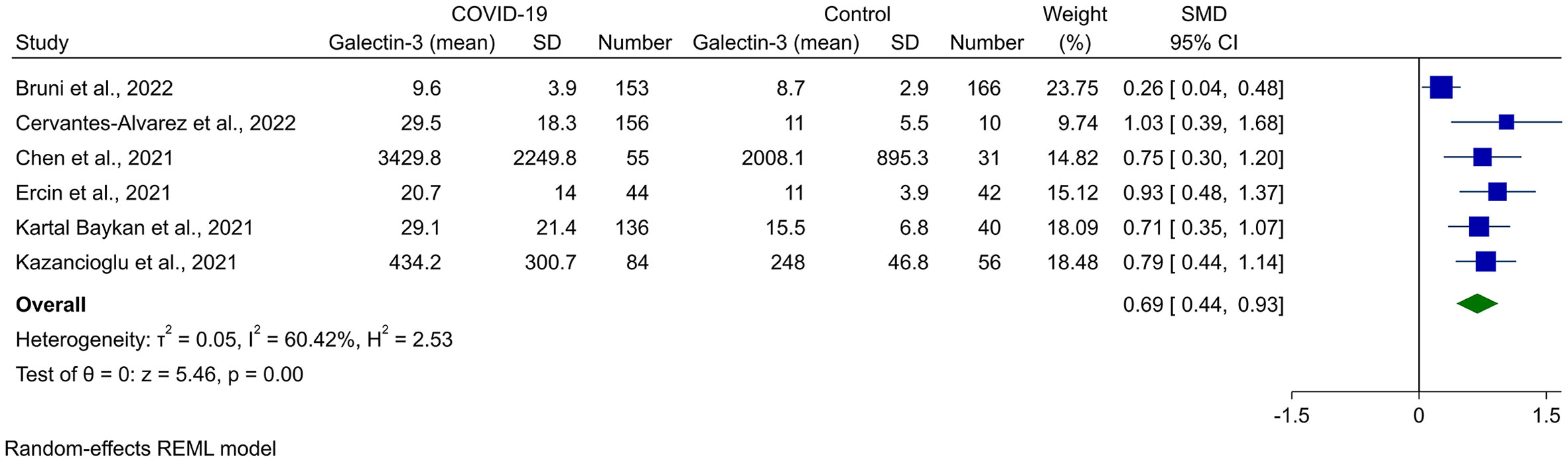
Figure 3 Forest plot for the meta-analysis of Gal-3 levels in COVID-19 patients vs. healthy controls after removing the outlier study (33).
Meta-regression was performed to find the source of heterogeneity for this analysis, and it was shown that mean age had a significant association with the result observed (P = 0.041). In addition, mean age contributed to the 44.05% of the heterogeneity observed. Sample size and female percentage did not have any association with the Gal-3 levels in COVID-19 cases (Table 2). The bubble plots for meta-regression based on sample size, mean age, and female percentage are shown in Supplementary Figures 2–4.
The funnel plot for the assessment of publication bias is shown in Supplementary Figure 5, which showed apparent asymmetry and introduced the possibility of publication bias. However, Begg’s and Egger’s statistical tests did not show any significant publication bias (P = 0.367 and P = 0.186, respectively).
3.3.2 Meta-analysis of Gal-3 levels in Severe vs. non-severe COVID-19
Five studies reported Gal-3 blood concentrations in severe COVID-19 cases and compared it with non-severe patients. Meta-analysis for these levels showed a tendency toward higher levels of Gal-3 in severe instances of COVID-19; however, this was not significant (SMD 0.45, 95% CI -0.17 to 1.07, P = 0.15, Figure 4). High heterogeneity was observed for this analysis as well (I2 = 91.63%). The Galbraith plot did not reveal any outlier study for this analysis (Supplementary Figure 6). However, in sensitivity analysis, removing Karsli et al. made the overall result significant (33).
In performing meta-regression for this meta-analysis, the sample size was found to have a direct association with the pooled effect size (P = 0.041). In addition, sample size and female percentage accounted for 48.90% and 3.53% of the observed heterogeneity, respectively (Table 2, bubble plots in Supplementary Figures 7–9).
