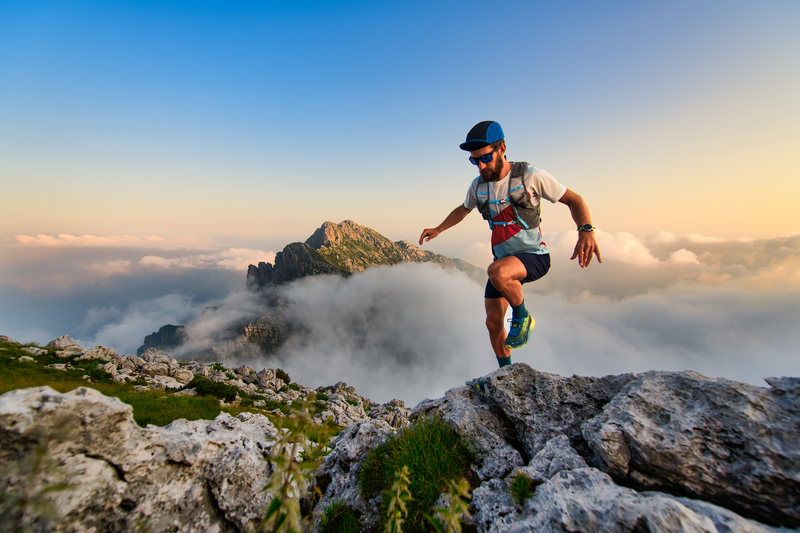
94% of researchers rate our articles as excellent or good
Learn more about the work of our research integrity team to safeguard the quality of each article we publish.
Find out more
REVIEW article
Front. Immunol. , 23 September 2022
Sec. Cancer Immunity and Immunotherapy
Volume 13 - 2022 | https://doi.org/10.3389/fimmu.2022.985863
This article is part of the Research Topic Unveiling the Tumor Microenvironment by Machine Learning to Develop New Immunotherapeutic Strategies View all 36 articles
Evaluation of tumor-host interaction and intratumoral heterogeneity in the tumor microenvironment (TME) is gaining increasing attention in modern cancer therapies because it can reveal unique information about the tumor status. As tumor-associated macrophages (TAMs) are the major immune cells infiltrating in TME, a better understanding of TAMs could help us further elucidate the cellular and molecular mechanisms responsible for cancer development. However, the high-dimensional and heterogeneous data in biology limit the extensive integrative analysis of cancer research. Machine learning algorithms are particularly suitable for oncology data analysis due to their flexibility and scalability to analyze diverse data types and strong computation power to learn underlying patterns from massive data sets. With the application of machine learning in analyzing TME, especially TAM’s traceable status, we could better understand the role of TAMs in tumor biology. Furthermore, we envision that the promotion of machine learning in this field could revolutionize tumor diagnosis, treatment stratification, and survival predictions in cancer research. In this article, we described key terms and concepts of machine learning, reviewed the applications of common methods in TAMs, and highlighted the challenges and future direction for TAMs in machine learning.
The tumor microenvironment (TME) is a complex system consisting of various components that would shape tumorigenesis, progression and metastasis. In addition to cancer cells, numerous innate immune cells reside within the TME, for instance, macrophages, dendritic cells, neutrophils, myeloid-derived suppressor cells, etc. In the complex environment, tumor-associated macrophages (TAMs), the major immune cells infiltrating tumors, can orchestrate various aspects of tumor biology, such as tumor initiation, progression, metastasis, and even anti-tumor immunosuppression. As crucial drivers in fostering tumor progression, TAMs are standing out as promising targets for diagnosis and new treatments in malignant tumors.
Machine Learning (ML) is a group of data-analytical methods to build predictive models by summarizing past empirical or theoretical literature. Deep learning (DL) is considered an evolution of machine learning. It uses a programmable artificial neural network (ANN) which is inspired by a biological nervous system to make accurate decisions. Recently, ML, DL, in particular, has exhibited a remarkable development with the support of the rapid increase in the storage capacity and processing power of computers. In the era of big data, ML methods have come to attention as their extraordinary ability to process large and heterogeneous data sets in complex biological systems. As P4 (Predictive, Preventive, Personalized, and Participatory) and precision medicine are emerging and gaining traction (1), ML has become integral to modern biological research for its ability to solve challenges not well addressed by traditional methods. There have been many applications of ML in medical research ranging from cancer classification, subtyping, new biomarker discovery, and drug discovery (2–5). Considering the crucial role of TAMs in TME and tumor biology, ML has been widely employed in TAMs-related studies and has achieved successful outcomes.
This review is intended for readers with little knowledge of ML algorithms. Firstly, we briefly review the origins, types, and functions of TAMs. Secondly, we introduce the basic principles and key concepts needed to understand how ML methods could be applied and utilized in cancer research. Thirdly, we discuss the methods and applications at the intersection of ML and TME, especially TAMs. In the end, we highlight the current challenges in ML that need to be addressed, as well as the future directions that could be used to fully realize the potential applications in cancer therapy.
TAMs comprise almost 50% of immune cells infiltrating tumors. They are highly heterogeneous cells that can be divided into two main origins: bone-marrow-derived macrophages (BMDMs) developing from hematopoietic stem cells and tissue-resident macrophages (TRMs) from progenitors seeded into tissues during embryonic development. For a long time, BMDMs have been considered the main effectors in TAMs, but nowadays, TRMs have emerged as an inseparable and essential component in TME (6).
In a simplified view, there are two distinct populations of polarized macrophages, the classical M1 [upon lipopolysaccharide (LPS) and IFNG stimulation] and the alternative M2 (upon IL4 or IL13 stimulation) phenotypes macrophages. Macrophages undergo polarization and get activated in multiple processes during physiological and disease processes (7, 8). M1 and M2 macrophages have different markers, including CD surface receptors, cytokines, chemokines, transcription profiles, etc. (Table 1). We have listed the characterized biomarkers, CDs, and cytokines for TAMs identification. M2 macrophages can be further classified into different subtypes, namely M2a (mediated by IL4 and IL13), M2b (mediated by immune complexes (IC) with LPS or IL1R ligand), M2c (mediated by TGFB1, IL10, and glucocorticoids), and M2d (activated by tumor-associated factors, the major part of TAMs) (44, 45). In contrast to proinflammatory, antibacterial, and anti-angiogenic M1 macrophages, M2 macrophages suppress inflammation, facilitate tissue repair, remodeling, angiogenesis, and retain homeostasis under physiological conditions (46, 47).
In general, TAMs contain M2 and small populations of M1 cells (48). However, the distinction between the M1 and M2 states is less clear in TME since TAMs probably display phenotypes anywhere in between these two extremes. Moreover, the phenotype of TAMs dynamically changes with the development and progression of tumors. Each macrophage in TME might show anti- or pro-tumorigenic properties to form a plastic and heterogeneous tumor-promoting totality in response to diverse microenvironmental signals (a mixed M1–M2 phenotype). In a word, the M1 or M2 only phenotype is too simple to elucidate the intricate roles of TAMs in the TME (49–53).
Macrophages are considered essential components in immune defense and immune sentinels combating tumor growth; however, accumulated evidence supports a new tumor-promoting role of macrophages as well. Different from the basic functions of phagocytizing pathogens and apoptotic cell debris, TAMs are equipped to execute a broad repertoire of pro-tumorigenic functions as heterogeneous effectors (Figure 1).
Figure 1 Roles of TAMs in tumor progression. Overview of TAMs in tumor progression. TAMs can derive from BMDMs and TRMs. TAMs provide a niche for tumor initiation and development, participate in angiogenesis, promote tumor metastasis, and enhance resistance to chemotherapy, radiotherapy and immunotherapy. (Created with BioRender.com).
TAMs profusely infiltrate TME with the ability to suppress anti-tumoral immune surveillance. Accumulating evidence has suggested that TAMs can express a variety of immunosuppressive chemokines and factors which promote tumor cell proliferation and survival, including platelet-derived growth factor (PDGF), epithelial growth factor (EGF), and transforming growth factor beta 1 (TGFB1) (54, 55). The abovementioned chemokines and factors lead to immune cell–cell interactions as well. For instance, TAMs can inhibit anti-tumor immunity by restraining antigen presentation and blocking T cells function, in which case T cells lose their capacity in recognizing and even killing tumor cells (45). Usually, activated cytotoxic T lymphocytes (CTLs) can attack cancer cells to suppress tumor growth, while TAMs express immunosuppressive cytokines, chemokines, and growth factors like IL10 and TGFB1 to make CTLs hyporesponsive (6). As a distinct T-cell subpopulation, regulatory T cells (Tregs) are actively engaged in the maintenance of immunological self-tolerance (56). IL10 and TGFB1 from TAMs can also induce Tregs-mediated immunosuppression (57). Besides, TAMs are able to recruit Tregs via CCL22 production, which further suppresses the antitumor immune response of T-cells and fosters tumor growth (58). Moreover, it is worth noting that cancer cells can strongly induce TAMs into pro-tumorigenic phenotype by secreting colony-stimulated factor 1, mucins and exosomes (59–61). To sum up, all these factors work together and make the TME a hospitable site.
