- 1Department of Radiation Oncology, Nanfang Hospital, Southern Medical University, Guangzhou, China
- 2Department of Radiation Oncology, Sun Yat-sen University Cancer Center, State Key Laboratory of Oncology in South China, Collaborative Innovation Center for Cancer Medicine, Guangdong Key Laboratory of Nasopharyngeal Carcinoma Diagnosis and Therapy, Guangzhou, China
- 3Department of Radiation Oncology, The First Affiliated Hospital, Sun Yat-Sen University, Guangzhou, China
Purpose: Head and neck squamous cell carcinoma (HNSCC) ranks sixth among all cancers globally regarding morbidity, and it has a poor prognosis, high mortality, and highly aggressive properties. In this study, we established a model for predicting prognosis based on immunohistochemical (IHC) scores.
Methods: Data on 402 HNSCC cases were collected, the glmnet Cox proportional hazards model was used, risk factors were analyzed for predicting the prognosis of survival, and the IHC score was established. We used the IHC score to predict disease-free survival (DFS) using training and independent validation cohorts, including 264 cases in total. Additionally, the accuracy of the IHC score and the TNM system (8th edition) was compared. A DFS prediction nomogram was established by combining the prognostic factors.
Results: The IHC scores included CK, Ki-67, p16, and p40 staining intensity. The concordance index and the Kaplan-Meier survival analysis showed that the IHC scores had high predictive power for HNSCC. Our results showed that the IHC score is an independent factor that can predict prognosis in a multivariate Cox regression analysis. When predicting DFS, the IHC score had a significantly higher value for the area under the ROC curve (AUC) than that of the TNM system. A nomogram was established and included the IHC score, age, tumor location, and the TNM stage. The calibration curves exhibited high consistency between the prognosis predicted by our nomogram and the actual prognosis.
Conclusions: The IHC score was more accurate than the eighth edition of the TNM system in predicting HNSCC prognosis. Therefore, combining the two methods can facilitate individualized patient consultation and care.
Introduction
Head and neck squamous cell carcinoma (HNSCC) includes nasopharyngeal cancer, oral cavity and oropharyngeal cancer, as well as laryngeal and hypopharyngeal cancer (1, 2). HNSCC is mainly treated by surgical resection, chemotherapy, and radiotherapy (3). Despite the development of comprehensive therapeutic strategies, a high recurrence rate and metastasis hinder the effective treatment of HNSCC (4). Therefore, early prognostic prediction and subsequent individualized treatment strategies are ideal for treating HNSCC patients. HNSCC is treated, and its prognosis is predicted mainly using the TNM classification system (8th edition) published by the American Joint Committee on Cancer (AJCC) (5). However, individual heterogeneity causes inaccurate prognosis prediction (6). Therefore, more accurate predictors are required.
As an inexpensive and convenient pathological technique, immunohistochemistry (IHC) is commonly used to analyze the occurrence, development, and invasiveness of HNSCC. Many studies have shown that IHC is an efficient approach for predicting survival in cancer patients. For example, cytokeratin (CK) is a specific marker of oral squamous epithelial cells and has a poor prognosis with oral squamous cell carcinoma (7, 8). Ki-67 is an immunohistochemical marker of cell proliferation and a predictor of local recurrence in papillary thyroid cancer (9). The protein p16Ink4a (p16) is a tumor suppressor protein that is underexpressed in different types of cancers, like bladder cancer, breast cancer, sarcoma, glioblastoma, lung cancer, colonic cancer, and hematologic neoplasms (10–12). The p16 protein is associated with a favorable prognosis (13). The p40 protein is an important prognostic factor for meningioma recurrence (14). The p53 protein is a ubiquitous protein in humans and a well-known tumor suppressor gene. Mutation or overexpression of the p53 gene is quite common in HNSCC (15, 16), but the prognostic significance of p53 is debatable (17, 18). The immunohistochemical marker p63 is a myoepithelial marker (19). The overexpression of p63 is associated with poor prognosis in oral cancer (20). Although IHC markers can predict tumor prognosis, a model based on multiple independent IHC prognostic factors is still lacking in HNSCC.
Therefore, in this study, we used the lmnet Cox proportional hazards model for constructing an IHC score to predict DFS in HNSCC patients. The predictive capacity of IHC score was validated using the concordance index (C-index) and by performing the Kaplan-Meier survival analysis. Additionally, a nomogram for the combined IHC score and other clinicopathological risk factors was established. This nomogram might be used for guiding management decisions in patients with HNSCC.
Materials and methods
Patients
From January 2009 to June 2014, the data on 666 patients with de novo HNSCC were collected from Sun Yat-Sen University Cancer Center. The inclusion criteria were: 1. Histopathologically confirmed non-metastatic HNSCC patients. 2. No evidence of tumors of other origins or other serious diseases. The exclusion criteria were: 1. Without tissue sections available for further immunohistochemistry staining. 2. Insufficient follow up data. The cases were randomized as training (n = 402) and validation (n = 264) cohorts at a ratio of 3:2. The flowchart for patient eligibility is presented in Figure 1. Information on age, sex, drinking status, smoking status, and tumor location of all patients was obtained from the clinical data. We re-recorded tumor staging for each patient using the TNM classification system (8th edition). The Institutional Review Board of Sun Yat-Sen University Cancer Center approved this study. No informed consent was required since we examined anonymous data.
Follow up
In this study, the disease-free survival (DFS) was considered to be the primary endpoint, which indicated the duration between the initial diagnosis date and the relapse or final follow-up date. All patients visited the clinic for follow-up at three-month intervals for the first 1-2 years, six-month intervals for the next 3-5 years, and one-year intervals thereafter till the last follow-up or death.
IHC staining and evaluation
The IHC assays of all patients were conducted at the Department of Pathology in our center. All specimens were tissue sections that were fixed in formalin and embedded in paraffin. The primary antibodies used were pan-cytokeratin (CK) (Santa Cruz, 1:500), Ki-67 (Dako, 1:100), p16 (Ventana, 1:500), p53 (Ventana, 1:500), p40 (Biocare, 1:100), and p63 (Dako, 1:100). The secondary antibody reagent used was a True Envision HRP Rabbit/Mouse Detection System (Dako Real Envision Systems). The sections were prepared using the 3,3’-diaminobenzidine stain, hematoxylin was used for control staining, and the rabbit immunoglobulin G (IgG; r&, d, Germany) or mouse IgG (Dako Cytomation, Glostrup, Denmark) was used as a negative control. The results of the IHC assay for all markers were semiquantitatively evaluated by two trained pathologists independently in a blinded manner. The staining intensity was categorized as positive (moderate/strong staining) or negative (weak/no staining), which depended on the reactivity of the IHC marker.