Publication bias investigation based on the funnel plot inspection did not show any sign of bias (Supplementary Figure 10). In line, Begg’s and Egger’s statistical tests were not significant for publication bias (P = 0.227 and P = 0.178, respectively).
3.3.3 Gal-3 levels’ association with COVID-19 complications
Intensive care unit (ICU) admission, need for ventilation, and death from COVID-19 had been assessed in the literature in association with Gal-3 levels. In a study by Kusnierz-Cabala et al. (34), the Gal-3 level was significantly higher in patients needing ICU treatment than those without it (4.77 [2.92-8.17] ng/ml vs. 2.30 [1.88-3.21] ng/ml, P = 0.001). Bruni et al. (29) study defined a composite endpoint of admission to ICU or 30-day mortality and found that COVID-19 cases with a composite endpoint had significantly higher levels of Gal-3 (12.1 [10.3-15.9] ng/ml vs. 8.1[6.5-11.3] ng/ml, P < 0.001). The outcome of death was the case investigated in studies by Portacci et al. (7) and Tawiah et al. (38). Portacci et al. reported median Gal-3 as 43.8 [36.2-59] ng/ml in dead patients, which was significantly higher than the ones with 30-day survival (21.9 [17.6-27.5] ng/ml, P < 0.001). In line, Tawiah et al. also measured higher Gal-3 levels in dead patients within 30 days of emergency department presentation. In a study conducted by Cannavo et al. (30), despite the observed higher mean value of Gal-3 serum levels in COVID-19 patients presenting cardiac function deterioration compared to those without cardiac complications, this difference was not statistically significant (Gal-3 levels between patients showing or non-showing cardiac complications: 4.7 ± 2.7 ng/mL vs. 3.5 ± 1.6 ng/mL, P = 0.35).
3.3.4 Diagnostic and prognostic use of Gal-3
With an area under the curve (AUC) of 0.622, Karsli et al. (33) reported Gal-3 as a potential biomarker for the diagnosis of COVID-19. Prediction of ICU admission based on Gal-3 showed an AUC of 0.7 in the Portacci et al. study (7), and 0.802 in Ozcan et al. study (36). Moreover, the threshold of 15.51 ng/ml resulted in a sensitivity of 78% and specificity of 90% in the prediction of ICU admission, according to Kusnierz-Cabala et al. (34). The prediction of in-hospital mortality reported an AUC of 0.843 with sensitivity and specificity of 75% and 76.8%, respectively (36). The 30-day mortality prediction showed an AUC of 0.906 and 0.681 in studies by Portacci et al. (7) and Tawiah et al. (38). A study conducted by Rodríguez-Tomàs et al. (37) reported an AUC of 0.709 in differentiating COVID-19 patients from controls.
3.4 Gal-8 and COVID-19
Only one study has investigated Gal-8 levels in different COVID-19 severities (19). All severities of COVID-19 had significantly lower levels of Gal-8 in comparison with healthy controls, representing 8.6 (6.87-9.84), 5.49 (4.7-6.53), and 6.38 (5.65-7.04) for mild, severe, and critical COVID-19, respectively, in addition to 6.6 (6.12-7.86) for healthy controls.
3.5 Gal-9 and COVID-19
Five studies investigated the association between Gal-9 and COVID-19. Bai et al. (26) reported significantly higher levels of full-length Gal-9 in COVID-19 associated with pneumonia than in healthy control (P < 0.0001). In line with these results, two other studies reported higher levels of Gal-9 in SARS-CoV-2-infected individuals compared to non-infected ones (20, 28).
Further to these studies, Chen et al. (21) reported that patients with both severe and non-severe forms of COVID-19 had significantly higher levels of Gal-9 than controls (2044 [1385-3303] pg/ml, and 2811 [1866-4371] pg/ml vs. 739 [495-960] pg/ml, respectively).
For their part, Bozorgmehr et al. (28) confirmed the results above, showing an increase in Gal-9 in COVID-19 patients compared to healthy controls. In addition, these authors determined a cutoff of Gal-9 plasmatic concentration of 2,042 pg/ml that allowed the COVID-19 diagnosis with a sensitivity of 94.96% and specificity of 94.92%.