Angiogenesis can be briefly defined as the formation of new capillaries from pre-existing blood vessels. It is generally accepted that tumor growth largely depends on angiogenesis since new vessels can supply fresh oxygen and nutrients as well as remove wastes and metabolites. Furthermore, angiogenesis is a vital event in hematogenous metastasis (62). Angiogenesis is activated when pro-angiogenic factors predominate over anti-angiogenic factors (63). As shown in Table 1, TAMs can produce diverse pro-angiogenic molecules (VEGF family, PDFG, TGFB1, etc.) and matrix metalloproteinases (MMP) to facilitate angiogenesis. In particular, developing tumors consume oxygen supply rapidly and tend to create an oxygen deficiency condition (hypoxia). It has been increasingly recognized that TAMs massively infiltrate hypoxic regions in tumors and hypoxic macrophages achieve a pro-angiogenic response by directly upregulating the abovementioned pro-angiogenic molecules through hypoxia-inducible factor-1 alpha (HIF1A) (64–67).
TAMs demonstrate lots of essential functions in tumor biology. In tumor metastasis, it is still a puzzling question how TAMs facilitate tumor spread specifically, though TAMs get involved in almost every process of metastasis. Herein, we provide a quick summary of the fundamental mechanics. First, TAMs within the TME can enhance tumor cell migration and invasion, thereby enabling the escape of tumor cell from the confines of the basement membrane into the surrounding tissues. Second, TAMs are associated with tumor angiogenesis, which, as was previously mentioned, results in tumor intravasation and vasculature-based tumor spread (68). Third, in the immunosuppressive TME, cancer cells can escape from being killed by T cells and prolong cell survival, which make it easier to spread to farther tissues and organs (69). It should be highlighted that tumor metastasis is a process that starts at a very early stage rather than a late event initiated and shaped in advanced cancers. Distant organs are conducive to the survival and outgrowth of primary cancer cells before their arrival. Those ‘primed’ sites are known as ‘pre-metastatic niches’ (PMNs) (70) and special attention has been given to the key role of TAMs in PMNs from clinical evidence (71). Upon the induction of many tumor-secreted factors, TAMs are recruited into the blood and then gather at the pre-metastatic sites (70, 72–74). Meanwhile, TRMs stemming from yolk sac progenitors, like cerebral microglia, liver Kupffer cells, pulmonary alveolar macrophages, and osteoclasts, have been resident in the distant sites before tumorigenesis and get involved in orchestrating PMNs formation following diverse stimulation as well. These macrophages guide circulating tumor cells (CTCs) into the PMNs through enhancing the expression of chemokines and remodeling the extracellular matrix (ECM) into more tumor-favorable structures (75).
Emerging cancer research depicts that a high proportion of TAMs infiltration in tumor samples is often associated with shortened survival and poor prognosis in many tumors (76–79). Furthermore, TAMs infiltration is thought to offset therapeutic response to radiotherapy, chemotherapy and targeted therapy, even leading to treatment failure (80, 81). Regarding underlying mechanisms, TAMs can reduce the efficacy of radiotherapy by triggering the anti-apoptotic programs in cancer cells that are resistant to radiotherapy. They also secrete a variety of cytokines and survival factors to mediate the resistance of the solid tumor to many chemotherapy drugs, including IL6 and milk-fat globule-epidermal growth factor-VIII (82, 83). Programmed death ligand 1 (PD-L1), which is thought to be carried by TAMs and is upregulated in response to stimulation of TME-derived factors, has been linked to immune exhaustion via the checkpoint ligand/receptor interaction. However, existing studies do not depict a comprehensive picture since another study comes to a contrary conclusion that PD-L1 expression on TAMs, instead of cancer cells, is positively associated with patients’ overall survival (84). Thus, further studies addressing the precise mechanisms involved are urgently needed.
Considering all these functions of TAMs, it is essential to comprehend heterogeneous TAMs and their roles in tumor biology to create and enhance more potent treatments. To date, various molecular strategies against TAMs are currently in preclinical or clinical trials, trying to overcome the knotty problem of immune suppression, such as TAMs recruitment, TAMs depletion and TAMs reprogramming (85).
The term machine learning was first coined in the 1950s by Arthur Samuel, a computer scientist at IBM (86). Since then, ML has evolved considerably and now is playing a critical role in modern medical science. ML is a subdivision of artificial intelligence and can be briefly defined as enabling algorithms to make accurate predictions based on prior experiences (87). The boundary between conventional statistical techniques and ML is obscure, whilst some terms in ML have similar functions to statistical methods. Some conventional statistical techniques, such as ridge regression can be combined with ML algorithms for prediction (88). One key distinction between ML and traditional statistical methods is that conventional statistic methods focus on the relationship between variables (89). However, ML contributes to identifying patterns from massive data and then performing predictions. Moreover, ML aims to solve more complicated problems, often dealing with high dimensional variables with the technique of feature selection, pattern analysis and dimensionality reduction. As a result, it extends and supplements existing statistical methods by offering tools and algorithms to decipher patterns in enormous, intricate and heterogeneous data sets. Common terminologies and explanations in ML can be seen in Table 2.
In oncology studies, ML can analyze large-scale data in different format and combine them into predictions for tumor staging, cancer susceptibility, tumor recurrence, and patient survival (90). The process of ML is to extract knowledge from massive data sets, identify the underlying patterns, build predictive models, and finally make predictions on unseen data. A basic explanation of ML in cancer research can be achieved by considering the example of tumor recurrence prediction. Features from heterogeneous sources of data (clinical, imaging and genomic) are extracted by the ML algorithm. ML algorithm identify the combinations of specific features and tumor recurrence risk, and then build a prediction model. After that, when presented with a new case, the algorithm could provide the likelihood of recurrence for the new case.
ML techniques can be generally categorized into three main groups based on whether the labels are required in the training data (91). Common categories of supervised and unsupervised learning can be found in Table 3.
The term ‘supervised’ refers to the technique where a model is supplied with labels, which are desired outcomes of the learning target (e.g., correct segmentation or classification results) (92). Generally, supervised learning is used to build a model to predict or categorize future events. It primarily focuses on classification (e.g., classifying benign or malignant tumors) and regression (calculating the risk of tumor relapse, estimating individualized disease-free survival, or predicting the length of patient life) (88).
Unsupervised learning is used when the input data has no labels. Hence, it learns the relationship between variables and uncovers patterns in unlabeled data. Supervised learning primarily addresses classification and regression issues, while unsupervised learning focuses more on dimensionality reduction and clustering (88). Clustering refers to identifying groups of similar cases within a data set based on some specific features; dimensionality reduction is used to reduce the complexity and heterogeneity of features extracted from massive biomedical data sets.
Semi-supervised learning combines supervised and unsupervised ML. It can be helpful when only a tiny fraction of the data is labeled, or the labels on the input data are incomplete (93). A lack of sufficient labeled data frequently occurs in medical contexts because, given the complexity and variability of biomedical data, labeling information (e.g., correctly delineating the target in auto-segmentation) can be labor- and time-consuming. From this respective, semi-supervised learning can improve the efficiency and accuracy of information extraction for large data sets.
ML workflow usually starts with data acquisition and pre-processing. Data sets are typically split into training, validation, and evaluation sets. The predictive model is constructed on the basis of the training set and tuned by the validation set; finally, the model performance is assessed by the held-out evaluation set (89). In practice, the training set usually accounts for a larger fraction of the data (70%), whereas validation and evaluation sets usually make up 15%, respectively.
The prerequisites of ML success are a sufficient number of samples and high-quality data. To make the most of ML, enough training data size should be ensured to extract more generic features from the whole data set without over-emphasizing the impact from a few certain samples. Besides, the data quality should be checked to ensure input data’s appropriateness, reproducibility, and versatility. Specifically, for supervised learning, the correctness of the ground truth labels is also quite essential. Incorrect labels can significantly downgrade the model performance and are difficult to detect during training (86).
The proper performance of the model relies heavily on features across sample sets, and model refinement can be achieved using the technique of feature selection. Inappropriate feature selection would undermine the training performance by straining computational resources, including time and memory. For ML application in TAMs, thousands of features can be used to predict the output variables (94), e.g., cell morphology, the molecular feature of TAMs, immune-related gene-based novel subtypes, patient characteristics, tumor infiltration, etc. After feature selection, ML would search for the optimal parameters and translate the features into accurate predictions. The parameters are created through a complicated calculation process.
After that, a validation set is also needed to optimize the parameters of the algorithm. In validation, a preliminary estimate of the model’s generalizability and accuracy is obtained; errors can be detected and corrected in this phase, and the process is then repeated (95). In other words, validation serves as a supplemental role in identifying the errors in a model in an early phase.
The input data is usually partitioned into k subsets of equal size. A single subset is retained as the validation set, and the remaining k-1 subsets are used as training data. The process of training and validation will continue until there is no further improvement in model performance.
The evaluation data is used to assess the performance of the final model on samples outside the input data set (training and validation set). This process aims to estimate the model performance in the real-world. The evaluation set should be utilized at the very end of the research, avoiding the model being tuned to fit the evaluation set (96). The performance of a specific model relies on many factors, such as the data size and quality of training data, as mentioned above. The complexity and the relationship between the input and output variables, as well as the computational resources such as available training time and memory, all play essential roles in achieving high model performance (94).