Establishment and validation of the IHC score
The data from 402 HNSCC cases were used as the training cohort to establish the IHC score. We used the “glmnet” package in the R software to determine the optimal model based on the Cox proportional hazards model under lasso penalty with 1,000 iterations (21). Then, the IHC score was established using the as-constructed optimal gene model. Later, the prediction ability of the signatures in the training and independent validation cohorts (264 cases) was verified by the concordance index (C-index) proposed by Harrell et al. (22).
The threshold for the low and high IHC score groups was determined based on the ROC survival analysis. Moreover, for graphically presenting the DFS of the low and high IHC score groups, we plotted the Kaplan-Meier (K-M) curves. The Cox regression analysis was performed to determine the prognostic significance of the IHC score.
Establishing and assessing an IHC score-based nomogram
Using the rms package in R, the IHC score-based nomograms were established based on all the identified factors that independently predicted prognosis. The C-index (with a 95% confidence interval) was used for determining the nomogram prediction performance of the training and validation cohorts. One-year and three-year DFS calibration plots were generated to compare the predicted DFS with the actual DFS. The IBM SPSS25.0 and the R software (version 4.2.0) were used for performing statistical analyses.
Results
Patient characteristics
We placed 402 and 264 cases into the training and validation cohorts, respectively. For the two cohorts, the ages were 20–89 (median = 59) and 18–90 (median = 58) years, respectively. The patients were followed up for 3–198 (median = 30) months, and 281 individuals experienced relapse or death. The three-year DFS rates of the two cohorts were 62.1% and 61.7%, respectively. The comparison of the baseline features between the two cohorts is presented in Table 1; the baseline features were similar in the training and validation cohorts. The representative immunohistochemical sections of the six IHC signatures (CK, Ki-67, p16, p40, p53, and p63) are shown in Figure 2.
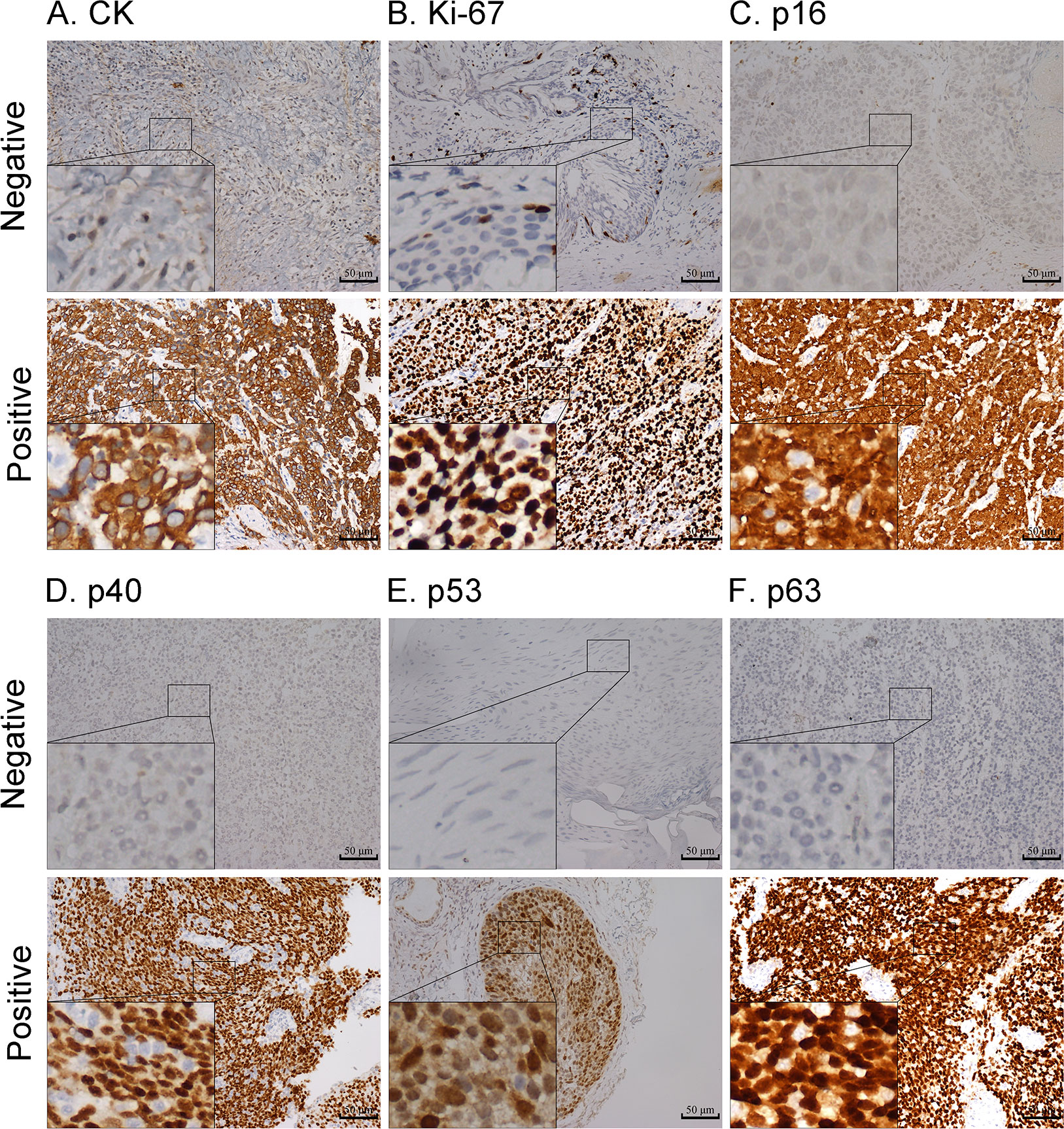
Figure 2 Representative images of negative and positive expression of CK (A), Ki-67 (B), p16 (C), p40 (D), p53 (E), and p63 (F) markers; magnification: 200x. Highly magnified images (800x) are shown in the inset (scale bar = 50 µm).
