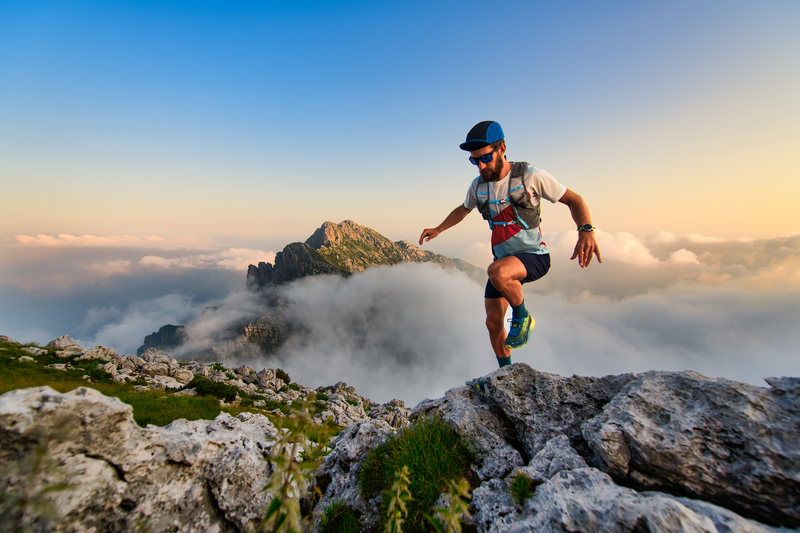
94% of researchers rate our articles as excellent or good
Learn more about the work of our research integrity team to safeguard the quality of each article we publish.
Find out more
OPINION article
Front. Immunol. , 31 January 2023
Sec. Viral Immunology
Volume 14 - 2023 | https://doi.org/10.3389/fimmu.2023.1075419
This article is part of the Research Topic New Approach Methods in Immunology View all 20 articles
A correction has been applied to this article in:
Corrigendum: Integration: gospel for immune bioinformatician on epitope-based therapy
Peptide-based vaccines are attracting considerable attention due to the potential to precisely tune the immune response using antigens fragments or peptides, as well as the relative ease of production (1). So far, peptide vaccines against viral infectious diseases have been widely developed and entered phase I/II clinical trials (2), which include COVID-19, HIV, influenza, hepatitis B, and hepatitis C, meaning the mature technology in research and development. Also, NeuVax, a peptide vaccine against breast cancer, completed phase III clinical trial and declared safety in vivo (3). This is the furthest step in the quest for epitope vaccines for use in humans to date (4, 5), suggesting the effectiveness of strategies and the bright application prospect to design peptide-based vaccines (6, 7).
Effective epitopes play a major therapeutic role in synthetic peptide vaccines. Identifying and screening epitopes, however, is usually an endless and complex process (8). Fortunately, researchers have developed in silico prediction methods that dramatically reduce the burden associated with epitope mapping by minimizing the list of potential epitope candidates for experimental testing (9). These tools allow investigators to analyze antigenic properties at multiple levels and multiple dimensions, such as affinity, immunogenicity, toxicity, and sensitization, which greatly promotes the further comprehension of pathogens and has a great role in promoting the research and development of drugs to benefit disease prevention and control (9–11).
However, the accuracy of single prediction analysis is often only 50%-70% (12–16). This is probably because of the inherent defects in computer algorithms based on epitope databases for fitting functions (8, 11). In view of above deficiencies, we propose a novel train of thought, “integration”, to augment the breadth and depth of bioinformatics in peptide-based therapeutics. At the same time, we call on researchers to excavate connotations with the characteristics of antigens behind the credit tool and to make breakthrough in the prevention and control of diseases such as infection and cancer.
