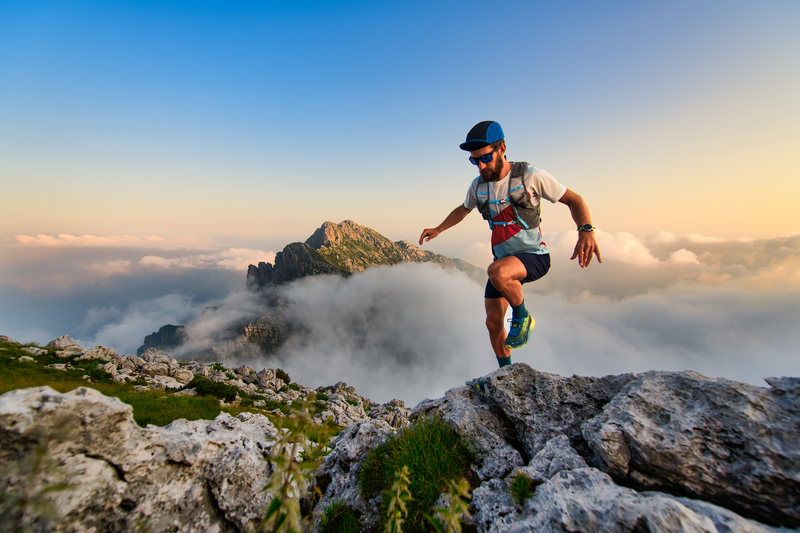
94% of researchers rate our articles as excellent or good
Learn more about the work of our research integrity team to safeguard the quality of each article we publish.
Find out more
PERSPECTIVE article
Front. Immunol. , 29 March 2023
Sec. Vaccines and Molecular Therapeutics
Volume 14 - 2023 | https://doi.org/10.3389/fimmu.2023.1107266
This article is part of the Research Topic Methods in Vaccines and Molecular Therapeutics: 2022 View all 17 articles
The human leukocyte antigen (HLA) proteins are an indispensable component of adaptive immunity because of their role in presenting self and foreign peptides to T cells. Further, many complex diseases are associated with genetic variation in the HLA region, implying an important role for specific HLA-presented peptides in the etiology of these diseases. Identifying the specific set of peptides presented by an individual’s HLA proteins in vivo, as a whole being referred to as the immunopeptidome, has therefore gathered increasing attention for different reasons. For example, identifying neoepitopes for cancer immunotherapy, vaccine development against infectious pathogens, or elucidating the role of HLA in autoimmunity. Despite the tremendous progress made during the last decade in these areas, several questions remain unanswered. In this perspective, we highlight five remaining key challenges in the analysis of peptide presentation and T cell immunogenicity and discuss potential solutions to these problems. We believe that addressing these questions would not only improve our understanding of disease etiology but will also have a direct translational impact in terms of engineering better vaccines and in developing more potent immunotherapies.
The human leukocyte antigen class II (HLA-II) proteins are a class of glycoproteins that are mainly expressed on the surface of antigen presenting cells (APCs) where they present peptides to CD4+ T cells. These peptides are derived from within the APCs itself, e.g. through autophagy (1), or from the cellular microenvironment, e.g. through receptor-mediated endocytosis (2) and macropinocytosis (3). As a result, APCs are able to present self-peptides together with foreign peptides, e.g. pathogens-driven peptides, to T cells. Thus, by identifying and analyzing the set of peptides presented on the surface of APCs, the set of potential T cell epitopes can be narrowed down considerably into a manageable set of peptides and proteins. Nonetheless, HLA-II genes are extremely polymorphic, with most of the observed genetic variants located inside the peptide binding pocket, indicating that different HLA-II alleles encode for proteins that present different peptides, i.e. different alleles result in different immunopeptidomes. Moreover, HLA-II proteins present a diverse set of peptides, hence, the combination of these two factors results in an astronomically large number of peptide HLA-II complexes. Consequently, a lot of effort has been directed toward identifying and characterizing the set of HLA-II presented peptides for a plethora of aims, ranging from investigating autoimmune diseases (4) to neoepitope identification and cancer immunotherapy (5–7).
The rise of MS-based immunopeptidomics during the last decade has revolutionized our ability to identify peptides presented by HLA proteins in vivo. This has led to a “Cambrian explosion” in the field of neoepitope identification where continuous efforts are being directed at improving the experimental (8) and the computational aspects (9–11) of the technology to enable an accurate identification of neoepitopes. For example, Feola et al. (12) have recently described an immunopeptidomics-based pipeline for neoantigen identification and cancer vaccine development. Meanwhile, Chong and colleagues have recently developed NewAnce for identifying non-canonical peptides presented by HLA proteins on tumors (13). Beside identifying presented peptides and neoepitopes, a lot of efforts has been directed at improving the quantification of peptides presented by HLA proteins (14, 15).