Construction of the IHC score
The Cox proportional hazards regression model was applied to six IHC markers (CK, Ki-67, p16, p40, p53, and p63) under 10-fold cross-validation to generate the optimal model. A total of 1,000 iterations were conducted, including three models for further screening. As shown in Figure 3A, the model with four IHC markers (CK, Ki-67, p16, and p40) had the highest (n = 688) frequency. This model was considered to be the optimal model for generating the IHC score regarding HNSCC. Thus, four IHC markers in this model were adopted to construct the IHC score. The coefficients of CK, Ki-67, p16, and p40 obtained from the LASSO Cox regression were used to construct the formula of IHC score:
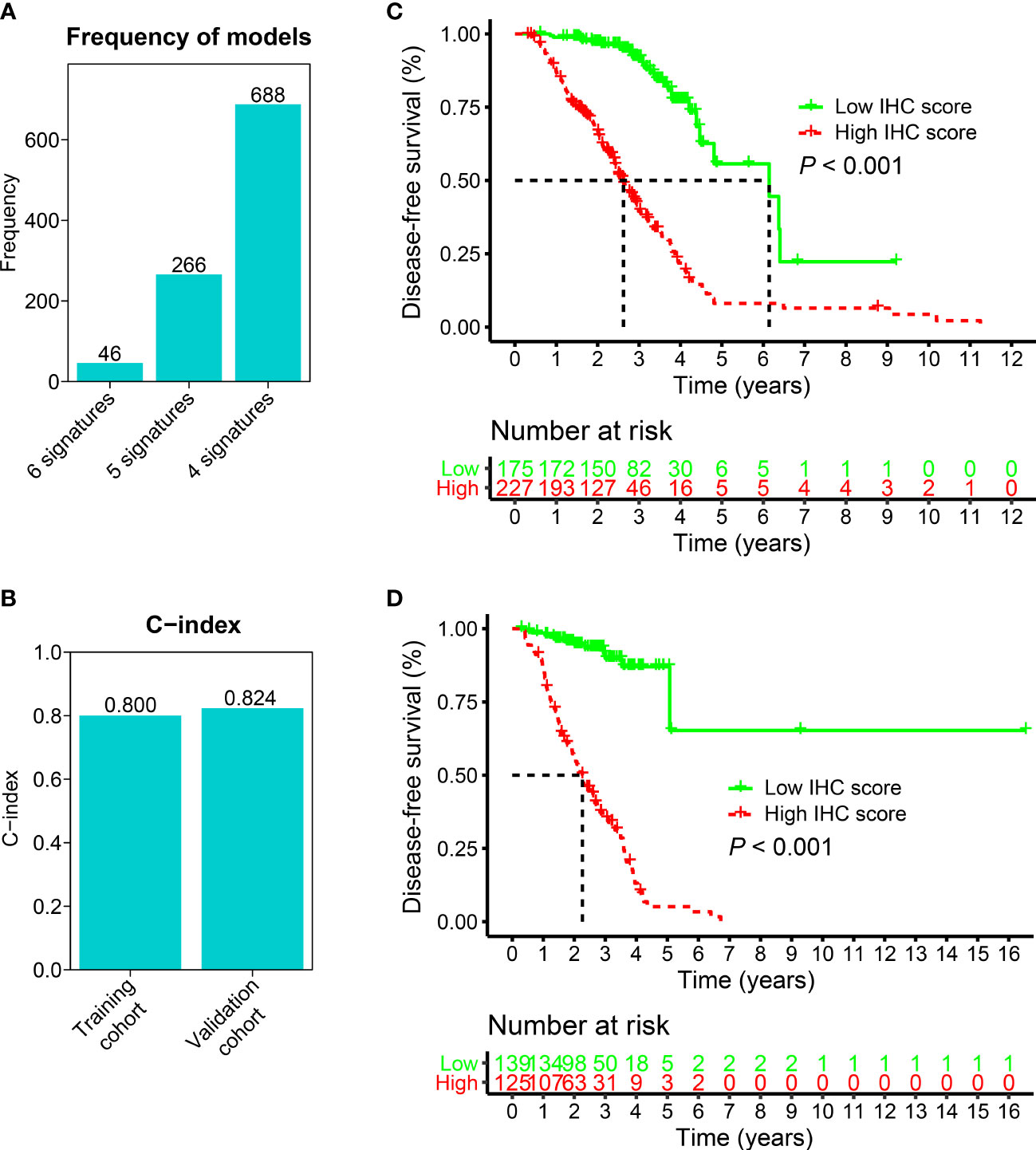
Figure 3 The construction and validation of the IHC score. (A) Generation of three models after 1,000 iterations. The model consisting of four IHC markers and with the highest frequency (688) was used to build the IHC score. 6 signatures: CK, Ki-67, p16, p40, p53, and p63; 5 signatures: CK, Ki-67, p16, p40, p53; 4 signatures: CK, Ki-67, p16, and p40. (B) For training and validation cohorts, the C-index values were 0.800 and 0.824, respectively. (C, D) K-M analyses were conducted on the IHC scores for the training and validation cohorts. In the training (C) and validation (D) cohorts, patients who had higher IHC scores showed poorer DFS, which suggested that the IHC score was a strong predictor of survival outcomes in the HNSCC patients (p< 0.001).
In the above formula, the IHC staining was scored as negative (score 0), and positive (score 1).
Validation of the IHC score
To validate the IHC score, we predicted the DFS by calculating the C-index. The results showed that the C-index values associated with the IHC score for training and validation cohorts were 0.800 and 0.824, respectively (p< 0.05, Figure 3B). This indicated that the IHC score had high predictive accuracy for survival. Then, based on the ROC threshold, all cases were classified into low and high IHC score groups. Patients with high IHC scores showed a lower DFS than those with low IHC scores in both the training and validation cohorts (Figures 3C, D). The results of the univariate analysis of IHC scores also revealed that the IHC scores were significantly related to the DFS for the HNSCC cases in both training and validation cohorts (p< 0.05, Figure 4). The results of the multivariate Cox regression analysis showed that the IHC score served as an independent factor for predicting the patient prognosis after clinicopathological characteristics like age, sex, smoking history, drinking history, tumor location, and the TNM stage were adjusted in the training cohort [Hazard ratio (HR) = 8.395, 95% confidence intervals (CI): 4.730–14.900, P< 0.001] and the validation cohort (HR = 13.613, 95% CI: 6.352–29.171, P< 0.001) (Figure 4).
