- 1The Second Clinical Medical College, Lanzhou University, Lanzhou, China
- 2Department of Head & Neck Oncology Surgery, the Cancer Hospital of the University of Chinese Academy of Sciences (Zhejiang Cancer Hospital), Hangzhou, China
- 3Key Laboratory of Head & Neck Cancer Translational Research of Zhejiang Province, Hangzhou, China
The groundbreaking research work about SIGLEC15 has raised it as a potential promising target in cancer immunotherapy. Unfortunately, the role of SIGLEC15 in thyroid carcinoma (THCA) remains obscure. Public and home multi-omics data were collected to investigate the role of SIGLEC15 in THCA in our study. SIGLEC15 was upregulated in THCA tumor tissue compared to nontumor tissue in both mRNA and protein levels; gene set enrichment analysis (GSEA) results showed that high SIGLEC15 mRNA expression was positively correlated to many immune pathways. Results of the examination of immunological landscape characteristics displayed high SIGLEC15 mRNA expression that mainly positively correlated with a large number of cancer immunity immunomodulators and pathways. In addition, upregulation of SIGLEC15 was positively correlated with an enhanced immune score, stromal score, and estimate score. However, higher SIGLEC15 mRNA also met high immune exhausted status. The majority of CpG methylation sites negatively correlated with SIGLEC15 mRNA expression. Analysis of clinical characteristics supported increased SIGLEC15 expression that was positively correlated with more extrathyroid extension and lymph node metastasis. We observed different single nucleotide variant (SNV) and copy number variation (CNV) patterns in high and low SIGLEC15 mRNA expression subgroups; some vital DNA damage repair deficiency scores addressed a negative correlation with SIGLEC15 mRNA expression. We also found that some commonly used chemotherapy drugs might be suitable for different SIGLEC15 mRNA expression subgroups. This study highlighted the vital role of SIGLEC15 in THCA. Targeting SIGLEC15 may offer a potential novel therapeutic opportunity for THCA patients. However, the detailed exact cellular mechanisms of SIGLEC15 in THCA still needed to be elucidated by further studies.
Introduction
Thyroid cancer is a common endocrine system tumor, and its incidence rate has been increasing steadily in recent years (1–3). What was worse is that it was reported that thyroid cancer is the fifth most common cancer in women (4). Although the increase in incidence rate could be largely attributed to the improvement in the detection and diagnosis technology of papillary thyroid cancer (PTC) (diameter <2 cm), the ratio of more invasive PTC (diameter 2.1–4.0 cm and >4 cm) increased by 1.5–5 times in the past 30 years (5), even if the majority of them had excellent long-term prognoses. However, some kind of thyroid cancer may also exhibit very aggressive behavior, and the mortality rate remains stubbornly high (6). There are still a small number of patients with advanced differentiated, poorly differentiated, and undifferentiated thyroid cancer with high mortality. To date, the most efficacious approach is targeted therapy with or without surgical resection, but the curative effect is still poor. Therefore, there is an urgent need for newer therapies.
More and more studies believed that the tumor microenvironment (TME) was an essential factor that affected tumor formation, development, and prognosis (7–10). Therefore, a lot of effort has been stimulated to identify immune factors that contribute to the prognosis of cancer patients. Checkpoint inhibitors, chemotherapy drugs, radiotherapy, and antiangiogenic drugs all enhanced T-cell infiltration in the tumor microenvironment (11). Immunotherapy has become the fourth primary treatment of tumors following surgery, radiotherapy, and chemotherapy. Immune checkpoint inhibitors, especially, have accomplished unprecedented success in the clinical treatment of multiple cancer types. There was also a growing body of cancer clinical trials that were approved by the US Food and Drug Administration (FDA) in which a single-agent checkpoint inhibitor or a kind of combination of checkpoint blockades was a treatment (12–14), demonstrating the breadth of interest from scientists and pharmaceutical factories in immuno-oncology and the great potential for additional immunotherapeutics.
Immune checkpoint-blocking therapy such as cytotoxic T lymphocyte-associated antigen 4 (CTLA4) (15, 16), programmed cell death protein 1 ligand 1 (PD-L1), and programmed cell death 1 (PD-1) have been widely used in many types of solid tumors (17, 18). Although anti-PD-1/PD-L1 therapy was the most famous and clinically effective immunotherapy, its effectiveness in human solid tumors remains only 20%–30% (19). In recent years, immunotherapy has been successfully applied to the treatment of advanced differentiated thyroid cancer and anaplastic thyroid cancer and changed the treatment paradigm (20, 21).
SIGLEC15, an alias of CD33L3 and HsT1361, belonged to the sialic acid-binding immunoglobulin-like lectin family. Angata et al. (22) first identified SIGLEC15 in 2007 and inferred that it probably played a conserved regulatory role in the immune system of vertebrates. The following studies demonstrated that SIGLEC15 played an important role in the development and differentiation of osteoclastogenesis, and it could also act as a potential therapeutic target with its versatile role of suppressed bone resorption but also facilitated bone remodeling (23–25). Jaeger et al. (26) identified SIGLEC15 as a susceptibility factor in recurrent vulvovaginal candidiasis. Wang et al. (27) first supported that SIGLEC15 could be an immune suppressor and potential target for normalization cancer immunotherapy by using a genome-scale T-cell activity array in 2019, and they also revealed upregulation of SIGLEC15 on various cancer types. Moreover, SIGLEC15 had unique molecular features when compared with the majority of known checkpoint inhibitory ligands and a mutually exclusive expression with PDL1, proposing that it could be a critical immune evasion mechanism in PD-L1-negative patients (27). SIGLEC15 was proven to be an immune suppressor in the premetastatic lymph node of colorectal cancer (28). Several studies displayed the complicated function of SIGLEC15 and validated that SIGLEC15 could act as a potential immunotherapeutic target for pancreatic ductal adenocarcinoma (29, 30). SIGLEC15 shaped a non-inflamed TME and predicted the molecular subtypes in bladder cancer (31). Furthermore, SIGLEC15 acted as a mediator of LINC00973t to suppress immune in clear-cell renal cell carcinoma (32). Most importantly, in a phase I clinical trial of SIGLEC15-positive patients who were diagnosed with advanced/metastatic solid tumors refractory or resistant to currently available therapies with a tumor proportion score Tumor Proportion Score (TPS) PDL1 score <50% could benefit from NC318 (anti-SIGLEC15 antibody) (33).
In the present study, we aimed to decipher the comprehensive picture of the role of SIGLEC15 in thyroid carcinoma (THCA) by datamining the well-known multi-omics databases, such as The Cancer Genome Atlas (TCGA) and Gene Expression Omnibus (GEO), and validated it in our own dataset by experiments.
Materials and methods
Data acquisition
We obtained TCGA and Genotype-Tissue Expression (GTEX) RNA sequencing data (FPKM), clinical data, and DNA methylation data from xenabrowser (https://xenabrowser.net/datapages/) (34, 35), and FPKM values were transformed to log2(TPM + 1) values. SIGLEC15 mRNA differential expression from the Pan-Cancer Analysis of Whole Genomes (PCAWG) was completed by R package UCSCXenaShiny (36). GEO datasets (GSE3467, GSE3678, GSE29265, GSE33630, GSE60542, GSE65144, GSE97001) were downloaded from GEO database (https://www.ncbi.nlm.nih.gov/geo/) (37). R package limma was used to get the mean value of repeated probes in GEO datasets (38). Wilcoxon rank-sum test was used to compare the differential expression of SIGLEC15 between tumor and normal samples in both The Cancer Genome Atlas, and GEO is Gene Expression Omnibus and GEO datasets; R package ggpubr and ggplot2 were used to visualize the difference (38). R package survival was used to explore the relationship between SIGLEC15 expression and overall survival. Somatic mutation MAF (Varscan2 version) file was downloaded from the GDC data portal (https://portal.gdc.cancer.gov/) and presented gene mutation between high and low SIGLEC15 (cut by median value of SIGLEC15) (39). Copy number information was harvested from Masked Copy Number Segment by R package TCGAbiolinks (40), then separated into two files by high and low SIGLEC15 (cut by median value of SIGLEC15); these two files were used as input for the online tools GenePattern module GISTIC_2.0 (version 6.15.28) (https://www.genepattern.org/#) to visualize the copy number variation (CNV) difference (41). We downloaded the genomic and molecular landscape of DNA damage repair deficiency scores file (42) and explored the correlation with SIGLEC15 mRNA expression. Online database Tumor Immune Single-cell Hub (TISCH) was conducted to explore the expression cell type of SIGLEC15 (43).
SIGLEC15 mRNA expression correlation with DNA methylation and N6-Methyladenosine regulator mRNA expression
We extracted DNA methylation site beta values 2 kb upstream to 0.5 kb downstream of the transcription start site (TSS) of gene SIGLEC15, then conducted a Spearman correlation analysis between SIGLEC15 DNA methylation and mRNA expression (including gene level and site level; gene level is the mean value of all site values). We also explored the correlation between SIGLEC15 mRNA expression and N6-methyladenosine (m6A) genes (including 13 readers, eight writers, and two erasers) (44).
Functional enrichment analysis
We used Wilcoxon rank-sum test to find the differentially expressed genes between high and low SIGLEC15 (cut by median value of SIGLEC15) groups and visualized by R package pheatmap. Gene set enrichment analysis (GSEA) software (GSEA v4.2.3) (45, 46), h.all.v7.5.1.symbols.gmt, and c5.go.v7.5.1.symbols.gmt were harvested from msigdb (http://www.gsea-msigdb.org/gsea/downloads.jsp) and then for GSEA with the high and low SIGLEC15 mRNA expression groups. Significant signaling pathways were selected by criteria false discovery rate (FDA) <0.25 and p-value <0.05.
Protein–protein interaction (PPI) network and hub genes
Significant differentially expressed genes between high and low SIGLEC15 (cut by median value of SIGLEC15) groups were based on the criteria of false discovery rate <0.05 and absolute value log2 fold change >1, then the selected genes were input into SRTING (v11.5, http://string-db.org/) for the retrieval of protein–protein interaction network information (47). A cutoff of 0.4 for minimum interaction score was set to get the biological functions with disconnected nodes hidden from the network, and the interaction file acted as input for Cytoscape3.9.1 to visualize the interaction network of these proteins (48); plug-in CytoHubba was applied to get hub genes with default parameters (49).
Evaluation of the immunological landscape characteristics with SIGLEC15 of the thyroid carcinoma
To decipher the immunological landscape characteristics of the TME in THCA, we firstly gained 122 immunomodulator genes [major histocompatibility complex (MHC), receptors, chemokines, and immune stimulators] (50), 47 immune checkpoint (ICP) genes, and 25 immunogenic cell death (ICD) genes (51). We displayed the different expressions between high and low SIGLEC15 (cut by median value of SIGLEC15) groups or correlation with gene SIGLEC15. The activity of the cancer immunity cycle data was obtained from online website TIP (http://biocc.hrbmu.edu.cn/TIP/index.jsp) (52) and immune features from the online website iAtlas Explorer (https://isb-cgc.shinyapps.io/iatlas/) were downloaded (53) and then were visualized by R package ggpurb between high and low SIGLEC15 (cut by median value of SIGLEC15) groups. R package estimate was used to output the estimated levels of infiltrating stromal and immune cells and calculated stromal score, immune score, and estimate score (54). The TIMER website (https://cistrome.shinyapps.io/timer/) was utilized to validate the influence of SIGLEC15 expression on immune cell infiltration in THCA (55). We also used single-sample gene set enrichment analysis (ssGSEA) to compare the immune infiltration scores between high and low SIGLEC15 (cut by median value of SIGLEC15) groups (56). The Tumor Immune Dysfunction and Exclusion (TIDE) score and exclusion score were evaluated using an online database (http://tide.dfci.harvard.edu/) (57).
