- 1Department of Obstetrics and Gynecology, The First Affiliated Hospital of Jinan University, Guangzhou, China
- 2International School, Jinan University, Guangzhou, China
- 3Shunde Hospital Affiliated to Jinan University, Guangzhou, China
- 4Department of General Surgery, The First Affiliated Hospital of Jinan University, Guangzhou, China
- 5College of Science and Engineering, Jinan University, Guangzhou, China
Background: Nowadays, immune checkpoint inhibitors (ICIs) have become one of the essential immunotherapies for cancer patients. However, the impact of antibiotic (ATB) use on cancer patients treated with ICIs remains controversial.
Methods: Our research included retrospective studies and a randomized clinical trial (RCT) with cancer patients treated with ICIs and ATB, from the public database of PubMed, Web of Science, Embase, Cochrane, clinical trials, and JAMA. The survival outcomes included progression-free survival (PFS) and overall survival (OS). Meanwhile, hazard ratios (HRs) and 95% confidence intervals (CIs) were calculated, and subgroup analyses were performed to determine the concrete association between ATB use and the prognosis of cancer patients treated in ICIs.
Results: Our results revealed that ATB use was associated with poor survival outcomes, including OS (HR: 1.94, 95% CI: 1.68–2.25, p <0.001) and PFS (HR: 1.83, 95% CI: 1.53–2.19, p <0.001). The subgroup analysis learned about the association between ATB use and the prognosis of cancer patients with ICI treatment, including 5 cancer types, 3 kinds of ICI, 5 different ATP windows, broad-spectrum ATB class, and ECOG score. ATB treatment was associated with poor OS of non-small-cell lung cancer (NSCLC), renal cell carcinoma (RCC), esophageal cancer (EC), and melanoma (MEL) in patients treated in ICIs, while non-small-cell lung cancer (NSCLC) and renal cell carcinoma (RCC) were associated with poor PFS. Meanwhile, it was strongly related to the ICI type and ATB window. Furthermore, it is firstly mentioned that the use of broad-spectrum ATB class was strongly associated with poor PFS.
Conclusion: In conclusion, our meta-analysis indicated that ATB use was significantly associated with poor OS and PFS of cancer patients treated with ICI immunotherapy, especially for patients with ATB use in the period of (−60 days; +30 days) near the initiation of ICI treatment. Also, different cancer types and the ICI type can also impact the survival outcome. This first reveals the strong relationship between the broad-spectrum ATB class and poor PFS. Still, more studies are needed for further study.
Introduction
Working via the anti-tumor immune response, immune checkpoint inhibitors (ICIs) have proved a promising therapeutic treatment in the clinic, which was designed to interfere with inhibitory pathways that naturally constrain T cell reactivity (1). ICIs reinvigorate anti-tumor immune responses by disrupting co-inhibitory T-cell signaling (2). In the last decade, ICIs have caused a major paradigm shift in cancer therapy. It has been approved for various cancers and has improved the survival outcome for many patients (3). However, although ICIs did improve the survival outcome of cancer treatment, the efficacy of the ICI drugs is still limited due to refractiveness, and there are still some uncertain points regarding ICI therapy (4). Additionally, the use of ICIs can induce unique side effects called immune-related adverse events, which can vary a lot in different individuals (5). Some patients exhibit an atypical treatment response pattern with new or enlarging lesions, which needs further observation to determine the process (6). The side effects of ICI therapy involve various organs and systems, including the thyroid and pituitary glands, skin, and digestive system and respiratory system, which can markedly affect the physiological function of organs and the quality of life of patients, even causing fatal consequences in some extreme cases (7). Thus, it is urgent and necessary to find the novel biomarkers to select the patients who can most benefit from the drugs that are in need of being identified.
Antibiotic (ATB) therapy has produced indispensable advances for patients with cancer, populations who are more easily get infected by bacterial because of treatment-related immune suppression. The derangement of the gut microbiota environment has been increasingly well-characterized because of the existence of tumor-specific immune tolerogenesis (8). However, the association between ATB use and the prognosis of cancer patients in ICI treatment remains controversial. Some studies have reported that antibiotic use can result in reduced efficacy of immune checkpoint inhibitors, which can be the consequence of dysbiosis of the intestinal microbiome, a main determinant of the cancer-immune set point of patients (9). Meanwhile, the perturbation of the gut microbiota has been indicated as a possible mechanism to explain the adverse effects attributed to antibiotic exposure in the context of ICI therapy (10). Some studies have found that exposure to antibiotic therapy can influence the probability of response to ICI and predict worse patient survival across malignancies (11). However, ATB use can eliminate the infection and improve the quality of infected patients. Therefore, it is necessary to determine whether ATB use affects the efficacy of ICI treatment and the prognosis of cancer patients.
To learn about the specific association between ATB use and ICI treatment of cancer patients and provide potential reference to clinic performance, the current meta-analysis was performed to clarify if ATB use will impact the survival outcome of cancer patients treated in ICIs, and whether any clinical factors could be used to predict the response of patients to ICIs.
Materials and methods
Literature searching strategy
Our meta-analysis protocol was submitted to the International Prospective Register of Systematic Reviews (PROSPERO CRD 42022330156), and this research followed the Preferred Reporting Items for Systematic Reviews and Meta-Analyses guidelines. Electronic databases including PubMed, Web of Science, Embase, Cochrane Library, and Clinical Trials were searched using MeSH words obtained from the National Center for Biotechnology Information (NCBI). Furthermore, the reference lists of eligible reports were also searched to identify potentially relevant studies (“Antibiotics, Antitubercular” AND “Antibiotics, Antineoplastic” AND “Anti-Bacterial Agents”) AND (“Immune Checkpoint Inhibitors” OR “Immune Checkpoint Inhibitors” (Pharmacological Action) OR “Immune Checkpoint Proteins”) were used as the search query.
Inclusion and exclusion criteria
These criteria were developed by all the authors. Inclusion criteria: (I) publications studied the ATB use in cancer patients with ICI treatment; (II) patients were divided into different groups, according to whether they were treated with ATB; (III) the studies should include standard and sufficient data; (IV) research data must be obtained independently by relative organizations; and (V) the publication language was English. Exclusion criteria: (I) duplicate publications and data; (II) relevant research data in the literature comes from public databases; (III) literature types are reviews, case reports, meeting abstracts, and basic experimental research literature; and (IV) literature language is not English.
Data extracting and quality assessment
From each of the included literature, the following data were collected: the name of author, publication year, country or area, the number of patients, study design, cancer type, ICI treatment, antibiotic treatment information (ATB window and drug type), median OS, median PFS, survival outcome (OS and PFS). Meanwhile, to learn about the concrete relationships between ATB treatment and clinical features of ICI-treated cancer patients, the baseline characteristics of patients, including gender, ICI line, cancer stage grade, and lung cancer, were also recorded. These data were reported in a standardized data extraction spreadsheet for further analysis. The quality assessment of eligible studies was done independently using the Newcastle–Ottawa scale.
Statistical analysis
The meta-analysis was conducted to calculate the pooled HRs with corresponding 95% Confidential Intervals (CIs) by using Review Manager 5.4 software for Mac. To avoid the potential heterogeneity affection, a random-effects model was chosen to analyze the survival outcome. Moreover, the dichotomous and generic inverse variance method models were adopted to analyze the extracted data. Statistical heterogeneity was assessed using the χ2 test and the I2 test, and publication bias was assessed by funnel plots. Statistical significance was considered in this study when p <0.05.
Results
Study selection
The initial literature search identified 772 reports, including 409 reports from PubMed, 188 reports from the Web of Science, 103 reports from the Embase database, 24 from the Cochrane database, 30 from clinical trials, and 18 from the JAMA database. After removing duplicate reports, 678 pieces of literature were considered potentially eligible. Finally, according to the above including and excluding criteria, 45 articles were selected, including 12,493 patients. The survival outcomes were composed of progress-free survival outcomes (PFS) and overall survival (OS). The study selection flowchart isshown in Figure 1.
Baseline characteristics of included studies
The eligible studies included 12,493 patients and 13 kinds of cancer types: lung cancer, head and neck cancers, renal cell carcinoma, acute myelocytic leukemia, melanoma, urothelial carcinoma, esophageal squamous cell carcinoma, liver cancer, porocarcinoma, digestive tract carcinoma, Hodgkin’s lymphoma, cervical cancer, and cholangiocarcinoma. The publication year ranged from 2017 to 2022, and the studies were performed in 17 different areas. Among all the studies, five of them used randomized controlled trial (RCT) designs, while other studies were retrospective. Seventeen of the studies used only one kind of ICI, including PD-1 (programmed cell death protein 1) inhibitor, PD-L1 (programmed cell death 1 ligand 1) inhibitor, and CTLA-4 (cytotoxic T-lymphocyte-associated protein 4) inhibitor (PD-1 inhibitors: n = 12; PD-L1 inhibitors: n = 4; CTLA-4 inhibitors: n = 1). While four of them were not definite, the ICI therapy and the other research used a combination of different ICI treatments for at least two of them. The detailed information is shown in Table 1.
