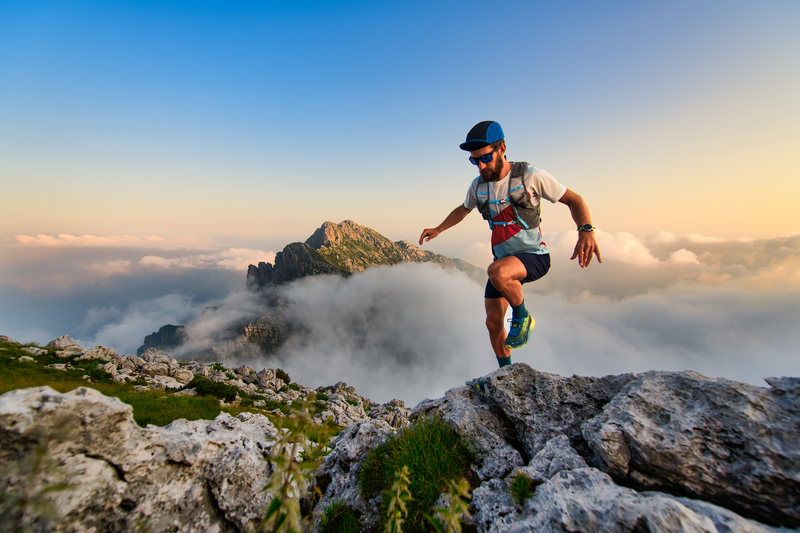
94% of researchers rate our articles as excellent or good
Learn more about the work of our research integrity team to safeguard the quality of each article we publish.
Find out more
ORIGINAL RESEARCH article
Front. Immunol. , 23 September 2022
Sec. Inflammation
Volume 13 - 2022 | https://doi.org/10.3389/fimmu.2022.988479
Background: The coronavirus disease (COVID-19) pandemic has posed a significant challenge for global health systems. Increasing evidence shows that asthma phenotypes and comorbidities are major risk factors for COVID-19 symptom severity. However, the molecular mechanisms underlying the association between COVID-19 and asthma are poorly understood. Therefore, we conducted bioinformatics and systems biology analysis to identify common pathways and molecular biomarkers in patients with COVID-19 and asthma, as well as potential molecular mechanisms and candidate drugs for treating patients with both COVID-19 and asthma.
Methods: Two sets of differentially expressed genes (DEGs) from the GSE171110 and GSE143192 datasets were intersected to identify common hub genes, shared pathways, and candidate drugs. In addition, murine models were utilized to explore the expression levels and associations of the hub genes in asthma and lung inflammation/injury.
Results: We discovered 157 common DEGs between the asthma and COVID-19 datasets. A protein–protein-interaction network was built using various combinatorial statistical approaches and bioinformatics tools, which revealed several hub genes and critical modules. Six of the hub genes were markedly elevated in murine asthmatic lungs and were positively associated with IL-5, IL-13 and MUC5AC, which are the key mediators of allergic asthma. Gene Ontology and pathway analysis revealed common associations between asthma and COVID-19 progression. Finally, we identified transcription factor–gene interactions, DEG–microRNA coregulatory networks, and potential drug and chemical-compound interactions using the hub genes.
Conclusion: We identified the top 15 hub genes that can be used as novel biomarkers of COVID-19 and asthma and discovered several promising candidate drugs that might be helpful for treating patients with COVID-19 and asthma.
Coronavirus disease 2019 (COVID-19) is a respiratory disease caused by severe acute respiratory syndrome coronavirus 2 (SARS-CoV-2) (1, 2). SARS-CoV-2 spreads quickly through respiratory droplets and aerosols produced by coughing and sneezing (3, 4). Coughs, wheezing, shortness of breath, and chest tightness are symptoms of asthma, which is a chronic inflammatory disease of the airways (5, 6). Both COVID-19 and asthma remain major global challenges for public health (7–9). Given the COVID-19 pandemic and the prevalence of allergic asthma, concerns have been raised regarding the possible increased susceptibility of patients with asthma to SARS-CoV-2 infection and disease severity (9, 10).
Previous studies have demonstrated a probable link between SARS-CoV-2 infection and the development of allergic asthma (11–14). Choi et al. discovered a higher prevalence of asthma in the COVID-19 patient population (2.9% versus 1.6–2.2%) (15). Conversely, Izquierdo et al. found a higher prevalence of COVID-19 amongst asthma patients than in the general population (1.41% versus 0.86%) (10). Furthermore, patients with asthma receiving biologic therapy had an even higher incidence of COVID-19 (10). Thus, both investigations imply that patients with asthma are more susceptible to COVID-19 infection than the general population, particularly those with severe asthma who are receiving biologic therapy.
Several studies have reported the potential mechanism associated with COVID-19 and asthma. SARS-CoV-2 enters host cells by binding to angiotensin-converting enzyme 2 (ACE2) via its spike protein, and this process is promoted by the host transmembrane protease serine 2 (TMPRSS2) (16–18). It has been proposed that allergic asthma can have a protective impact via inhibition of the ACE2 receptor or, more speculatively, by counterbalancing the heightened antiviral immune response seen in patients with severe COVID-19 (19, 20). Furthermore, pre-existing eosinophilia—a biomarker of allergic asthma—was found to be protective against hospitalization for COVID-19 infection in a single-center retrospective investigation (21). However, there has also been concern that higher expression of TMPRSS2 found among asthmatics may facilitate SARS-CoV-2 cell entry (22, 23).
Taken together, these findings suggest the strong association between COVID-19 and asthma. However, no definitive conclusions can be drawn yet as many factors can influence the reported incidences of (severe) COVID-19 and asthma. Therefore, it is critical to learn how COVID-19 affects patients with asthma and identify potential beneficial medications for COVID-19-positive asthmatic patients that could minimize the risk of hospitalization or death.
In this study, we discovered several critical cellular-signaling pathways and gene networks that are commonly associated with COVID-19 and asthma. Furthermore, we identified 15 hub genes that might be used as novel biomarkers for targeted therapy in patients with COVID-19 and asthma, and validated them in murine models of asthma and lung inflammation/injury. We also screened out several promising candidate drugs that might be effective therapeutic agents in this population. Herein, we analyzed potential molecular mechanisms and identified several biomarkers and drugs that might be useful for treating patients with COVID-19 and allergic asthma, using a bioinformatics and a systems-biology approach.
To identify common genetic interrelations and correlations between patients with COVID-19 and allergic asthma, we used both RNA-seq and microarray analysis datasets from the Gene Expression Omnibus (GEO) database (National Center for Biotechnology Information; https://www.ncbi.nlm.nih.gov/geo/) (24). For the COVID-19 dataset, we used GEO accession number GSE171110 (25), which contains whole-blood RNA-sequencing (RNA-seq) data from patients with COVID-19 and healthy donors. The data were obtained through high throughput sequencing using the Illumina HiSeq 2500 V4 system. The allergic asthma dataset (GSE143192) (26) was collected from peripheral blood mononuclear cell samples from asthmatic patients and healthy volunteers. The sequencing data were obtained using the Agilent-078298 human ceRNA array, V1.0 4X180K. A summary of the information contained in both datasets is provided in Table 1.
Table 1 Overview of the datasets with their geo-features and quantitative measurements in this analysis.
A gene is considered differentially expressed when a statistically significant difference is observed under different experimental conditions at the transcriptional level (27). The key goal of this analysis was to identify DEGs between case and control data from the datasets GSE171110 and GSE143192, respectively. The DEGs were identified from the long-expression values using the “limma” package of R software (version 4.2.0) with the Benjamini–Hochberg correction to control for the false-discovery rate. Significant DEGs were detected using cut-off criteria (adjusted P-value or P-value < 0.05 and |log fold-change| ≥ 1.0). Common DEGs between both datasets were obtained using Jvenn, an online Venn-analysis tool (http://jvenn.toulouse.inra.fr/app/example.html) (28).
We used EnrichR (https://maayanlab.cloud/enrichr/) (29), a comprehensive online gene-set enrichment tool, to analyze biological processes and signaling pathways associated with the common DEGs. A P-value < 0.05 and a Q value < 0.25 were used as standardized metrics to quantify the top functional items and pathways.
PPIs were identified using the STRING database (https://string-db.org/) (30). The resulting PPI network was processed and examined using Cytoscape (version 3.7.1). The PPI network was built using proteins encoded by the shared DEGs between COVID-19 and asthma datasets. The Markov cluster method, included in the STRING database, was used to find gene clusters. The PPI network of frequent DEGs was built using a composite score greater than 0.4. Genes showing a significant correlation in candidate modules are referred to as hub genes (31). We then used the Cytoscape plug-in, cytoHubba, to rank and examine prominent nodes in the PPI network modules and predict hub genes.
We discovered DEG–microRNA (miRNA)-interaction networks and DEG–transcription factor (TF)-interaction networks using NetworkAnalyst (32). DEG–miRNA-interaction networks were discovered using the TarBase (33) and miRTarBase (34) databases. The JASPAR database (35) was utilized to analyze the TF–DEG-interaction networks. We used common DEGs for the GRN analysis to uncover the transcriptional elements and miRNAs that regulate DEGs at the post-transcriptional level.
We used the DSigDB database to predict protein–drug interactions and identify candidate pharmacological compounds associated with DEGs. We identified drugs targeting common DEGs between COVID-19 and asthma datasets using the Enrichr web server and the DSigDB database based on a statistical threshold of an adjusted P-value <0.05.
DisGeNET (http://www.disgenet.org/) is a knowledge-management platform to integrate and standardize data for disease-associated genes and variations from diverse sources. DisGeNET currently has information related to over 24,000 diseases and characteristics, 17,000 genes, and 117,000 genomic variations (36). The platform is focused on increasing the understanding of human genetic disorders. We also used NetworkAnalyst to investigate gene–disease associations to uncover diseases and chronic issues linked to common DEGs (37).
To establish the house dust mite (HDM)-induced murine asthma model (38), mice were sensitized with 5 µg of HDM (Greer Laboratories) intranasally on days 0, 1, and 2, and subsequently challenged with 5 µg of HDM intranasally on days 8 to 12 to induce allergic asthma. Control mice received PBS during both the sensitization and challenge phases. The mice were euthanized for analysis 2 days after the last challenge.