Accuracy has always been the goal pursued by various bioinformatics algorithms. Therefore, researchers have relied on a variety of algorithms, such as artificial neural networks (ANNs) (17–19), the stabilized matrix method (SMM) (20), and the consensus method (21), and tested them in tens of thousands of epitopes in multiple databases (17, 18). However, fully accurate algorithmic toolkits have not been developed to date. Meanwhile, in silico studies usually reveal their results with limited or even single predictive tools in respective orientations, which greatly hinders the application in general. Therefore, the concept of “integration” is advocated, which is the use of multiple analysis algorithms for an identical antigen, selecting the dominant epitopes predicted by each tool or adopting higher criteria. Considering this strategy in a purpose-oriented way, alternative methods output epitopes by different algorithms, collateral with false positives due to inherent defects of each toolkit. Theoretically, dominant epitopes should be defined through the intersection of multiple tools, which greatly reduces the probability of nondominant epitope output, thereby improving the accuracy of prediction.
Affinity analyses have been widely used in the study of infectious diseases (22–24), neoplasms (25) and autoimmune diseases (26). Table 1 lists the current international mainstream epitope analysis algorithms. There are multiple algorithms and platforms to analyze affinity, and the IEDB analysis resource (MHC-I binding and MHC-II binding) and DTU HEALTH TECH (NetMHCpan and NetMHCIIpan) platforms have taken over the absolute principal position. The functionality of affinity analysis provided by the IEDB Analytical Resources integrates multiple analytical methods (27), including Artificial neural network (ANN) (19), Stabilized matrix method (SMM) (20), SMM with a Peptide: major histocompatibility complex(MHC) Binding Energy Covariance matrix (SMMPMBEC) (28), and so on. Why not make full use of these algorithms to sharpen the accuracy of affinity analysis? Recently, immunologists followed this thought in glycoprotein epitope studies of Hantaan virus and Ebola virus, in which five or four affinity algorithms predicted MHC-I and MHC-II restricted epitopes, respectively, selecting epitopes with Rank ≤ 2% 3 or 2 times among toolkits. Strong cellular responses in enzyme-linked immunospot assays with corresponding epitopes validated the effectiveness of this strategy in infectious diseases such as viral hemorrhagic fever virus (29–31).
Most studies on bioinformatics exploration of peptide-based therapeutics lacked validation of relevant results (32–34). This may be related to the time- and resource-consuming experimental conditions. For example, considering the risk of Ebola virus and the harsh nature of the required test conditions, Alizadeh et al. resigned the evaluation after designing a multiepitope vaccine against Ebola virus (35). However, we found a connection between different types of epitope analysis, so the results of multiple analyses can be compared to validate the results at the bioinformatics level.
Take an example of the depth implication in affinity analysis and molecular docking (8). Peptide-MHC interactions are crucial in immune functions. Therefore, determining the structure of Peptide-MHC complexes is important for understanding the molecular mechanism of related biological processes and developing peptide-based immunotherapy. Typically, the RCSB PDB (https://www.rcsb.org/) is used to obtain models of MHC molecules and then HEPEDOCK 2.0 is used to predict the possibility of MHC-peptide complexes and provide multiple docking models (36). EpiDOCK toolkit can be also used in MHC-II-peptide docking and predicting binding energy (37). Binding affinity and molecular docking share a similar connotation, indicating the binding ability of MHC molecules and epitopes (38, 39). Therefore, the results of both analyses can mutually validate each other in silico. However, if there is a gap in the above comparison, it may be derived from the defects in algorithms’ intrinsic variety. More importantly, by simulating the docking between epitopes and MHC, the location and tightness of the docking can be directly figured out, so that the affinity data can be more intuitively reflected.
Most algorithms give only a large number of “cold” numbers in processing antigens. How to convert these numbers into meaningful models or illustrations related to the body’s immunologic nature is an aporia faced by every bioinformatics researcher. Here are three approaches to delineate the above issues.
A large amount of data will be generated in the affinity analysis of multiepitopes together with multi-MHC genotypes. It would not be state-of-art to present the whole things directly in the main text. By looking at these data using a holistic view, that is, using a heatmap form, which can show three dimensions: the epitopes, MHC genotypes and affinity ranks (40, 41). It is intuitive to exhibit the epitopes that are dominant in MHC genotypes. At the same time, bihierarchical clustering would reveal the cross-reactivity between different genotypes based on affinity analysis data (42, 43). This intuitively reflects genotype proximity according to pathogen specificity, further understanding of susceptibility and resistance against pathogens in different races, geographies, populations, and even species. Using these forms to present data not only makes the expression more intuitive and concise but also fully interprets the deep-seated meaning of the data.