MS-immunopeptidomics has been also utilized for vaccine candidate prioritization. Recently, Bettencourt et al. (16) utilized this technology for identifying Mycobacterium tuberculosis (Mtb) presented peptides, subsequently, a proof-of-concept vaccine against Mtb was developed using a subset of the presented peptides. Further, an mRNA-based vaccine against Listeria monocytogenes was recently developed by Mayer and colleagues (17) using an MS-immunopeptidomics guided approach. MS-immunopeptidomics was also utilized for mapping the presentation of SARS-CoV-2 spike glycoprotein proteins by common HLA-DR proteins (18).
Despite the recent progress, deciphering the principles governing peptide generation from potential protein candidates has proven to be an extremely challenging task. A reliable prediction of immunogenicity and immunodominance is therefore still lacking. In addition, the impact of the cellular microenvironment, genetic alterations in the non-HLA components of the peptide presentation machinery, the ageing of the host cells and other environmental factors on peptide presentation by HLA-II proteins is still far from being completely understood. In this perspective, we thus highlight five open questions that are likely to improve our understanding of the biology of HLA-II peptide presentation and its influence on T cell immunogenicity.
Arguably, one of the crucial and, nonetheless, not-completely understood steps in peptide presentation by HLA-II proteins is the loading of HLA-II molecules. Conceptually, loading can be divided into two processes that operate asynchronously. Firstly, peptide generation, and secondly, the actual molecular loading. The former happens through the sequential cleavage of proteins inside the lysosome (19–21) which can be derived from, for example, self-proteins (22, 23), from microbes (16) or from environmental sources such as pollen allergens (24). Proteins in the lysosomes are mainly cleaved using a group of proteases referred to as cathepsins (Cat) (25). Although some cathepsins are ubiquitously expressed in all tissues, e.g. Cat B (26), Cat D (27), and Cat L (28), some show a tissue-restricted expression, for example, Cat S (28) in APCs.
Recent findings have suggested an important role for cathepsins in shaping peptide presentation and immunogenicity. For example, Riese et al. (29) identified a critical role for Cat S in antigen presentation in B cells and dendritic cells. Meanwhile, Dheilly et al. (30) have shown that the same enzyme also regulates antigen presentation by non-Hodgkin Lymphoma. Besides Cat S, Cat L has been shown to be a major regulator of presentation by epithelium cells (31) and in the production of an IgA antibodies against M. pneumonia (32). Cat L has also been shown to impact the specialization of the generated T cell response where treating Leishmania major infected mice with Cat L inhibitors worsen the disease by changing the T cell response from a Th1 to a Th2 response (33). Moreover, inhibiting Cat L in Non-obese diabetic (NOD) mice protected these mice form developing type I diabetes supposedly by increasing the fraction of T regulatory cells in these mice relative to untreated mice (34). These findings illustrate the need to study the influence of cathepsins on HLA-II peptide presentation in depth using recent technological advances in MS-immunopeptidomics and other omics fields. The provided insights would help in establishing a link between the digestive capacity of the cell (i.e. which digestive enzyme is expressed and at what level) and presentation by HLA-II proteins.
Different methods can be used to delineate the role of cathepsins in shaping HLA-II immunopeptidomes such as the combination of mass spectrometry (MS)-based immunopeptidomics and gene-editing methods. This can be accomplished either by knocking down one of the cathepsins in a cell culture and measure its impact on the immunopeptidome and/or by using a mixture of knock-ins and knockouts of different combinations of digestive enzymes and subsequently investigate the impact on the immunopeptidome. Machine-learning methods could then be set up to extract generalizable patterns from these experiments.
Although the experiments proposed above would provide much-needed insights into the role of proteases in shaping HLA-II immunopeptidomes, it is only one piece of the larger puzzle as it only delineates the determinants of the digestive process. Nonetheless, the actual pool of proteins available to these proteases, i.e. the pool of substrate proteins, is also still poorly understood. Recently, Wang et al. (4) have described differences in peptides presented by B cells and monocytes, and argued that differences in the preprocessing machinery and the available proteins to each cell-type might explain this difference. Along these lines, Marcu et al. (35) have revealed qualitative and quantitative differences in the immunopeptidome of different tissues. Further, presentation of viral and tumors proteins has been shown to increase after tagging these proteins for autophagy (36–39), pointing to an important rule for autophagy and the non-HLA components of the presentation machinery in modeling the set of presented peptides. These components can also be influenced by external and internal signals such as inflammation, stress, and calorie restriction (40–42). Therefore, future efforts should focus on deciphering the link among the cellular status, the cellular micro-environment and HLA-II immunopeptidomes, for example, using simultaneous deep multi-omics profiling, e.g. proteomics and transcriptomics, coupled with MS-based immunopeptidomics across different cellular states and across different tissues and organs.
An additional challenge is the extent of variation in the duration of presentation, i.e. the duration a given peptide is presented on the cell surface by an HLA-II protein. Differences in the lifespan, i.e. duration of presentation, of different peptide HLA-I complexes have been proposed to be a major factor shaping the immunogenicity of different peptides (43, 44). For example, Micheletti et al. (45) have identified an important rule for the lifespan of HLA-I peptide complexes and the efficiency of the generated T cell response. Despite the prominent role of presentation dynamics in shaping immunogenicity, it is a poorly understood and characterized process.
