- 1Department of Integrative Medicine, Huashan Hospital, Fudan University, Shanghai, China
- 2The Institutes of Integrative Medicine, Fudan University, Shanghai, China
Asthma is a complex and heterogeneous disease with multicellular involvement, and knowledge gaps remain in our understanding of the pathogenesis of asthma. Efforts are still being made to investigate the immune pathogenesis of asthma in order to identify possible targets for prevention. Single cell RNA sequencing (scRNA-seq) technology is a useful tool for exploring heterogeneous diseases, identifying rare cell types and distinct cell subsets, enabling elucidation of key processes of cell differentiation, and understanding regulatory gene networks that predict immune function. In this article, we provide an overview of the importance of scRNA-seq for asthma research, followed by an in-depth discussion of the results in recent years, in order to provide new ideas for the pathogenesis, drug development and treatment of asthma.
Introduction: Current status of asthma treatment
Asthma is a heterogeneous disease characterized by chronic airway inflammation. Multiple types of cells and their products play an important role in its pathogenesis (1). Asthma is a major public health challenge, affecting approximately 300 million people worldwide, and this number is constantly rising (2, 3). In addition to high mortality, asthma also reduces the quality of life and physical functioning of asthmatics and imposes an economic burden (4). The combination of beta2-adrenergic receptor agonists and steroids is effective in the treatment of asthma, but some patients have poor response or tolerance to beta2-agonists and glucocorticoids due to disease heterogeneity or individual differences (5). Corticosteroids also do not cure or significantly alter the course of asthma, as asthma symptoms return quickly after the drug is discontinued (6). Systemic corticosteroid use may also cause hypothalamic-pituitary-adrenal axis dysfunction. By contrast, treating asthma with biological agents may increase costs and side effects (7–9). Therefore, the pathogenesis of asthma needs to be further studied. The emergence of scRNA-seq technology provides a new research idea and solution for the comprehensive and systematic study of effector cells and key molecular mechanisms of asthma.
The complex pathogenesis of asthma
The pathogenesis of asthma is partially unknown, and genome-wide association studies have shown that immune responses and epithelial barrier dysfunction contribute to the pathogenesis of asthma (10, 11).
The pathological process of asthma is involved in a variety of cells and cellular components, including dendritic cells (DCs), mast cells, eosinophils, T lymphocytes, B lymphocytes and neutrophils, as well as epithelial cells, which interact with inflammatory cells and the external environment, leading to chronic airway inflammation, reversible airflow limitation, airway hyperresponsiveness, and airway remodeling. When exposed to allergens, epithelial cells monitor specific antigens and release pro-inflammatory factors that promote the recruitment and activation of DCs, thereby inducing allergen-specific type 2 T helper (Th2) cell activation (12). Upon re-exposure to allergens, Th2 effector cells are stimulated and activated to secrete type 2 inflammatory factors such as IL-4, IL-5 and IL-13, recruit and activate eosinophils and mast cells, and stimulate B lymphocytes to produce IgE, which mediates eosinophil inflammation and regulates cellular and humoral immunity (13, 14). Type 2 innate lymphoid cells (ILC2s) are also important mediators of asthma by producing IL-5, IL-13 and crossregulating conventional T cells (15, 16). Increased neutrophils and enhanced Th17 immune responses are also considered to be involved in the pathological progression of severe asthma (17–19).
Airway epithelial cells are located at the junction between the inhalation environment and the lung. They are the first barrier to protect the human body from pathogens, and are crucial for the occurrence and development of asthmatic airway inflammation (20). When the epithelial barrier is disrupted, it promotes the secretion of growth factors from epithelial cells, which activates fibroblasts and myofibroblasts, which in turn promotes excessive collagen deposition, leading to increased smooth muscle mass (21). In addition, epithelial cells can produce cytokines crucial for innate immunity and promote immune cell activity. For example, IL-25 and IL-33 secreted by airway epithelium can activate immune cells and recruit inflammatory cells to initiate Th2-type cell responses or ILC2 activation. On the other hand, IL-13, a type 2 inflammatory factor that plays an important role in structural cells (22, 23), such as epithelial goblet cell metaplasia (24) and mucin glycoprotein MUC5AC secretion (25, 26), is a critical factor in lethal airway obstruction (25, 27).
In conclusion, the pathological process of asthma is participated by a variety of cells and their components, such as eosinophils, T lymphocytes, B lymphocytes, macrophages, neutrophils and epithelial cells, which interact with the external environment, leading to chronic airway inflammation, reversible airflow limitation, and airway remodeling in asthmatic patients, showing the diversity of asthma phenotypes and the complexity of pathogenesis.
Application and research progress of scRNA-seq technology in asthma pathogenesis
Transcriptome sequencing is widely used to study the mechanisms of asthma. Traditional transcriptome sequencing methods based on blood or tissue samples require a large number of cell populations, presenting the average expression levels in all samples, and cannot present the characteristics of individual cells. Therefore, the pathogenesis of heterogeneous disease has not been fully elucidated. ScRNA-seq is a technology that parses RNA sequences at the single-cell level. It takes a single cell as a unit, separates the cell population in a tissue or body fluid sample into a single cell, performs whole transcriptome amplification and high-throughput sequencing, and obtains the corresponding data and information analysis. This disruptive technology enables higher-resolution analysis of cellular differences and a better understanding of how individual cells function in their specific microenvironment, specific therapeutic and disease context. In addition, scRNA-seq predicts cell-to-cell interactions and state changes in extraordinary detail and characterizes the molecular cellular phenotypes (or cell “states”) (20). Conceivably, scRNA-seq technique can achieve more unique targets than traditional approaches, such as identifying rare cells, defining disease subtypes, discovering new cellular markers, characterizing cellular heterogeneity and subsets, elucidating disease mechanisms, and enabling precise and personalized drugs.
As shown in Figure 1, the main steps of scRNA-seq include single cell preparation, single-cell library construction and sequencing, and bioinformatics data analysis (28). There are a variety of single-cell isolation techniques. Nucleic acid is obtained after isolation and lysis of single-cell and then amplified for subsequent sequencing. Various single cell isolation methods have been developed depending on the state of the sample, the number of cells required, and the purpose of the analysis (Table 1). Based on microdroplet or microfluidic chip technology, many manufacturers have launched their own high-throughput scRNA Seq platforms. At present, there are four mainstream commercial platforms: ICELL8 Single-Cell System, BD Rhapsody™ Single-Cell Analysis System, Illumina Bio-Rad Single-Cell Sequencing Solution and 10x Chromium Single Cell Gene Expression Solution.