Alignment of variants has been implicated in many studies but is limited to the step of multiple sequence alignment, finding mutation sites, and exploring evolutionary clades (42, 44, 45). On this basis, another direction — further research on the impact of mutation sites — should also be taken into consideration. That is, the mutation site is brought into the original sequence to explore the impact of the mutation site on the affinity and immunogenicity of the specific epitopes (29, 31). This analysis of the mutated sequences in silico predicts the approximate direction of evolutionary impacts on immunogenetic variation and deepens the understanding of pathogen conservation.
Epitope studies always aim to design drugs or vaccines for clinical use. Therefore, building of molecular model will be a complex and important work. At present, there are two channels to obtain molecular structure models: one is based on the existing RCSB PDB database to retrieve the discovered molecular structures, and the other is ab initio prediction method based on molecular dynamic (MD). That is, 3D atomic models were building from different stringing arrangements and iterative structural assembly simulations according to amino acid sequences, and then the models are optimized by MD. MD is a computer simulation method which is employed in various engineering and science disciplines to calculate motion and equilibrium of each individual atom or molecule. It can be used to explore conformational space, and is often the method of choice for large molecules such as proteins. Recently, due to the continuous development of MD, molecular models and docking are closer to the real response (46). Here, we recommend a method, the integration of I-TASSER and GalaxyRefine server. I-TASSER integrates inter-residue contact maps from deep neural-network learning with the cutting-edge fragment assembly simulations (47). GalaxyRefine performs repeated structure perturbation and subsequent overall structural relaxation by molecular dynamics simulation (48). The integration of I-TASSER and GalaxyRefine server allows the molecular structure to be rebuilt and optimized, providing an overall intuitive molecular structure (8, 49).
In recent years, bioinformatics has been widely used on epitope studies of pathogenic microorganisms. However, in silico analysis is not static and needs to be adjusted according to the characteristics of antigens and the purposes of the research. The contradictions investigated in tumor immunity and autoimmune diseases are distinguishing. Also, the “elegance” of integration should be noted.
Tumor antigens are usually cancer-specific peptides (neoepitopes) (50)generated by somatic mutations or genomic aberrations, posttranslational modifications (PTMs) (51), and translation from noncoding regions (52). The aim of tumor immunity is to circumvent immune suppressive evasion mechanisms used by cancer cells through modulation of T cell responses (2). Therefore, in silico analysis of tumor antigens mainly focuses on interrogating cytotoxic T (CTL) cell epitopes and promoting cytotoxicity (53). On the other hand, autoimmune diseases arise from immune responses to self-antigens and reflect a breakdown in immunological tolerance (54). Most classical autoimmune diseases have associations with genes in the MHC-II region (55), leading to the production of autoantibodies (56) or the activation of CTL cells (57) to autoantigens. Therefore, pathognomonic autoimmune diseases need to be specifically designed according to the disease mechanism and site of immunoreaction.
T-cell (CD8 + and CD4 + T-cell) epitopes and B-cell epitopes are the material basis of adaptive immune responses as allure to most of studies. However, few has addressed the association among them so far. It is well known that CD4+ T cells, as helper cells, promote both killer T cells and B cells and then strengthen humoral and cellular immunity (58). Obeying this immune principle, following routine can be considered: epitopes should be validated, and the links between genuine MHC-I and MHC-II dominance, perhaps MHC-II and B-cell antigenic determinants can be found to mine the substantial bases of cellular and humoral immunity.
The above renders multiple “integration” ideas. Figure 1 shows the structure diagram of recommendatory integration strategy. However, attentions should be paid in the practice: an epitope could be jointly selected with affinity, immunogenicity, conservation, toxicity, allergen analysis, etc. It would be interesting but challenging to figure out what impacts the different orders will have on the results and what particular connotations the epitopes will be endued with. Additionally, for each operation, the results will be tested once. It is intractable to face false positives, false negatives or overfitting of the integrated results due to multiple testing. Finally, toolkits of the algorithms and datasets do not update in a timely manner, which directly affects the tool selection, priority of use, and settings of various parameters.