Finally, against this trend, the study from Yaşar et al. failed to find a statistical difference between mild COVID-19 and healthy controls (19). However, these authors found that severe and critical cases of the disease had significantly higher Gal-9 levels than patients with mild symptoms or healthy controls.
4 Discussion
Overall, our study demonstrated that the assessment of variation in circulating Gal-1, -3, -8, and -9 levels in response to SARS-CoV-2 infection could be used as potential diagnostic and prognostic non-invasive biomarkers of COVID-19.
In detail, our systematic review revealed that Gal-1 release is increased in COVID-19 patients compared to controls. However, the data about its relevance to disease severity was contradictory. For instance, in one study, it has been reported no significant difference between severe and non-severe COVID-19 patients (22). In comparison, another study reported a higher level of Gal-1 in critical cases compared to those with severe or mild COVID-19 (35).
Concerning Gal-3, our meta-analysis showed that the circulating levels of Gal-3 were significantly higher in COVID-19 patients than in healthy controls, with no significance between severe and non-severe cases. Moreover, our review support that Gal-3 levels are positively associated with COVID-19 complications. A huge body of literature indicates cytokine release as the main contributing factor to morbidity and mortality of COVID-19 (39). Gal-3 is also correlated with several inflammatory biomarkers, which may be the pathophysiology behind this capability to be a biomarker. Classic inflammatory biomarkers such as C-Reactive Protein (CRP), Ferritin, neutrophil count, and D-dimer have been shown to have associations with disease severity (40, 41); however, as stated by Cervantes-Alvarez et al., there is collinearity between them. In this study, after multivariate analysis, only CRP and Gal-3 were recognized as independent predictors of disease severity (31). Moreover, due to various cut-offs available for CRP, Gal-3 may even be a better candidate for measurement to stratify disease severity. However, it is worth noting that three reports are currently contrasting with this trend. For instance, Bruni et al. (29) stated that COVID-19 patients had insignificant levels of Gal-3 compared to controls. In line with this, Kusnierz-Cabala et al. (34) reported no significant difference in serum Gal-3 levels in COVID-19 patients compared with healthy controls. Meanwhile, Karsli et al. (33) reported that Gal-3 levels are lower in severe COVID-19 patients than in healthy controls.
In addition, despite limited, our systematic review of Gal-8 and -9 showed that lower Gal-8 and higher Gal-9 levels could be observed in COVID-19 patients compared with healthy controls. Concerning the correlation between Gal-8 and -9 to the disease severity, the data available are not conclusive. Indeed, our study showed a tendency toward higher levels in severe cases of Gal-9. Conversely, for Gal-8 the data are very limited as only one study investigated whether the serum level of this factor is differently modulated by COVID-19 severities (19). In this context, Yasar and colleagues reported a reverse trend of Gal-8 levels compared with other galectins. Nevertheless, it is worth noting that recent studies revealed Gal-8 binds exclusively to a specific motif of the viral receptor-binding domain (RBD) of SARS-CoV-2, called 30SLacNAc, while other galectins identify and bind to other additional motifs of the RBD as well (42). However, more studies are required to confirm this different trend seen in Gal-8 and look for possible explanations as well.
Together, these data suggest that further investigation into the clinical relevance and biological role of Gal-8 and -9 is needed. Indeed, understanding the role of these galectins will not only increase their diagnostic and prognostic significance but may also have therapeutic use in the fight against COVID-19 as well as other inflammatory disorders, as per Gal-1 and -3 which are currently considered two of the most critical therapeutic and drug target in various diseases (43). Studies regarding their use in COVID-19 patients showed that inhibition of Gal-3 could modulate the host immune response and reduce cytokine storm syndrome, a major cause of death in COVID-19, alongside preventing viral entry and reducing post-COVID-19 pulmonary fibrosis (44, 45). Also, different clinical trials are currently targeting Gal-3 for treating fibrosis in various diseases, such as idiopathic pulmonary fibrosis and non-alcoholic steatohepatitis-related liver fibrosis (13). In this context, a phase Ib/IIa randomized controlled platform trial is evaluating the potential effects of the Gal-3 inhibitor GB0139 in hospitalized patients with confirmed COVID-19 pneumonitis (46).