Analysis of ATB use and clinic features
In this study, we performed a meta-analysis between ATB use and clinic features, including ECOG score (≤), PD-L1 expression (<1%), non-small-cell lung cancer (NSCLC) patients, gender (male) and ICI therapy line (0–1 prior), which were shown in Supplementary Material. However, we did not observe a clear significant association of these factors (p >0.05), except for the ECOG score (≤1). Among all the eligible studies, 20 studies were chosen to analyze the relationship between ATB use in ICI treatment and ECOG scores. The results showed that the cancer patients with ATB use in the clinic were associated with a lower ECOG score (≤1) in importance significance (HR: 0.69, 95% CI: 0.49–0.98, p = 0.04).
The association between ATB use and survival outcomes (OS + PFS)
Thirty-seven studies were selected to analyze overall survival (OS). The results revealed that ATB use was strongly associated with the increased risk of poor OS (HR: 1.94, 95% CI: 1.68–2.25, p <0.00001), shown in Figure 2A. However, a clear heterogeneity was observed in this analysis (I2 = 84%). Moreover, 31 studies were selected to perform progression-free survival (PFS). The results shown in Figure 2B indicated that ATB use was significantly associated with worse PFS (HR: 1.83, 95% CI: 1.53–2.19, p <0.00001), but also with an obvious heterogeneity (I2 = 86%).
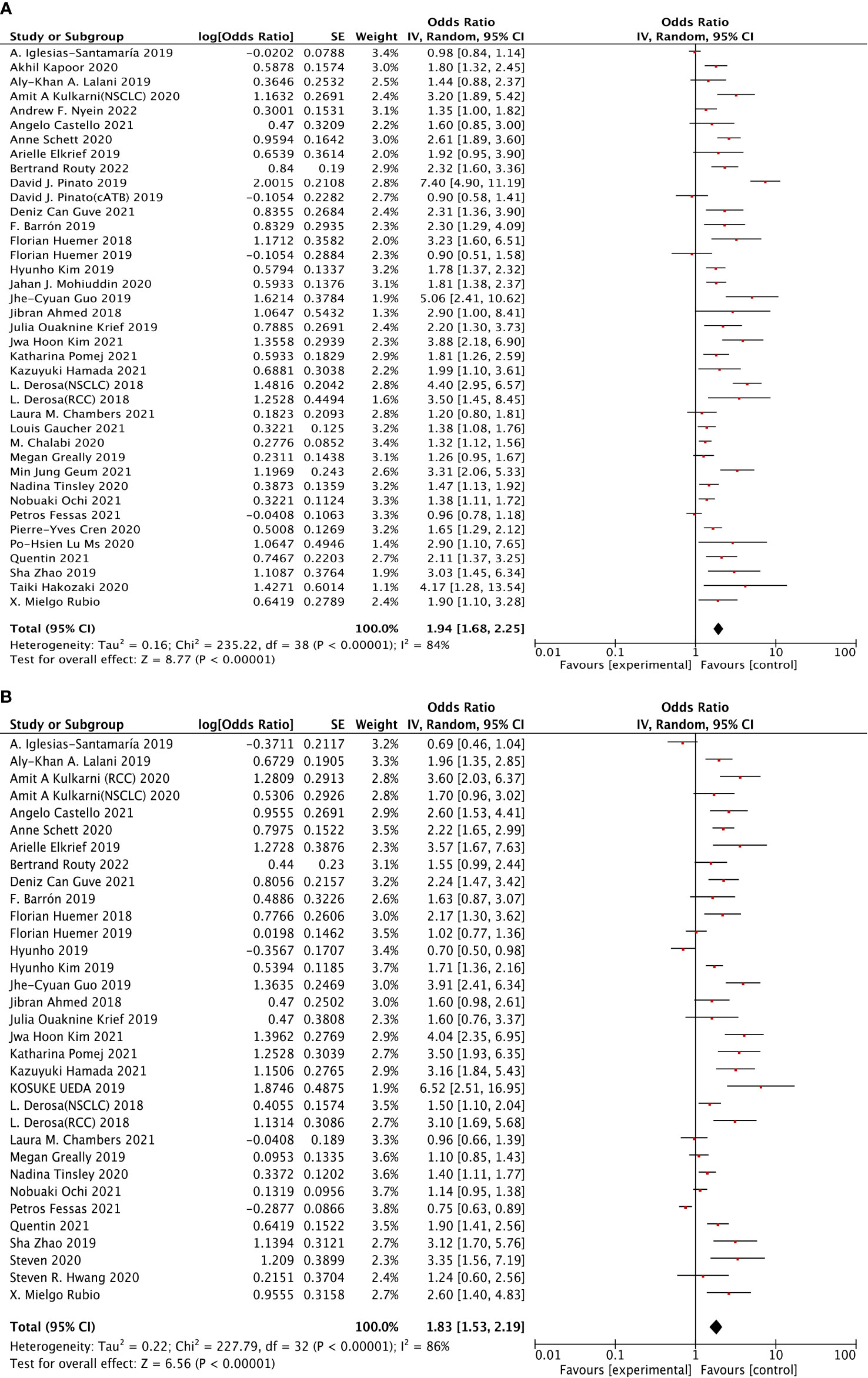
Figure 2 The forest plot showing the relationship between ATB use and OS, PFS in cancer patients treated with ICIs. Overall survival (OS), progress-free survival (PFS); CI, confidential interval; Random, random-effects model; The random-effects model was adopted. (A) Overall survival (OS). (B) Progress-free survival (PFS). (A) Relationship between ATB use and OS in cancer patients treated with ICIs. (B) Relationship between ATB use and PFS in cancer patients treated with ICIs.
Sensitivity analysis
For further verification to identify the association between ATB use and the survival outcomes (OS + PFS) in ICI-treated cancer patients, we performed the same analysis in randomized controlled trial studies as the sensitivity analysis. Three RCT studies were selected. It revealed a similar result as above, that in cancer patients treated with ICIs, ATB use was significantly related to poor OS (HR: 3.13, 95% CI: 1.25–7.84, p <0.001, I2 = 90%) and poor PFS (HR: 2.54, 95% CI: 1.38–4.68, p <0.001, I2 = 70%) (Figure 3).
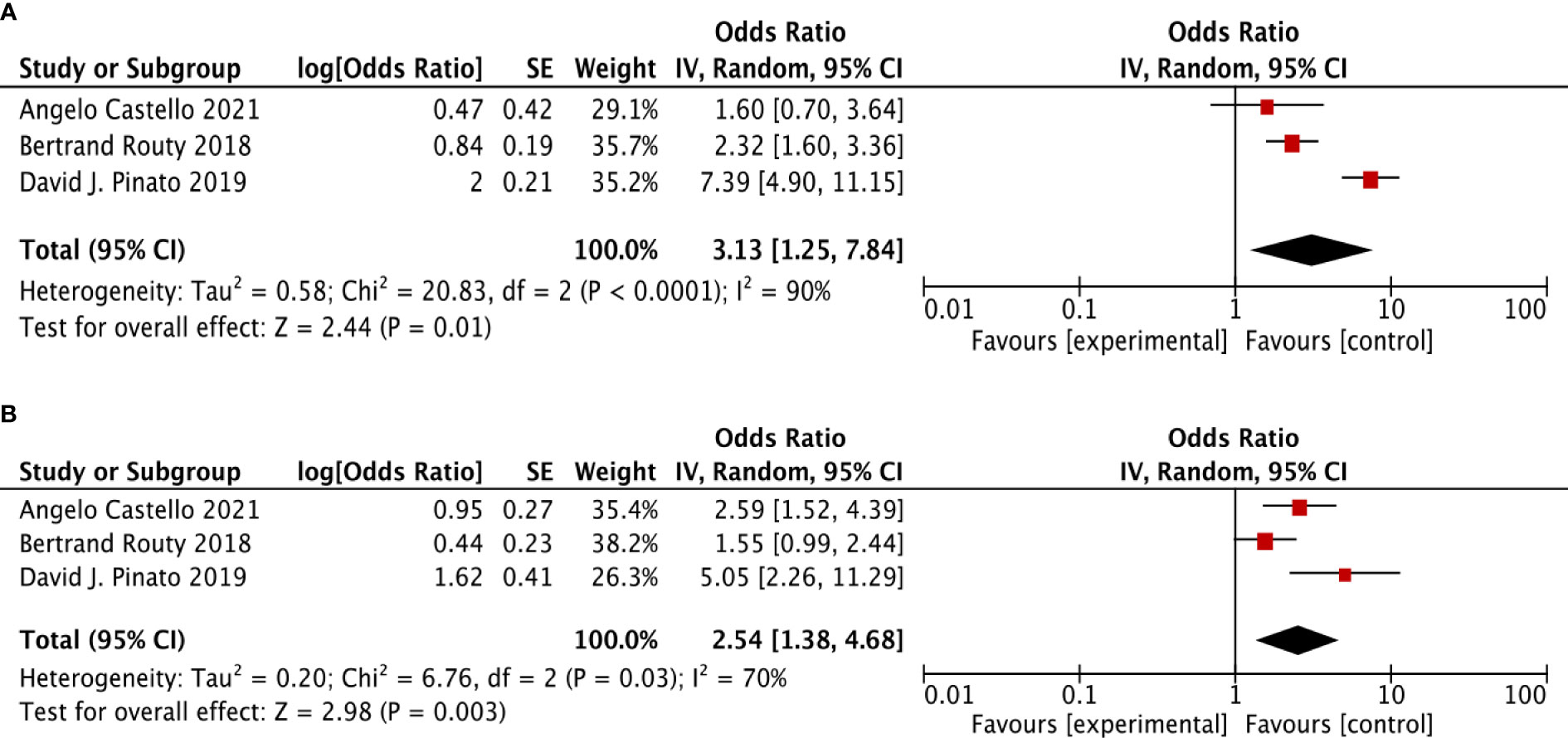
Figure 3 The forest plot showing the relationship between ATB use and OS, PFS in cancer patients treated with ICIs, based on randomized controlled trial (RCT). Overall survival (OS), progress-free survival (PFS); CI, confidential interval; Random, random-effects model. The random-effects model was adopted. (A) Overall survival (OS). (B) progress-free survival (PFS).