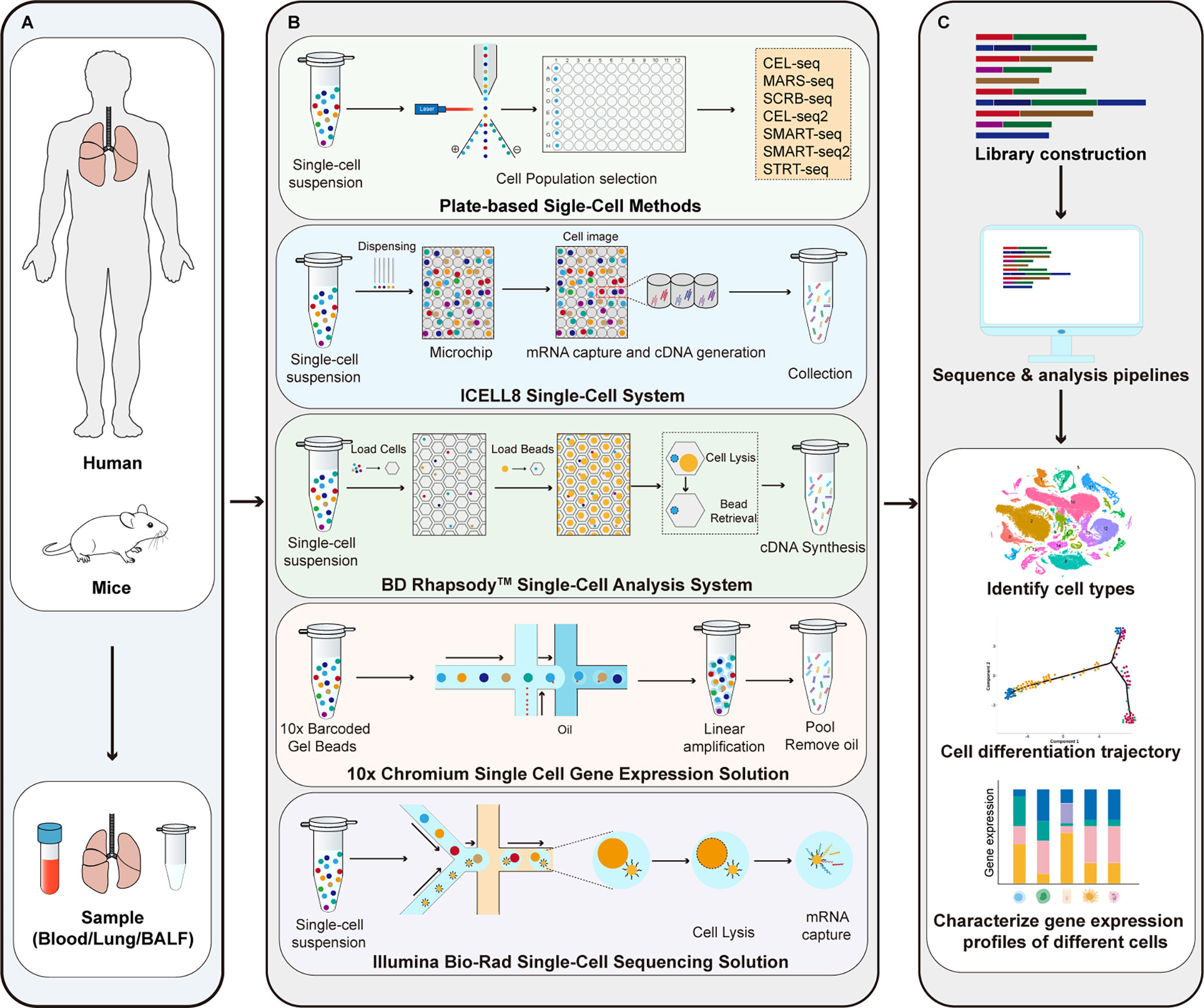
Figure 1 Principle of scRNA-seq technologies. (A) Sample preparation; (B) Single-cell library construction and sequencing; (C) Bioinformatics data analysis. CEL-seq, cell expression by linear amplification and sequencing; MARS-seq, massively parallel single-cell RNA sequencing; SCRB-seq, single-cell RNA barcoding and sequencing; SMART-seq, switching mechanism at 5’ end of the RNA transcript; STRT-seq, single-cell tagged reverse transcription sequencing.
ScRNA-seq can obtain the transcriptome expression data of each cell, accurately present the differences in gene expression between different cells, so as to re-understand the role of various tissues and organs in the pathogenesis of disease at the single-cell level, and has irreplaceable advantages in the study of heterogeneous diseases (35). ScRNA-seq has been applied in the study of asthma, mainly targeting immune cells (T cells, DCs, ILCs, macrophages, mast cells, neutrophils, eosinophils, etc.) and affected histiocytes (airway epithelial cells, goblet cells, etc.) involved in the pathogenesis. Therefore, it provides new technical support for elucidating its pathogenesis and exploring new therapeutic targets. This article categorizes published studies by cell type to reveal the role of different cell populations and interactions between populations in asthma pathogenesis (Table 2). The main findings of scRNA-seq in asthma are summarized in Figure 2. The key words used in the literature retrieval were “asthma” and “single cell transcriptome” or “scRNA”. We manually screened the literatures, excluding reviews and literature with non-asthmatic patients or non-asthmatic models.
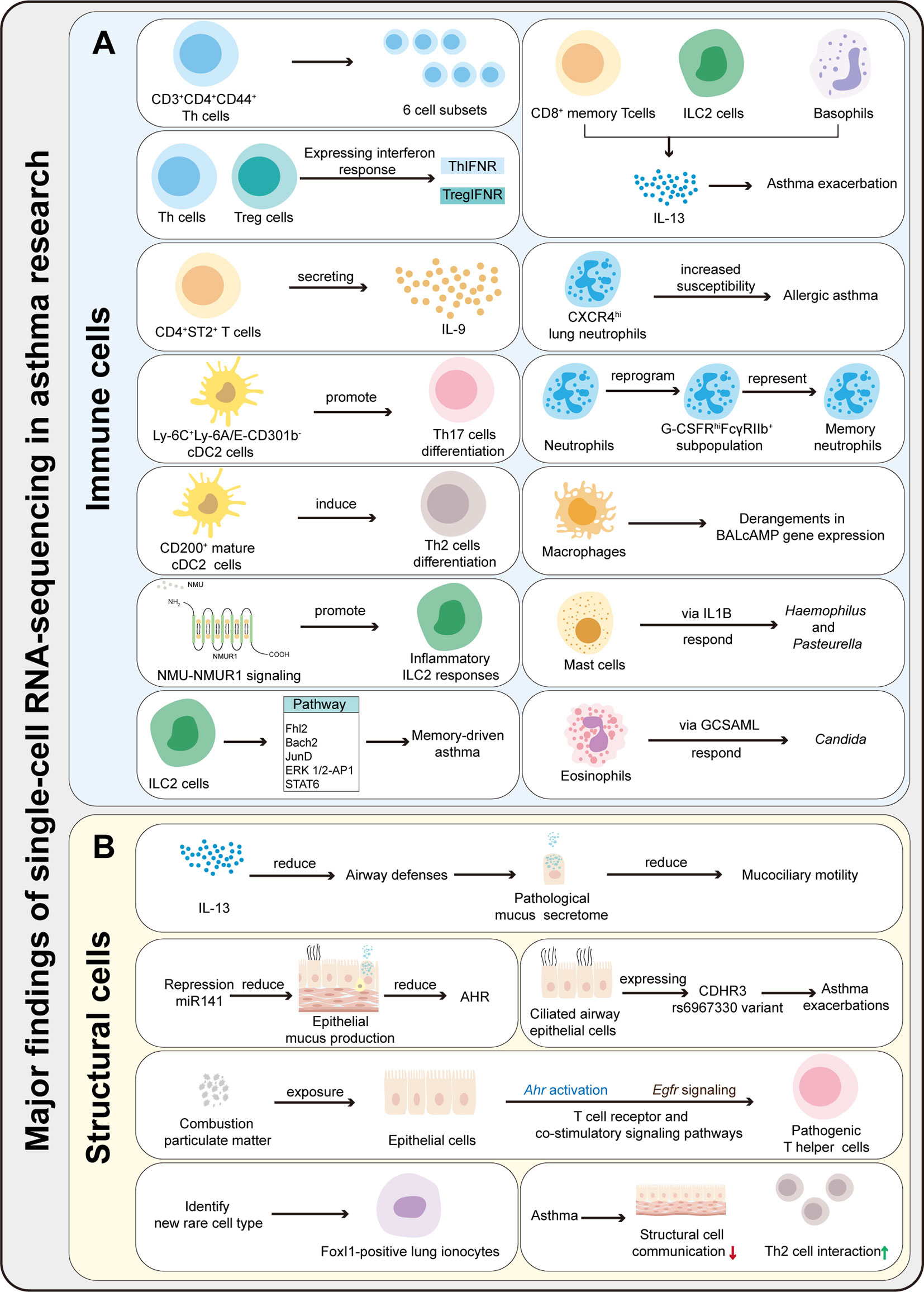
Figure 2 Summary of the major findings resulting from scRNA-seq application in asthma research. (A) ScRNA-seq can be used to characterize the heterogeneity and function of immune cells, identify rare cells and new cell subsets, and explores immune cells differentiation mechanisms and pathways in asthma. (B) ScRNA-seq can be used to characterize the function of structural cells, identify rare cells, and explore the communication between immune and structural cells.