5 Limitations
There are some study limitations to note. First, Gal-1, -8, and -9 lacked sufficient original studies to perform a meta-analysis. Secondly, regarding the measurement methods, the studies used various techniques and equipment that consequently resulted in heterogeneity, which despite performing meta-regression, may add limitations. Finally, with the present data, we could not compare the effectiveness of galectins as diagnostic and prognostic tools to each other. Thus, additional studies are needed to compare different galectins not only with each other but also with other known COVID-19 biomarkers.
6 Conclusion
With the emergence of the COVID-19 pandemic, the healthcare systems now face a monumental challenge worldwide to find effective treatments and develop tools for disease diagnosis and monitoring disease progression. To our knowledge, this is the first comprehensive systematic review and meta-analysis evaluating the role of Gal-1, -3, -8, and -9 as potential serum and/or plasma biomarkers for the diagnosis and prognosis of COVID-19. Although we have identified several galectins differentially modulated in response to SARS-CoV-2 infection, our analysis supports a potential prognostic and diagnostic role for Gal-3, in particular, due to its elevated levels in patients compared with healthy controls and its positive correlation with the disease severity. However, additional studies are needed to corroborate our findings and further examine Gal-3’s levels in conjunction with other diagnostics or clinical parameters assessing host immunity, infection history, and other complications associated with SARS-CoV-2 infections. Similarly, further investigation is necessary to shed light on the role of the Gal-1, -8, and -9 that, based on the evidence provided here, may have a similar valuable diagnostic and prognostic role and be at the same time considered a potential therapeutic target, but that remain still poorly studied.
Author contributions
AHB: Writing - original draft/Conceptualization/Formal analysis/Visualization. AKh: Writing - original draft, review and editing/Conceptualization/Supervision. SYA, AKa: Writing - original draft/Data curation. AC, CJD: Writing - review and editing. All authors read and approved the final manuscript.
Funding
This work was supported, in part, by the NIH/NCI Alliance of Glycobiologists for Cancer Research, U01CA225644 (CJ Dimitroff).
Conflict of interest
The authors declare that the research was conducted in the absence of any commercial or financial relationships that could be construed as a potential conflict of interest.
Publisher’s note
All claims expressed in this article are solely those of the authors and do not necessarily represent those of their affiliated organizations, or those of the publisher, the editors and the reviewers. Any product that may be evaluated in this article, or claim that may be made by its manufacturer, is not guaranteed or endorsed by the publisher.
Supplementary material
The Supplementary Material for this article can be found online at: https://www.frontiersin.org/articles/10.3389/fimmu.2023.1127247/full#supplementary-material
References
1. Lu G, Wang J. Dynamic changes in routine blood parameters of a severe COVID-19 case. Clin Chim Acta (2020) 508:98–102. doi: 10.1016/j.cca.2020.04.034
2. Struyf T, Deeks JJ, Dinnes J, Takwoingi Y, Davenport C, Leeflang MM, et al. Signs and symptoms to determine if a patient presenting in primary care or hospital outpatient settings has COVID-19. Cochrane Database Syst Rev (2022) 5(5):Cd013665. doi: 10.1002/14651858.CD013665
3. Malik P, Patel U, Mehta D, Patel N, Kelkar R, Akrmah M, et al. Biomarkers and outcomes of COVID-19 hospitalisations: Systematic review and meta-analysis. BMJ Evid Based Med (2021) 26(3):107–8. doi: 10.1136/bmjebm-2020-111536
4. Ali N. Elevated level of c-reactive protein may be an early marker to predict risk for severity of COVID-19. J Med Virol (2020) 92(11):2409–11. doi: 10.1002/jmv.26097