To establish the lipopolysaccharide (LPS)-induced acute lung inflammation/injury model (39), mice were anesthetized and instilled with 10 μg LPS O111 (Sigma) intranasally or PBS (control). On day 4 after intranasal LPS administration, mice were euthanized for analysis.
RNA from lungs was extracted using an RNA isolation kit (Tiangen) and reversely transcribed (Tiangen). Actin served as the internal control, and the ΔΔCt quantification method was employed to calculate the relative gene expression.
All animal procedures were approved by the Committee of Animal Experiments in Guangzhou Medical University (Project number: 2019-273). All protocols adhered to the Guide for the Care and Use of Laboratory Animals.
Data are presented as the mean ± standard error of the mean (SEM). If the data were normally distributed, a two-tailed unpaired Student’s t-test was used to assess the differences between the two groups. A Mann-Whitney U unpaired test was used for populations that were not normally distributed. Correlation analysis was conducted with GraphPad Prism (version 8.0.1) with the Pearson method. R software (version 4.2.0) and GraphPad Prism were used for all statistical analyses. A P-value less than 0.05 was considered statistically significant (*, P < 0.05; **, P < 0.01; ***, P < 0.001; ****, P < 0.0001, n.s., not significant.).
Figure 1 depicts the crucial procedures used in this study. To examine shared genetic interrelations between allergic asthma and COVID-19, we evaluated human RNA-seq and microarray datasets from GEO and identified the common DEGs that trigger COVID-19 and allergic asthma. First, we analyzed the transcriptomic datasets of patients with COVID-19 and identified 4,082 genes that showed differential expression compared to the corresponding levels in healthy controls. Similarly, we identified 1,137 DEGs in the asthma dataset (Table 1). The volcano plots presented in Figures 2A, 2B show the DEGs (upregulated genes, red dots; downregulated genes, blue dots) for COVID-19 and asthma. Next, using Jvenn, a dependable web service for Venn analysis, we discovered 157 common DEGs between the asthma and COVID-19 datasets (Figure 2C). All 157 DEGs are listed in Table S1.
Figure 2 Volcano plots of (A) COVID-19 and (B) allergic asthma datasets, with genes having a log fold-change of at least 1 and P-value < 0.05. (C) The Venn diagram depicts the shared DEGs among COVID-19 and asthma.
The GO enrichment method is often used to reflect connections between genes and GO terms, whereas the Kyoto Encyclopedia of Genes and Genomes (KEGG) enrichment method can uncover gene–pathway correlations (40, 41). We used the Enrichr tool (29) to run a functional-enrichment test on common DEGs to find significantly enriched functional GO terms and cell-signaling pathways. The GO database was used as an annotation source for the GO analysis, which is divided into three categories (biological processes, cellular components, and molecular functions). Table 2 summarizes the top 10 terms related to biological processes, molecular functions, and cellular components. The overall ontological analysis is depicted in Figures 3A–C as a linear bar graph for each category. The differentially expressed genes were significantly enriched in cellular response to type I interferon, integral component of plasma membrane, and carboxylic acid transmembrane transporter activity in the biological processes, cell compartments, and molecular functions subsets, respectively.
Figure 3 Ontological analysis and pathway enrichment analysis of shared differentially expressed genes (DEGs) between patients with COVID-19 and asthma. Ontological analysis: (A) biological processes, (B) cellular components, and (C) molecular function. Pathway enrichment analysis: (D) Wikipathway 2021, (E) KEGG 2021 human pathway, (F) Reactome pathway, and the (G) Bioplanet pathway.
KEGG pathway analysis is a modeling tool used to highlight how essential molecular or biological processes interact to reveal the reciprocal influences of different diseases (42). Four global databases, including KEGG, WikiPathways, Reactome, and Bioplanet, were used to assemble the most enriched pathways based on the common DEGs between asthma and COVID-19 datasets. KEGG pathway analysis revealed the following top 10 pathways: legionellosis, coronavirus disease, NOD-like receptor signaling pathway, measles, influenza A, hepatitis C, gastric cancer, Staphylococcus aureus infection, longevity regulating pathway, and NF-kappa B signaling pathway. The top pathways found in the examined datasets are listed in Table 3. Figures 3D–G shows more precise representations of the pathway-enrichment analysis with bar graphs.
Common DEGs between COVID-19 and asthma datasets were uploaded to the STRING database to investigate PPIs and uncover common DEG interactions and adhesion pathways. Figure 4A shows the PPI network of common DEGs between COVID-19 and asthma datasets. The most interconnected nodes are presented as hub genes in the PPI network. CytoHubba has 11 methods for investigating networks from various viewpoints, and Maximal Clique Centrality (MCC) is the best of the 11 methods (27, 43). Based on the PPI network analysis performed using the cytoHubba plug-in of Cytoscape, we identified the top 15 (9.55%) most influential DEGs, which included MX1, RSAD2, IFIT3, OAS1, OAS2, IFIT1, IFIH1, IFI6, IFIT2, IFI27, OAS3, SAMD9L, CMPK2, IFI35, and IFNL1 by applying the MCC method of cytoHubba. Here, the cutoff (parameter) of the topological metric for hub genes was 13 (degree). These hub genes can potentially be used as biomarkers that could lead to new therapeutic approaches for the diseases being studied. Moreover, we used the cytoHubba plug-in to establish a submodule network and adequately capture the intimate relationships and vicinity of these genes (Figure 4B).
Figure 4 Protein–protein interaction (PPI) network and hub genes of differentially expressed genes (DEGs) common to COVID-19 and asthma. (A) Rectangle nodes represent DEGs and edges represent the interactions between nodes. STRING was used to create the PPI network, which was then visualized in Cytoscape. (B) The cytoHubba plug-in in Cytoscape was used to identify hub genes from the PPI network. The red and yellow nodes show the top 15 hub genes and their interactions with other molecules (green).
To determine whether the identified hub genes could be used as biomarkers to predict asthma and COVID-induced lung inflammation/injury, we utilized murine models of HDM-induced asthma and LPS-induced lung inflammation/injury. Bronchoalveolar lavage fluid (BALF) from HDM-treated mice had more eosinophils, neutrophils, and lymphocytes than BALF from PBS-treated control mice (Figures 5A, B; Figure S1A), and the lungs from HDM-treated mice showed much more peri-bronchial and peri-vascular leukocyte infiltration (Figure 5C), and higher IL-4, IL-5, IL-13 and MUC5AC levels (Figure S1B). These results demonstrate that HDM induced allergic asthma in mice. Moreover, MX1, RSAD2, IFIT1, IFI27, OAS3, and SAMD9L were markedly elevated in HDM-treated mice (Figure 5D). Furthermore, MX1, RSAD2, IFIT1, IFI27, OAS3, and SAMD9L levels correlated positively with IL-5, IL-13, and MUC5AC levels (Figures 5E–G; Figure S1C), which are the key mediators of allergic asthma (5).
Figure 5 Validation of the identified hub genes with a murine model of asthma. (A) Schematic representation of the experimental protocol used for the murine model of house dust mite (HDM)-induced asthma. (B) Differential cell counts in bronchoalveolar lavage fluid (BALF) samples from PBS- or HDM-treated mice. The data shown were combined from two experiments. n = 6–8. (C) Representative hematoxylin and eosin-stained lung sections. n = 5–6. Scale bars, 200 μm. (D) Cytokine mRNA abundances in homogenized lung tissues. The data shown were combined from two experiments. n = 6–8. (E-G) Corresponding scatterplot showing the relationships between mRNA-expression levels of the identified hub genes and those of MUC5AC, IL-5, and IL-13 in the lungs, as determined based on Spearman’s rank correlation (R). Student’s t-test was used to evaluate the differences. *P < 0.05, **P < 0.01, ***P < 0.001. The results shown are presented as the mean ± SEM.
Next, we explored the lung levels of the hub genes using a murine model of LPS-induced lung inflammation/injury. We utilized an LPS-induced lung inflammation/injury model to partially mimic COVID-19-induced lung injury because we lacked a biosafety level 3 facility to develop a murine model of SARS-CoV-2 infection. IFN-γ, TNF-α, MX1, RSAD2, IFIT1, OAS2, and IFI27 were markedly elevated in LPS-treated mice when compared to levels in the PBS control (Figures 6A, B). Moreover, RSAD2 was positively correlated with IFN-γ and TNF-α (Figures 6C, D), which are key mediators of cytokine shock during SARS-CoV-2 infection (44).
Figure 6 Validation of the identified hub genes with a murine lung inflammation/injury model. (A, B) Cytokine mRNA abundance in homogenized lung tissue. Data were combined from two experiments. n = 5–7. (C, D) Corresponding scatterplot showing the identified hub gene mRNA levels versus IFN-γ and TNF-α mRNA levels in the lung determined based on Spearman’s rank correlation (R). Student’s t-test was used to evaluate the differences. *, P < 0.05, **, P < 0.01. Results are shown as the mean ± SEM.
To determine the major changes at the transcriptional level and better understand the regulatory hub proteins, we employed network analysis to discover regulatory transcription factors (TFs) and miRNAs. Figure 7 depicts the interactions between TF regulators and the identified hub genes. The interactions of the miRNA regulators with the identified hub genes are shown in Figure 8. We found that 65 TFs and 119 post-transcriptional (miRNA) regulatory signals were predicted to govern multiple identified hub genes, implying a significant interplay between them. Tables S2, S3 show the construction and analysis of the regulatory target TF–gene and target miRNA–gene networks, as well as the topology table, respectively.
Figure 7 Interconnected regulatory interaction network of differentially expressed genes (DEGs)–transcription factors (TFs) created using the Network Analyst. Herein, blue nodes represent TFs, and pink nodes represent the interaction between gene symbols and TFs.
Figure 8 Interconnected regulatory interaction network of differentially expressed genes (DEGs)–miRNAs. Herein, the pink circle node indicates the gene symbols that interact with miRNAs.
Analyses of protein–drug interactions help to understand the structural features recommended for receptor sensitivity, which may also be useful in drug discovery (45, 46). The hub genes identified based on COVID-19–asthma interactions were used in this analysis. Ten potential therapeutic drugs were identified with Enrichr based on transcriptional characteristics from the DSigDB database, and the top 10 candidate compounds were retrieved based on their P-values. Table 4 shows the top 10 enriched medications in the DSigDB database (3′-azido-3′-deoxythymidine, acetohexamide, chlorophyllin, suloctidil, estradiol, prenylamine, progesterone, benzene, clioquinol, and LY-294002).