Figure 1 The structure diagram of recommendatory integration strategy. The orange is the purpose, blue is the method and yellow is the tool involved.
The evolution of epitope-based vaccines is one of the most promising developments arising from bioinformatics-based research (8), and the development of bioinformatics has also made pathogen epitope exploration convenient and cost-effective (11). We put forward a point of view, “Integration”, aiming to improve the accuracy and fit-in the body’s immune response. It says the opinion that integration manners are advances in bioinformatics rather than just a single algorithmic innovation. The acquisition of analytical results is by no means the terminus of epitopes studies. How to apply the toolkits in silico under the precondition for immunology principles will be critical issues to be addressed. In this opinion, the “integration” tenet was first put forward, dedicated to compensating for inherent deficiencies in current algorithms and simulating the realistic immune response model. It provided a novel train of thought for peptide-based immunotherapy in silico against infection, neoplasms and autoimmune diseases, and would by all odds promote the development and progress of the bioinformatics discipline.
Conceptualization: DJ, BS, and KY. Methodology: DJ, BS, and JZ. Formal analysis: SB and DJ. Investigation: BS, DJ, and YoW. Resources, DJ, and KY. Writing—original draft preparation: BS and JZ. Writing—review and editing: DJ, JZ, ZL, MX, and YoW. Peer discussion: YoW, CL, LC, and YuW. Visualization, BS, JZ, ZL, and MX. Supervision, DJ and KY. Project administration, DJ and KY. Funding acquisition: DJ and KY. All authors contributed to the article and approved the submitted version.
This study was supported by the General Program of National Nature Science Foundation of China (No. 82073154 to KY), the Youth Program of National Nature Science Foundation of China (No. 82203510 to DJ), the Key Research and Development Program of Shaanxi Province (2023-YBSF-198 to DJ), and the Promotion Program of Air Force Medical University (2020SWAQ03 to KY).
The authors declare that the research was conducted in the absence of any commercial or financial relationships that could be construed as a potential conflict of interest.
All claims expressed in this article are solely those of the authors and do not necessarily represent those of their affiliated organizations, or those of the publisher, the editors and the reviewers. Any product that may be evaluated in this article, or claim that may be made by its manufacturer, is not guaranteed or endorsed by the publisher.
1. Malonis RJ, Lai JR, Vergnolle O. Peptide-based vaccines: Current progress and future challenges. Chem Rev (2020) 120(6):3210–29. doi: 10.1021/acs.chemrev.9b00472
2. Hamley IW. Peptides for vaccine development. ACS Appl Bio Mater (2022) 5(3):905–44. doi: 10.1021/acsabm.1c01238
3. Mittendorf EA, Lu B, Melisko M, Price Hiller J, Bondarenko I, Brunt AM, et al. Efficacy and safety analysis of nelipepimut-s vaccine to prevent breast cancer recurrence: A randomized, multicenter, phase III clinical trial. Clin Cancer Res (2019) 25(14):4248–54. doi: 10.1158/1078-0432.CCR-18-2867
4. Nicolás-Morales ML, Luisa-Sanjuan A, Gutiérrez-Torres M, Vences-Velázquez A, Ortuño-Pineda C, Espinoza-Rojo M, et al. Peptide-based vaccines in clinical phases and new potential therapeutic targets as a new approach for breast cancer: A review. Vaccines (Basel) (2022) 10(8). doi: 10.3390/vaccines10081249