Studies on immune cells associated with asthma
T cell subsets, as the key participants of airway inflammation in asthma, are the focus of research on the pathogenesis of asthma. There is meaningful heterogeneity between and within Th subsets. Th1/Th2 imbalance caused by T cell differentiation disorder is one of the important mechanisms of asthma (56, 57). In recent years, some new T cell subtypes and their roles in asthma have been gradually recognized, including regulatory T (Treg), Th17 etc (58).
Th cells play an important role in the pathogenesis of asthma, but their transcriptional characteristics in the pathological progression of asthma remain unclear. Christopher Andrew Tibbitt performed scRNA-seq on Th cells in the house dust mite (HDM) model (49), and found that there were a large number of Foxp3+Treg, Th1, Th2 and Th17 cell subsets in the airway, while there were fewer Th-related cell subsets in lung tissue and lymph nodes. In this study, SMART-Seq2 platform was used to perform scRNA-seq on CD3+CD4+CD44+ Th cells in the airway. Through two independent experiments, the expression of 2000-2500 genes was detected in each cell, and a total of more than 12000 genes were detected in all cells, of which 1971 genes were significantly differentially expressed. Further analysis showed that these CD3+CD4+CD44+Th cells were divided into 6 subpopulations, all expressing Cd4, Cd3e, Cd3g, Cd3d and Cd44. Cd200r1, Il6, Plac8 and Igfbp7 were characteristic genes of Th2 cells, which were significantly enriched in the airway. ScRNA-seq revealed the diversity of Th cells, enriched the related pathways of lipid metabolism in Th2 subsets, elucidated the pathways of Th2 cell differentiation, and suggested that glycolysis and lipid metabolism play a significant role in driving Th2 cell-mediated immune response (49).
Grégory Seumois et al. analyzed the scRNA-seq of Th cells and Treg cells from asthmatics with HDM allergy and revealed new T cell subsets (36). Th and Treg subsets expressed interferon response signature in asthmatic patients without HDM allergy compared with asthmatic patients with HDM allergy. TNFSF10 was enriched in these subsets, and its product, tumor necrosis factor-related apoptosis-inducing ligand, inhibited Th cell activation (36). These findings suggested that these subsets might suppress allergic responses, which helped to explain why only a small percentage of people developed Th2 responses to the nearly ubiquitous allergens. In peripheral blood of patients with allergy and asthma, allergen-specific CD4+ T cells secreted IL-9, which was significantly reduced after successful immunotherapy, suggesting that IL-9 was an important secreted product of long-term stimulation of allergenic T cells (36, 59).
Similarly, Benjamin J. Ulrich et al. found that IL-9, which was produced by allergen-specific recall and amplified by IL-33, was required for recall responses (45). More importantly, they discovered a special subset of IL-9-secreting CD4+ ST2+ T cells by scRNA-seq and scATAC-seq, which were tissue resident cells and acted as a long-term memory population, mediating rapid responses to allergen challenges. Meanwhile, using whole lung scRNA-seq, they found that IL-9 could affect the expansion and gene expression of many cells, such as CD4+ T cells, ILC2s and B cells. ScRNA-seq analysis identified and discovered the roles of IL-9-secreting CD4+ ST2+ T subsets in specific environments and interactions with other cells, more specific and detailed than previously known T cell subsets.
As antigen-presenting cells, DCs play an important role in the allergen-driven Th2 immune response in asthmatic airways (60). Gentaro Izumi et al. identified five distinct lung conventional CD11b+ DCs (cDC2) clusters by scRNA-Seq technology, in which the Ly-6C+ cDC2 subgroup rapidly accumulated in the lung after allergic sensitization and could strongly promote Th17 differentiation in vitro. Pseudo-temporal analysis of scRNA-Seq data and transfer experiments of cDC2 subsets revealed that partially mature Ly-6C+Ly-6A/E-CD301b-cDC2 expressed Il1b can promoted Th17 differentiation. In contrast, CD200+ mature cDC2 strongly induced Th2 differentiation, but not Th17 differentiation. Therefore, lung cDC2 promoted Th17 and Th2 differentiation at different maturation stages (48).
Although ILCs represent only a small part of the lung immune system, they play a crucial role in early response to pathogens and in facilitating the acquisition of adaptive immunity, and are active participants in the development of asthma (61). Antonia Wallrapp et al. used scRNA-seq to analyze ILCs in mouse lungs at steady state or stimulation with IL-25 and IL-33, and identified that Neuromedin U (NMU)-NMUR1 signaling promoted inflammatory ILC2 responses. Consistent with reports that NMU knockout mice had attenuated airway inflammation after sensitization (62), Antonia Wallrapp et al. found that NMU, NMUR1, could activate ILC2 in vitro, and concurrent administration of NMU and IL-25 amplified inflammation in vivo. Conversely, loss of this signal reduced the number and function of ILC2s and altered the transcriptional program after excitation (40).
