- 1Department of Anesthesiology, Shanghai Pulmonary Hospital, School of Medicine, Tongji University, Shanghai, China
- 2Department of Thoracic Surgery, Shanghai Pulmonary Hospital, School of Medicine, Tongji University, Shanghai, China
- 3Department of Cardiothoracic Surgery, Changzheng Hospital, Naval Medical University, Shanghai, China
- 4Department of Health Statistics, Naval Medical University, Shanghai, China
- 5Department of Orthopaedics, Changzheng Hospital, Naval Medical University, Shanghai, China
Background: This research aimed to investigate the predictive performance of log odds of positive lymph nodes (LODDS) for the long-term prognosis of patients with node-positive lung neuroendocrine tumors (LNETs).
Methods: We collected 506 eligible patients with resected N1/N2 classification LNETs from the Surveillance, Epidemiology, and End Results (SEER) database between 2004 and 2015. The study cohort was split into derivation cohort (n=300) and external validation cohort (n=206) based on different geographic regions. Nomograms were constructed based on the derivation cohort and validated using the external validation cohort to predict the 1-, 3-, and 5-year cancer-specific survival (CSS) and overall survival (OS) of patients with LNETs. The accuracy and clinical practicability of nomograms were tested by Harrell’s concordance index (C-index), integrated discrimination improvement (IDI), net reclassification improvement (NRI), calibration plots, and decision curve analyses.
Results: The Cox proportional-hazards model showed the high LODDS group (-0.79≤LODDS) had significantly higher mortality compared to those in the low LODDS group (LODDS<-0.79) for both CSS and OS. In addition, age at diagnosis, sex, histotype, type of surgery, radiotherapy, and chemotherapy were also chosen as predictors in Cox regression analyses using stepwise Akaike information criterion method and included in the nomograms. The values of C-index, NRI, and IDI proved that the established nomograms were better than the conventional eighth edition of the TNM staging system. The calibration plots for predictions of the 1-, 3-, and 5-year CSS/OS were in excellent agreement. Decision curve analyses showed that the nomograms had value in terms of clinical application.
Conclusions: We created visualized nomograms for CSS and OS of LNET patients, facilitating clinicians to bring individually tailored risk assessment and therapy.
Introduction
Lung neuroendocrine tumors (LNETs) originate from pulmonary neuroendocrine cells, accounting for approximately 25% of primary lung neoplasms (1). The incidence of LNET is less than 0.002% as reported in some countries (2–4). Owing to the increased lung cancer screening, the annual incidence of LNET has substantially increased, rising from 0.0003% in 1973 to 0.0014% in 2004 in the United States (5–7). Currently, the 2015 World Health Organization (WHO) classification has grouped LNETs into four histologic variants based on their histopathologic features: typical carcinoid (TC), atypical carcinoid (AC), large cell neuroendocrine carcinoma (LCNEC), and small cell lung carcinoma (SCLC) (8). The 5-year survival rate of non-small cell lung cancer (NSCLC) was approximately 16%, while the corresponding rate of SCLC, LCNEC, AC, and TC patients was 5%, 17%, 64%, and 84%, respectively (9, 10). Due to the rarity and morphological heterogeneity of these tumors, there have been limited clinical data available regarding LNETs, thus making their diagnosis, staging, risk assessment, and treatment challenging (1). Although specific to NSCLC, the international American Joint Committee on Cancer/Union for International Cancer Control (AJCC/UICC) tumor-node-metastasis (TNM) staging system has been applied to LNETs (11, 12). However, several studies have shown an overlapping survival of patients with LNETs, particularly in stages II and III (11–15). Therefore, further investigation is warranted to optimize the staging system for LNETs.
Lymph node (LN) involvement is a significant prognostic factor for staging and risk stratification. A combination of the number of positive lymph nodes (NPLN), the number of dissected lymph nodes (NDLN), and the anatomic location of LN metastasis have been applied in the staging of many malignancies (16–20). However, the latest version of AJCC/UICC TNM classification of lung cancer did not take account of any number- or ratio-based LN staging system, which could affect the precision of prognosis evaluation (21, 22). A novel prognostic indicator known as log odds of positive lymph nodes (LODDS) is being utilized to identify patients with a homogeneous prognosis in many malignant tumors, including NSCLC (23–27). However, no specific study has focused on its prognostic significance in LNETs till now.
The present study aimed to determine whether LODDS could be utilized to predict the cancer-specific survival (CSS) or overall survival (OS) of patients with node-positive (N1/N2 classification) LNET using the Surveillance, Epidemiology, and End Results (SEER) database. Based on LODDS, the research intended to construct two visualized and online nomograms which are practical tools for clinical prediction used in many diseases (28–32). To facilitate clinical use, we also constructed two visualized and online nomograms for LNETs.
Methods
Study design, data screening, and ethical statement
This is a multi-center retrospective cohort study according to the parts of the methods described in our previous studies (25, 26, 33). We use the Transparent Reporting of a multivariate prediction model for Individual Prediction or Diagnosis (TRIPOD) for reporting (34). The data of this study were downloaded from the SEER 18 registries research database, covering approximately 28% of the population of USA (35). Data were extracted using the SEER*Stat version 8.3.9 software. The requirement for approval by the institutional review board and individual patient consent was waived since the study made use of the database's anonymous data. In summary, this study complied with the Declaration of Helsinki (36).
Population selection
Data on the patients with lung cancer was obtained from the SEER database. Inclusion criteria were as followed (1): diagnosed from 2004 to 2015; (2) site recode “ICD-O-3/WHO 2008” restricted to “Lung and Bronchus”; and (3) pathologically confirmed as TC (ICD-O-3 code: 8240/3), AC (ICD-O-3 code: 8249/3) or LCNEC (ICD-O-3 code: 8013/3). The study period was set from 2004 to 2015, as the sixth or seventh edition of TMN classification and Collaborative Stage information was available in the database since 2004. Besides, we reclassified the TNM staging system according to the 8th version of TNM classification because the TNM staging system had multiple versions in the SEER database and did not apply to all patients (37). Furthermore, considering its strong invasion ability and unique pathological characteristics limiting the surgical options, SCLC was not included in the present study (38, 39). Although LCNEC was reported to contain subgroups of tumors showing SCLC characteristics and others with NSCLC-like features, surgery could be considered for early and locally advanced LCNEC (40–42). Therefore, we enrolled patients with LCNEC in this study. Patients were excluded who (1) aged<18 years; (2) had a diagnosis of any other cancer; (3) did not undergo radical surgery with systematic LN dissection; (4) had the diagnosis lacking pathological evidence; (5) were at pN0/pN3 disease; (6) had distant metastasis (M1); (7) received preoperative radiotherapy; (8) died within one month after surgery; (9) had unknown information of race, laterality, tumor location, radiotherapy, TNM staging system, and CSS/OS.
Variable extraction, preparation, grouping, and calculation
The baseline demographics data including age at diagnosis (<65 and ≥65), sex (male and female), and race (white, black, and other) were extracted from the SEER database. Baseline tumor-related characteristics included primary site (upper lobe, middle lobe, lower lobe, and other), laterality (right and left), histotype (TC, AC, and LCNEC), tumor differentiation (well/moderately differentiated, poorly differentiated/undifferentiated, and unknown), T classification (T1, T2, T3, and T4), and N classification (N1 and N2). In addition, treatment information including surgical intervention (sublobectomy, lobectomy, and pneumonectomy), radiotherapy (yes and no/unknown), chemotherapy (yes and no/unknown), NDLN, and NPLN was also extracted from the database. LODDS was calculated as: . To avoid an infinite number, 0.50 was added to both the numerator and denominator. CSS and OS were two of the study endpoints. The period from diagnosis to all-cause death was referred to as OS, while the time from diagnosis to LNET-related death was referred to as CSS. For censored data, the follow-up duration was computed as the number of months between diagnosis and death or the last follow-up (December 31, 2016).
Construction and validation of nomograms
Baseline features of the study groups stratified by LODDS were compared using Pearson’s χ2 test, Fisher’s exact test, Student t test, or Mann-Whitney test as appropriate. Categorical variables were presented as counts and percentages, while continuous variables were reported as the mean (standard deviation [SD]) or the median (interquartile range [IQR]).
Patients from purchased/referred care delivery areas (PRCDA) of Northern plains, East, and Alaska were considered to be the derivation cohort, whereas the external validation cohort includes patients from PRCDA of Southwest and Pacific coast. LODDS was dichotomized via the X-tile software to achieve the largest difference in survival outcome by selecting the highest χ2 value in survival analysis indicating the largest survival difference (43). First, age at diagnosis, sex, race, laterality, primary site, histotype, tumor differentiation, T classification, N classification, surgery, radiotherapy, chemotherapy, and LODDS were analyzed by univariable Cox-proportional-hazards regression analysis. Second, the potential predictors with P<0.1 were put into the multivariable Cox-proportional-hazards regression analysis using the stepwise Akaike information criterion method (stepAIC) to select the optimal predictors for the final models (44). The results are presented as hazard ratios (HRs) with 95% confidence intervals (CIs). Using these identified prognostic factors, we constructed two nomograms for predicting 1-, 3-, and 5-year CSS and OS in LNET patients.
Several indexes and methods were used to assess the precision of our nomograms. First, Harrell’s concordance index (C-index) was used to evaluate the discrimination power of the nomograms. Second, a calibration plot, a curve presenting the conformity between predicted and actual survival rate at 1, 3, and 5 years, along with bootstrapped sampling of the population, was used to assess the calibration. Third, a comparison between our nomograms and the conventional 8th version of the TNM staging system was conducted by calculating the net reclassification improvement (NRI) and integrated discrimination improvement (IDI) (45). Z test was used to examine the difference. Fourth, the receiver operating characteristic (ROC) curve and decision curve analysis (DCA) were performed to test the clinical usefulness of the nomograms and TNM classification. Kaplan-Maier curves were applied for comparing the discriminative power of nomograms and TNM staging system in the entire study cohort. Finally, to facilitate patients and doctors in using the models, two user-friendly webservers for our nomograms were established.