In NSCLC, RCC, HCC, EC, and MEL, how does the ATB use impact the prognosis (OS + PFS) in patients treated in ICIs?
For cancer types, we chose NSCLC, RCC, HCC, EC, and MEL to observe. In the OS sub-group analysis, seventeen studies were selected for NSCLC, two studies were selected for RCC, two studies were selected for HCC, three studies were selected for EC, and four studies were selected for MEL. NSCLC (HR: 2.09, 95% CI: 1.69–2.58), RCC (HR: 1.81, 95% CI: 1.14–2.87), EC (HR: 2.80, 95% CI: 1.08–7.25), and MEL (HR: 1.94, 95% CI: 1.41–2.67) were shown to be strongly associated with poor OS. However, no significant relationship was observed for HCC. Moreover, four different cancer types were included in the PFS subgroup analysis, including NSCLC, RCC, HCC, and EC, which indicated that NSCLC (HR: 1.81, 95% CI: 1.47–2.24) and RCC (HR: 3.14, 95% CI: 2.16–4.58) cancer types were associated with poor PFS with a strong effect and HCC, whereas EC was not significantly related (Figure 4).
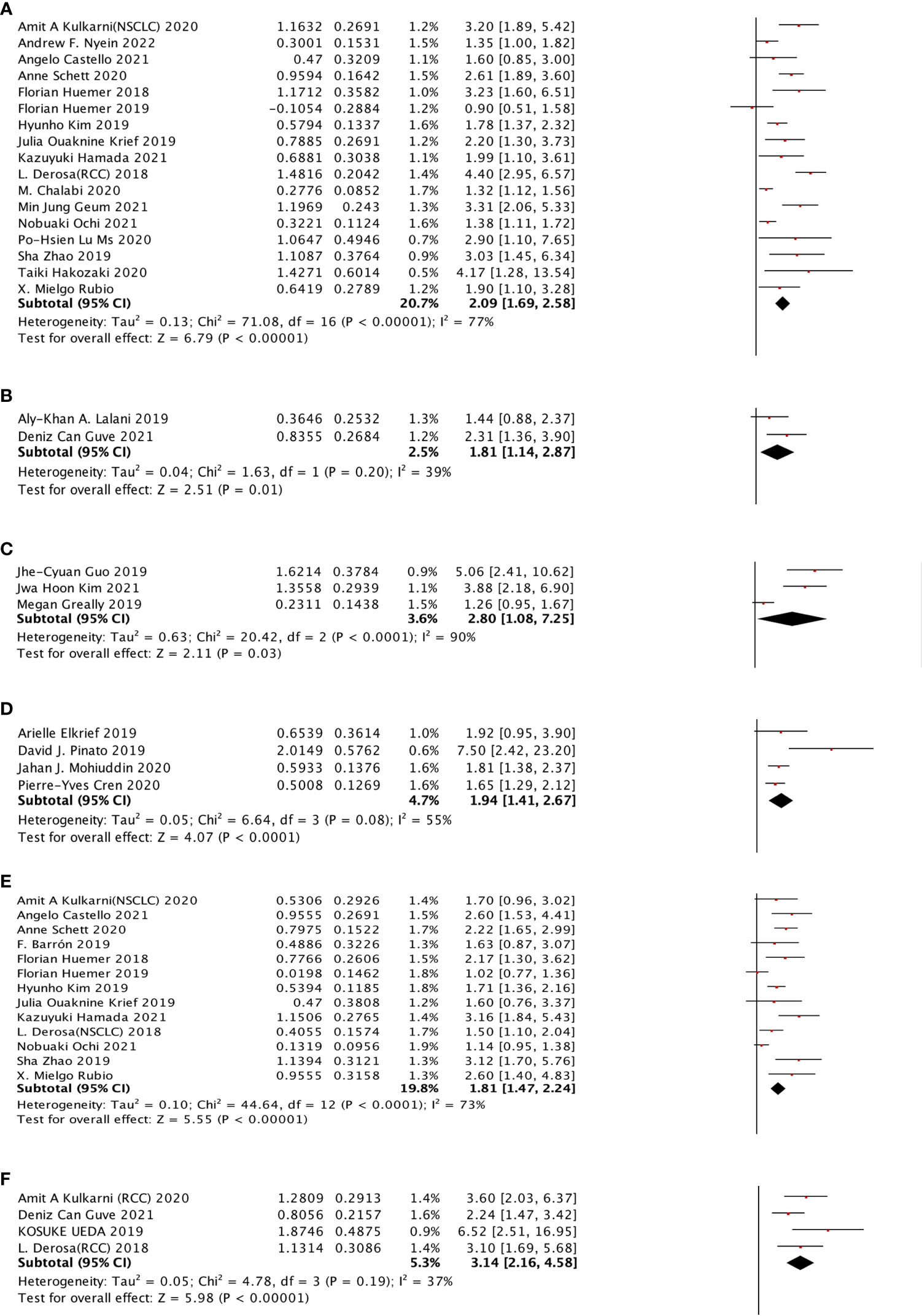
Figure 4 The subgroup analysis between ATB use and cancer prognosis (OS + PFS) of RCC and NSCLC cancer patients treated with ICIs. (A) The relationship between ATB use and OS of NSCLC patients treated with ICIs. (B) The relationship between ATB use and PFS of RCC patients treated with ICIs. (C) The relationship between ATB use and OS of esophagus cancer patients treated with ICIs. (D) The relationship between ATB use and OS of melanoma patients treated with ICIs. (E) The relationship between ATB use and PFS of NSCLC patients treated with ICIs. (F) The relationship between ATB use and PFS of RCC patients treated with ICIs.
In cancer patients treated in PD-1 or PD-L1 ICI type, how does the ATB use impact the prognosis (OS + PFS)?
PD-1 inhibitor, PD-L1 inhibitor, and the combination of PD-1 inhibitor and PD-L1 inhibitor were selected to do the sub-analysis for ICI type. The results showed that all the three types showed a stronger effect on OS (PD-1 inhibitor: HR: 2.20, 95% CI: 1.87–2.60, p <0.00001, I2 = 25%; PD-L1 inhibitor: HR: 1.47, 95% CI: 1.19–1.82; combination of PD-1 inhibitor and PD-L1 inhibitor: HR: 2.30, 95% CI: 1.41–3.75). Meanwhile, the same inhibitor types were observed in the PFS sub-analysis, and only the PD-1 inhibitor (HR: 2.32, 95% CI: 1.83–2.95) and the combination of PD-1 inhibitor and PD-L1 inhibitor (HR: 1.81, 95% CI: 1.20–2.73) showed a significant relationship with PFS (Figure 5).
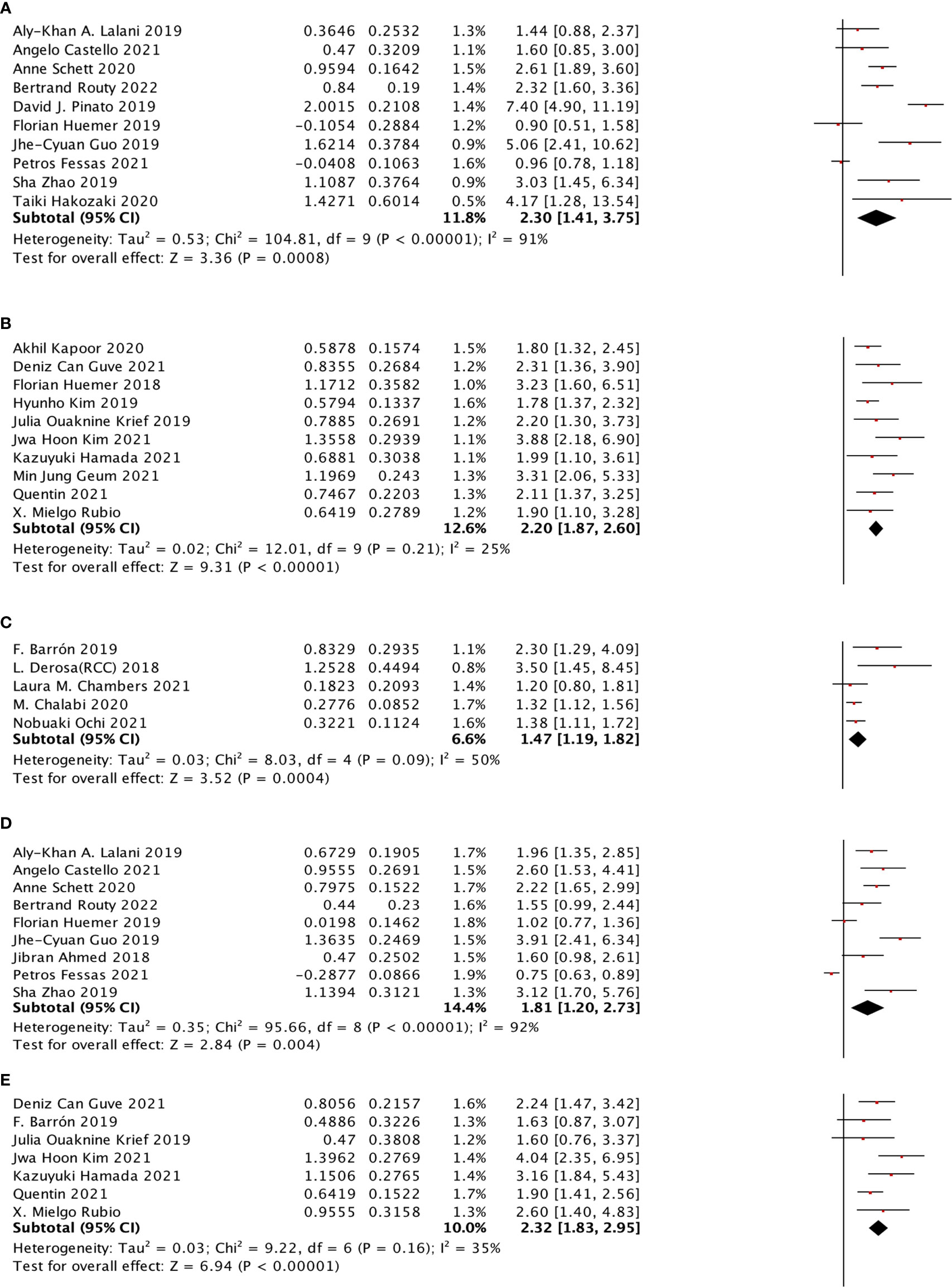
Figure 5 The subgroup analysis between ATB use and different immune checkpoint inhibitors of cancer patients treated with ICIs. (A) The association between ATB use and OS in cancer patients treated with the combination of PD-1 inhibitor and PD-L1 inhibitor. (B) The association between ATB use and OS in cancer patients treated with PD-1 inhibitor. (C) The association between ATB use and OS in cancer patients treated with PD-L1 inhibitor. (D) The association between ATB use and PFS in cancer patients treated with the combination of PD-1 inhibitor and PD-L1 inhibitor. (E) The association between ATB use and PFS in cancer patients treated with PD-1 inhibitor.