Different experimental approaches can be utilized to investigate this problem, for example, by recording the presentation dynamic of a knocked-in gene-of-interest, e.g., specific antigenic proteins or tumor-associated neoantigen with a controllable level of expression. In such systems the link between expression levels and temporal presentation behavior can be delineated by varying either the strength and/or the duration of expression and quantifying the effect on presentation dynamics using immunopeptidomics.
As illustrated above, the immunopeptidome is an extremely complex, dynamic, and only partially understood entity. One of the factors that require further investigation are post-translational modifications (PTMs), for example phosphorylation, acylation or deamination. Such PTM can change the physicochemical interaction between peptides and HLA-proteins (46) as well as the interaction between a peptide-HLA complex and TCRs and, as a result, affect the generated T cell response (47). Indeed, in different autoimmune diseases such as type I diabetes some of the target antigens were found to harbor PTMs, for example, insulin/proinsulin (48) and GRP7 (49).
Multiple challenges render the identification of PTMs in the immunopeptidome troublesome. For example, peptides with PTMs have a low abundance or at least a lower abundance relative to the un-modified peptides (50) (at least this holds true in proteomics). Moreover, some PTMs might interfere with peptide ionization and hence decrease the detectability by MS (50). Besides these experimental challenges, there are computational challenges associated with identifying PTMs. Mainly, the database search which becomes computationally impractical when a large number of PTMs is included (51). Nonetheless, through the development of PTM-aware search engines such as MSFragger (52), and MODa (53), or through the developed of tailored pipelines such as PROMISE (54) progress is being made in addressing this challenging task.
Conceptually, factors shaping the interaction between an APC and a T cell can be broken-down into five interwoven layers (Figure 1): starting with (A) the molecular layer, then (B) the supramolecular layer, followed by (C) the cellular layer, then (D) the micro-environmental layer, and finally (E) the temporal layer (see below).
Figure 1 A hierarchal model of factors influencing or shaping the T cell response. (A) The molecular layer which focuses on HLA-peptide-TCR interaction. Here, the interaction between HLA- DQ 2.2 loaded with a gluten-derived peptide and a TCR is shown. The panel was created using ChimeraX (55), the protein 3D structure was obtained from Protein Data Bank (56) under the identifier 6PX6 (57). (B) The supramolecular layer which describes the impact of co-stimulatory molecules and the count of HLA-P complexes, among others. (C) Describes the impact of the cell types on both sides of the immunological synapse,i.e. the type of APCs and T cells, on shaping the generated T cell response. (D) The CME layer which describes the impact of the cellular micro-environment on shaping T cell response where extra cellular signaling can influence both antigen presentation, T cell priming and subsequently the generated T cell response. (E) The impact of aging on shaping T cell response where the T cell pool becomes dominated by expanded memory T cells and chronological old naïve T cells.
The molecular layer refers to the interaction between an HLA-peptide complex and a TCR. At this level, the sequence-based physicochemical properties of the three molecular components would determine which set of TCRs will recognize a particular peptide-HLA complex. The strength of this interaction also governs different aspects of the generated T cell response, for example, Tubo et al. (58) have recently shown that the strength of TCR-peptide-MHCII interaction have an important role in shaping the differentiation of naïve CD4+ T cells.
The supramolecular layer involves the formation of an immunological synapse (59) between an APC and a T cell where a collection of co-stimulatory molecules and their receptors, along with the TCR and its intracellular signaling components, assemble to form an efficient signaling and communication channel between the two cells. One of the open questions here is the impact of presentation density on T cell activation, i.e. the absolute number of HLA-II proteins presenting the same peptide, and which HLA-II proteins present the peptide to the T cell. Previous work by Irvine et al. (60) illustrated the exceptional sensitivity of CD4+ T cells towards MHC-II molecules where a single MHC-peptide complex is sufficient for driving the T cell response. Hence, a potential future direction is to disentangle the impact of affinity, i.e. the affinity of a particular TCR toward a particular HLA-peptide complex, from the impact of the count, i.e. the number of HLA-peptide complexes.
The cellular level refers to the type of APCs and T cells: although HLA-II molecules are mainly expressed on professional APCs, their expression can be induced on a variety of other cell types by inflammatory cytokines, e.g. INF-γ (61, 62). Additionally, different cell types can act as professional APCs such as dendritic cells and macrophages (63) each of which is also subclassified further into different cell types. For example, dendritic cells are classified into conventional dendritic cells (cDCs) and plasmacytoid dendritic cells, inflammatory dendritic cells and Langerhans cells (64). cDCs are further subclassified into cDC1 and cDC2 (65), each with a different phenotype and function. For example, cDC1 are efficient cross-presenters (65, 66) while cDC2 cells can efficiently prime naïve CD4+ T cells (65, 67, 68). On the other side of the immunological synapse, T cells can exist in different states, for example, naïve, effector, memory, and anergic T cells, each of which have a different antigenic sensitivity (69, 70), and even utilize different metabolic pathways (71). Hence, characterizing the cellular impact on presentation and T cell activation is of paramount importance for a deeper understanding of immunogenicity.