All statistical analyses were performed using R software (version.3.6.14.1.0; The R Project for Statistical Computing, TX, USA; http://www.r-project.org) and EmpowerStats (version 2.0; http://www.empowerstats.com). Two-tailed P<0.05 was deemed as statistical significance.
Results
Patients characteristics and cutoff value for LOODS
The SEER database collected 11,870 patients diagnosed with LNETs from January 2004 to December 2015. After employing the inclusion and exclusion criteria, 506 patients remained in the final study cohort. The selection process was summarized in Figure 1. According to the X-tile software's calculations, the optimal cutoff value of LODDS was set as -0.79. Baseline demographic and clinicopathological variables of participants in the derivation dataset and external validation dataset were summarized in Table 1. The median number of NDLN was 9.00 (IQR: 6.00-14.00) in the derivation dataset and 9.00 (IQR: 6.00-15.00) in the validation dataset, whereas the median number of NPLN was 1.00 (IQR: 1.00-2.00) in the derivation dataset and 2.00 (IQR: 1.00-3.00) in the validation dataset. Compared with the derivation dataset, the validation dataset had more patients with other race (P-value<0.001). No difference was observed in other variables (all P-values>0.05).
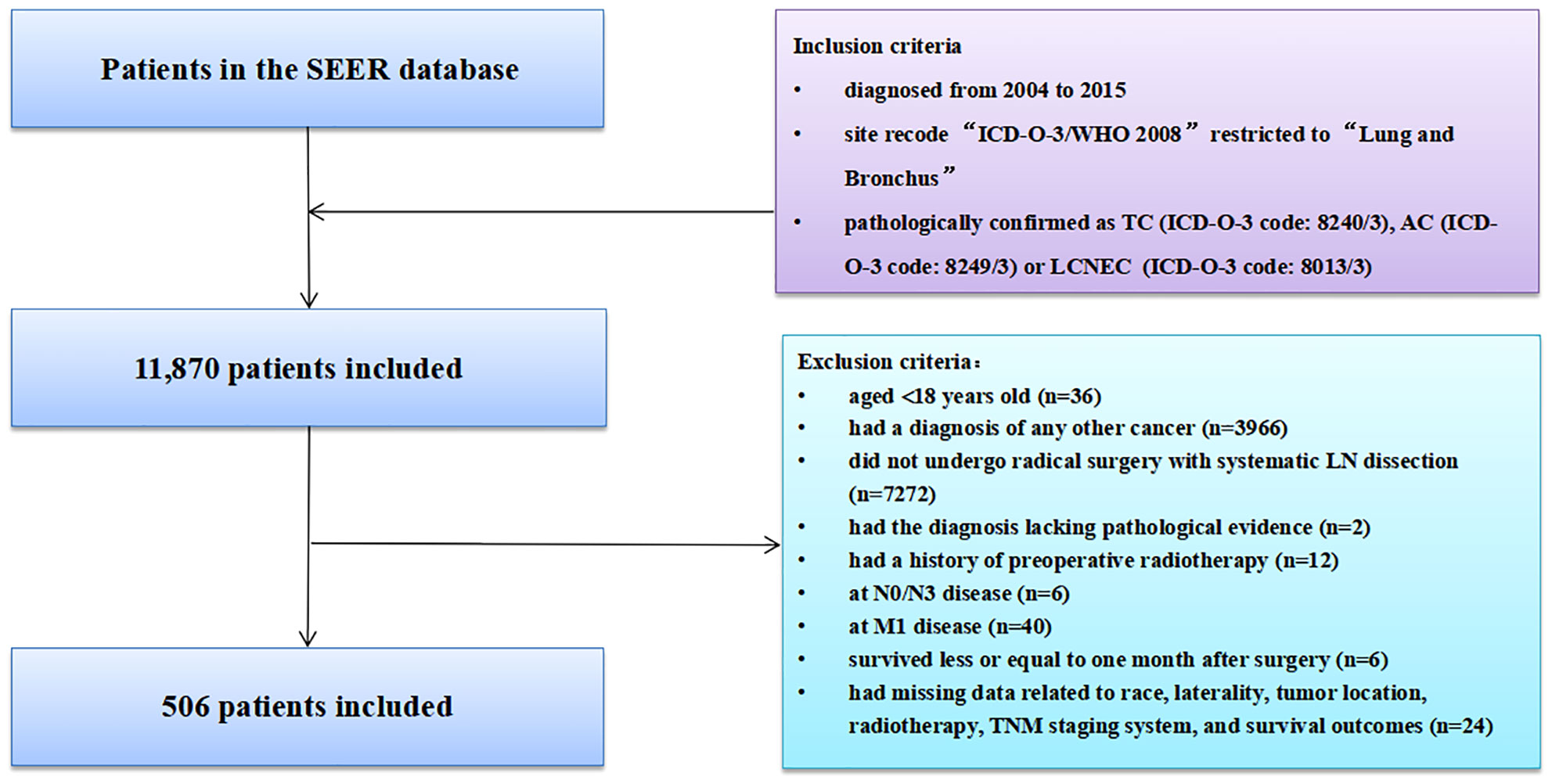
Figure 1 Selection of study cohort from the SEER database. AC, atypical carcinoid; LCNEC, large cell neuroendocrine carcinoma; SCLC, small cell lung carcinoma; SEER, Surveillance, Epidemiology, and End Results; LN, lymph node; TNM, tumor-node-metastasis.
Survival analysis
The median follow-up time for the entire cohort was 42 months (IQR: 19-77 months). Among 506 participants, 180 (35.57%) participants died from any cause, and 135 (26.68%) participants died from LNET-related death in the end of the follow-up. Multivariable Cox regression analysis showed that participants in the high LODDS group (-0.79≤LODDS) was associated with reducing CSS compared to those in the low LODDS group (LODDS<-0.79) (HR=2.21, 95% CI: 1.38-3.52, P<0.001) (Table 2). The multivariable Cox regression analysis for OS yielded similar results (HR=1.76, 95% CI: 1.20-2.58, P=0.004, Table 3). To diminish the potential bias caused by the LN fragments, we performed a sensitivity analysis by restricting the resected LN count to fewer than or equal to 20, and found that LODDS remained statistically significant (CSS: HR=2.06, 95% CI: 1.28-3.31, P=0.003; OS: HR=1.68, 95% CI: 1.12-2.53, P=0.012) (Tables S1, S2). Furthermore, the extent of LN management should be in accordance with the IASLC recommendations, which recommended examination of at least 6 nodes/stations, therefore we excluded patients with the examined LN count to fewer than 6. The multivariable Cox regression analysis yielded similar results (CSS: HR=3.64, 95% CI: 1.99-6.68, P<0.001; OS: HR=2.00, 95% CI: 1.23-3.23, P=0.005) (Tables S3, S4).
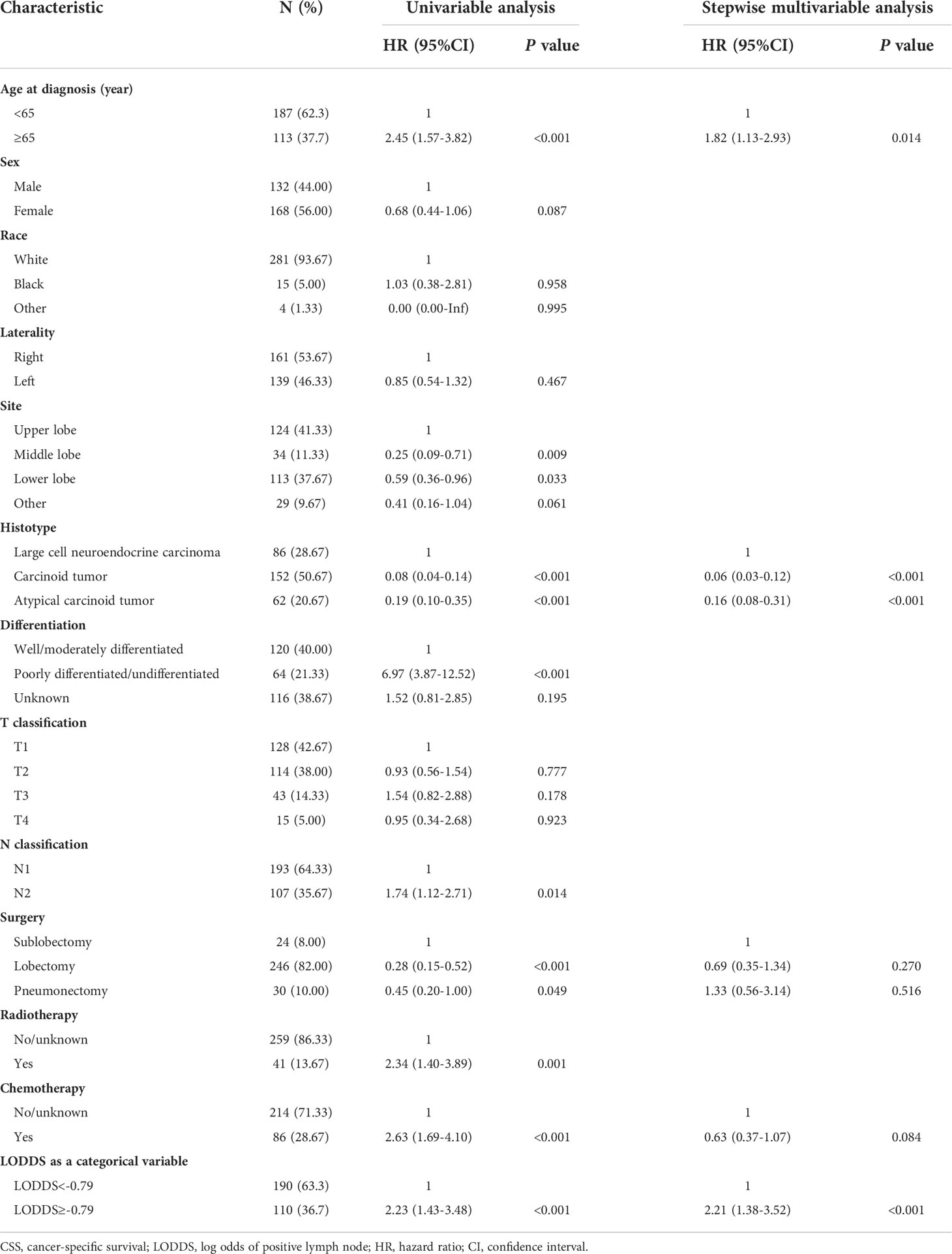
Table 2 Univariable and stepwise multivariable Cox proportional regression analysis for CSS of the derivation dataset.