What is the relationship between ATB use and survival outcome (OS + PFS) of patients according to different ATB windows?
The selected ATB window included (−60 days, +30 days), (−60 days, 0 day), (−30 days, 30 days), (−30 days, 0 day), (0 day, +30 days) for OS subgroup analysis and (−60 days, +30 days), (−60 days, 0 day), (−30 days, 30 days), (0 day, +30 days) were selected for PFS subgroup analysis. All of these groups were shown to be significantly associated with poor survival outcomes. We also performed the PFS subgroup analysis for using ATB treatment during ICI treatment, and no significant relation was observed (Figure 6).
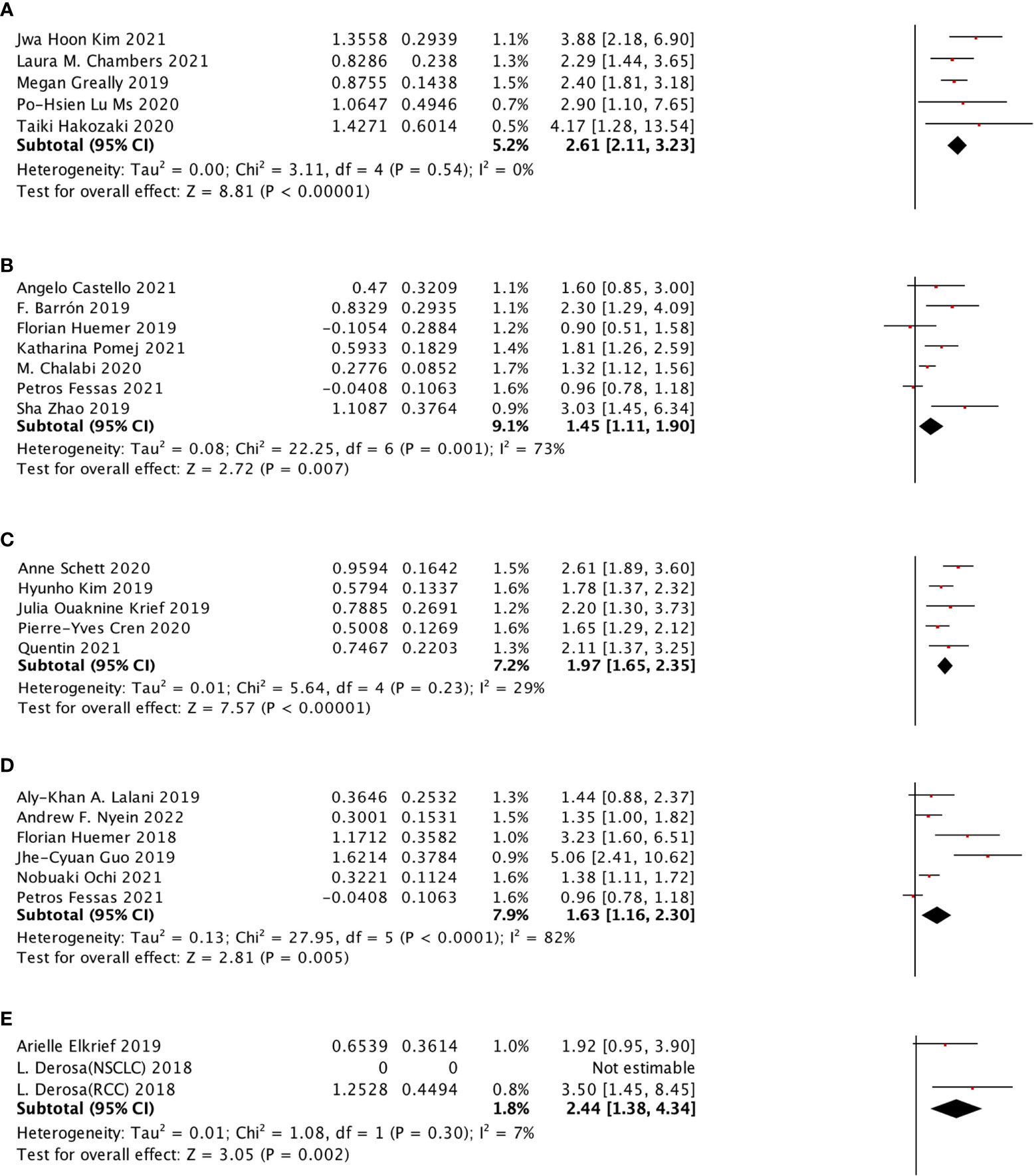
Figure 6 In different ATB windows, the subgroup analysis between ATB use and OS of cancer patients treated with ICIs. (A) ATB window (−30 days, 0 day); (B) ATB window (−30 days, 30 days); (C) ATB window (−60 days, 0 days); (D) ATB window (−60 days, 30 days); and (E) ATB window (0 days, 30 day).
In broad-spectrum ATB class, the relationship of ATB use and PFS
The analysis between the use of broad-spectrum ATB class and PFS of ICI treated cancer patients was also performed, as shown in Figure 7, which was the first mentioned in this research. The result, with no heterogeneity (I2 = 0), revealed that this class was strongly related to poor PFS (HR: 1.86, 95% CI: 1.44–2.41) Figure 8.