Different diseases can be linked or correlated in certain situations, such as when they share one or more similar genes (47). Deciphering links between genes and diseases is a crucial first step in developing treatments. Our investigation of gene–disease relationships with NetworkAnalyst showed that influenza, myocardial ischemia, spontaneous abortion, Fanconi anemia, leukemia, stomach neoplasms, diabetes mellitus, viral diseases, depressive disorders, asthma, and COVID-19 were most coordinated with the hub genes identified in this study. Figure 9 depicts the gene–disease relationships.
Figure 9 Gene-disease association network. Herein, the diseases represented by the pink square nodes and the green circle nodes indicate the gene symbols that interact with the disease.
Acute asthma exacerbations are frequently caused by respiratory infections (21, 48, 49). Since the world is currently facing the COVID-19 pandemic, concerns have arisen regarding a possible increased risk of COVID-19 severity and exacerbations in people with asthma (50, 51). High-throughput sequencing datasets have become valuable resources for identifying biomarkers for many disorders (52, 53). In this context, combining bioinformatics and systems biology analytics with patient sequencing data can improve our understanding of the effects of COVID-19 on asthmatic patients and reveal new therapeutic tactics and management alternatives (54–56). In this study, network analyses were used to explore gene-expression patterns from patient datasets related to asthma and COVID-19, which yielded potential COVID-19 and asthma biomarkers and candidate drugs.
Our analysis of asthma and COVID-19 transcriptomics revealed 157 common DEGs. The biological relevance of the common DEGs was assessed through GO pathway analysis to gain insights into the pathophysiology of asthma and COVID-19. Using the Enrichr platform (29), three GO analyses were conducted with the GO database as the annotation source for biological processes (molecular activities), cellular components (genes that regulate functions), and molecular function (activities at the molecular level). For the biological processes, cellular responses to type-I interferon (IFN-I; 11 genes) and the IFN-I signaling pathway (11 genes) were among the top GO terms. IFN-I induces the production of several genes that contribute to autocrine and paracrine antiviral activities in host cells (57–59). Increasing evidence suggests that patients with severe COVID-19 have a strong IFN-I response, which contrasts with the delayed, possibly suppressed, interferon response seen during early infection (57, 60, 61). Through various pathways, a potent IFN-I response could increase hyperinflammation, leading to severe COVID-19 (57, 62, 63). Furthermore, IFN-Is inhibit type-2 asthma immunopathology and fibrotic illness by regulating ILC2 cells (64). Notably, interferon treatment benefits patients with severe asthma (64, 65).
The top GO terms based on the cellular component were “integral component of the plasma membrane” (30 genes) and the “serine C-palmitoyltransferase (SPT) complex” (two genes). During SARS-CoV-2 replication, the ribosome produces the viral membrane protein, spike glycoprotein, and envelope protein, which incorporate into the endoplasmic reticulum membrane (27, 66). The earliest and rate-limiting step in the de novo production of all sphingolipids is catalyzed by the human SPT complex (67, 68). SPT function is regulated by ORMDLs, with the human ORMDL3 being linked to asthma (67). The top two GO pathways in the molecular function category were “carboxylic acid transmembrane transporter activity” (six genes) and “SPT activity” (two genes). Carboxylic acid transmembrane transporter activity affects several pulmonary disorders, including asthma, acute respiratory distress syndrome, lung cancer, and pulmonary fibrosis (69–71).
KEGG pathway analysis of 157 common DEGs revealed similar pathways for asthma and COVID-19. The top KEGG pathway terms were legionellosis, coronavirus disease, NOD-like receptor signaling pathway, measles, influenza A, hepatitis C, gastric cancer, Staphylococcus aureus infection, longevity regulating pathway, and NF-kappa B signaling pathway. Previous evidence suggests that legionellosis, measles, and influenza A have strong relationships with acute respiratory tract infections, which are implicated in community-acquired pneumonia, acute exacerbations of chronic bronchitis, asthma, and COVID-19 (72–75).
We also established a PPI network based on DEGs to study common functional features of proteins and predict therapeutic targets. Various pathogeneses were linked to hub genes that could be important therapeutic targets or biomarkers for COVID-19 and asthma. We used the MCC method to identify the top 15 hub genes (MX1, RSAD2, IFIT3, OAS1, OAS2, IFIT1, IFIH1, IFI6, IFIT2, IFI27, OAS3, SAMD9L, CMPK2, IFI35, and IFNL1) associated with both asthma and COVID-19. We utilized a mouse model of allergic asthma with HDM (which can induce T-helper-cell-mediated airway inflammation reminiscent of human asthma) to ascertain whether the identified hub genes could be useful biomarkers for predicting asthma. MX1, RSAD2, IFIT1, IFI27, OAS3, and SAMD9L were markedly elevated in HDM-treated mice and positively associated with IL-5, IL-13 and MUC5AC (key mediators of allergic asthma). Moreover, MX1, RSAD2, IFIT1, OAS2, and IFI27 were markedly elevated in a murine model of LPS-induced lung inflammation/injury and RSAD2 was positively associated with INF-γ and TNF-α, the key mediators of cytokine shock during SARS-CoV-2 infection.
MX genes are found in nearly all vertebrate genomes and are primarily active against RNA viruses (76, 77). MX1 is a member of the effector protein family of the IFN system. MX1 induces a protective antiviral response by modulating the expression of critical molecules linked to influenza A virus lethality; these effects are amplified under IFN-ɑ stimulation (78, 79). Moreover, MX1 expression is higher in patients with COVID-19 and may have antiviral properties against SARS-COV-2 (76, 80). MX1 also has antiviral properties against other viruses, including influenza A, measles, and type-3 parainfluenza viruses (76, 79–81). In addition, flow-cytometric detection of MX1 protein expression in whole blood appears to be an easy and valuable method for studying viral infections during acute asthma exacerbations (82).
Gaskill et al. demonstrated that RASD2 plays a role in idiopathic pulmonary fibrosis (IPF), asthma, and chronic obstructive pulmonary disease (COPD) (83). Furthermore, RASD2 has been implicated as a target for SARS-CoV-2 infection (27). IFN-induced proteins with tetratricopeptide repeats (IFITs), such as IFIT1, IFIT2, and IFIT3, mediate IFN-related pathways and may suppress viral replication (84, 85), suggesting that they could be targeted to inhibit SARS-CoV-2 replication. OAS1, OAS2, and OAS3 homologous are members of the 2′-5′-oligoadenylate synthetase (OAS) family, which inhibits cellular protein synthesis and confers resistance to viral infection (86). Polymorphisms in the interferon-stimulated gene, OAS1, have been linked to host immune responses against several classes of viral infections (86, 87), and extracellular OAS1 may play a role in antiviral immune responses (88). A two-sample Mendelian randomization study of 931 proteins showed that increased OAS1 levels in the non-infectious state strongly correlated with reduced risks for SARS-CoV-2 susceptibility and COVID-19 severity (86).
We also discovered links between COVID-19 and asthma in terms of TFs and miRNAs. TFs drive mRNA expression, whereas miRNAs regulate gene expression via RNA silencing at the post-transcription level (89, 90). Thus, TFs and miRNAs play essential roles in disease development. Our analysis revealed relationships among the common DEGs, TFs, and miRNAs. We identified TFs, such as GATA3, STAT3, STAT1, CEBPB, FOS, FOXD1, MAX, GATA2, RELA, and JUN, which are associated with diffident types of respiratory diseases, such as asthma, COPD, IPF, and COVID-19 (88, 91–100). In the context of determining the relationship between DEGs and miRNAs, hsa-miR-335-5p, hsa-miR-146a-5p, hsa-miR-5192, hsa-miR-375, and hsa-miR-124-3p found to be associated with the pathogenesis and exacerbation of asthma (101–104). In patients with asthma treated with inhaled corticosteroids, hsa-miR-335-5p was strongly related to changes in the FEV1 ratio (102). MiR-146a is an immune-regulatory miRNA that executes key functions in allergies and asthma (105, 106). An miR-146a polymorphism has been linked to asthma in several studies (101, 105, 106). Previous data implicated miR-146a in immunoglobulin E (IgE) synthesis and promoting the IgE-class transition in B cells (101, 107). Furthermore, children with asthma had considerably greater plasma miR-146a levels than healthy controls (101, 108). In asthmatic patients, hsa-miR-124-3p was adversely linked to the risk of exacerbation, severity, and inflammation, but was positively associated with lung function (104). Remarkably, we also identified five miRNAs (hsa-miR-1-3p, hsa-miR-17-5p, hsa-miR-20a-5p, hsa-miR-5192, and hsa-miR-26b-5p) that were predicted to be associated with DEGs in patients with COVID-19 (109–111). Most of these miRNAs have been implicated in tumorigenesis of several types of cancer, particularly lung cancer (112–115).
Our gene–disease analysis predicted relationships between common DEGs and various disorders, including COVID-19. For example, several genes related to depressive disorders (major depressive disorder, drug-induced depressive state, unipolar depression, and mental depression) were found in the study. Mental disorders are major causes of global health burdens, with depressive and anxiety disorders being the leading contributors (116–118). Mental depression and major depressive disorder appear to synergistically influence asthma control and the quality of life (119–121). The COVID-19 pandemic has exacerbated many determinants of poor mental health (116, 122) by negatively influencing the mental health of those with anxiety, depression, or obsessive–compulsive disorders, necessitating strict therapeutic monitoring (116, 122, 123). Furthermore, our network analysis revealed associations with the identified DEGs and other viral diseases, such as influenza. Acute asthma exacerbations are frequently caused by respiratory viruses such as influenza (124, 125). The danger of co-infection with influenza A virus and SARS-CoV-2 is a serious concern for public health officials and clinicians (126, 127). Furthermore, our results suggest that individuals with COVID-19 and asthma can also be affected by myocardial ischemia, stomach neoplasms, acute myelocytic leukemia, Fanconi anemia, spontaneous abortion, and insulin-dependent diabetes.