Tissue microarray analysis
This study was approved by the institutional review board of Zhejiang Cancer Hospital. The informed consents were signed from all subjects in the study. The tissue microarray (TMA) chips were obtained from Wuhan Xavier Biotechnology Co., Ltd. A total of 110 thyroid cancer tissue specimens and 54 adjacent tissue specimens were obtained; each formalin-fixed paraffin-embedded block was cut into 4-µm-thick sections for arraying. SIGLEC15 antibody (GTX32061, GeneTex, CA, USA) was used for immunohistochemistry (IHC); representative cancer tissue areas were marked on hematoxylin–eosin-stained slides, and TMA construction sampling was performed using tissue chip scanner (3DHistech®, Pannoramic MIDI, Hungary). The Densito Quant software in Quant Center was used to automatically identify and set all dark brown on the tissue section as strong positive, brown yellow as medium positive, light yellow as weak positive, and blue nucleus as negative. Then, each tissue point was identified; the strong positive, medium positive, weak positive, and negative areas (unit: pixel) were analyzed; and the positivity percentage and histochemistry score (H-score) were calculated.
Evaluation of potential chemotherapy drugs to SIGLEC15 mRNA expression
Cellminer™ database [Version: 2022.1 (database: 2.8.1)] was used for the research of pharmacological data of the NCI-60 cancerous cell lines (58).
Statistical analysis
Statistical analysis was finished with R software (v4.1.1, https://www.r-project.org/) and its corresponding packages. Comparison between two groups was conducted utilizing Wilcoxon rank-sum test, and Kruskal–Wallis test was carried out for normal multiple groups. Spearman correlation test was adopted to determine the correlation between variables. Fisher exact test was performed to analyze the correlation between SIGLEC15 and clinicopathologic characteristics. p-value <0.05 was set as the threshold; if not specially noted, ns, *, **, ***, and **** stand for p-value >0.05, p-value <=0.05, pvalue <=0.01, pvalue <=0.001 and pvalue <=0.0001, respectively.
Results
Analysis of expression of SIGLEC15 in thyroid carcinoma samples
Figure 1 showed the workflow of this study, which was presented for SIGLEC15 differential expression, immune genes, cells, pathways, immune infiltration scores, clinical features, mutations, CNV, DNA methylation, and m6A genes with SIGLEC15. After a comprehensive analysis of the expression data from The Cancer Genome Atlas, and GEO is Gene Expression Omnibus, Genotype-Tissue Expression, and PCAWG database, we found that SIGLEC15 was highly expressed in THCA compared with normal tissues in all databases (Figure 2A; Supplementary Figure S1A). In addition, SIGLEC15 mRNA expression from database The Cancer Genome Atlas, and GEO is Gene Expression Omnibus of THCA in tumor and normal samples was shown in Figure 2B. Paired tumor and normal samples in Supplementary Figure S1B, SIGLEC15 was also found to be highly expressed in tumor samples in gene expression profiles (GSE3467, GSE3678, GSE29265, GSE33630, GSE60542, GSE65144, GSE97001) from the GEO database in Figure 2B. The expression level and the positive rates of SIGLEC15 were compared between thyroid cancer tissue and adjacent normal tissue samples by immunohistochemistry on microarray. Figures 2C, D were a representative area of TMA, a classic pair of samples. Adjacent tissue had lower positive staining (Figure 2E) than thyroid cancer tissue (Figure 2F); the concrete H-score was in Table 1.
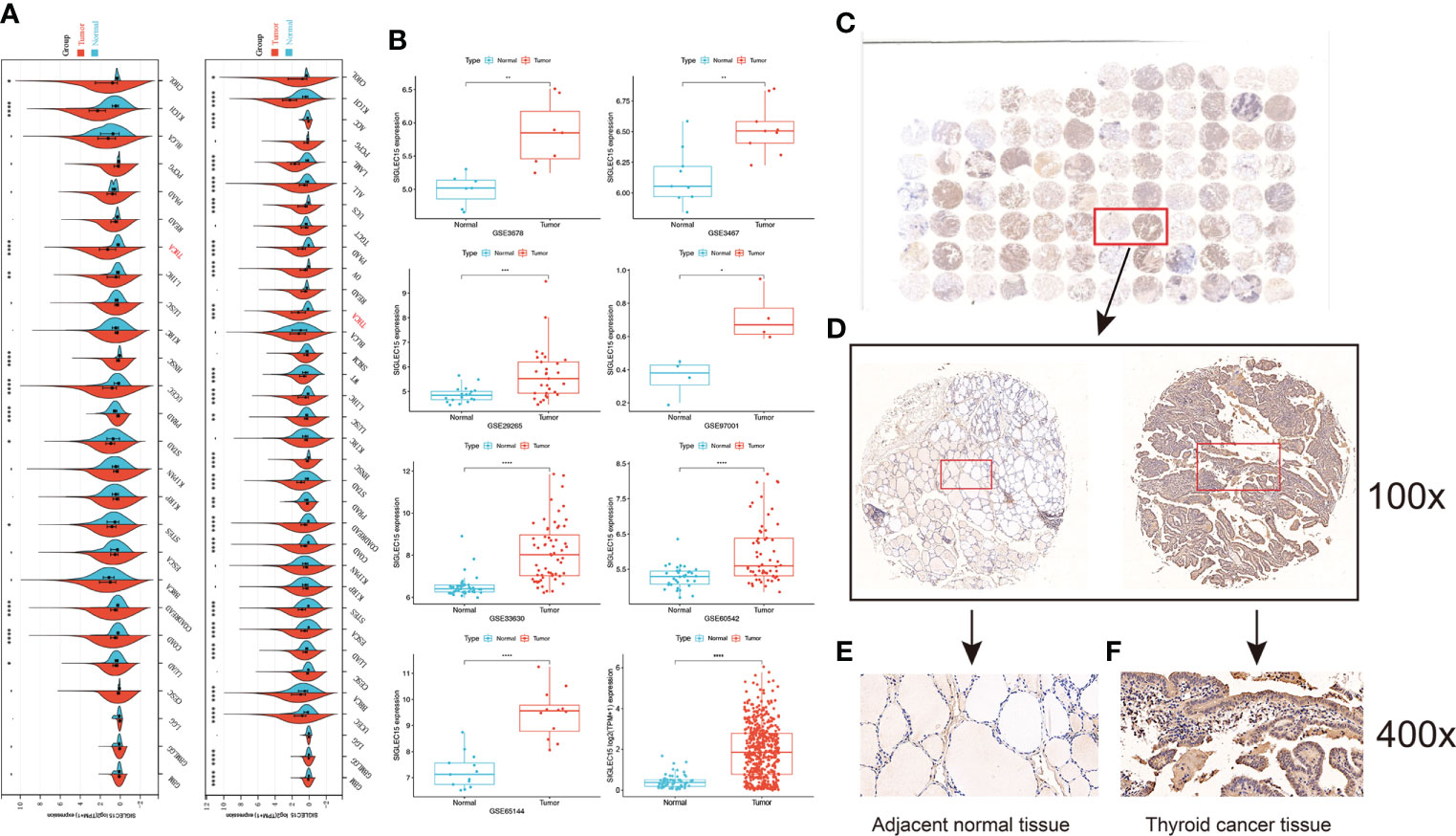
Figure 2 Analysis of the expression of SIGLEC15. (A) Pan-cancer mRNA expression of SIGLEC15 between tumor and normal tissues from The Cancer Genome Atlas, and GEO is Gene Expression Omnibus and Genotype-Tissue Expression database. (B) mRNA expression of SIGLEC15 between tumor and normal tissues from GEO and The Cancer Genome Atlas, and GEO is Gene Expression Omnibus database. (C) A classic pair of samples. (D–F) Left sample was adjacent normal tissue. Right sample was thyroid carcinoma tissue. Thyroid carcinoma tissue has higher SIGLEC15-positive staining than the adjacent normal tissue. ns, *, **, ***, and **** stand for p-value >0.05, p-value <=0.05, p-value <=0.01, pvalue <=0.001 and pvalue <=0.0001, respectively.
Differential genes, signaling pathways, and hub genes associated with SIGLEC15 mRNA expression groups
We obtained significant differentially expressed genes between high and low SIGLEC15 groups. The 20 most highly and lowly expressed genes were presented, and we noted that the SIGLEC family genes SIGLEC15 and SIGLEC6 were in the 20 most highly expressed genes in tumor samples (Figure 3A). We further analyzed the signaling pathways involving SIGLEC15 via GSEA; high SIGLEC15 mRNA expression was positively correlated with pathways such as the regulation of adaptive immune response, positive regulation of cytokine production, T cell-mediated immunity (Figure 3B), inflammatory response, interferon alpha response, and interferon gamma response (Figure 3C). Thereafter, we used the methods mentioned above to identify hub genes; 10 hub genes were harvested, as Figure 3D presented; several CXC family genes were in the hub gene list, such as genes CXCL1, CXCL2, and CXCL8 (Figure 3D).
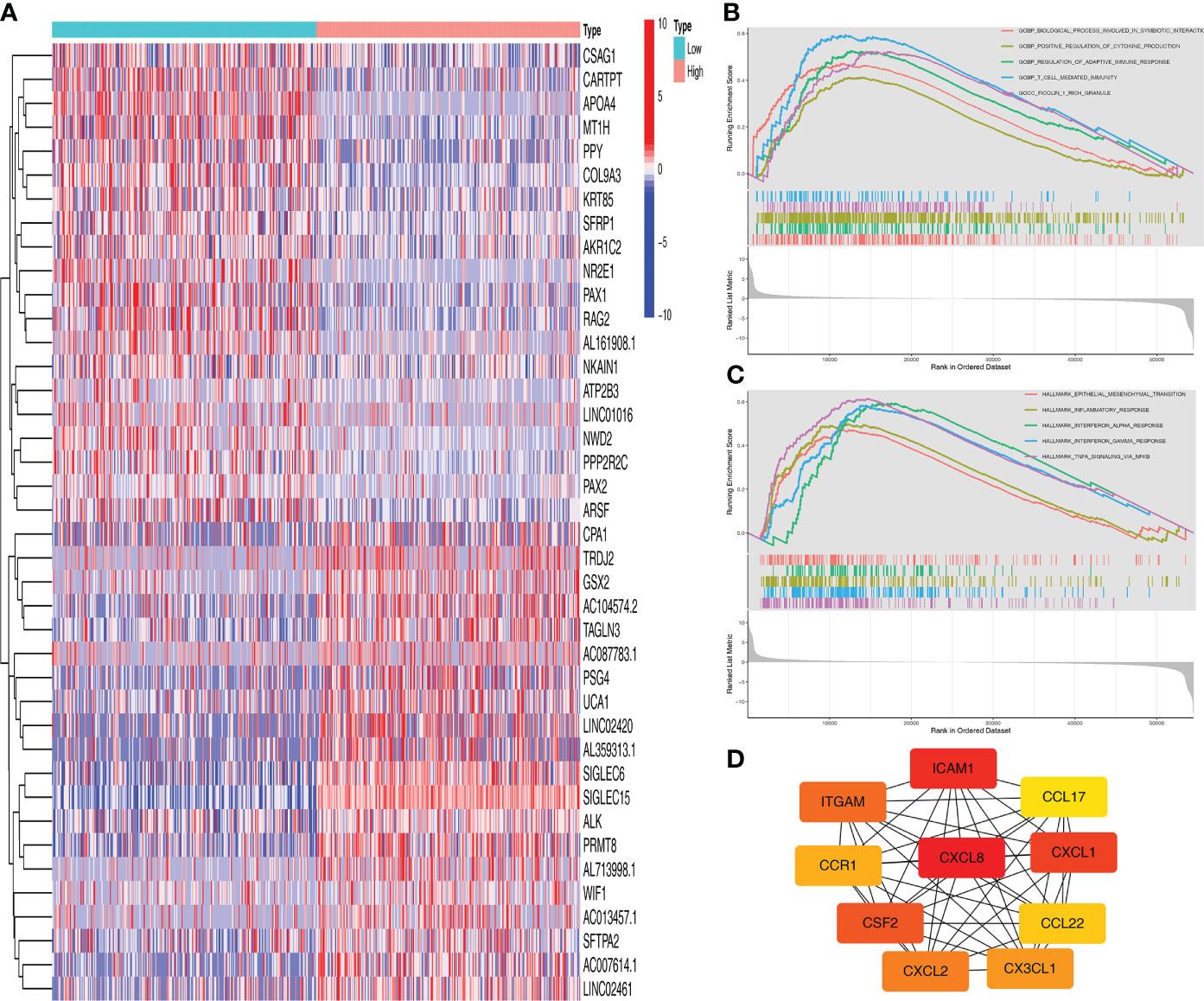
Figure 3 Functional enrichment analysis of SIGLEC15. (A) Top 20 differentially expressed genes between high and low SIGLEC15 groups. (B, C) GSEA for the signaling pathways activated in the high SIGLEC15 mRNA expression group with (B) GO pathways and (C) HALLMARK pathways. (D) Hub genes related to SIGLEC15.