In this section, we are going to introduce the most common utilized ML algorithms applied in cancer research, especially, TAMs. We also compared the advantages and disadvantages of different algorithms in Table 4 (97–101). Since the combination of ML and TAMs is an emerging cross-cutting research field, most studies were published in the last five years. All the matches were reviewed for suitability and significance for this review. Table 5 depicts the publications we found most pertinent to our topic. Cancer type, sample size, research purpose, as well as the ML applications are presented in the table.
Dimensionality reduction refers to techniques that transform data in high dimensions into a lower-dimensional form while preserving the relationships between the data points as much as possible. In a nutshell, it is a data preparation technique used for downsizing the input variables and performed before modeling. By far, Principal Component Analysis (PCA) is the most popular multidimensional data analysis technique (126). It reduces the dimensionality by eliminating less important components to omit the redundant dimensions and focusing only on the most important components that could best explain the heterogeneity in the data (Figure 2A). Other dimensionality reduction algorithms include t-distributed stochastic neighbor embedding and uniform manifold approximation and projection.
Figure 2 Basic principles of standard ML algorithms. (A) PCA reduces the dimensionality of a data set consisting of plenty of interrelated variables. (A) illustrates a series of data points viewed from another angle with approximately the same value on that dimension. It shows that the distinction between the data points can be represented by a principal component. (B) Regression analysis determines the relationship between factors and disease outcomes or identifies relevant prognostic factors for diseases. (B) illustrates regression estimating a mathematical formula that relates input variables to the output variable. (C) SVM generates a hyperplane in higher-dimensional feature space and maximizes the margin of error to select the best hyperplane. The best hyperplane would serve as a decision boundary for classification. (D) RF model ensembles a large number of small decision trees. Each tree is capable of making an individual prediction. (E) Neural networks tend to resemble the connections of neurons and synapses in human brain. The input data is assigned initial weights and transferred to output layers for classification. Hidden layers would tune the initial wrights to minimize the neural network’s prediction error.
PCA is primarily applied to problems where there are a large number of features, which are referred to as high-dimensional problems (127). Generally, there are many important applications of PCA in cancer research because the input variables in oncology data are complex and massive. For example, PCA is used to extract principal components as signature score to calculate the patients’ risk scores based on meaningful macrophage-related genes (105, 106). Zhang et al. performed PCA on 487 patients to reduce the feature dimensions and clearly distinguished high-risk and low-risk patients (107). Autoencoder in deep learning neural networks is another method to perform dimensionality reduction. Encoder is the part of the model prior to the bottleneck. It aims to compress the data dimension to a bottleneck layer that is much smaller than the initial input data. Shen et al. developed a deep learning model through self-supervised feature representation learning to characterize immune infiltration from transcriptome (116). The developed model was used to distill expression signatures of the transcriptome in brain tumor samples. The application of PCA in TAMs research could potentially be promising in enhancing predictive accuracy when input variables and their inter-connections are remarkably complicated.
Regression analysis is a method to mathematically describe the relationships between the outcome of interest (e.g., patient survival or relapse risk) and one or more features, also termed as variables (Figure 2B) (128). It answers the questions: Which variable is the most significant? What’s the connection among these variables? And, perhaps most importantly, how certain are we about all of these variables? Regression analysis has been applied to cancer research for decades, from survival analysis with Cox’s proportional hazard regression to Least Absolute Shrinkage Selection Operator Regression (LASSO) regression for significant feature selection.
Linear regression is the most common and simplest model for discovering how one or more explanatory variables determine the dependent variable (129). Logistic regression is extended by a linear regression model for classification problems. However, it differs from linear regression by being employed when the outcome variable is binary. Yin et al. built a diagnosis signature by logistic regression based on selected significant factors correlated with TAMs. They found that these factors were conducive to distinguish normal tissues from tumor (108). Cox proportional hazard is generally used when the outcome is the time to an occurrence (for example, time to death, time to relapse). The results of Cox are explained in terms of a hazard ratio, indicating the risk of an event at a given time. Ridge regression and LASSO regression are variants of linear regression (linear regression appended with a regularization term) introduced for more accurate prediction. Ridge and LASSO are commonly used to reduce model complexity and prevent potential over-fitting. Typically, LASSO and Cox are combined together for disease prognosis. These studies generally use univariate Cox regression and LASSO regression to identify the significant characteristics and multivariate Cox regression to build risk score models (108).
Another variant of linear regression is elastic net regression. It integrates the LASSO and ridge regression methods by learning from their drawbacks to improve the regularization of statistical models. Thus, it achieves a more stable and better prediction than LASSO and ridge regression in less training samples. In two studies that intended to develop a prognostic model based on the molecular feature of TAMs, they both used elastic net to construct risk scores (105, 106). Especially, in Zhang et al’s study, they found that glioma with higher risk scores is populated by macrophages comprising both the traditional M1 and M2 phenotypes, which further indicates that M0/M1/M2 is a continuum rather than two extremes (106).
Support Vector Machine (SVM) is a powerful method that can be used for both regression and classification tasks (130). However, it mostly works as a classifier and aims to create a decision boundary, also termed as hyperplane, between two classes that distinctly classifies the data into different categories (131). The objective of SVM is to maximize the margin to select the best hyperplane, which offers some reinforcement so that subsequent data points can be classified with greater confidence. The margin is determined by a series of hyperplanes parallel to the decision boundary whose distance to the nearest data point is the largest in either the positive or negative class, as depicted in Figure 2C.
As a classifier, SVM is frequently used in TAMs. Patients can be classified into different groups based on the significant tumor-infiltrating immune cell proportions. For instance, patients with rectal cancer can be classified into responsive and non-responsive groups through the ML method based on the tumor-infiltrating immune cell composition and achieved an accuracy of 65% (104). Nakamura et al. applied SVM to discriminate between malignant and non-malignant tissues in ovarian cancer patients and malignant ovary samples through the immune signatures including M1 macrophage mediator signatures (117). Yan et al. used SVM to explore prognostic genes associated with immune infiltration and the classification accuracy reached as high as 0.934. Also of note, the high and low-risk groups exhibited significantly different proportions of TAMs (104). Some researchers used SVM to further validate the clustering results (105, 106). In an article by Liang, the authors applied six ML algorithms to predict inflammasome clusters, in which macrophages were the major immune cell population enriched in inflammasome complexMid and inflammasome complexHigh clusters. In this paper, SVM achieved a highest prediction accuracy of 96% (118). Some researchers also use SVM-RFE, a feature selection algorithm that ranks the features according to the recursive feature deletion sequence, to identify prognostic genes associated with TAMs infiltration (109, 111).
The strength of SVM is that it can be used for complex data sets with many variables or dimensions. However, when it comes to high dimensions, SVM achieves a powerful model at the cost of easy interpretation of which features are influencing the model.
Random forest (RF) is an ensemble decision tree classifier combining multiple tree predictors introduced by Leo Breiman (132). As an ML algorithm near the top of the classifier hierarchy, the RF classifier is capable of ranking the predictive ability of each variable and constructing a predictive model (110). Generally, RF is based on the aggregation of a large number of uncorrelated and weak decision trees, and each uncorrelated tree casts an individual prediction. The final decision is made by majority voting of all trees, which outperform any single classifier (Figure 2D). RF models are considered less vulnerable to overfit the training data set given the large number of trees built, making each tree an independent model. Given a large number of trees ensembled and each tree indicating an independent model, random forest models are thought to be less susceptible to overfitting. The ability of RF to precisely classify observations is extremely valuable in oncology applications, such as predicting patient death or tumor relapse. So far, RF has been applied to many TAMs studies for classification. They are generally used to screen TAMs-related markers and construct an immune-related risk score for risk prediction (110, 121, 123, 125). By utilizing RFs, a diagnostic model based on immune infiltration can accurately perform the differential diagnosis of bone-related malignancies (119). Nakamura et al. used RF to investigate whether genes identified by literature search or other analysis can distinguish between normal tissues and cancer tissues (117). In many studies, RFs also worked with other algorithms to screen the overlapping markers, e.g., LASSO (121, 125).
Overall, the advantage of RF is that it is an ensemble algorithm which has more accuracy than any individual prediction, especially when multi-modality variables are combined (133). However, the high dimension of all the features in cancer research and their complex interactions make it very difficult for humans to interpret the model and results.
Deep learning (DL) is a notable sub-class of ML which has a remarkable ability to learn patterns from raw, unstructured input data by incorporating artificial neural networks (ANN) (134). ANN is inspired by the structure and function of the brain. It attempts to use multiple layers of calculation units to imitate how the human brain processes input information. It is essentially a mathematical model consisting of an input layer, multiple hidden layers, and an output layer, as shown in Figure 2E. Each layer has multiple artificial neurons, also known as nodes in neural network. The nodes in input layers gather source material such as image pixels and numerical data. Hidden layers in the middle connect nodes to the next layer, creating non-linear representations between source data and the output layer (135).
Despite deriving from ANN, the DL framework differs from a straightforward neural network. Overall, DL networks are larger and consist of more layers and nodes, making it possible to reflect complicated interrelationships precisely. DL is able to process plenty of features across a large number of samples and derive neural network-based ‘representations’ quickly. Many specialized DL models have outperformed traditional ML models for various tasks, such as medical image segmentation and image-based tumor staging. Classical DL algorithms include Convolutional Neural Network (136), Recurrent Neural Networks (137), Radial Basis Function Networks (138), Long Short-Term Memory Networks (LSTMs) (139), Self-Organizing Maps (140), Autoencoders (141), etc., which have been proved to achieve state-of-the-art performance in specific applications (142–144).
