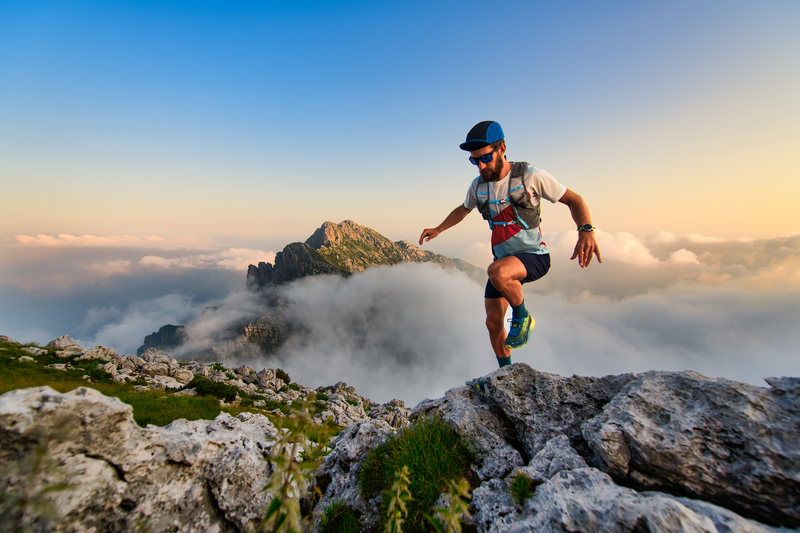
95% of researchers rate our articles as excellent or good
Learn more about the work of our research integrity team to safeguard the quality of each article we publish.
Find out more
ORIGINAL RESEARCH article
Front. Immunol. , 25 August 2022
Sec. Cancer Immunity and Immunotherapy
Volume 13 - 2022 | https://doi.org/10.3389/fimmu.2022.973153
This article is part of the Research Topic Tumor Ablation and Immunity View all 36 articles
Purpose: As a coreceptor in Wnt and HGF signaling, glypican-3 (GPC-3) promotes the progression of tumor and is associated with a poor prognosis in hepatocellular carcinoma (HCC). GPC-3 has evolved as a target molecule in various immunotherapies, including chimeric antigen receptor T cell. However, its evaluation still relies on invasive histopathologic examination. Therefore, we aimed to develop an easy-to-use and noninvasive risk score integrating preoperative gadoxetic acid–enhanced magnetic resonance imaging (EOB-MRI) and clinical indicators to predict positive GPC-3 expression in HCC.
Methods and materials: Consecutive patients with surgically-confirmed solitary HCC who underwent preoperative EOB-MRI between January 2016 and November 2021 were retrospectively included. EOB-MRI features were independently evaluated by two masked abdominal radiologists and the expression of GPC-3 was determined by two liver pathologists. On the training dataset, a predictive scoring system for GPC-3 was developed against pathology via logistical regression analysis. Model performances were characterized by computing areas under the receiver operating characteristic curve (AUCs).
Results: A total of 278 patients (training set, n=156; internal validation set, n=39; external validation set, n=83) with solitary HCC (208 [75%] with positive GPC-3 expression) were included. Serum alpha-fetoprotein >10 ng/ml (AFP, odds ratio [OR]=2.3, four points) and five EOB-MR imaging features, including tumor size >3.0cm (OR=0.5, -3 points), nonperipheral “washout” (OR=3.0, five points), infiltrative appearance (OR=9.3, 10 points), marked diffusion restriction (OR=3.3, five points), and iron sparing in solid mass (OR=0.2, -7 points) were significantly associated with positive GPC-3 expression. The optimal threshold of scoring system for predicting GPC-3 positive expression was 5.5 points, with AUC 0.726 and 0.681 on the internal and external validation sets, respectively.
Conclusion: Based on serum AFP and five EOB-MRI features, we developed an easy-to-use and noninvasive risk score which could accurately predict positive GPC-3 HCC, which may help identify potential responders for GPC-3-targeted immunotherapy.
Primary liver cancer is the sixth most commonly diagnosed cancer and the third leading cause of cancer-related death worldwide, and hepatocellular carcinoma (HCC) comprises 75% to 85% of cases (1). Immunotherapies play an increasingly central role in the management of HCC, among which chimeric antigen receptor (CAR) T cell therapy is regarded as a promising next-generation immunotherapy regimen with remarkable safety and efficacy profiles (2). However, CAR T cell therapy was shown to provide clinical benefit in limited HCC patients, possibly due to a lack of tumor specific antigens and an immunosuppressive tumor microenvironment (3). Therefore, there remains an unmet need for biomarker discovery which could help identify potential responders and direct individualized treatment decision-making.
In HCC, Wnt signaling has been reported to promote hepatocarcinogenesis, tumor growth and dissemination, while the hepatocyte growth factor (HGF) is also associated with increased hepatocarcinogenesis and metastasis. Glypican-3 (GPC-3) is a heparan sulfate glycoprotein which serves as a coreceptor in Wnt and HGF signaling. In specific, GPC-3 binds to the cell membrane and is involved in organ morphogenesis by regulating cell proliferation through modulation of Wnt signaling. Moreover, it is also involved in HCC cell migration and motility through heparan sulfate chain-mediated cooperation with the HGF/Met pathway (4, 5). Therefore, it has also been reported to promote tumor progression and associated with a poor prognosis in HCC (6–8). Currently, GPC-3 is widely used for diagnostic purposes because of it is specifically expressed in around 70–80% of HCCs (9). More recently, GPC-3 has gained much attention as a novel target molecule in immunotherapies. In specific, Shi D et al. (10) firstly published a phase I trial of CAR-GPC-3 T-cell therapy in patients with advanced HCC, and their results demonstrated the initial safety profile and early signs of antitumor activity of CAR-GPC-3 T cells. In addition, Liu et al. (5) reported that a novel human monoclonal antibody (32A9), as a GPC-3-specifc antibody which efficiently eliminated GPC3-positive HCC cells in vitro and induced HCC xenograft tumor regressions in vivo. Comparison with traditional therapies, tumor antigen-targeting antibody- and immune modulating antibody-based immunotherapies represent emerging approaches that may improve HCC treatment outcomes (11). Therefore, GPC-3 holds potential to serve as an effective biomarker for patient selection in HCC immunotherapy. However, the assessment of GPC-3 expression still mandates invasive histopathologic examination, which is sensitive to sampling errors and not routinely performed in the clinical practice.