5. Dhar SK KV, Damodar S, Gujar S, Das M. IL-6 and IL-10 as predictors of disease severity in COVID-19 patients: Results from meta-analysis and regression. Heliyon. (2021) 7(2):e06155. doi: 10.1016/j.heliyon.2021.e06155
6. Lagunas-Rangel FA, Chávez-Valencia V. High IL-6/IFN-γ ratio could be associated with severe disease in COVID-19 patients. J Med Virol (2020) 92(10):1789–90. doi: 10.1002/jmv.25900
7. Portacci A, Diaferia F, Santomasi C, Dragonieri S, Boniello E, Di Serio F, et al. Galectin-3 as prognostic biomarker in patients with COVID-19 acute respiratory failure. Respir Med (2021) 187:106556. doi: 10.1016/j.rmed.2021.106556
8. Leffler H, Carlsson S, Hedlund M, Qian Y, Poirier F. Introduction to galectins. Glycoconj J (2002) 19(7-9):433–40. doi: 10.1023/B:GLYC.0000014072.34840.04
9. Thijssen VL, Heusschen R, Caers J, Griffioen AW. Galectin expression in cancer diagnosis and prognosis: A systematic review. Biochim Biophys Acta (2015) 1855(2):235–47. doi: 10.1016/j.bbcan.2015.03.003
10. Tribulatti MV, Carabelli J, Prato CA, Campetella O. Galectin-8 in the onset of the immune response and inflammation. Glycobiology (2020) 30(3):134–42. doi: 10.1093/glycob/cwz077
11. Chou FC, Chen HY, Kuo CC, Sytwu HK. Role of galectins in tumors and in clinical immunotherapy. Int J Mol Sci (2018) 19(2):430. doi: 10.3390/ijms19020430
12. Zick Y. Galectin-8, cytokines, and the storm. Biochem Soc Trans (2022) 50(1):135–49. doi: 10.1042/BST20200677
13. Oatis D, Simon-Repolski E, Balta C, Mihu A, Pieretti G, Alfano R, et al. Cellular and molecular mechanism of pulmonary fibrosis post-COVID-19: Focus on galectin-1, -3, -8, -9. Int J Mol Sci (2022) 23(15):8210. doi: 10.3390/ijms23158210
14. Rabinovich GA. Galectin-1 as a potential cancer target. Br J Cancer (2005) 92(7):1188–92. doi: 10.1038/sj.bjc.6602493
15. Chalasani N, Abdelmalek MF, Garcia-Tsao G, Vuppalanchi R, Alkhouri N, Rinella M, et al. Effects of belapectin, an inhibitor of galectin-3, in patients with nonalcoholic steatohepatitis with cirrhosis and portal hypertension. Gastroenterology (2020) 158(5):1334–45.e5. doi: 10.1053/j.gastro.2019.11.296
16. Mansour AA, Krautter F, Zhi Z, Iqbal AJ, Recio C. The interplay of galectins-1, -3, and -9 in the immune-inflammatory response underlying cardiovascular and metabolic disease. Cardiovasc Diabetol (2022) 21(1):253. doi: 10.1186/s12933-022-01690-7
17. Blanda V, Bracale UM, Di Taranto MD, Fortunato G. Galectin-3 in cardiovascular diseases. Int J Mol Sci (2020) 21(23):9232. doi: 10.3390/ijms21239232
18. d’Alessandro M, De Vita E, Bergantini L, Mazzei MA, di Valvasone S, Bonizzoli M, et al. Galactin-1, 3 and 9: Potential biomarkers in idiopathic pulmonary fibrosis and other interstitial lung diseases. Respir Physiol Neurobiol (2020) 282:103546. doi: 10.1016/j.resp.2020.103546
19. Yaşar Ş, Çolak C, Yoloğlu S. Artificial intelligence-based prediction of covid-19 severity on the results of protein profiling. Comput Methods Programs Biomedicine. (2021) 202:105996. doi: 10.1016/j.cmpb.2021.105996
20. De Biasi S, Meschiari M, Gibellini L, Bellinazzi C, Borella R, Fidanza L, et al. Marked T cell activation, senescence, exhaustion and skewing towards TH17 in patients with COVID-19 pneumonia. Nat Commun (2020) 11(1):3434. doi: 10.1038/s41467-020-17292-4
21. Chen PK, Lan JL, Huang PH, Hsu JL, Chang CK, Tien N, et al. Interleukin-18 is a potential biomarker to discriminate active adult-onset still’s disease from COVID-19. Front Immunol (2021) 12. doi: 10.3389/fimmu.2021.719544
22. Kazancioglu S, Yilmaz FM, Bastug A, Ozbay BO, Aydos O, Yücel Ç, et al. Assessment of galectin-1, galectin-3, and prostaglandin e2 levels in patients with covid-19. Japanese J Infect Dis (2021) 74(6):530–6. doi: 10.7883/yoken.JJID.2021.020
23. Wells G, Shea B, O'Connell D, Peterson j, Welch V, Losos M, et al. The Newcastle–Ottawa scale (NOS) for assessing the quality of non-randomized studies in meta-analysis. (2000).