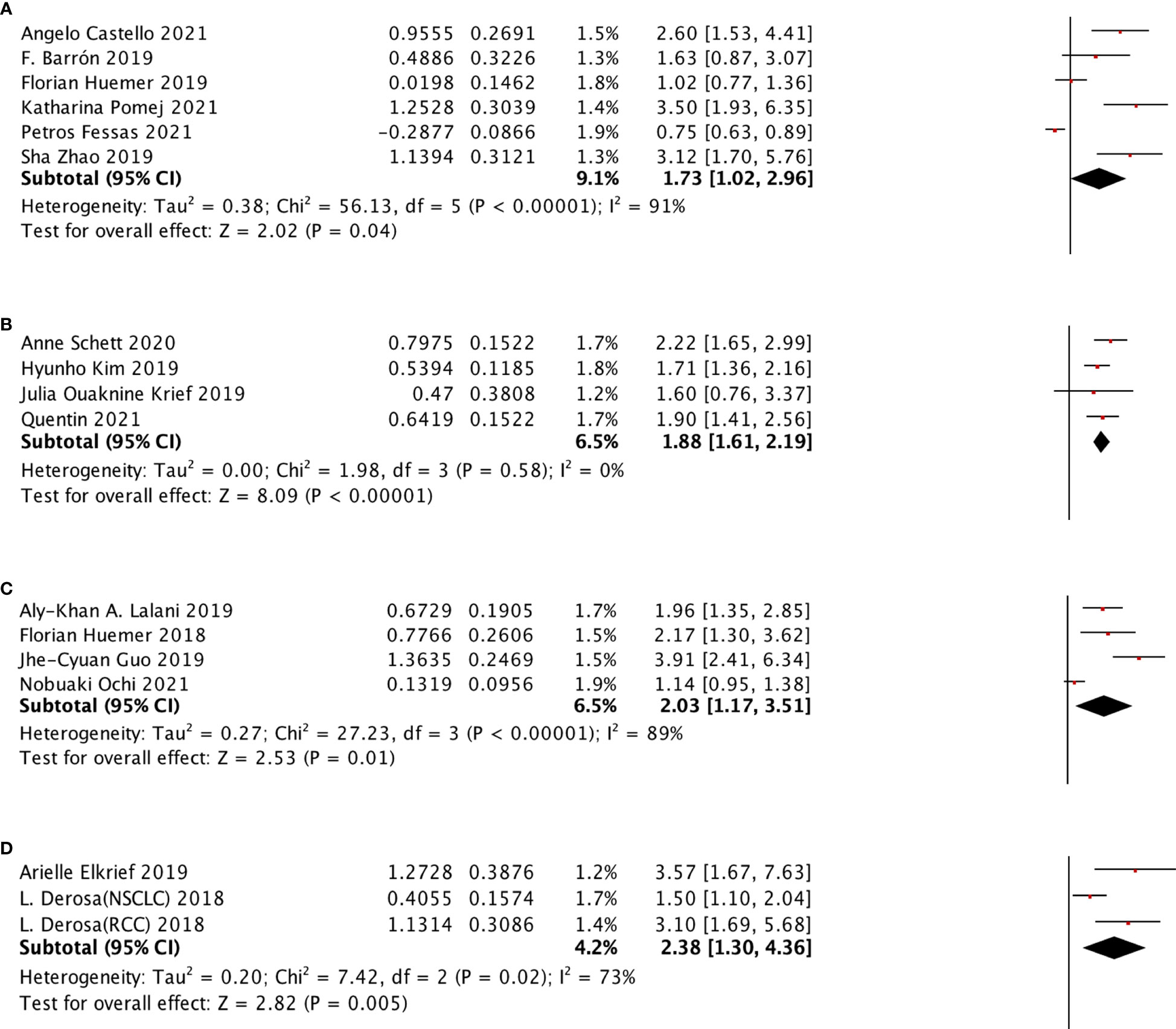
Figure 7 In different ATB window, the subgroup analysis between ATB use and PFS of cancer patients treated with ICIs. (A) ATB window (-30 days, 30 day); (B) ATB window (-60 days, 0 days); (C) ATB window (-60 days, 30 days); (D) ATB window (0 days, 30 days).

Figure 8 In broad- spectrum ATB class, the subgroup analysis between ATB use and PFS of cancer patients treated with ICIs.
ECOG score and OS
No significant difference was observed between higher ECOG score and OS, compared with lower ECOG score (≤1) (HR: 0.49, 95% CI: 0.09–2.76, p = 0.42).
Assessment of publication bias
The publication bias for this research was evaluated by funnel plots, which were collected and shown in the Supplementary Material. There was no obvious publication bias in this research. Newcastle-Ottawa scale scores from 6 to 9 (Table 2). The heterogeneity value also indicated a low publication bias (Table 3).
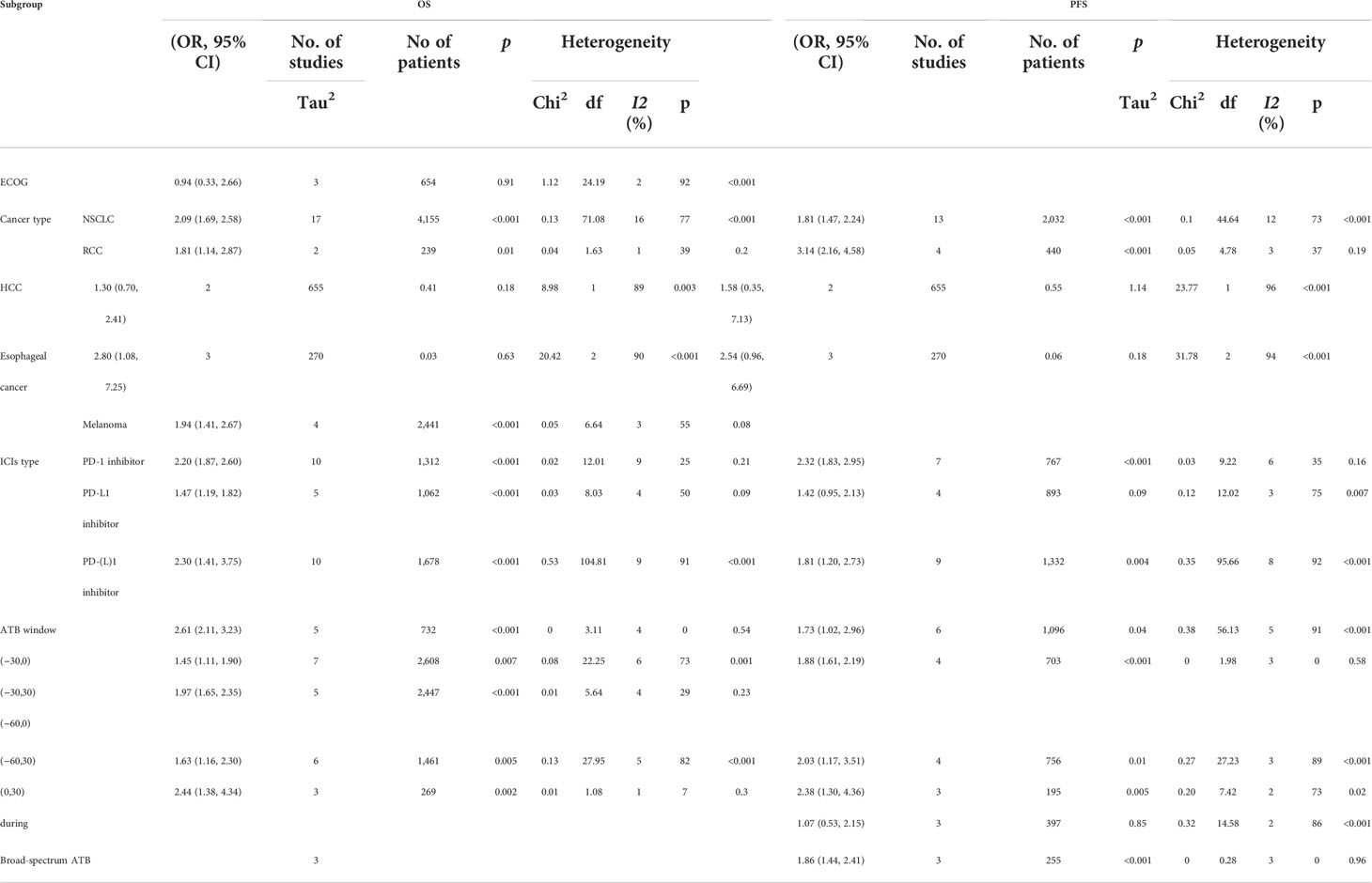
Table 3 Subgroup analysis of ECOG, cancer type, ICI type, and ATB window based on OS (overall survival) and PFS (progress-free survival).
Discussion
This research is the most comprehensive study on the effect of antibiotic use on the clinical features and survival outcomes of cancer patients treated with ICIs, compared with previous meta-analysis until now. In this meta-analysis, from 45 studies with 12,493 patients, the effects of ATB use on OS, PFS, and clinical features were included to study the impacts of ATB use on cancer patients treated with ICI therapy, with RCT analysis as verification. Based on OS analysis and PFS analysis, we performed several subgroup analyses from 5 aspects, cancer type (NSCLC, RCC, HCC, EC, and MEL), ICI therapy type (PD-1, PD-L1), ATB window (−60 days, +30 days), ATB class (broad-spectrum ATB class) and ECOG score (2–5 vs 0–1).
Our findings revealed that the ATB use was related with worse OS and PFS, which was similar with previous study (6). ATB treatment is commonly performed in clinic for cancer patients, who are more susceptible to getting infected, but the ATBs can alter the composition and diversity of the gut microbiota. Therefore, ATB use can significantly impact the efficacy of ICIs. In subgroup analysis, for various cancer types, we analyzed non-small-cell lung cancer (NSCLC), renal cell carcinoma (RCC), hepatocellular carcinoma (HCC), esophageal cancer (EC), and melanoma (MEL). All of the five cancer types were shown to be at a higher risk of poor OS except HCC, while only NSCLC and RCC were shown to be at a higher risk of poor PFS. Among all the five types, EC was at the highest risk (HR = 2.8) in OS analysis, and RCC was at the highest risk in PFS analysis, even higher than 3 (HR = 3.14). Interestingly, in PFS analysis, no significant association was observed between EC and PFS of cancer patients treated in ICIs (p = 0.06), with only three eligible studies and high heterogeneity, which could be a focus of future research. HCC was shown not to be significantly associated with both OS and PFS, but with only two studies, which needs more studies for further verification. Various cancer types have different impacts on the human body. For the gut environment, a favorable gut microbiota can enhance antigen presentation and T-cell function related to the systemic and anti-tumor immune response, which was demonstrated in a mouse experiment (7). The diversity of gut microbiota increases from infancy to adulthood and decreases in the elderly, with metabolic, defensive, and trophic functions (56). Induction and regulation of the adaptive immune system is one of the essential aspects of the gut microbiota trophic function, and intestinal immunity is the largest and most complex part of the overall immune system of the human body, with at least 80% of all antibodies produced in the intestinal mucosa for adults (57). Thus, ATB use may reduce the efficacy of ICI immunotherapy through altering the diversity and composition of the gut microbiota, which still needs more evidence to prove.