Magnetic resonance imaging (MRI) could be used for visualization of specific target or drug delivery carrier accumulation in tumors (12, 13). Similarly, GPC-3 expression can be evaluated via noninvasive imaging techniques. For example, MRI specific superparamagnetic iron oxide (SPIO) anti-GPC-3 molecular probe has demonstrated the effectiveness for assessing GPC-3 expression in HCC tissues (14–16), and a few studies revealed the associations between GPC-3 expression with various MRI morphological features and quantitative parameters (e.g., MRI-based radiomics and iterative decomposition of water and fat with echo asymmetry and least squares estimation [IDEAL-IQ] parameters) (17–19). Despite promising results so far, existing evidence were not yet ready to be transformed into routine practice due to the limited application of SPIO and poor interpretability of quantitative techniques.
Therefore, in patients who underwent curative-intent surgery for solitary HCC, this study aimed to develop and validate a simple, noninvasive, and interpretable model to predict GPC-3 expression based on gadoxetic acid (EOB)-enhanced MRI and clinical features and to explore the model’s efficacy in stratifying postoperative survival.
This retrospective study was approved by the Ethics Committee of West China Hospital, Sichuan University (Chengdu, China), and the requirements to obtain written informed consent were waived (Approved No. 2022-651).
Between January 2016 and November 2021, consecutive patients who fulfilled the following inclusion criteria were enrolled: (a) age ≥18 years;(b) underwent curative-intent liver resection; (c) with pathologically-confirmed solitary HCC; and (d) underwent EOB-MRI within 30 days prior to surgery. The exclusion criteria were as follows: (a) received any prior treatment for HCC (e.g., hepatectomy, radiofrequency ablation and transhepatic arterial chemotherapy and embolization); (b) the MR images were of insufficient quality for analysis (e.g., severe artifact); (c) with other malignant tumors than HCC (n=9); and (d) inadequate information on postoperative pathology report to determine GPC-3 expression. Detailed patient inclusion and exclusion are illustrated in Figure 1.
Figure 1 The flowchart of the retrospective study cohort. A total of 278 participants with solitary hepatocellular carcinoma were included in this study.
Baseline clinical information, including patient demographics, causes of liver diseases, and key laboratory test results (alpha-fetoprotein [AFP], aspartate aminotransferase [AST], alanine aminotransferase [ALT] and serum total bilirubin [TBIL]) were collected from electronic medical records.
Four 3.0 T MR scanners (Discovery 750, SIGNA™ Architect, and SIGNA™ Premier, GE Healthcare; and MAGNETOM Skyra, Siemens Healthineers) and one 1.5 T MRI scanner (uMR588, United Imaging Healthcare) were used to acquire MR images. The sequences included: T2-weighted imaging, diffusion-weighted imaging with apparent diffusion coefficient maps, T1-weighted in-phase and opposed-phase imaging, and T1-weighted dynamic imaging with gadoxetic acid disodium (Primovist®, Bayer Pharma AG). Details of the MRI technique are provided in Supplementary A1 and Table S1.
Two fellowship-trained abdominal radiologists (with 8 and 6 years of experiences in liver MR imaging, respectively) who were blinded of the clinical, laboratory, histopathologic and follow-up information independently reviewed all MR images. Any discrepancy in imaging interpretation was resolved by a third radiologist who had over 20 years of experience in liver MR imaging.
The reviewers evaluated the presence/absence of a total of 37 imaging features which were reported to associate with the underlying liver disease (e.g., radiologically-evident cirrhosis), tumor burden (e.g., bilobar involvement), tumor extent (e.g., lymph node metastasis, macrovascular invasion), tumor aggressiveness (e.g., intratumoral artery, non-smooth tumor margin, peritumoral hepatobiliary phase hypointensity) and the Liver Imaging Reporting and Data System (LI-RADS) version 2018 features and categories. Definitions of the evaluated imaging features are summarized in Table S2.
Information on GPC-3 expression, as documented by another radiologist without knowing the patient’s imaging and clinical data, was retrieved from routine pathological reports as the reference standard in the current study.
In specific, complete HCC specimens were obtained after hepatectomy, and a standard seven-point sampling method (20) was used to assess tumor and peritumoral pathologic features. To accurately evaluate the expression of GPC-3, we adopted the scoring scale proposed by Takai et al. (21) which took into account positive cell rate, staining intensity, and staining pattern. Based on this scoring scale, the positive cell rate was graded from 0 to 3+ as 0 (<5% tumor cells positive), 1+ (5–10% tumor cells positive), 2+ (10–50% tumor cells positive), and 3+ (>50% tumor cells positive). The staining intensity was classified as weak, moderate, and strong staining. The staining pattern was graded on a scale of I–III based on whether the cell membrane manifested as incomplete (I: globally incomplete; II: generally incomplete with some complete staining) or complete circumferential staining (III: generally complete with some incomplete staining). In the current study, grade 0 positive cell rate with any staining intensity or grade 1+ positive cell rate with weak staining were regarded as GPC-3 negative.
Patients underwent routine postoperative follow-up at 1 month, every 3 months for the first 2 years and every 6 months thereafter with serum AFP and contrast-enhanced imaging modality (ultrasound, CT, or MRI). Tumor recurrence was confirmed by imaging or pathologic examinations during follow-up. Recurrence-free survival (RFS) was defined as the time from the date of surgery to that of tumor recurrence or the last follow-up date (May 1, 2022), whichever occurred first. Overall survival (OS) was defined as the time from the date of surgery to that of death by any cause, or the last follow-up date, whichever occurred first.
Differences in continuous variables were compared using either the Student’s t test or the Mann-Whitney U test, while those in categorical variables were investigated by either the chi-square test or the Fisher’s exact test, as appropriate.