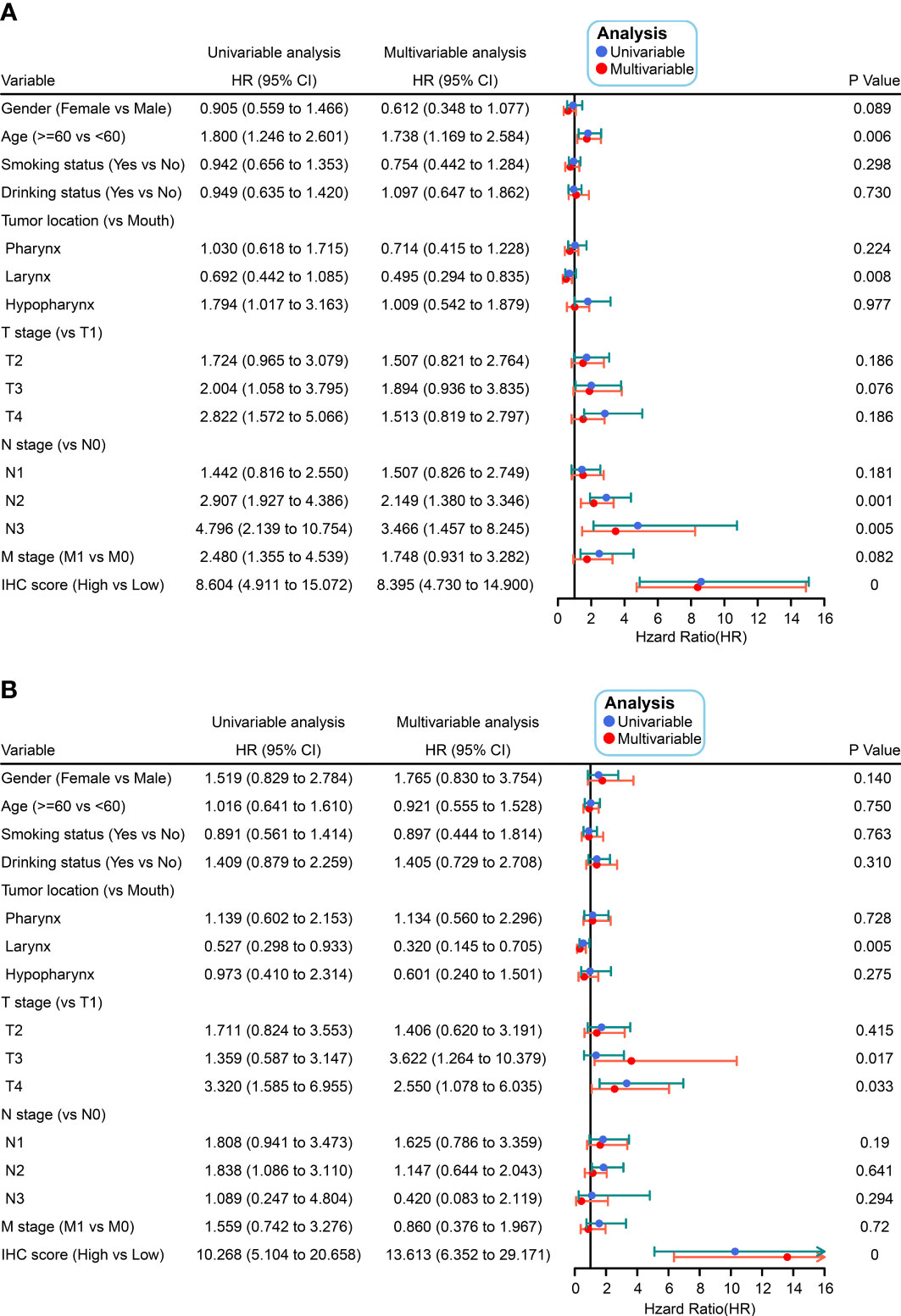
Figure 4 Univariate and multivariate Cox analyses were conducted on IHC scores as a function of clinicopathological characteristics in the training (A) and validation (B) cohorts.
Comparison of the IHC score with the TNM system
The prediction accuracy of the IHC score and the TNM system (8th edition) was compared for the DFS between the two cohorts. The values of the area under the ROC curve (AUC) for the IHC score related to the prediction of the one-year and three-year DFS were 0.787/0.857 and 0.775/0.817 for the training and validation cohorts, respectively, which were considerably higher than those of the TNM stage (Figure 5). The results showed that the prediction accuracy and the discriminative power of the IHC score for DFS were significantly higher than that of the TNM system.