Mukesh Verma et al. suggested that in asthma, ILCs and other cells establish a mutually balanced gene repression program (repressors Nr4a2, Zeb1, Bach2 and JunD) and preparation programs (signaling activators Fhl2, FosB, Stat6, Srebf2 and MPP7) in response to repeated stimulation of allergens. ILC2 were found to play an important role in memory-driven asthma. Reciprocal regulation between these two programs established and maintained memory-driven asthma. Activation of Fhl2 by recall allergen stimuli downregulated Bach2 and JunD and activated the ERK1/2-AP1 and STAT6 pathways, inducing a memory-driven asthma phenotype (42).
Lingli Wang et al. (43) established a mouse model of HDM/LPS-induced exacerbation of steroid-resistant asthma and analyzed immune cells in the lung by scRNA-seq. CD11b+macrophages, multiple DCs subpopulations, and ILC2 were found to be potentially involved in steroid-resistant AHR and airway inflammation. They identified that IL-13 was predominantly expressed by CD8+ memory T cells, ILC2, and basophils after HDM and LPS challenge, and its expression was largely steroid-resistant in the first two cells, suggesting that IL-13 was a key cytokine driving the pathogenesis of asthma exacerbation. At the same time, they identified multicellular signaling pathways (“EIF2 signaling”, “oxidative phosphorylation”, “Rho family GTPases signaling”) that were strongly associated with disease progression during exacerbation. Their study found that common or unique host responses at the single-cell level were regulated by a large number of low-risk intracellular events that were unlikely to be easily identified by conventional analytical methods, showing the research advantages of scRNA-seq. Furthermore, their single-cell analysis of lung immune cells added to the understanding of the cellular composition of lung and provided an important resource of information on immune cell networks in the lung during asthma exacerbation and steroid resistance, providing a comprehensive reference data of mouse cell atlas.
Neutrophils are crucial in type 2 allergic immunity, as well as the pro-allergic environment (low-dose of microorganisms, respiratory viral infections, and air pollutants) and host allergy susceptibility. Using scRNA-seq technology to study three different mouse models of allergic asthma, Coraline Radermecker et al. found that neutrophil extracellular traps released CXCR4hi lung neutrophils as an early stage of type 2 inflammation triggering factors. These neutrophils promoted allergen uptake by lung CD11b+Ly-6C+ DCs and increased susceptibility. In this study, scRNA-seq analysis confirmed that there were significant differences in lung neutrophils in the inflammatory environment induced by low-dose and high-dose lipopolysaccharide exposure (41). Furthermore, Zhiwei Li et al. identified the inflammatory neutrophils with specific molecular features of innate immune memory (G-CSFRhiFcγRIIb+) by scRNA-seq on lung tissues of asthmatic mice, which suggested that inflammatory memory neutrophils might aggravate asthma (46).
Ailu Chen et al. used DropSeq for scRNA-Seq technology to study the response of peripheral blood mononuclear cells (PBMCs) from severe asthmatic individuals and healthy individuals to the TLR3 agonist Poly I:C. They found that CD4+ T cells were predominant cell type in severe asthma, and exhibited a pro-inflammatory signature of increased JAK1 expression. Following Poly I:C stimulation, PBMCs from severe asthma strongly induced the interferon pathway compared with controls. This study found that the response of cells from asthmatics to interferon stimulation was not impaired (37).
Kaveh Moghbeli et al. measured the cell specificity of the bronchoalveolar lavage (BAL) cAMP gene network through transcriptome analysis of human lung parenchyma, BAL, and monocyte cell lines. They found that expression of the BALcAMP gene network was restrained under the influence of severe asthma and prolonged β-agonist exposure. Disturbances in this signaling were seen in many cell populations, especially macrophages in the alveolar and interstitial spaces, and might have implications for immune effector function (44).
Hui Li et al. used scRNA-seq to explore the immune status of BALF in patients with asthma exacerbations and found increased levels of CD8+ T cells, several monocyte clusters, and a monocyte-derived macrophage subset (39).They also found that an additional group of core exacerbation-associated modules was activated, including eukaryotic initiation factor 2 signaling, ephrin receptor signaling, and C-X-C chemokine receptor type 4 signaling in the subpopulations of CD8+ T cells (C1-a) and monocyte clusters (C7 clusters), which were associated with infection. Their analysis provided an insightful framework for understanding the shared and distinct expression patterns of inflammatory genes at the individual-cell level.
Airway microbial disturbances are strongly linked to the onset and severity of asthma, but the underlying link is unknown. Daniel Spakowicz et al. developed an integrated dimensionality reduction and statistical modeling approach, linking human genes, cells as well as microbes and identified their relationships. They validated the method with scRNA-seq and studied sputum from asthmatics and found unknown interactions between microbes and genes, such as mast cells responding to Haemophilus and Pasteurella via IL1B, while eosinophils responding to Candida via GCSAML (38).
Studies on structural cells associated with asthma
Mucin is the main component of respiratory mucus, which can moisten airway, absorb impurities, clean and expel foreign bodies. If the secretion of mucin increased in the airway, small airway lumen will be obstructed or spasm, which will induce asthma. Therefore, epithelial disfunction and airway remodeling (pathological mucus increase due to goblet cell metaplasia, submucosal gland hyperplasia, etc.) are typical features of asthma (20). The development of scRNA-seq technology has enabled people to clearly understand the role of various structural cells in the airway during asthma attacks.
The type 2 inflammatory factor IL-13 has vital roles in structural cells (22, 23), such as epithelial goblet cell metaplasia (24) and mucin glycoprotein MUC5AC secretion (25, 26). Nathan D. Jackson et al. performed scRNA-seq using cultured airway epithelial cells and found that IL-13 induced an emergent mucus secretion state in multiple cell types. It was demonstrated that IL-13-induced airway mucus obstruction was an important feature of type 2 high asthma, and that IL-13 generally reduced innate airway defenses, resulting in a pathological mucus secretome that prevented mucociliary motility (52). Asthma is associated with changes in the structure and function of the airway epithelium, a key site of SARS-CoV-2 infection (63, 64). The altered expression of many asthmatic airway epithelial genes is driven by the type 2 cytokine IL-13 (26). Luke R. Bonser et al. used scRNA-seq to identify cytokine-induced changes in SARS-CoV-2-related gene expression in human bronchial epithelial cells (HBECs) and correlated with gene expression changes in the airway epithelium of patients with mild-to-moderate asthma (65). Their results identified that many IL-13-regulated SARS-CoV-2-related genes detected in HBECs were also altered in type 2 high asthma. IL-13 pretreatment reduced viral RNA recovery from SARS-CoV-2 infected cells and reduced dsRNA, a marker of viral replication, and mucus also inhibited viral infection. It was suggested that the stimulation of HBECs with IL-13 affected the expression of many SARS-CoV-2-related genes and largely inhibited the infection of these cells against SARS-CoV-2, which just explained the relatively low prevalence of asthma in patients diagnosed with COVID-19.
MiR-141 is abundantly expressed in branching airways (66) and has many predicted mucus-related targets (67). Sana Siddiqui et al. found that disruption of miR-141 resulted in reduced numbers of goblet cells, MUC5AC and total secreted mucus induced by IL-13 stimulation. The results were consistent with a decrease in goblet cell gene expression and an increase in basal cell gene expression obtained by scRNA-seq analysis (50).