Applications of neural networks and DL in TAMs focus more on classification and medical image segmentation. Li et al. developed an MRI radiomics approach to predict survival and tumor-infiltrating macrophages in gliomas (120). They used two neural network models and one long short-term memory DL model to divide patients into long and short-term survival clusters. In research conducted by Wang et al. (112), Mask R-CNN, a DL-based model, was applied to segment the nuclei of the tumor, lymphocyte, stroma, karyorrhexis, red blood cells and macrophage from pathology images. In addition to the existing segmentation algorithms, some studies developed their own DL segmentation models to characterize immune infiltration. Risom et al. segmented cell nuclei using Msmer, a DL-based algorithm developed in their lab (145), and Hagos et al. used ConCORDe-Net to detect cells in multiplex immunohistochemistry images (122). Meanwhile, commercial and Open-source software could also be used for segmentation in cancer research. For example, inForm software package (Akoya Biosciences) has been applied in some studies to automatically perform tissue category segmentation, cell segmentation, and cell type classification (113, 114). InForm software is a powerful software that enables per-cell analysis of immunohistochemistry and immunofluorescence. It allows the separation and measurement of weak and spectrally overlapping markers and automatic detection and segmentation of specific tissues. Orange Data Mining Toolbox is another open-source software. Rostam et al. used it to automatically identify different macrophage functional phenotypes based on cell size and morphology (103).
Interest in DL models has grown in recent decades owing to rapid advances in high-performance computing infrastructure, such as cloud and GPU computing (146). However, it is still far from meeting the vast amounts of data needed for medical research. Developing deep neural networks and then training is time-consuming and computationally expensive compared with traditional ML methods.
Despite such exciting research, various limitations or requirements must be addressed before ML can realize its full potential in the studies focusing on TAMs. As most ML models are data-driven, the most critical challenge is the requirement of tremendous and valuable data sets (147). Generally, data related to TAMs can be incredibly complex, with thousands of variables capturing different facets of the TME system. However, these data sets are still too small for ML modeling, especially for unsupervised learning. The lack of sample size might lead to poor model performance or overfitting. Deep neural networks are especially vulnerable to overfitting because they have thousands to millions of parameters.
Moreover, data quality and completeness are also challenging in the studies of tumor prognosis, in which patient follow-up might be irregularly collected or lost, and different institutions may use various standards of testing. In response to the challenge of massive clinical data acquisition, some cloud-based cancer repositories such as Gene Expression Omnibus (GEO) and The Cancer Genome Atlas (TCGA) have been created to enable cross-institution data sharing and data quality assurance. We hope with the emergence of more open-source data sets and data standardization, these restrictions will be less of an issue in the future.
Clinical translation is also a challenge for ML. Many trials are still in the stage of by-proof-test. Research groups and companies are facing the challenges of making their products more reliable and practical in large-scale implementations or even real usage scenarios. Similarly, many innovative solutions, generated from the frontiers of ML research and shown to be theoretically powerful, have yet to integrate into day-to-day clinical use. In modeling, most models take fixed training and testing data set, which is impractical in real clinical practice. Considering the rapid changes in tumor data, continuous updating and reevaluation are required to monitor the model performance and guarantee model consistency. In addition, most of the current ML-based tumor models are single-center studies. There are considerably fewer external validation studies of TAMs in the published papers. Future studies should involve external or cross-institution validation to ensure the test set is diversified enough with different clinic scenarios involved. We believe the robust external validation and improvements in interpretability and generalizability may boost clinician confidence in ML and facilitate further incorporation of ML models into clinical practice.
Furthermore, after reviewing papers combining ML with TAMs, we come to realize that the complexity and heterogeneity of TAMs in TME are far from being fully elucidated. As discussed above, the dichotomy of TAMs is too simple to clarify macrophage activation states in vivo. What should be noted is that M0/M1/M2 is a continuum in vivo instead of well-delineated categories. TAMs are characterized by its remarkable plasticity. The phenotypes can switch between the two extremes, while most existing studies still regard TAMs as two distinct extremes. Besides, subtypes of M2-TAMs can be further identified and classified as M2a, M2b, M2c (148, 149), and M2d in TME. Identifying complexity and heterogeneity of TAMs in vivo and the subtypes of M2 macrophages more precisely to reduce side effects of cancer therapy using ML methods can be challenging but promising. Therapies addressing the recruitment, depletion and repolarization of M2 are promising strategies for tumor treatment. With the help of ML, many studies are enabled to identify specific molecules involved in polarization of M0 macrophages towards M1/M2 macrophages and TAMs recruitment. However, the key biomarkers in depletion and repolarization of M2 based on ML have not received a lot of attention. By integrating more medical images and omics data, it is anticipated that ML will have broader prospects on exploring, validating and implementing critical genes in the repolarization of TAMs to further facilitate precision oncology.
ML in cancer research is still in the early stage of exploration. More investigations and efforts are required to break through current limitations. In terms of reducing the need for a large data set, Generative Adversarial Networks (GAN) are receiving attention. GAN has two neural networks, which are generative and discriminator networks. They contest with each other in a zero-sum game and generate new and synthetic instances of data that can ‘fool’ the discriminator network.
Precision medicine is the future direction of cancer therapy, in which case patients can get optimized management and treatment to improve survival. An important part of precision oncology involves understanding cancer genomics, radiomics and the complex heterogeneity of TME. With the help of ML, scientists are able to disentangle more cancer characteristics, enabling precision oncology. One of the popular and evolutionary directions in ML is reinforcement learning. It learns to achieve goals in an uncertain and complex environment. Due to the non-stationary tumor environment with changing conditions and stimuli, reinforcement learning has the potential to offer computer-guided decision support for personalized treatment. Currently, its applications in medicine are mainly focus on medical image analysis, disease screening and personalized treatment recommendations. In the future, we envision that it could be employed for dynamic cancer treatment regimens after personalized tumor prognosis, tailoring the treatment for each individual.
Overall, the combination of ML and TAMs is relatively young and far from fulfilling its potential in cancer research. The distinctive nature of cancer studies makes accuracy and interpretability extremely crucial. We still have a long way to go to uncover and harness the intricacies of ML and the complexities of TME. Hopefully, with ever-evolving algorithms, more potent supercomputers, and substantial investment being involved in this field, these applications will be more intelligent, cost-effective, and time-efficient. In the future, ML is expected to play a more critical role in TAMs analysis and precision oncology.
ZL and QY wrote the manuscript; QZ and XY reviewed and edited the paper; ZBL and JF offered technological guidance. All authors reviewed the results and approved the final version of the manuscript. ZL and QY are the co-first authors and JF is the corresponding author.
This study was supported by the Science and Technology Project of Shanghai Municipal Science and Technology Commission (No.22Y31900500).
The authors declare that the research was conducted in the absence of any commercial or financial relationships that could be construed as a potential conflict of interest.
All claims expressed in this article are solely those of the authors and do not necessarily represent those of their affiliated organizations, or those of the publisher, the editors and the reviewers. Any product that may be evaluated in this article, or claim that may be made by its manufacturer, is not guaranteed or endorsed by the publisher.
1. König IR, Fuchs O, Hansen G., Mutius von E, M, Kopp V, et al. What is precision medicine? Eur Respir J (2017) 50(4). doi: 10.1183/13993003.00391-2017
2. Issa NT, Stathias V, Schürer S, Dakshanamurthy S. Machine and deep learning approaches for cancer drug repurposing. Semin Cancer Biol (2021) 68:132–42. doi: 10.1016/j.semcancer.2019.12.011
3. Ngiam KY, Khor IW. Big data and machine learning algorithms for health-care delivery. Lancet Oncol (2019) 20(5):e262–73. doi: 10.1016/S1470-2045(19)30149-4
4. Vamathevan J, Clark D, Czodrowski P, Dunham I, Ferran E, Lee G, et al. Applications of machine learning in drug discovery and development. Nat Rev Drug Discov (2019) 18(6):463–77. doi: 10.1038/s41573-019-0024-5
5. Lynch CM, Abdollahi B, Fuqua JD, Carlo AR, Bartholomai JA, Balgemann RN, et al. Prediction of lung cancer patient survival via supervised machine learning classification techniques. Int J Med Inform (2017) 108:1–8. doi: 10.1016/j.ijmedinf.2017.09.013
6. Pathria P, Louis TL, Varner JA. Targeting tumor-associated macrophages in cancer. Trends Immunol (2019) 40(4):310–27.
7. Wynn TA, Chawla A, Pollard JW. Macrophage biology in development, homeostasis and disease. Nature (2013) 496(7446):445–55.
8. Krenkel O, Tacke F. Liver macrophages in tissue homeostasis and disease. Nat Rev Immunol (2017) 17(5):306–21.
9. Shapouri-Moghaddam A, Mohammadian S, Vazini H, Taghadosi MS, Esmaeili A, Mardani F, et al. Macrophage plasticity, polarization, and function in health and disease. J Cell Physiol (2018) 233(9):6425–40.