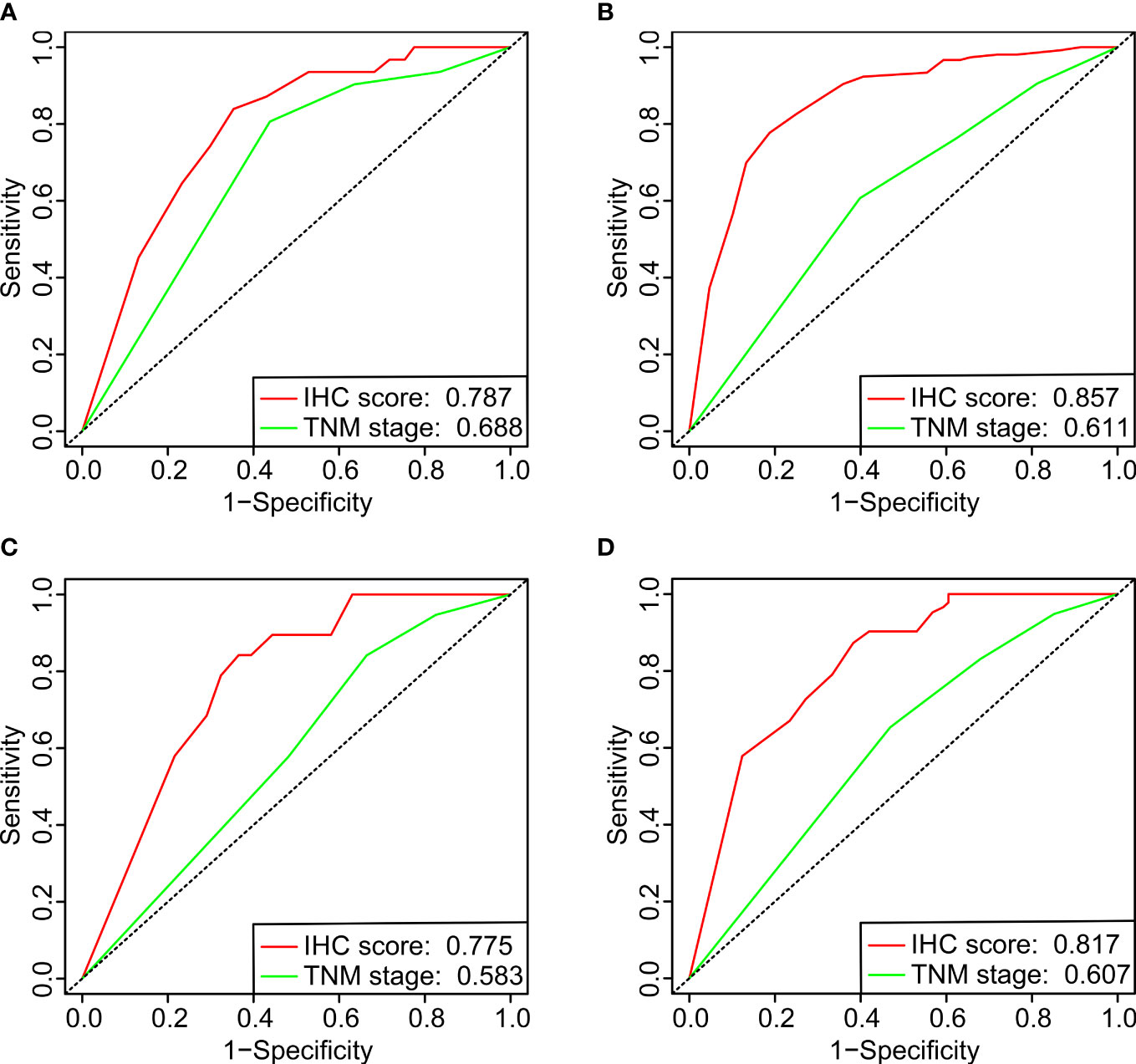
Figure 5 The ROC curves of one-year (A, C) and three-year (B, D) DFS for comparing the IHC scores with the UICC/AJCC TNM classification system (8th edition) in the training (A, B) and validation (C, D) cohorts.
Establishment and verification of a nomogram
We established a nomogram by incorporating key prognostic factors, including the IHC score, age, tumor location, T stage, N stage, and M stage, to provide a quantitative tool for clinically predicting the probability of DFS in HNSCC patients (Figure 6A). The C-index values for predicting the DFS were estimated to be 0.803 and 0.800 (95% CI: 0.768–0.838 and 0.747–0.853), respectively, for the training and validation cohorts. The C-index in all the cohorts was greater than 0.7, indicating that the model was satisfactory. Calibration plots for the one-year and three-year DFS probabilities also showed significant correspondence between predictions and observations for all cohorts (Figures 6B, C).
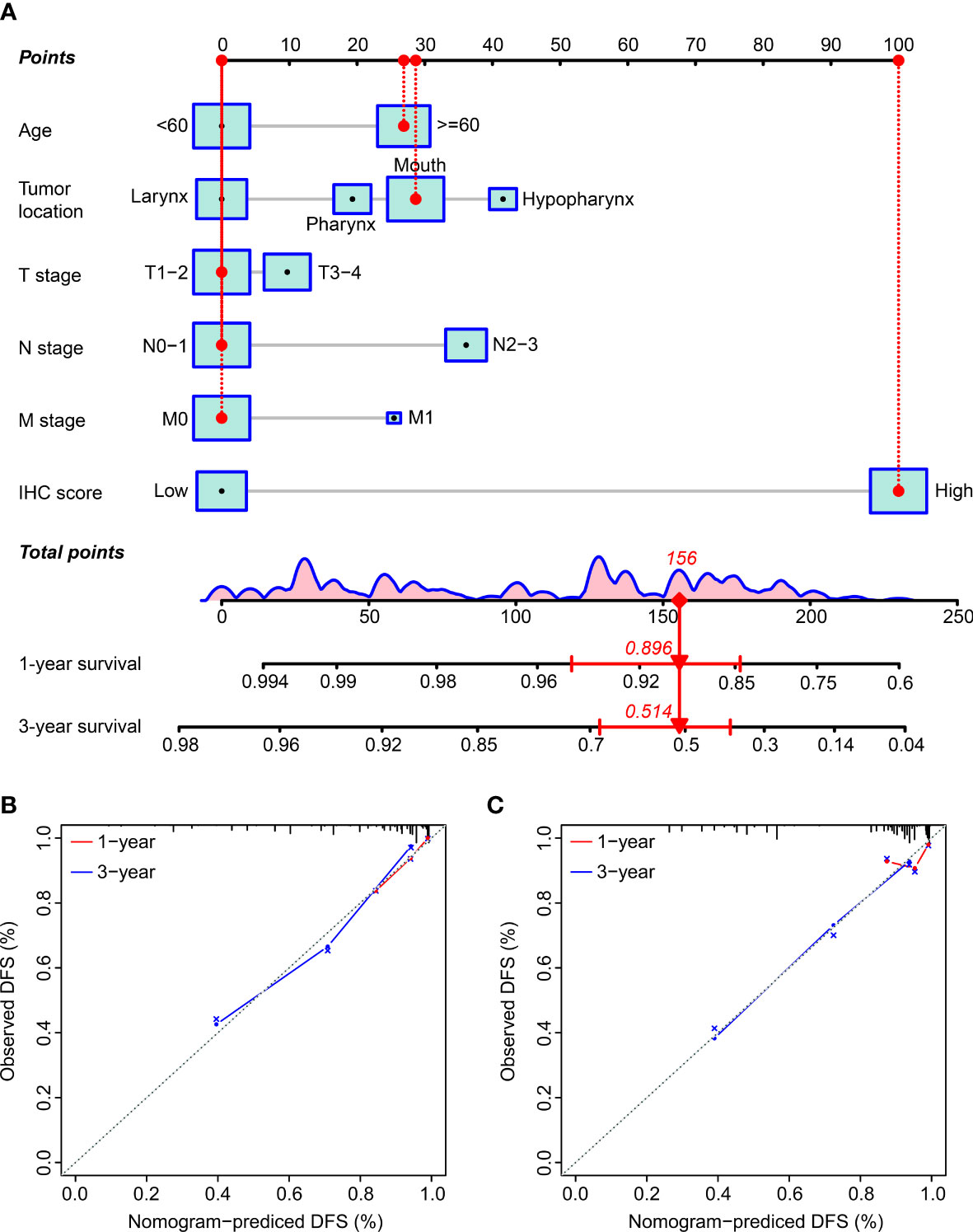
Figure 6 The establishment and verification of the nomogram. (A) The nomogram incorporated the IHC score and clinicopathological factors. (B, C) The predictive power of the nomogram was assessed for the training (B) and validation cohorts (C) based on the one-year and three-year DFS using the calibration curves. The dashed line below the line at an angle of 45° indicates the equivalent nomogram-predicted probability and the measured counterpart.