The cellular micro-environment (CME) can play a vital role in shaping either antigen presentation and/or the resulting T cell response. In the former, the CME plays a pivotal role in determining which proteins are available to the presentation machinery along with finetuning the cellular state, e.g. which digestive enzymes are expressed and/or which co-stimulatory molecules are expressed through cellular signaling (72–74). For the T cell response, CME signaling can have a crucial role in shaping the behavior of T cells, e.g. TGF-β and the induction of regulatory T cells (75).
Finally, the temporal level which refers to the (immune system) age of the host along with previous exposure to the same antigen or to a similar antigen. These two factors will likely shape the resulting response greatly by changing the pool of available T cells, e.g. in young individuals the T cell pool is shaped by more dynamic naïve T cells, while in older individuals it is mainly characterized by expanded memory T cells and chronologically older naïve T cells (76). Hence, a deeper characterization of the impact of aging on antigen-presentation and T cell expansion is vital because it might provide strategies to design vaccines that would work on the elderly, which has proven to be a challenging task, too (77, 78).
Recent advances in computational (9, 10) and experimental approaches, e.g. improved sensitivity of MS analysis, are enabling much deeper and more accurate characterization of the immunopeptidome. Nonetheless, we are still far from a complete understanding of all the factors shaping the formation of the immunopeptidome and its dynamics. Furthermore, despite the recent improvement in predicting peptide-presentation in vivo, this improvement has not translated into a reliable in silico prediction of immunogenicity. Indeed, factors governing immunogenicity and immunodominance are still poorly understood. In this perspective we aimed at highlighting gaps in our understanding of peptide presentation and T cell activation, and discussed approaches for further investigation. Coordinated, systematic and large-scale efforts are urgently needed to provide deeper insights into antigen presentation and T cell activation. These insights would help developing more efficient vaccines, better immunotherapies, and also improve our understanding of immunity and host-pathogen interactions more generally.
The original contributions presented in the study are included in the article/supplementary material. Further inquiries can be directed to the corresponding author.
HE and AF conceived the manuscript. HE wrote the manuscript with input from TL, AT, PB and AF. All authors read and approved the final manuscript.
The manuscript was funded by the German Research Foundation DFG Research Unit 5042: miTarget—miTarget—The Microbiome as a Therapeutic Target in Inflammatory Bowel Diseases along with funding from the Cluster of Excellence 2167 “Precision Medicine in Chronic Inflammation (PMI)”. TL was funded by the Deutsche Forschungsgemeinschaft (DFG, German Research Foundation) – 437857095. The funding agencies had no role in the design, collection, analysis, and interpretation of data and neither in writing the manuscript.
Figure 1 was created with BioRender.com.
The authors declare that the research was conducted in the absence of any commercial or financial relationships that could be construed as a potential conflict of interest.
All claims expressed in this article are solely those of the authors and do not necessarily represent those of their affiliated organizations, or those of the publisher, the editors and the reviewers. Any product that may be evaluated in this article, or claim that may be made by its manufacturer, is not guaranteed or endorsed by the publisher.
1. Schuck S. Microautophagy – distinct molecular mechanisms handle cargoes of many sizes. J Cell Sci (2020) 133, jcs246322. doi: 10.1242/jcs.246322
2. Kaksonen M, Roux A. Mechanisms of clathrin-mediated endocytosis. Nat Rev Mol Cell Biol (2018) 19:313–326. doi: 10.1038/nrm.2017.132
3. Liu Z, Roche PA. Macropinocytosis in phagocytes: regulation of MHC class-II-restricted antigen presentation in dendritic cells. Front Physiol (2015) 6. doi: 10.3389/fphys.2015.00001
4. Wang J, Jelcic I, Mühlenbruch L, Haunerdinger V, Toussaint NC, Zhao Y, et al. HLA-DR15 molecules jointly shape an autoreactive T cell repertoire in multiple sclerosis. Cell (2020) 183:1264–81. doi: 10.1016/j.cell.2020.09.054
5. Bassani-Sternberg M, Bräunlein E, Klar R, Engleitner T, Sinitcyn P, Audehm S, et al. Direct identification of clinically relevant neoepitopes presented on native human melanoma tissue by mass spectrometry. Nat Commun (2016) 7, 13404. doi: 10.1038/ncomms13404
6. Chong C, Coukos G, Bassani-Sternberg M. Identification of tumor antigens with immunopeptidomics. Nat Biotechnol (2022) 40:175–188. doi: 10.1038/s41587-021-01038-8
7. Rivero-Hinojosa S, Grant M, Panigrahi A, Zhang H, Caisova V, Bollard CM, et al. Proteogenomic discovery of neoantigens facilitates personalized multi-antigen targeted T cell immunotherapy for brain tumors. Nat Commun (2021) 12, 6689. doi: 10.1038/s41467-021-26936-y
8. Abelin JG, Harjanto D, Malloy M, Suri P, Colson T, Goulding SP, et al. Defining HLA-II ligand processing and binding rules with mass spectrometry enhances cancer epitope prediction. Immunity (2019) 51:766–779.e17. doi: 10.1016/j.immuni.2019.08.012. Elsevier.