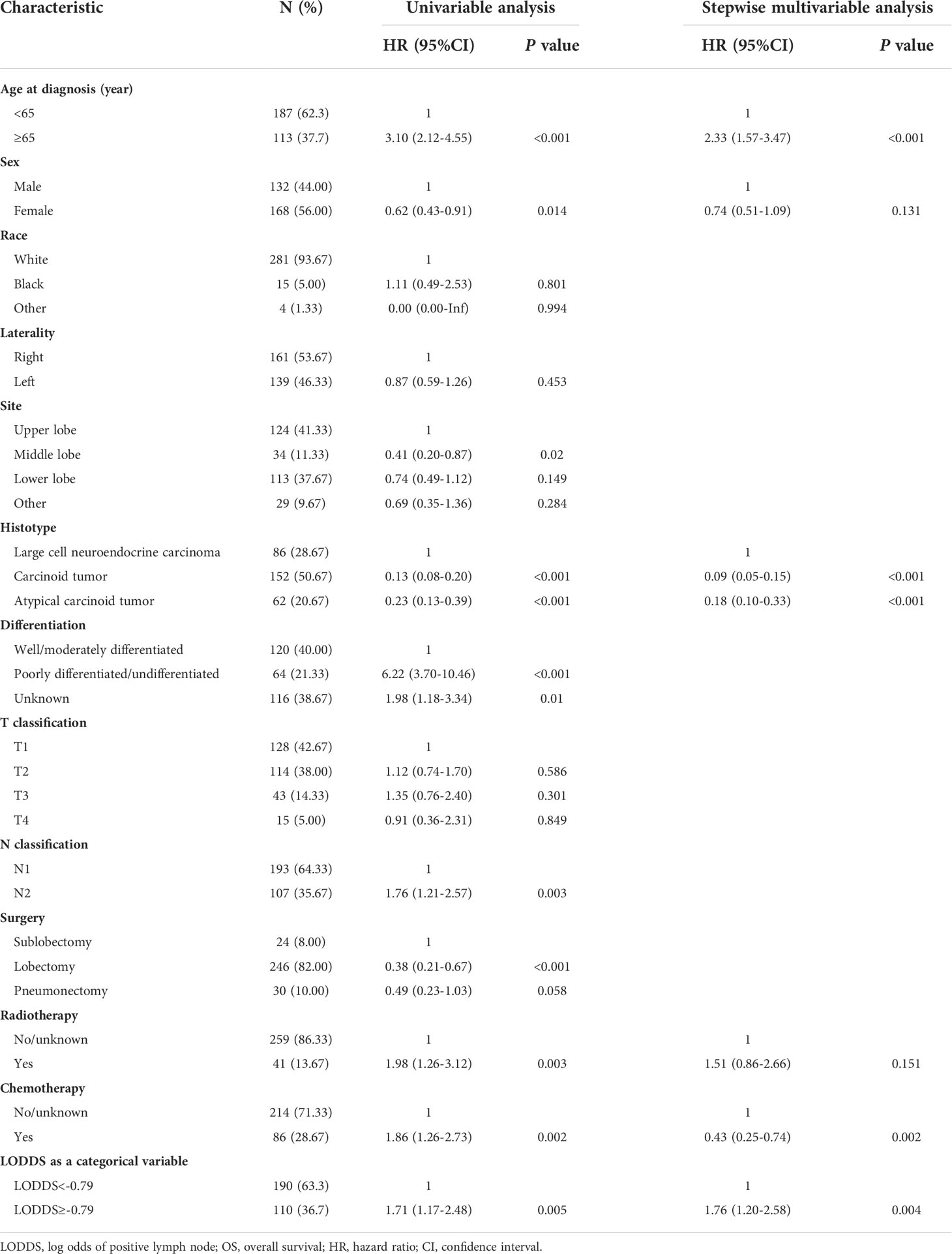
Table 3 Univariable and stepwise multivariable Cox proportional regression analysis for OS of the derivation dataset.
Construction and validation of the nomograms
Prognostic nomograms for CSS and OS were established including optimal indicators selected by stepAIC method in multivariable Cox regression analysis. For nomogram construction and validation, among the final study cohort including 506 patients, 300 of them were assigned to the derivation cohort (PRCDA=Northern plains, East, and Alaska) and 206 of them were assigned to the validation cohort (PRCDA=Southwest and Pacific coast). The nomogram of CSS showed that histotype made the largest contribution to prediction, followed by LODDS, surgery, age at diagnosis, and chemotherapy (Figure 2A and Table 2). Similarly, the nomogram of OS showed that histotype made the largest contribution to prediction, followed by chemotherapy, age at diagnosis, LODDS, radiotherapy and sex (Figure 2B and Table 3). The top point reference scale of the nomograms assigned a score for each category of these predictive variables. After adding up the total score and locating the sum on the total points reference scale, a straight line was drawn to the bottom survival probability scale to find the estimated 1-/3-/5- survival rate. For example, for a LNET patient aged 65 (21 points) who had a diagnosis of large cell neuroendocrine carcinoma (100 points) and underwent lobectomy (0 points) with LODDS<-0.79 (0 points) and received chemotherapy (0 points), the total points were 121 points, corresponding to a 1-year CSS of 83%, in addition, the online dynamic nomogram could give a 95%CI of 74-92% (Figure 2A).
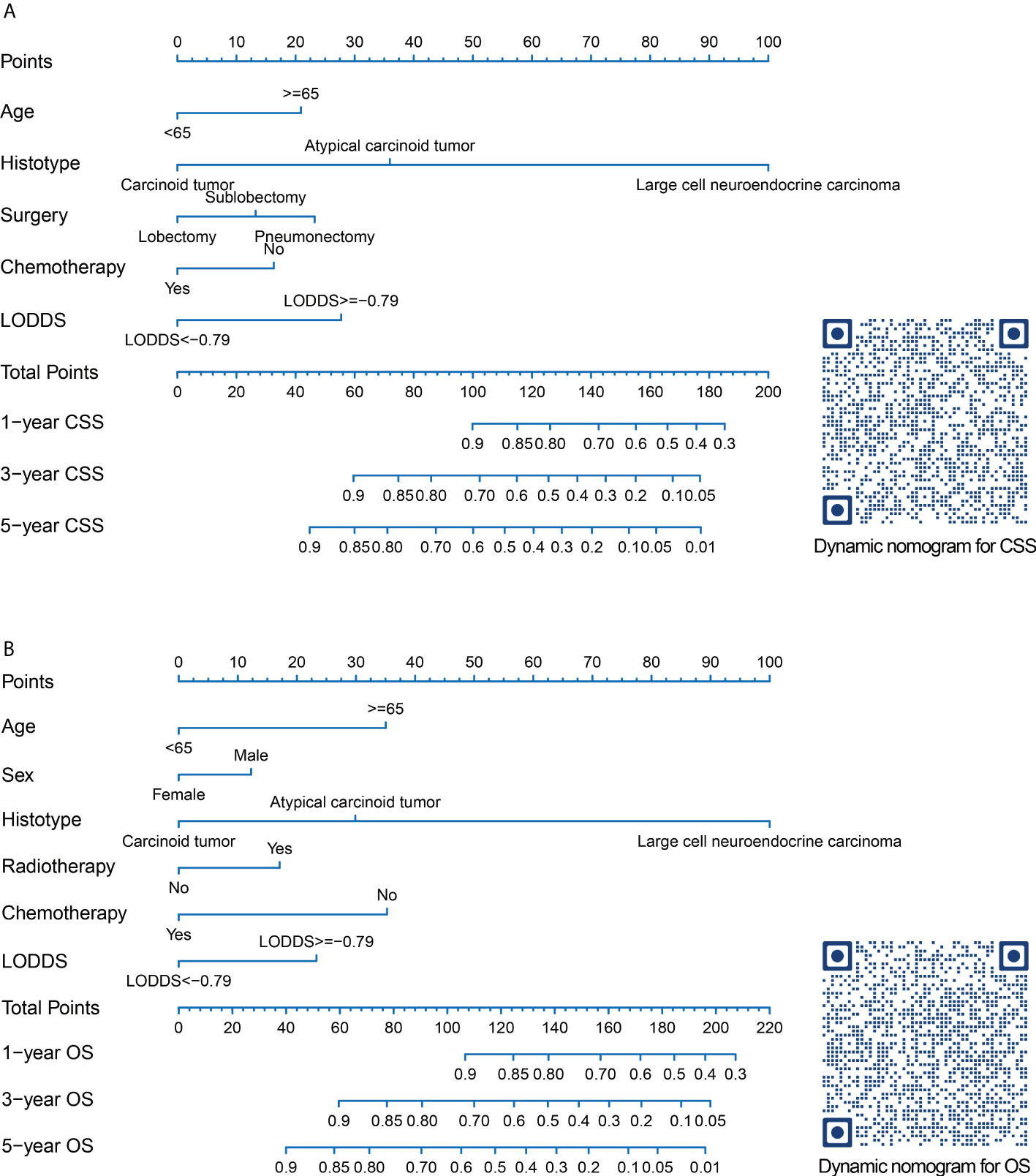
Figure 2 Nomograms and quick response codes of the webservers of the nomograms to predict 1-, 3- and 5-year CSS (A) and OS (B) for patients with node-positive lung neuroendocrine carcinoma after surgery. CSS, lung cancer-specific survival; OS, overall survival; LODDS, log odds of positive lymph nodes.