Discussion
HNSCC shows high aggressiveness and poor prognosis. Significant progress has been made in elucidating the mechanism related to the pathogenesis of HNSCC, but molecular biomarkers are unavailable for prognosis prediction in the clinic. Thus, we focused on identifying an IHC classifier capable of predicting the prognosis in HNSCC patients. We conducted IHC staining for six immunohistochemical markers (CK, Ki-67, p16, p40, p53, and p63) in paraffin-embedded tumor sections of HNSCC patients. Four markers (CK, Ki-67, p16, and p40) were selected to derive the formula of the IHC score. In prognostic analysis, we found a higher three-year DFS rate in the low IHC score group than in the high IHC score group, which suggested that an increase in the IHC score was associated with poor prognosis. The IHC score, along with age, gender, drinking status, smoking status, TNM stage, and tumor location, were independent prognostic factors in the multivariate Cox model.
Based on previous studies, these IHC markers (CK, Ki-67, p16, and p40) have been implicated in tumor development and progression. Cytokeratins (CKs) are epithelial-specific intermediate filament proteins associated with cell adhesion and migration (23, 24). There is evidence that CKs play an oncogenic role in the proliferation and metastasis of cancer cells. As reported by Jang TH, et al, CK17 activates integrin β4/α6 and increases FAK, Src, and ERK phosphorylation in oral cancer cells to enhance cancer stemness (25). Human melanoma and breast cancer cells with high expression of CK8 and CK18 exhibit higher migration and invasion ability (7, 26). Similarly, our research showed that patients with CK expression had worse survival outcomes than those with low CK expression. Known as the most reliable proliferation marker, Ki-67 is a nuclear non-histone protein that is detected in actively proliferating normal and neoplastic cells (27). Ki-67 immunohistochemistry is considered an important prognostic indicator in oesophageal, lung, kidney, and breast carcinomas (28), which is consistent with our results. The cyclin-dependent kinase inhibitor p16 decreases pRb phosphorylation and inhibits cell cycle progression from G1 to S (29). Deficiency of p16 is a potential druggable target. Overexpression of p16 induced by recombinant adenovirus has significant antitumor effects on human HNSCC (30). In our study, p16 was found to be associated with a better prognosis in HNSCC patients, which is consistent with the findings of these previous studies. ΔNp63 detected by p40 antibody serves as a specific marker for the differentiation of squamous epithelial cells (31). The anti-p40 antibody has gained diagnostic utility in recent years for distinguishing head and neck sarcomatoid squamous cell carcinoma from mesenchymal tumors (32). Several studies demonstrates that ΔNp63 plays a pro-tumorigenic role. For instance, ΔNp63 enhances Wnt signaling by increasing the expression of the Wnt receptor Fzd7, and thereby promotes normal mammary stem cell activity (33). Another major function of ΔNp63 is to inhibit the activity of some tumor suppressor genes, such as p53, TAp63 and TAp73 (34, 35). In line with previous studies, our results showed that HNSCC patients with high p40 expression exhibited poor outcomes. In addition to playing significant roles in tumor initiation and progression, these genes have also been associated with patient outcomes, which makes them suitable for developing a prediction model for prognostic stratification of HNSCC patients. Previous studies have mainly used RNA sequencing or gene expression microarray data to build models. Unlike previous studies, we used immunohistochemical data to construct a prognostic model based on the expression levels of these genes. As one of the most commonly used and convenient methods, immunohistochemical analyses would greatly contribute to the utility and generalizability of the model.
After evaluation of these IHC markers, our results suggested that the IHC score based on the combined expression of the four markers (CK, Ki-67, p16, and p40) can predict survival in HNSCC patients. Kaplan-Meier survival curves supported the strong ability of the IHC score to distinguish cases with high recurrence risk from those with low recurrence risk. Moreover, the IHC score was determined by the univariate analysis along with the multivariate Cox regression analysis to be an independent prognostic factor. Additionally, the ROC analysis showed that the IHC score had stronger predictive power than the TNM stage. Finally, a nomogram was constructed, based on the IHC score, along with additional critical clinicopathological characteristics, for accurately predicting survival in HNSCC patients. After evaluating the calibration curve and the C-index, the results showed that the performance of the nomogram was good.
Our model had several advantages. First, the large study population reinforced the reliability of the results. Second, the IHC scores and other clinical features incorporated into the nomogram were inexpensive and readily available markers. Finally, the nomogram can be used to calculate individualized prognostic estimates without complex mathematical calculations, further demonstrating the clinical utility of the model.
However, our study had some limitations. First, the data were collected from a single institution. Larger studies are needed to confirm our results. Another limitation was that we conducted a retrospective analysis, which might have introduced some bias in the study. Further prospective studies are required to verify the hypothesis.
Conclusions
We demonstrated that the IHC score based on four immunohistochemical markers, including CK, Ki-67, p16, and p40, was significantly associated with prognosis in HNSCC patients. A nomogram integrating the IHC scores and multiple clinical factors can be a powerful tool for evaluating clinical outcomes in patients with HNSCC.
Data availability statement
The raw data supporting the conclusions of this article will be made available by the authors, without undue reservation.
Ethics statement
This study was approved by The Institutional Review Board of Sun Yat-Sen University Cancer Center.