Inter-rater agreement between the two reviewers were assessed by calculating the intraclass correlation coefficient for continuous variables, Cohen’s κ values for binary variables and weighted κ values for ordinal/categorical variables, respectively.
On a per-patient basis, 70% of randomly selected patients (n=195) were assigned into the training dataset, while the remaining 30% (n=83) into an independent external validation dataset.
On the training dataset, clinical and imaging predictors for GPC-3 were selected via univariable logistic regression analysis. To improve clinical utility and model simplicity, continuous variables were converted to categorical or dichotomized variables according to ranges of normality or clinical relevance (showed in Table 2). Thereafter, all predictors with P values < 0.1 were fit into a multivariable logistic regression model with backward stepwise method and 5-fold cross validation (creating the “internal validation” dataset) while controlled for patient age, gender, and HBV infection status (infected vs. non-infected). The Akaike Information Criterion was used to obtain the most parsimonious feature combination. A scoring system and nomogram was constructed based on the significant predictors at multivariable regression analysis to estimate the probability of GPC-3 expression, and the optimal threshold of the nomogram was determined by receiver operating characteristic analysis with the Youden’s index.
On the external validation dataset, the diagnostic performances of the predictive model for assessing GPC-3 expression were evaluated by computing area under the receiver operating characteristic curve (AUC), sensitivity, specificity, positive predictive value (PPV), negative predictive value (NPV), and accuracy. Calibration curves were plotted to investigate the model calibration by the Hosmer-Lemeshow test, and decision curve analysis was conducted to estimate the model’s clinical usefulness by quantifying the net benefits at different threshold probabilities. Survival outcomes were evaluated using the Kaplan-Meier method and compared with the log-rank test.
All statistical analyses were conducted with R software (version 3.5.1; http://www.Rproject.org) and SPSS (version 22.0). A two-sided p<0.05 was used to indicate statistically significant difference.
A total of 278 eligible patients (222 male; 53.6 ± 11.6 years) were enrolled during the study period (Figure 1), 208 (74.8%) with GPC-3 positive HCC and 70 (25.2%) with GPC-3 negative HCC. In the GPC-3 positive and negative groups, the mean patient age was 52.9 ± 11.7 and 55.8 ± 11.3 years, respectively (P=0.066); male and female patients were 165 (79.3%) and 43 (20.7%) in positive group, 57 (81.4%) and 13 (18.6%) in negative group (P=0.735); the number of patients with hepatitis B was 193 (92.8%) and 60 (85.7%), respectively, the cause of liver disease was no significant difference (P=0.304); the median of serum AFP was 40.6 (range 0.98–25451) ng/mL and 6.73 (range 1.0~2484.0) ng/mL, respectively (P=0.009); serum AST was 29.0 (13.0~723.0) U/L and 35.0 (13.0~243.0) U/L, respectively (P=0.043); serum ALB was 43.1 (30.6~66.5) g/L and 41.9 (27.2~51.0) g/L, respectively (P=0.043); no significant difference in other clinical characteristics was detected between the GPC-3 positive and negative groups. (Table 1)
No difference in baseline clinical features was detected between the training and validation datasets (P > 0.05 for all; Supplementary Table S3).
In the groups of GPC-3 positive and negative HCC, the tumor size were 3.73 ± 2.7 cm and 4.4 ± 2.8 cm, respectively (P=0.091); the number of patients presenting nonperipheral “washout” were 182 (87.5%) and 65 (92.9%), respectively (P=0.095); the number of patients presenting “infiltrative appearance” were 28 (13.5%) and three (4.3%), respectively (P=0.046); the number of patients presenting “marked diffusion restriction” were 87 (41.8%) and 17 (24.3%), respectively (P=0.01); the number of patients presenting “iron sparing in solid mass” were 34 (16.3%) and 18 (25.7%), respectively (P=0.11). The number of patients with LI-RADS categories of 4, 5 and M were 15 (7.2%), 175 (84.1%),18 (8.7%) for patients with positive GPC-3 expressions, and three (4.3%), 64 (91.4%), three (4.3%) for patients with negative GPC-3 expressions, respectively (P=0.399); no significant difference in other EOB-MRI features was detected between the GPC-3 positive and negative groups. (Table 2)
On the training dataset, 14 clinical variables and 37 imaging features were significantly associated with GPC-3 expression at univariate analysis; while serum AFP (odds ratio [OR]=2.3, 95% confidence interval [CI]: 1.1-4.7, corresponding to four points in the GPC-3 score for >10ng/mL), tumor size (OR=0.5, 95%CI: 0.2-1.0, corresponding to -3 points in the GPC-3 score for >3.0 cm), nonperipheral “washout” (OR=3.0, 95%CI: 1.3-7.2, corresponding to five points in the GPC-3 score for its presence), infiltrative appearance (OR=9.3, 95% CI: 1.8-46.4, corresponding to 10 points in the GPC-3 score for its presence), marked diffusion restriction (OR=3.3, 95%CI: 1.5-7.4, corresponding to five points in the GPC-3 score for its presence) and iron sparing in solid mass (OR=0.2, 95% CI: 0.1-0.6, corresponding to -7 points in the GPC-3 score for its presence) were significantly associated with GPC-3 expression at multivariate logistic regression analysis (Figure 2; Table 3).
Figure 2 Gadoxetate disodium-enhanced MRI and histopathologic images of hepatocellular carcinoma (HCC) with different glypican-3 (GPC-3) expressions. A 72-year-old male patient with GPC-3 positive expression HCC (A–F), and a 77-year-old male patient with GPC-3 negative expression HCC (G–L). Pre-contrast T1-weighted images showed a hypointense lesion (3.54cm) in the right liver (A) and a hypointense lesion (3.97cm) in the mid liver (G); T2-weighted images showed hyperintense lesions with [(B), blue arrow] and without [(H), blue arrow] “iron sparing in solid mass”; diffusion-weighted images demonstrated presence [(C), yellow arrow] and absence [(I), yellow arrow] of “marked diffusion restriction”; arterial phase images showed “nonperipheral-nonglobal arterial phase hyperenhancement” [(D), green arrow) and “global arterial phase hyperenhancement” [(J), green arrow]; portal venous phase images showed “nonperipheral washout” and infiltrative appearance [(E), orange and red arrow] and “no-washout” and smooth margin [(K), orange and red arrow]; immunohistochemical staining revealed the GPC-3 positive (F) and negative (L) expressions.
