- Department of Hepatobiliary and Pancreaticosplenic Surgery, Beijing ChaoYang Hospital, Capital Medical University, Beijing, China
Background: Preoperative inflammatory status plays an important role in the prognosis of malignancy. We sought to explore the value of preoperative inflammatory biomarkers in predicting long-term outcomes of liver transplantation (LT) in patients with hepatocellular carcinoma (HCC).
Method: Patients who underwent LT for HCC in our hospital between January 2010 and June 2020 were included in this study. Demographic, clinical, laboratory, and outcome data were obtained. The area under the curve (AUC) of the receiver operating characteristic curve was used to evaluate the predictive value of inflammatory biomarkers. The effectiveness of inflammatory biomarkers in predicting outcomes was analyzed by univariate and multivariate Cox proportional hazards analyses.
Results: A total of 218 patients were included in the study, with a mean age of 53.9 ± 8.5 years. The AUC of neutrophil-to-lymphocyte ratio (NLR), platelet-to-lymphocyte ratio (PLR), monocyte-to-lymphocyte ratio (MLR), systemic immune inflammation index (SII), and systemic inflammatory response index (SIRI) for overall survival (OS) were 0.741, 0.731, 0.756, 0.746, and 0.749, respectively. Cox proportional hazards model indicated that SIRI > 1.25 was independently associated with low OS [hazard ratio (HR) = 2.258, P = 0.024]. PLR > 82.15 and SIRI > 0.95 were independently associated with low disease-free survival (HR = 1.492, P = 0.015; and HR = 1.732, P = 0.008, respectively). In the survival analysis, the prognosis of patients with high preoperative SIRI and PLR was significantly worse (P < 0.001).
Conclusion: SIRI and PLR were useful prognostic markers for predicting patients with HCC after LT.
Introduction
Hepatocellular carcinoma (HCC) is the most common pathological type of primary liver cancer, and the incidence is increasing year by year (1, 2). It is also an important factor of cancer-related death (3, 4). For patients with early-stage HCC, liver transplantation (LT) is undoubtedly one of the most effective forms of treatment. LT can radically remove the carcinoma and also cure liver disease such as cirrhosis, which removes major risk factors for new-onset tumors. Therefore, of all the treatments, LT is the most likely to cure the patient (5–7). Although patients with HCC were screened according to selection criteria before receiving LT, about 10%–20% of patients still experience recurrence after LT (8, 9). This also severely limits the long-term survival of these patients. Therefore, clinicians need to screen out patients who will have better survival benefits after LT to make more efficient use of scarce donor liver sources.
Recently, clinical biomarkers based on laboratory results help to objectively assess the patient’s status and have predictive value for some patient outcomes (10–13). These include several inflammatory and immune scores, such as platelet-to-lymphocyte ratio (PLR), systemic immune inflammation index (SII), neutrophil-to-lymphocyte ratio (NLR), systemic inflammatory response index (SIRI), and monocyte-to-lymphocyte ratio (MLR). NLR, PLR, and MLR reflect immune function and inflammatory states, which are also useful for predicting tumor recurrence and early death (11–17). SII, calculated from platelet, neutrophil, and lymphocyte counts, is a strong predictor of poor prognosis for patients with HCC and helps physicians make clinical decisions (18–20). SIRI may be effective in reflecting the dynamics of inflammation and immune status, predicting prognosis after resection of HCC and response to systemic treatment for HCC (21–23).
We conducted this study to determine the utility of preoperative inflammatory biomarkers in predicting long-term outcomes in patients with HCC after LT.
Materials and methods
Study population
We retrospectively collected patients who received LT for HCC in our hospital between January 2010 and June 2020. The selection of the study population is represented by a flowchart (Figure 1).
Exclusion criteria are as follows: (1) combined acute infection, (2) preoperative diagnosis of hypersplenism, and (3) incomplete data.
Data collection and follow-up
We applied electronic medical record system to collect the following data: demographic and laboratory characteristics. All enrolled patients had venous blood samples taken within 24 h of admission and performed complete blood count analysis. About biomarkers, NLR was the ratio of neutrophil count to lymphocyte count, PLR was the ratio of platelet count to lymphocyte count, SII was defined as platelet count * neutrophil count/lymphocyte count, MLR was the ratio of monocyte count to lymphocyte count, and SIRI was defined as monocyte count * neutrophil count/lymphocyte count.
We followed all patients by telephone and outpatient or inpatient observation until June 2022 or death. Follow-ups were performed every 3 months for 2 years after surgery and every 6 months thereafter. If there was a suspicious lesion in the liver or lungs, then biopsy was performed to determine whether it was recurrence or metastasis. The time recurrence started was defined as the time when AFP levels started to rise. LT to death or last observation was defined as overall survival (OS), and LT to recurrence was defined as disease-free survival (DFS).
Statistical analysis
Continuous variables were compared between groups by either the Student’s t-test or the Mann-Whitney U-test. Categorical variables were compared between groups by Pearson’s chi-square test or Fisher’s exact test. The optimal cutoff value was determined by receiver operating characteristic (ROC) curve. Independent prognostic factors related to OS and DFS were identified by Cox regression models. Variables were included in multivariate analysis if P < 0.2 in univariate analysis. Kaplan–Meier curves with the log-rank test were used to survival analysis. Statistical significance was defined as two-sided P < 0.05. All statistical analyses were performed using SPSS 26.0 and GraphPad Prism 8.0.
Results
Characteristics of patients
Flowchart shows the included and excluded patients (Figure 1). A total of 218 patients were recruited. The mean age of all patients was 53.9 ± 8.5 years, in which 90.4% are men (197 of 218). The median follow-up was 39.4 months. Survival outcomes at 2 years of follow-up determined patient grouping.
The baseline characteristics are shown in Table 1. Compared to the non-survival group, the survival group had a significantly higher body mass index (BMI) of 25.0 ± 3.9 (P = 0.014). There were no significant differences in age and sex (P > 0.05). Common comorbidities such as diabetes, hypertension, hepatic encephalopathy, and HBV positivity were also not significantly different between groups (P > 0.05). In the pathological information, tumor number, total tumor size, largest tumor size, and degree of tumor differentiation were significantly different between groups (P < 0.001).