Author contributions
Study conception and design: Q-QX, Q-JL, and XW. Data acquisition: Q-QX, Q-JL, ZX, L-LL, ZH and JL. Data analysis and interpretation: Q-QX, Q-JL, and ZX. Quality control of data and algorithms: LXL, Y-YC, R-ZC, and XW. Manuscript writing: Q-QX, Q-JL, and XW. Manuscript reviewing and approving: LXL, Y-YC, R-ZC, and XW. All authors have read and agreed to the published version of the manuscript. All authors contributed to the article and approved the submitted version.
Funding
This research was funded by the National Natural Science Foundation of China (82003214) and Guangdong Basic and Applied Basic Research Foundation (2019A1515110076).
Conflict of interest
The authors declare that the research was conducted in the absence of any commercial or financial relationships that could be construed as a potential conflict of interest.
Publisher’s note
All claims expressed in this article are solely those of the authors and do not necessarily represent those of their affiliated organizations, or those of the publisher, the editors and the reviewers. Any product that may be evaluated in this article, or claim that may be made by its manufacturer, is not guaranteed or endorsed by the publisher.
References
1. Hunter KD, Parkinson EK, Harrison PR. Profiling early head and neck cancer. Nat Rev Cancer (2005) 5(2):127–35. doi: 10.1038/nrc1549
2. Bray F, Ferlay J, Soerjomataram I, Siegel RL, Torre LA, Jemal A. Global cancer statistics 2018: GLOBOCAN estimates of incidence and mortality worldwide for 36 cancers in 185 countries. CA: Cancer J Clin (2018) 68(6):394–424. doi: 10.3322/caac.21492
3. Ang KK, Chen A, Curran WJ Jr., Garden AS, Harari PM, Murphy BA, et al. Head and neck carcinoma in the united states: First comprehensive report of the longitudinal oncology registry of head and neck carcinoma (LORHAN). Cancer (2012) 118(23):5783–92. doi: 10.1002/cncr.27609
4. Gatta G, Botta L, Sánchez MJ, Anderson LA, Pierannunzio D, Licitra L. Prognoses and improvement for head and neck cancers diagnosed in Europe in early 2000s: The EUROCARE-5 population-based study. Eur J Cancer (Oxford England: 1990) (2015) 51(15):2130–43. doi: 10.1016/j.ejca.2015.07.043
5. Edge SB, Compton CC. The American joint committee on cancer: the 7th edition of the AJCC cancer staging manual and the future of TNM. Ann Surg Oncol (2010) 17(6):1471–4. doi: 10.1245/s10434-010-0985-4
6. Muzaffar J, Bari S, Kirtane K, Chung CH. Recent advances and future directions in clinical management of head and neck squamous cell carcinoma. Cancers (2021) 13(2). doi: 10.3390/cancers13020338
7. Moll R, Divo M, Langbein L. The human keratins: biology and pathology. Histochem Cell Biol (2008) 129(6):705–33. doi: 10.1007/s00418-008-0435-6
8. Fillies T, Werkmeister R, Packeisen J, Brandt B, Morin P, Weingart D, et al. Cytokeratin 8/18 expression indicates a poor prognosis in squamous cell carcinomas of the oral cavity. BMC cancer (2006) 6:10. doi: 10.1186/1471-2407-6-10
9. Viana AOR, Gonçalves Filho J, Francisco ALN, Pinto CAL, Kowalski LP. Ki-67 and CK-19 are predictors of locoregional recurrence in papillary thyroid carcinoma. Acta otorhinolaryngologica Italica: organo ufficiale della Societa italiana di otorinolaringologia e chirurgia cervico-facciale (2020) 40(3):190–7. doi: 10.14639/0392-100x-n0311
10. Baur AS, Shaw P, Burri N, Delacrétaz F, Bosman FT, Chaubert P. Frequent methylation silencing of p15(INK4b) (MTS2) and p16(INK4a) (MTS1) in b-cell and T-cell lymphomas. Blood (1999) 94(5):1773–81. doi: 10.1182/blood.V94.5.1773
11. Knösel T, Altendorf-Hofmann A, Lindner L, Issels R, Hermeking H, Schuebbe G, et al. Loss of p16(INK4a) is associated with reduced patient survival in soft tissue tumours, and indicates a senescence barrier. J Clin pathology (2014) 67(7):592–8. doi: 10.1136/jclinpath-2013-202106
12. Yu DH, Waterland RA, Zhang P, Schady D, Chen MH, Guan Y, et al. Targeted p16(Ink4a) epimutation causes tumorigenesis and reduces survival in mice. J Clin Invest (2014) 124(9):3708–12. doi: 10.1172/jci76507
13. Alsbeih G, Al-Harbi N, Bin Judia S, Al-Qahtani W, Khoja H, El-Sebaie M, et al. Prevalence of human papillomavirus (HPV) infection and the association with survival in Saudi patients with head and neck squamous cell carcinoma. Cancers (2019) 11(6). doi: 10.3390/cancers11060820
14. Guadagno E, Del Basso De Caro M, Pignatiello S, Sciammarella C, Solari D, Cappabianca P, et al. Expression of p40 (ΔNp63) protein in meningiomas, an unexpected finding: immunohistochemical study and evaluation of its possible prognostic role. J neuro-oncology (2016) 129(3):405–13. doi: 10.1007/s11060-016-2205-y
15. Nogueira CP, Dolan RW, Gooey J, Byahatti S, Vaughan CW, Fuleihan NS, et al. Inactivation of p53 and amplification of cyclin D1 correlate with clinical outcome in head and neck cancer. Laryngoscope (1998) 108(3):345–50. doi: 10.1097/00005537-199803000-00007
16. Carlos de Vicente J, Junquera Gutiérrez LM, Zapatero AH, Fresno Forcelledo MF, Hernández-Vallejo G, López Arranz JS. Prognostic significance of p53 expression in oral squamous cell carcinoma without neck node metastases. Head neck (2004) 26(1):22–30. doi: 10.1002/hed.10339
17. Bosch FX, Ritter D, Enders C, Flechtenmacher C, Abel U, Dietz A, et al. Head and neck tumor sites differ in prevalence and spectrum of p53 alterations but these have limited prognostic value. Int J cancer (2004) 111(4):530–8. doi: 10.1002/ijc.11698