Jamie L. Everman et al. performed scRNA-seq on whole lung cell suspensions and found that the cadherin-related family member 3 (CDHR3) protein was exclusively expressed in ciliated airway epithelial cells. They also revealed the underlying mechanism on the risk of asthma exacerbations conferred by the CDHR3 rs6967330 variant (53).
Using scRNA-seq analysis, Jeffrey N. Harding et al. found increased Il-17 signaling, Ahr activation, Egfr signaling, T cell receptor and co-stimulatory signaling pathways in epithelial cells when exposed to particulate matter. Their data proved that particulate matter promoted an eTh17-specific inflammatory response leading to neutrophilic asthma through pathways in epithelial, DCs and T cells (55).
As a relatively rare cell type, lung ionocytes can express the ion transporter and cystic fibrosis CFTR gene and play a role in regulating the transport of ions and fluids through the airway epithelium and the pH of the mucosal surface. Daniel T. Montoro et al. studied the cellular composition of mouse tracheal epithelium and identified FoxI1-positive lung ionocytes using scRNA-seq and in vivo lineage tracing. Deletion of the related gene Foxi1 in mouse ionocyte might result in loss of Cftr expression and disrupted airway mucus physiology (51).
The interaction of lung structural cells and immune cells is critical for maintaining lung homeostasis. Felipe A. Vieira Braga et al. used scRNA-seq to understand the cellular distribution of the lower airways in healthy and asthmatic patients. At the same time, they identified a new subset of tissue-resident memory T cells and a new mucociliary cell state in asthmatic airway epithelium. In addition, they found other asthma-related changes, including an increase in goblet cells, intraepithelial mast cells, and pathologic effector Th2 cells in the airway wall. Analysis of cell interactions in healthy subjects and asthmatic patients showed that structural cell interactions were significantly reduced with a significant increase in Th2 cells interactions. This study provided new insights into changes in epithelial cells, suggesting that altered patterns of communication between immune and structural cells underlied asthmatic airway inflammation (54). It’s worth noting that Vieira Braga et al. did not identify specific clusters of neuroendocrine cells by scRNA-seq, so supervised analysis using neuroendocrine cell marker genes identified a small number of cells with neuroendocrine-like features present only in lower airways.
Discussion
The advent and rapid development of scRNA-seq technology has made it possible to study individual cells in tissues in depth, classify these cells into cell types, and characterize variations in their molecular profiles as a function of genetics, environment, cell–cell interactions, developmental processes, aging, or disease. Only by understanding the heterogeneity of cells can we make a breakthrough in the study of their common manifestations. The pathogenesis of asthma is affected by a variety of factors. There are acute phase and chronic remission phase of asthma, and the status of related cells may be different in different periods. The identification pathways and molecular events of asthma exacerbation are key areas of asthma. However, previous asthma transcriptomic studies have been limited in their understanding of the cellular and molecular composition of the lung immune response by the lack of adequate tools to study differences between cells that are histologically indistinguishable. Th cells, in particular, have subsets that are limited by assessment at independent time points and in response to different cytokine environments (68–70). These differences need to be further verified by methods such as single-cell sequencing.
The currently published literatures on lung tissue scRNA-seq has increased the understanding of lung cell composition and provided a comprehensive and important source of data for constructing cell atlas of human and mouse. Kyle J. Travaglini et al. applied droplet- and plate-based scRNA-seq to systematically identify the cell populations and gene expression profiles involved in lung tissue, created a molecular cell atlas (71). This atlas elucidated the biochemical functions of lung cell types and cell-selective transcription factors, while also provided a molecular basis for studying how lung cell identity, function, and interactions altered in development and asthma.
Although scRNA-seq technology has matured, there is still room for further improvement. Firstly, the preparation of tissue single cell suspensions is complicated, which poses a challenge to the classification of some cell populations in samples. Some single-cell isolation technology may have impact on cell integrity, resulting in data distortion or loss (29), such as FACS sorting may have non-negligible effects on cell viability of Chinese Hamster Ovary cells and for a human monocytic cell line (THP1) (72). The different types of cells that make up the tissue have different conditions of response to different digestive enzymes. In order to obtain as many kinds of tissue cells as possible, it is necessary to explore experimental methods of enzymatic digestion. Secondly, maintaining fidelity and avoiding bias during large-scale amplification is not an easy task. Moreover, another limitation of this technology is the relatively shallow sequencing “depth” of individual cells, which largely limits the discovery of high expressed genes (73), such as neuroendocrine cells. Limits on the number of cells that can be sequenced can hamper the identification of rare cells. The cost of scRNA-seq technology is still high, which affects its wide application. The bioinformatics analysis of scRNA-seq is currently carried out by experts in professional fields. Creating simplified data analysis methods and unified processing strategies is also an urgent problem to be solved.
Currently, there are only a limited number of scRNA-seq studies on asthma, and most have used mice models. Although there are many similarities between asthma patients and airway allergic mice, and mice are widely used in preclinical studies of asthma, there are crucial differences between human and mice lungs at the anatomical and cellular levels that should be taken with caution when extrapolating human asthma from mice data (74, 75). Lung organoids also have considerable potential to discover new therapeutic approaches for diseases such as asthma (76), and scRNA-seq can be used to identify the origin of cellular composition of lung organoids (77) to explore their differentiation mechanisms and pathways involved (78). Recent studies showed that single-cell omics researches had been extended from transcriptome to multi-omics, and single-cell multi-omics sequencing technology will more comprehensively and accurately reveal the specific mechanism of various lung cells and their subtypes in the pathogenesis of asthma.
Author contributions
WW, WT and JD carried out the concepts, design, definition of intellectual content, literature search and manuscript preparation. WW, WT drafted the manuscript and figures. ML carried out the literature search and manuscript modification. JD helped perform the manuscript with constructive discussions. FT and JC provided assistance for literature search and manuscript editing. All authors have read and approved the content of the manuscript.
Funding
This work was supported by grants from the National Natural Science Foundation of China (Grant No. 82174170), Research Fund of Huashan Hospital affiliated to Fudan University (2021QD042), the Innovative Research Team of High-level Local Universities in Shanghai, and Expert workstation for Jingcheng Dong in Yunnan Province (20210101) Province (20210101).
Conflict of interest
The authors declare that the research was conducted in the absence of any commercial or financial relationships that could be construed as a potential conflict of interest.
Publisher’s note
All claims expressed in this article are solely those of the authors and do not necessarily represent those of their affiliated organizations, or those of the publisher, the editors and the reviewers. Any product that may be evaluated in this article, or claim that may be made by its manufacturer, is not guaranteed or endorsed by the publisher.