Compared with the survival group, the white blood cells, neutrophil, lymphocyte, monocyte counts, and AFP of the non-survival group increased significantly (P = 0.018, 0.008, 0.003, 0.026, and 0.034, respectively). There were significant differences in preoperative NLR, PLR, MLR, SII, and SIRI between groups (P = 0.001, < 0.001, < 0.001, < 0.001, and < 0.001, respectively).
Preoperative inflammatory biomarkers and prognosis
The ROC curves were used for exploring the correlation between preoperative inflammatory biomarkers and outcomes after LT (Figures 2A, B). The optimal cutoff values for NLR, PLR, MLR, SII, and SIRI to predict postoperative OS were 4.1, 82.15, 0.45, 179.8, and 1.25, respectively [the area under the curve (AUC) = 0.741, 0.731, 0.756, 0.746, and 0.749, respectively; Supplementary Table 1]. For DFS, the optimal cutoff values were 2.35, 82.15, 0.45, 86.3, and 0.95, respectively (AUC = 0.658, 0.647, 0.599, 0.649, and 0.601, respectively; Supplementary Table 2).
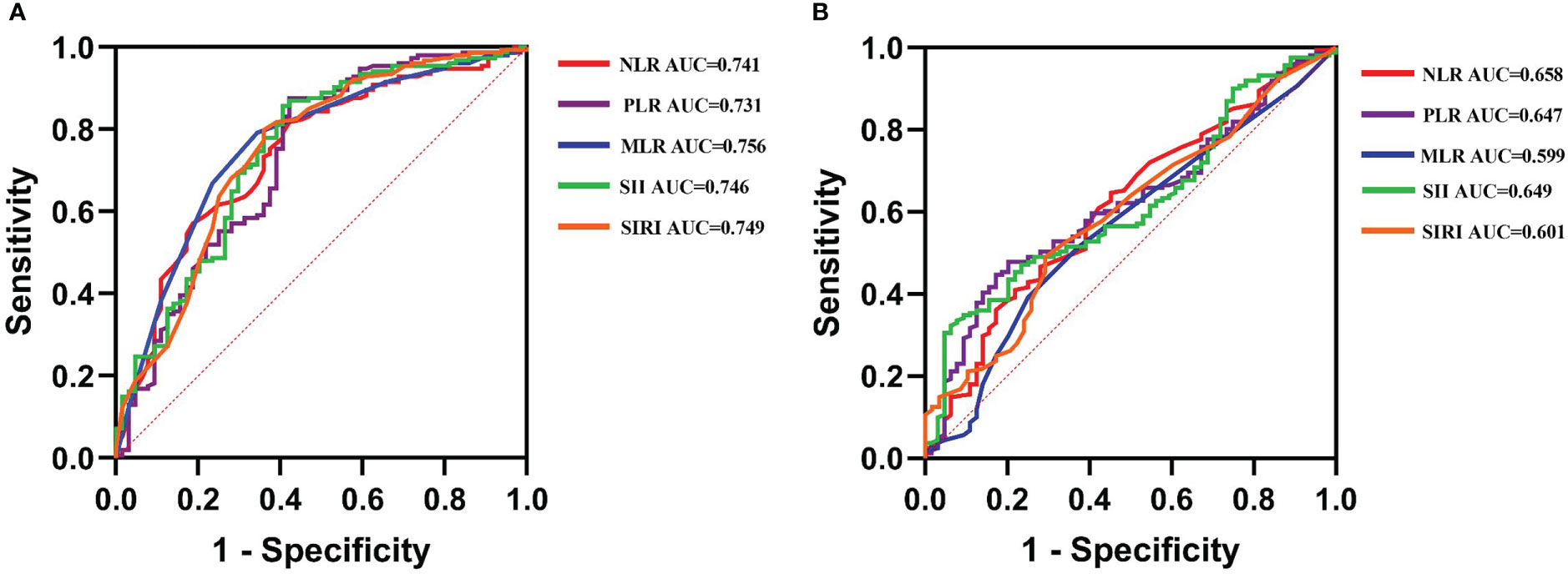
Figure 2 The receiver operating characteristic (ROC) curves explore the value of preoperative inflammatory biomarkers in predicting the long-time prognosis in liver transplantation (LT) for hepatocellular carcinoma (HCC). (A) The value of ROC in predicting overall survival in LT for HCC. (B) The value of ROC in predicting disease-free survival in LT for HCC.
Tables 2 and 3 show the results of Cox regression analysis. Multivariate Cox proportional hazards regression analysis showed that BMI (>25.55), AFP (>73.2), tumor number (>3), differentiation (1–2), and SIRI (>1.25) were independent related factors for OS [hazard ratio (HR) = 0.924, P = 0.029; HR = 2.376, P = 0.002; HR = 2.193, P = 0.002; HR = 3.006, P = 0.024; and HR = 2.258, P = 0.024, respectively] (Table 2). AFP (>73.2), tumor number (>3), differentiation (1–2), PLR (>82.15), and SIRI (>0.95) were the independent risk factors for DFS (HR = 1.626, P = 0.005; HR = 1.861, P = 0.001; HR = 2.435, P = 0.001; HR = 1.492, P = 0.015; and HR = 1.732, P = 0.008, respectively) (Table 3).