The nomograms were validated internally in the derivation cohort and externally in the validation cohort. C-indexes of nomogram for CSS in derivation cohort and validation cohort were 0.843 (0.801-0.885), and 0.809 (0.755-0.863). C-indexes of OS for OS in derivation cohort and validation cohort were 0.813 (0.774-0.852), and 0.801 (0.753-0.848). Additionally, a good similarity between the nomogram-predicted and actual survival rates was demonstrated by the calibration plots(Figure 3), which showed that the spots were near to the 45-degree line.
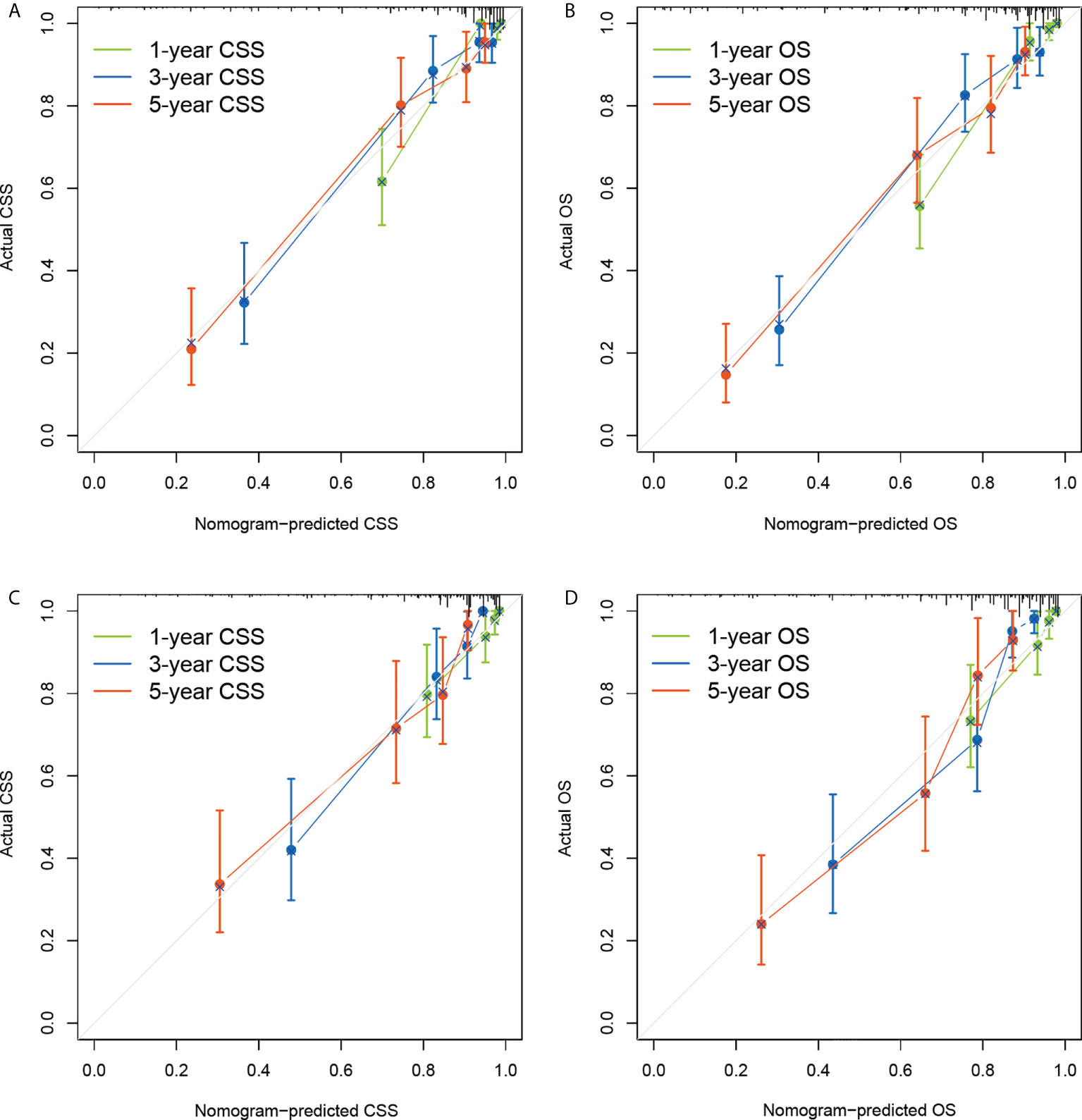
Figure 3 Calibration plots of the nomograms to predict CSS and OS of the derivation dataset (A, B) and external validation dataset (C, D). CSS, lung cancer-specific survival; OS, overall survival.
Comparison of the nomograms and the eighth edition TNM staging system
The comparisons between the nomograms and the TNM staging system were also performed (Table S5). Analysis of accuracy showed that the IDI or NRI for the 1-, 3-, and 5-year CSS or OS in derivation or validation dataset were all larger than 0 with all P<0.001, indicating a better prediction power of the nomograms compared with TNM staging system. The area under ROC curve of the nomograms was larger than TNM staging system for the 1-, 3-, and 5-year CSS or OS prediction in derivation or validation dataset (Figure 4). Furthermore, the DCA was applied to determine the clinical applicability. The DCA showed that our nomograms were better than the TNM staging system, as it added more net benefits than the TNM classification for nearly all threshold probabilities based on both the derivation cohort and validation cohort (Figure 5). The nomogram-calculated total points of the patients were divided into low- (CSS: <80; OS: <83), medium- (CSS: 80 to 133; OS: 83 to 135), and high-risk (CSS: >133; OS: >135) subgroups using X-tile software, and this classification method exhibited better discriminative power than the eighth edition of TNM staging system as shown in the Kaplan-Maier curve analysis (Figure 6).
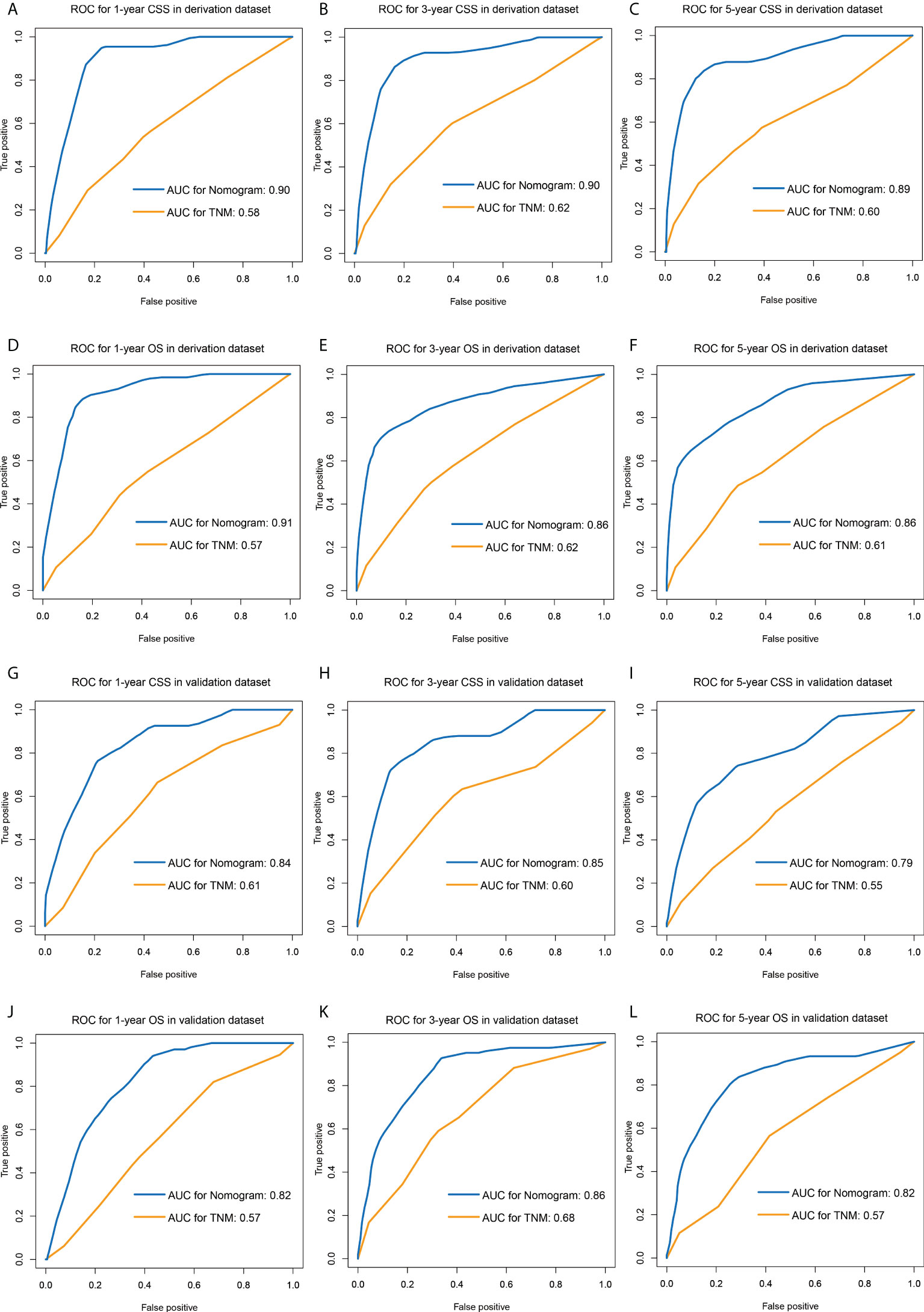
Figure 4 ROC curves of the nomograms and TNM staging system for predicting 1-, 3-, 5-year CSS in the derivation dataset (A–C) and external validation dataset (D–F), and 1-, 3-, 5-year OS in the derivation dataset (G–I) and external validation dataset (J–L). ROC, receiver operating characteristic; CSS, lung cancer-specific survival; OS, overall survival.