Inter-rater agreement was moderate for nonperipheral “washout” (κ=0.400, 95%CI: 0.307-0.494), infiltrative appearance (κ=0.430, 95%CI: 0.266-0.576), marked diffusion restriction (κ=0.387, 95%CI: 0.261-0.497) and iron sparing in solid mass (κ= 0.432, 95%CI: 0.293-0.561). Inter-rater agreements on the remaining imaging features are summarized in Table 2.
The AUC for the GPC-3 prediction model was 0.775 (95% CI, 0.694- 0.855), 0.726 (95% CI, 0.558- 0.894) and 0.681 (95% CI, 0.547-0.81) for the training, internal validation, and external validation datasets, respectively (Table 4).
According to Youden’s index, the optimal threshold of scoring system for predicting GPC-3 expression was 5.5 points, and patients with the GPC-3 scores ≥5.5 points were categorized as at high-risk for positive GPC-3 expression in HCC. Based on this threshold, the sensitivity, specificity, PPV, NPV, and accuracy of the GPC-3 score on the internal validation dataset was 50.7% (95% CI, 42.3%-59%), 87.8% (95% CI, 75.2%-95.4%), 92.5% (95% CI, 84.4%-97.2%), 37.4% (95% CI, 28.5%-46.9%), and 60% (95% CI, 52.8%-66.9%), respectively. On the external validation dataset, these measures were 43.5% (95% CI, 31%-56.7%), 81.0% (95% CI, 58.1%-94.6%), 87.1% (95% CI, 70.2%-96.4%), 32.7% (95% CI, 20.3%-47.1%), and 53% (95% CI, 41.7%-64.1%), respectively.
The nomogram and decision curves revealed substantial clinical benefit of the prediction model in predicting GPC-3 expression (Figure 3). The calibration curves showed good agreement between predicted and observed probabilities of positive GPC-3 expression for both the training (P=0.895) and validation (P=0.264) datasets (Figure 4).
Figure 3 The nomogram and decision curve to predict glypican-3 positive expression in hepatocellular carcinoma. (A) The nomogram was developed based on serum alpha-fetoprotein and five MRI features (tumor size, nonperipheral “washout”, infiltrative appearance, marked diffusion restriction, and iron sparing in solid mass). (B) Decision curve analysis of the prediction model for external validation set. Y-axis represents the net benefit, which is calculated by gaining true positives and deleting false positives. The X-axis is the probability threshold. The curve of the predictive model over the AFP and MRI features that showed the good benefit.
Figure 4 Model calibration curves on the training (A) and validation sets (B) to predict glypican-3 positive expression in hepatocellular carcinoma.
A total of 187 (62%) patients had complete RFS and OS information, thus were included in the survival analyses. Median follow-up was 668 days (95% CI: 589-734 days). Among them, 14 (7%) patients died, and 66 (35%) patients experienced tumor recurrence. Median OS was 1613 days (95% CI: 972-1973 days), while median RFS was 1548 days (95% CI: 1113-2281 days). No difference in RFS and OS was detected between patients with pathologically or model-predicted confirmed GPC-3 expressions. (P values= 0.13, 0.20, 0.52 and 0.150, respectively), despite a trend-wise longer survival in patients with GPC-3 negative HCC (Figure 5).
Figure 5 The Kaplan-Meier curves of pathologically-confirmed (A, C) and model-predicted glypican-3 expressions (B, D) in stratifying postoperative overall survival (A, B) and recurrence-free survival (B, D).
In patients who underwent curative-intent liver resection for solitary HCC, we developed and validated a noninvasive risk score to predict GPC-3 expression based on serum AFP and five EOB-MRI features (tumor size, nonperipheral “washout”, infiltrative appearance, marked diffusion restriction, and iron sparing in solid mass). On the independent validation testing set, the prediction model demonstrated moderate diagnostic performance and gool calibration for GPC-3 expression.
Previous studies have revealed that GPC-3 is actively involved in regulating HCC tumor growth (22, 23). Shirakawa et al. (24) reported that positive GPC-3 expression is correlated with poor clinical prognosis for HCC patients, and Li et al. (25) illustrated that GPC-3 is a coreceptor with the activation of the Wnt signaling pathway. The authors considered it to be a potential target site for HCC therapy that restrains Wnt signaling. Currently, novel treatments for HCC have been explored and assessed in in vitro and in vivo experiments and clinical trials targeting GPC-3, such as CAR T cell therapy (26), immunotoxin therapy (27), and GPC-3-derived peptide vaccines (28). Therefore, it is of great significance to evaluate the expression of GPC-3 in HCC preoperatively, noninvasively and precisely. Chen et al. (19) reported that the R2* value yielded from iterative decomposition of water and fat with echo asymmetry and least squares estimation MRI could reliably predict GPC-3 expression in HCC prior to surgery. In addition, Gu et al. (18) demonstrated that the proposed MR-based radiomics signature is strongly related to GPC-3 positivity and incorporating AFP levels and radiomics signatures may noninvasively and individually predict GPC-3-positive HCC. In our study, 278 solitary patients were included who underwent hepatectomy, and postoperative pathology assessed GPC-3 expression. A total of 208 (74.8%) of the HCC lesions were GPC-3 positive, and 70 (25.2%) were negative. The results revealed that AFP>10 ng/ml, tumor size>3.0 cm, nonperipheral “washout”, infiltrative appearance, marked diffusion restriction, and iron sparing in solid mass were significantly associated with GPC-3 positive expression. Chu et al. (29) illustrated a GPC3-based immunomagnetic fluorescent system (C6/MMSN-GPC3) that showed the high-specific isolation and instant observation of HCC circulating tumor cells. But in our study, we directly assessed the GPC-3 expression in HCC tumor tissues by MRI features which no need peripheral blood and without radiation.