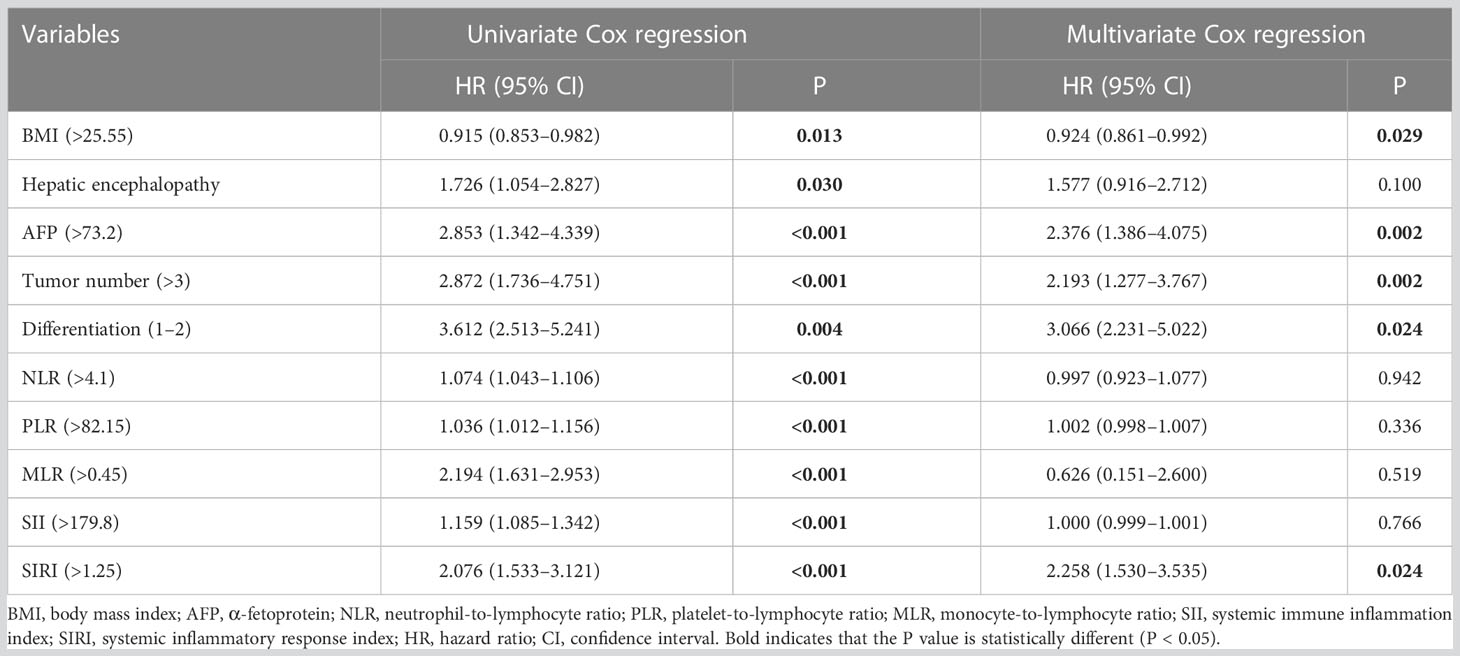
Table 2 Cox regression analysis of the effects of clinicopathological factors on the overall survival of patients.
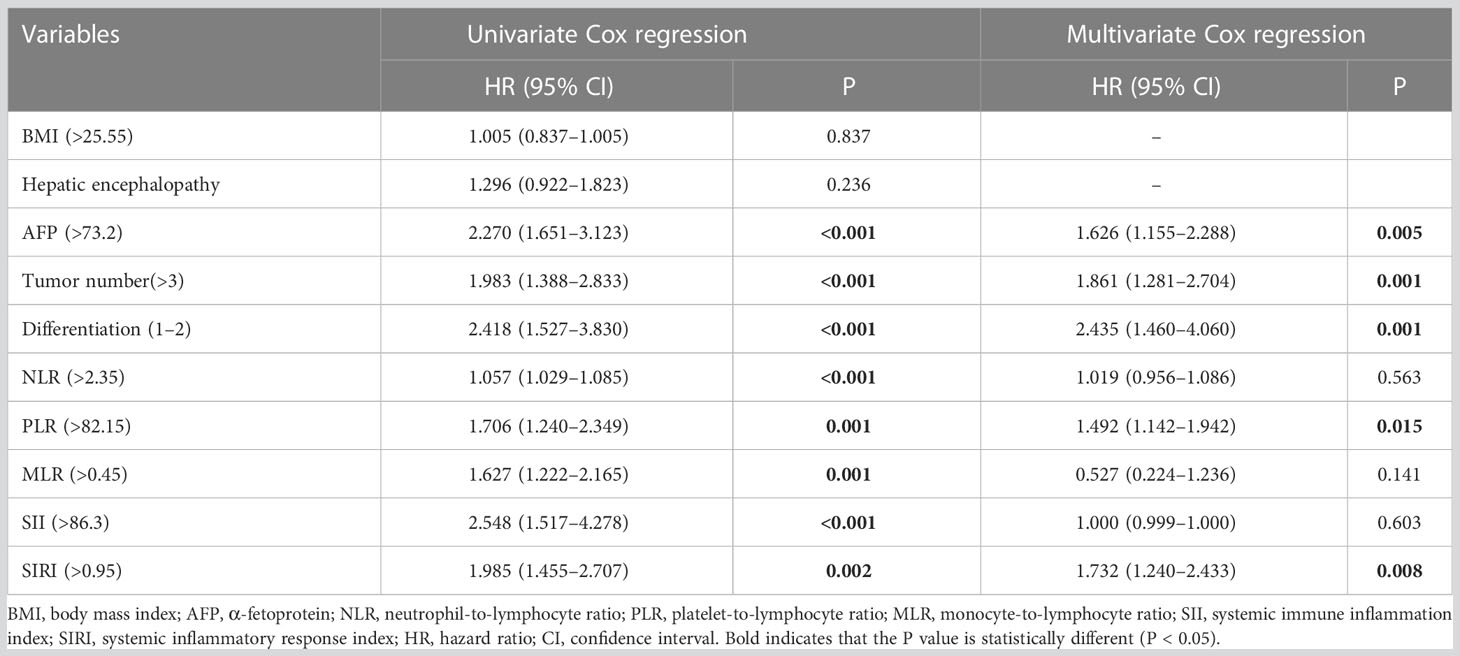
Table 3 Cox regression analysis of the effects of clinicopathological factors on the disease-free survival of patients.
Kaplan–Meier survival analysis for long-term prognosis was shown in Figures 3A–C. Compared with the high-SIRI group, the 5-year OS rate and DFS rate of the low-SIRI group were significantly higher (40.2% vs. 81.9%, log-rank test, P < 0.001; 16.8% vs. 33.3%, log-rank test, P < 0.001; Figures 3A, B). In addition, compared with the high-PLR group, the 5-year DFS rate of the low-PLR group was significantly higher (20.6% vs. 33.7%, log-rank test, P = 0.001; Figure 3C).