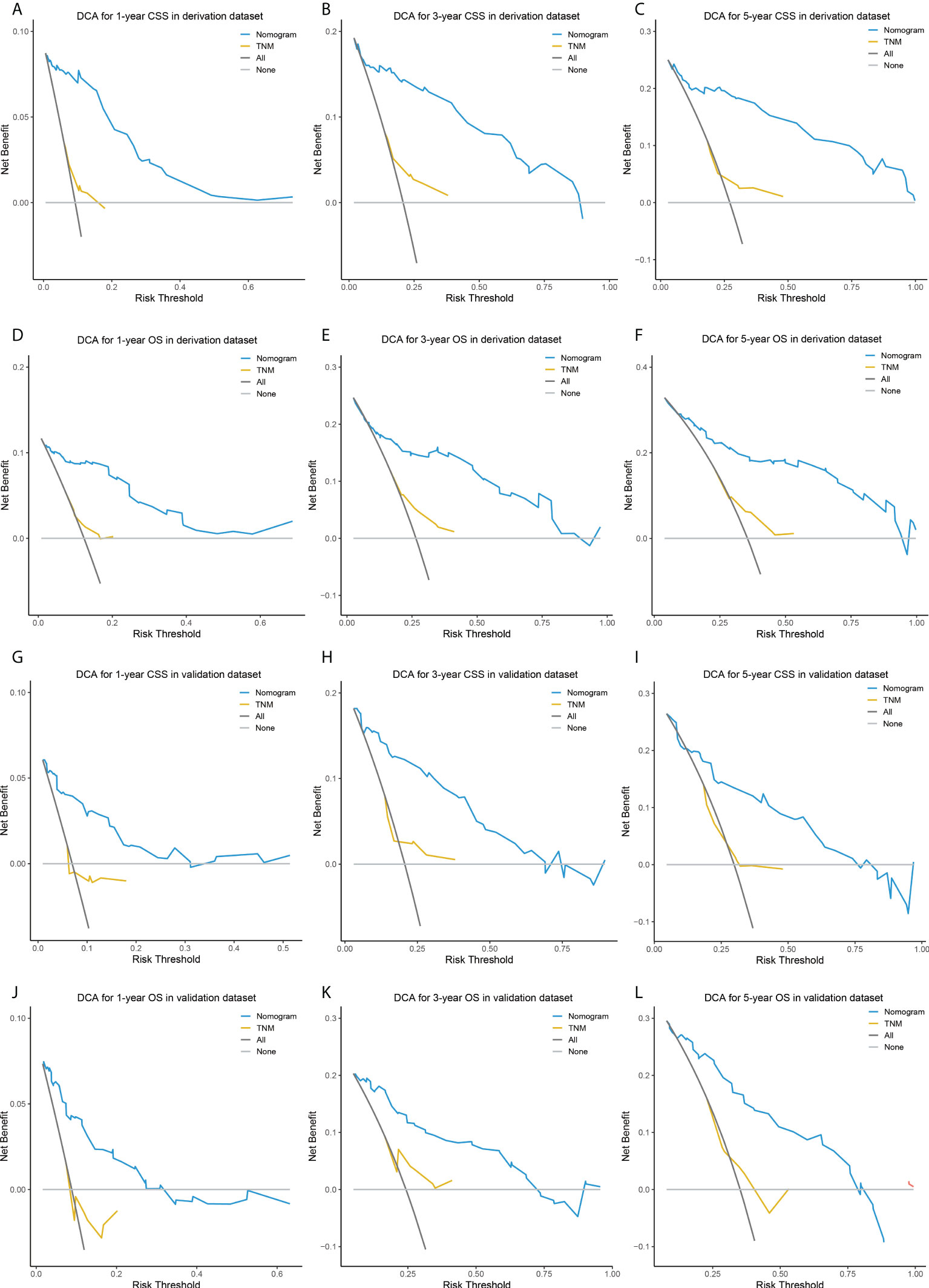
Figure 5 DCA of the nomograms and TNM staging system for predicting 1-, 3-, 5-year CSS in the derivation dataset (A–C) and external validation dataset (D–F), and 1-, 3-, 5-year OS in the derivation dataset (G–I) and external validation dataset (J–L). DCA, decision curve analysis; CSS, lung cancer-specific survival; OS, overall survival.
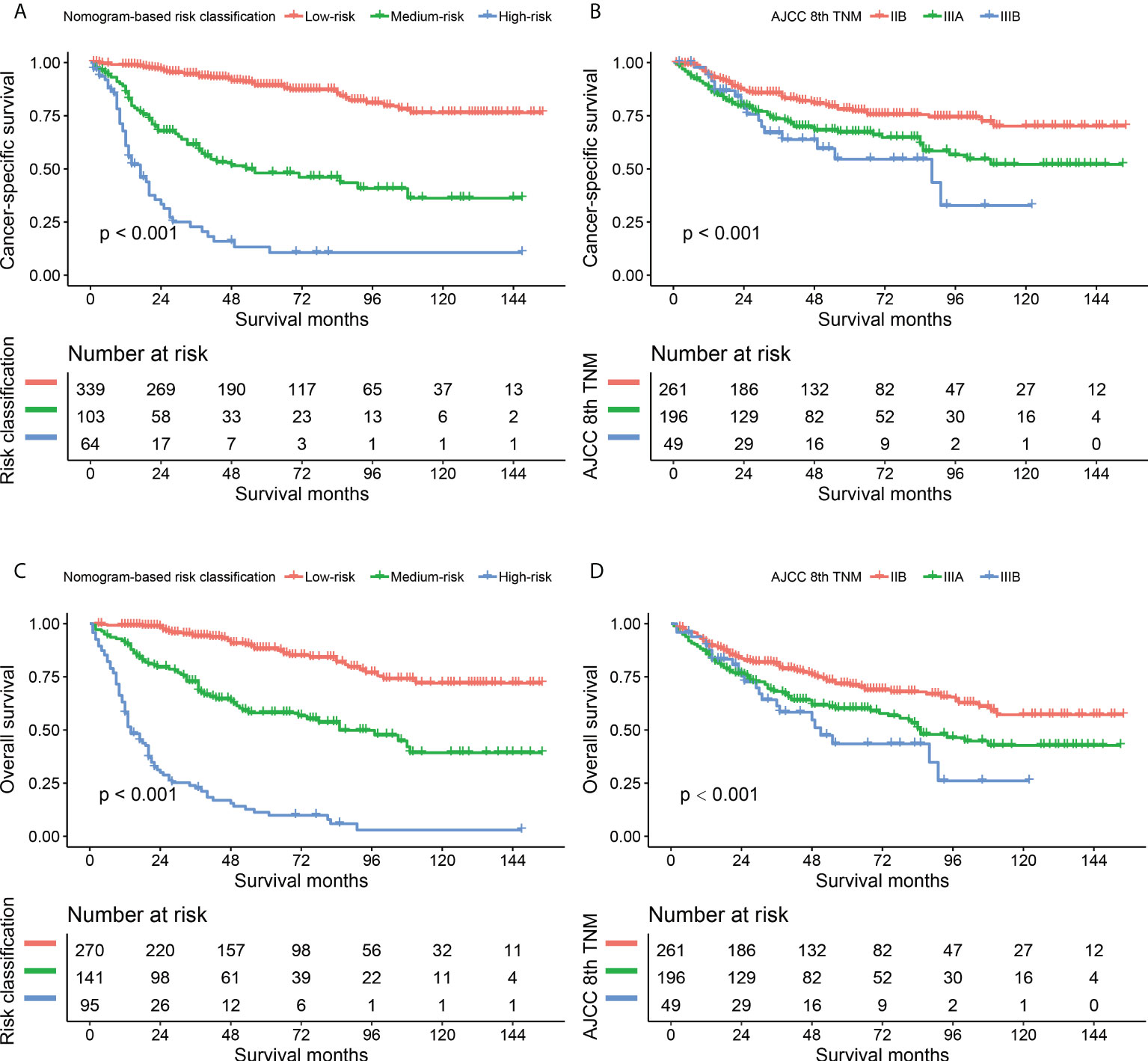
Figure 6 Kaplan-Maier curves comparing nomogram-based classification with 8th AJCC TNM staging system in CSS (A, B) and OS (C, D) prediction. CSS, cancer-specific survival; OS, overall survival.
Establishment of online models for convenient clinical use
Two online nomograms with interactive interface based on the multivariable Cox regression models were built (CSS: https://drboidedwater.shinyapps.io/DynNom-CSS-lungneuroendocrinecarcinoma/; OS: https://drboidedwater.shinyapps.io/DynNom-OS-lungneuroendocrinecarcinoma/). To facilitate the access of these two links, the quick response codes were demonstrated in Figure 2. The webservers may generate estimated survival rate and Kaplan-Meier curves by entering the covariates.
Discussion
LNETs constitute a unique clinical subgroup of primary pulmonary tumors. Due to their relatively low incidence, no specific staging system exists for LNETs. An exact and reasonable classification of the lymph node status is critical in the staging and prognosis evaluation of patients with LNCTs. In this study, LODDS was proved to be independently related to long-term clinical prognosis among patients with resectable LNETs. These results were robust to a series of sensitivity analyses. Second, we constructed two visualized and publicly accessible online nomograms, incorporating LODDS and routinely available demographic, staging and treatment information, to predict the survival probability for individual LNET patients. To our knowledge, the present study is the first to explore the prognostic value of LODDS for LNET based on a multi-center cohort with a relatively large sample size.