Several substances and drugs have been tested as potential COVID-19 therapeutic agents. Remdesivir, a new nucleoside analog with broad-spectrum antiviral activity against RNA viruses, was the first licensed treatment for severe COVID-19 (128). Furthermore, favipiravir, a new antiviral medication that inhibits viral transcription and replication by competitively inhibiting RNA-dependent RNA polymerase, has been used to treat SARS-CoV-2 infection (129). We discovered that 3′-azido-3′-deoxythymidine (also known as azidothymidine, zidovudine, or Retrovir), acetohexamide, chlorophyllin, suloctidil, estradiol, prenylamine, progesterone, benzene, clioquinol, and LY-294002 are candidate drugs for treating COVID-19 and asthma. Interestingly, 3′-azido-3′-deoxythymidine is a nucleoside reverse-transcriptase inhibitor (NRTI) that was the first drug approved for treating acquired immunodeficiency syndrome, which is caused by human immunodeficiency virus (HIV) (130, 131). The approval of zidovudine and other HIV NRTIs by the Food and Drug Administration accelerated the development of antivirals for various viruses, including SARS-CoV-2 (132). A case study on COVID-19 revealed zidovudine as a drug candidate for SARS-CoV-2 (133). Acetohexamide is a first-generation sulfonylurea derivative used to treat type-2 diabetes, especially in people whose blood glucose cannot be controlled by diet (134, 135). Acetohexamide also shows promise for disrupting SARS-CoV-2 binding and replication by targeting cell surface-binding immunoglobins (136). Chlorophyllin is a central component generated from chlorophyll that has been widely employed as a green pigment in the food industry (137, 138). Previous findings demonstrated that chlorophyllin significantly suppresses IL-6 (138, 139). Chlorophyllin could be used to alleviate harsh immune-modulated outcomes in patients infected with SARS-CoV-2 by blocking IL-6 trans-signaling, thereby decreasing proinflammatory cytokine effects, lymphocyte recruitment, and neutrophil overproduction (137).
Progesterone and estradiol were also identified as candidate drugs. These endogenous reproductive hormones are abundantly produced in the periphery by the adrenal glands and ovaries and de novo by the brain (140). They play significant physiological roles by altering inflammatory processes and behaviors (141, 142). Recently, progesterone was demonstrated to mitigate the severity of COVID-19 pneumonia in a Syrian hamster model (143). Moreover, patients infected with SARS-CoV-2 have higher progesterone levels, which are linked to decreased COVID-19 severity (140).
Another candidate drug identified here was suloctidil, which is a new medication being tested in several clinical trials for its potential use against dementia and thrombotic diseases (144, 145). Moreover, prenylamine, iodochlorhydroxyquin, clioquinol, and LY294002 were also found as drug candidates in this study. The World Health Organization has classified prenylamine as a class V calcium channel antagonist that blocks diphenylalkylamine calcium channels (146, 147). Iodochlorhydroxyquin, also known as clioquinol, is a type of mycophenolic acid with antimicrobial and antifungal properties. Skin infections can be treated with topical medicines containing clioquinol (148). Furthermore, clioquinol was once widely used as an anti-infective, particularly for diarrhea (149). LY294002 is a highly selective inhibitor of phosphatidylinositol 3 kinase (150, 151). Beyond respiratory diseases, several other conditions are associated with SARS-CoV-2 infection, including thrombosis, blood-related problems, diarrhea, and different types of cancer. Therefore, those predicted drugs are also candidates for the treatment of other COVID-19-related diseases.
Despite presenting some interesting findings, this study has some limitations. These results, including hub genes, regulatory networks, and candidate drug identification, were obtained from bioinformatics calculations and analyses and the hub genes were validated using a murine HDM-induced asthma model and LPS-induced lung inflammation/injury model. However, the murine LPS-induced lung inflammation/injury model can only partially mimic COVID-19-induced lung injury, as acute lung inflammation/injury in this model is caused by opportunistic gram-negative bacteria (39, 152). Thus, further research studies and clinical trials are needed to validate the biological functions of the hub genes, as well as the efficacy and safety of the discovered candidate drugs.
In conclusion, we utilized transcriptomic analysis to identify common pathways and molecular biomarkers in asthma and COVID-19 to help understand the link between the SARS-CoV-2 infection and asthma. We identified 157 common DEGs between COVID-19 and asthma and conducted gene-expression analysis to discover the relevant GO terms and cell-signaling pathways. In addition, we constructed a PPI network based on the common DEGs and identified the top 15 hub genes from the PPI network and validated their association with asthma and COVID-19 infection utilizing murine models that mimic asthma and lung inflammation/injury. Finally, several drugs and drug–target interactions were identified to be associated with the 15 hub genes. Many vaccines are being employed to combat the COVID-19 pandemic, but SARS-CoV-2 continues to acquires novel mutations. COVID-19 can only be defeated by developing new and effective COVID-19 vaccines and therapies. We hope that the findings of this study will provide key insights that may help in developing novel drugs or repurposing existing therapeutic agents to combat COVID-19, particularly in patients who suffer from asthma.
The original contributions presented in the study are included in the article/Supplementary Material. Further inquiries can be directed to the corresponding authors.
This study was reviewed and approved by the Committee of Animal Experiments in Guangzhou Medical University (Project number: 2019-273).
GQ and HF conceptualized the study. HWF, ZS and ZC collected the transcriptome and clinical data. HWF, ZS, ZC, AC, DS and YK conducted the experiments and participated in the data analysis. GQ and HWF drafted the manuscript. HF revised the final manuscript. All authors contributed to the article and approved the submitted version.
This work was supported by grants from the National Natural Science Foundation of China (81901633 to GQ; 81971863 to HF), the Natural Science Foundation of Shanghai, China (22ZR1444700 to HF), and the Guangzhou Key Medical Discipline Construction Project Fund.
We thank all the patients who participated in this study and donated samples, as well as the GEO database for providing their platform. We thank Editage for English language editing.
The authors declare that the research was conducted in the absence of any commercial or financial relationships that could be construed as a potential conflict of interest.
All claims expressed in this article are solely those of the authors and do not necessarily represent those of their affiliated organizations, or those of the publisher, the editors and the reviewers. Any product that may be evaluated in this article, or claim that may be made by its manufacturer, is not guaranteed or endorsed by the publisher.
The Supplementary Material for this article can be found online at: https://www.frontiersin.org/articles/10.3389/fimmu.2022.988479/full#supplementary-material
BALF, bronchoalveolar lavage fluid; COVID-19, coronavirus disease 2019; DEG, differentially expressed gene; GO, Gene Ontology; GRN, gene-regulatory network; HDM, house dust mite; HIV, human immunodeficiency virus; IFN-I, type-I interferon; IgE, immunoglobulin E; IPF, idiopathic pulmonary fibrosis; KEGG, Kyoto Encyclopedia of Genes and Genomes; LPS, lipopolysaccharide; miRNA, microRNA; PPI, protein–protein interaction; SARS-CoV-2, severe acute respiratory syndrome coronavirus 2; SEM, standard error of the mean; TF, transcription factor.
1. West R, Kobokovich A, Connell N, Gronvall GK. COVID-19 antibody tests: A valuable public health tool with limited relevance to individuals. Trends Microbiol (2021) 29(3):214–23. doi: 10.1016/j.tim.2020.11.002
2. Thompson MG, Burgess JL, Naleway AL, Tyner H, Yoon SK, Meece J, et al. Prevention and attenuation of covid-19 with the BNT162b2 and mRNA-1273 vaccines. N Engl J Med (2021) 385(4):320–9. doi: 10.1056/NEJMoa2107058
3. Yuce M, Filiztekin E, Ozkaya KG. COVID-19 diagnosis -a review of current methods. Biosens Bioelectron. (2021) 172:112752. doi: 10.1016/j.bios.2020.112752
4. Kevadiya BD, Machhi J, Herskovitz J, Oleynikov MD, Blomberg WR, Bajwa N, et al. Diagnostics for SARS-CoV-2 infections. Nat Mater (2021) 20(5):593–605. doi: 10.1038/s41563-020-00906-z
5. Hammad H, Lambrecht BN. The basic immunology of asthma. Cell (2021) 184(6):1469–85. doi: 10.1016/j.cell.2021.02.016