18. Mineta H, Borg A, Dictor M, Wahlberg P, Akervall J, Wennerberg J. p53 mutation, but not p53 overexpression, correlates with survival in head and neck squamous cell carcinoma. Br J cancer (1998) 78(8):1084–90. doi: 10.1038/bjc.1998.632
19. Tse GM, Tan PH, Lui PC, Gilks CB, Poon CS, Ma TK, et al. The role of immunohistochemistry for smooth-muscle actin, p63, CD10 and cytokeratin 14 in the differential diagnosis of papillary lesions of the breast. J Clin pathology (2007) 60(3):315–20. doi: 10.1136/jcp.2006.036830
20. Zhuang Z, Xie N, Hu J, Yu P, Wang C, Hu X, et al. Interplay between ΔNp63 and miR-138-5p regulates growth, metastasis and stemness of oral squamous cell carcinoma. Oncotarget (2017) 8(13):21954–73. doi: 10.18632/oncotarget.15752
21. Friedman J, Hastie T, Tibshirani R. Regularization paths for generalized linear models via coordinate descent. J Stat Software (2010) 33(1):1–22. doi: 10.18637/jss.v033.i01
22. Harrell FE Jr., Lee KL, Mark DB. Multivariable prognostic models: Issues in developing models, evaluating assumptions and adequacy, and measuring and reducing errors. Stat Med (1996) 15(4):361–87. doi: 10.1002/(sici)1097-0258(19960229)15:4<361::Aid-sim168>3.0.Co;2-4
23. Schweizer J, Bowden PE, Coulombe PA, Langbein L, Lane EB, Magin TM, et al. New consensus nomenclature for mammalian keratins. J Cell Biol (2006) 174(2):169–74. doi: 10.1083/jcb.200603161
24. de Pereda JM, Lillo MP, Sonnenberg A. Structural basis of the interaction between integrin alpha6beta4 and plectin at the hemidesmosomes. EMBO J (2009) 28(8):1180–90. doi: 10.1038/emboj.2009.48
25. Jang TH, Huang WC, Tung SL, Lin SC, Chen PM, Cho CY, et al. MicroRNA-485-5p targets keratin 17 to regulate oral cancer stemness and chemoresistance via the integrin/FAK/Src/ERK/β-catenin pathway. J Biomed science (2022) 29(1):42. doi: 10.1186/s12929-022-00824-z
26. Karantza V. Keratins in health and cancer: More than mere epithelial cell markers. Oncogene (2011) 30(2):127–38. doi: 10.1038/onc.2010.456
27. Pich A, Chiusa L, Navone R. Prognostic relevance of cell proliferation in head and neck tumors. Ann oncology: Off J Eur Soc Med Oncol (2004) 15(9):1319–29. doi: 10.1093/annonc/mdh299
28. Gioacchini FM, Alicandri-Ciufelli M, Magliulo G, Rubini C, Presutti L, Re M. The clinical relevance of ki-67 expression in laryngeal squamous cell carcinoma. Eur Arch oto-rhino-laryngology: Off J Eur Fed Oto-Rhino-Laryngological Societies (EUFOS): affiliated German Soc Oto-Rhino-Laryngology - Head Neck Surgery (2015) 272(7):1569–76. doi: 10.1007/s00405-014-3117-0
29. Zhang HS, Postigo AA, Dean DC. Active transcriptional repression by the Rb-E2F complex mediates G1 arrest triggered by p16INK4a, TGFbeta, and contact inhibition. Cell (1999) 97(1):53–61. doi: 10.1016/s0092-8674(00)80714-x
30. Rocco JW, Li D, Liggett WH Jr., Duan L, Saunders JK Jr., Sidransky D, et al. p16INK4A adenovirus-mediated gene therapy for human head and neck squamous cell cancer. Clin Cancer research: an Off J Am Assoc Cancer Res (1998) 4(7):1697–704.
31. Bishop JA, Teruya-Feldstein J, Westra WH, Pelosi G, Travis WD, Rekhtman N. p40 (ΔNp63) is superior to p63 for the diagnosis of pulmonary squamous cell carcinoma. Modern pathology: An Off J United States Can Acad Pathology Inc (2012) 25(3):405–15. doi: 10.1038/modpathol.2011.173
32. Bishop JA, Montgomery EA, Westra WH. Use of p40 and p63 immunohistochemistry and human papillomavirus testing as ancillary tools for the recognition of head and neck sarcomatoid carcinoma and its distinction from benign and malignant mesenchymal processes. Am J Surg pathology (2014) 38(2):257–64. doi: 10.1097/pas.0000000000000119
33. Chakrabarti R, Wei Y, Hwang J, Hang X, Andres Blanco M, Choudhury A, et al. ΔNp63 promotes stem cell activity in mammary gland development and basal-like breast cancer by enhancing Fzd7 expression and wnt signalling. Nat Cell Biol (2014) 16(10):1004–15, 1-13. doi: 10.1038/ncb3040
34. Su X, Chakravarti D, Flores ER. p63 steps into the limelight: Crucial roles in the suppression of tumorigenesis and metastasis. Nat Rev Cancer (2013) 13(2):136–43. doi: 10.1038/nrc3446
Keywords: head and neck squamous cell carcinoma, immunohistochemistry, nomogram, prognosis, TNM system
Citation: Xu Q-Q, Li Q-J, Xu Z, Lan L-L, Hou Z, Liu J, Lu L, Chen Y-Y, Chen R-Z and Wen X (2023) Prognostic value of the immunohistochemical score based on four markers in head and neck squamous cell carcinoma. Front. Immunol. 14:1076890. doi: 10.3389/fimmu.2023.1076890
Received: 22 October 2022; Accepted: 13 February 2023;
Published: 22 February 2023.
Edited by:
Wenxue Ma, University of California, San Diego, United StatesReviewed by:
William J. Magner, University at Buffalo, United StatesYao Xiao, Wuhan University, China
Copyright © 2023 Xu, Li, Xu, Lan, Hou, Liu, Lu, Chen, Chen and Wen. This is an open-access article distributed under the terms of the Creative Commons Attribution License (CC BY). The use, distribution or reproduction in other forums is permitted, provided the original author(s) and the copyright owner(s) are credited and that the original publication in this journal is cited, in accordance with accepted academic practice. No use, distribution or reproduction is permitted which does not comply with these terms.
*Correspondence: Xin Wen, wenx1990@126.com; Yuan-Yuan Chen, chenyy2@sysucc.org.cn; Run-Zhe Chen, chenrz@sysucc.org.cn
†These authors have contributed equally to this work
‡ORCID: Xin Wen, orcid.org/0000-0001-8530-9848