Immunological and biological significance of SIGLEC15 in thyroid carcinoma
The majority of 122 highly expressed immunomodulators were found in the high SIGLEC15 mRNA expression group, such as MHC family member genes, CXC family member genes, CXC chemokine receptors that played important roles in cancer immunity (Figure 4A), and ICPs and ICD genes, which played critical roles in modulating the host antitumor immunity. SIGLEC15 had a positive correlation with most of the ICPs, and some had a significant positive correlation (e.g., CD200, CD276, CD40) but had no significant correlation with PDCD1 (PD-1) (Figure 4B). SIGLEC15 also had a significant positive correlation with some ICDs (e.g., ANXA1, MET) and significant negative correlation with CALR (Figure 4C). We also found a significant positive correlation between SIGLEC15 and CD44 (Supplementary Figure S1C; Supplementary Table S1). Systematically tracking the activity of anticancer immunity and the extent of tumor-infiltrating immune cells were important for cancer immunotherapy. The majority of the steps of the cancer immunity cycle were found to be significantly upregulated, including step 3 (priming and activation), step 5 (infiltration of immune cells into tumors), and most parts of step 4 (trafficking of immune cells to tumors) (Figure 5A). Furthermore, we also assessed the correlation between SIGLEC15 mRNA expression and 56 previously defined immune-related molecular features; the expressions of 11 molecular features were significantly higher in the high SIGLEC15 group, including Dendritic Activated, IFN gamma Response, and Leukocyte Fraction (Supplementary Figures S2A–K). We further evaluated the correlation between SIGLEC15 expression and immunocyte infiltration and observed that SIGLEC15 significantly positively correlated with the infiltration of B cells, CD4 T cells, macrophages, neutrophils, and dendritic cells (Figure 5B). Moreover, the high SIGLEC15 group employed a higher stromal score, immune score, and estimate score (Figure 5C). We also used the ssGSEA algorithm to calculate immunocyte infiltration; it was easy to see that all of the 16 immune cells and 13 immune-related pathway scores were significantly upregulated in the high SIGLEC15 group (Figures 5E, F). Although the high SIGLEC15 group had a higher proportion of immunocytes and an elevated level of immune checkpoints, we also observed that the high SIGLEC15 group linked with an increased score of TIDE, immune exclusion by TIDE database (Figure 5D). Moreover, by integrating some known gene sets correlated with exhausted immunity, we found that although patients with high SIGLEC15 had a high proportion of immunocytes, they also had higher scores of immune checkpoint blockade (ICB) resistance, exhausted CD8, T-cell exhaustion, immune checkpoint, and T-cell regulatory, which would lead to immune exhaustion. So, high SIGLEC15 subgroup patients met immune exhaustion, which indicated that patients with a low SIGLEC15 expression could benefit more from Immune checkpoint inhibitor (ICI) therapy than patients with a high SIGLEC15 expression (Supplementary Figure S2L). We also figured out that mainly immune cells express SIGLEC15, especially on Monocytes and Macrophages (Supplementary Figure S3A).
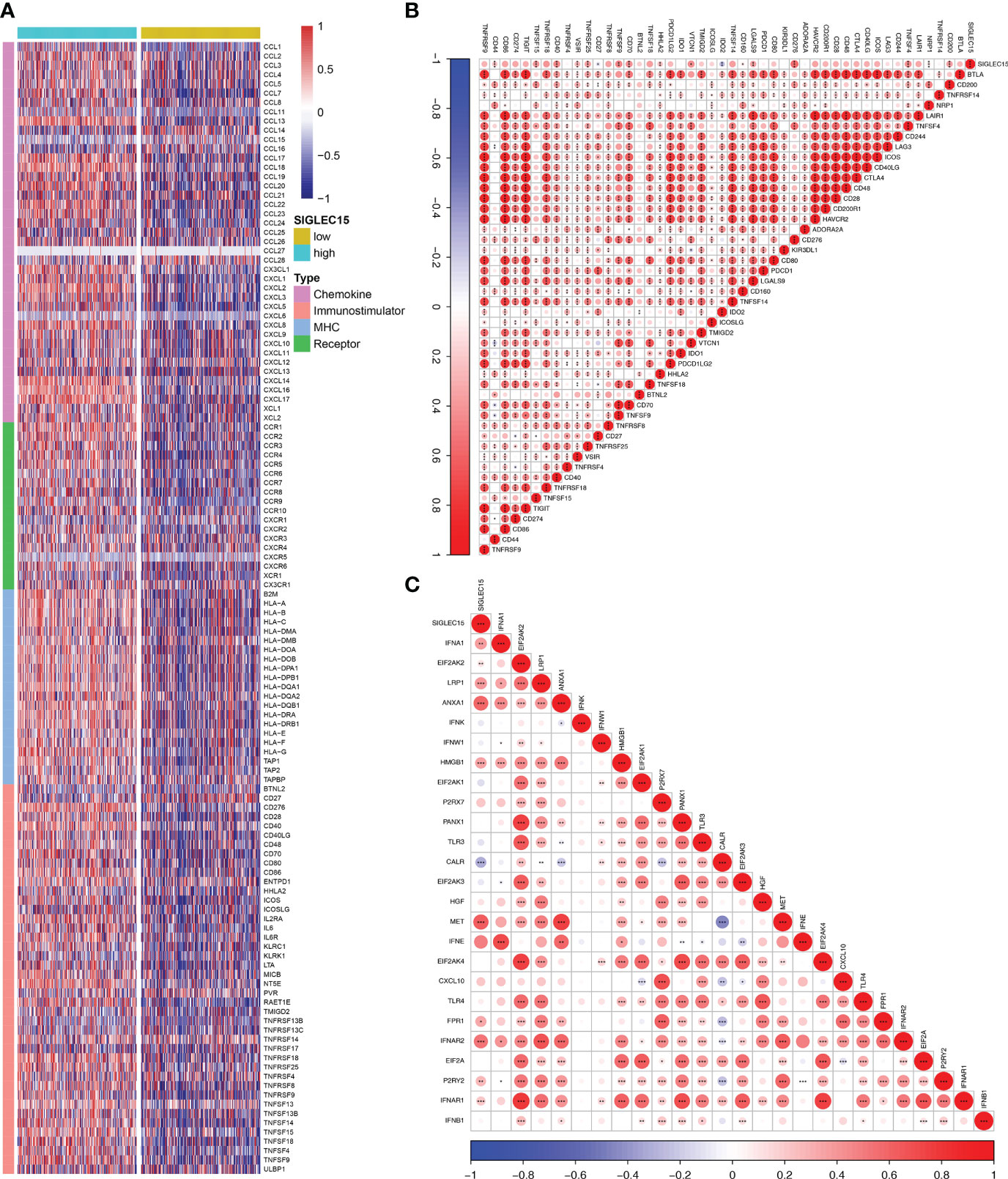
Figure 4 Immunological gene patterns related to SIGLEC15. (A) Differential expression of 122 immunomodulators (chemokines, receptors, MHC, and immunostimulators) between the high and low SIGLEC15 groups. (B) ICP modulator relationship with SIGLEC15. (C) ICD modulator relationship with SIGLEC15. ns, *, **, and *** stand for p-value ≤0.05, p-value ≤0.01, pvalue ≤0.001.
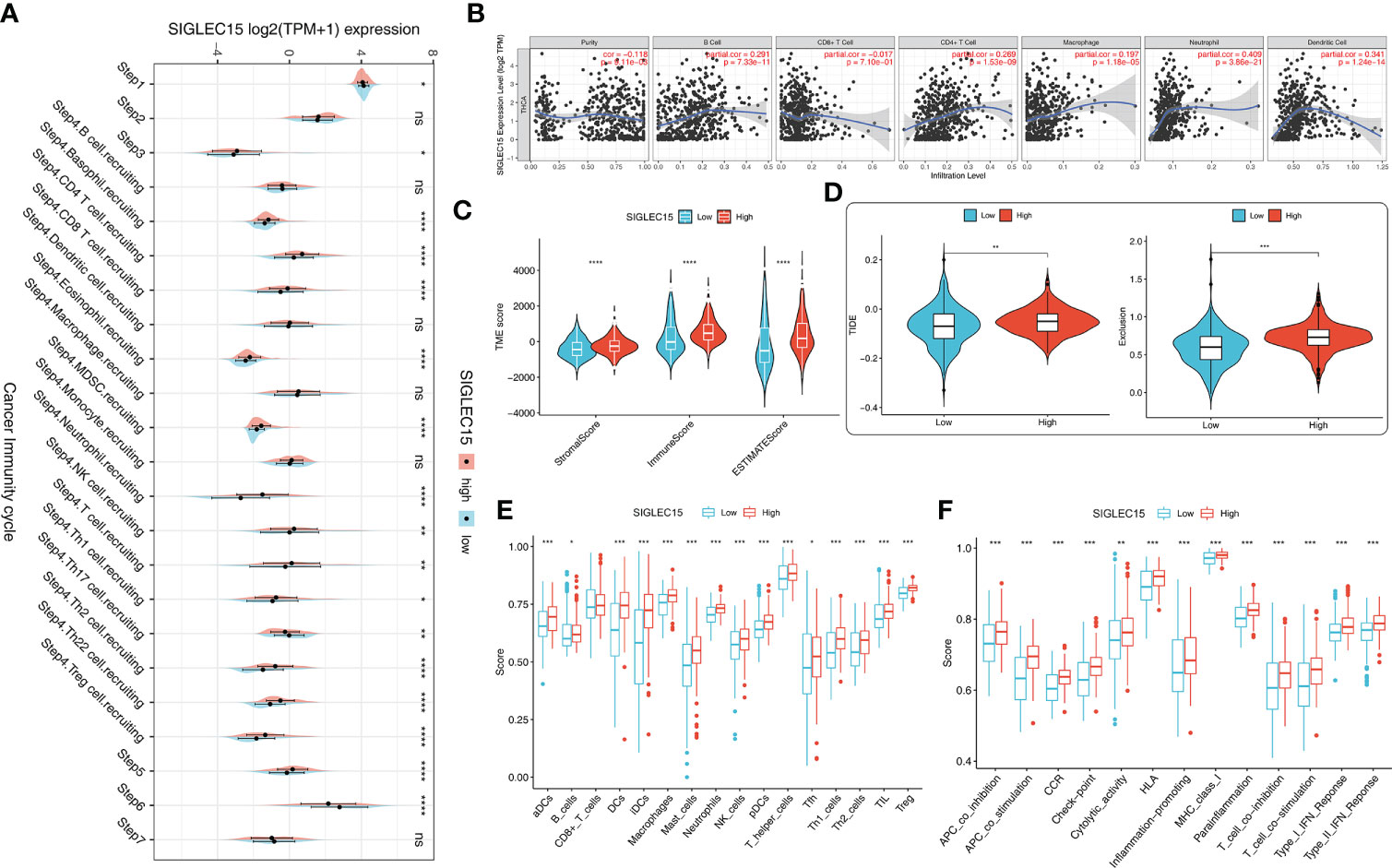
Figure 5 Immunological cell patterns related to SIGLEC15. (A) Differences in the seven steps of the cancer immunity cycle between the high and low SIGLEC15 groups. (B) SIGLEC15 was associated with immune cell infiltration in THCA obtained from the TIMER database. (C) TME scores were compared between the high and low SIGLEC15 groups. (D) Scores were compared between the low and high SIGLEC15 groups in TIDE score and Exclusion score. (E) Enrichment scores for 16 immunocytes were compared between the low and high SIGLEC15 groups. (F) Enrichment scores for 13 immune-related pathways were compared between the low and high SIGLEC15 groups. ns, *, **, ***, and **** stand for p-value >0.05, p-value <=0.05, p-value <=0.01, pvalue <=0.001 and pvalue <=0.0001, respectively.