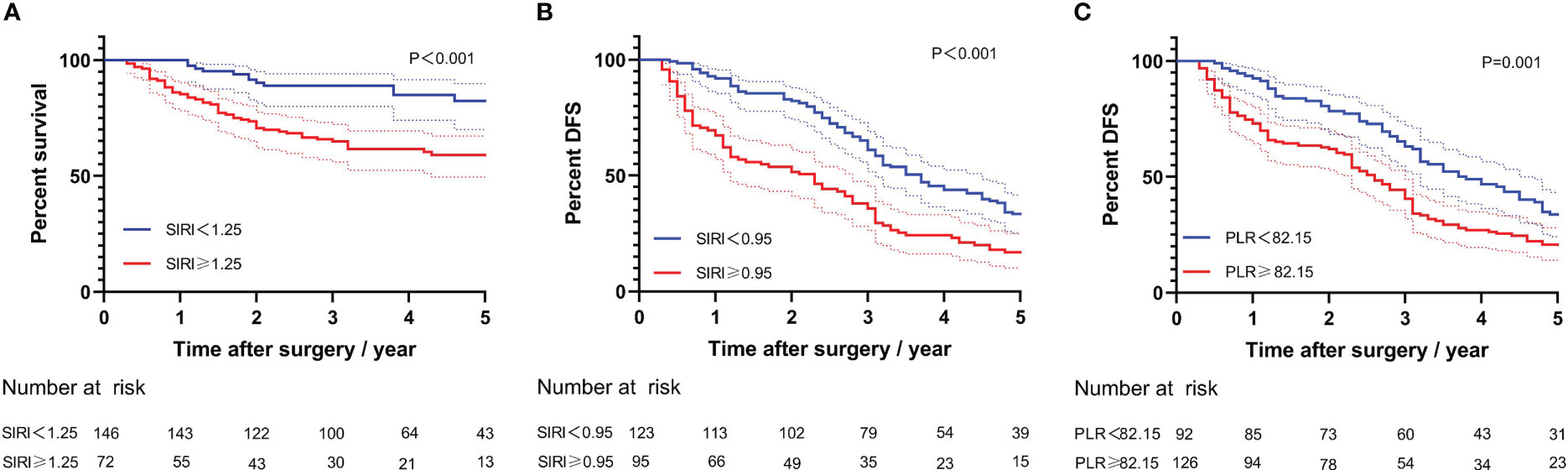
Figure 3 Kaplan–Meier curves for long-time prognosis by PLR and SIRI. (A) Kaplan–Meier curves for overall survival by SIRI. (B) Kaplan–Meier curves for disease-free survival by SIRI. (C) Kaplan–Meier curves for disease-free survival by PLR.
Discussion
Our study found that inflammatory biomarkers such as PLR and SIRI were significantly related to long-term prognosis after LT for HCC. High SIRI and high PLR were the independent risk factors for poor prognosis, particularly SIRI, which was clearly associated with both OS and DFS.
Over the past 10 years, NLR has received increasing attention as an emerging disease biomarker. NLR is the ratio of neutrophil count to lymphocyte count, combining the innate immune response and acquired immunity in the immune system (24). NLR has been shown to be critical in sepsis, pneumonia, COVID-19, cardiovascular disease, cancer, and other diseases and is independently related to prognosis (25–31). In terms of cancers, it is pointed out that inflammation contributes to the development and progression of cancers (32). The correlation between NLR and HCC prognosis has also been actively explored. Studies found that patients with HCC with low NLR were more likely to have a better long-term prognosis (33–35). Moreover, NLR also has clear correlation with long-term prognosis after surgical resection, LT, transarterial chemoembolization, and radiofrequency ablation, with HRs ranging from 1.16 to 4.22 (33, 36–39).
PLR, MLR, and SII have been widely used in predicting the death and recurrence of HCC (17–20, 40–44). This study showed a significant correlation between high preoperative PLR and low DFS in patients with HCC receiving LT. This is also consistent with some previous findings (45–47). The correlation of PLR with tumor characteristics may play an important role. High PLR has been shown to be associated with larger tumor size and lymph node metastasis (48, 49). As for HCC, studies have shown that high PLR means a high likelihood of advanced tumor staging and aggressive tumor phenotype (45). On the one hand, tumor cells can activate platelets, and activated platelets will directly adhere to tumor cells, helping tumor cells to escape immunity (50, 51). On the other hand, activated platelets can also promote tumor angiogenesis and development by expressing cytokines (52, 53). In addition, activated platelets can cause cancer-associated thrombosis, resulting in a poor prognosis (54, 55). Lymphocytes are also an important part of anti-tumor immunity, which can secrete cytokines to activate anti-tumor immunity and directly kill tumor cells, thereby inhibiting the proliferation and migration of tumor cells. Therefore, when the number of lymphocytes in the peripheral blood decreases, the body’s defenses against cancer cells are also weakened, which can lead to tumor progression and recurrence (56).
SIRI is calculated from lymphocyte, neutrophil, and monocyte counts, and its predictive value for malignancy prognosis has been demonstrated (57–60). Nevertheless, SIRI still does not receive enough attention in patients with HCC with LT. This study showed a clear correlation between high SIRI and poor long-term outcomes in this population. In addition to the lymphocytes mentioned above, neutrophils and monocytes also play critical roles in the development of tumors. At present, the mechanism has not been fully clarified. Tumor cells can secrete cytokines to stimulate the proliferation and differentiation of neutrophils and promote the increase of circulating neutrophil levels (61). High levels of circulating neutrophils lead to decreased function of T cells and lymphocytes, leading to weakened immune function (62, 63). Clinically, elevated peripheral neutrophil counts are clearly related to high polymorphonuclear neutrophil myeloid-derived suppressor cells (PMN-MDSCs), resulting in poor prognosis for patients with HCC (64). PMN-MDSCs have immunosuppressive ability, and, in HCC, they can regulate arginase-1 and reactive oxygen species to inhibit T cells (65, 66). Notably, it is pointed out that PMN-MDSCs and tumor-associated neutrophils have some similarities in origin, morphology, and molecular phenotype and also have some functional overlap (67). Similarly, previous studies have confirmed that the number of circulating monocytes determines the number of tumor-associated macrophages (68–70). As for TAM, it is able to induce apoptosis of T cells with anticancer functions and promote tumor angiogenesis, thereby promoting tumor growth, invasion, and migration, resulting in a poor prognosis (71, 72).