5. Davodabadi F, Sarhadi M, Arabpour J, Sargazi S, Rahdar A, Díez-Pascual AM. Breast cancer vaccines: New insights into immunomodulatory and nano-therapeutic approaches. J Control Release (2022) 349:844–75. doi: 10.1016/j.jconrel.2022.07.036
6. Mittendorf EA, Clifton GT, Holmes JP, Schneble E, van Echo D, Ponniah S, et al. Final report of the phase I/II clinical trial of the E75 (nelipepimut-s) vaccine with booster inoculations to prevent disease recurrence in high-risk breast cancer patients. Ann Oncol (2014) 25(9):1735–42. doi: 10.1093/annonc/mdu211
7. Errico A. Breast cancer: E75-a safe and effective vaccine for the prevention of disease recurrence. Nat Rev Clin Oncol (2014) 11(8):440. doi: 10.1038/nrclinonc.2014.11
8. Yurina V, Adianingsih OR. Predicting epitopes for vaccine development using bioinformatics tools. Ther Adv Vaccines Immunother (2022) 10:25151355221100218. doi: 10.1177/25151355221100218
9. Sanchez-Trincado JL, Gomez-Perosanz M, Reche PA. Fundamentals and methods for T- and b-cell epitope prediction. J Immunol Res (2017) 2017:2680160. doi: 10.1155/2017/2680160
10. Bahrami AA, Payandeh Z, Khalili S, Zakeri A, Bandehpour M. Immunoinformatics: In silico approaches and computational design of a multi-epitope, immunogenic protein. Int Rev Immunol (2019) 38(6):307–22. doi: 10.1080/08830185.2019.1657426
11. Kazi A, Chuah C, Majeed ABA, Leow CH, Lim BH, Leow CY. Current progress of immunoinformatics approach harnessed for cellular- and antibody-dependent vaccine design. Pathog Glob Health (2018) 112(3):123–31. doi: 10.1080/20477724.2018.1446773
12. Jurtz V, Paul S, Andreatta M, Marcatili P, Peters B, Nielsen M. NetMHCpan-4.0: Improved peptide-MHC class I interaction predictions integrating eluted ligand and peptide binding affinity data. J Immunol (2017) 199(9):3360–8. doi: 10.4049/jimmunol.1700893
13. Nielsen M, Lundegaard C, Lund O. Prediction of MHC class II binding affinity using SMM-align, a novel stabilization matrix alignment method. BMC Bioinf (2007) 8:238. doi: 10.1186/1471-2105-8-238
14. Jensen KK, Andreatta M, Marcatili P, Buus S, Greenbaum JA, Yan Z, et al. Improved methods for predicting peptide binding affinity to MHC class II molecules. Immunol (2018) 154(3):394–406. doi: 10.1111/imm.12889
15. Wang P, Sidney J, Dow C, Mothé B, Sette A, Peters B. A systematic assessment of MHC class II peptide binding predictions and evaluation of a consensus approach. PloS Comput Biol (2008) 4(4):e1000048. doi: 10.1371/journal.pcbi.1000048
17. Reynisson B, Barra C, Kaabinejadian S, Hildebrand WH, Peters B, Nielsen M. Improved prediction of MHC II antigen presentation through integration and motif deconvolution of mass spectrometry MHC eluted ligand data. J Proteome Res (2020) 19(6):2304–15. doi: 10.1021/acs.jproteome.9b00874
18. Alvarez B, Reynisson B, Barra C, Buus S, Ternette N, Connelley T, et al. NNAlign_MA; MHC peptidome deconvolution for accurate MHC binding motif characterization and improved T-cell epitope predictions. Mol Cell Proteomics (2019) 18(12):2459–77. doi: 10.1074/mcp.TIR119.001658
19. Andreatta M, Nielsen M. Gapped sequence alignment using artificial neural networks: application to the MHC class I system. Bioinformatics (2016) 32(4):511–7. doi: 10.1093/bioinformatics/btv639