SIGLEC15 mRNA expression correlates with methylation
m6A RNA methylation was a kind of epigenetic modification measured by methyltransferases, demethylases, and binding proteins, which were also called “writers,” “erasers,” and “readers.” We conducted the relationship analysis of these regulated genes with SIGLEC15 mRNA expression levels; it could be easily seen that the majority of reader genes had a significant positive correlation with SIGLEC15 (Figure 6A), and all of the writer genes except RBM15 had a significant positive correlation with SIGLEC15 (Figure 6B). However, eraser genes had no significant correlation with SIGLEC15 (Figure 6C). We also analyzed the extent to which SIGLEC15 mRNA expression correlated with CpG methylation and the whole CpG methylation site levels of SIGLEC15, which varied from a broad scope (Figure 6D). Interestingly, the averaged SIGLEC15 promoter and body hypermethylation were associated with decreased SIGLEC15 mRNA expression, indicated by a strong negative correlation coefficient (Figure 6E). Moreover, we measured each CpG methylation site level with SIGLEC15 mRNA expression levels; we found that six out of nine sites had a negative correlation with SIGLEC15, and CpG methylation site cg05752393 had a positive correlation with SIGLEC15 (Figures 6F–L). However, the CpG methylation site cg13741394 and cg00425636 had no significant correlation with SIGLEC15 (Figures 6M, N).
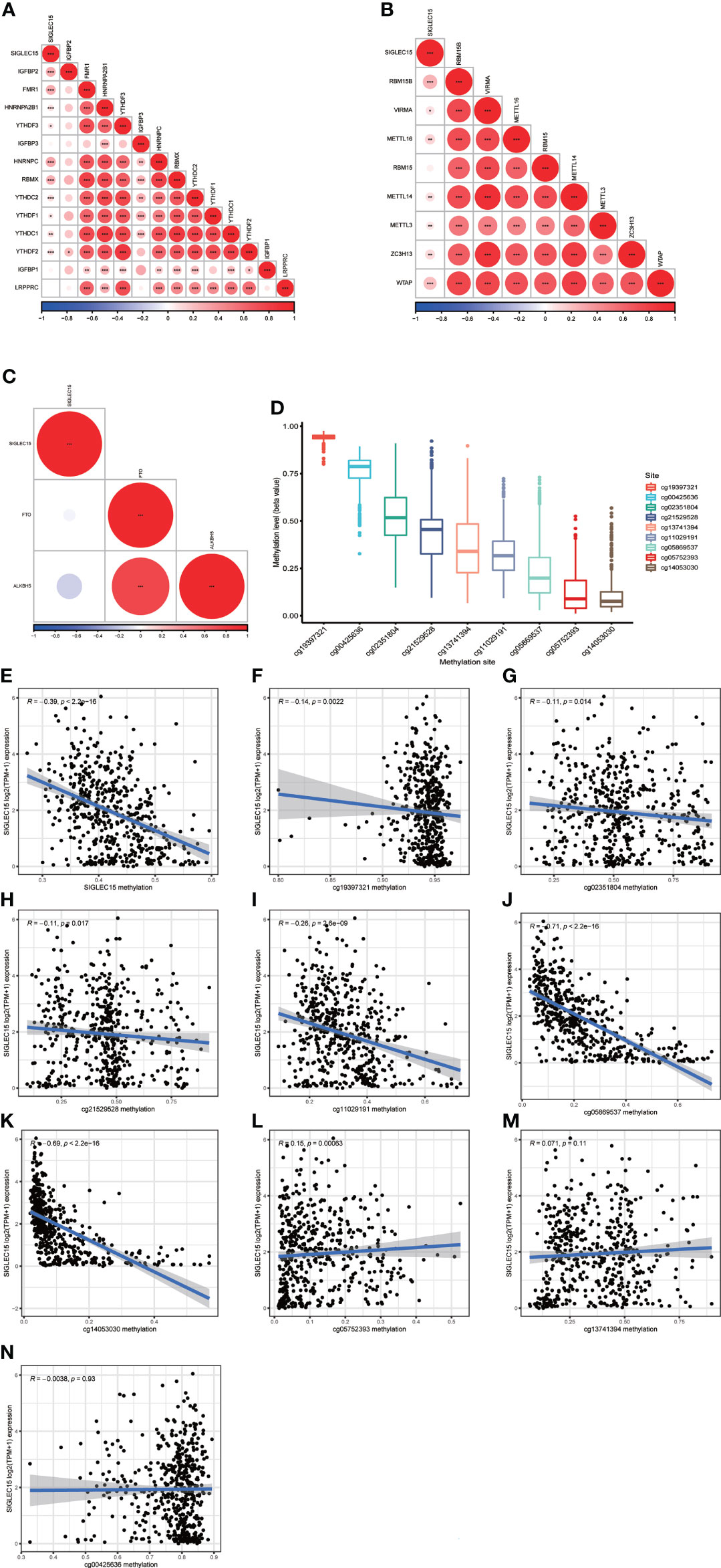
Figure 6 SIGLEC15 mRNA expression correlation with DNA methylation and m6A regulator mRNA expression. (A–C) SIGLEC15 mRNA expression correlation with (A) m6A readers, (B) writers, and (C) erasers. (D) Each CpG methylation site level of SIGLEC15. (E) SIGLEC15 mRNA expression correlation with averaged CpG methylation site. (F–N) SIGLEC15 mRNA expression correlation with DNA methylation of each CpG methylation site.
Clinical significance of SIGLEC15
We quantified vital clinical feature associations with SIGLEC15 mRNA expression in THCA, and stage, lymph node metastasis (N stage), extrathyroid extension, and BRAF V600E status were found to be positively correlated with SIGLEC15 mRNA expression levels; other clinical factors (e.g., age, gender, residual tumor) indicated no significant relationship with SIGLEC15 mRNA expression (Figures 7A–I). Furthermore, we analyzed the clinical feature associations with SIGLEC15 IHC level, and we revealed that N stage and extrathyroid extension were positively related to SIGLEC15 in Table 2. However, there was no significant relationship between SIGLEC15 expression and overall survival (Supplementary Figure S4A).
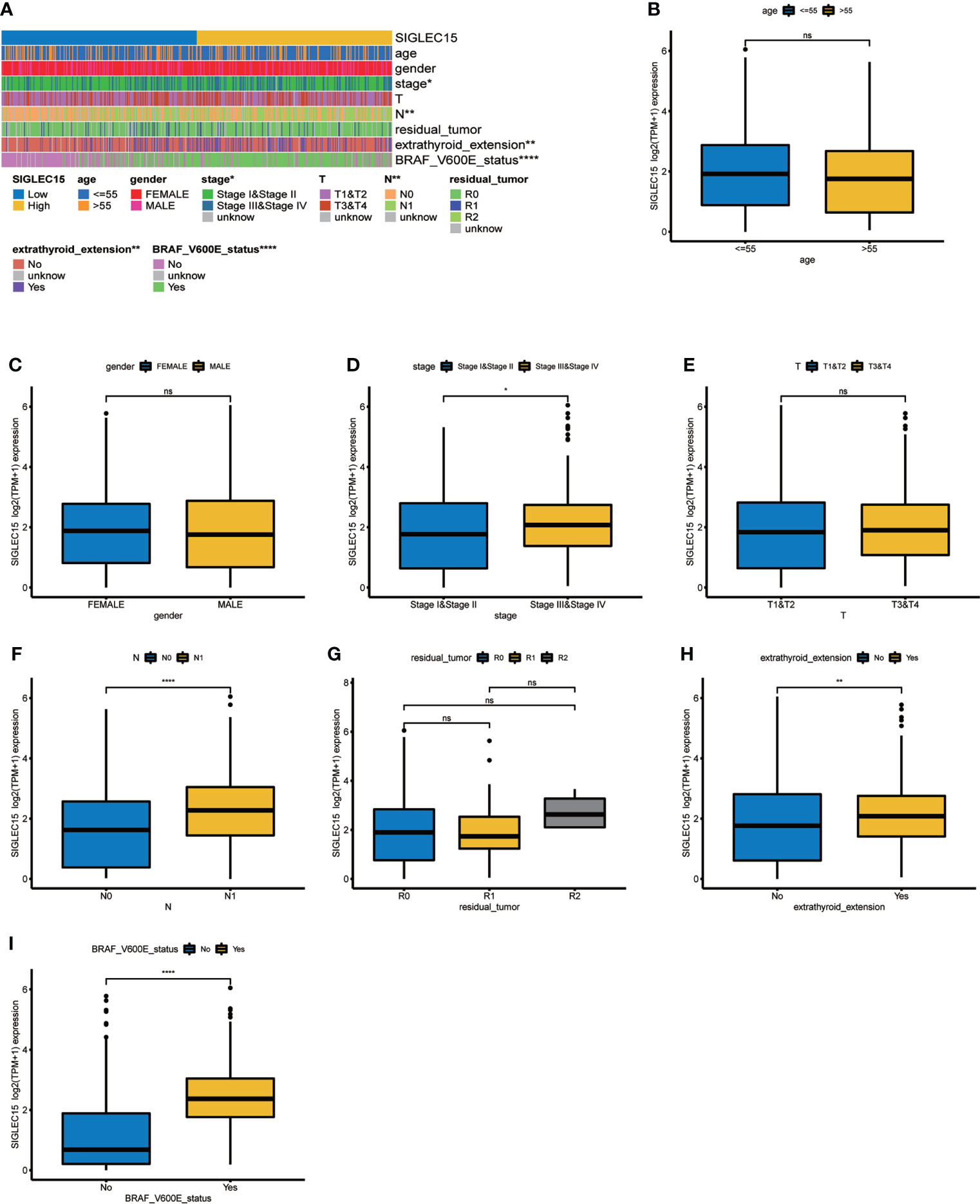
Figure 7 Clinical significance of SIGLEC15. (A) Heatmap of clinical feature correlation with SIGLEC15. (B–I) High and low SIGLEC15 mRNA expression group difference in (B) Age, (C) Gender, (D) Stage, (E) T stage, (F) N stage, (G) Tumor residual size, (H) Extrathyroid extension, and (I) BRAF V600E status. ns, *, **, ***, and **** stand for p-value >0.05, p-value <=0.05, p-value <=0.01, pvalue <=0.001 and pvalue <=0.0001, respectively.