The liver imaging reporting and data system (LI-RADS) is supported by the American College of Radiology (https://www.acr.org) and provides standardization of liver imaging and reporting. It offers four individual imaging algorithms designed for different clinical contexts of HCC risk patients, which include ultrasound LI-RADS for surveillance, contrast-enhanced US, CT or MRI LI-RADS for diagnosis and staging, and treatment response LI-RADS to assess response to local-regional therapies. All LI-RADS algorithms are built on the foundation of standardized lexicon, technique, management, and reporting guidelines (30). LI-RADS assigns features that reflect the probability of HCC, nonHCC malignancy, or benignity (31), assesses the association between LI-RADS categories with microvascular invasion (MVI) and histologic grade of HCC (32), predicts the recurrence of HCC after primary liver transplantation within the Milan criteria (33), and evaluates the molecular alterations during hepatocarcinogenesis (34). Zhao et al. (17) enrolled 43 and 100 patients with pathologically confirmed GPC-3 negative and positive patients with contrast-enhanced MRI and diffusion-weighted imaging (DWI) to explore the potential MRI findings in predicting GPC-3 positive HCCs. The results showed that the serum AFP levels and lower 75th percentile apparent diffusion coefficient (ADC) values were helpful in differentiating GPC-3 positive HCCs. However, the 75th percentile ADC value was not included in clinical LI-RADS analysis; in addition to requiring professional software measurement, it has disadvantages such as poor interpretability and inconveniences of clinical application. Therefore, our study comprehensively analyzed the differential ability of LI-RADS MRI features and categories for GPC-3-positive HCC that has not been previously reported in the literature.
After univariate and multivariate regression analyses, AFP, tumor size, nonperipheral “washout”, infiltrative appearance, marked diffusion restriction, and iron sparing in solid mass were selected to construct the prediction model of this study. Based on the 5.5 points threshold, our model demonstrated a specificity of 87.8% in predicting positive GPC-3 expression in HCC. Therefore, considering remarkable incidence of adverse effects and high cost of HCC immunotherapy, our model may help avoid inappropriate treatment initiation, minimize unwanted adverse effect, reduce unnecessary cost, and improve individualized treatment decision-making. AFP is a progenitor cell marker that has been considered useful in the early screening and assisting diagnosis of HCC and as a biomarker helping select candidates in testing drugs in Phase III trials or evaluating curative effects (35, 36). Ye et al. illustrated that patients with high serum AFP levels and GPC-3 positive expression were associated with a poor prognosis (37). Our results showed that there was a positive association between serum AFP levels >10 ng/ml with GPC-3 positive expression.
Nonperipheral “washout” appearance is an important major imaging feature of LI-RADS, it is a visually assessed temporal reduction in enhancement of an observation relative to composite liver tissue from an earlier to a later phase resulting in hypoenhancement on the portal venous (PVP) or delayed phases (38), Kim et al. (39) revealed that nonperipheral “washout” appearance on the PVP or transitional phase is the most reliable MR imaging feature for differentiating hepatocellular carcinoma with paradoxical uptake on the hepatobiliary phase from focal nodular hyperplasia-like nodules. Additionally, our study showed that the nonperipheral “washout” feature is an independent risk factor for evaluating HCC with positive GPC-3. The infiltrative appearance may represent true infiltration of tumor cells into the liver parenchyma, which commonly indicates malignancy with a permeative growth pattern and is associated with macrovascular invasion (40), tumor metastasis and a short survival time (41), Sun et al. (42) reported that the infiltrative tumor margin has the potential to identify PD-L1-positive HCC, similarly our results demonstrated that this MR imaging feature also hints at GPC-3 positive expression. Tumor size is also included as a major feature of LI-RADS since the likelihood of malignancy in a cirrhosis-associated nodule is positively correlated with the size of the observation (43), but the tumor size exceeding 3.0 cm was considered to be more likely associated with negative GPC-3 expression in our study.
Diffusion restriction is an ancillary MR imaging feature favoring malignancy in general. The minimum apparent diffusion coefficient is a significantly independent risk factor for early HCC recurrence after surgery (44), and Joo et al. (45) showed that the diffusion restriction feature was an independent predictor to help differentiate progressed HCC from low- or high-grade nodules because the expression of GPC-3 in HCC is obviously higher than that in low- or high-grade nodules; thus, the finding may confirm our results that marked diffusion restriction is also an independent predictor for GPC-3 positive expression. Also, iron sparing in solid masses is an ancillary LI-RADS feature that usually favors liver malignancy and is defined as a paucity of iron in solid masses relative to iron-overloaded livers or in inner nodules relative to siderotic outer nodules, a paucity of iron suggests clonal expansion of a cell with iron resistance distinct from the background parenchyma (46), the results of our study showed that this LI-RADS feature strongly indicates the GPC-3 negative expression in HCC, which may indicate that malignant hepatic cells with reduced iron intake is more likely to be accompanied by GPC-3 negative expression.
In this study, during the follow-up, 14 (7%) patients died, and 66 (35%) patients experienced tumor recurrence. Despite no significant difference in RFS or OS detected between patients with pathologically or model-predicted confirmed GPC-3 expression, Kaplan–Meier survival curves still showed a trend wise longer survival in patients with GPC-3-negative HCC. The possible reason is that the follow-up time was not long enough, which led to the small number of patients with OS and RFS events, only 7% and 35%, respectively. Nevertheless, this study also suggests that GPC-3 expression is of great significance for the prognosis of HCC patients.