There were several limitations in our study. First, the study was retrospective, single-center that would skew the results. The results still need to be further validated in multi-center prospective studies. Second, preoperative inflammatory biomarkers were affected by many factors, such as heavy bleeding and instrument errors, making the measurement results inaccurate. Last, this study did not take repeated measurements at different time points to observe the dynamics of these biomarkers.
Conclusion
Elevated preoperative SIRI and PLR were the independent risk factors for poor prognosis in LT for HCC. This result will help clinicians to screen out patients with better prognosis before surgery and to provide some reference for decision-making on comprehensive treatment after surgery.
Data availability statement
The original contributions presented in the study are included in the article/Supplementary Material. Further inquiries can be directed to the corresponding author.
Ethics statement
The study involving human participants were reviewed and approved by Beijing Chaoyang Hospital ethics committee (No.2020-D.304). The patients/participants provided their written informed consent to participate in this study.
Author contributions
SPC and SC conceived and designed the study. SC and QC collected the data. SC and QC analyzed the data. SPC wrote the original draft. QH and RL revised the manuscript. RL administered and supervised the study. All authors contributed to the article and approved the submitted version.
Conflict of interest
The authors declare that the research was conducted in the absence of any commercial or financial relationships that could be construed as a potential conflict of interest.
Publisher’s note
All claims expressed in this article are solely those of the authors and do not necessarily represent those of their affiliated organizations, or those of the publisher, the editors and the reviewers. Any product that may be evaluated in this article, or claim that may be made by its manufacturer, is not guaranteed or endorsed by the publisher.
Supplementary material
The Supplementary Material for this article can be found online at: https://www.frontiersin.org/articles/10.3389/fimmu.2023.1118053/full#supplementary-material
References
1. El-Serag HB, Davila JA, Petersen NJ, McGlynn KA. The continuing increase in the incidence of hepatocellular carcinoma in the united states: An update. Ann Intern Med (2003) 139(10):817–23. doi: 10.7326/0003-4819-139-10-200311180-00009
2. El-Serag HB, Kanwal F. Epidemiology of hepatocellular carcinoma in the united states: Where are we? where do we go? Hepatology (2014) 60(5):1767–75. doi: 10.1002/hep.27222
3. Bray F, Ferlay J, Soerjomataram I, Siegel RL, Torre LA, Jemal A. Global cancer statistics 2018: GLOBOCAN estimates of incidence and mortality worldwide for 36 cancers in 185 countries. CA Cancer J Clin (2018) 68(6):394–424. doi: 10.3322/caac.21492
4. Yang JD, Hainaut P, Gores GJ, Amadou A, Plymoth A, Roberts LR. A global view of hepatocellular carcinoma: Trends, risk, prevention and management. Nat Rev Gastroenterol Hepatol (2019) 16(10):589–604. doi: 10.1038/s41575-019-0186-y
5. Forner A, Llovet JM, Bruix J. Hepatocellular carcinoma. Lancet (2012) 379(9822):1245–55. doi: 10.1016/S0140-6736(11)61347-0
6. de Lope CR, Tremosini S, Forner A, Reig M, Bruix J. Management of HCC. J Hepatol (2012) 56 Suppl 1:S75–87. doi: 10.1016/S0168-8278(12)60009-9
7. Adam R, Bhangui P, Vibert E, Azoulay D, Pelletier G, Duclos-Vallee JC, et al. Resection or transplantation for early hepatocellular carcinoma in a cirrhotic liver: Does size define the best oncological strategy? Ann Surg (2012) 256(6):883–91. doi: 10.1097/SLA.0b013e318273bad0
8. Vivarelli M, Cucchetti A, La Barba G, Ravaioli M, Del Gaudio M, Lauro A, et al. Liver transplantation for hepatocellular carcinoma under calcineurin inhibitors: Reassessment of risk factors for tumor recurrence. Ann Surg (2008) 248(5):857–62. doi: 10.1097/SLA.0b013e3181896278
9. Bhangui P, Allard MA, Vibert E, Cherqui D, Pelletier G, Cunha AS, et al. Salvage versus primary liver transplantation for early hepatocellular carcinoma: Do both strategies yield similar outcomes? Ann Surg (2016) 264(1):155–63. doi: 10.1097/SLA.0000000000001442
10. Citores MJ, Lucena JL, de la Fuente S, Cuervas-Mons V. Serum biomarkers and risk of hepatocellular carcinoma recurrence after liver transplantation. World J Hepatol (2019) 11(1):50–64. doi: 10.4254/wjh.v11.i1.50
11. Lai Q, Melandro F, Larghi Laureiro Z, Giovanardi F, Ginanni Corradini S, Ferri F, et al. Platelet-to-lymphocyte ratio in the setting of liver transplantation for hepatocellular cancer: A systematic review and meta-analysis. World J Gastroenterol (2018) 24(15):1658–65. doi: 10.3748/wjg.v24.i15.1658
12. Kwon HM, Moon YJ, Jung KW, Park YS, Jun IG, Kim SO, et al. Neutrophil-to-lymphocyte ratio is a predictor of early graft dysfunction following living donor liver transplantation. Liver Int (2019) 39(8):1545–56. doi: 10.1111/liv.14103