6. Barcik W, Boutin RCT, Sokolowska M, Finlay BB. The role of lung and gut microbiota in the pathology of asthma. Immunity (2020) 52(2):241–55. doi: 10.1016/j.immuni.2020.01.007
7. Lambrecht BN, Hammad H, Fahy JV. The cytokines of asthma. Immunity (2019) 50(4):975–91. doi: 10.1016/j.immuni.2019.03.018
8. Wang JY, Pawankar R, Tsai HJ, Wu LS, Kuo WS. COVID-19 and asthma, the good or the bad? Allergy (2021) 76(2):565–7. doi: 10.1111/all.14480
9. Song J, Zeng M, Wang H, Qin C, Hou HY, Sun ZY, et al. Distinct effects of asthma and COPD comorbidity on disease expression and outcome in patients with COVID-19. Allergy (2021) 76(2):483–96. doi: 10.1111/all.14517
10. Izquierdo JL, Almonacid C, Gonzalez Y, Del Rio-Bermudez C, Ancochea J, Cardenas R, et al. The impact of COVID-19 on patients with asthma. Eur Respir J (2021) 57(3):2003142. doi: 10.1183/13993003.03142-2020
11. Green I, Merzon E, Vinker S, Golan-Cohen A, Magen E. COVID-19 susceptibility in bronchial asthma. J Allergy Clin Immunol Pract (2021) 9(2):684–92.e1. doi: 10.1016/j.jaip.2020.11.020
12. Adir Y, Saliba W, Beurnier A, Humbert M. Asthma and COVID-19: an update. Eur Respir Rev (2021) 30(162):210152. doi: 10.1183/16000617.0152-2021
13. Wang Y, Chen J, Chen W, Liu L, Dong M, Ji J, et al. Does asthma increase the mortality of patients with COVID-19? A systematic review and meta-analysis. Int Arch Allergy Immunol (2021) 182(2):76–82. doi: 10.1159/000510953
14. Du H, Dong X, Zhang JJ, Cao YY, Akdis M, Huang PQ, et al. Clinical characteristics of 182 pediatric COVID-19 patients with different severities and allergic status. Allergy (2021) 76(2):510–32. doi: 10.1111/all.14452
15. Choi YJ, Park JY, Lee HS, Suh J, Song JY, Byun MK, et al. Effect of asthma and asthma medication on the prognosis of patients with COVID-19. Eur Respir J (2021) 57(3):2002226. doi: 10.1183/13993003.02226-2020
16. Gao YD, Agache I, Akdis M, Nadeau K, Klimek L, Jutel M, et al. The effect of allergy and asthma as a comorbidity on the susceptibility and outcomes of COVID-19. Int Immunol (2022) 34(4):177–88. doi: 10.1093/intimm/dxab107
17. Riggioni C, Comberiati P, Giovannini M, Agache I, Akdis M, Alves-Correia M, et al. A compendium answering 150 questions on COVID-19 and SARS-CoV-2. Allergy (2020) 75(10):2503–41. doi: 10.1111/all.14449
18. Xie YZ, Peng CW, Su ZQ, Huang HT, Liu XH, Zhan SF, et al. A practical strategy for exploring the pharmacological mechanism of luteolin against COVID-19/Asthma comorbidity: Findings of system pharmacology and bioinformatics analysis. Front Immunol (2021) 12:769011. doi: 10.3389/fimmu.2021.769011
19. Jackson DJ, Busse WW, Bacharier LB, Kattan M, O'Connor GT, Wood RA, et al. Association of respiratory allergy, asthma, and expression of the SARS-CoV-2 receptor ACE2. J Allergy Clin Immunol (2020) 146(1):203–6 e3. doi: 10.1016/j.jaci.2020.04.009
20. Eggert LE, He Z, Collins W, Lee AS, Dhondalay G, Jiang SY, et al. Asthma phenotypes, associated comorbidities, and long-term symptoms in COVID-19. Allergy (2022) 77(1):173–85. doi: 10.1111/all.14972
21. Ferastraoaru D, Hudes G, Jerschow E, Jariwala S, Karagic M, de Vos G, et al. Eosinophilia in asthma patients is protective against severe COVID-19 illness. J Allergy Clin Immunol Pract (2021) 9(3):1152–62.e3. doi: 10.1016/j.jaip.2020.12.045
22. Radzikowska U, Ding M, Tan G, Zhakparov D, Peng Y, Wawrzyniak P, et al. Distribution of ACE2, CD147, CD26, and other SARS-CoV-2 associated molecules in tissues and immune cells in health and in asthma, COPD, obesity, hypertension, and COVID-19 risk factors. Allergy (2020) 75(11):2829–45. doi: 10.1111/all.14429
23. Bloom CI, Cullinan P, Wedzicha JA. Asthma phenotypes and COVID-19 risk: A population-based observational study. Am J Respir Crit Care Med (2022) 205(1):36–45. doi: 10.1164/rccm.202107-1704OC
24. Barrett T, Wilhite SE, Ledoux P, Evangelista C, Kim IF, Tomashevsky M, et al. NCBI GEO: archive for functional genomics data sets–update. Nucleic Acids Res (2013) 41(Database issue):D991–5. doi: 10.1093/nar/gks1193
25. Levy Y, Wiedemann A, Hejblum BP, Durand M, Lefebvre C, Surenaud M, et al. CD177, a specific marker of neutrophil activation, is associated with coronavirus disease 2019 severity and death. iScience (2021) 24(7):102711. doi: 10.1016/j.isci.2021.102711
26. Xia L, Wang X, Liu L, Fu J, Xiao W, Liang Q, et al. Lnc-BAZ2B promotes M2 macrophage activation and inflammation in children with asthma through stabilizing BAZ2B pre-mRNA. J Allergy Clin Immunol (2021) 147(3):921–32.e9. doi: 10.1016/j.jaci.2020.06.034
27. Mahmud SMH, Al-Mustanjid M, Akter F, Rahman MS, Ahmed K, Rahman MH, et al. Bioinformatics and system biology approach to identify the influences of SARS-CoV-2 infections to idiopathic pulmonary fibrosis and chronic obstructive pulmonary disease patients. Brief Bioinform (2021) 22(5):bbab115. doi: 10.1093/bib/bbab115
28. Bardou P, Mariette J, Escudie F, Djemiel C, Klopp C. Jvenn: an interactive Venn diagram viewer. BMC Bioinf (2014) 15:293. doi: 10.1186/1471-2105-15-293
29. Kuleshov MV, Jones MR, Rouillard AD, Fernandez NF, Duan Q, Wang Z, et al. Enrichr: a comprehensive gene set enrichment analysis web server 2016 update. Nucleic Acids Res (2016) 44(W1):W90–7. doi: 10.1093/nar/gkw377
30. Szklarczyk D, Gable AL, Lyon D, Junge A, Wyder S, Huerta-Cepas J, et al. STRING v11: protein-protein association networks with increased coverage, supporting functional discovery in genome-wide experimental datasets. Nucleic Acids Res (2019) 47(D1):D607–D13. doi: 10.1093/nar/gky1131
31. Liu Y, Gu HY, Zhu J, Niu YM, Zhang C, Guo GL. Identification of hub genes and key pathways associated with bipolar disorder based on weighted gene Co-expression network analysis. Front Physiol (2019) 10:1081. doi: 10.3389/fphys.2019.01081
32. Xia J, Gill EE, Hancock RE. NetworkAnalyst for statistical, visual and network-based meta-analysis of gene expression data. Nat Protoc (2015) 10(6):823–44. doi: 10.1038/nprot.2015.052
33. Sethupathy P, Corda B, Hatzigeorgiou AG. TarBase: A comprehensive database of experimentally supported animal microRNA targets. RNA (2006) 12(2):192–7. doi: 10.1261/rna.2239606
34. Hsu SD, Lin FM, Wu WY, Liang C, Huang WC, Chan WL, et al. miRTarBase: a database curates experimentally validated microRNA-target interactions. Nucleic Acids Res (2011) 39(Database issue):D163–9. doi: 10.1093/nar/gkq1107
35. Khan A, Fornes O, Stigliani A, Gheorghe M, Castro-Mondragon JA, van der Lee R, et al. JASPAR 2018: update of the open-access database of transcription factor binding profiles and its web framework. Nucleic Acids Res (2018) 46(D1):D260–D6. doi: 10.1093/nar/gkx1126
36. Pinero J, Ramirez-Anguita JM, Sauch-Pitarch J, Ronzano F, Centeno E, Sanz F, et al. The DisGeNET knowledge platform for disease genomics: 2019 update. Nucleic Acids Res (2020) 48(D1):D845–D55. doi: 10.1093/nar/gkz1021
37. Zhou G, Soufan O, Ewald J, Hancock REW, Basu N, Xia J. NetworkAnalyst 3.0: a visual analytics platform for comprehensive gene expression profiling and meta-analysis. Nucleic Acids Res (2019) 47(W1):W234–W41. doi: 10.1093/nar/gkz240.
38. Qian G, Jiang W, Zou B, Feng J, Cheng X, Gu J, et al. LPS inactivation by a host lipase allows lung epithelial cell sensitization for allergic asthma. J Exp Med (2018) 215(9):2397–412. doi: 10.1084/jem.20172225
39. Zou B, Jiang W, Han H, Li J, Mao W, Tang Z, et al. Acyloxyacyl hydrolase promotes the resolution of lipopolysaccharide-induced acute lung injury. PloS Pathog (2017) 13(6):e1006436. doi: 10.1371/journal.ppat.1006436
40. Nashiry MA, Sumi SS, Sharif Shohan MU, Alyami SA, Azad AKM, Moni MA. Bioinformatics and system biology approaches to identify the diseasome and comorbidities complexities of SARS-CoV-2 infection with the digestive tract disorders. Brief Bioinform (2021) 22(6):bbab126. doi: 10.1093/bib/bbab126
41. Thomas PD, Hill DP, Mi H, Osumi-Sutherland D, Van Auken K, Carbon S, et al. Gene ontology causal activity modeling (GO-CAM) moves beyond GO annotations to structured descriptions of biological functions and systems. Nat Genet (2019) 51(10):1429–33. doi: 10.1038/s41588-019-0500-1
42. Kanehisa M, Furumichi M, Sato Y, Ishiguro-Watanabe M, Tanabe M. KEGG: integrating viruses and cellular organisms. Nucleic Acids Res (2021) 49(D1):D545–D51. doi: 10.1093/nar/gkaa970
43. Chin CH, Chen SH, Wu HH, Ho CW, Ko MT, Lin CY. cytoHubba: identifying hub objects and sub-networks from complex interactome. BMC Syst Biol (2014) 8 Suppl 4:S11. doi: 10.1186/1752-0509-8-S4-S11
44. Karki R, Sharma BR, Tuladhar S, Williams EP, Zalduondo L, Samir P, et al. Synergism of TNF-alpha and IFN-gamma triggers inflammatory cell death, tissue damage, and mortality in SARS-CoV-2 infection and cytokine shock syndromes. Cell (2021) 184(1):149–68 e17. doi: 10.1016/j.cell.2020.11.025
45. Nashiry A, Sarmin Sumi S, Islam S, Quinn JMW, Moni MA. Bioinformatics and system biology approach to identify the influences of COVID-19 on cardiovascular and hypertensive comorbidities. Brief Bioinform (2021) 22(2):1387–401. doi: 10.1093/bib/bbaa426