Several limitations in this study should be noted. First, the retrospective nature could have introduced selection biases to the study cohort, and in this predictive model was developed in patients with solitary HCCs to improve radiology-pathology correlations. However, this design also hampered the extrapolation of our findings to multiple tumors. Therefore, future studies are encouraged to explore the correlation between MRI features and GPC-3 expressions in multiple tumors while guaranteeing rigorous radiology-pathology spatial correlation. Second, the number of enrolled patients with GPC-3-negative HCC was relatively small (70 patients [25.2%]), and the imbalanced sample size may have reduced the effectiveness of model training. However, this was in part because we only analyzed data from patients with solitary HCC. This was determined to improve radiology-pathology correlation and to minimize the interference of confounding factors. Third, although CAR T cell targeting GPC-3 therapy for advanced HCC patients is considered next-generation immunotherapy, our study did not directly evaluate the efficacy of CAR T cell therapy. Nevertheless, this study constructed an easy-to-use predictive model that could be used for noninvasive assessment for GPC-3 expression in HCC, which may provide useful information regarding patient selection for future GPC-3-targeted immunotherapy. Fourth, at present, we only established the model based on the qualitative features of EOB-MRI, although it has the advantages of simple operation and strong interpretation, but it does not use any functional/molecular imaging techniques. Other molecular imaging techniques, for example, positron emission tomography-computed tomography and single-photon emission computed tomography-computed tomography, allow direct depiction for the expression of tumor-specific targets, thus may serve as promising noninvasive tools for GPC-3 expression assessment in HCC (47).
In conclusion, in patients with surgically-confirmed solitary HCC, we developed and validated an easy-to-use noninvasive prediction model which could accurately predict positive GPC-3 HCC based on serum AFP and five EOB-MR imaging features in HCC patients. Our findings may help identify potential responders for GPC-3-targeted immunotherapy and direct personalized treatment decision-making.
The original contributions presented in the study are included in the article/Supplementary Material. Further inquiries can be directed to the corresponding authors.
The studies involving human participants were reviewed and approved by the ethics committee of West China Hospital, Sichuan University. Written informed consent for participation was not required for this study in accordance with the national legislation and the institutional requirements.
YC, YQ and HJ conceived of and designed this study. ZZ and TD performed the experiments. YNW and HJ performed the data analysis. BS and YW reviewed this manuscript. All authors contributed to the article and approved the submitted version.
This work was funded by the National Natural Science Foundation of China (Grant No. 82101997, 81971571) and the Science and Technology Department of Sichuan Province (Grant No. 2022YFS0071, 2021YFS0021, 2021YFS0141).
The authors declare that the research was conducted in the absence of any commercial or financial relationships that could be construed as a potential conflict of interest.
All claims expressed in this article are solely those of the authors and do not necessarily represent those of their affiliated organizations, or those of the publisher, the editors and the reviewers. Any product that may be evaluated in this article, or claim that may be made by its manufacturer, is not guaranteed or endorsed by the publisher.
The Supplementary Material for this article can be found online at: https://www.frontiersin.org/articles/10.3389/fimmu.2022.973153/full#supplementary-material
1. Sung H, Ferlay J, Siegel RL, Laversanne M, Soerjomataram I, Jemal A, et al. Global cancer statistics 2020: Globocan estimates of incidence and mortality worldwide for 36 cancers in 185 countries. CA: Cancer J Clin (2021) 71(3):209–49. doi: 10.3322/caac.21660
2. Singh AK, McGuirk JP. Car T cells: Continuation in a revolution of immunotherapy. Lancet Oncol (2020) 21(3):e168–e78. doi: 10.1016/s1470-2045(19)30823-x
3. Larson RC, Maus MV. Recent advances and discoveries in the mechanisms and functions of car T cells. Nat Rev Cancer (2021) 21(3):145–61. doi: 10.1038/s41568-020-00323-z
4. Gao W, Kim H, Ho M. Human monoclonal antibody targeting the heparan sulfate chains of glypican-3 inhibits hgf-mediated migration and motility of hepatocellular carcinoma cells. PloS One (2015) 10(9):e0137664. doi: 10.1371/journal.pone.0137664
5. Liu X, Gao F, Jiang L, Jia M, Ao L, Lu M, et al. 32a9, a novel human antibody for designing an immunotoxin and car-T cells against glypican-3 in hepatocellular carcinoma. J Trans Med (2020) 18(1):295. doi: 10.1186/s12967-020-02462-1
6. Capurro M, Martin T, Shi W, Filmus J. Glypican-3 binds to frizzled and plays a direct role in the stimulation of canonical wnt signaling. J Cell Sci (2014) 127(Pt 7):1565–75. doi: 10.1242/jcs.140871
7. Filmus J, Capurro M. Glypican-3: A marker and a therapeutic target in hepatocellular carcinoma. FEBS J (2013) 280(10):2471–6. doi: 10.1111/febs.12126
8. Haruyama Y, Kataoka H. Glypican-3 is a prognostic factor and an immunotherapeutic target in hepatocellular carcinoma. World J Gastroenterol (2016) 22(1):275–83. doi: 10.3748/wjg.v22.i1.275