For ICI therapy type, we selected PD-1 inhibitor type, PD-L1 inhibitor type, and the combination of both PD-1 inhibitor and PD-L1 inhibitor. The results revealed that the PD-1 inhibitor and the combination were strongly associated with a higher risk of poorer prognosis, while PD-L1 was shown to be out of meaningful relationship with PFS. Interestingly, we found that the HR value of the combination was quite lower than the HR value of the PD-1 inhibitor alone, which may indicate that the PD-L1 inhibitor matters a lot in this process. Rounis and his team analyzed 66 patients who received PD-1 inhibitors or PD-L1 inhibitors and found that ATB administration did not affect the survival outcome of ICI patients, but prolonged ATB use was related to poor survival (58). This contradiction may be attributed to different varieties, such as the amount of study population, cancer type, and ATB type. In our research, it was indicated that different ATB windows had effects on the survival outcome of ICI patients, when the ATB window was in the period between 60 days before ICI initiation and 30 days after ICI initiation. Especially when ATB window was (−30 days,0 day) of ICI initiation, the risk was the highest in OS analysis (HR = 2.61), with no heterogeneity (I2 = 0). When ATB window was (0 day, +30 days) of ICI initiation, the risk was the highest in the PFS analysis (HR = 2.38). Some studies have already revealed that the short-term decrease in bacterial richness after treatment in ATB (59). Meanwhile, the status of gut microbiota can recover to a baseline within 3 months after ATB discontinuation (60). So, using ATB treatment is essential, which can significantly influence the survival outcome of cancer patients on ICI therapy. Also, we also found the relationship of clinic feature, it was revealed that patients with a lower ECOG score (≤1) were more pretended to undergo ATB treatment. While the other aspects (PD-1 inhibitor type, NSCLC, gender type, cancer stage, and ICI line therapy) were observed to have no significant association.
Until now, the concrete mechanisms of how the use of antibiotics can impact the ICI therapy efficiency for cancer patients are still unknown, but some studies have shown that it may also be associated with the tumor microenvironment (61). An intact commensal microbiota is necessary for cancer therapy, which can mediate therapy effects through modulating the myeloid-derived cell functions in the tumor microenvironment. For example, in one experiment with ATB-treated mice, the tumor-infiltrating myeloid-derived cells responded poorly to the therapy, leading to lower cytokine production and tumor necrosis after CpG-oligonucleotide treatment, and it also showed deficient production of reactive oxygen species (ROS) and cytotoxicity after chemotherapy (62). Another research has indicated that ATB may change the equilibrium of commensal bacteria, conducive for ICB efficacy, which may result in possible resistance to ICIs (63). Meanwhile, the local microbiota was demonstrated to make up an important part of the tumor microenvironment in many types of cancer, which may be affected by ATB use (64). Many researchers have proved that local bacterial dysbiosis can cause a pro-inflammatory immune response and thereby promote cancer growth (65).
Compared with the previous meta-analysis, our research is the most comprehensive, which included the largest number of studies, the largest population, and studied the most comprehensive aspects of subgroup analysis. Yu et al. (66) and Jiang et al. (67) performed a similar meta-analysis, although their subgroup was not as comprehensive as ours. Lurienne et al. (68) and Chen et al. (69) only included NSCLC patients with great limitations. The research by Elkrief (70) missed relative statistical analysis. However, there are still several limitations to our current study. First, the heterogeneity of the included research cannot be ignored. Different responses to drugs, different intervals of administration, and different individual cancer status can result in high heterogeneity. Second, the included studies did not provide enough details. Although we recorded the baseline characteristics of the population and performed the subgroup analysis, some concrete aspects are still unclear, such as infection type and infection site. The subgroup analysis for the ICI type lacked CTLA-4 inhibitor, which was inadequate. Thirdly, most of the studies were retrospective, and only five of the studies contained randomized controlled trials.
Conclusion
In this research, it was revealed that ATB use was strongly associated with worse OS and PFS in cancer patients treated with ICI immunotherapy, especially during the period between 60 days before ICI initiation and 30 days after ICI initiation, which indicated that ATBs should be used cautiously and strictly to avoid a worse survival outcome. The immunotherapy inhibitor type and ATB class can also impact the prognosis. Moreover, it was found that different cancer types are also essentially associated with a survival outcome, including NSCLC, RCC, EC, and MEL. Still, more studies are needed to find the concrete mechanism between ATB use and ICIs and further improve the clinical treatment.
Data availability statement
The original contributions presented in the study are included in the article/Supplementary Material. Further inquiries can be directed to the corresponding authors.
Author contributions
JZ conceived the project and wrote the manuscript. GH, W-CW, D-hH, J-wZ, and HZ participated in data analysis. HT participated in language editing. RL and HZ reviewed the manuscript. All authors contributed to the article and approved the submitted version.
Funding
This work was partially supported by the Guangdong Province Medical Science and Technology Research Fund Project (A2021056), funded by the Special Fund for Scientific Research and Cultivation of Shunde Hospital Affiliated to Jinan University (202101011); and the Guangdong Innovation and Entrepreneurship Training Program for Undergraduate (S202110559091X, S202210559078).
Acknowledgments
We would like to thank Taiwen Li and Shirley Liu from Harvard University for helpful suggestions.
Conflict of interest
The authors declare that the research was conducted in the absence of any commercial or financial relationships that could be construed as a potential conflict of interest.
Publisher’s Note
All claims expressed in this article are solely those of the authors and do not necessarily represent those of their affiliated organizations, or those of the publisher, the editors and the reviewers. Any product that may be evaluated in this article, or claim that may be made by its manufacturer, is not guaranteed or endorsed by the publisher.
Supplementary Material
The Supplementary Material for this article can be found online at: https://www.frontiersin.org/articles/10.3389/fimmu.2022.968729/full#supplementary-material
References
1. Hargadon KM, Johnson CE, Williams CJ. Immune checkpoint blockade therapy for cancer: an overview of FDA-approved immune checkpoint inhibitors. Int Immunopharmacol (2018) 62:29–39. doi: 10.1016/j.intimp.2018.06.001
2. Jenkins RW, Barbie DA, Flaherty KT. Mechanisms of resistance to immune checkpoint inhibitors. Br J Cancer (2018) 118(1):9–16. doi: 10.1038/bjc.2017.434
3. Chen DS, Mellman I. Elements of cancer immunity and the cancer-immune set point. Nature (2017) 541(7637):321–30. doi: 10.1038/nature21349
4. Baba Y, Nomoto D, Okadome K, Ishimoto T, Iwatsuki M, Miyamoto Y, et al. Tumor immune microenvironment and immune checkpoint inhibitors in esophageal squamous cell carcinoma. Cancer Sci (2020) 111(9):3132–41. doi: 10.1111/cas.14541
5. Nakamura Y. Biomarkers for immune checkpoint inhibitor-mediated tumor response and adverse events. Front Med (2019) 6:119. doi: 10.3389/fmed.2019.00119
6. Yu Y, Zheng P, Gao L, Li H, Tao P, Wang D, et al. Effects of antibiotic use on outcomes in cancer patients treated using immune checkpoint inhibitors: a systematic review and meta-analysis. J Immunother (2021) 44(2):76–85. doi: 10.1097/CJI.0000000000000346
7. Routy B, Le Chatelier E, Derosa L, Duong CP, Alou MT, Daillère R, et al. Gut microbiome influences efficacy of PD-1–based immunotherapy against epithelial tumors. Science (2018) 359(6371):91–7. doi: 10.1126/science.aan3706
8. Zitvogel L, Galluzzi L, Viaud S, Vétizou M, Daillère R, Merad M, et al. Cancer and the gut microbiota: an unexpected link. Sci Transl Med (2015) 7(271):271ps1. doi: 10.1126/scitranslmed.3010473