References
1. Victor JR, Lezmi G, Leite-de-Moraes M. New insights into asthma inflammation: Focus on inkt, mait, and gammadeltat cells. Clin Rev Allergy Immunol (2020) 59(3):371–81. doi: 10.1007/s12016-020-08784-8
2. Barcik W, Boutin RCT, Sokolowska M, Finlay BB. The role of lung and gut microbiota in the pathology of asthma. Immunity (2020) 52(2):241–55. doi: 10.1016/j.immuni.2020.01.007
3. Huang K, Yang T, Xu J, Yang L, Zhao J, Zhang X, et al. Prevalence, risk factors, and management of asthma in China: A national cross-sectional study. Lancet (2019) 394(10196):407–18. doi: 10.1016/S0140-6736(19)31147-X
4. Papi A, Brightling C, Pedersen SE, Reddel HK. Asthma. Lancet (2018) 391(10122):783–800. doi: 10.1016/S0140-6736(17)33311-1
5. Caramori G, Nucera F, Mumby S, Lo Bello F, Adcock IM. Corticosteroid resistance in asthma: Cellular and molecular mechanisms. Mol Aspects Med (2022) 85:100969. doi: 10.1016/j.mam.2021.100969
6. Akdis CA. Therapies for allergic inflammation: Refining strategies to induce tolerance. Nat Med (2012) 18(5):736–49. doi: 10.1038/nm.2754
7. Rabe KF, Nair P, Brusselle G, Maspero JF, Castro M, Sher L, et al. Efficacy and safety of dupilumab in glucocorticoid-dependent severe asthma. N Engl J Med (2018) 378(26):2475–85. doi: 10.1056/NEJMoa1804093
8. Pelaia C, Pelaia G, Longhini F, Crimi C, Calabrese C, Gallelli L, et al. Monoclonal antibodies targeting alarmins: A new perspective for biological therapies of severe asthma. Biomedicines (2021) 9(9):1108. doi: 10.3390/biomedicines9091108
9. Chupp GL, Kaur R, Mainardi A. New therapies for emerging endotypes of asthma. Annu Rev Med (2020) 71:289–302. doi: 10.1146/annurev-med-041818-020630
10. Moffatt MF, Gut IG, Demenais F, Strachan DP, Bouzigon E, Heath S, et al. A Large-scale, consortium-based genomewide association study of asthma. N Engl J Med (2010) 363(13):1211–21. doi: 10.1056/NEJMoa0906312
11. Portelli MA, Hodge E, Sayers I. Genetic risk factors for the development of allergic disease identified by genome-wide association. Clin Exp Allergy (2015) 45(1):21–31. doi: 10.1111/cea.12327
12. Zaongo SD, Harypursat V, Chen Y. Single-cell sequencing facilitates elucidation of hiv immunopathogenesis: A review of current literature. Front Immunol (2022) 13:828860. doi: 10.3389/fimmu.2022.828860
13. Van Hulst G, Bureau F, Desmet CJ. Eosinophils as drivers of severe eosinophilic asthma: Endotypes or plasticity? Int J Mol Sci (2021) 22(18):10150. doi: 10.3390/ijms221810150
14. Dash B, Sun X. Eosinophils set DNA traps in allergic asthma. Nat Cell Biol (2021) 23(10):1057–9. doi: 10.1038/s41556-021-00765-z
15. Monticelli LA, Osborne LC, Noti M, Tran SV, Zaiss DM, Artis D. Il-33 promotes an innate immune pathway of intestinal tissue protection dependent on amphiregulin-egfr interactions. Proc Natl Acad Sci U.S.A. (2015) 112(34):10762–7. doi: 10.1073/pnas.1509070112
16. Monticelli LA, Sonnenberg GF, Abt MC, Alenghat T, Ziegler CG, Doering TA, et al. Innate lymphoid cells promote lung-tissue homeostasis after infection with influenza virus. Nat Immunol (2011) 12(11):1045–54. doi: 10.1031/ni.2131
17. Ekstedt S, Stenberg H, Tufvesson E, Diamant Z, Bjermer L, Kumlien Georen S, et al. The potential role of Cd16(High) Cd62l(Dim) neutrophils in the allergic asthma. Allergy (2019) 74(11):2265–8. doi: 10.1111/all.13861
18. Krishnamoorthy N, Douda DN, Bruggemann TR, Ricklefs I, Duvall MG, Abdulnour RE, et al. Neutrophil cytoplasts induce Th17 differentiation and skew inflammation toward neutrophilia in severe asthma. Sci Immunol (2018) 3(26):eaao4747. doi: 10.1126/sciimmunol.aao4747
19. Radermecker C, Louis R, Bureau F, Marichal T. Role of neutrophils in allergic asthma. Curr Opin Immunol (2018) 54:28–34. doi: 10.1016/j.coi.2018.05.006
20. Heijink IH, Kuchibhotla VNS, Roffel MP, Maes T, Knight DA, Sayers I, et al. Epithelial cell dysfunction, a major driver of asthma development. Allergy (2020) 75(8):1902–17. doi: 10.1111/all.14421
21. Fehrenbach H, Wagner C, Wegmann M. Airway remodeling in asthma: What really matters. Cell Tissue Res (2017) 367(3):551–69. doi: 10.1007/s00441-016-2566-8
22. Chen G, Korfhagen TR, Xu Y, Kitzmiller J, Wert SE, Maeda Y, et al. Spdef is required for mouse pulmonary goblet cell differentiation and regulates a network of genes associated with mucus production. J Clin Invest (2009) 119(10):2914–24. doi: 10.1172/JCI39731
23. Park SW, Verhaeghe C, Nguyenvu LT, Barbeau R, Eisley CJ, Nakagami Y, et al. Distinct roles of Foxa2 and Foxa3 in allergic airway disease and asthma. Am J Respir Crit Care Med (2009) 180(7):603–10. doi: 10.1164/rccm.200811-1768OC
24. Yu H, Li Q, Kolosov VP, Perelman JM, Zhou X. Interleukin-13 induces mucin 5ac production involving Stat6/Spdef in human airway epithelial cells. Cell Commun Adhes (2010) 17(4-6):83–92. doi: 10.3109/15419061.2010.551682
25. Bonser LR, Zlock L, Finkbeiner W, Erle DJ. Epithelial tethering of Muc5ac-rich mucus impairs mucociliary transport in asthma. J Clin Invest (2016) 126(6):2367–71. doi: 10.1172/JCI84910
26. Woodruff PG, Modrek B, Choy DF, Jia G, Abbas AR, Ellwanger A, et al. T-Helper type 2-driven inflammation defines major subphenotypes of asthma. Am J Respir Crit Care Med (2009) 180(5):388–95. doi: 10.1164/rccm.200903-0392OC
27. Hansson GC. Mucus and mucins in diseases of the intestinal and respiratory tracts. J Intern Med (2019) 285(5):479–90. doi: 10.1111/joim.12910
28. Potter SS. Single-cell rna sequencing for the study of development, physiology and disease. Nat Rev Nephrol (2018) 14(8):479–92. doi: 10.1038/s41581-018-0021-7
29. Gross A, Schoendube J, Zimmermann S, Steeb M, Zengerle R, Koltay P. Technologies for single-cell isolation. Int J Mol Sci (2015) 16(8):16897–919. doi: 10.3390/ijms160816897