9. Capurro M, Wanless IR, Sherman M, Deboer G, Shi W, Miyoshi E, et al. Glypican-3: A novel serum and histochemical marker for hepatocellular carcinoma. Gastroenterology (2003) 125(1):89–97. doi: 10.1016/s0016-5085(03)00689-9
10. Shi D, Shi Y, Kaseb AO, Qi X, Zhang Y, Chi J, et al. Chimeric antigen receptor-Glypican-3 T-cell therapy for advanced hepatocellular carcinoma: Results of phase I trials. Clin Cancer Res (2020) 26(15):3979–89. doi: 10.1158/1078-0432.Ccr-19-3259
11. Du K, Li Y, Liu J, Chen W, Wei Z, Luo Y, et al. A bispecific antibody targeting Gpc3 and Cd47 induced enhanced antitumor efficacy against dual antigen-expressing hcc. Mol Ther J Am Soc Gene Ther (2021) 29(4):1572–84. doi: 10.1016/j.ymthe.2021.01.006
12. Cheng H, Fan X, Ye E, Chen H, Yang J, Ke L, et al. Dual tumor microenvironment remodeling by glucose-contained radical copolymer for mri-guided photoimmunotherapy. Advanced Materials (2022) 34(25):2107674. doi: 10.1002/adma.202107674
13. Cao L, Cheng H, Jiang Q, Li H, Wu Z. Apex1 is a novel diagnostic and prognostic biomarker for hepatocellular carcinoma. Aging (2020) 12(5):4573–91. doi: 10.18632/aging.102913
14. Zhao M, Liu Z, Dong L, Zhou H, Yang S, Wu W, et al. A Gpc3-specific aptamer-mediated magnetic resonance probe for hepatocellular carcinoma. Int J nanomed (2018) 13:4433–43. doi: 10.2147/ijn.S168268
15. Ma XH, Wang S, Liu SY, Chen K, Wu ZY, Li DF, et al. Development and in vitro study of a bi-specific magnetic resonance imaging molecular probe for hepatocellular carcinoma. World J Gastroenterol (2019) 25(24):3030–43. doi: 10.3748/wjg.v25.i24.3030
16. Li Y, Chen Z, Li F, Wang J, Zhang Z. Preparation and in vitro studies of mri-specific superparamagnetic iron oxide Antigpc3 probe for hepatocellular carcinoma. Int J nanomed (2012) 7:4593–611. doi: 10.2147/ijn.S32196
17. Zhao J, Gao S, Sun W, Grimm R, Fu C, Han J, et al. Magnetic resonance imaging and diffusion-weighted imaging-based histogram analyses in predicting glypican 3-positive hepatocellular carcinoma. Eur J Radiol (2021) 139:109732. doi: 10.1016/j.ejrad.2021.109732
18. Gu D, Xie Y, Wei J, Li W, Ye Z, Zhu Z, et al. Mri-based radiomics signature: A potential biomarker for identifying glypican 3-positive hepatocellular carcinoma. J Magn Reson Imaging (2020) 52(6):1679–87. doi: 10.1002/jmri.27199
19. Chen R, Bai Y, Liu T, Zhang G, Han Y, Chen L, et al. Evaluation of glypican-3 expression in hepatocellular carcinoma by using ideal iq magnetic resonance imaging. Acad Radiol (2021) 28(8):e227–e34. doi: 10.1016/j.acra.2020.05.015
20. Liao B, Liu L, Wei L, Wang Y, Chen L, Cao Q, et al. Innovative synoptic reporting with seven-point sampling protocol to improve detection rate of microvascular invasion in hepatocellular carcinoma. Front Oncol (2021) 11:726239. doi: 10.3389/fonc.2021.726239
21. Takai H, Kato A, Ishiguro T, Kinoshita Y, Karasawa Y, Otani Y, et al. Optimization of tissue processing for immunohistochemistry for the detection of human glypican-3. Acta histochem (2010) 112(3):240–50. doi: 10.1016/j.acthis.2008.11.025
22. Gao W, Kim H, Feng M, Phung Y, Xavier CP, Rubin JS, et al. Inactivation of wnt signaling by a human antibody that recognizes the heparan sulfate chains of glypican-3 for liver cancer therapy. Hepatol (Baltimore Md) (2014) 60(2):576–87. doi: 10.1002/hep.26996
23. Lai JP, Oseini AM, Moser CD, Yu C, Elsawa SF, Hu C, et al. The oncogenic effect of sulfatase 2 in human hepatocellular carcinoma is mediated in part by glypican 3-dependent wnt activation. Hepatol (Baltimore Md) (2010) 52(5):1680–9. doi: 10.1002/hep.23848
24. Shirakawa H, Suzuki H, Shimomura M, Kojima M, Gotohda N, Takahashi S, et al. Glypican-3 expression is correlated with poor prognosis in hepatocellular carcinoma. Cancer Sci (2009) 100(8):1403–7. doi: 10.1111/j.1349-7006.2009.01206.x
25. Li N, Wei L, Liu X, Bai H, Ye Y, Li D, et al. A frizzled-like cysteine-rich domain in glypican-3 mediates wnt binding and regulates hepatocellular carcinoma tumor growth in mice. Hepatol (Baltimore Md) (2019) 70(4):1231–45. doi: 10.1002/hep.30646
26. Pan Z, Di S, Shi B, Jiang H, Shi Z, Liu Y, et al. Increased antitumor activities of glypican-3-Specific chimeric antigen receptor-modified T cells by coexpression of a soluble Pd1-Ch3 fusion protein. Cancer immunol immunother CII (2018) 67(10):1621–34. doi: 10.1007/s00262-018-2221-1
27. Gao W, Tang Z, Zhang YF, Feng M, Qian M, Dimitrov DS, et al. Immunotoxin targeting glypican-3 regresses liver cancer Via dual inhibition of wnt signalling and protein synthesis. Nat Commun (2015) 6:6536. doi: 10.1038/ncomms7536
28. Sawada Y, Yoshikawa T, Ofuji K, Yoshimura M, Tsuchiya N, Takahashi M, et al. Phase ii study of the Gpc3-derived peptide vaccine as an adjuvant therapy for hepatocellular carcinoma patients. Oncoimmunology (2016) 5(5):e1129483. doi: 10.1080/2162402x.2015.1129483
29. Chu Q, Mu W, Lan C, Liu Y, Gao T, Guan L, et al. High-specific isolation and instant observation of circulating tumour cell from hcc patients Via glypican-3 immunomagnetic fluorescent nanodevice. Int J nanomed (2021) 16:4161–73. doi: 10.2147/ijn.S307691
30. Chernyak V, Fowler KJ, Kamaya A, Kielar AZ, Elsayes KM, Bashir MR, et al. Liver imaging reporting and data system (Li-rads) version 2018: Imaging of hepatocellular carcinoma in at-risk patients. Radiology (2018) 289(3):816–30. doi: 10.1148/radiol.2018181494
31. Santillan C, Chernyak V, Sirlin C. Li-Rads categories: Concepts, definitions, and criteria. Abdominal Radiol (New York) (2018) 43(1):101–10. doi: 10.1007/s00261-017-1334-x