46. Mahmud SMH, Chen W, Meng H, Jahan H, Liu Y, Hasan SMM. Prediction of drug-target interaction based on protein features using undersampling and feature selection techniques with boosting. Anal Biochem (2020) 589:113507. doi: 10.1016/j.ab.2019.113507
47. Rahaman M, Komanapalli J, Mukherjee M, Byram PK, Sahoo S, Chakravorty N. Decrypting the role of predicted SARS-CoV-2 miRNAs in COVID-19 pathogenesis: A bioinformatics approach. Comput Biol Med (2021) 136:104669. doi: 10.1016/j.compbiomed.2021.104669
48. Garcia-Pachon E, Ruiz-Alcaraz S, Baeza-Martinez C, Zamora-Molina L, Soler-Sempere MJ, Padilla-Navas I, et al. Symptoms in patients with asthma infected by SARS-CoV-2. Respir Med (2021) 185:106495. doi: 10.1016/j.rmed.2021.106495
49. Carli G, Cecchi L, Stebbing J, Parronchi P, Farsi A. Is asthma protective against COVID-19? Allergy (2021) 76(3):866–8. doi: 10.1111/all.14426
50. Eger K, Bel EH. Asthma and COVID-19: do we finally have answers? Eur Respir J (2021) 57(3):2004451. doi: 10.1183/13993003.04451-2020
51. Hyams C, Marlow R, Maseko Z, King J, Ward L, Fox K, et al. Effectiveness of BNT162b2 and ChAdOx1 nCoV-19 COVID-19 vaccination at preventing hospitalisations in people aged at least 80 years: a test-negative, case-control study. Lancet Infect Dis (2021) 21(11):1539–48. doi: 10.1016/S1473-3099(21)00330-3
52. Piazza I, Beaton N, Bruderer R, Knobloch T, Barbisan C, Chandat L, et al. A machine learning-based chemoproteomic approach to identify drug targets and binding sites in complex proteomes. Nat Commun (2020) 11(1):4200. doi: 10.1038/s41467-020-18071-x
53. Al-Mustanjid M, Mahmud SMH, Royel MRI, Rahman MH, Islam T, Rahman MR, et al. Detection of molecular signatures and pathways shared in inflammatory bowel disease and colorectal cancer: A bioinformatics and systems biology approach. Genomics (2020) 112(5):3416–26. doi: 10.1016/j.ygeno.2020.06.001
54. Abrams EM, Sinha I, Fernandes RM, Hawcutt DB. Pediatric asthma and COVID-19: The known, the unknown, and the controversial. Pediatr Pulmonol. (2020) 55(12):3573–8. doi: 10.1002/ppul.25117
55. Cevhertas L, Ogulur I, Maurer DJ, Burla D, Ding M, Jansen K, et al. Advances and recent developments in asthma in 2020. Allergy (2020) 75(12):3124–46. doi: 10.1111/all.14607
56. Johnston SL. Asthma and COVID-19: Is asthma a risk factor for severe outcomes? Allergy (2020) 75(7):1543–5. doi: 10.1111/all.14348
57. Lee JS, Shin EC. The type I interferon response in COVID-19: implications for treatment. Nat Rev Immunol (2020) 20(10):585–6. doi: 10.1038/s41577-020-00429-3
58. De Souza DP, Achuthan A, Lee MK, Binger KJ, Lee MC, Davidson S, et al. Autocrine IFN-I inhibits isocitrate dehydrogenase in the TCA cycle of LPS-stimulated macrophages. J Clin Invest. (2019) 129(10):4239–44. doi: 10.1172/JCI127597
59. Hadjadj J, Yatim N, Barnabei L, Corneau A, Boussier J, Smith N, et al. Impaired type I interferon activity and inflammatory responses in severe COVID-19 patients. Science (2020) 369(6504):718–24. doi: 10.1126/science.abc6027
60. Lee JS, Park S, Jeong HW, Ahn JY, Choi SJ, Lee H, et al. Immunophenotyping COVID-19 influenza highlights role type I interferons Dev Severe COVID-19. Sci Immunol (2020) 5(49):eabd1554. doi: 10.1126/sciimmunol.abd1554
61. Channappanavar R, Fehr AR, Vijay R, Mack M, Zhao J, Meyerholz DK, et al. Dysregulated type I interferon and inflammatory monocyte-macrophage responses cause lethal pneumonia in SARS-CoV-Infected mice. Cell Host Microbe (2016) 19(2):181–93. doi: 10.1016/j.chom.2016.01.007
62. Neil JA, Matsuzawa-Ishimoto Y, Kernbauer-Holzl E, Schuster SL, Sota S, Venzon M, et al. IFN-I and IL-22 mediate protective effects of intestinal viral infection. Nat Microbiol (2019) 4(10):1737–49. doi: 10.1038/s41564-019-0470-1
63. Venet F, Cour M, Rimmele T, Viel S, Yonis H, Coudereau R, et al. Longitudinal assessment of IFN-I activity and immune profile in critically ill COVID-19 patients with acute respiratory distress syndrome. Crit Care (2021) 25(1):140. doi: 10.1186/s13054-021-03558-w
64. Duerr CU, McCarthy CD, Mindt BC, Rubio M, Meli AP, Pothlichet J, et al. Type I interferon restricts type 2 immunopathology through the regulation of group 2 innate lymphoid cells. Nat Immunol (2016) 17(1):65–75. doi: 10.1038/ni.3308
65. Kroegel C, Bergmann N, Foerster M, Workalemahu G, Machnik A, Mock B, et al. Interferon-alphacon-1 treatment of three patients with severe glucocorticoid-dependent asthma. effect on disease control and systemic glucocorticosteroid dose. Respiration (2006) 73(4):566–70. doi: 10.1159/000088660
66. Marques-Pereira C, Pires MN, Gouveia RP, Pereira NN, Caniceiro AB, Rosario-Ferreira N, et al. SARS-CoV-2 membrane protein: From genomic data to structural new insights. Int J Mol Sci (2022) 23(6):2986. doi: 10.3390/ijms23062986
67. Li S, Xie T, Liu P, Wang L, Gong X. Structural insights into the assembly and substrate selectivity of human SPT-ORMDL3 complex. Nat Struct Mol Biol (2021) 28(3):249–57. doi: 10.1038/s41594-020-00553-7
68. Harayama T, Riezman H. Understanding the diversity of membrane lipid composition. Nat Rev Mol Cell Biol (2018) 19(5):281–96. doi: 10.1038/nrm.2017.138
69. Iwabuchi Y. Directing carboxylic acid dehydrogenation. Science (2021) 374(6572):1199. doi: 10.1126/science.abm4457
70. Chidambaram A, Sundararaju K, Chidambaram RK, Subbiah R, Jayaraj JM, Muthusamy K, et al. Design, synthesis, and characterization of alpha, beta-unsaturated carboxylic acid, and its urea based derivatives that explores novel epigenetic modulators in human non-small cell lung cancer A549 cell line. J Cell Physiol (2018) 233(7):5293–309. doi: 10.1002/jcp.26333
71. Rezazadeh Azari M, Mohammadian Y, Pourahmad J, Khodagholi F, Peirovi H, Mehrabi Y, et al. Individual and combined toxicity of carboxylic acid functionalized multi-walled carbon nanotubes and benzo a pyrene in lung adenocarcinoma cells. Environ Sci pollut Res Int (2019) 26(13):12709–19. doi: 10.1007/s11356-019-04795-x
72. Fischer FB, Mausezahl D, Wymann MN. Temporal trends in legionellosis national notification data and the effect of COVID-19, Switzerland, 2000-2020. Int J Hyg Environ Health (2022), 113970. doi: 10.1016/j.ijheh.2022.113970
73. Leuchter RK, Jackson NJ, Mafi JN, Sarkisian CA. Association between covid-19 vaccination and influenza vaccination rates. N Engl J Med (2022) 386(26):2531–2. doi: 10.1056/NEJMc2204560
74. Mittal S, Pahuja S, Madan M, Agarwal D, Mohan A, Madan K, et al. A case of legionellosis during the COVID-19 pandemic. J Clin Rheumatol (2021) 27(8S):S632–S3. doi: 10.1097/RHU.0000000000001689
75. Rana MS, Alam MM, Ikram A, Salman M, Mere MO, Usman M, et al. Emergence of measles during the COVID-19 pandemic threatens pakistan's children and the wider region. Nat Med (2021) 27(7):1127–8. doi: 10.1038/s41591-021-01430-6
76. Bizzotto J, Sanchis P, Abbate M, Lage-Vickers S, Lavignolle R, Toro A, et al. SARS-CoV-2 infection boosts MX1 antiviral effector in COVID-19 patients. iScience (2020) 23(10):101585. doi: 10.1016/j.isci.2020.101585
77. Zav'yalov VP, Hamalainen-Laanaya H, Korpela TK, Wahlroos T. Interferon-inducible myxovirus resistance proteins: Potential biomarkers for differentiating viral from bacterial infections. Clin Chem (2019) 65(6):739–50. doi: 10.1373/clinchem.2018.292391
78. Pillai PS, Molony RD, Martinod K, Dong H, Pang IK, Tal MC, et al. Mx1 reveals innate pathways to antiviral resistance and lethal influenza disease. Science (2016) 352(6284):463–6. doi: 10.1126/science.aaf3926
79. Haller O, Staeheli P, Schwemmle M, Kochs G. Mx GTPases: dynamin-like antiviral machines of innate immunity. Trends Microbiol (2015) 23(3):154–63. doi: 10.1016/j.tim.2014.12.003
80. Andolfo I, Russo R, Lasorsa VA, Cantalupo S, Rosato BE, Bonfiglio F, et al. Common variants at 21q22.3 locus influence MX1 and TMPRSS2 gene expression and susceptibility to severe COVID-19. iScience (2021) 24(4):102322. doi: 10.1016/j.isci.2021.102322
81. Chen Y, Graf L, Chen T, Liao Q, Bai T, Petric PP, et al. Rare variant MX1 alleles increase human susceptibility to zoonotic H7N9 influenza virus. Science (2021) 373(6557):918–22. doi: 10.1126/science.abg5953
82. Itazawa T, Adachi Y, Imamura H, Okabe Y, Yamamoto J, Onoue Y, et al. Increased lymphoid MxA expression in acute asthma exacerbation in children. Allergy (2001) 56(9):895–8. doi: 10.1034/j.1398-9995.2001.00312.x
83. Gaskill C, Marriott S, Pratap S, Menon S, Hedges LK, Fessel JP, et al. Shared gene expression patterns in mesenchymal progenitors derived from lung and epidermis in pulmonary arterial hypertension: identifying key pathways in pulmonary vascular disease. Pulm Circ (2016) 6(4):483–97. doi: 10.1086/688314
84. Diamond MS, Farzan M. The broad-spectrum antiviral functions of IFIT and IFITM proteins. Nat Rev Immunol (2013) 13(1):46–57. doi: 10.1038/nri3344