30. Guo F, Li L, Li J, Wu X, Hu B, Zhu P, et al. Single-cell multi-omics sequencing of mouse early embryos and embryonic stem cells. Cell Res (2017) 27(8):967–88. doi: 10.1038/cr.2017.82
31. Nichterwitz S, Chen G, Aguila Benitez J, Yilmaz M, Storvall H, Cao M, et al. Laser capture microscopy coupled with smart-Seq2 for precise spatial transcriptomic profiling. Nat Commun (2016) 7:12139. doi: 10.1038/ncomms12139
32. Shapiro E, Biezuner T, Linnarsson S. Single-cell sequencing-based technologies will revolutionize whole-organism science. Nat Rev Genet (2013) 14(9):618–30. doi: 10.1038/nrg3542
33. Saei A, Asfia S, Kouchakzadeh H, Rahmandoust M. Antibody-modified magnetic nanoparticles as specific high-efficient cell-separation agents. J BioMed Mater Res B Appl Biomater (2020) 108(6):2633–42. doi: 10.1002/jbm.b.34595
34. Gierahn TM, Wadsworth MH 2nd, Hughes TK, Bryson BD, Butler A, Satija R, et al. Seq-well: Portable, low-cost rna sequencing of single cells at high throughput. Nat Methods (2017) 14(4):395–8. doi: 10.1038/nmeth.4179
35. Papalexi E, Satija R. Single-cell rna sequencing to explore immune cell heterogeneity. Nat Rev Immunol (2018) 18(1):35–45. doi: 10.1038/nri.2017.76
36. Seumois G, Ramirez-Suastegui C, Schmiedel BJ, Liang S, Peters B, Sette A, et al. Single-cell transcriptomic analysis of allergen-specific T cells in allergy and asthma. Sci Immunol (2020) 5(48):eaba6087. doi: 10.1126/sciimmunol.aba6087
37. Chen A, Diaz-Soto MP, Sanmamed MF, Adams T, Schupp JC, Gupta A, et al. Single-cell characterization of a model of poly I:C-stimulated peripheral blood mononuclear cells in severe asthma. Respir Res (2021) 22(1):122. doi: 10.1186/s12931-021-01709-9
38. Spakowicz D, Lou S, Barron B, Gomez JL, Li T, Liu Q, et al. Approaches for integrating heterogeneous rna-seq data reveal cross-talk between microbes and genes in asthmatic patients. Genome Biol (2020) 21(1):150. doi: 10.1186/s13059-020-02033-z
39. Li H, Wang H, Sokulsky L, Liu S, Yang R, Liu X, et al. Single-cell transcriptomic analysis reveals key immune cell phenotypes in the lungs of patients with asthma exacerbation. J Allergy Clin Immunol (2021) 147(3):941–54. doi: 10.1016/j.jaci.2020.09.032
40. Wallrapp A, Riesenfeld SJ, Burkett PR, Abdulnour RE, Nyman J, Dionne D, et al. The neuropeptide nmu amplifies Ilc2-driven allergic lung inflammation. Nature (2017) 549(7672):351–6. doi: 10.1038/nature24029
41. Radermecker C, Sabatel C, Vanwinge C, Ruscitti C, Marechal P, Perin F, et al. Locally instructed Cxcr4(Hi) neutrophils trigger environment-driven allergic asthma through the release of neutrophil extracellular traps. Nat Immunol (2019) 20(11):1444–55. doi: 10.1038/s41590-019-0496-9
42. Verma M, Michalec L, Sripada A, McKay J, Sirohi K, Verma D, et al. The molecular and epigenetic mechanisms of innate lymphoid cell (Ilc) memory and its relevance for asthma. J Exp Med (2021) 218(7):e20201354. doi: 10.1084/jem.20201354
43. Wang L, Netto KG, Zhou L, Liu X, Wang M, Zhang G, et al. Single-cell transcriptomic analysis reveals the immune landscape of lung in steroid-resistant asthma exacerbation. Proc Natl Acad Sci U.S.A. (2021) 118(2):e2005590118. doi: 10.1073/pnas.2005590118
44. Moghbeli K, Valenzi E, Naramore R, Sembrat JC, Chen K, Rojas MM, et al. B-agonist exposure preferentially impacts lung macrophage cyclic amp-related gene expression in asthma and asthma copd overlap syndrome. Am J Physiol Lung Cell Mol Physiol (2021) 321(5):L837–l43. doi: 10.1152/ajplung.00260.2021
45. Ulrich BJ, Kharwadkar R, Chu M, Pajulas A, Muralidharan C, Koh B, et al. Allergic airway recall responses require il-9 from resident memory Cd4(+) T cells. Sci Immunol (2022) 7(69):eabg9296. doi: 10.1126/sciimmunol.abg9296
46. Li Z, Feng Y, Liu H, Yang H, Li J, Zhou Y, et al. Single-cell transcriptomics of mouse lung reveal inflammatory memory neutrophils in allergic asthma. Allergy (2022) 77(6):1911–5. doi: 10.1111/all.15286
47. Jiang J, Faiz A, Berg M, Carpaij OA, Vermeulen CJ, Brouwer S, et al. Gene signatures from scrna-seq accurately quantify mast cells in biopsies in asthma. Clin Exp Allergy (2020) 50(12):1428–31. doi: 10.1111/cea.13732
48. Izumi G, Nakano H, Nakano K, Whitehead GS, Grimm SA, Fessler MB, et al. Cd11b(+) lung dendritic cells at different stages of maturation induce Th17 or Th2 differentiation. Nat Commun (2021) 12(1):5029. doi: 10.1038/s41467-021-25307-x
49. Tibbitt CA, Stark JM, Martens L, Ma J, Mold JE, Deswarte K, et al. Single-cell rna sequencing of the T helper cell response to house dust mites defines a distinct gene expression signature in airway Th2 cells. Immunity (2019) 51(1):169–84.e5. doi: 10.1016/j.immuni.2019.05.014
50. Siddiqui S, Johansson K, Joo A, Bonser LR, Koh KD, Le Tonqueze O, et al. Epithelial mir-141 regulates il-13-Induced airway mucus production. JCI Insight (2021) 6(5):e139019. doi: 10.1172/jci.insight.139019
51. Montoro DT, Haber AL, Biton M, Vinarsky V, Lin B, Birket SE, et al. A revised airway epithelial hierarchy includes cftr-expressing ionocytes. Nature (2018) 560(7718):319–24. doi: 10.1038/s41586-018-0393-7
52. Jackson ND, Everman JL, Chioccioli M, Feriani L, Goldfarbmuren KC, Sajuthi SP, et al. Single-cell and population transcriptomics reveal pan-epithelial remodeling in type 2-high asthma. Cell Rep (2020) 32(1):107872. doi: 10.1016/j.celrep.2020.107872
53. Everman JL, Sajuthi S, Saef B, Rios C, Stoner AM, Numata M, et al. Functional genomics of Cdhr3 confirms its role in hrv-c infection and childhood asthma exacerbations. J Allergy Clin Immunol (2019) 144(4):962–71. doi: 10.1016/j.jaci.2019.01.052
54. Vieira Braga FA, Kar G, Berg M, Carpaij OA, Polanski K, Simon LM, et al. A cellular census of human lungs identifies novel cell states in health and in asthma. Nat Med (2019) 25(7):1153–63. doi: 10.1038/s41591-019-0468-5