13. Templeton AJ, McNamara MG, Seruga B, Vera-Badillo FE, Aneja P, Ocana A, et al. Prognostic role of neutrophil-to-lymphocyte ratio in solid tumors: A systematic review and meta-analysis. J Natl Cancer Inst (2014) 106(6):dju124. doi: 10.1093/jnci/dju124
14. Lai Q, Castro Santa E, Rico Juri JM, Pinheiro RS, Lerut J. Neutrophil and platelet-to-lymphocyte ratio as new predictors of dropout and recurrence after liver transplantation for hepatocellular cancer. Transpl Int (2014) 27(1):32–41. doi: 10.1111/tri.12191
15. Dolan RD, McSorley ST, Horgan PG, Laird B, McMillan DC. The role of the systemic inflammatory response in predicting outcomes in patients with advanced inoperable cancer: Systematic review and meta-analysis. Crit Rev Oncol Hematol (2017) 116:134–46. doi: 10.1016/j.critrevonc.2017.06.002
16. Feng Y, Zhang N, Wang S, Zou W, He Y, Ma JA, et al. Systemic inflammation response index is a predictor of poor survival in locally advanced nasopharyngeal carcinoma: A propensity score matching study. Front Oncol (2020) 10:575417. doi: 10.3389/fonc.2020.575417
17. Mao S, Yu X, Shan Y, Fan R, Wu S, Lu C. Albumin-bilirubin (ALBI) and monocyte to lymphocyte ratio (MLR)-based nomogram model to predict tumor recurrence of AFP-negative hepatocellular carcinoma. J Hepatocell Carcinoma (2021) 8:1355–65. doi: 10.2147/JHC.S339707
18. Hu B, Yang XR, Xu Y, Sun YF, Sun C, Guo W, et al. Systemic immune-inflammation index predicts prognosis of patients after curative resection for hepatocellular carcinoma. Clin Cancer Res (2014) 20(23):6212–22. doi: 10.1158/1078-0432.CCR-14-0442
19. Yang J, Bao Y, Chen W, Duan Y, Sun D. Nomogram based on systemic immune inflammation index and prognostic nutrition index predicts recurrence of hepatocellular carcinoma after surgery. Front Oncol (2020) 10:551668. doi: 10.3389/fonc.2020.551668
20. Wang BL, Tian L, Gao XH, Ma XL, Wu J, Zhang CY, et al. Dynamic change of the systemic immune inflammation index predicts the prognosis of patients with hepatocellular carcinoma after curative resection. Clin Chem Lab Med (2016) 54(12):1963–9. doi: 10.1515/cclm-2015-1191
21. Mao S, Yu X, Sun J, Yang Y, Shan Y, Sun J, et al. Development of nomogram models of inflammatory markers based on clinical database to predict prognosis for hepatocellular carcinoma after surgical resection. BMC Cancer (2022) 22(1):249. doi: 10.1186/s12885-022-09345-2
22. Zhao M, Duan X, Han X, Wang J, Han G, Mi L, et al. Sarcopenia and systemic inflammation response index predict response to systemic therapy for hepatocellular carcinoma and are associated with immune cells. Front Oncol (2022) 12:854096. doi: 10.3389/fonc.2022.854096
23. Wang TC, An TZ, Li JX, Pang PF. Systemic inflammation response index is a prognostic risk factor in patients with hepatocellular carcinoma undergoing TACE. Risk Manag Healthc Policy (2021) 14:2589–600. doi: 10.2147/RMHP.S316740
24. Song M, Graubard BI, Rabkin CS, Engels EA. Neutrophil-to-lymphocyte ratio and mortality in the united states general population. Sci Rep (2021) 11(1):464. doi: 10.1038/s41598-020-79431-7
25. Huang Z, Fu Z, Huang W, Huang K. Prognostic value of neutrophil-to-lymphocyte ratio in sepsis: A meta-analysis. Am J Emerg Med (2020) 38(3):641–7. doi: 10.1016/j.ajem.2019.10.023
26. Dragoescu AN, Padureanu V, Stanculescu AD, Chiutu LC, Tomescu P, Geormaneanu C, et al. Neutrophil to lymphocyte ratio (NLR)-a useful tool for the prognosis of sepsis in the ICU. Biomedicines (2021) 10(1):75. doi: 10.3390/biomedicines10010075
27. Feng DY, Zhou YQ, Zhou M, Zou XL, Wang YH, Zhang TT. Risk factors for mortality due to ventilator-associated pneumonia in a Chinese hospital: A retrospective study. Med Sci Monit (2019) 25:7660–5. doi: 10.12659/MSM.916356
28. Seyit M, Avci E, Nar R, Senol H, Yilmaz A, Ozen M, et al. Neutrophil to lymphocyte ratio, lymphocyte to monocyte ratio and platelet to lymphocyte ratio to predict the severity of COVID-19. Am J Emerg Med (2021) 40:110–4. doi: 10.1016/j.ajem.2020.11.058
29. Grasselli G, Greco M, Zanella A, Albano G, Antonelli M, Bellani G, et al. Risk factors associated with mortality among patients with COVID-19 in intensive care units in Lombardy, Italy. JAMA Intern Med (2020) 180(10):1345–55. doi: 10.1001/jamainternmed.2020.3539
30. Shah N, Parikh V, Patel N, Patel N, Badheka A, Deshmukh A, et al. Neutrophil lymphocyte ratio significantly improves the framingham risk score in prediction of coronary heart disease mortality: Insights from the national health and nutrition examination survey-III. Int J Cardiol (2014) 171(3):390–7. doi: 10.1016/j.ijcard.2013.12.019
31. Lianos GD, Alexiou GA, Exarchos C, Rausei S, Mitsis M, Voulgaris S. Prognostic significance of neutrophil-to-lymphocyte ratio in several malignancies: Where do we stand? biomark Med (2020) 14(3):169–72. doi: 10.2217/bmm-2019-0497
32. Hanahan D, Weinberg RA. Hallmarks of cancer: The next generation. Cell (2011) 144(5):646–74. doi: 10.1016/j.cell.2011.02.013
33. Xiao WK, Chen D, Li SQ, Fu SJ, Peng BG, Liang LJ. Prognostic significance of neutrophil-lymphocyte ratio in hepatocellular carcinoma: a meta-analysis. BMC Cancer (2014) 14:117. doi: 10.1186/1471-2407-14-117