85. Li D, Swaminathan S. Human IFIT proteins inhibit lytic replication of KSHV: A new feed-forward loop in the innate immune system. PloS Pathog (2019) 15(2):e1007609. doi: 10.1371/journal.ppat.1007609
86. Zhou S, Butler-Laporte G, Nakanishi T, Morrison DR, Afilalo J, Afilalo M, et al. A Neanderthal OAS1 isoform protects individuals of European ancestry against COVID-19 susceptibility and severity. Nat Med (2021) 27(4):659–67. doi: 10.1038/s41591-021-01281-1
87. Larsen S, Kawamoto S, Tanuma S, Uchiumi F. The hematopoietic regulator, ELF-1, enhances the transcriptional response to interferon-beta of the OAS1 anti-viral gene. Sci Rep (2015) 5:17497. doi: 10.1038/srep17497
88. Kristiansen H, Scherer CA, McVean M, Iadonato SP, Vends S, Thavachelvam K, et al. Extracellular 2'-5' oligoadenylate synthetase stimulates RNase l-independent antiviral activity: a novel mechanism of virus-induced innate immunity. J Virol (2010) 84(22):11898–904. doi: 10.1128/JVI.01003-10
89. Politano G, Di Carlo S, Benso A. 'One DB to rule them all'-the RING: a regulatory INteraction graph combining TFs, genes/proteins, SNPs, diseases and drugs. Database (Oxford) (2019) 2019:baz108. doi: 10.1093/database/baz108
90. Liu J, Ding D, Zhong J, Liu R. Identifying the critical states and dynamic network biomarkers of cancers based on network entropy. J Transl Med (2022) 20(1):254. doi: 10.1186/s12967-022-03445-0
91. Augert A, Mathsyaraja H, Ibrahim AH, Freie B, Geuenich MJ, Cheng PF, et al. MAX functions as a tumor suppressor and rewires metabolism in small cell lung cancer. Cancer Cell (2020) 38(1):97–114.e7. doi: 10.1016/j.ccell.2020.04.016
92. Blanco-Melo D, Nilsson-Payant BE, Liu WC, Uhl S, Hoagland D, Moller R, et al. Imbalanced host response to SARS-CoV-2 drives development of COVID-19. Cell (2020) 181(5):1036–45.e9. doi: 10.1016/j.cell.2020.04.026
93. Bloomfield M, Parackova Z, Hanzlikova J, Lastovicka J, Sediva A. Immunogenicity and safety of COVID-19 mRNA vaccine in STAT1 GOF patients. J Clin Immunol (2022) 42(2):266–9. doi: 10.1007/s10875-021-01163-8
94. Ghaedi M, Shen ZY, Orangi M, Martinez-Gonzalez I, Wei L, Lu X, et al. Single-cell analysis of RORalpha tracer mouse lung reveals ILC progenitors and effector ILC2 subsets. J Exp Med (2020) 217(3):1–19. doi: 10.1084/jem.20182293
95. Luo W, Ding R, Guo X, Zhan T, Tang T, Fan R, et al. Clinical data mining reveals gancao-banxia as a potential herbal pair against moderate COVID-19 by dual binding to IL-6/STAT3. Comput Biol Med (2022) 145:105457. doi: 10.1016/j.compbiomed.2022.105457
96. Okazaki K, Anzawa H, Katsuoka F, Kinoshita K, Sekine H, Motohashi H. CEBPB is required for NRF2-mediated drug resistance in NRF2-activated non-small cell lung cancer cells. J Biochem (2022) 171(5):567–78. doi: 10.1093/jb/mvac013
97. Peebles RS Jr.. A new horizon in asthma: inhibiting ILC function. Sci Transl Med (2013) 5(174):174fs7. doi: 10.1126/scitranslmed.3005881
98. Ruiz EJ, Lan L, Diefenbacher ME, Riising EM, Da Costa C, Chakraborty A, et al. JunD, not c-jun, is the AP-1 transcription factor required for ras-induced lung cancer. JCI Insight (2021) 6(13):e124985. doi: 10.1172/jci.insight.124985
99. Steffen R, Lautenschlager S, Fehr J. Travel restrictions and lockdown during the COVID-19 pandemic-impact on notified infectious diseases in Switzerland. J Travel Med (2020) 27(8):taaa180. doi: 10.1093/jtm/taaa180
100. Zong S, Jiao Y, Liu X, Mu W, Yuan X, Qu Y, et al. FKBP4 integrates FKBP4/Hsp90/IKK with FKBP4/Hsp70/RelA complex to promote lung adenocarcinoma progression via IKK/NF-kappaB signaling. Cell Death Dis (2021) 12(6):602. doi: 10.1038/s41419-021-03857-8
101. Tsai MJ, Tsai YC, Chang WA, Lin YS, Tsai PH, Sheu CC, et al. Deducting MicroRNA-mediated changes common in bronchial epithelial cells of asthma and chronic obstructive pulmonary disease-a next-generation sequencing-guided bioinformatic approach. Int J Mol Sci (2019) 20(3):553. doi: 10.3390/ijms20030553
102. Li J, Panganiban R, Kho AT, McGeachie MJ, Farnam L, Chase RP, et al. Circulating MicroRNAs and treatment response in childhood asthma. Am J Respir Crit Care Med (2020) 202(1):65–72. doi: 10.1164/rccm.201907-1454OC
103. Bleck B, Grunig G, Chiu A, Liu M, Gordon T, Kazeros A, et al. MicroRNA-375 regulation of thymic stromal lymphopoietin by diesel exhaust particles and ambient particulate matter in human bronchial epithelial cells. J Immunol (2013) 190(7):3757–63. doi: 10.4049/jimmunol.1201165
104. Li X, Ye S, Lu Y. Long non-coding RNA NEAT1 overexpression associates with increased exacerbation risk, severity, and inflammation, as well as decreased lung function through the interaction with microRNA-124 in asthma. J Clin Lab Anal (2020) 34(1):e23023. doi: 10.1002/jcla.23023
105. Trinh HKT, Pham DL, Kim SC, Kim RY, Park HS, Kim SH. Association of the miR-196a2, miR-146a, and miR-499 polymorphisms with asthma phenotypes in a Korean population. Mol Diagn Ther (2017) 21(5):547–54. doi: 10.1007/s40291-017-0280-1
106. Sun W, Ma Y, Chen P, Wang D. MicroRNA-10a silencing reverses cisplatin resistance in the A549/cisplatin human lung cancer cell line via the transforming growth factor-beta/Smad2/STAT3/STAT5 pathway. Mol Med Rep (2015) 11(5):3854–9. doi: 10.3892/mmr.2015.3181
107. Carreras-Badosa G, Runnel T, Plaas M, Karner J, Ruckert B, Lattekivi F, et al. microRNA-146a is linked to the production of IgE in mice but not in atopic dermatitis patients. Allergy (2018) 73(12):2400–3. doi: 10.1111/all.13579
108. Hammad Mahmoud Hammad R, Hamed D, Eldosoky M, Ahmad A, Osman HM, Abd Elgalil HM, et al. Plasma microRNA-21, microRNA-146a and IL-13 expression in asthmatic children. Innate Immun (2018) 24(3):171–9. doi: 10.1177/1753425918763521
109. Sardar R, Satish D, Gupta D. Identification of novel SARS-CoV-2 drug targets by host MicroRNAs and transcription factors Co-regulatory interaction network analysis. Front Genet (2020) 11:571274. doi: 10.3389/fgene.2020.571274
110. Bundgaard H, Bundgaard JS, Raaschou-Pedersen DET, von Buchwald C, Todsen T, Norsk JB, et al. Effectiveness of adding a mask recommendation to other public health measures to prevent SARS-CoV-2 infection in Danish mask wearers : A randomized controlled trial. Ann Intern Med (2021) 174(3):335–43. doi: 10.7326/M20-6817
111. Paniri A, Hosseini MM, Moballegh-Eslam M, Akhavan-Niaki H. Comprehensive in silico identification of impacts of ACE2 SNPs on COVID-19 susceptibility in different populations. Gene Rep (2021) 22:100979. doi: 10.1016/j.genrep.2020.100979
112. Gong C, Cheng Z, Yang Y, Shen J, Zhu Y, Ling L, et al. A 10-miRNA risk score-based prediction model for pathological complete response to neoadjuvant chemotherapy in hormone receptor-positive breast cancer. Sci China Life Sci (2022). doi: 10.1007/s11427-022-2104-3
113. Sartorius K, An P, Winkler C, Chuturgoon A, Li X, Makarova J, et al. The epigenetic modulation of cancer and immune pathways in hepatitis b virus-associated hepatocellular carcinoma: The influence of HBx and miRNA dysregulation. Front Immunol (2021) 12:661204. doi: 10.3389/fimmu.2021.661204
114. Wu D, Deng S, Li L, Liu T, Zhang T, Li J, et al. TGF-beta1-mediated exosomal lnc-MMP2-2 increases blood-brain barrier permeability via the miRNA-1207-5p/EPB41L5 axis to promote non-small cell lung cancer brain metastasis. Cell Death Dis (2021) 12(8):721. doi: 10.1038/s41419-021-04004-z
115. Zhang W, Zhang Q, Che L, Xie Z, Cai X, Gong L, et al. Using biological information to analyze potential miRNA-mRNA regulatory networks in the plasma of patients with non-small cell lung cancer. BMC Cancer (2022) 22(1):299. doi: 10.1186/s12885-022-09281-1
116. Pan KY, Kok AAL, Eikelenboom M, Horsfall M, Jorg F, Luteijn RA, et al. The mental health impact of the COVID-19 pandemic on people with and without depressive, anxiety, or obsessive-compulsive disorders: a longitudinal study of three Dutch case-control cohorts. Lancet Psychiatry (2021) 8(2):121–9. doi: 10.1016/S2215-0366(20)30491-0
117. Sabatini S, Bennett HQ, Martyr A, Collins R, Gamble LD, Matthews FE, et al. Minimal impact of COVID-19 pandemic on the mental health and wellbeing of people living with dementia: Analysis of matched longitudinal data from the IDEAL study. Front Psychiatry (2022) 13:849808. doi: 10.3389/fpsyt.2022.849808
118. Hu H, Tang N, Zhang F, Li L, Li L. Bioinformatics and system biology approach to identify the influences of COVID-19 on rheumatoid arthritis. Front Immunol (2022) 13:860676. doi: 10.3389/fimmu.2022.860676
119. Wisnivesky JP, Markowitz SB, James S, Stone K, Dickens B, Busse P, et al. Comorbid posttraumatic stress disorder and major depressive disorder are associated with asthma morbidity among world trade center workers. Ann Allergy Asthma Immunol (2021) 126(3):278–83. doi: 10.1016/j.anai.2020.10.007
120. Filipowski M, Bozek A, Kozlowska R, Czyzewski D, Jarzab J. The influence of hospitalizations due to exacerbations or spontaneous pneumothoraxes on the quality of life, mental function and symptoms of depression and anxiety in patients with COPD or asthma. J Asthma (2014) 51(3):294–8. doi: 10.3109/02770903.2013.862543