9. Khan U, Ho K, Hwang EK, Peña C, Brouwer J, Hoffman K, et al. Impact of use of antibiotics on response to immune checkpoint inhibitors and tumor microenvironment. Am J Clin Oncol (2021) 44(6):247–53. doi: 10.1097/COC.0000000000000813
10. Pinato DJ, Gramenitskaya D, Altmann DM, Boyton RJ, Mullish BH, Marchesi JR, et al. Antibiotic therapy and outcome from immune-checkpoint inhibitors. J Immunother Cancer (2019) 7(1):1–8. doi: 10.1186/s40425-019-0775-x
11. Routy B, Chatelier EL, Derosa L, Duong CPM, Alou MT, Daillère R, et al. Gut microbiome influences efficacy of PD-1–based immunotherapy against epithelial tumors. Science (2018) 359(6371):91–7. doi: 10.1126/science.aan3706
12. Iglesias-Santamaría A. Impact of antibiotic use and other concomitant medications on the efficacy of immune checkpoint inhibitors in patients with advanced cancer. Clin Transl Oncol (2020) 22(9):1481–90. doi: 10.1007/s12094-019-02282-w
13. Kapoor A, Noronha V, Patil VM, Joshi A, Menon N, Mahajan A, et al. Concomitant use of antibiotics and immune checkpoint inhibitors in patients with solid neoplasms: retrospective data from real-world settings. Ecancermedicalscience (2020) 14:1038. doi: 10.3332/ecancer.2020.1038
14. Lalani AA, Xie W, Braun DA, Kaymakcalan M, Bossé D, Steinharter JA, et al. Effect of antibiotic use on outcomes with systemic therapies in metastatic renal cell carcinoma. Eur Urol Oncol (2020) 3(3):372–81. doi: 10.1016/j.euo.2019.09.001
15. Kulkarni AA, Ebadi M, Zhang S, Meybodi MA, Ali AM, DeFor T, et al. Comparative analysis of antibiotic exposure association with clinical outcomes of chemotherapy versus immunotherapy across three tumour types. ESMO Open (2020) 5(5):e000803. doi: 10.1136/esmoopen-2020-000803
16. Nyein AF, Bari S, Hogue S, Zhao Y, Maller B, Sha S, et al. Effect of prior antibiotic or chemotherapy treatment on immunotherapy response in non-small cell lung cancer. BMC Cancer (2022) 22(1):101. doi: 10.1186/s12885-022-09210-2
17. Castello A, Rossi S, Toschi L, Lopci E. Impact of antibiotic therapy and metabolic parameters in non-small cell lung cancer patients receiving checkpoint inhibitors. J Clin Med (2021) 10(6):247–53. doi: 10.3390/jcm10061251
18. Schett A, Rothschild SI, Curioni-Fontecedro A, Krähenbühl S, Früh M, Schmid S, et al. Predictive impact of antibiotics in patients with advanced non small-cell lung cancer receiving immune checkpoint inhibitors : Antibiotics immune checkpoint inhibitors in advanced NSCLC. Cancer Chemother Pharmacol (2020) 85(1):121–31. doi: 10.1007/s00280-019-03993-1
19. Elkrief A, El Raichani L, Richard C, Messaoudene M, Belkaid W, Malo J, et al. Antibiotics are associated with decreased progression-free survival of advanced melanoma patients treated with immune checkpoint inhibitors. Oncoimmunology (2019) 8(4):e1568812. doi: 10.1080/2162402X.2019.1568812
20. Routy B, Le Chatelier E, Derosa L, Duong CPM, Alou MT, Daillère R, et al. Gut microbiome influences efficacy of PD-1-based immunotherapy against epithelial tumors. Science (2018) 359(6371):91–7. doi: 10.1126/science.aan3706
21. Hogue C, Kuzel TM, Borgia JA, Marwaha G, Bonomi PD, Fidler MJ, et al. P2.04-69 impact of antibiotic usage on survival during checkpoint inhibitor treatment of non-small cell lung cancer (NSCLC). J Thorac Oncol (2019) 146(6):1534–46.e3. doi: 10.1016/j.jtho.2019.08.1574
22. Kaderbhai C, Richard C, Fumet JD, Aarnink A, Foucher P, Coudert B, et al. Antibiotic use does not appear to influence response to nivolumab. Anticancer Res (2017) 37(6):3195–200. doi: 10.1097/CJI.0000000000000346
23. Pinato DJ, Howlett S, Ottaviani D, Urus H, Patel A, Mineo T, et al. Association of prior antibiotic treatment with survival and response to immune checkpoint inhibitor therapy in patients with cancer. JAMA Oncol (2019) 5(12):1774–8. doi: 10.1001/jamaoncol.2019.2785
24. Guven DC, Acar R, Yekeduz E, Bilgetekin I, Baytemur NK, Erol C, et al. The association between antibiotic use and survival in renal cell carcinoma patients treated with immunotherapy: a multi-center study. Curr Probl Cancer (2021) 45(6):100760. doi: 10.1016/j.currproblcancer.2021.100760
25. Barrón F, Arrieta O, Cardona A, Ruiz-Patiño A, Barrón ZZ, Rojas L, et al. Relevance of antibiotic use on clinical activity of immune checkpoint inhibitors in Hispanic patients with advanced NSCLC (CLICAP-ABs) EP1. Technol Cancer Res Treat (2019) 04-46. doi: 10.1177/15330338211033498
26. Huemer F, Lang D, Westphal T, Gampenrieder SP, Hutarew G, Weiss L, et al. Baseline absolute lymphocyte count and ECOG performance score are associated with survival in advanced non-small cell lung cancer undergoing PD-1/PD-L1 blockade. J Clin Med (2019) 8(7):1572–79. doi: 10.3390/jcm8071014
27. Huemer F, Rinnerthaler G, Westphal T, Hackl H, Hutarew G, Gampenrieder SP, et al. Impact of antibiotic treatment on immune-checkpoint blockade efficacy in advanced non-squamous non-small cell lung cancer. Oncotarget (2018) 9(23):16512–20. doi: 10.18632/oncotarget.24751
28. Kim H, Lee JE, Hong SH, Lee MA, Kang JH, Kim IH. The effect of antibiotics on the clinical outcomes of patients with solid cancers undergoing immune checkpoint inhibitor treatment: a retrospective study. BMC Cancer (2019) 19(1):1100. doi: 10.1186/s12885-019-6267-z
29. Mohiuddin JJ, Chu B, Facciabene A, Poirier K, Wang X, Doucette A, et al. Association of antibiotic exposure with survival and toxicity in patients with melanoma receiving immunotherapy. J Natl Cancer Inst (2021) 113(2):162–70. doi: 10.1093/jnci/djaa057
30. Guo JC, Lin CC, Lin CY, Hsieh MS, Kuo HY, Lien MY, et al. Neutrophil-to-lymphocyte ratio and use of antibiotics associated with prognosis in esophageal squamous cell carcinoma patients receiving immune checkpoint inhibitors. Anticancer Res (2019) 39(10):5675–82. doi: 10.21873/anticanres.13765
31. Ahmed J, Kumar A, Parikh K, Anwar A, Knoll BM, Puccio C, et al. Use of broad-spectrum antibiotics impacts outcome in patients treated with immune checkpoint inhibitors. Oncoimmunology (2018) 7(11):e1507670. doi: 10.1080/2162402X.2018.1507670
32. Ouaknine Krief J, Helly de Tauriers P, Dumenil C, Neveux N, Dumoulin J, Giraud V, et al. Role of antibiotic use, plasma citrulline and blood microbiome in advanced non-small cell lung cancer patients treated with nivolumab. J Immunother Cancer (2019) 7(1):176. doi: 10.3390/jcm10061251
33. Kim JH, Ahn B, Hong SM, Jung HY, Kim DH, Choi KD, et al. Real-world efficacy data and predictive clinical parameters for treatment outcomes in advanced esophageal squamous cell carcinoma treated with immune checkpoint inhibitors. Cancer Res Treat (2022) 54(2):505–16. doi: 10.4143/crt.2020.1198
34. Cheung KS, Lam LK, Seto WK, Leung WK. Use of antibiotics during immune checkpoint inhibitor treatment is associated with lower survival in hepatocellular carcinoma. Liver Cancer (2021) 10(6):606–14. doi: 10.1159/000518090
35. Pomej K, Balcar L, Scheiner B, Semmler G, Meischl T, Mandorfer M, et al. Antibiotic therapy is associated with worse outcome in patients with hepatocellular carcinoma treated with sorafenib. J Hepatocell Carcinoma (2021) 8:1485–93. doi: 10.2147/JHC.S317957
36. Hamada K, Yoshimura K, Hirasawa Y, Hosonuma M, Murayama M, Narikawa Y, et al. Antibiotic usage reduced overall survival by over 70% in non-small cell lung cancer patients on anti-PD-1 immunotherapy. Anticancer Res (2021) 41(10):4985–93. doi: 1016/j.jtho.2019.08.1574
37. Ueda K, Yonekura S, Ogasawara N, Matsunaga Y, Hoshino R, Kurose H, et al. The impact of antibiotics on prognosis of metastatic renal cell carcinoma in Japanese patients treated with immune checkpoint inhibitors. Anticancer Res (2019) 39(11):6265–71. doi: 10.21873/anticanres.11680
38. Derosa L, Hellmann MD, Spaziano M, Halpenny D, Fidelle M, Rizvi H, et al. Negative association of antibiotics on clinical activity of immune checkpoint inhibitors in patients with advanced renal cell and non-small-cell lung cancer. Ann Oncol (2018) 29(6):1437–44. doi: 10.1001/jamaoncol.2019.2785
39. Chambers LM, Michener CM, Rose PG, Reizes O, Yao M, Vargas R. Impact of antibiotic treatment on immunotherapy response in women with recurrent gynecologic cancer. Gynecol Oncol (2021) 161(1):211–20. doi: 10.1016/j.currproblcancer.2021.100760
40. Gaucher L, Adda L, Séjourné A, Joachim C, Guillaume C, Poulet C, et al. Associations between dysbiosis-inducing drugs, overall survival and tumor response in patients treated with immune checkpoint inhibitors. Ther Adv Med Oncol (2021) 13:17588359211000591. doi: 10.1016/j.jtho.2019.08.2131