34. Xue TC, Zhang L, Xie XY, Ge NL, Li LX, Zhang BH, et al. Prognostic significance of the neutrophil-to-lymphocyte ratio in primary liver cancer: A meta-analysis. PloS One (2014) 9(5):e96072. doi: 10.1371/journal.pone.0096072
35. Limaye AR, Clark V, Soldevila-Pico C, Morelli G, Suman A, Firpi R, et al. Neutrophil-lymphocyte ratio predicts overall and recurrence-free survival after liver transplantation for hepatocellular carcinoma. Hepatol Res (2013) 43(7):757–64. doi: 10.1111/hepr.12019
36. Huang ZL, Luo J, Chen MS, Li JQ, Shi M. Blood neutrophil-to-lymphocyte ratio predicts survival in patients with unresectable hepatocellular carcinoma undergoing transarterial chemoembolization. J Vasc Interv Radiol (2011) 22(5):702–9. doi: 10.1016/j.jvir.2010.12.041
37. Gomez D, Farid S, Malik HZ, Young AL, Toogood GJ, Lodge JP, et al. Preoperative neutrophil-to-lymphocyte ratio as a prognostic predictor after curative resection for hepatocellular carcinoma. World J Surg (2008) 32(8):1757–62. doi: 10.1007/s00268-008-9552-6
38. Zhou D, Zhang Y, Xu L, Zhou Z, Huang J, Chen M. A monocyte/granulocyte to lymphocyte ratio predicts survival in patients with hepatocellular carcinoma. Sci Rep (2015) 5:15263. doi: 10.1038/srep15263
39. Pravisani R, Mocchegiani F, Isola M, Lorenzin D, Adani GL, Cherchi V, et al. Postoperative trends and prognostic values of inflammatory and nutritional biomarkers after liver transplantation for hepatocellular carcinoma. Cancers (Basel) (2021) 13(3):513. doi: 10.3390/cancers13030513
40. Schobert IT, Savic LJ, Chapiro J, Bousabarah K, Chen E, Laage-Gaupp F, et al. Neutrophil-to-lymphocyte and platelet-to-lymphocyte ratios as predictors of tumor response in hepatocellular carcinoma after DEB-TACE. Eur Radiol (2020) 30(10):5663–73. doi: 10.1007/s00330-020-06931-5
41. Guo L, Ren H, Pu L, Zhu X, Liu Y, Ma X. The prognostic value of inflammation factors in hepatocellular carcinoma patients with hepatic artery interventional treatments: A retrospective study. Cancer Manag Res (2020) 12:7173–88. doi: 10.2147/CMAR.S257934
42. Zhang L, Yan ZP, Hou ZH, Huang P, Yang MJ, Zhang S, et al. Neutrophil-to-Lymphocyte and platelet-to-Lymphocyte ratios as predictors of outcomes in patients with unresectable hepatocellular carcinoma undergoing transarterial chemoembolization plus sorafenib. Front Mol Biosci (2021) 8:624366. doi: 10.3389/fmolb.2021.624366
43. Chen Y, Yang Y, Zhang XY, Fan QS, Li X, Xin YJ, et al. Nomogram based on neutrophil-to-Lymphocyte ratio and platelet-to-Lymphocyte ratio to predict recurrence in patients with hepatocellular carcinoma after radiofrequency ablation. Cardiovasc Intervent Radiol (2021) 44(10):1551–60. doi: 10.1007/s00270-021-02872-8
44. van der Zee-Neuen A, Putrik P, Ramiro S, Keszei AP, Hmamouchi I, Dougados M, et al. Large Country differences in work outcomes in patients with RA - an analysis in the multinational study COMORA. Arthritis Res Ther (2017) 19(1):216. doi: 10.1186/s13075-017-1421-y
45. Xia W, Ke Q, Wang Y, Wang W, Zhang M, Shen Y, et al. Predictive value of pre-transplant platelet to lymphocyte ratio for hepatocellular carcinoma recurrence after liver transplantation. World J Surg Oncol (2015) 13:60. doi: 10.1186/s12957-015-0472-2
46. Ismael MN, Forde J, Milla E, Khan W, Cabrera R. Utility of inflammatory markers in predicting hepatocellular carcinoma survival after liver transplantation. BioMed Res Int (2019) 2019:7284040. doi: 10.1155/2019/7284040
47. Ren A, Li Z, Zhang X, Deng R, Ma Y. Inflammation-based prognostic scores in patients with hepatitis b virus-related hepatocellular carcinoma after liver transplantation. J Hepatocell Carcinoma (2020) 7:101–6. doi: 10.2147/JHC.S259992
48. Kwon HC, Kim SH, Oh SY, Lee S, Lee JH, Choi HJ, et al. Clinical significance of preoperative neutrophil-lymphocyte versus platelet-lymphocyte ratio in patients with operable colorectal cancer. Biomarkers (2012) 17(3):216–22. doi: 10.3109/1354750X.2012.656705
49. Azab B, Shah N, Radbel J, Tan P, Bhatt V, Vonfrolio S, et al. Pretreatment neutrophil/lymphocyte ratio is superior to platelet/lymphocyte ratio as a predictor of long-term mortality in breast cancer patients. Med Oncol (2013) 30(1):432. doi: 10.1007/s12032-012-0432-4
50. Labelle M, Begum S, Hynes RO. Direct signaling between platelets and cancer cells induces an epithelial-mesenchymal-like transition and promotes metastasis. Cancer Cell (2011) 20(5):576–90. doi: 10.1016/j.ccr.2011.09.009
51. Sierko E, Wojtukiewicz MZ. Platelets and angiogenesis in malignancy. Semin Thromb Hemost (2004) 30(1):95–108. doi: 10.1055/s-2004-822974
52. Bambace NM, Holmes CE. The platelet contribution to cancer progression. J Thromb Haemost (2011) 9(2):237–49. doi: 10.1111/j.1538-7836.2010.04131.x
53. Ferroni P, Spila A, D'Alessandro R, Martini F, Iacovone F, Ettorre GM, et al. Platelet activation and vascular endothelial growth factor 165 release in hepatocellular cancer. Clin Chim Acta (2011) 412(5-6):450–4. doi: 10.1016/j.cca.2010.11.026
54. Plantureux L, Crescence L, Dignat-George F, Panicot-Dubois L, Dubois C. Effects of platelets on cancer progression. Thromb Res (2018) 164 Suppl 1:S40–S7. doi: 10.1016/j.thromres.2018.01.035