20. Peters B, Sette A. Generating quantitative models describing the sequence specificity of biological processes with the stabilized matrix method. BMC Bioinf (2005) 6:132. doi: 10.1186/1471-2105-6-132
21. Moutaftsi M, Peters B, Pasquetto V, Tscharke DC, Sidney J, Bui HH, et al. A consensus epitope prediction approach identifies the breadth of murine T(CD8+)-cell responses to vaccinia virus. Nat Biotechnol (2006) 24(7):817–9. doi: 10.1038/nbt1215
22. Cheng P, Wang L, Gong W. In silico analysis of peptide-based biomarkers for the diagnosis and prevention of latent tuberculosis infection. Front Microbiol (2022) 13:947852. doi: 10.3389/fmicb.2022.947852
23. Jiang S, Wu S, Zhao G, He Y, Guo X, Zhang Z, et al. Identification of a promiscuous conserved CTL epitope within the SARS-CoV-2 spike protein. Emerg Microbes Infect (2022) 11(1):730–40. doi: 10.1080/22221751.2022.2043727
24. Ullah A, Shahid FA, Haq MU, Tahir Ul Qamar M, Irfan M, Shaker B, et al. An integrative reverse vaccinology, immunoinformatic, docking and simulation approaches towards designing of multi-epitopes based vaccine against monkeypox virus. J Biomol Struct Dyn (2022), 1–14. doi: 10.1080/07391102.2022.2125441
25. Ragone C, Manolio C, Mauriello A, Cavalluzzo B, Buonaguro FM, Tornesello ML, et al. Molecular mimicry between tumor associated antigens and microbiota-derived epitopes. J Transl Med (2022) 20(1):316. doi: 10.1186/s12967-022-03512-6
26. Repac J, Mandić M, Lunić T, Božić B, Božić Nedeljković B. Mining the capacity of human-associated microorganisms to trigger rheumatoid arthritis-a systematic immunoinformatics analysis of T cell epitopes. PloS One (2021) 16(6):e0253918. doi: 10.1371/journal.pone.0253918
27. Vita R, Mahajan S, Overton JA, Dhanda SK, Martini S, Cantrell JR, et al. The immune epitope database (IEDB): 2018 update. Nucleic Acids Res (2019) 47(D1):D339–d43. doi: 10.1093/nar/gky1006
28. Kim Y, Sidney J, Pinilla C, Sette A, Peters B. Derivation of an amino acid similarity matrix for peptide: MHC binding and its application as a Bayesian prior. BMC Bioinf (2009) 10:394. doi: 10.1186/1471-2105-10-394
29. Sun B, Zhang J, Wang J, Liu Y, Sun H, Lu Z, et al. Comparative immunoreactivity analyses of hantaan virus glycoprotein-derived MHC-I epitopes in vaccination. Vaccines (Basel) (2022) 10(4):564. doi: 10.3390/vaccines10040564
30. Sun H, Lu Z, Xuan G, Liu N, Wang T, Liu Y, et al. Integrative analysis of HTNV glycoprotein derived MHC II epitopes by in silico prediction and experimental validation. Front Cell Infect Microbiol (2021) 11:671694. doi: 10.3389/fcimb.2021.671694
31. Liu Y, Sun B, Wang J, Sun H, Lu Z, Chen L, et al. In silico analyses and experimental validation of the MHC class-I restricted epitopes of ebolavirus GP. Int Immunol (2022) 34(6):313–25. doi: 10.1093/intimm/dxac006
32. Fereshteh S, Noori Goodarzi N, Sepehr A, Shafiei M, Ajdary S, Badmasti F. In silico analyses of extracellular proteins of acinetobacter baumannii as immunogenic candidates. Iran J Pharm Res (2022) 21(1):e126559. doi: 10.5812/ijpr-126559
33. Mia MM, Hasan M, Ahmed S, Rahman MN. Insight into the first multi-epitope-based peptide subunit vaccine against avian influenza a virus (H5N6): An immunoinformatics approach. Infect Genet Evol (2022) 104:105355. doi: 10.1016/j.meegid.2022.105355
34. Shantier SW, Mustafa MI, Abdelmoneim AH, Fadl HA, Elbager SG, Makhawi AM. Novel multi epitope-based vaccine against monkeypox virus: vaccinomic approach. Sci Rep (2022) 12(1):15983. doi: 10.1038/s41598-022-20397-z