10. Jaguin M, Houlbert N, Fardel O, Lecureur V, et al. Polarization profiles of human m-CSF-generated macrophages and comparison of M1-markers in classically activated macrophages from GM-CSF and m-CSF origin. Cell Immunol (2013) 281(1):51–61.
11. Orecchioni M, Ghosheh Y, Pramod AB, Ley K. Macrophage polarization: Different gene signatures in M1(LPS+) vs. classically and M2(LPS-) vs. alternatively activated macrophages. Front Immunol (2019) 10:1084. doi: 10.3389/fimmu.2019.01084
12. Trombetta AC, Soldano S, Contini P, Tomatis V, Ruaro B, Paolino S, et al. A circulating cell population showing both M1 and M2 monocyte/macrophage surface markers characterizes systemic sclerosis patients with lung involvement. Respir Res (2018) 19(1):186. doi: 10.1186/s12931-018-0891-z
13. Feng D, Huang WY, Niu XL, Hao S, Zhang LN, Hu YJ, et al. Significance of macrophage subtypes in the peripheral blood of children with systemic juvenile idiopathic arthritis. Rheumatol Ther (2021) 8(4):1859–70. doi: 10.1007/s40744-021-00385-x
14. Cunha C, Gomes C, Vaz AR, Brites D. Exploring new inflammatory biomarkers and pathways during LPS-induced M1 polarization. Med Inflamm (2016) 2016:6986175. doi: 10.1155/2016/6986175
15. Yamaguchi T, Fushida S, Yamamoto Y, Tsukada T, Kinoshita J, Oyama K, et al. Tumor-associated macrophages of the M2 phenotype contribute to progression in gastric cancer with peritoneal dissemination. Gastric Cancer (2016) 19(4):1052–65. doi: 10.1007/s10120-015-0579-8
16. Nakagawa M, Karim MR, Izawa T, Kuwamura M, Yamate J. Immunophenotypical characterization of M1/M2 macrophages and lymphocytes in cisplatin-induced rat progressive renal fibrosis. Cells (2021) 10(2). doi: 10.3390/cells10020257
17. Su MJ, Aldawsari H, Amiji M. Pancreatic cancer cell exosome-mediated macrophage reprogramming and the role of MicroRNAs 155 and 125b2 transfection using nanoparticle delivery systems. Sci Rep (2016) 6:30110. doi: 10.1038/srep30110
18. Qiu Y, Xu J, Yang L, Zhao G, Ding J, Chen Q, et al. MiR-375 silencing attenuates pro-inflammatory macrophage response and foam cell formation by targeting KLF4. Exp Cell Res (2021) 400(1):112507. doi: 10.1016/j.yexcr.2021.112507
19. Jang JY, Lee JK, Jeon YK, Kim CW. Exosome derived from epigallocatechin gallate treated breast cancer cells suppresses tumor growth by inhibiting tumor-associated macrophage infiltration and M2 polarization. BMC Cancer (2013) 13:421. doi: 10.1186/1471-2407-13-421
20. Weng YS, Tseng HY, Chen YA, Shen PC, Haq Al AT, Chen LM, et al. MCT-1/miR-34a/IL-6/IL-6R signaling axis promotes EMT progression, cancer stemness and M2 macrophage polarization in triple-negative breast cancer. Mol Cancer (2019) 18(1):42. doi: 10.1186/s12943-019-0988-0
21. Tong F, Mao X, Zhang S, Xie H, Yan B, Wang B, et al. HPV + HNSCC-derived exosomal miR-9 induces macrophage M1 polarization and increases tumor radiosensitivity. Cancer Lett (2020) 478:34–44. doi: 10.1016/j.canlet.2020.02.037
22. Wang X, Luo G, Zhang K, Cao J, Huang C, Jiang T, et al. Hypoxic tumor-derived exosomal miR-301a mediates M2 macrophage polarization via PTEN/PI3Kγ to promote pancreatic cancer metastasis. Cancer Res (2018) 78(16):4586–98. doi: 10.1158/0008-5472.CAN-17-3841
23. Luo YY, Yang ZQ, Lin XF, Zhao FL, Tu HT, Wang LJ, et al. Knockdown of lncRNA PVT1 attenuated macrophage M1 polarization and relieved sepsis induced myocardial injury via miR-29a/HMGB1 axis. Cytokine (2021) 143:155509. doi: 10.1016/j.cyto.2021.155509
24. Zhao S, Mi Y, Guan B, Zheng B, Wei P, Gu Y, et al. Tumor-derived exosomal miR-934 induces macrophage M2 polarization to promote liver metastasis of colorectal cancer. J Hematol Oncol (2020) 13(1):156. doi: 10.1186/s13045-020-00991-2
25. Jiang M, Dai J, Yin M, Jiang C, Ren M, Tian L, et al. LncRNA MEG8 sponging miR-181a-5p contributes to M1 macrophage polarization by regulating SHP2 expression in henoch-schonlein purpura rats. Ann Med (2021) 53(1):1576–88. doi: 10.1080/07853890.2021.1969033
26. Chen X, Ying X, Wang X, Wu X, Zhu Q, Wang X, et al. Exosomes derived from hypoxic epithelial ovarian cancer deliver microRNA-940 to induce macrophage M2 polarization. Oncol Rep (2017) 38(1):522–8. doi: 10.3892/or.2017.5697
27. Chi X, Ding B, Zhang L, Zhang J, Wang J, Zhang W, et al. lncRNA GAS5 promotes M1 macrophage polarization via miR-455-5p/SOCS3 pathway in childhood pneumonia. J Cell Physiol (2019) 234(8):13242–51. doi: 10.1002/jcp.27996
28. Rong J, Xu L, Hu Y, Liu F, Yu Y, Guo H, et al. Inhibition of let-7b-5p contributes to an anti-tumorigenic macrophage phenotype through the SOCS1/STAT pathway in prostate cancer. Cancer Cell Int (2020) 20:470. doi: 10.1186/s12935-020-01563-7
29. Pasca S, Jurj A, Petrushev B, Tomuleasa C, Matei D. MicroRNA-155 implication in M1 polarization and the impact in inflammatory diseases. Front Immunol (2020) 11:625. doi: 10.3389/fimmu.2020.00625
30. Ge X, Tang P, Rong Y, Jiang D, Lu X, Ji C, et al. Exosomal miR-155 from M1-polarized macrophages promotes EndoMT and impairs mitochondrial function via activating NF-κB signaling pathway in vascular endothelial cells after traumatic spinal cord injury. Redox Biol (2021) 41:101932. doi: 10.1016/j.redox.2021.101932
31. Banerjee S, Xie N, Cui H, Tan Z, Yang S, Icyuz M, et al. MicroRNA let-7c regulates macrophage polarization. J Immunol (2013) 190(12):6542–9. doi: 10.4049/jimmunol.1202496
32. Xu S, Wei J, Wang F, Kong LY, Ling XY, Nduom E, et al. Effect of miR-142-3p on the M2 macrophage and therapeutic efficacy against murine glioblastoma. J Natl Cancer Inst (2014) 106(8). doi: 10.1093/jnci/dju162
33. Baer C, Squadrito ML, Laoui D, Thompson D, Hansen SK, Kiialainen A, et al. Suppression of microRNA activity amplifies IFN-γ-induced macrophage activation and promotes anti-tumour immunity. Nat Cell Biol (2016) 18(7):790–802. doi: 10.1038/ncb3371
34. Chen J, Zhang K, Zhi Y, Wu Y, Chen B, Bai J, et al. Tumor-derived exosomal miR-19b-3p facilitates M2 macrophage polarization and exosomal LINC00273 secretion to promote lung adenocarcinoma metastasis via hippo pathway. Clin Transl Med (2021) 11(9):e478. doi: 10.1002/ctm2.478
35. Cao J, Dong R, Jiang L, Gong Y, Yuan M, You J, et al. LncRNA-MM2P identified as a modulator of macrophage M2 polarization. Cancer Immunol Res (2019) 7(2):292–305. doi: 10.1158/2326-6066.CIR-18-0145
36. Mills CD. M1 and M2 macrophages: Oracles of health and disease. Crit Rev Immunol (2012) 32(6):463–88. doi: 10.1615/CritRevImmunol.v32.i6.10
37. De Santa F, Vitiello L, Torcinaro A, Ferraro E. The role of metabolic remodeling in macrophage polarization and its effect on skeletal muscle regeneration. Antioxid Redox Signal (2019) 30(12):1553–98. doi: 10.1089/ars.2017.7420
38. Wang J, Li R, Peng Z, Hu B, Rao X, Li J, et al. HMGB1 participates in LPS−induced acute lung injury by activating the AIM2 inflammasome in macrophages and inducing polarization of M1 macrophages via TLR2, TLR4, and RAGE/NF−κB signaling pathways. Int J Mol Med (2020) 45(1):61–80.