55. Timp JF, Braekkan SK, Versteeg HH, Cannegieter SC. Epidemiology of cancer-associated venous thrombosis. Blood (2013) 122(10):1712–23. doi: 10.1182/blood-2013-04-460121
56. Dunn GP, Old LJ, Schreiber RD. The immunobiology of cancer immunosurveillance and immunoediting. Immunity (2004) 21(2):137–48. doi: 10.1016/j.immuni.2004.07.017
57. Qi Q, Zhuang L, Shen Y, Geng Y, Yu S, Chen H, et al. A novel systemic inflammation response index (SIRI) for predicting the survival of patients with pancreatic cancer after chemotherapy. Cancer (2016) 122(14):2158–67. doi: 10.1002/cncr.30057
58. Chao B, Ju X, Zhang L, Xu X, Zhao Y. A novel prognostic marker systemic inflammation response index (SIRI) for operable cervical cancer patients. Front Oncol (2020) 10:766. doi: 10.3389/fonc.2020.00766
59. Sun L, Hu W, Liu M, Chen Y, Jin B, Xu H, et al. High systemic inflammation response index (SIRI) indicates poor outcome in gallbladder cancer patients with surgical resection: A single institution experience in China. Cancer Res Treat (2020) 52(4):1199–210. doi: 10.4143/crt.2020.303
60. Wang L, Zhou Y, Xia S, Lu L, Dai T, Li A, et al. Prognostic value of the systemic inflammation response index (SIRI) before and after surgery in operable breast cancer patients. Cancer biomark (2020) 28(4):537–47. doi: 10.3233/CBM-201682
61. Chan SL, Chan AW, Chan AK, Jian P, Mo F, Chan CM, et al. Systematic evaluation of circulating inflammatory markers for hepatocellular carcinoma. Liver Int (2017) 37(2):280–9. doi: 10.1111/liv.13218
62. De Larco JE, Wuertz BR, Furcht LT. The potential role of neutrophils in promoting the metastatic phenotype of tumors releasing interleukin-8. Clin Cancer Res (2004) 10(15):4895–900. doi: 10.1158/1078-0432.CCR-03-0760
63. Ardi VC, Kupriyanova TA, Deryugina EI, Quigley JP. Human neutrophils uniquely release TIMP-free MMP-9 to provide a potent catalytic stimulator of angiogenesis. Proc Natl Acad Sci USA (2007) 104(51):20262–7. doi: 10.1073/pnas.0706438104
64. Li X, Xing YF, Lei AH, Xiao Q, Lin ZH, Hong YF, et al. Neutrophil count is associated with myeloid derived suppressor cell level and presents prognostic value of for hepatocellular carcinoma patients. Oncotarget (2017) 8(15):24380–8. doi: 10.18632/oncotarget.15456
65. Bronte V, Brandau S, Chen SH, Colombo MP, Frey AB, Greten TF, et al. Recommendations for myeloid-derived suppressor cell nomenclature and characterization standards. Nat Commun (2016) 7:12150. doi: 10.1038/ncomms12150
66. Nan J, Xing YF, Hu B, Tang JX, Dong HM, He YM, et al. Endoplasmic reticulum stress induced LOX-1(+ ) CD15(+) polymorphonuclear myeloid-derived suppressor cells in hepatocellular carcinoma. Immunology (2018) 154(1):144–55. doi: 10.1111/imm.12876
67. Coffelt SB, Wellenstein MD, de Visser KE. Neutrophils in cancer: Neutral no more. Nat Rev Cancer (2016) 16(7):431–46. doi: 10.1038/nrc.2016.52
68. Wang H, Shao Q, Wang J, Zhao L, Wang L, Cheng Z, et al. Decreased CXCR2 expression on circulating monocytes of colorectal cancer impairs recruitment and induces re-education of tumor-associated macrophages. Cancer Lett (2022) 529:112–25. doi: 10.1016/j.canlet.2022.01.004
69. Patysheva M, Larionova I, Stakheyeva M, Grigoryeva E, Iamshchikov P, Tarabanovskaya N, et al. Effect of early-stage human breast carcinoma on monocyte programming. Front Oncol (2021) 11:800235. doi: 10.3389/fonc.2021.800235
70. Patysheva M, Frolova A, Larionova I, Afanas'ev S, Tarasova A, Cherdyntseva N, et al. Monocyte programming by cancer therapy. Front Immunol (2022) 13:994319. doi: 10.3389/fimmu.2022.994319
71. Liao R, Jiang N, Tang ZW, Li de W, Huang P, Luo SQ, et al. Systemic and intratumoral balances between monocytes/macrophages and lymphocytes predict prognosis in hepatocellular carcinoma patients after surgery. Oncotarget (2016) 7(21):30951–61. doi: 10.18632/oncotarget.9049
Keywords: hepatocellular carcinoma, liver transplantation, biomarkers, systemic inflammatory response index, prognosis
Citation: Cui S, Cao S, Chen Q, He Q and Lang R (2023) Preoperative systemic inflammatory response index predicts the prognosis of patients with hepatocellular carcinoma after liver transplantation. Front. Immunol. 14:1118053. doi: 10.3389/fimmu.2023.1118053
Received: 07 December 2022; Accepted: 14 March 2023;
Published: 27 March 2023.
Edited by:
Xiao Xu, Zhejiang University, ChinaReviewed by:
Jinzhen Cai, The Affiliated Hospital of Qingdao University, ChinaGenshu Wang, Sun Yat-sen University, China
Copyright © 2023 Cui, Cao, Chen, He and Lang. This is an open-access article distributed under the terms of the Creative Commons Attribution License (CC BY). The use, distribution or reproduction in other forums is permitted, provided the original author(s) and the copyright owner(s) are credited and that the original publication in this journal is cited, in accordance with accepted academic practice. No use, distribution or reproduction is permitted which does not comply with these terms.
*Correspondence: Ren Lang, dr_langren@126.com; Qiang He, heqiang349@sina.com
†These authors have contributed equally to this work