35. Alizadeh M, Amini-Khoei H, Tahmasebian S, Ghatrehsamani M, Ghatreh Samani K, Edalatpanah Y, et al. Designing a novel multi−epitope vaccine against Ebola virus using reverse vaccinology approach. Sci Rep (2022) 12(1):7757. doi: 10.1038/s41598-022-11851-z
36. Tao H, Zhao X, Zhang K, Lin P, Huang SY. Docking cyclic peptides formed by a disulfide bond through a hierarchical strategy. Bioinformatics (2022) 38(17):4109–16. doi: 10.1093/bioinformatics/btac486
37. Atanasova M, Patronov A, Dimitrov I, Flower DR, Doytchinova I. EpiDOCK: a molecular docking-based tool for MHC class II binding prediction. Protein Eng Des Sel (2013) 26(10):631–4. doi: 10.1093/protein/gzt018
38. Reynisson B, Alvarez B, Paul S, Peters B, Nielsen M. NetMHCpan-4.1 and NetMHCIIpan-4.0: Improved predictions of MHC antigen presentation by concurrent motif deconvolution and integration of MS MHC eluted ligand data. Nucleic Acids Res (2020) 48(W1):W449–w54. doi: 10.1093/nar/gkaa379
39. Nielsen M, Andreatta M. NetMHCpan-3.0; improved prediction of binding to MHC class I molecules integrating information from multiple receptor and peptide length datasets. Genome Med (2016) 8(1):33. doi: 10.1186/s13073-016-0288-x
40. Sankaranarayanan S, Mohkhedkar M, Janakiraman V. Mutations in spike protein T cell epitopes of SARS-COV-2 variants: Plausible influence on vaccine efficacy. Biochim Biophys Acta Mol Basis Dis (2022) 1868(9):166432. doi: 10.1016/j.bbadis.2022.166432
41. Salaikumaran MR, Kasamuthu PS, Aathmanathan VS, Burra V. An in silico approach to study the role of epitope order in the multi-epitope-based peptide (MEBP) vaccine design. Sci Rep (2022) 12(1):12584. doi: 10.1038/s41598-022-16445-3
42. Wu M, Chen Y, Lang W, Qin X, Ruan L, Su M, et al. Genetic variation analysis of porcine circovirus type 4 in south China in 2019 to 2021. Viruses (2022) 14(8):1736. doi: 10.3390/v14081736
43. Sami SA, Marma KKS, Mahmud S, Khan MAN, Albogami S, El-Shehawi AM, et al. Designing of a multi-epitope vaccine against the structural proteins of marburg virus exploiting the immunoinformatics approach. ACS Omega (2021) 6(47):32043–71. doi: 10.1021/acsomega.1c04817
44. Agarwal A, Beck KL, Capponi S, Kunitomi M, Nayar G, Seabolt E, et al. Predicting epitope candidates for SARS-CoV-2. Viruses (2022) 14(8):1837. doi: 10.3390/v14081837
45. Vale FF, Vítor JMB, Marques AT, Azevedo-Pereira JM, Anes E, Goncalves J. Origin, phylogeny, variability and epitope conservation of SARS-CoV-2 worldwide. Virus Res (2021) 304:198526. doi: 10.1016/j.virusres.2021.198526
46. Hu X, Zeng Z, Zhang J, Wu D, Li H, Geng F. Molecular dynamics simulation of the interaction of food proteins with small molecules. Food Chem (2023) 405(Pt A):134824. doi: 10.1016/j.foodchem.2022.134824
47. Zheng W, Zhang C, Li Y, Pearce R, Bell EW, Zhang Y. Folding non-homologous proteins by coupling deep-learning contact maps with I-TASSER assembly simulations. Cell Rep Methods (2021) 1(3):100014. doi: 10.1016/j.crmeth.2021.100014
48. Heo L, Park H, Seok C. GalaxyRefine: Protein structure refinement driven by side-chain repacking. Nucleic Acids Res (2013) 41(Web Server issue):W384–8. doi: 10.1093/nar/gkt458
49. Prawiningrum AF, Paramita RI, Panigoro SS. Immunoinformatics approach for epitope-based vaccine design: Key steps for breast cancer vaccine. Diagnostics (Basel) (2022) 12(12):2981. doi: 10.3390/diagnostics12122981