55. Harding JN, Gross M, Patel V, Potter S, Cormier SA. Association between particulate matter containing epfrs and neutrophilic asthma through ahr and Th17. Respir Res (2021) 22(1):275. doi: 10.1186/s12931-021-01867-w
56. Lloyd CM, Snelgrove RJ. Type 2 immunity: Expanding our view. Sci Immunol (2018) 3(25):eaat1604. doi: 10.1126/sciimmunol.aat1604
57. Alobaidi AH, Alsamarai AM, Alsamarai MA. Inflammation in asthma pathogenesis: Role of T cells, macrophages, epithelial cells and type 2 inflammation. Antiinflamm Antiallergy Agents Med Chem (2021) 20(4):317–32. doi: 10.2174/1871523020666210920100707
58. Moldaver DM, Larche M, Rudulier CD. An update on lymphocyte subtypes in asthma and airway disease. Chest (2017) 151(5):1122–30. doi: 10.1016/j.chest.2016.10.038
59. Wambre E, Bajzik V, DeLong JH, O'Brien K, Nguyen QA, Speake C, et al. A phenotypically and functionally distinct human Th2 cell subpopulation is associated with allergic disorders. Sci Transl Med (2017) 9(401):eaam9171. doi: 10.1126/scitranslmed.aam9171
60. Morianos I, Semitekolou M. Dendritic cells: Critical regulators of allergic asthma. Int J Mol Sci (2020) 21(21):7930. doi: 10.3390/ijms21217930
61. Hsu AT, Gottschalk TA, Tsantikos E, Hibbs ML. The role of innate lymphoid cells in chronic respiratory diseases. Front Immunol (2021) 12:733324. doi: 10.3389/fimmu.2021.733324
62. Moriyama M, Fukuyama S, Inoue H, Matsumoto T, Sato T, Tanaka K, et al. The neuropeptide neuromedin U activates eosinophils and is involved in allergen-induced eosinophilia. Am J Physiol Lung Cell Mol Physiol (2006) 290(5):L971–7. doi: 10.1152/ajplung.00345.2005
63. Zou L, Ruan F, Huang M, Liang L, Huang H, Hong Z, et al. Sars-Cov-2 viral load in upper respiratory specimens of infected patients. N Engl J Med (2020) 382(12):1177–9. doi: 10.1056/NEJMc2001737
64. Wang W, Xu Y, Gao R, Lu R, Han K, Wu G, et al. Detection of sars-Cov-2 in different types of clinical specimens. JAMA (2020) 323(18):1843–4. doi: 10.1001/jama.2020.3786
65. Bonser LR, Eckalbar WL, Rodriguez L, Shen J, Koh KD, Zlock LT, et al. The type 2 asthma mediator il-13 inhibits sars-Cov-2 infection of bronchial epithelium. bioRxiv (2021) 66(4):391–401. doi: 10.1165/rcmb.2021-0364OC
66. Park CY, Jeker LT, Carver-Moore K, Oh A, Liu HJ, Cameron R, et al. A resource for the conditional ablation of micrornas in the mouse. Cell Rep (2012) 1(4):385–91. doi: 10.1016/j.celrep.2012.02.008
67. Li JH, Zhang Z, Du MZ, Guan YC, Yao JN, Yu HY, et al. Microrna-141-3p fosters the growth, invasion, and tumorigenesis of cervical cancer cells by targeting Foxa2. Arch Biochem Biophys (2018) 657:23–30. doi: 10.1016/j.abb.2018.09.008
68. Tuzlak S, Dejean AS, Iannacone M, Quintana FJ, Waisman A, Ginhoux F, et al. Repositioning Th cell polarization from single cytokines to complex help. Nat Immunol (2021) 22(10):1210–7. doi: 10.1038/s41590-021-01009-w
69. Tortola L, Jacobs A, Pohlmeier L, Obermair FJ, Ampenberger F, Bodenmiller B, et al. High-dimensional T helper cell profiling reveals a broad diversity of stably committed effector states and uncovers interlineage relationships. Immunity (2020) 53(3):597–613 e6. doi: 10.1016/j.immuni.2020.07.001
70. O'Shea JJ, Paul WE. Mechanisms underlying lineage commitment and plasticity of helper Cd4+ T cells. Science (2010) 327(5969):1098–102. doi: 10.1126/science.1178334
71. Travaglini KJ, Nabhan AN, Penland L, Sinha R, Gillich A, Sit RV, et al. A molecular cell atlas of the human lung from single-cell rna sequencing. Nature (2020) 587(7835):619–25. doi: 10.1038/s41586-020-2922-4
72. Mollet M, Godoy-Silva R, Berdugo C, Chalmers JJ. Computer simulations of the energy dissipation rate in a fluorescence-activated cell sorter: Implications to cells. Biotechnol Bioeng (2008) 100(2):260–72. doi: 10.1002/bit.21762
73. Royer DJ, Cook DN. Regulation of immune responses by nonhematopoietic cells in asthma. J Immunol (2021) 206(2):292–301. doi: 10.4049/jimmunol.2000885
74. Finkelman FD, Wills-Karp M. Usefulness and optimization of mouse models of allergic airway disease. J Allergy Clin Immunol (2008) 121(3):603–6. doi: 10.1016/j.jaci.2008.01.008
75. Irvin CG, Bates JH. Measuring the lung function in the mouse: The challenge of size. Respir Res (2003) 4:4. doi: 10.1186/rr199
76. Barkauskas CE, Chung MI, Fioret B, Gao X, Katsura H, Hogan BL. Lung organoids: Current uses and future promise. Development (2017) 144(6):986–97. doi: 10.1242/dev.140103
77. Salahudeen AA, Choi SS, Rustagi A, Zhu J, de la OS, Flynn RA, et al. Progenitor identification and sars-Cov-2 infection in long-term human distal lung organoid cultures. Nature (2020). 588(7839):670–675 doi: 10.1038/s41586-020-3014-1
Keywords: asthma, scRNA-seq, mechanism, immune cell, structural cell
Citation: Tang W, Li M, Teng F, Cui J, Dong J and Wang W (2022) Single-cell RNA-sequencing in asthma research. Front. Immunol. 13:988573. doi: 10.3389/fimmu.2022.988573
Received: 07 July 2022; Accepted: 15 November 2022;
Published: 29 November 2022.
Edited by:
Hiroki Kabata, Keio University School of Medicine, JapanReviewed by:
Hiromasa Inoue, Kagoshima University, JapanZhaowei Yang, Guangzhou Institute of Respiratory Health, China
Copyright © 2022 Tang, Li, Teng, Cui, Dong and Wang. This is an open-access article distributed under the terms of the Creative Commons Attribution License (CC BY). The use, distribution or reproduction in other forums is permitted, provided the original author(s) and the copyright owner(s) are credited and that the original publication in this journal is cited, in accordance with accepted academic practice. No use, distribution or reproduction is permitted which does not comply with these terms.
*Correspondence: Wenqian Wang, d3F3YW5nMTk4OEAxNjMuY29t; Jingcheng Dong, amNkb25nMjAwNEAxMjYuY29t
†These authors have contributed equally to this work