41. Chalabi M, Cardona A, Nagarkar DR, Dhawahir Scala A, Gandara DR, Rittmeyer A, et al. Efficacy of chemotherapy and atezolizumab in patients with non-small-cell lung cancer receiving antibiotics and proton pump inhibitors: pooled post hoc analyses of the OAK and POPLAR trials. Ann Oncol (2020) 31(4):525–31. doi: 10.1016/j.annonc.2020.01.006
42. Greally M, Chou JF, Chatila WK, Margolis M, Capanu M, Hechtman JF, et al. Clinical and molecular predictors of response to immune checkpoint inhibitors in patients with advanced esophagogastric cancer. Clin Cancer Res (2019) 25(20):6160–9. doi: 10.1158/1078-0432.CCR-18-3603
43. Metges J-P, Michaud E, Deniel Lagadec D, Marhuenda F, Chaslerie A, Grude F. Impact of anti-infectious and corticosteroids on immunotherapy: Nivolumab and pembrozilumab follow-up in a French study. Am Soc Clin Oncol (2018) 19(1):1100. doi: 10.1093/annonc/mdy288.087
44. Geum MJ, Kim C, Kang JE, Choi JH, Kim JS, Son ES, et al. Broad-spectrum antibiotic regimen affects survival in patients receiving nivolumab for non-small cell lung cancer. Pharm (Basel) (2021) 14(5):162–70. doi: 10.3390/ph14050445
45. Tinsley N, Zhou C, Tan G, Rack S, Lorigan P, Blackhall F, et al. Cumulative antibiotic use significantly decreases efficacy of checkpoint inhibitors in patients with advanced cancer. Oncologist (2020) 25(1):55–63. doi: 10.1634/theoncologist.2019-0160
46. Ochi N, Ichihara E, Takigawa N, Harada D, Inoue K, Shibayama T, et al. The effects of antibiotics on the efficacy of immune checkpoint inhibitors in patients with non-small-cell lung cancer differ based on PD-L1 expression. Eur J Cancer (2021) 149:73–81. doi: 10.1016/j.ejca.2021.02.040
47. Fessas P, Naeem M, Pinter M, Marron TU, Szafron D, Balcar L, et al. Early antibiotic exposure is not detrimental to therapeutic effect from immunotherapy in hepatocellular carcinoma. Liver Cancer (2021) 10(6):583–92. doi: 10.1159/000519108
48. Cren PY, Bertrand N, Le Deley MC, Génin M, Mortier L, Odou P, et al. Is the survival of patients treated with ipilimumab affected by antibiotics? an analysis of 1585 patients from the French national hospital discharge summary database (PMSI). Oncoimmunology (2020) 9(1):1846914. doi: 10.1080/2162402X.2020.1846914
49. Lu PH, Tsai TC, Chang JW, Deng ST, Cheng CY. Association of prior fluoroquinolone treatment with survival outcomes of immune checkpoint inhibitors in Asia. J Clin Pharm Ther (2021) 46(2):408–14. doi: 10.1111/jcpt.13298
50. Giordan Q, Salleron J, Vallance C, Moriana C, Clement-Duchene C. Impact of antibiotics and proton pump inhibitors on efficacy and tolerance of anti-PD-1 immune checkpoint inhibitors. Front Immunol (2021) 12:716317. doi: 10.3389/fimmu.2021.716317
51. Zhao S, Gao G, Li W, Li X, Zhao C, Jiang T, et al. Antibiotics are associated with attenuated efficacy of anti-PD-1/PD-L1 therapies in Chinese patients with advanced non-small cell lung cancer. Lung Cancer (2019) 130:10–7. doi: 10.1016/j.lungcan.2019.01.017
52. Hwang SR, Higgins A, Castillo Almeida NE, LaPlant B, Maurer MJ, Ansell SM, et al. Effect of antibiotic use on outcomes in patients with Hodgkin lymphoma treated with immune checkpoint inhibitors. Leuk Lymphoma (2021) 62(1):247–51. doi: 10.1080/10428194.2020.1827250
53. Hakozaki T, Richard C, Elkrief A, Hosomi Y, Benlaïfaoui M, Mimpen I, et al. The gut microbiome associates with immune checkpoint inhibition outcomes in patients with advanced non-small cell lung cancer. Cancer Immunol Res (2020) 8(10):1243–50. doi: 10.1158/2326-6066.CIR-20-0196
54. Mielgo Rubio X, Aguado C, Sereno M, Chara L, Cabezón L, Velastegui A, et al. Early antibiotic use affects the efficacy of first line immunotherapy in lung cancer patients but route of administration seems to be decisive. J Thorac Oncol (2019). ELSEVIER SCIENCE INC STE 800, 230 PARK AVE, NEW YORK, NY 10169 USA. doi: 10.1016/j.ygyno.2021.01.015
55. Jing Y, Chen X, Li K, Liu Y, Zhang Z, Chen Y, et al. Association of antibiotic treatment with immune-related adverse events in patients with cancer receiving immunotherapy. J ImmunoTher Cancer (2022) 10(1):e003779. doi: 10.1136/jitc-2021-003779
56. Ramirez J, Guarner F, Bustos Fernandez L, Maruy A, Sdepanian VL, Cohen H. Antibiotics as major disruptors of gut microbiota. Front Cell Infection Microbiol (2020) 10. doi: 10.3389/fcimb.2020.572912
57. Brandtzaeg P. Function of mucosa-associated lymphoid tissue in antibody formation. Immunol Investigations (2010) 39(4-5):303–55. doi: 10.3109/08820131003680369
58. Rounis K, Makrakis D, Papadaki C, Monastirioti A, Vamvakas L, Kalbakis K, et al. Prediction of outcome in patients with non-small cell lung cancer treated with second line PD-1/PDL-1 inhibitors based on clinical parameters: results from a prospective, single institution study. PLoS One (2021) 16(6):e0252537. doi: 10.1371/journal.pone.0252537
59. Raymond F, Déraspe M, Boissinot M, Bergeron MG, Corbeil J. Partial recovery of microbiomes after antibiotic treatment. Gut Microbes (2016) 7(5):428–34. doi: 10.1080/19490976.2016.1216747
60. Huang X-Z, Gao P, Song Y-X, Xu Y, Sun J-X, Chen X-W, et al. Antibiotic use and the efficacy of immune checkpoint inhibitors in cancer patients: a pooled analysis of 2740 cancer patients. Oncoimmunology (2019) 8(12):e1665973. doi: 10.1080/2162402X.2019.1665973
61. Khan U, Ho K, Hwang EK, Peña C, Brouwer J, Hoffman K, et al. Impact of use of antibiotics on response to immune checkpoint inhibitors and tumor microenvironment. Am J Clin Oncol (2021) 44(6):247–53. doi: 10.1097/COC.0000000000000813
62. Iida N, Dzutsev A, Stewart CA, Smith L, Bouladoux N, Weingarten RA, et al. Commensal bacteria control cancer response to therapy by modulating the tumor microenvironment. science (2013) 342(6161):967–70. doi: 10.1126/science.1240527
63. Gong J, Chehrazi-Raffle A, Placencio-Hickok V, Guan M, Hendifar A, Salgia R. The gut microbiome and response to immune checkpoint inhibitors: preclinical and clinical strategies. Clin Trans Med (2019) 8(1):1–14. doi: 10.1186/s40169-019-0225-x
64. Wong-Rolle A, Wei HK, Zhao C, Jin C. Unexpected guests in the tumor microenvironment: microbiome in cancer. Protein Cell (2021) 12(5):426–35. doi: 10.1007/s13238-020-00813-8
65. Abreu MT, Peek RM Jr. Gastrointestinal malignancy and the microbiome. Gastroenterology (2014) 146(6):1534–46.e3. doi: 10.1053/j.gastro.2014.01.001
66. Yu Y, Zheng P, Gao L, Li H, Tao P, Wang D, et al. Effects of antibiotic use on outcomes in cancer patients treated using immune checkpoint inhibitors: A systematic review and meta-analysis. J Immunother (2021) 44(2):76–85. doi: 10.1097/CJI.0000000000000346
67. Jiang S, Geng S, Chen Q, Zhang C, Cheng M, Yu Y, et al. Effects of concomitant antibiotics use on immune checkpoint inhibitor efficacy in cancer patients. Front Oncol (2022) 12:823705. doi: 10.3389/fonc.2022.823705
68. Lurienne L, Cervesi J, Duhalde L, de Gunzburg J, Andremont A, Zalcman G, et al. NSCLC immunotherapy efficacy and antibiotic use: A systematic review and meta-analysis. J Thorac Oncol (2020) 15(7):1147–59. doi: 10.1016/j.jtho.2020.03.002
69. Chen H, Han KD, He ZJ, Huang YS. How to choose a survival period? the impact of antibiotic use on OS or PFS in NSCLC patients treated with immune checkpoint inhibitors: A systematic review and meta-analysis. Technol Cancer Res Treat (2021) 20:15330338211033498. doi: 10.1177/15330338211033498
Keywords: antibiotic, immune checkpoint inhibitor, PD-1, PD-L1, survival outcomes
Citation: Zhou J, Huang G, Wong W-C, Hu D-h, Zhu J-w, Li R and Zhou H (2022) The impact of antibiotic use on clinical features and survival outcomes of cancer patients treated with immune checkpoint inhibitors. Front. Immunol. 13:968729. doi: 10.3389/fimmu.2022.968729
Received: 14 June 2022; Accepted: 27 June 2022;
Published: 28 July 2022.
Edited by:
Fu Wang, Xi’an Jiaotong University, ChinaReviewed by:
Bin Liu, University of Arizona, United StatesFan Pan, German Cancer Research Center (DKFZ), Germany
Copyright © 2022 Zhou, Huang, Wong, Hu, Zhu, Li and Zhou. This is an open-access article distributed under the terms of the Creative Commons Attribution License (CC BY). The use, distribution or reproduction in other forums is permitted, provided the original author(s) and the copyright owner(s) are credited and that the original publication in this journal is cited, in accordance with accepted academic practice. No use, distribution or reproduction is permitted which does not comply with these terms.
*Correspondence: Ruiman Li, liruimanssr@163.com; Hong Zhou, zhouhongjnu@foxmail.com
†These authors have contributed equally to this work