32. Wei H, Jiang H, Liu X, Qin Y, Zheng T, Liu S, et al. Can Li-rads imaging features at gadoxetic acid-enhanced mri predict aggressive features on pathology of single hepatocellular carcinoma? Eur J Radiol (2020) 132:109312. doi: 10.1016/j.ejrad.2020.109312
33. Lee S, Kim KW, Jeong WK, Jeong SY, Hwang JA, Choi JS, et al. Liver imaging reporting and data system category on magnetic resonance imaging predicts recurrence of hepatocellular carcinoma after liver transplantation within the Milan criteria: A multicenter study. Ann Surg Oncol (2021) 28(11):6782–9. doi: 10.1245/s10434-021-09772-8
34. Narsinh KH, Cui J, Papadatos D, Sirlin CB, Santillan CS. Hepatocarcinogenesis and Li-rads. Abdominal Radiol (New York) (2018) 43(1):158–68. doi: 10.1007/s00261-017-1409-8
35. Park H, Kim SU, Park JY, Kim DY, Ahn SH, Chon CY, et al. Clinical usefulness of double biomarkers afp and pivka-ii for subdividing prognostic groups in locally advanced hepatocellular carcinoma. Liver Int (2014) 34(2):313–21. doi: 10.1111/liv.12274
36. Zhu AX, Park JO, Ryoo BY, Yen CJ, Poon R, Pastorelli D, et al. Ramucirumab versus placebo as second-line treatment in patients with advanced hepatocellular carcinoma following first-line therapy with sorafenib (Reach): A randomised, double-blind, multicentre, phase 3 trial. Lancet Oncol (2015) 16(7):859–70. doi: 10.1016/s1470-2045(15)00050-9
37. Ye L, Li D, Chen Y, Yu X. Evaluation for clinical and prognostic implications of glypican-3 and A-fetoprotein in hepatocellular carcinoma: A new subtype? Trans Cancer Res (2020) 9(5):3443–52. doi: 10.21037/tcr-19-1803
38. Santillan C, Fowler K, Kono Y, Chernyak V. Li-Rads major features: Ct, mri with extracellular agents, and mri with hepatobiliary agents. Abdominal Radiol (New York) (2018) 43(1):75–81. doi: 10.1007/s00261-017-1291-4
39. Kim JW, Lee CH, Kim SB, Park BN, Park YS, Lee J, et al. Washout appearance in gd-Eob-Dtpa-Enhanced Mr imaging: A differentiating feature between hepatocellular carcinoma with paradoxical uptake on the hepatobiliary phase and focal nodular hyperplasia-like nodules. J Magn Reson Imaging (2017) 45(6):1599–608. doi: 10.1002/jmri.25493
40. Vernuccio F, Porrello G, Cannella R, Vernuccio L, Midiri M, Giannitrapani L, et al. Benign and malignant mimickers of infiltrative hepatocellular carcinoma: Tips and tricks for differential diagnosis on ct and mri. Clin Imaging (2021) 70:33–45. doi: 10.1016/j.clinimag.2020.10.011
41. An C, Zuo M, Li W, Chen Q, Wu P. Infiltrative hepatocellular carcinoma: Transcatheter arterial chemoembolization versus hepatic arterial infusion chemotherapy. Front Oncol (2021) 11:747496. doi: 10.3389/fonc.2021.747496
42. Sun L, Mu L, Zhou J, Tang W, Zhang L, Xie S, et al. Imaging features of gadoxetic acid-enhanced Mr imaging for evaluation of tumor-infiltrating Cd8 cells and pd-L1 expression in hepatocellular carcinoma. Cancer immunol immunother CII (2022) 71(1):25–38. doi: 10.1007/s00262-021-02957-w
43. Forner A, Vilana R, Ayuso C, Bianchi L, Solé M, Ayuso JR, et al. Diagnosis of hepatic nodules 20 mm or smaller in cirrhosis: Prospective validation of the noninvasive diagnostic criteria for hepatocellular carcinoma. Hepatol (Baltimore Md) (2008) 47(1):97–104. doi: 10.1002/hep.21966
44. Lee S, Kim SH, Hwang JA, Lee JE, Ha SY. Pre-operative adc predicts early recurrence of hcc after curative resection. Eur Radiol (2019) 29(2):1003–12. doi: 10.1007/s00330-018-5642-5
45. Joo I, Kim SY, Kang TW, Kim YK, Park BJ, Lee YJ, et al. Radiologic-pathologic correlation of hepatobiliary phase hypointense nodules without arterial phase hyperenhancement at gadoxetic acid-enhanced mri: A multicenter study. Radiology (2020) 296(2):335–45. doi: 10.1148/radiol.2020192275
46. Chernyak V, Tang A, Flusberg M, Papadatos D, Bijan B, Kono Y, et al. Li-Rads(®) ancillary features on ct and mri. Abdominal Radiol (New York) (2018) 43(1):82–100. doi: 10.1007/s00261-017-1220-6
Keywords: magnetic resonance imaging, glypican-3, hepatocellular carcinoma, immunotherapy, diagnosis
Citation: Chen Y, Qin Y, Wu Y, Wei H, Wei Y, Zhang Z, Duan T, Jiang H and Song B (2022) Preoperative prediction of glypican-3 positive expression in solitary hepatocellular carcinoma on gadoxetate-disodium enhanced magnetic resonance imaging. Front. Immunol. 13:973153. doi: 10.3389/fimmu.2022.973153
Received: 19 June 2022; Accepted: 26 July 2022;
Published: 25 August 2022.
Edited by:
Yueyong Xiao, Chinese People’s Liberation Army General Hospital, ChinaCopyright © 2022 Chen, Qin, Wu, Wei, Wei, Zhang, Duan, Jiang and Song. This is an open-access article distributed under the terms of the Creative Commons Attribution License (CC BY). The use, distribution or reproduction in other forums is permitted, provided the original author(s) and the copyright owner(s) are credited and that the original publication in this journal is cited, in accordance with accepted academic practice. No use, distribution or reproduction is permitted which does not comply with these terms.
*Correspondence: Hanyu Jiang, aGFueXVfamlhbmdAZm94bWFpbC5jb20=; Bin Song, Y2pyLnNvbmdiaW5AdmlwLjE2My5jb20=
†These authors have contributed equally to this work
Disclaimer: All claims expressed in this article are solely those of the authors and do not necessarily represent those of their affiliated organizations, or those of the publisher, the editors and the reviewers. Any product that may be evaluated in this article or claim that may be made by its manufacturer is not guaranteed or endorsed by the publisher.
Research integrity at Frontiers
Learn more about the work of our research integrity team to safeguard the quality of each article we publish.