Mutational analyses of SIGLEC15 in thyroid carcinoma
No mutations were found in the MAF file of the gene SIGLEC15 of THCA patients produced by varscan2 software obtained from The Cancer Genome Atlas, and GEO is Gene Expression Omnibus. We also investigated mutational profiles of low and high SIGLEC15 groups; it could be clearly seen that more patient samples in the high SIGLEC15 group had gene BRAF mutations. Moreover, the majority of mutations in patient samples of the high SIGLEC15 group were located in gene BRAF (Figure 8A); patient samples in the low SIGLEC15 group had mutations in genes BRAF, NRAS, and HRAS (Figure 8B), not focused as that in the high SIGLEC15 group. The GISTIC2.0 results indicated that amplification displayed a lower frequency in the high SIGLEC15 mRNA expression group compared to the low SIGLEC15 expression group (Figures 8C, D) and similar frequency of deletion in the two groups (Figures 8C, D). Furthermore, we calculated the G-score, which also showed more amplification events in the low SIGLEC15 expression group (Supplementary Figures S5A, S5B).
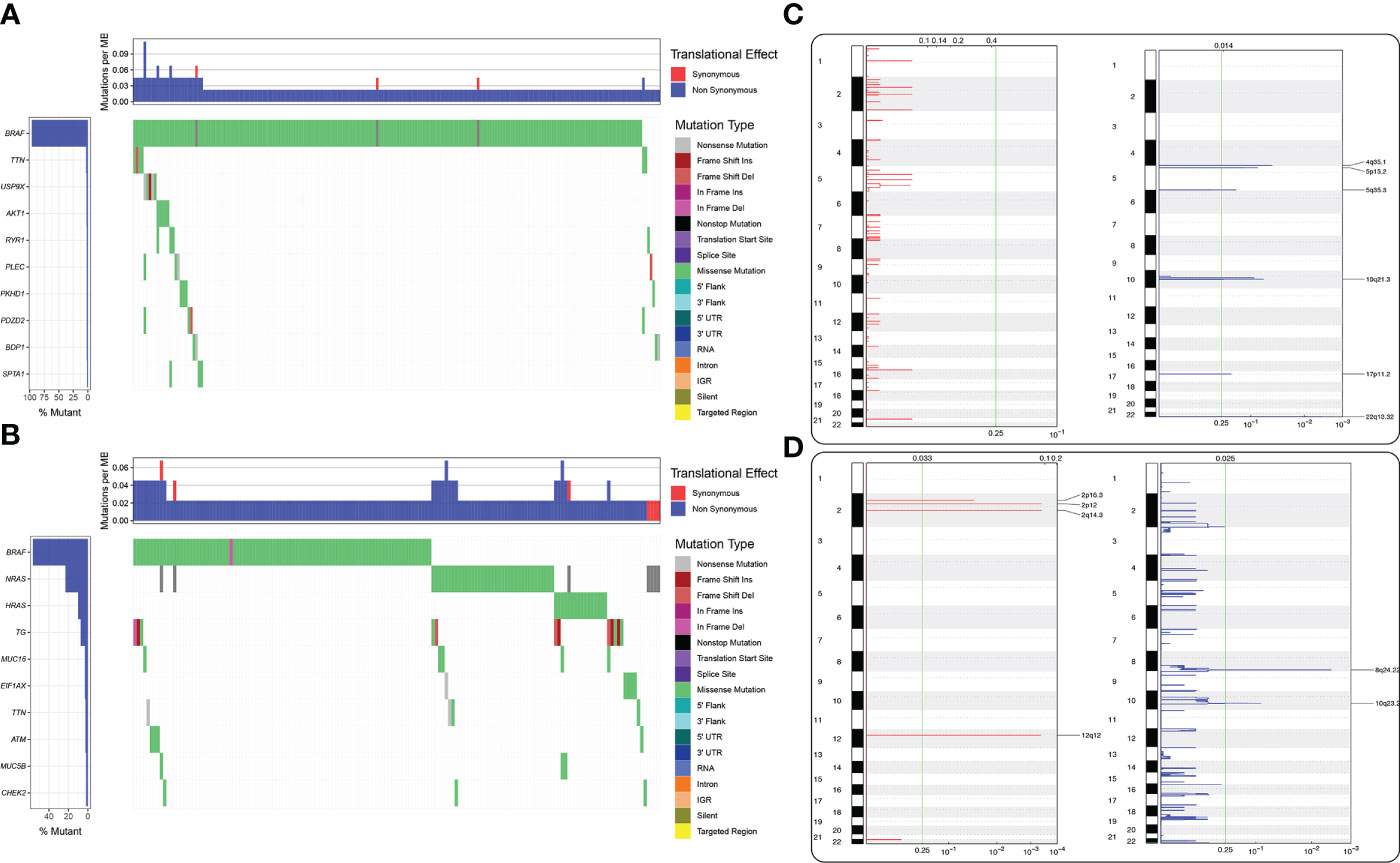
Figure 8 Differential mutational landscape in the high and low SIGLEC15 mRNA expression groups. (A) Genes were ranked according to the mutational frequency. SNV and Indel mutations in the high SIGLEC15 mRNA expression group. (B) Genes were ranked according to the mutational frequency. SNV and Indel mutations in the low SIGLEC15 mRNA expression group. (C, D) CNV landscape of (C) amplification and (D) deletion in the high and low SIGLEC15 mRNA expression groups; the chromosome was oriented vertically from top to bottom and GenePattern GISTIC2.0 q-value at each locus was placed from left to right. The green line displayed the cutoff value of q-value = 0.25.
DDR deficiency association with SIGLEC15
DDR genes played vital roles in maintaining genomic stability, so the relationship between DDR deficiency scores and SIGLEC15 was evaluated. We observed that many scores had a negative correlation with SIGLEC15, aneuploidy score prime (correlation coefficient = -0.23) (Figure 9A), aneuploidy score (correlation coefficient = -0.18) (Figure 9B), CNA frac altered (correlation coefficient = -0.19) (Figure 9C), LOH frac altered (correlation coefficient = -0.15) (Figure 9D), expression CDF trAnsform of Rank Distribution (eCARD) (correlation coefficient = -0.22) (Figure 9E), and repair proficiency scoring (RPS) (correlation coefficient = -0.24) (Figure 9F); nevertheless, PARPi7 (7-gene DNA repair deficiency expression signature) had a positive correlation with SIGLEC15 mRNA expression (correlation coefficient = 0.34) (Figure 9G).
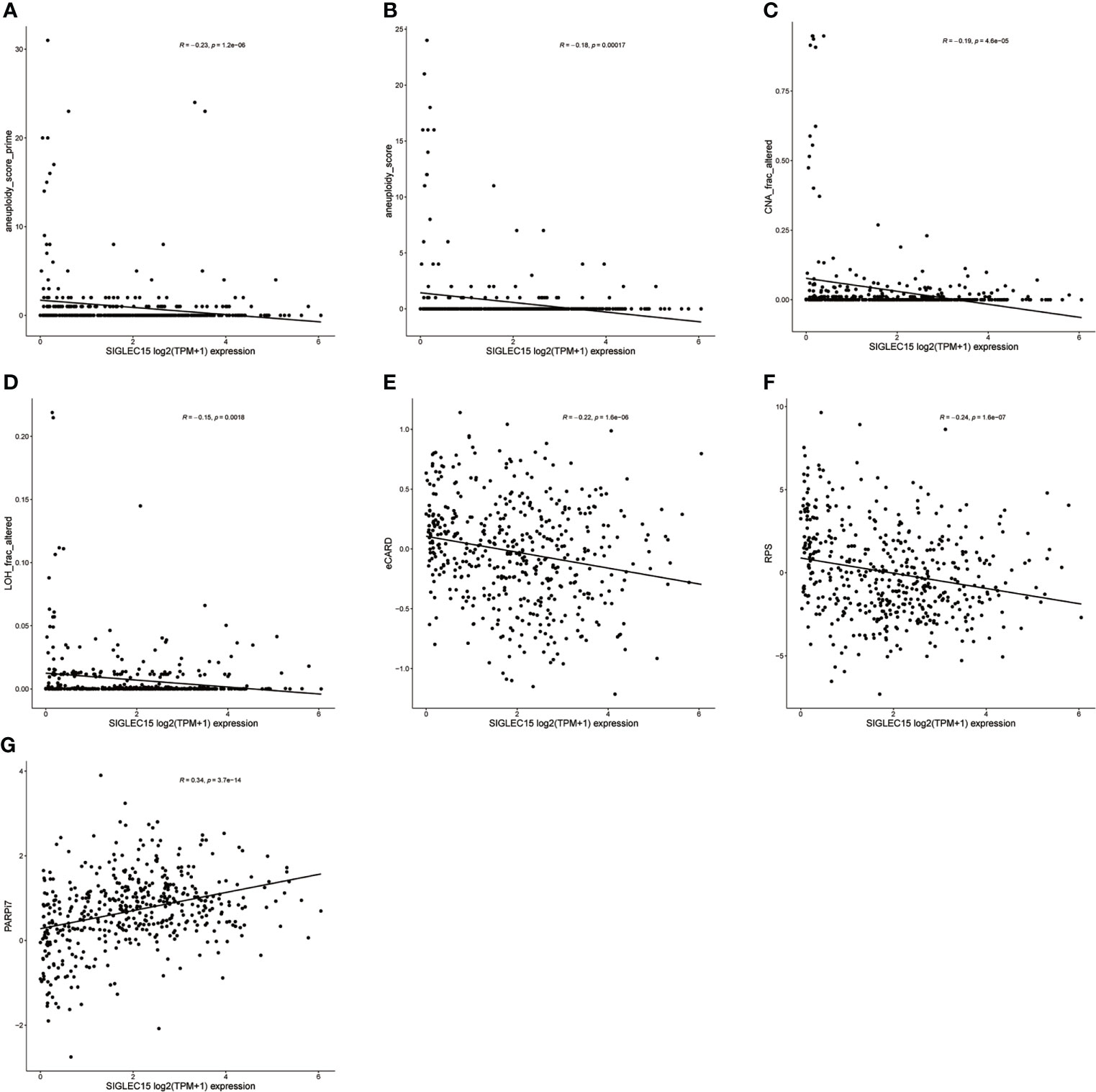
Figure 9 DDR deficiency score correlation with SIGLEC15 mRNA expression. (A–G) SIGLEC15 mRNA expression with score of (A) aneuploidy score prime, (B) aneuploidy score, (C) CNA frac altered, (D) LOH frac altered, (E) eCARD, (F) RPS, and (G) PARPi7.
Potential chemotherapy drugs for SIGLEC15 determined thyroid carcinoma progress
Combining chemotherapy drugs with a single-agent immune checkpoint therapeutic approach may enhance antitumor immune response and overcome primary resistance. We revealed that SIGLEC15 mRNA expression was negatively associated with the IC50 of tyrothricin, estramustine, pipamperone, fulvestrant, and salinomycin and implied that these selected chemotherapeutic drugs may be suitable for the treatment of those with a high expression level of SIGLEC15, while selected chemotherapeutic drugs like pelitrexol, triciribine phosphate, staurosporine, dasatinib, amonafide, and midostaurin might exert an opposite effect for the treatment of those with a high expression level of SIGLEC15 (Figures 10A–K).