9. Bichmann L, Nelde A, Ghosh M, Heumos L, Mohr C, Peltzer A, et al. MHCquant: Automated and reproducible data analysis for immunopeptidomics. J Proteome Res Am Chem Soc (2019) 18:3876–84. doi: 10.1021/acs.jproteome.9b00313
10. ElAbd H, Degenhardt F, Koudelka T, Kamps A-K, Tholey A, Bacher P, et al. Immunopeptidomics toolkit library (IPTK): A python-based modular toolbox for analyzing immunopeptidomics data. BMC Bioinf (2021) 22, 405. doi: 10.1186/s12859-021-04315-0
11. ElAbd H, Degenhardt F, Lenz TL, Franke A, Wendorff M. VCF2Prot: An efficient and parallel tool for generating personalized proteomes from VCF files. bioRxiv (2022). doi: 10.1101/2022.01.21.477084
12. Feola S, Chiaro J, Martins B, Russo S, Fusciello M, Ylösmäki E, et al. A novel immunopeptidomic-based pipeline for the generation of personalized oncolytic cancer vaccines. Elife (2022) 11:e71156. doi: 10.7554/eLife.71156
13. Chong C, Müller M, Pak HS, Harnett D, Huber F, Grun D, et al. Integrated proteogenomic deep sequencing and analytics accurately identify non-canonical peptides in tumor immunopeptidomes. Nat Commun (2020) 11. doi: 10.1038/s41467-020-14968-9
14. Stopfer LE, Mesfin JM, Joughin BA, Lauffenburger DA, White FM. Multiplexed relative and absolute quantitative immunopeptidomics reveals MHC I repertoire alterations induced by CDK4/6 inhibition. Nat Commun (2020) 11, 2760. doi: 10.1038/s41467-020-16588-9
15. Pollock SB, Rose CM, Darwish M, Bouziat R, Delamarre L, Blanchette C, et al. Sensitive and quantitative detection of MHC-I displayed neoepitopes using a semiautomated workflow and TOMAHAQ mass spectrometry. Mol Cell Proteomics (2021) 20, 100108. doi: 10.1016/j.mcpro.2021.100108
16. Bettencourt P, Müller J, Nicastri A, Cantillon D, Madhavan M, Charles PD, et al. Identification of antigens presented by MHC for vaccines against tuberculosis. NPJ Vaccines (2020) 5:2. doi: 10.1038/s41541-019-0148-y.
17. Mayer RL, Verbeke R, Asselman C, Aernout I, Gul A, Eggermont D, et al. Immunopeptidomics-based design of mRNA vaccine formulations against listeria monocytogenes. Nat Commun (2022) 13:6075. doi: 10.1038/s41467-022-33721-y
18. Knierman MD, Lannan MB, Spindler LJ, McMillian CL, Konrad RJ, Siegel RW. The human leukocyte antigen class II immunopeptidome of the SARS-CoV-2 spike glycoprotein. Cell Rep (2020) 33. doi: 10.1016/j.celrep.2020.108454. Elsevier.
19. Neefjes J. CIIV, MIIC and other compartments for MHC class II loading. Eur J Immunol (1999) 29:1421–1425. doi: 10.1002/(SICI)1521-4141(199905)29:05<1421::AID-IMMU1421>3.0.CO;2-C
20. Michelet X, Garg S, Wolf BJ, Tuli A, Ricciardi-Castagnoli P, Brenner MB. MHC class II presentation is controlled by the lysosomal small GTPase, Arl8b. J Immunol (2015) 194:2079–88. doi: 10.4049/jimmunol.1401072
21. Lützner N, Kalbacher H. Quantifying cathepsin s activity in antigen presenting cells using a novel specific substrate*. J Biol Chem (2008) 283:36185–94. doi: 10.1074/jbc.M806500200
22. Nimmerjahn F, Milosevic S, Behrends U, Jaffee EM, Pardoll DM, Bornkamm GW, et al. Major histocompatibility complex class II-restricted presentation of a cytosolic antigen by autophagy. Eur J Immunol (2003) 33:1250–92003. doi: 10.1002/eji.200323730
23. Dengjel J, Schoor O, Fischer R, Reich M, Kraus M, Müller M, et al. Autophagy promotes MHC class II presentation of peptides from intracellular source proteins. Proc Natl Acad Sci Proc Natl Acad Sci (2005) 102:7922–7. doi: 10.1073/pnas.0501190102
24. Blume C, Foerster S, Gilles S, Becker W-M, Ring J, Behrendt H, et al. Human epithelial cells of the respiratory tract and the skin differentially internalize grass pollen allergens. J Invest Dermatol (2009) 129:1935–44. doi: 10.1038/jid.2008.459
25. Yadati T, Houben T, Bitorina A, Shiri-Sverdlov R. The ins and outs of cathepsins: Physiological function and role in disease management. Cells (2020) 9. doi: 10.3390/cells9071679
26. Ni J, Lan F, Xu Y, Nakanishi H, Li X. Extralysosomal cathepsin b in central nervous system: Mechanisms and therapeutic implications. Brain Pathol (2022) 32:e13071. doi: 10.1111/bpa.13071. John Wiley & Sons, Ltd.