24. Begg CB, Mazumdar M. Operating characteristics of a rank correlation test for publication bias. Biometrics (1994) 50(4):1088–101. doi: 10.2307/2533446
25. Egger M, Davey Smith G, Schneider M, Minder C. Bias in meta-analysis detected by a simple, graphical test. Bmj (1997) 315(7109):629–34. doi: 10.1136/bmj.315.7109.629
26. Bai G, Furushima D, Niki T, Matsuba T, Maeda Y, Takahashi A, et al. High levels of the cleaved form of galectin-9 and osteopontin in the plasma are associated with inflammatory markers that reflect the severity of covid-19 pneumonia. Int J Mol Sci (2021) 22(9):4978. doi: 10.3390/ijms22094978
27. Baykan EK, Şebin E, Karaşahin Ö, Baykan AR, Cerrah S, Göğebakan H, et al. Galectin-3: Can it be a diagnostic tool for pneumonia in covid-19 patients? Turkish J Med Sci (2021) 51(5):2256–62. doi: 10.3906/sag-2102-202
28. Bozorgmehr N, Mashhouri S, Rosero EP, Xu L, Shahbaz S, Sligl W, et al. Galectin-9, a player in cytokine release syndrome and a surrogate diagnostic biomarker in SARS-CoV-2 infection. mBio (2021) 12(3):e00384-21. doi: 10.1128/mBio.00384-21
29. Bruni F, Charitos P, Lampart M, Moser S, Siegemund M, Bingisser R, et al. Complement and endothelial cell activation in COVID-19 patients compared to controls with suspected SARS-CoV-2 infection: A prospective cohort study. Front Immunol (2022) 13:941742. doi: 10.3389/fimmu.2022.941742
30. Cannavo A, Liccardo D, Gelzo M, Amato F, Gentile I, Pinchera B, et al. Serum galectin-3 and aldosterone: potential biomarkers of cardiac complications in patients with COVID-19. Minerva Endocrinol (2022) 47(3):270–8. doi: 10.23736/S2724-6507.22.03789-7
31. Cervantes-Alvarez E, Limon-de la Rosa N, Salgado-de la Mora M, Valdez-Sandoval P, Palacios-Jimenez M, Rodriguez-Alvarez F, et al. Galectin-3 as a potential prognostic biomarker of severe COVID-19 in SARS-CoV-2 infected patients. Sci Rep (2022) 12(1):1856. doi: 10.1038/s41598-022-05968-4
32. Ercin U, Aribas ET, Tuncbilek S, Kaya C, Dincel AS, Bilgihan A, et al. Comparison of biochemical and immunological biomarker levels of patients with COVID-19 with healthy individuals. Turkish J Biochem (2021) 46(4):365–75. doi: 10.1515/tjb-2020-0624
33. Karsli E, Anabarli Metin D, Canacik O, Sabirli R, Kaymaz B, Kurt O, et al. Galectin-3 as a potential prognostic biomarker for COVID-19 disease: A case-control study. Cureus (2022) 14(9):e28805. doi: 10.7759/cureus.28805
34. Kusnierz-Cabala B, Maziarz B, Dumnicka P, Dembinski M, Kapusta M, Bociasa-Jasik M, et al. Diagnostic significance of serum galectin-3 in hospitalized patients with COVID-19 a preliminary study. BIOMOLECULES (2021) 11(8):1136. doi: 10.3390/biom11081136
35. Markovic SS, Gajovic N, Jurisevic M, Jovanovic M, Jovicic BP, Arsenijevic N, et al. Galectin-1 as the new player in staging and prognosis of COVID-19. Sci Rep (2022) 12(1):1272. doi: 10.1038/s41598-021-04602-z
36. Özcan S, Dönmez E, Yavuz ST, Ziyrek M, İnce O, Küçük HS, et al. Prognostic significance of serum galectin-3 in hospitalized patients with COVID-19. Cytokine (2022) 158:155970. doi: 10.1016/j.cyto.2022.155970