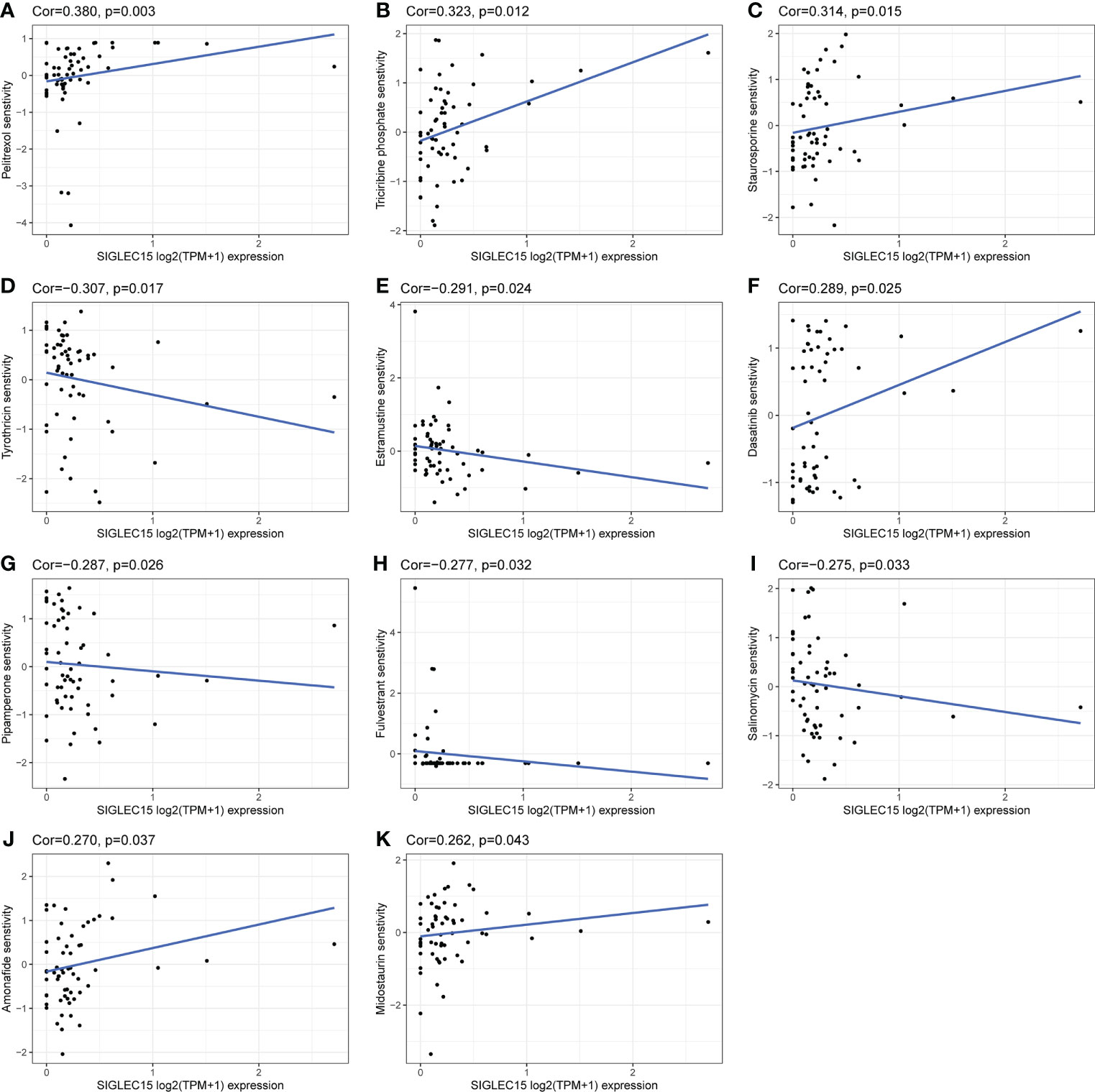
Figure 10 Potential effective chemotherapy drugs with SIGLEC15. (A–K) Correlation of SIGLEC15 mRNA expression level and IC50 of different drugs based on the CellMiner database.
Discussion
Thyroid cancer was one of the most prevalent endocrine cancers with an elevated incidence rate over the past decades, and it was the fifth leading incidence of cancer in women (4). Although the low mortality and moderate prognosis were frequently mentioned, the recurrence and the complications were still obscure. In these years, immunotherapy was applied to the treatment of advanced differentiated thyroid cancer and anaplastic thyroid cancer, with some success (20). Despite the immense success of multiple antibody-based immune therapies targeting PD-1/PD-L1 in common clinical regimens, there were still many non-responding patients (59). Since PD-1/PD-L1 represented only one of many inhibitory immune checkpoints, exploration of other potential new immune modulators that could be blocked to expand the success of cancer immunotherapy and promote the anticancer immune response is urgently needed.
In this study, the features of SIGLEC15 in multi-omics data in THCA cases were comprehensively characterized for the first time. We revealed that SIGLEC15 was overexpressed in THCA. Consistent with our result, previous studies through integrative data mining of SIGLEC15 mRNA expression in human tumors showed that higher SIGLEC15 levels were observed in colon adenocarcinoma and thyroid carcinoma (60), colon adenocarcinoma, esophageal carcinoma and thyroid carcinoma (61). Chen et al. found that SIGLEC15-knockout mice exhibited retarded tumor growth and prolonged survival compared to wild-type mice. Hao et al. (62) showed that SIGLEC15 mRNA expression was not associated with the prognosis of early non-small cell lung cancer. Liang et al. (63) proved that high SIGLEC15 mRNA expression was not related to either overall survival or disease-free survival in patients with non-small cell lung cancer. Quirino et al. (64) found that SIGLEC15 was also not correlated with either overall survival or relapse-free survival in gastric adenocarcinoma. In contrast, SIGLEC15 positivity had better disease-specific survival and progression-free survival compared to SIGLEC15 negativity in pancreatic ductal adenocarcinoma (30). Nevertheless, Li et al. (65) demonstrated that patients with a high SIGLEC15 mRNA expression had worse overall survival and disease-free survival than patients with low SIGLEC15 in the PACA-AU database, but no association was observed between SIGLEC15 and prognosis in their own microarray cohort. Thus, it remained to be determined which biomarkers (SIGLEC15 IHC or mRNA) could better guide patient selection for treatment response to SIGLEC15-associated therapy, and there has exited a companion diagnostic assay of SIGLEC5 by immunohistochemical was conducted by Shafi et al. (66). In addition, the pan-cancer analysis and our result showed that the expression of SIGLEC15 may play distinctive roles in different human cancers, such as acting as an immunosuppressor in “hot tumor” non-small cell lung cancer, so anti-SIGLEC15 therapy was suitable for such tumor. Meanwhile, in our study, we proved that increased SIGLEC15 expression positively correlated with more extrathyroid extension and lymph node metastasis, indicating the vital role of SIGLEC15 in the malignant progression of THCA; thus, THCA patients may benefit from the block antibodies for SIGLEC15.
Hu et al. (31) indicated that anti-SIGLEC15 therapy could be feasible for bladder cancer treatment as SIGLEC15 related to a non-inflamed TME in bladder cancer. Chen et al. (30) revealed that SIGLEC15 was related to a low density of Regulatory T cells (Tregs) and CD45RO T cells, and Wang et al. (27) also showed that SIGLEC15 suppressed antigen-specific T-cell responses. Liu et al. (67) demonstrated that SIGLEC15 promoted the migration of hepatoma cells through regulating the CD44 protein stability in liver cancer. Li et al. showed that SIGLEC15 performed immunosuppressive functions by directly inducing immunosuppressive tumor-associated macrophages (TAMs) via binding to α-2,3 sialic acid. Liu et al. (32) clarified the importance of LINC00973-miR-7109- SIGLEC15 regulation axis in immune evasion of clear-cell renal cell carcinoma. Our results showed that immunomodulators such as HLA class I and II and chemokines were upregulated in the higher SIGLEC15 group, which were vital molecules that induced adaptive immune responses (68); our results also showed a significant positive correlation between SIGLEC15 and CD44. In the cancer immunity cycle process, there existed seemingly contradictory results, step 1 (release of cancer cell antigens), step 6 (recognition of cancer cells by T cells), and step 4 (Th17 cell recruiting) were downregulated in the higher SIGLEC15 group; nevertheless, the higher SIGLEC15 group also met immune exhaustion and thus may induce an immune escape environment for patients and finally responded less to ICB therapy. In addition, patients who were in the higher SIGLEC15 group had more BRAF V600E mutation, which was a poor prognosis factor in THCA. Previous studies evidenced that treatment with inhibitors that target the BRAF kinase combined with anti-PD-1 therapy improved antitumor immunity in BRAF-mutant melanoma (69, 70). Clinical trial NCT02130466 showed that combined dabrafenib (a BRAF inhibitor) plus trametinib (a MEK1 and MEK2 (MEK1/2) inhibitor) plus pembrolizumab (an anti-PD-1 antibody) had more antitumor activity than dabrafenib plus trametinib plus placebo (71, 72). Our result also found some potent chemotherapy drugs for the high and low SIGLEC15 groups, so this may provide a rationale for using immuno-oncology agent combinations for THCA patients. The mentioned above result also signified the complex TME in THCA.
DNA methylation and m6A methylation were two epigenetic mechanisms for the regulation of gene expression in eukaryotes and acted as vital regulators in cancer (73–75). We firstly fully described the negative correlation of DNA methylation and expression of SIGLEC15 and prognosis in THCA in detail. Another pan-cancer also revealed the negative correlation in bladder cancer, uterine corpus endometrial carcinoma, breast invasive carcinoma, pancreatic ductal adenocarcinoma, etc. (61). We also firstly revealed the m6A methylation regulator relationship with SIGLEC15; regulating the expression of SIGLEC15 via methylation in cancer may be another road.
In addition to its function in immune regulation, Chen et al. (30) demonstrated that SIGLEC15 mRNA expression had a positive correlation with high BRCA1 status by IHC, and combining SIGLEC15 with different DDR molecular statuses may be a potential prognosis predictor. Read et al. (76) revealed that elevated pituitary tumor transform gene (PTTG) and pituitary tumor transforming gene binding factor (PBF) modulate DNA damage response genes in thyroid cancer. We found that SIGLEC15 was negatively related to BRCA1 in the mRNA level but no correlation with BRCA2. We also found that high SIGLEC15 had a negative correlation with DDR deficiency scores, such as aneuploidy score, CNA frac altered, and LOH frac altered, and these results implied that SIGLEC15 may affect thyroid cancer progression through interacting with DDR genes.
There were some limitations in the current work. Firstly, more experiments need to be done to figure out the cellular mechanism of SIGLEC15 in THCA. Secondly, there was no animal model experiment, so mouse models and either humanized or spontaneous but containing genomic features relevant to THCA animal models were needed to prove the results. Therefore, animal models, clinical verification data from multiple centers, and prospective studies were required to confirm our findings.
Conclusion
In conclusion, we found that SIGLEC15 mRNA expression was upregulated in tumor tissue and validated that by TMA. Clinical feature integration supported that increased SIGLEC15 mRNA expression promoted extrathyroid extension and lymph node metastasis, and elevated SIGLEC15 group patients met immune exhaustion. Specifying the role of SIGLEC15 in THCA could represent a potential next-generation cancer immunotherapy option for patients.
Data availability statement
The original contributions presented in the study are included in the article/Supplementary Material. Further inquiries can be directed to the corresponding authors.
Ethics statement
The studies involving human participants were reviewed and approved by Ethics Committee of Zhejiang Cancer Hospital. The patients/participants provided their written informed consent to participate in this study.
Author contributions
XFH, CC, XBL, and XDH have contributed equally to this work. XDH, XBL conceptualized and designed this study. CC, XFH Provision and collection of study materials, XFH, XBL, and XDH wrote the first draft of the manuscript. All authors contributed to the article and approved the submitted version.
Funding
This study was funded by the Medical and Health Research Program of Zhejiang Province 2022 Grant/Award Number: 2022PY005.
Conflict of interest
The authors declare that the research was conducted in the absence of any commercial or financial relationships that could be construed as a potential conflict of interest.
Publisher’s note
All claims expressed in this article are solely those of the authors and do not necessarily represent those of their affiliated organizations, or those of the publisher, the editors and the reviewers. Any product that may be evaluated in this article, or claim that may be made by its manufacturer, is not guaranteed or endorsed by the publisher.