27. Hoes M, Tromp J, Ouwerkerk W, Bomer N, Oberdorf-Maass S, Samani N, et al. The role of cathepsin d in the pathophysiology of heart failure and its potentially beneficial properties: A translational approach. Eur J Heart Fail (2019) 22:2102–11. doi: 10.1002/ejhf.1674
28. Mohamed MM, Sloane BF. Multifunctional enzymes in cancer. Nat Rev Cancer (2006) 6:764–75. doi: 10.1038/nrc1949
29. Riese RJ, Mitchell RN, Villadangos JA, Shi GP, Palmer JT, Karp ER, et al. Cathepsin s activity regulates antigen presentation and immunity. J Clin Invest (1998) 101:2351–63. doi: 10.1172/JCI1158. The American Society for Clinical Investigation.
30. Dheilly E, Battistello E, Katanayeva N, Sungalee S, Michaux J, Duns G, et al. Cathepsin s regulates antigen processing and T cell activity in non-Hodgkin lymphoma. Cancer Cell (2020) 37:674–89. doi: 10.1016/j.ccell.2020.03.016
31. Beck H, Schwarz G, Schröter CJ, Deeg M, Baier D, Stevanovic S, et al. Cathepsin s and an asparagine-specific endoprotease dominate the proteolytic processing of human myelin basic protein in vitro. Eur J Immunol (2001) 31:3726–36. doi: 10.1002/1521-4141(200112)31:12<3726::AID-IMMU3726>3.0.CO;2-O. John Wiley & Sons, Ltd.
32. Zhang N, Gao P, Yin B, Li J, Wu T, Kuang Y, et al. Cathepsin l promotes secretory IgA response by participating in antigen presentation pathways during mycoplasma hyopneumoniae infection. PLoS One (2019) 14:e0215408. doi: 10.1371/journal.pone.0215408
33. Onishi K, Li Y, Ishii K, Hisaeda H, Tang L, Duan X, et al. Cathepsin l is crucial for a Th1-type immune response during leishmania major infection. Microbes Infect (2004) 6:468–74. doi: 10.1016/j.micinf.2004.01.008
34. Maehr R, Mintern JD, Herman AE, Lennon-Duménil A-M, Mathis D, Benoist C, et al. Cathepsin l is essential for onset of autoimmune diabetes in NOD mice. J Clin Invest (2005) 115:2934–43. doi: 10.1172/JCI25485. The American Society for Clinical Investigation.
35. Marcu A, Bichmann L, Kuchenbecker L, Kowalewski DJ, Freudenmann LK, Backert L, et al. HLA ligand atlas: A benign reference of HLA-presented peptides to improve T-cell-based cancer immunotherapy. J Immunother Cancer (2021) 9:e002071. doi: 10.1136/jitc-2020-002071
36. Schmid D, Pypaert M, Münz C. MHC class II antigen loading compartments continuously receive input from autophagosomes. Immunity (2007) 26:792007. doi: 10.1016/j.immuni.2006.10.018
37. Jin Y, Sun C, Feng L, Li P, Xiao L, Ren Y, et al. Regulation of SIV antigen-specific CD4+ T cellular immunity via autophagosome-mediated MHC II molecule-targeting antigen presentation in mice. PLoS One (2014) 9:e931432014. doi: 10.1371/journal.pone.0093143
38. Fonteneau JF, Brilot F, Münz C, Gannagé M. The tumor antigen NY-ESO-1 mediates direct recognition of melanoma cells by CD4+ T cells after intercellular antigen transfer. J Immunol (2016) 196:64–71. doi: 10.4049/jimmunol.1402664
39. Münz C. The macroautophagy machinery in MHC restricted antigen presentation. Front Immunol (2021) 12:628429. doi: 10.3389/fimmu.2021.628429
40. Dikic I, Elazar Z. Mechanism and medical implications of mammalian autophagy. Nat Rev Mol Cell Biol (2018) 19:349–64. doi: 10.1038/s41580-018-0003-4
41. He L, Zhang J, Zhao J, Ma N, Kim SW, Qiao S, et al. Autophagy: The last defense against cellular nutritional stress. Adv Nutr (2018) 9:493–504. doi: 10.1093/advances/nmy011
42. Chung KW, Chung HY. The effects of calorie restriction on autophagy: Role on aging intervention. Nutr (2019) 11. doi: 10.3390/nu11122923
43. Levitsky V, Zhang QJ, Levitskaya J, Masucci MG. The life span of major histocompatibility complex-peptide complexes influences the efficiency of presentation and immunogenicity of two class I-restricted cytotoxic T lymphocyte epitopes in the Epstein-Barr virus nuclear antigen 4. J Exp Med (1996) 183:915–26. doi: 10.1084/jem.183.3.915
44. Nelson CA, Petzold SJ, Unanue ER. Peptides determine the lifespan of MHC class II molecules in the antigen-presenting cell. Nature (1994) 371:250–2. doi: 10.1038/371250a0
45. Micheletti B, Canella M, Traniello G. The lifespan of major histocompatibility complex class i/peptide complexes determines the efficiency of cytotoxic T-lymphocyte responses. Immunology (1999) 96:411–5. doi: 10.1046/j.1365-2567.1999.00707.x.