37. Rodriguez-Tomas E, Iftimie S, Castane H, Baiges-Gaya G, Hernandez-Aguilera A, Gonzalez-Vinas M, et al. Clinical performance of paraoxonase-1-Related variables and novel markers of inflammation in coronavirus disease-19. a machine learning approach. ANTIOXIDANTS (2021) 10(6):991. doi: 10.3390/antiox10060991
38. Tawiah K, Jackson L, Omosule C, Ballman C, Shahideh B, Scott MG, et al. Serial cardiac biomarkers for risk stratification of patients with COVID-19. Clin Biochem (2022) 107:24–32. doi: 10.1016/j.clinbiochem.2022.06.002
39. Moore JB, June CH. Cytokine release syndrome in severe COVID-19. Science. (2020) 368(6490):473–4. doi: 10.1126/science.abb8925
40. Luo X, Zhou W, Yan X, Guo T, Wang B, Xia H, et al. Prognostic value of c-reactive protein in patients with coronavirus 2019. Clin Infect Dis (2020) 71(16):2174–9. doi: 10.1093/cid/ciaa641
41. Wang L. C-reactive protein levels in the early stage of COVID-19. Med Mal Infect (2020) 50(4):332–4. doi: 10.1016/j.medmal.2020.03.007
42. Lenza MP, Oyenarte I, Diercks T, Quintana JI, Gimeno A, Coelho H, et al. Structural characterization of n-linked glycans in the receptor binding domain of the SARS-CoV-2 spike protein and their interactions with human lectins. Angew Chem Int Ed Engl (2020) 59(52):23763–71. doi: 10.1002/anie.202011015
43. Modenutti CP, Capurro JIB, Di Lella S, Martí MA. The structural biology of galectin-ligand recognition: Current advances in modeling tools, protein engineering, and inhibitor design. Front Chem (2019) 7:823. doi: 10.3389/fchem.2019.00823
44. Garcia-Revilla J, Deierborg T, Venero JL, Boza-Serrano A. Hyperinflammation and fibrosis in severe COVID-19 patients: Galectin-3, a target molecule to consider. Front Immunol (2020) 11. doi: 10.3389/fimmu.2020.02069
45. Caniglia JL, Asuthkar S, Tsung AJ, Guda MR, Velpula KK. Immunopathology of galectin-3: an increasingly promising target in COVID-19. F1000Res (2020) 9:1078. doi: 10.12688/f1000research.25979.2
Keywords: COVID-19, galectin, biomarker, systematic review, meta-analysis
Citation: Behnoush AH, Khalaji A, Alemohammad SY, Kalantari A, Cannavo A and Dimitroff CJ (2023) Galectins can serve as biomarkers in COVID-19: A comprehensive systematic review and meta-analysis. Front. Immunol. 14:1127247. doi: 10.3389/fimmu.2023.1127247
Received: 20 December 2022; Accepted: 13 February 2023;
Published: 23 February 2023.
Edited by:
Milos Jesenak, Comenius University, SlovakiaReviewed by:
Umberto Basile, Catholic University of the Sacred Heart, Rome, ItalyAntonio Vella, University Hospital of Verona, Italy
Copyright © 2023 Behnoush, Khalaji, Alemohammad, Kalantari, Cannavo and Dimitroff. This is an open-access article distributed under the terms of the Creative Commons Attribution License (CC BY). The use, distribution or reproduction in other forums is permitted, provided the original author(s) and the copyright owner(s) are credited and that the original publication in this journal is cited, in accordance with accepted academic practice. No use, distribution or reproduction is permitted which does not comply with these terms.
*Correspondence: Amirmohammad Khalaji, amirm.khalaji@gmail.com