121. Zhu W, Xu D, Li H, Xu G, Tian J, Lyu L, et al. Impact of long-term home quarantine on mental health and physical activity of people in shanghai during the COVID-19 pandemic. Front Psychiatry (2021) 12:782753. doi: 10.3389/fpsyt.2021.782753
122. Barrett EA, Simonsen C, Aminoff SR, Hegelstad WTV, Lagerberg TV, Melle I, et al. The COVID-19 pandemic impact on wellbeing and mental health in people with psychotic and bipolar disorders. Brain Behav (2022) 12(5):e2559. doi: 10.1002/brb3.2559
123. Collaborators C-MD. Global prevalence and burden of depressive and anxiety disorders in 204 countries and territories in 2020 due to the COVID-19 pandemic. Lancet (2021) 398(10312):1700–12. doi: 10.1016/S0140-6736(21)02143-7
124. Skevaki C, Karsonova A, Karaulov A, Xie M, Renz H. Asthma-associated risk for COVID-19 development. J Allergy Clin Immunol (2020) 146(6):1295–301. doi: 10.1016/j.jaci.2020.09.017
125. Oreskovic NM, Kinane TB, Aryee E, Kuhlthau KA, Perrin JM. The unexpected risks of COVID-19 on asthma control in children. J Allergy Clin Immunol Pract (2020) 8(8):2489–91. doi: 10.1016/j.jaip.2020.05.027
126. Solomon DA, Sherman AC, Kanjilal S. Influenza in the COVID-19 era. JAMA (2020) 324(13):1342–3. doi: 10.1001/jama.2020.14661
127. Mehta P, Sanchez E, Moraitis E, Longley N, Lendrem DW, Giles IP, et al. Influenza vaccination and interruption of methotrexate in adult patients in the COVID-19 era: an ongoing dilemma. Lancet Rheumatol (2021) 3(1):e9–e10. doi: 10.1016/S2665-9913(20)30392-1
128. Malin JJ, Suarez I, Priesner V, Fatkenheuer G, Rybniker J. Remdesivir against COVID-19 and other viral diseases. Clin Microbiol Rev (2020) 34(1):e00162-20. doi: 10.1128/CMR.00162-20
129. Lagocka R, Dziedziejko V, Klos P, Pawlik A. Favipiravir in therapy of viral infections. J Clin Med (2021) 10(2):273. doi: 10.3390/jcm10020273
130. Im J, Kim W, Kim KT, Chung SK. Preparation of a 3'-azido-3'-deoxythymidine (AZT) derivative, which is blood-brain barrier permeable. Chem Commun (Camb) (2009) 31:4669–71. doi: 10.1039/b909541a
131. Gedela K, Rajus N, Luis H, Fridayantara WD, Irwanto I, Sukmaningrum E, et al. Antiretroviral drug switches to zidovudine-based regimens and loss to follow-up during the first COVID-19 lockdown in Bali, Indonesia. HIV Med (2022). doi: 10.1111/hiv.13298
132. Tompa DR, Immanuel A, Srikanth S, Kadhirvel S. Trends and strategies to combat viral infections: A review on FDA approved antiviral drugs. Int J Biol Macromol. (2021) 172:524–41. doi: 10.1016/j.ijbiomac.2021.01.076
133. McCoy K, Gudapati S, He L, Horlander E, Kartchner D, Kulkarni S, et al. Biomedical text link prediction for drug discovery: A case study with COVID-19. Pharmaceutics (2021) 13(6):794. doi: 10.3390/pharmaceutics13060794
134. Szkudlarek A, Wilk M, Maciazek-Jurczyk M. In vitro investigations of acetohexamide binding to glycated serum albumin in the presence of fatty acid. Molecules (2020) 25(10):2340. doi: 10.3390/molecules25102340
135. Endo S, Miyagi N, Matsunaga T, Ikari A. Rabbit dehydrogenase/reductase SDR family member 11 (DHRS11): Its identity with acetohexamide reductase with broad substrate specificity and inhibitor sensitivity, different from human DHRS11. Chem Biol Interact (2019) 305:12–20. doi: 10.1016/j.cbi.2019.03.026
136. Zhang Y, Greer RA, Song Y, Praveen H, Song Y. In silico identification of available drugs targeting cell surface BiP to disrupt SARS-CoV-2 binding and replication: Drug repurposing approach. Eur J Pharm Sci (2021) 160:105771. doi: 10.1016/j.ejps.2021.105771
137. Clark NF, Taylor-Robinson AW. COVID-19 therapy: Could a copper derivative of chlorophyll a be used to treat lymphopenia associated with severe symptoms of SARS-CoV-2 infection? Front Med (Lausanne) (2021) 8:620175. doi: 10.3389/fmed.2021.620175
138. Zheng H, You Y, Hua M, Wu P, Liu Y, Chen Z, et al. Chlorophyllin modulates gut microbiota and inhibits intestinal inflammation to ameliorate hepatic fibrosis in mice. Front Physiol (2018) 9:1671. doi: 10.3389/fphys.2018.01671
139. Yang Y, Jiang X, Pandol SJ, Han YP, Zheng X. Green plant pigment, chlorophyllin, ameliorates non-alcoholic fatty liver diseases (NAFLDs) through modulating gut microbiome in mice. Front Physiol (2021) 12:739174. doi: 10.3389/fphys.2021.739174
140. Su S, Hua D, Li JP, Zhang XN, Bai L, Cao LB, et al. Modulation of innate immune response to viruses including SARS-CoV-2 by progesterone. Signal Transduct Target Ther (2022) 7(1):137. doi: 10.1038/s41392-022-00981-5
141. Shah SB. COVID-19 and progesterone: Part 1. SARS-CoV-2, progesterone and its potential clinical use. Endocr Metab Sci (2021) 5:100109. doi: 10.1016/j.endmts.2021.100109
142. Yang C, Pan X, Huang Y, Cheng C, Xu X, Wu Y, et al. Drug repurposing of itraconazole and estradiol benzoate against COVID-19 by blocking SARS-CoV-2 spike protein-mediated membrane fusion. Adv Ther (Weinh) (2021) 4(5):2000224. doi: 10.1002/adtp.202000224
143. Yuan L, Zhu H, Wu K, Zhou M, Ma J, Chen R, et al. Female sex hormone, progesterone, ameliorates the severity of SARS-CoV-2-caused pneumonia in the Syrian hamster model. Signal Transduct Target Ther (2022) 7(1):47. doi: 10.1038/s41392-021-00860-5
144. Zeng B, Li J, Wang Y, Chen P, Wang X, Cui J, et al. In vitro and in vivo effects of suloctidil on growth and biofilm formation of the opportunistic fungus candida albicans. Oncotarget (2017) 8(41):69972–82. doi: 10.18632/oncotarget.19542
145. Chung MW, Komorowski RA, Varma RR. Suloctidil-induced hepatotoxicity. Gastroenterology (1988) 95(2):490–1. doi: 10.1016/0016-5085(88)90510-0
146. Joseph A, Kumar GJ, Pawar SD, Hirlekar BU, Bharatam PV, Konda S, et al. Analytical developments of p-hydroxy prenylamine reference material for dope control research: Characterization and purity assessment. Drug Test Anal (2022) 14(2):224–32. doi: 10.1002/dta.3171
147. Shah RR, Stonier PD. Withdrawal of prenylamine: perspectives on pharmacological, clinical and regulatory outcomes following the first QT-related casualty. Ther Adv Drug Saf (2018) 9(8):475–93. doi: 10.1177/2042098618780854
148. Kehinde IA, Egbeyemi A, Kaur M, Onyenaka C, Adebusuyi T, Olaleye OA. Inhibitory mechanism of clioquinol and its derivatives at the exopeptidase site of human angiotensin-converting enzyme-2 and receptor binding domain of SARS-CoV-2 viral spike. J Biomol Struct Dyn (2022) 1–10. doi: 10.1080/07391102.2022.2043938
149. Lin G, Zhu F, Kanaan NM, Asano R, Shirafuji N, Sasaki H, et al. Clioquinol decreases levels of phosphorylated, truncated, and oligomerized tau protein. Int J Mol Sci (2021) 22(21):12063. doi: 10.3390/ijms222112063
150. Huang A, Zeng P, Li Y, Lu W, Lai Y. LY294002 is a promising inhibitor to overcome sorafenib resistance in FLT3-ITD mutant AML cells by interfering with PI3K/Akt signaling pathway. Front Oncol (2021) 11:782065. doi: 10.3389/fonc.2021.782065
151. Caglioti C, Palazzetti F, Monarca L, Lobello R, Ceccarini MR, Iannitti RG, et al. LY294002 inhibits intermediate conductance calcium-activated potassium (KCa3.1) current in human glioblastoma cells. Front Physiol (2021) 12:790922. doi: 10.3389/fphys.2021.790922
Keywords: coronavirus disease 2019, allergic asthma, disease biomarker, hub gene, gene ontology, drug, systems-biology, bioinformatics
Citation: Fang H, Sun Z, Chen Z, Chen A, Sun D, Kong Y, Fang H and Qian G (2022) Bioinformatics and systems-biology analysis to determine the effects of Coronavirus disease 2019 on patients with allergic asthma. Front. Immunol. 13:988479. doi: 10.3389/fimmu.2022.988479
Received: 07 July 2022; Accepted: 30 August 2022;
Published: 23 September 2022.
Edited by:
Weishan Huang, Louisiana State University, United StatesReviewed by:
Linxiang Yin, Harvard Medical School, United StatesCopyright © 2022 Fang, Sun, Chen, Chen, Sun, Kong, Fang and Qian. This is an open-access article distributed under the terms of the Creative Commons Attribution License (CC BY). The use, distribution or reproduction in other forums is permitted, provided the original author(s) and the copyright owner(s) are credited and that the original publication in this journal is cited, in accordance with accepted academic practice. No use, distribution or reproduction is permitted which does not comply with these terms.
*Correspondence: Guojun Qian, cWlhbmd1b2p1bkBnemhtdS5lZHUuY24=; Hao Fang, ZHJmYW5naGFvQDE2My5jb20=
†These authors have contributed equally to this work
Disclaimer: All claims expressed in this article are solely those of the authors and do not necessarily represent those of their affiliated organizations, or those of the publisher, the editors and the reviewers. Any product that may be evaluated in this article or claim that may be made by its manufacturer is not guaranteed or endorsed by the publisher.
Research integrity at Frontiers
Learn more about the work of our research integrity team to safeguard the quality of each article we publish.