46. Mei S, Ayala R, Ramarathinam SH, Illing PT, Faridi P, Song J, et al. Immunopeptidomic analysis reveals that deamidated HLA-bound peptides arise predominantly from deglycosylated precursors. Mol Cell Proteomics (2020) 19:1236–47. doi: 10.1074/mcp.RA119.001846
47. McGinty JW, Marré ML, Bajzik V, Piganelli JD, James EA. T Cell epitopes and post-translationally modified epitopes in type 1 diabetes. Curr Diabetes Rep (2015) 15:90. doi: 10.1007/s11892-015-0657-7
48. Mannering SI, Harrison LC, Williamson NA, Morris JS, Thearle DJ, Jensen KP, et al. The insulin a-chain epitope recognized by human T cells is posttranslationally modified. J Exp Med (2005) 202:1191–7. doi: 10.1084/jem.20051251. 2005/10/31The Rockefeller University Press.
49. Wicker LS, Chen SL, Nepom GT, Elliott JF, Freed DC, Bansal A, et al. Naturally processed T cell epitopes from human glutamic acid decarboxylase identified using mice transgenic for the type 1 diabetes-associated human MHC class II allele, DRB1*0401. J Clin Invest (1996) 98:2597–603. doi: 10.1172/JCI119079
50. Larsen M, Trelle M, Thingholm T, Jensen O. Analysis of posttranslational modifications of proteins by tandem mass spectrometry. Biotechniques (2006) 40:790–8. doi: 10.2144/000112201
51. Yuan Z-F, Lin S, Molden RC, Garcia BA. Evaluation of proteomic search engines for the analysis of histone modifications. J Proteome Res (2014) 13:4470–8. doi: 10.1021/pr5008015
52. Yu F, Teo GC, Kong AT, Haynes SE, Avtonomov DM, Geiszler DJ, et al. Identification of modified peptides using localization-aware open search. Nat Commun (2020) 11:4065. doi: 10.1038/s41467-020-17921-y
53. Na S, Bandeira N, Paek E. Fast multi-blind modification search through tandem mass spectrometry. Mol Cell Proteomics (2012) 11:M111.010199. doi: 10.1074/mcp.M111.010199
54. Kacen A, Javitt A, Kramer MP, Morgenstern D, Tsaban T, Solomon A, et al. Uncovering the modified immunopeptidome reveals insights into principles of PTM-driven antigenicity. bioRxiv (2021). doi: 10.1101/2021.04.10.438991
55. Pettersen EF, Goddard TD, Huang CC, Meng EC, Couch GS, Croll TI, et al. UCSF ChimeraX: Structure visualization for researchers, educators, and developers. Protein Sci (2021) 30:70–82. doi: 10.1002/pro.3943
56. Berman HM, Westbrook J, Feng Z, Gilliland G, Bhat TN, Weissig H, et al. The protein data bank. Nucleic Acids Res (2000) 28:235–42. doi: 10.1093/nar/28.1.235
57. Tian TY, Shiva D-K, Keshia KHS, Shuo-Wang Q, Neumann RS, Lundin KEA, et al. A molecular basis for the T cell response in HLA-DQ2.2 mediated celiac disease. Proc Natl Acad Sci (2020) 117:3063–73. doi: 10.1073/pnas.1914308117. Proceedings of the National Academy of Sciences.
58. Tubo NJ, Pagán AJ, Taylor JJ, Nelson RW, Linehan JL, Ertelt JM, et al. Single naive CD4+ T cells from a diverse repertoire produce different effector cell types during infection. Cell (2013) 53:758–96. doi: 10.1016/j.cell.2013.04.007
59. Dustin ML. The immunological synapse. Cancer Immunol Res (2014) 2:1023–33. doi: 10.1158/2326-6066.CIR-14-0161
60. Irvine DJ, Purbhoo MA, Krogsgaard M, Davis MM. Direct observation of ligand recognition by T cells. Nature (2002) 419:845–9. doi: 10.1038/nature01076
61. Thelemann C, Eren RO, Coutaz M, Brasseit J, Bouzourene H, Rosa M, et al. Interferon-γ induces expression of MHC class II on intestinal epithelial cells and protects mice from colitis. PLoS One (2014) 9:e86844–e86844. doi: 10.1371/journal.pone.0086844
62. Helbig H, Kittredge KL, Palestine AG, Coca-Prados M, Nussenblatt RB. Gamma-interferon induces differential expression of HLA-DR, -DP and -DQ in human ciliary epithelial cells. Graefe’s Arch Clin Exp Ophthalmol (1991) 229:191–4. doi: 10.1007/BF00170556
63. Gaudino SJ, Kumar P. Cross-talk between antigen presenting cells and T cells impacts intestinal homeostasis, bacterial infections, and tumorigenesis. Front Immunol (2019) 10. doi: 10.3389/fimmu.2019.00360
64. Balan S, Saxena M, Bhardwaj N. Chapter one - dendritic cell subsets and locations. In: Lhuillier C, Galluzzi L, editors. Immunobiol dendritic cells part a. (2019) Amsterdam: Academic Press.