39. Loeuillard E, Yang J, Buckarma E, Wang J, Liu Y, Conboy C, et al. Targeting tumor-associated macrophages and granulocytic myeloid-derived suppressor cells augments PD-1 blockade in cholangiocarcinoma. J Clin Invest (2020) 130(10):5380–96. doi: 10.1172/JCI137110
40. Gordon SR, Maute RL, Dulken BW, Hutter G, George BM, McCracken MN, et al. PD-1 expression by tumour-associated macrophages inhibits phagocytosis and tumour immunity. Nature (2017) 545(7655):495–9. doi: 10.1038/nature22396
41. Chen J, Lin Z, Liu L, Zhang R, Geng Y, Fan M, et al. GOLM1 exacerbates CD8(+) T cell suppression in hepatocellular carcinoma by promoting exosomal PD-L1 transport into tumor-associated macrophages. Signal Transduct Target Ther (2021) 6(1):397. doi: 10.1038/s41392-021-00784-0
42. Arlauckas SP, Garren SB, Garris CS, Kohler RH, Oh J, Pittet MJ, et al. Arg1 expression defines immunosuppressive subsets of tumor-associated macrophages. Theranostics (2018) 8(21):5842–54. doi: 10.7150/thno.26888
43. Ren J, Han X, Lohner H, Liang R, Liang S, Wang H, et al. Serum- and glucocorticoid-inducible kinase 1 promotes alternative macrophage polarization and restrains inflammation through FoxO1 and STAT3 signaling. J Immunol (2021) 207(1):268–80. doi: 10.4049/jimmunol.2001455
44. Mohapatra S, Pioppini C, Ozpolat B, Calin GA. Non-coding RNAs regulation of macrophage polarization in cancer. Mol Cancer (2021) 20(1):24. doi: 10.1186/s12943-021-01313-x
45. Zhou J, Tang Z, Gao S, Li C, Feng Y, Zhou X, et al. Tumor-associated macrophages: Recent insights and therapies. Front Oncol (2020) 10:188. doi: 10.3389/fonc.2020.00188
46. Leibovich SJ, Ross R. The role of the macrophage in wound repair. a study with hydrocortisone and antimacrophage serum. Am J Pathol (1975) 78(1):71–100.
47. Polverini PJ, Cotran PS, Gimbrone MA Jr, Unanue ER. Activated macrophages induce vascular proliferation. Nature (1977) 269(5631):804–6. doi: 10.1038/269804a0
48. Engblom C, Pfirschke C, Pittet MJ. The role of myeloid cells in cancer therapies. Nat Rev Cancer (2016) 16(7):447–62. doi: 10.1038/nrc.2016.54
49. Guillot A, Tacke F. Liver macrophages: Old dogmas and new insights. Hepatol Commun (2019) 3(6):730–43. doi: 10.1002/hep4.1356
50. Wu K, Lin K, Li X, Yuan X, Xu P, Ni P, et al. Redefining tumor-associated macrophage subpopulations and functions in the tumor microenvironment. Front Immunol (2020) 11:1731. doi: 10.3389/fimmu.2020.01731
51. Helm O, Mennrich R, Petrick D, Goebel L, Freitag-Wolf S, Röder C, et al. Comparative characterization of stroma cells and ductal epithelium in chronic pancreatitis and pancreatic ductal adenocarcinoma. PloS One (2014) 9(5):e94357. doi: 10.1371/journal.pone.0094357
52. Wu MF, Lin CA, Yuan TH, Yeh HY, Su SF, Gu CL, et al. The M1/M2 spectrum and plasticity of malignant pleural effusion-macrophage in advanced lung cancer. Cancer Immunol Immunother (2021) 70(5):1435–50. doi: 10.1007/s00262-020-02781-8
53. Cai H, Zhang Y, Wang J, Gu J. Defects in macrophage reprogramming in cancer therapy: The negative impact of PD-L1/PD-1. Front Immunol (2021) 12:690869. doi: 10.3389/fimmu.2021.690869
54. Yin M, Li X, Tan S, Zhou HJ, Ji W, Bellone S, et al. Tumor-associated macrophages drive spheroid formation during early transcoelomic metastasis of ovarian cancer. J Clin Invest (2016) 126(11):4157–73. doi: 10.1172/JCI87252
55. Pan Y, Yu Y, Wang X, Zhang T. Tumor-associated macrophages in tumor immunity. Front Immunol (2020) 11:583084. doi: 10.3389/fimmu.2020.583084
56. Tanaka A, Sakaguchi S. Targeting treg cells in cancer immunotherapy. Eur J Immunol (2019) 49(8):1140–6. doi: 10.1002/eji.201847659
57. Noy R, Pollard JW. Tumor-associated macrophages: from mechanisms to therapy. Immunity (2014) 41(1):49–61. doi: 10.1016/j.immuni.2014.06.010
58. Wang D, Yang L, Yue D, Cao L, Li L, Wang D, et al. Macrophage-derived CCL22 promotes an immunosuppressive tumor microenvironment via IL-8 in malignant pleural effusion. Cancer Lett (2019) 452:244–53. doi: 10.1016/j.canlet.2019.03.040
59. Allavena P, Chieppa M, Bianchi G, Solinas G, Fabbri M, Laskarin G, et al. Engagement of the mannose receptor by tumoral mucins activates an immune suppressive phenotype in human tumor-associated macrophages. Clin Dev Immunol (2010) 2010:547179. doi: 10.1155/2010/547179
60. Thibault B, Castells M, Delord JP, Couderc B. Ovarian cancer microenvironment: implications for cancer dissemination and chemoresistance acquisition. Cancer Metastasis Rev (2014) 33(1):17–39. doi: 10.1007/s10555-013-9456-2
61. Chen YC, Lai YS, Hsuuw YD, Chang KT. Withholding of m-CSF supplement reprograms macrophages to M2-like via endogenous CSF-1 activation. Int J Mol Sci (2021) 22(7). doi: 10.3390/ijms22073532
62. Folkman J. What is the evidence that tumors are angiogenesis dependent? J Natl Cancer Inst (1990) 82(1):4–6. doi: 10.1093/jnci/82.1.4
63. Baeriswyl V, Christofori G. The angiogenic switch in carcinogenesis. Semin Cancer Biol (2009) 19(5):329–37. doi: 10.1016/j.semcancer.2009.05.003
64. Biswas SK, Sica A, Lewis CE. Plasticity of macrophage function during tumor progression: regulation by distinct molecular mechanisms. J Immunol (2008) 180(4):2011–7. doi: 10.4049/jimmunol.180.4.2011
65. White JR, Harris RA, Lee SR, Craigon MH, Binley K, Price T, et al. Genetic amplification of the transcriptional response to hypoxia as a novel means of identifying regulators of angiogenesis. Genomics (2004) 83(1):1–8. doi: 10.1016/S0888-7543(03)00215-5
66. Werno C, Menrad H, Weigert A, Dehne N, Goerdt S, Schledzewski K, et al. Knockout of HIF-1α in tumor-associated macrophages enhances M2 polarization and attenuates their pro-angiogenic responses. Carcinogenesis (2010) 31(10):1863–72. doi: 10.1093/carcin/bgq088
67. Li N, Li Y, Li Z, Huang C, Yang Y, Lang M, et al. Hypoxia inducible factor 1 (HIF-1) recruits macrophage to activate pancreatic stellate cells in pancreatic ductal adenocarcinoma. Int J Mol Sci (2016) 17(6). doi: 10.3390/ijms17060799
68. Cassetta L, Pollard JW. Targeting macrophages: therapeutic approaches in cancer. Nat Rev Drug Discov (2018) 17(12):887–904. doi: 10.1038/nrd.2018.169
69. Wang HW, Joyce JA. Alternative activation of tumor-associated macrophages by IL-4: priming for protumoral functions. Cell Cycle (2010) 9(24):4824–35. doi: 10.4161/cc.9.24.14322
70. Peinado H, Zhang H, Matei IR, Costa-Silva B, Hoshino A, Rodrigues G, et al. Pre-metastatic niches: organ-specific homes for metastases. Nat Rev Cancer (2017) 17(5):302–17. doi: 10.1038/nrc.2017.6
71. Sleeman JP. The lymph node pre-metastatic niche. J Mol Med (Berl) (2015) 93(11):1173–84. doi: 10.1007/s00109-015-1351-6
72. Kaplan RN, Riba RD, Zacharoulis S, Bramley AH, Vincent L, Costa C, et al. VEGFR1-positive haematopoietic bone marrow progenitors initiate the pre-metastatic niche. Nature (2005) 438(7069):820–7. doi: 10.1038/nature04186
73. Joyce JA, Pollard JW. Microenvironmental regulation of metastasis. Nat Rev Cancer (2009) 9(4):239–52. doi: 10.1038/nrc2618
74. Sceneay J, Smyth MJ, Möller A. The pre-metastatic niche: finding common ground. Cancer Metastasis Rev (2013) 32(3-4):449–64. doi: 10.1007/s10555-013-9420-1
75. Lin Y, Xu J, Lan H. Tumor-associated macrophages in tumor metastasis: biological roles and clinical therapeutic applications. J Hematol Oncol (2019) 12(1):76. doi: 10.1186/s13045-019-0760-3
76. Werner L, Dreyer JH, Hartmann D, Barros MHM, Büttner-Herold M, Grittner U, et al. Tumor-associated macrophages in classical Hodgkin lymphoma: hormetic relationship to outcome. Sci Rep (2020) 10(1):9410. doi: 10.1038/s41598-020-66010-z
77. Hwang I, Kim JW, Ylaya K, Chung EJ, Kitano H, Perry C, et al. Tumor-associated macrophage, angiogenesis and lymphangiogenesis markers predict prognosis of non-small cell lung cancer patients. J Transl Med (2020) 18(1):443. doi: 10.1186/s12967-020-02618-z