The involvement of regional lymph nodes in malignancies has been considered as one of the most important prognostic factors. The 8th version of the TNM staging system of NSCLC divided metastasis to intrapulmonary LNs and ipsilateral peribronchial and/or hilar LNs as N1 classification, and metastasis into ipsilateral mediastinal and/or subcarinal LNs into N2 classification without taking into account numbers of examined and metastatic lymph nodes (46). LODDS is a new LN ratio-based index and has been reported to be as an independent predictor in many malignancies such as rectal cancer (47), pancreatic cancer (48), gallbladder cancer (49), gastric cancer (50), and colon cancer (51). Recently, several studies were attempting to explore the prognostic value of LODDS for NSCLC. In 2020, we did a research and found that the high value of LODDS>-0.37 was independently related to worse CSS/OS in patients with node-positive lung squamous cell cancer (25). Dziedzic et al. (24) found that it is possible to discriminate NSCLC patients more effectively by using LODDS compared to conventional N classification. Deng et al. (23) found LODDS and lymph node ratio (LNR) staging schemes outperformed NPLN for predicting CSS/OS among patients with node-positive NSCLC. However, most previous studies only focused on NSCLC, and few reports have detected the predictive value of LODDS in LNETs.
In the present study, the high value of LODDS was associated with worse survival for N1/N2 stage patients with LNETs. However, LODDS must be used and calculated with caution, because the value of LODDS is influenced by the number of dissected LN. Therefore, sensitivity analyses were performed. For LNETs, the extent of LN management should conform to the International Association for the Study of Lung Cancer (IASLC) recommendations, which suggested examination of at least 6 nodes/stations, 3 of which should be mediastinal including the subcarinal station (52). Considering that the accurate value of LODDS was dependent on the adequate NDLN, we excluded patients with examined LN count less than 6 and found that LODDS could still serve as an independent predictor for LNETs. What's more, it is quite easy to break the integrity of the LN during surgery, especially when the LNs are adherent to one another or challenging to be removed from the dissected tissues (53). To avoid the potential bias led by fragmented LNs, we excluded patients with examined LN count of more than 20, and the results did not change. In summary, LODDS is the ratio-based LN staging system that combines NPLN and NDLN, which might be superior to some number-based LN assessment methods. Furthermore, in LODDS, the numerator and denominator are both added with a value of 0.5, eliminating the singularities caused by null data, therefore LODDS might be used to estimate survival of node-negative patients, as opposed to LNR.
The LODDS was not the only prognostic factor included in our nomograms. Similar to previous studies (54–56), age at diagnosis, sex, histotype, surgery, and radiotherapy were chosen as prognostic factors of CSS or OS. In this study, the nomograms showed that histotype contributed the most to the prognosis, which indicated tumor histotype is a crucial determinant of the clinical behavior of LNETs. Our nomograms indicated that LCNEC showed the worst prognosis followed by AC and TC. Growing evidence also suggests that high-grade LCNEC is biologically distinct from low-grade TC and intermediate-grade AC in view of clinical behavior, pathologic features, molecular alterations as well as possible precursor lesions (57). All-stage 5-year OS for LCNEC fluctuated between 13% and 57% (58). Different from LCNEC, TC and AC are more commonly found in younger patients without smoking histories. AC is significantly more aggressive than TC, with a higher frequency of nodal and distant metastases, and 5-year survival of 60%. In this study, we did not include SCLC patients, because SCLC is usually deemed as nonsurgical (59).
The optimal surgical choice for LNETs is controversial. The surgical type is associated with tumor site, tumor size, and precise assessment of preoperative biopsy specimen. Several studies reported that wedge resection might be correlated with the increased tumor recurrence rate especially in node-positive TC or AC (60, 61). Lobectomy is reported as superior to segmentectomy in terms of OS in some, but not in all pulmonary carcinoids (62–64). Similar to these studies, our study showed that lobectomy was superior to sublobectomy and pneumonectomy in the nomogram for CSS. Furthermore, there is an absence of high-quality evidence to show whether or not chemotherapy could provide clinical benefits for LNETs. Although our nomogram showed that chemotherapy might be associated with more favorable prognoses. However, the results need to be interpreted with caution. In the SEER database, patients without receiving chemotherapy and those with unknown information about adjuvant therapy were classified into one category, which might lead to potential bias. Until now, for pulmonary carcinoids, routine adjuvant therapy may only be considered in selected fit patients (AC, N2 stage) with a particularly high risk of relapse (65). Besides, Iyoda et al. (66) suggested that platinum-based adjuvant chemotherapy after surgery could help patients with LCNEC prevent recurrence.
Because LNET is a heterogeneous disease, each LNET patient requires an individualized and timely risk assessment, which allows for more precise therapeutic strategies and medical resource allocation decisions. In this study, we developed and validated two nomograms to predict prognosis in patients with LNET. Our nomograms based on LODDS were more accurate and obtained more clinical net benefit than the conventional AJCC/UICC TNM staging system. In summary, the online nomograms, composed of several easily obtained predictors, could be a simpler way to engage clinicians in death risks, patient counseling, and decision-making. To put it another way, LNET patients with poorer clinical results estimated by nomograms may require more aggressive therapy (39).
Several limitations of this study should be noted. First, the SEER database lacks some detailed data, such as smoking history, some promising molecular markers (e.g. Ki-67), imaging techniques used before surgery, histological and morphological data (e.g. mitotic rate), type of resection (R0, R1, or R2), and use of systemic therapies. Therefore, they could not be included as covariables in the multivariable Cox models. Second, information about comorbidities, and tumor recurrence is also not available in the database. Third, although we reclassified the TNM classification according to the eighth edition of AJCC/UICC TNM classification, the TNM staging system, which was derived from the SEER database’s collaborative stage system, is a combination of clinical and pathologic stages. Because of the distinction between clinical and pathologic stages, more subgroup analysis is required using a single clinical or pathologic staging system.
Conclusions
LODDS was found to be useful in predicting CSS/OS in LNET patients who underwent surgery. Webservers of nomograms including LODDS to assess CSS and OS were established. The well-executed nomograms may aid clinicians in providing reasonable, customized therapeutic strategies for LNET patients.
Data availability statement
The raw data supporting the conclusions of this article will be made available by the authors, without undue reservation.
Ethics statement
Ethical review and approval was not required for the study on human participants in accordance with the local legislation and institutional requirements. Written informed consent for participation was not required for this study in accordance with the national legislation and the institutional requirements.
Author contributions
Conception/Design: SW, YY, and ML. Collection and/or assembly of data: JW, YG and ML. Data analysis and interpretation: SW and QX. Manuscript writing: YY, XL, and QX. Final approval of manuscript: All authors. Funding support: XL. All authors contributed to the article and approved the submitted version.
Funding
This study was funded by Program of Shanghai Academic Research Leader (No. 21XD1402800) and Shanghai “Rising Stars of Medical Talent” Youth Development Program: Outstanding Youth Medical Talents.
Acknowledgments
The authors would like to thank all patients and staff who have participated in the SEER program.
Conflict of interest
The authors declare that the research was conducted in the absence of any commercial or financial relationships that could be construed as a potential conflict of interest.
Publisher’s note
All claims expressed in this article are solely those of the authors and do not necessarily represent those of their affiliated organizations, or those of the publisher, the editors and the reviewers. Any product that may be evaluated in this article, or claim that may be made by its manufacturer, is not guaranteed or endorsed by the publisher.
Supplementary material
The Supplementary Material for this article can be found online at: https://www.frontiersin.org/articles/10.3389/fimmu.2022.987881/full#supplementary-material
Abbreviations
AC, atypical carcinoid; AJCC, American Joint Committee on Cancer; CI, confidence interval; C-index, concordance index; CSS, cancer-specific survival; DCA, decision curve analysis; HR, hazard ratio; IASLC, International Association for the Study of Lung Cancer; IDI, integrated discrimination improvement; IQR, interquartile range; LCNEC, large cell neuroendocrine carcinoma; LN, lymph node; LNET, lung neuroendocrine tumor; LNR, lymph node ratio; LODDS, log odds of positive lymph nodes; NDLN, number of dissected lymph nodes; NPLN, number of positive lymph nodes; NRI, net reclassification improvement; NSCLC, non-small cell lung cancer; OS, overall survival; PRCDA, purchased/referred care delivery area; SCLC, small cell lung carcinoma; SD, standard deviation; SEER, Surveillance, Epidemiology, and End Results; TC, typical carcinoid; TNM, tumor-node-metastasis; UICC, Union for International Cancer Control; WHO, World Health Organization.