65. Sasaki I, Kato T, Hemmi H, Fukuda-Ohta Y, Wakaki-Nishiyama N, Yamamoto A, et al. Conventional type 1 dendritic cells in intestinal immune homeostasis. Front Immunol (2022) 13. doi: 10.3389/fimmu.2022.857954
66. Heath WR, Carbone FR. Cross-presentation, dendritic cells, tolerance and immunity. Annu Rev Immunol (2001) 19:472001. doi: 10.1146/annurev.immunol.19.1.47
67. Kumamoto Y, Linehan M, Weinstein JS, Laidlaw BJ, Craft JE, Iwasaki A. CD301b+ dermal dendritic cells drive T helper 2 cell-mediated immunity. Immunity (2013) 39:733–432013. doi: 10.1016/j.immuni.2013.08.029
68. Plantinga M, Guilliams M, Vanheerswynghels M, Deswarte K, Branco-Madeira F, Toussaint W, et al. Conventional and monocyte-derived CD11b+ dendritic cells initiate and maintain T helper 2 cell-mediated immunity to house dust mite allergen. Immunity (2013) 38:322–352013. Elsevier. doi: 10.1016/j.immuni.2012.10.016
69. Grayson JM, Harrington LE, Lanier JG, Wherry EJ, Ahmed R. Differential sensitivity of naive and memory CD8+ T cells to apoptosis in vivo. J Immunol (2002) 169:3760–70. doi: 10.4049/jimmunol.169.7.3760
70. Kumar R, Ferez M, Swamy M, Arechaga I, Rejas MT, Valpuesta JM, et al. Increased sensitivity of antigen-experienced T cells through the enrichment of oligomeric T cell receptor complexes. Immunity (2011) 35:375–87. doi: 10.1016/j.immuni.2011.08.010
71. Gerriets VA, Rathmell JC. Metabolic pathways in T cell fate and function. Trends Immunol (2012) 33:168–73. doi: 10.1016/j.it.2012.01.010
72. Kobie JJ, Wu RS, Kurt RA, Lou S, Adelman MK, Whitesell LJ, et al. Transforming growth factor β inhibits the antigen-presenting functions and antitumor activity of dendritic cell vaccines. Cancer Res (2011) 63:1860–42003.
73. Strobl H, Knapp W. TGF-β1 regulation of dendritic cells. Microbes Infect (1999) 1:1283–90. doi: 10.1016/S1286-4579(99)00256-7
74. Oh J, Shin J-S. Molecular mechanism and cellular function of MHCII ubiquitination. Immunol Rev (2015) 266:134–44. doi: 10.1111/imr.12303
75. Wan YY, Flavell RA. ‘Yin–yang’ functions of transforming growth factor-β and T regulatory cells in immune regulation. Immunol Rev (2007) 220:199–213. doi: 10.1111/j.1600-065X.2007.00565.x.
76. Salam N, Rane S, Das R, Faulkner M, Gund R, Bughani U, et al. T Cell ageing: Effects of age on development, survival & function. Indian J Med Res (2013) 138:595–6082013.
77. Ciabattini A, Nardini C, Santoro F, Garagnani P, Franceschi C, Medaglini D. Vaccination in the elderly: The challenge of immune changes with aging. Semin Immunol (2018) 40:83–94. doi: 10.1016/j.smim.2018.10.010
Keywords: HLA-II & autoimmunity, immunogenicitiy, TCR - T cell receptor, mass-spectrometry (MS) based immunopeptidomics, neoantigen, immunotherapy
Citation: ElAbd H, Bacher P, Tholey A, Lenz TL and Franke A (2023) Challenges and opportunities in analyzing and modeling peptide presentation by HLA-II proteins. Front. Immunol. 14:1107266. doi: 10.3389/fimmu.2023.1107266
Received: 24 November 2022; Accepted: 13 March 2023;
Published: 29 March 2023.
Edited by:
P Bernard Fourie, University of Pretoria, South AfricaReviewed by:
Jinghua Lu, National Institute of Allergy and Infectious Diseases (NIH), United StatesCopyright © 2023 ElAbd, Bacher, Tholey, Lenz and Franke. This is an open-access article distributed under the terms of the Creative Commons Attribution License (CC BY). The use, distribution or reproduction in other forums is permitted, provided the original author(s) and the copyright owner(s) are credited and that the original publication in this journal is cited, in accordance with accepted academic practice. No use, distribution or reproduction is permitted which does not comply with these terms.
*Correspondence: Andre Franke, YS5mcmFua2VAaWttYi51bmkta2llbC5kZQ==
Disclaimer: All claims expressed in this article are solely those of the authors and do not necessarily represent those of their affiliated organizations, or those of the publisher, the editors and the reviewers. Any product that may be evaluated in this article or claim that may be made by its manufacturer is not guaranteed or endorsed by the publisher.
Research integrity at Frontiers
Learn more about the work of our research integrity team to safeguard the quality of each article we publish.