78. Nie Y, Huang H, Guo M, Chen J, Wu W, Li W, et al. Breast phyllodes tumors recruit and repolarize tumor-associated macrophages via secreting CCL5 to promote malignant progression, which can be inhibited by CCR5 inhibition therapy. Clin Cancer Res (2019) 25(13):3873–86. doi: 10.1158/1078-0432.CCR-18-3421
79. Kleinschmidt J, Zucker CL, Yazulla S. Neurotoxic action of kainic acid in the isolated toad and goldfish retina: II. mechanism of action. J Comp Neurol (1986) 254(2):196–208. doi: 10.1002/cne.902540205
80. Xiang X, Wang J, Lu D, Xu X. Targeting tumor-associated macrophages to synergize tumor immunotherapy. Signal Transduct Target Ther (2021) 6(1):75. doi: 10.1038/s41392-021-00484-9
81. Li D, Ji H, Niu X, Yin L, Wang Y, Gu Y. Tumor-associated macrophages secrete CC-chemokine ligand 2 and induce tamoxifen resistance by activating PI3K/Akt/mTOR in breast cancer. Cancer Sci (2020) 111(1):47–58. doi: 10.1111/cas.14230
82. Jinushi M, Chiba S, Yoshiyama H, Masutomi K, Kinoshita I, Dosaka-Akita H, et al. Tumor-associated macrophages regulate tumorigenicity and anticancer drug responses of cancer stem/initiating cells. Proc Natl Acad Sci USA (2011) 108(30):12425–30. doi: 10.1073/pnas.1106645108
83. Kong L, Zhou Y, Bu H, Lv T, Shi Y, Yang J, et al. Deletion of interleukin-6 in monocytes/macrophages suppresses the initiation of hepatocellular carcinoma in mice. J Exp Clin Cancer Res (2016) 35(1):131. doi: 10.1186/s13046-016-0412-1
84. Liu CQ, Xu J, Zhou ZG, Jin LL, Yu XJ, Xiao G, et al. Expression patterns of programmed death ligand 1 correlate with different microenvironments and patient prognosis in hepatocellular carcinoma. Br J Cancer (2018) 119(1):80–8. doi: 10.1038/s41416-018-0144-4
85. Kimm MA, Klenk C, Alunni-Fabbroni M, Kästle S, Stechele M, Ricke J, et al. Tumor-associated macrophages-implications for molecular oncology and imaging. Biomedicines (2021) 9(4). doi: 10.3390/biomedicines9040374
86. MacEachern SJ, Forkert ND. Machine learning for precision medicine. Genome (2021) 64(4):416–25. doi: 10.1139/gen-2020-0131
87. Deo RC. Machine learning in medicine. Circulation (2015) 132(20):1920–30. doi: 10.1161/CIRCULATIONAHA.115.001593
88. Handelman GS, Kok HK, Chandra RV, Razavi AH, Lee MJ, Asadi H, et al. eDoctor: machine learning and the future of medicine. J Intern Med (2018) 284(6):603–19. doi: 10.1111/joim.12822
89. Shameer K, Johnson KW, Glicksberg BS, Dudley JT, Sengupta PP, et al. Machine learning in cardiovascular medicine: are we there yet? Heart (2018) 104(14):1156–64. doi: 10.1136/heartjnl-2017-311198
90. Cammarota G, Ianiro G, Ahern A, Carbone C, Temko A, Claesson MJ, et al. Gut microbiome, big data and machine learning to promote precision medicine for cancer. Nat Rev Gastroenterol Hepatol (2020) 17(10):635–48. doi: 10.1038/s41575-020-0327-3
91. Berry MW, Mohamed A, Yap BW. Supervised and unsupervised learning for data science. Springer (2019).
92. Cunningham P, Cord M, Delany SJ. Supervised learning. In: Machine learning techniques for multimedia. Springer (2008). p. 21–49.
93. Zhu X, Goldberg AB. Introduction to semi-supervised learning. Synthesis Lectures Artif Intell Mach Learn (2009) 3(1):1–130. doi: 10.1007/978-3-031-01548-9
95. Abbasi B, Goldenholz DM. Machine learning applications in epilepsy. Epilepsia (2019) 60(10):2037–47. doi: 10.1111/epi.16333
96. Greener JG, Kandathil SM, Moffat L, Jones DT. A guide to machine learning for biologists. Nat Rev Mol Cell Biol (2022) 23(1):40–55. doi: 10.1038/s41580-021-00407-0
97. Gupta S. Pros and cons of various machine learning algorithms (2020). Available at: https://towardsdatascience.com/pros-and-cons-of-various-classification-ml-algorithms-3b5bfb3c87d6.
98. (2019). Available at: https://www.i2tutorials.com/what-are-the-pros-and-cons-of-the-pca/.
99. Kriegeskorte N, Golan T. Neural network models and deep learning. Curr Biol (2019) 29(7):R231–r236. doi: 10.1016/j.cub.2019.02.034
100. Sugahara S, Ueno M. Exact learning augmented naive bayes classifier. Entropy (Basel) (2021) 23(12). doi: 10.3390/e23121703
101. Stoltzfus JC. Logistic regression: a brief primer. Acad Emerg Med (2011) 18(10):1099–104. doi: 10.1111/j.1553-2712.2011.01185.x
102. Chang H, Zhu Y, Zheng J, Chen L, Lin J, Yao J, et al. Construction of a macrophage infiltration regulatory network and related prognostic model of high-grade serous ovarian cancer. J Oncol (2021) 2021:1331031. doi: 10.1155/2021/1331031
103. Rostam HM, Reynolds PM, Alexander MR, Gadegaard N, Ghaemmaghami AM. Image based machine learning for identification of macrophage subsets. Sci Rep (2017) 7(1):3521. doi: 10.1038/s41598-017-03780-z
104. Zhu M, Li X, Ge Y, Nie J, Li X. (2019). The tumor infiltrating leukocyte cell composition are significant markers for prognostics of radiotherapy of rectal cancer as revealed by cell type deconvolution, in: 2019 IEEE Fifth International Conference on Big Data Computing Service and Applications (BigDataService) , IEEE.
105. Zhang N, Dai Z, Wu W, Wang Z, Cao H, Zhang Y, et al. The predictive value of monocytes in immune microenvironment and prognosis of glioma patients based on machine learning. Front Immunol (2021) 12:656541. doi: 10.3389/fimmu.2021.656541
106. Zhang H, Luo YB, Wu W, Zhang L, Wang Z, Dai Z, et al. The molecular feature of macrophages in tumor immune microenvironment of glioma patients. Comput Struct Biotechnol J (2021) 19:4603–18. doi: 10.1016/j.csbj.2021.08.019
107. Zhang E, He J, Zhang H, Shan L, Wu H, Zhang M, et al. Immune-related gene-based novel subtypes to establish a model predicting the risk of prostate cancer. Front Genet (2020) 11:595657. doi: 10.3389/fgene.2020.595657
108. Yin R, Zhai X, Han H, Tong X, Li Y, Deng K, et al. Characterizing the landscape of cervical squamous cell carcinoma immune microenvironment by integrating the single-cell transcriptomics and RNA-seq. Immun Inflammation Dis (2022) 10(6):e608. doi: 10.1002/iid3.608
109. Yan S, Fang J, Chen Y, Xie Y, Zhang S, Zhu X, et al. Comprehensive analysis of prognostic gene signatures based on immune infiltration of ovarian cancer. BMC Cancer (2020) 20(1):1205. doi: 10.1186/s12885-020-07695-3
110. Wu XR, Peng HX, He M, Zhong R, Liu J, Wen YK, et al. Macrophages-based immune-related risk score model for relapse prediction in stage I-III non-small cell lung cancer assessed by multiplex immunofluorescence. Transl Lung Cancer Res (2022) 11(4):523–42. doi: 10.21037/tlcr-21-916
111. Wei S, Lu J, Lou J, Shi C, Mo S, Shao Y, et al. Gastric cancer tumor microenvironment characterization reveals stromal-related gene signatures associated with macrophage infiltration. Front Genet (2020) 11:663. doi: 10.3389/fgene.2020.00663
112. Wang S, Rong R, Yang DM, Fujimoto J, Yan S, Cai L, et al. Computational staining of pathology images to study the tumor microenvironment in lung cancer. Cancer Res (2020) 80(10):2056–66. doi: 10.1158/0008-5472.CAN-19-1629
113. Väyrynen JP, Haruki K, Lau MC, Väyrynen SA, Zhong R, Costa Dias A, et al. The prognostic role of macrophage polarization in the colorectal cancer microenvironment. Cancer Immunol Res (2021) 9(1):8–19. doi: 10.1158/2326-6066.CIR-20-0527
114. Ugai T, Väyrynen JP, Haruki K, Akimoto N, Lau MC, Zhong R, et al. Smoking and incidence of colorectal cancer subclassified by tumor-associated macrophage infiltrates. J Natl Cancer Inst (2022) 114(1):68–77. doi: 10.1093/jnci/djab142
115. Starosolski Z, Courtney AN, Srivastava M, Guo L, Stupin I, Metelitsa LS, et al. A nanoradiomics approach for differentiation of tumors based on tumor-associated macrophage burden. Contrast Media Mol Imaging (2021) 2021:6641384. doi: 10.1155/2021/6641384
116. Shen X, Wang X, Shen H, Feng M, Wu D, Yang Y, et al. Transcriptomic analysis identified two subtypes of brain tumor characterized by distinct immune infiltration and prognosis. Front Oncol (2021) 11:734407. doi: 10.3389/fonc.2021.734407