References
1. Hendifar AE, Marchevsky AM, Tuli R. Neuroendocrine tumors of the lung: Current challenges and advances in the diagnosis and management of well-differentiated disease. J Thorac Oncol (2017) 12(3):425–36. doi: 10.1016/j.jtho.2016.11.2222
2. Hemminki K, Li X. Incidence trends and risk factors of carcinoid tumors: a nationwide epidemiologic study from Sweden. Cancer (2001) 92(8):2204–10. doi: 10.1002/1097-0142(20011015)92:8<2204::AID-CNCR1564>3.0.CO;2-R
3. Quaedvlieg PF, Visser O, Lamers CB, Janssen-Heijen ML, Taal BG. Epidemiology and survival in patients with carcinoid disease in the netherlands. an epidemiological study with 2391 patients. Ann Oncol (2001) 12(9):1295–300. doi: 10.1023/A:1012272314550
4. Hauso O, Gustafsson BI, Kidd M, Waldum HL, Drozdov I, Chan AK, et al. Neuroendocrine tumor epidemiology: contrasting Norway and north America. Cancer (2008) 113(10):2655–64. doi: 10.1002/cncr.23883
5. Dasari A, Shen C, Halperin D, Zhao B, Zhou S, Xu Y, et al. Trends in the incidence, prevalence, and survival outcomes in patients with neuroendocrine tumors in the united states. JAMA Oncol (2017) 3(10):1335–42. doi: 10.1001/jamaoncol.2017.0589
6. Hallet J, Law CH, Cukier M, Saskin R, Liu N, Singh S. Exploring the rising incidence of neuroendocrine tumors: a population-based analysis of epidemiology, metastatic presentation, and outcomes. Cancer (2015) 121(4):589–97. doi: 10.1002/cncr.29099
7. Naalsund A, Rostad H, Strøm EH, Lund MB, Strand TE. Carcinoid lung tumors–incidence, treatment and outcomes: a population-based study. Eur J Cardio-thoracic Surg (2011) 39(4):565–9. doi: 10.1016/j.ejcts.2010.08.036
8. Travis WD, Brambilla E, Nicholson AG, Yatabe Y, Austin JHM, Beasley MB, et al. The 2015 world health organization classification of lung tumors: Impact of genetic, clinical and radiologic advances since the 2004 classification. J Thorac Oncol (2015) 10(9):1243–60. doi: 10.1097/JTO.0000000000000630
9. Kumar V, Yadavilli S, Kannan R. A review on RNAi therapy for NSCLC: Opportunities and challenges. Wiley Interdiscip Rev Nanomed Nanobiotechnol (2021) 13(2):e1677. doi: 10.1002/wnan.1677
10. Shah S, Gosain R, Groman A, Gosain R, Dasari A, Halfdanarson TR, et al. Incidence and survival outcomes in patients with lung neuroendocrine neoplasms in the united states. Cancers (Basel) (2021) 13(8):1753. doi: 10.3390/cancers13081753
11. Yoon JY, Sigel K, Martin J, Jordan R, Beasley MB, Smith C, et al. Evaluation of the prognostic significance of TNM staging guidelines in lung carcinoid tumors. J Thorac Oncol (2019) 14(2):184–92. doi: 10.1016/j.jtho.2018.10.166
12. Yeh YC, Chou TY. Pulmonary neuroendocrine tumors: study of 90 cases focusing on clinicopathological characteristics, immunophenotype, preoperative biopsy, and frozen section diagnoses. J Surg Oncol (2014) 109(3):280–6. doi: 10.1002/jso.23497
13. Robelin P, Hadoux J, Forestier J, Planchard D, Hervieu V, Berdelou A, et al. Characterization, prognosis, and treatment of patients with metastatic lung carcinoid tumors. J Thorac Oncol (2019) 14(6):993–1002. doi: 10.1016/j.jtho.2019.02.002
14. Filosso PL, Oliaro A, Ruffini E, Bora G, Lyberis P, Asioli S, et al. Outcome and prognostic factors in bronchial carcinoids: a single-center experience. J Thorac Oncol (2013) 8(10):1282–8. doi: 10.1097/JTO.0b013e31829f097a
15. Aydin E, Yazici U, Gulgosteren M, Agackiran Y, Kaya S, Gulhan E, et al. Long-term outcomes and prognostic factors of patients with surgically treated pulmonary carcinoid: our institutional experience with 104 patients. Eur J Cardio-thoracic Surg: Off J Eur Assoc Cardio-thoracic Surg (2011) 39(4):549–54. doi: 10.1016/j.ejcts.2010.08.010
16. Kodera Y, Yamamura Y, Shimizu Y, Torii A, Hirai T, Yasui K, et al. The number of metastatic lymph nodes: a promising prognostic determinant for gastric carcinoma in the latest edition of the TNM classification. J Am Coll Surgeon (1998) 187(6):597–603. doi: 10.1016/S1072-7515(98)00229-4
17. Sinn HP, Helmchen B, Wittekind CH. TNM classification of breast cancer: changes and comments on the 7th edition. Der Pathologe (2010) 31(5):361–6. doi: 10.1007/s00292-010-1307-0
18. Gallegos-Hernandez JF, Hernandez-Hernandez DM, Flores-Diaz R, Sierra-Santiesteban I, Pichardo-Romero P, Arias-Ceballos H, et al. The number of sentinel nodes identified as prognostic factor in oral epidermoid cancer. Oral Oncol (2005) 41(9):947–52. doi: 10.1016/j.oraloncology.2005.05.010
19. Yang L, Xiong Z, Xie Q, He W, Liu S, Kong P, et al. Prognostic value of total number of lymph nodes retrieved differs between left-sided colon cancer and right-sided colon cancer in stage III patients with colon cancer. BMC Canc (2018) 18(1):558. doi: 10.1186/s12885-018-4431-5
20. Chu X, Yang ZF. Impact on survival of the number of lymph nodes resected in patients with lymph node-negative gastric cancer. World J Surg Oncol (2015) 13:192. doi: 10.1186/s12957-015-0602-x
21. Tsim S, O’Dowd CA, Milroy R, Davidson S. Staging of non-small cell lung cancer (NSCLC): A review. Respir Med (2010) 104(12):1767–74. doi: 10.1016/j.rmed.2010.08.005
22. Lv P, Chen G, Zhang P. Log odds of positive lymph nodes are superior to other measures for evaluating the prognosis of non-small cell lung cancer. Thorac Canc (2014) 5(6):570–5. doi: 10.1111/1759-7714.12145
23. Deng W, Xu T, Wang Y, Xu Y, Yang P, Gomez D, et al. Log odds of positive lymph nodes may predict survival benefit in patients with node-positive non-small cell lung cancer. Lung Canc (2018) 122:60–6. doi: 10.1016/j.lungcan.2018.05.016
24. Dziedzic D, Piotr R, Langfort R, Orlowski T, Polish Lung Cancer Study G. Log odds of positive lymph nodes as a novel prognostic indicator in NSCLC staging. Surg Oncol (2017) 26(1):80–5. doi: 10.1016/j.suronc.2017.01.004
25. Yu Y, Zhang P, Yao R, Wang J, Wang P, Xue X, et al. Prognostic value of log odds of positive lymph nodes in node-positive lung squamous cell carcinoma patients after surgery: a SEER population-based study. Trans Lung Cancer Res (2020) 9(4):1285–301. doi: 10.21037/tlcr-20-193
26. Wang S, Yu Y, Xu W, Lv X, Zhang Y, Liu M. Dynamic nomograms combining n classification with ratio-based nodal classifications to predict long-term survival for patients with lung adenocarcinoma after surgery: a SEER population-based study. BMC Canc (2021) 21(1):653. doi: 10.1186/s12885-021-08410-6
27. Wang Q, Wang S, Sun Z, Cao M, Zhao X. Evaluation of log odds of positive lymph nodes in predicting the survival of patients with non-small cell lung cancer treated with neoadjuvant therapy and surgery: a SEER cohort-based study. BMC Canc (2022) 22(1):801. doi: 10.1186/s12885-022-09908-3
28. Huang Y, Liang C, Liu Z. Nomogram for predicting pulmonary hypertension in patients without pulmonary embolism. Radiology (2016) 280(1):327–8. doi: 10.1148/radiol.2016152584
29. Eisner BH, Goldfarb DS. A nomogram for the prediction of kidney stone recurrence. J Am Soc Nephrol (2014) 25(12):2685–7. doi: 10.1681/ASN.2014060631
30. Li W, Dong S, Wang H, Wu R, Wu H, Tang ZR, et al. Risk analysis of pulmonary metastasis of chondrosarcoma by establishing and validating a new clinical prediction model: a clinical study based on SEER database. BMC Musculoskelet Disord (2021) 22(1):529. doi: 10.1186/s12891-021-04414-2
31. Li W, Wang H, Dong S, Tang ZR, Chen L, Cai X, et al. Establishment and validation of a nomogram and web calculator for the risk of new vertebral compression fractures and cement leakage after percutaneous vertebroplasty in patients with osteoporotic vertebral compression fractures. Eur Spine J (2022) 31(5):1108–21. doi: 10.1007/s00586-021-07064-z
32. Wang S, Chen A, Zhu W, Feng D, Wei J, Li Q, et al. Characterization of fatty acid metabolism in lung adenocarcinoma. Front Genet (2022) 13:905508. doi: 10.3389/fgene.2022.905508
33. Yu Y, Huang R, Wang P, Wang S, Ling X, Zhang P, et al. Sublobectomy versus lobectomy for long-term survival outcomes of early-stage non-small cell lung cancer with a tumor size ≤2 cm accompanied by visceral pleural invasion: a SEER population-based study. J Thorac Dis (2020) 12(3):592–604. doi: 10.21037/jtd.2019.12.121
34. Collins GS, Reitsma JB, Altman DG, Moons KG. Transparent reporting of a multivariable prediction model for individual prognosis or diagnosis (TRIPOD): the TRIPOD statement. BMJ (Clinical Res ed) (2015) 350:g7594. doi: 10.1161/CIRCULATIONAHA.114.014508
35. Cronin KA, Ries LA, Edwards BK. The surveillance, epidemiology, and end results (SEER) program of the national cancer institute. Cancer (2014) 120 Suppl 23:3755–7. doi: 10.1002/cncr.29049