50. Schaap-Johansen AL, Vujović M, Borch A, Hadrup SR, Marcatili P. T Cell epitope prediction and its application to immunotherapy. Front Immunol (2021) 12:712488. doi: 10.3389/fimmu.2021.712488
51. Malaker SA, Penny SA, Steadman LG, Myers PT, Loke JC, Raghavan M, et al. Identification of glycopeptides as posttranslationally modified neoantigens in leukemia. Cancer Immunol Res (2017) 5(5):376–84. doi: 10.1158/2326-6066.CIR-16-0280
52. Laumont CM, Vincent K, Hesnard L, Audemard É, Bonneil É, Laverdure JP, et al. Noncoding regions are the main source of targetable tumor-specific antigens. Sci Transl Med (2018) 10(470):eaau5516. doi: 10.1126/scitranslmed.aau5516
53. Duan F, Duitama J, Al Seesi S, Ayres CM, Corcelli SA, Pawashe AP, et al. Genomic and bioinformatic profiling of mutational neoepitopes reveals new rules to predict anticancer immunogenicity. J Exp Med (2014) 211(11):2231–48. doi: 10.1084/jem.20141308
54. Streeter HB, Wraith DC. Manipulating antigen presentation for antigen-specific immunotherapy of autoimmune diseases. Curr Opin Immunol (2021) 70:75–81. doi: 10.1016/j.coi.2021.03.019
55. Libert C, Dejager L, Pinheiro I. The X chromosome in immune functions: when a chromosome makes the difference. Nat Rev Immunol (2010) 10(8):594–604. doi: 10.1038/nri2815
56. Smith EL, Peakman M. Peptide immunotherapy for type 1 diabetes-clinical advances. Front Immunol (2018) 9:392. doi: 10.3389/fimmu.2018.00392
57. Matsoukas JM, Ligielli I, Chasapis CT, Kelaidonis K, Apostolopoulos V, Mavromoustakos T. Novel approaches in the immunotherapy of multiple sclerosis: Cyclization of myelin epitope peptides and conjugation with mannan. Brain Sci (2021) 11(12):1583. doi: 10.3390/brainsci11121583
Keywords: integration, epitope, immunotherapy, in silico, bioinformatics, immune response
Citation: Sun B, Zhang J, Li Z, Xie M, Luo C, Wang Y, Chen L, Wang Y, Jiang D and Yang K (2023) Integration: Gospel for immune bioinformatician on epitope-based therapy. Front. Immunol. 14:1075419. doi: 10.3389/fimmu.2023.1075419
Received: 20 October 2022; Accepted: 17 January 2023;
Published: 31 January 2023.
Edited by:
Shisong Jiang, University of Oxford, United KingdomReviewed by:
Ali Namvar, Iranian Comprehensive Hemophilia Care Center, IranCopyright © 2023 Sun, Zhang, Li, Xie, Luo, Wang, Chen, Wang, Jiang and Yang. This is an open-access article distributed under the terms of the Creative Commons Attribution License (CC BY). The use, distribution or reproduction in other forums is permitted, provided the original author(s) and the copyright owner(s) are credited and that the original publication in this journal is cited, in accordance with accepted academic practice. No use, distribution or reproduction is permitted which does not comply with these terms.
*Correspondence: Dongbo Jiang, c3VwZXJqYW1lczE5OTFAZm94bWFpbC5jb20=; Kun Yang, eWFuZ2t1bmt1bkBmbW11LmVkdS5jbg==
†These authors share first authorship
Disclaimer: All claims expressed in this article are solely those of the authors and do not necessarily represent those of their affiliated organizations, or those of the publisher, the editors and the reviewers. Any product that may be evaluated in this article or claim that may be made by its manufacturer is not guaranteed or endorsed by the publisher.
Research integrity at Frontiers
Learn more about the work of our research integrity team to safeguard the quality of each article we publish.