117. Nakamura M, Bax HJ, Scotto D, Souri EA, Sollie S, Harris RJ, et al. Immune mediator expression signatures are associated with improved outcome in ovarian carcinoma. Oncoimmunology (2019) 8(6):e1593811. doi: 10.1080/2162402X.2019.1593811
118. Liang Q, Wu J, Zhao X, Shen S, Zhu C, Liu T, et al. Establishment of tumor inflammasome clusters with distinct immunogenomic landscape aids immunotherapy. Theranostics (2021) 11(20):9884–903. doi: 10.7150/thno.63202
119. Li GQ, Wang YK, Zhou H, Jin LG, Wang CY, Albahde M, et al. Application of immune infiltration signature and machine learning model in the differential diagnosis and prognosis of bone-related malignancies. Front Cell Dev Biol (2021) 9:630355. doi: 10.3389/fcell.2021.630355
120. Li G, Li L, Li Y, Qian Z, Wu F, He Y, et al. An MRI radiomics approach to predict survival and tumour-infiltrating macrophages in gliomas. Brain (2022) 145(3):1151–61. doi: 10.1093/brain/awab340
121. Kuang Z, Tu J, Li X. Combined identification of novel markers for diagnosis and prognostic of classic Hodgkin lymphoma. Int J Gen Med (2021) 14:9951–63. doi: 10.2147/IJGM.S341557
122. Hagos YB, Akarca AU, Ramsay A, Rossi RL, Pomplun S, Ngai V, et al. High inter-follicular spatial co-localization of CD8+FOXP3+ with CD4+CD8+ cells predicts favorable outcome in follicular lymphoma. Hematol Oncol (2022). doi: 10.1002/hon.3003
123. Guo H, Li B, Diao L, Wang H, Chen P, Jiang M, et al. An immune-based risk-stratification system for predicting prognosis in pulmonary sarcomatoid carcinoma (PSC). Oncoimmunology (2021) 10(1):1947665. doi: 10.1080/2162402X.2021.1947665
124. de Lange MJ, Nell RJ, Lalai RN, Versluis M, Jordanova ES, Luyten GPM, et al. Digital PCR-based T-cell quantification-assisted deconvolution of the microenvironment reveals that activated macrophages drive tumor inflammation in uveal melanoma. Mol Cancer Res (2018) 16(12):1902–11. doi: 10.1158/1541-7786.MCR-18-0114
125. Lin D, Zhao W, Yang J, Wang H, Zhang H. Integrative analysis of biomarkers and mechanisms in adamantinomatous craniopharyngioma. Front Genet (2022) 13:830793. doi: 10.3389/fgene.2022.830793
126. Giuliani A. The application of principal component analysis to drug discovery and biomedical data. Drug Discovery Today (2017) 22(7):1069–76. doi: 10.1016/j.drudis.2017.01.005
127. Liu C, Reynolds PM, Alexander MR, Gadegaard N, Ghaemmaghami AM. Partial least squares regression and principal component analysis: similarity and differences between two popular variable reduction approaches. Gen Psychiatr (2022) 35(1):e100662. doi: 10.1136/gpsych-2021-100662
128. Montgomery DC, Peck EA, Vining GG. Introduction to linear regression analysis. John Wiley & Sons (2021).
129. DeGregory KW, Kuiper P, DeSilvio T, Pleuss JD, Miller R, Roginski JW, et al. A review of machine learning in obesity. Obes Rev (2018) 19(5):668–85. doi: 10.1111/obr.12667
130. Noble WS. What is a support vector machine? Nat Biotechnol (2006) 24(12):1565–7. doi: 10.1038/nbt1206-1565
131. Huang S, Cai N, Pacheco PP, Narrandes S, Wang Y, Xu W, et al. Applications of support vector machine (SVM) learning in cancer genomics. Cancer Genomics Proteomics (2018) 15(1):41–51.
132. Blanchet L, Vitale R, Vorstenbosch van R, Stavropoulos G, Pender J, Jonkers D, et al. Constructing bi-plots for random forest: Tutorial. Anal Chim Acta 2020 (1131) p:146–55.
133. Sarica A, Cerasa A, Quattrone A. Random forest algorithm for the classification of neuroimaging data in alzheimer's disease: A systematic review. Front Aging Neurosci (2017) 9:329. doi: 10.3389/fnagi.2017.00329
134. Shrestha A, Mahmood A. Review of deep learning algorithms and architectures. IEEE Access (2019) 7:53040–65. doi: 10.1109/ACCESS.2019.2912200
135. Radakovich N, Nagy M, Nazha A. Machine learning in haematological malignancies. Lancet Haematol (2020) 7(7):e541–50. doi: 10.1016/S2352-3026(20)30121-6
136. Sarıgül M, Ozyildirim BM, Avci M. Differential convolutional neural network. Neural Netw (2019) 116:279–87. doi: 10.1016/j.neunet.2019.04.025
137. Cossu A, Carta A, Lomonaco V, Bacciu D. Continual learning for recurrent neural networks: An empirical evaluation. Neural Netw (2021) 143:607–27. doi: 10.1016/j.neunet.2021.07.021
138. Orr MJ. Introduction to radial basis function networks. In: Technical report, center for cognitive science. University of Edinburgh (1996).
139. Tai KS, Socher R, Manning CD. Improved semantic representations from tree-structured long short-term memory networks. arXiv (2015). arXiv:1503.00075. doi: 10.3115/v1/P15-1150
142. Carreras J, Kikuti YY, Miyaoka M, Hiraiwa S, Tomita S, Ikoma H, et al. A combination of multilayer perceptron, radial basis function artificial neural networks and machine learning image segmentation for the dimension reduction and the prognosis assessment of diffuse large b-cell lymphoma. AI (2021) 2(1):106–34. doi: 10.3390/ai2010008
143. Carreras J, Kikuti YY, Miyaoka M, Hiraiwa S, Tomita S, Ikoma H, et al. The use of the random number generator and artificial intelligence analysis for dimensionality reduction of follicular lymphoma transcriptomic data. BioMedInformatics (2022) 2(2):268–80. doi: 10.3390/biomedinformatics2020017
144. Carreras J, Kikuti YY, Miyaoka M, Hiraiwa S, Tomita S, Ikoma H, et al. Artificial intelligence analysis of the gene expression of follicular lymphoma predicted the overall survival and correlated with the immune microenvironment response signatures. Mach Learn Knowledge Extraction (2020) 2(4):647–71. doi: 10.3390/make2040035
145. Risom T, Glass DR, Averbukh I, Liu CC, Baranski A, Kagel A, et al. Transition to invasive breast cancer is associated with progressive changes in the structure and composition of tumor stroma. Cell (2022) 185(2):299–310.e18. doi: 10.1016/j.cell.2021.12.023
146. Gupta R, Srivastava D, Sahu M, Tiwari S, Ambasta RK, Kumar P, et al. Artificial intelligence to deep learning: machine intelligence approach for drug discovery. Mol Diversity (2021) 25(3):1315–60. doi: 10.1007/s11030-021-10217-3
147. Schelter S, Biessmann F, Januschowski T, Salinas D, Seufert S, Szarvas G, et al. On challenges in machine learning model management. (2018).
148. Arora S, Dev K, Agarwal B, Das P, Syed MA, et al. Macrophages: Their role, activation and polarization in pulmonary diseases. Immunobiology (2018) 223(4-5):383–96. doi: 10.1016/j.imbio.2017.11.001
Keywords: machine learning, tumor microenvironment, tumor-associated macrophages (TAMs), deep learning, artificial intelligence
Citation: Li Z, Yu Q, Zhu Q, Yang X, Li Z and Fu J (2022) Applications of machine learning in tumor-associated macrophages. Front. Immunol. 13:985863. doi: 10.3389/fimmu.2022.985863
Received: 04 July 2022; Accepted: 07 September 2022;
Published: 23 September 2022.
Edited by:
Ping Zheng, The University of Melbourne, AustraliaCopyright © 2022 Li, Yu, Zhu, Yang, Li and Fu. This is an open-access article distributed under the terms of the Creative Commons Attribution License (CC BY). The use, distribution or reproduction in other forums is permitted, provided the original author(s) and the copyright owner(s) are credited and that the original publication in this journal is cited, in accordance with accepted academic practice. No use, distribution or reproduction is permitted which does not comply with these terms.
*Correspondence: Jie Fu, ZnVqaWU3NEBzanR1LmVkdS5jbg==
†These authors share first authorship
Disclaimer: All claims expressed in this article are solely those of the authors and do not necessarily represent those of their affiliated organizations, or those of the publisher, the editors and the reviewers. Any product that may be evaluated in this article or claim that may be made by its manufacturer is not guaranteed or endorsed by the publisher.
Research integrity at Frontiers
Learn more about the work of our research integrity team to safeguard the quality of each article we publish.