36. Goodyear MD, Krleza-Jeric K, Lemmens T. The declaration of Helsinki. BMJ (Clinical Res ed) (2007) 335(7621):624–5. doi: 10.1136/bmj.39339.610000.BE
37. Detterbeck FC. The eighth edition TNM stage classification for lung cancer: What does it mean on main street? J Thorac Cardiovasc Surg (2018) 155(1):356–9. doi: 10.1016/j.jtcvs.2017.08.138
38. Jackson AS, Rosenthal A, Cattoni M, Bograd AJ, Farivar AS, Aye RW, et al. Staging system for neuroendocrine tumors of the lung needs to incorporate histologic grade. Ann Thorac Surg (2020) 109(4):1009–18. doi: 10.1016/j.athoracsur.2019.09.053
39. Yi C, Dai J, Song N, Wu C, Zhang L, Zhu Y, et al. Improvement of pathological staging system for neuroendocrine tumors of the lung. Ann Transl Med (2021) 9(6):447. doi: 10.21037/atm-20-5910
40. Iyoda A, Makino T, Koezuka S, Otsuka H, Hata Y. Treatment options for patients with large cell neuroendocrine carcinoma of the lung. Gen Thorac Cardiovasc Surg (2014) 62(6):351–6. doi: 10.1007/s11748-014-0379-9
41. Iyoda A, Azuma Y, Sano A. Neuroendocrine tumors of the lung: clinicopathological and molecular features. Surg Today (2020) 50(12):1578–84. doi: 10.1007/s00595-020-01988-7
42. Raman V, Jawitz OK, Yang CJ, Voigt SL, Tong BC, D’Amico TA, et al. Outcomes for surgery in Large cell lung neuroendocrine cancer. J Thorac Oncol (2019) 14(12):2143–51. doi: 10.1016/j.jtho.2019.09.005
43. Camp RL, Dolled-Filhart M, Rimm DL. X-Tile: a new bio-informatics tool for biomarker assessment and outcome-based cut-point optimization. Clin Cancer Res (2004) 10(21):7252–9. doi: 10.1158/1078-0432.CCR-04-0713
44. Jungk C, Reinhardt A, Warta R, Capper D, Deimling AV, Herold-Mende C, et al. Extent of resection, MGMT promoter methylation status and tumor location independently predict progression-free survival in adult sporadic pilocytic astrocytoma. Cancers (Basel) (2019) 11(8):1072. doi: 10.3390/cancers11081072
45. Vickers AJ, Elkin EB. Decision curve analysis: a novel method for evaluating prediction models. Med Dec Mak (2006) 26(6):565–74. doi: 10.1177/0272989X06295361
46. Taylor MD, LaPar DJ, Thomas CJ, Persinger M, Stelow EB, Kozower BD, et al. Lymph node ratio predicts recurrence and survival after R0 resection for non-small cell lung cancer. Ann Thorac Surg (2013) 96(4):1163–70. doi: 10.1016/j.athoracsur.2013.04.031
47. Huang B, Ni M, Chen C, Cai G, Cai S. LODDS is superior to lymph node ratio for the prognosis of node-positive rectal cancer patients treated with preoperative radiotherapy. Tumori (2017) 103(1):87–92. doi: 10.5301/tj.5000560
48. Ramacciato G, Nigri G, Petrucciani N, Pinna AD, Ravaioli M, Jovine E, et al. Prognostic role of nodal ratio, LODDS, pN in patients with pancreatic cancer with venous involvement. BMC Surg (2017) 17(1):109. doi: 10.1186/s12893-017-0311-1
49. Xiao Z, Shi Z, Hu L, Gao Y, Zhao J, Liu Y, et al. A new nomogram from the SEER database for predicting the prognosis of gallbladder cancer patients after surgery. Ann Trans Med (2019) 7(23):738. doi: 10.21037/atm.2019.11.112
50. Liu H, Deng J, Zhang R, Hao X, Jiao X, Liang H. The RML of lymph node metastasis was superior to the LODDS for evaluating the prognosis of gastric cancer. Int J Surg (London England) (2013) 11(5):419–24. doi: 10.1016/j.ijsu.2013.03.009
51. Fortea-Sanchis C, Martínez-Ramos D, Escrig-Sos J. The lymph node status as a prognostic factor in colon cancer: comparative population study of classifications using the logarithm of the ratio between metastatic and nonmetastatic nodes (LODDS) versus the pN-TNM classification and ganglion ratio systems. BMC Canc (2018) 18(1):1208. doi: 10.1186/s12885-018-5048-4
52. Caplin ME, Baudin E, Ferolla P, Filosso P, Garcia-Yuste M, Lim E, et al. Pulmonary neuroendocrine (carcinoid) tumors: European neuroendocrine tumor society expert consensus and recommendations for best practice for typical and atypical pulmonary carcinoids. Ann Oncol (2015) 26(8):1604–20. doi: 10.1093/annonc/mdv041
53. Dai J, Liu M, Yang Y, Li Q, Song N, Rocco G, et al. Optimal lymph node examination and adjuvant chemotherapy for stage I lung cancer. J Thorac Oncol (2019) 14(7):1277–85. doi: 10.1016/j.jtho.2019.03.027
54. He Y, Zhao F, Han Q, Zhou Y, Zhao S. Prognostic nomogram for predicting long-term cancer-specific survival in patients with lung carcinoid tumors. BMC Canc (2021) 21(1):141. doi: 10.1186/s12885-021-07832-6
55. Dong S, Liang J, Zhai W, Yu Z. Development and validation of an individualized nomogram for predicting overall survival in patients with typical lung carcinoid tumors. Am J Clin Oncol (2020) 43(9):607–14. doi: 10.1097/COC.0000000000000715
56. Chen X, Pang Z, Wang Y, Bie F, Zeng Y, Wang G, et al. The role of surgery for atypical bronchopulmonary carcinoid tumor: Development and validation of a model based on surveillance, epidemiology, and end results (SEER) database. Lung Canc (2020) 139:94–102. doi: 10.1016/j.lungcan.2019.11.006
57. Hiroshima K, Mino-Kenudson M. Update on large cell neuroendocrine carcinoma. Trans Lung Cancer Res (2017) 6(5):530–9. doi: 10.21037/tlcr.2017.06.12
58. Rossi G, Cavazza A, Marchioni A, Longo L, Migaldi M, Sartori G, et al. Role of chemotherapy and the receptor tyrosine kinases KIT, PDGFRalpha, PDGFRbeta, and met in large-cell neuroendocrine carcinoma of the lung. J Clin Oncol (2005) 23(34):8774–85. doi: 10.1200/JCO.2005.02.8233
59. Lim E, Goldstraw P, Nicholson AG, Travis WD, Jett JR, Ferolla P, et al. Proceedings of the IASLC international workshop on advances in pulmonary neuroendocrine tumors 2007. J Thorac Oncol (2008) 3(10):1194–201. doi: 10.1097/JTO.0b013e3181861d7b
60. García-Yuste M, Matilla JM, Cañizares MA, Molins L, Guijarro R. Surgical treatment of low and intermediate grade lung net. J Thorac Dis (2017) 9(Suppl 15):S1435–s41. doi: 10.21037/jtd.2017.09.83
61. García-Yuste M, Matilla JM, Cueto A, Paniagua JM, Ramos G, Cañizares MA, et al. Typical and atypical carcinoid tumours: analysis of the experience of the Spanish multi-centric study of neuroendocrine tumours of the lung. Eur J Cardio-thoracic Surg (2007) 31(2):192–7. doi: 10.1016/j.ejcts.2006.11.031
62. Filosso PL, Rena O, Guerrera F, Moreno Casado P, Sagan D, Raveglia F, et al. Clinical management of atypical carcinoid and large-cell neuroendocrine carcinoma: a multicentre study on behalf of the European association of thoracic surgeons (ESTS) neuroendocrine tumours of the lung working group†. Eur J Cardio-thoracic Surg (2015) 48(1):55–64. doi: 10.1093/ejcts/ezu404
63. Steuer CE, Behera M, Kim S, Chen Z, Saba NF, Pillai RN, et al. Atypical carcinoid tumor of the lung: a surveillance, epidemiology, and end results database analysis. J Thorac Oncol (2015) 10(3):479–85. doi: 10.1097/JTO.0000000000000419
64. Marciello F, Mercier O, Ferolla P, Scoazec JY, Filosso PL, Chapelier A, et al. Natural history of localized and locally advanced atypical lung carcinoids after complete resection: A joined French-Italian retrospective multicenter study. Neuroendocrinology (2018) 106(3):264–73. doi: 10.1159/000480015
65. Baudin E, Caplin M, Garcia-Carbonero R, Fazio N, Ferolla P, Filosso PL, et al. Lung and thymic carcinoids: ESMO clinical practice guidelines for diagnosis, treatment and follow-up(☆). Ann Oncol (2021) 32(4):439–51. doi: 10.1016/j.annonc.2021.01.003
Keywords: lung neuroendocrine tumor, log odds of positive lymph nodes, predictor, survival, nomogram
Citation: Wang S, Wei J, Guo Y, Xu Q, Lv X, Yu Y and Liu M (2022) Construction and validation of nomograms based on the log odds of positive lymph nodes to predict the prognosis of lung neuroendocrine tumors. Front. Immunol. 13:987881. doi: 10.3389/fimmu.2022.987881
Received: 06 July 2022; Accepted: 11 August 2022;
Published: 23 September 2022.
Edited by:
Kathleen Wilkie, Ryerson University, CanadaReviewed by:
Albino Eccher, Integrated University Hospital Verona, ItalyWenle Li, Xiamen University, China
Jie Tian, Shanghai Jiao Tong University, China
Haosheng Wang, Nanjing Drum Tower Hospital, China
Copyright © 2022 Wang, Wei, Guo, Xu, Lv, Yu and Liu. This is an open-access article distributed under the terms of the Creative Commons Attribution License (CC BY). The use, distribution or reproduction in other forums is permitted, provided the original author(s) and the copyright owner(s) are credited and that the original publication in this journal is cited, in accordance with accepted academic practice. No use, distribution or reproduction is permitted which does not comply with these terms.
*Correspondence: Meiyun Liu, bGl1bWVpeXVuMEAxMjYuY29t; Yue Yu, MTUwMjE1NjcxNzBAMTYzLmNvbQ==
†These authors have contributed equally to this work and share first authorship