Supplementary material
The Supplementary Material for this article can be found online at: https://www.frontiersin.org/articles/10.3389/fimmu.2022.975787/full#supplementary-material
Supplementary Figure 1 | Analysis of expression of SIGLEC15. (A) pan-cancer mRNA expression of SIGLEC15 between tumor and normal tissues from PCAWG database. (B) mRNA expression of SIGLEC15 between paired tumor and normal tissues from THCA (Thyroid carcinoma). (C) Correlation between mRNA expression of SIGLEC15 and CD44.
Supplementary Figure 2 | (A-K) Differential enrichment scores of immune signatures between high and low SIGLEC15 groups. (L) Enrichment scores of immune exhausted scores in low and high SIGLEC15 groups.
Supplementary Figure 3 | Single-cell analysis exploration of the expression cell type of SIGLEC15.
Supplementary Figure 4 | Kaplan-Meier survival curves between SIGLEC15 expression and survival.
Supplementary Figure 5 | (A, B) Comparison of amplification and deletion of CNV in high and low SIGLEC15 mRNA expression groups.
Supplementary Table 1 | Correlation details in expression of SIGLEC15 and CD44.
Abbreviations
Abbreviations: ACC, Adrenocortical carcinoma; BLCA, Bladder Urothelial Carcinoma; BRCA, Breast invasive carcinoma; CESC, Cervical squamous cell carcinoma and endocervical adenocarcinoma; CHOL, Cholangiocarcinoma; COAD, Colon adenocarcinoma; COADREAD, Colon adenocarcinoma/Rectum adenocarcinoma Esophageal carcinoma; ESCA, Esophageal carcinoma; GBM, Glioblastoma multiforme; GBMLGG, Glioma; HNSC, Head and Neck squamous cell carcinoma; KICH, Kidney Chromophobe; KIPAN, Pan-kidney cohort (KICH+KIRC+KIRP); KIRC, Kidney renal clear cell carcinoma; KIRP, Kidney renal papillary cell carcinoma; LAML, Acute Myeloid Leukemia; LGG, Brain Lower Grade Glioma; LIHC, Liver hepatocellular carcinoma; LUAD, Lung adenocarcinoma; LUSC, Lung squamous cell carcinoma; OV, Ovarian serous cystadenocarcinoma; PAAD, Pancreatic adenocarcinoma; PCPG, Pheochromocytoma and Paraganglioma; PRAD, Prostate adenocarcinoma; READ, Rectum adenocarcinoma; STAD, Stomach adenocarcinoma; SKCM, Skin Cutaneous Melanoma; STES, Stomach and Esophageal carcinoma; TGCT, Testicular Germ Cell Tumors; THCA, Thyroid carcinoma; UCEC, Uterine Corpus Endometrial Carcinoma; UCS, Uterine Carcinosarcoma; ALL, Acute Lymphoblastic Leukemia; WT, High-Risk Wilms Tumor.
References
1. Lim H, Devesa SS, Sosa JA, Check D, Kitahara CM. Trends in Thyroid Cancer Incidence and Mortality in the United States, 1974–2013. JAMA (2017) 317(13):1338–48. doi: 10.1001/jama.2017.2719
2. Ancker OV, Wehland M, Bauer J, Infanger M, Grimm D. Adverse Effect of Hypertension in the Treatment of Thyroid Cancer with Multi-Kinase Inhibitors. Int J Mol Sci (2017) 18(3):625. doi: 10.3390/ijms18030625
3. Siegel RL, Miller KD, Jemal A. Cancer statistics, 2015. CA Cancer J Clin (2015) 65(1):5–29. doi: 10.3322/caac.21254
4. Bray F, Ferlay J, Soerjomataram I, Siegel RL, Torre LA, Jemal A. Global cancer statistics 2018: GLOBOCAN estimates of incidence and mortality worldwide for 36 cancers in 185 countries. CA Cancer J Clin (2018) 68(6):394–424. doi: 10.3322/caac.21492
5. Mazzaferri EL, Kloos RT. Current approaches to primary therapy for papillary and follicular thyroid cancer. J Clin Endocrinol Metab (2001) 86(4):1447–63. doi: 10.1210/jcem.86.4.7407
6. Cabanillas ME, McFadden DG, Durante C. Thyroid cancer. Lancet (2016) 388(10061):2783–95. doi: 10.1016/S0140-6736(16)30172-6
7. Fridman WH, Sautès-Fridman C, Galon J. The immune contexture in human tumours: Impact on clinical outcome. Nat Rev Cancer (2012) 12(4):298–306. doi: 10.1038/nrc3245
8. Giraldo NA, Sanchez-Salas R, Peske JD, Vano Y, Becht E, Petitprez F, et al. The clinical role of the tme in solid cancer. Br J Cancer (2019) 120(1):45–53. doi: 10.1038/s41416-018-0327-z
9. Maman S, Witz IP. A history of exploring cancer in context. Nat Rev Cancer (2018) 18(6):359–76. doi: 10.1038/s41568-018-0006-7
10. Klemm F, Joyce JA. Microenvironmental regulation of therapeutic response in cancer. Trends Cell Biol (2015) 25(4):198–213. doi: 10.1016/j.tcb.2014.11.006
11. Kubli SP, Berger T, Araujo DV, Siu LL, Mak TW. Beyond immune checkpoint blockade: Emerging immunological strategies. Nat Rev Drug Discov (2021) 20(12):899–919. doi: 10.1038/s41573-021-00155-y
12. Vaddepally RK, Kharel P, Pandey R, Garje R, Chandra AB. Review of Indications of FDA-Approved Immune Checkpoint Inhibitors per NCCN Guidelines with the Level of Evidence. Cancers (Basel) (2020) 12(3):738. doi: 10.3390/cancers12030738
13. Twomey JD, Zhang B. Cancer Immunotherapy Update: FDA-Approved Checkpoint Inhibitors and Companion Diagnostics. AAPS J (2021) 23(2):39. doi: 10.1208/s12248-021-00574-0
14. Hargadon KM, Johnson CE, Williams CJ. Immune checkpoint blockade therapy for cancer: An overview of FDA-approved immune checkpoint inhibitors. Int Immunopharmacol (2018) 62:29–39. doi: 10.1016/j.intimp.2018.06.001
15. Rowshanravan B, Halliday N, Sansom DM. Ctla-4: A moving target in immunotherapy - sciencedirect. Blood (2018) 131(1):58–67. doi: 10.1182/blood-2017-06-741033
16. Wright JJ, Powers AC, Johnson D. Endocrine toxicities of immune checkpoint inhibitors. Nat Rev Endocrinol (2021) 17(7):389–99. doi: 10.1038/s41574-021-00484-3
17. Doroshow DB, Bhalla S, Beasley MB, Sholl LM, Kerr KM, Gnjatic S, et al. Pd-L1 as a biomarker of response to immune-checkpoint inhibitors. Nat Rev Clin Oncol (2021) 18(6):345–62. doi: 10.1038/s41571-021-00473-5
18. Sharpe AH, Pauken KE. The diverse functions of the Pd1 inhibitory pathway. Nat Rev Immunol (2018) 18(3):153–67. doi: 10.1038/nri.2017.108
19. Jiang Y, Zhao X, Fu J, Wang H. Progress and challenges in precise treatment of tumors with pd-1/Pd-L1 blockade. Front Immunol (2020) 11:339. doi: 10.3389/fimmu.2020.00339
20. Capdevila J, Wirth LJ, Ernst T, Aix SP, Taylor M. Pd-1 blockade in anaplastic thyroid carcinoma. J Clin Oncol (2020) 38(23):2620–7. doi: 10.1200/JCO.19.02727
21. Ahn S, Kim TH, Kim SW, Ki CS, Jang HW, Kim JS, et al. Comprehensive screening for pd-L1 expression in thyroid cancer. Endocrine-related Cancer (2017) 24(2):97. doi: 10.1530/ERC-16-0421
22. Angata T, Tabuchi Y, Nakamura K, Nakamura M. Siglec-15: An immune system siglec conserved throughout vertebrate evolution. Glycobiology (2007) 17(8):838–46. doi: 10.1093/glycob/cwm049
23. Shimizu T, Takahata M, Kameda Y, Endo T, Hamano H, Hiratsuka S, et alSialic acid-binding immunoglobulin-like lectin 15 (Siglec-15) mediates periarticular bone loss, but not joint destruction, in murine antigen-induced arthritis. Bone (2015) 79:65–70. doi: 10.1016/j.bone.2015.05.029
24. YK A, MT A, SM B, TS A, HH A, TA D, et al. Siglec-15 is a potential therapeutic target for postmenopausal osteoporosis. Bone (2015) 71:217–26. doi: 10.1016/j.bone.2014.10.027
25. Sato D, Takahata M, Ota M, Fukuda C, Tsuda E, Shimizu T, et al. Siglec-15-Targeting therapy increases bone mass in rats without impairing skeletal growth. Bone (2018) 116:172–80. doi: 10.1016/j.bone.2018.07.026
26. Jaeger M, Pinelli M, Borghi M, Constantini C, Dindo M, van Emst L, et al. A systems genomics approach identifies SIGLEC15 as a susceptibility factor in recurrent vulvovaginal candidiasis. Sci Transl Med (2019) 11(496):eaar3558. doi: 10.1126/scitranslmed.aar3558
27. Wang J, Sun J, Liu LN, Flies DB, Nie X, Toki M, et al. Siglec-15 as an immune suppressor and potential target for normalization cancer immunotherapy. Nat Med (2019) 25(4):656–66. doi: 10.1038/s41591-019-0374-x
28. Du H, Tang J, Li X, Wang X, Wu L, Zhang R, et al. Siglec-15 is an immune suppressor and potential target for immunotherapy in the pre-metastatic lymph node of colorectal cancer. Front Cell Dev Biol (2021) 9:691937. doi: 10.3389/fcell.2021.691937
29. Li TJ, Jin KZ, Li H, Ye LY, Li PC, Jiang B, et al. SIGLEC15 amplifies immunosuppressive properties of tumor-associated macrophages in pancreatic cancer. Cancer Lett (2022) 530:142–55. doi: 10.1016/j.canlet.2022.01.026
30. Chen X, Mo S, Zhang Y, Ma H, Lu Z, Yu S, et al. Analysis of a novel immune checkpoint, siglec-15, in pancreatic ductal adenocarcinoma. J Pathol Clin Res (2022) 8(3):268–78. doi: 10.1002/cjp2.260
31. Hu J, Yu A, Othmane B, Qiu D, Li H, Li C, et al. SIGLEC15 shapes a non-inflamed tumor microenvironment and predicts the molecular subtype in bladder cancer. Theranostics (2021) 11(7):3089–108. doi: 10.7150/thno.53649
32. Liu Y, Li X, Zhang C, Zhang H, Huang Y. Linc00973 is involved in cancer immune suppression through positive regulation of siglec-15 in clear-cell renal cell carcinoma. Cancer Sci (2020) 111(10):3693–704. doi: 10.1111/cas.14611
33. Shum E, Myint H, Shaik J, Zhou Q, Barbu E, Morawski A, et al. 490 clinical benefit through siglec-15 targeting with Nc318 antibody in subjects with siglec-15 positive advanced solid tumors. Journal for ImmunoTherapy of Cancer (2021) 9:A520–1. doi: 10.1136/jitc-2021-SITC2021.490
34. Goldman MJ, Craft B, Hastie M, Repečka K, McDade F, Kamath A, et al. Visualizing and interpreting cancer genomics data Via the xena platform. Nat Biotechnol (2020) 38(6):675–8. doi: 10.1038/s41587-020-0546-8
35. Izzi V, Davis MN, Naba A. A. Pan-Cancer Analysis of the Genomic Alterations and Mutations of the Matrisome. Cancers (Basel) 12(8):2046. doi: 10.3390/cancers12082046
36. Wang S, Xiong Y, Zhao L, Gu K, Li Y, Zhao F, et al. Ucscxenashiny: An R/Cran package for interactive analysis of ucsc xena data. Bioinformatics (2021) 2):2. doi: 10.1093/bioinformatics/btab561
37. Edgar R, Domrachev M, Lash AE. Gene expression omnibus: Ncbi gene expression and hybridization array data repository. Nucleic Acids Res (2002) 30(1):207–10. doi: 10.1093/nar/30.1.207
38. Ritchie ME, Phipson B, Wu D, Hu Y, Law CW, Shi W, et al. Limma powers differential expression analyses for rna-sequencing and microarray studies. Nucleic Acids Res (2015) 43(7):e47–e. doi: 10.1093/nar/gkv007
39. Grossman RL, Heath AP, Ferretti V, Varmus HE, Lowy DR, Kibbe WA, et al. Toward a shared vision for cancer genomic data. N Engl J Med (2016) 375(12):1109–12. doi: 10.1056/NEJMp1607591
40. Colaprico A, Silva TC, Olsen C, Garofano L, Cava C, Garolini D, et al. Tcgabiolinks: An R/Bioconductor package for integrative analysis of tcga data. Nucleic Acids Res (2015) 44(8):e71. doi: 10.1093/nar/gkv1507
41. Reich M, Liefeld T, Gould J, Lerner J, Tamayo P, Mesirov JP. Genepattern 2.0. Nat Genet (2006) 38(5):500–1. doi: 10.1038/ng0506-500
42. Knijnenburg TA, Wang L, Zimmermann MT, Chambwe N, Gao GF, Cherniack AD, et al. Genomic and molecular landscape of DNA damage repair deficiency across the cancer genome atlas. Cell Rep (2018) 23(1):239–54.e6. doi: 10.1016/j.celrep.2018.03.076
43. Sun D, Wang J, Han Y, Dong X, Ge J, Zheng R, et al. Tisch: A comprehensive web resource enabling interactive single-cell transcriptome visualization of tumor microenvironment. Nucleic Acids Res (2020) 49(D1):D1420–D30. doi: 10.1093/nar/gkaa1020
44. Zhang C, Fu J, Zhou Y. A review in research progress concerning M6a methylation and immunoregulation. Front Immunol (2019) 10:922. doi: 10.3389/fimmu.2019.00922
45. Subramanian A, Tamayo P, Mootha VK, Mukherjee S, Ebert BL, Gillette MA, et al. Gene set enrichment analysis: A knowledge-based approach for interpreting genome-wide expression profiles. Proc Natl Acad Sci USA (2005) 102(43):15545–50. doi: 10.1073/pnas.0506580102
46. Mootha VK, Lindgren CM, Eriksson KF, Subramanian A, Sihag S, Lehar J, et al. PGC-1α-Responsive genes involved in oxidative phosphorylation are coordinately downregulated in human diabetes. Nat Genet (2003) 34(3):267–73. doi: 10.1038/ng1180
47. Szklarczyk D, Gable AL, Lyon D, Junge A, Wyder S, Huerta-Cepas J, et al. String V11: Protein–protein association networks with increased coverage, supporting functional discovery in genome-wide experimental datasets. Nucleic Acids Res (2018) 47(D1):D607–13. doi: 10.1093/nar/gky1131
48. Shannon P. Cytoscape: A software environment for integrated models of biomolecular interaction networks. Genome Res (2003) 13(11):2498–504. doi: 10.1101/gr.1239303
49. Chin C-H, Chen S-H, Wu H-H, Ho C-W, Ko M-T, Lin C-Y. Cytohubba: Identifying hub objects and Sub-networks from complex interactome. BMC Syst Biol (2014) 8(4):S11. doi: 10.1186/1752-0509-8-S4-S11
50. Charoentong P, Finotello F, Angelova M, Mayer C, Efremova M, Rieder D, et al. Pan-cancer immunogenomic analyses reveal genotype-immunophenotype relationships and predictors of response to checkpoint blockade. Cell Rep (2017) 18(1):248–62. doi: 10.1016/j.celrep.2016.12.019
51. Huang X, Zhang G, Tang T, Liang T. Identification of tumor antigens and immune subtypes of pancreatic adenocarcinoma for mrna vaccine development. Mol Cancer (2021) 20(1):44. doi: 10.1186/s12943-021-01310-0
52. Xu L, Deng C, Pang B, Zhang X, Liu W, Liao G, et al. Tip: A web server for resolving tumor immunophenotype profiling. Cancer Res (2018) 78(23):6575–80. doi: 10.1158/0008-5472.Can-18-0689
53. Eddy JA, Thorsson V, Lamb AE, Gibbs DL, Heimann C, Yu JX, et al. Cri iatlas: An interactive portal for immuno-oncology research. F1000Res (2020) 9:1028. doi: 10.12688/f1000research.25141.1
54. Yoshihara K, Shahmoradgoli M, Martínez E, Vegesna R, Kim H, Torres-Garcia W, et al. Inferring tumour purity and stromal and immune cell admixture from expression data. Nat Commun (2013) 4:2612. doi: 10.1038/ncomms3612
55. Li T, Fan J, Wang B, Traugh N, Chen Q, Liu JS, et al. Timer: A web server for comprehensive analysis of tumor-infiltrating immune cells. Cancer Res (2017) 77(21):e108–10. doi: 10.1158/0008-5472.Can-17-0307
56. Hänzelmann S, Castelo R, Guinney J. GSVA: gene set variation analysis for microarray and RNA-seq data. BMC Bioinformatics (2013) 14:7–21. doi: 10.1186/1471-2105-14-7
57. Jiang P, Gu S, Deng P, Fu J, Sahu A, Hu X, et al. Signatures of T cell dysfunction and exclusion predict cancer immunotherapy response. Nat Med (2018) 24(10):1550–58. doi: 10.1038/s41591-018-0136-1
58. Luna A, Elloumi F, Varma S, Wang Y, Rajapakse VN, Aladjem MI, et al. CellMiner Cross-Database (CellMinerCDB) version 1.2: Exploration of patient-derived cancer cell line pharmacogenomics. Nucleic Acids Res (2021) 49(D1):D1083–93. doi: 10.1093/nar/gkaa968
59. O’Donnell J, Long GV, Scolyer RA, Teng MW, Smyth MJ. Resistance to Pd1/Pdl1 checkpoint inhibition. Cancer Treat Rev (2017) 52:71–81. doi: 10.1016/j.ctrv.2016.11.007
60. Li QT, Huang ZZ, Chen YB, Yao HY, Ke ZH, He XX, et al. Integrative analysis of siglec-15 mrna in human cancers based on data mining. J Cancer (2020) 11(9):2453–64. doi: 10.7150/jca.38747
61. Li B, Zhang B, Wang X, Zeng Z, Huang Z, Zhang L, et al. Expression signature, prognosis value, and immune characteristics of siglec-15 identified by pan-cancer analysis. Oncoimmunology (2020) 9(1):1807291. doi: 10.1080/2162402x.2020.1807291
62. Hao JQ, Nong JY, Zhao D, Li HY, Wang JH. The significance of siglec-15 expression in resectable non-small cell lung cancer. Neoplasma (2020) 67(6):1214–22. doi: 10.4149/neo_2020_200220N161
63. Liang H, Chen Q, Hu Z, Zhou L, Meng Q, Zhang T, et al. SIGLEC15 facilitates the progression of non-small cell lung cancer and is correlated with spinal metastasis. Ann Transl Med (2022) 10(6):281. doi: 10.21037/atm-22-764
64. Quirino MWL, Pereira MC, Deodato de Souza MF, Pitta IDR, Da Silva Filho AF, Albuquerque MSS, et al. Immunopositivity for siglec-15 in gastric cancer and its association with clinical and pathological parameters. Eur J Histochem (2021) 65(1). doi: 10.4081/ejh.2021.3174
65. Li TJ, Jin KZ, Li H, Ye LY, Li PC, Jiang B, et al. SIGLEC15 amplifies immunosuppressive properties of tumor-associated macrophages in pancreatic cancer. Cancer Lett (2022) 530:142–55. doi: 10.1016/j.canlet.2022.01.026
66. Shafi S, Aung TN, Robbins C, Zugazagoitia J, Vathiotis I, Gavrielatou N, et al. Development of an immunohistochemical assay for siglec-15. Lab Invest (2022) 102(7):771–8. doi: 10.1038/s41374-022-00785-9
67. Liu W, Ji Z, Wu B, Huang S, Chen Q, Chen X, et al. Siglec-15 promotes the migration of liver cancer cells by repressing lysosomal degradation of Cd44. FEBS Lett (2021) 595(17):2290–302. doi: 10.1002/1873-3468.14169
68. Wang C, Xiong C, Hsu Y-C, Wang X, Chen L. Human leukocyte antigen (Hla) and cancer immunotherapy: Hla-dependent and-independent adoptive immunotherapies. (2020) 5(14):14–26. doi: 10.21037/aob-20-27
69. Salmon H, Idoyaga J, Rahman A, Leboeuf M, Remark R, Jordan S, et al. Expansion and activation of Cd103(+) dendritic cell progenitors at the tumor site enhances tumor responses to therapeutic pd-L1 and braf inhibition. Immunity (2016) 44(4):924–38. doi: 10.1016/j.immuni.2016.03.012
70. Cooper ZA, Frederick DT, Ahmed Z, Wargo JA. Combining checkpoint inhibitors and braf-targeted agents against metastatic melanoma. Oncoimmunology (2013) 2(5):e24320. doi: 10.4161/onci.24320
71. Ascierto P, Ferrucci P, Stephens R, Del Vecchio M, Atkinson V, Schmidt H, et al. Keynote-022 part 3: Phase ii randomized study of 1l dabrafenib (D) and trametinib (T) plus pembrolizumab (Pembro) or placebo (Pbo) for braf-mutant advanced melanoma. Ann Oncol (2018) 29:viii442. doi: 10.1093/annonc/mdy289
72. Ascierto PA, Ferrucci PF, Fisher R, Del Vecchio M, Atkinson V, Schmidt H, et al. Dabrafenib, trametinib and pembrolizumab or placebo in braf-mutant melanoma. Nat Med (2019) 25(6):941–6. doi: 10.1038/s41591-019-0448-9
73. Fu Y, Dominissini D, Rechavi G, He C. Gene expression regulation mediated through reversible M6a rna methylation. Nat Rev Genet (2014) 15(5):293–306. doi: 10.1038/nrg3724
74. Bradner JE, Hnisz D, Young RA. Transcriptional addiction in cancer. Cell (2017) 168(4):629–43. doi: 10.1016/j.cell.2016.12.013
75. Chen XY, Zhang J, Zhu JS. The role of M(6)a rna methylation in human cancer. Mol Cancer (2019) 18(1):103. doi: 10.1186/s12943-019-1033-z
Keywords: thyroid cancer, SIGLEC15, mRNA expression, clinical characteristics, immune dysfunction
Citation: Hou X, Chen C, Lan X and He X (2022) Unveiling the molecular features, relevant immune and clinical characteristics of SIGLEC15 in thyroid cancer. Front. Immunol. 13:975787. doi: 10.3389/fimmu.2022.975787
Received: 22 June 2022; Accepted: 17 August 2022;
Published: 09 September 2022.
Edited by:
Liusheng Peng, Third Military Medical University, ChinaReviewed by:
Huimin Cui, Yantaishan Hospital, ChinaTakashi Tanikawa, Josai University, Japan
Hongbo Xu, Wenzhou Medical University, China
Copyright © 2022 Hou, Chen, Lan and He. This is an open-access article distributed under the terms of the Creative Commons Attribution License (CC BY). The use, distribution or reproduction in other forums is permitted, provided the original author(s) and the copyright owner(s) are credited and that the original publication in this journal is cited, in accordance with accepted academic practice. No use, distribution or reproduction is permitted which does not comply with these terms.
*Correspondence: Xiaodong He, hxd@lzu.edu.cn; Xiabin Lan, Ianxia99@sina.com