- 1The First Clinical Medical College, Guangzhou University of Chinese Medicine, Guangzhou, China
- 2Guangdong Provincial Hospital of Chinese Medicine, Guangzhou, China
- 3The First Affiliated Hospital of Guangzhou University of Chinese Medicine, Guangzhou, China
Background: The COVID-19 pandemic, caused by severe acute respiratory syndrome coronavirus 2 (SARS-CoV-2), is a global crisis. Although many people recover from COVID-19 infection, they are likely to develop persistent symptoms similar to those of myalgic encephalomyelitis/chronic fatigue syndrome (ME/CFS) after discharge. Those constellations of symptoms persist for months after infection, called Long COVID, which may lead to considerable financial burden and healthcare challenges. However, the mechanisms underlying Long COVID and ME/CFS remain unclear.
Methods: We collected the genes associated with Long COVID and ME/CFS in databases by restricted screening conditions and clinical sample datasets with limited filters. The common genes for Long COVID and ME/CFS were finally obtained by taking the intersection. We performed several advanced bioinformatics analyses based on common genes, including gene ontology and pathway enrichment analyses, protein–protein interaction (PPI) analysis, transcription factor (TF)–gene interaction network analysis, transcription factor–miRNA co-regulatory network analysis, and candidate drug analysis prediction.
Results: We found nine common genes between Long COVID and ME/CFS and gained a piece of detailed information on their biological functions and signaling pathways through enrichment analysis. Five hub proteins (IL-6, IL-1B, CD8A, TP53, and CXCL8) were collected by the PPI network. The TF–gene and TF–miRNA coregulatory networks were demonstrated by NetworkAnalyst. In the end, 10 potential chemical compounds were predicted.
Conclusion: This study revealed common gene interaction networks of Long COVID and ME/CFS and predicted potential therapeutic drugs for clinical practice. Our findings help to identify the potential biological mechanism between Long COVID and ME/CFS. However, more laboratory and multicenter evidence is required to explore greater mechanistic insight before clinical application in the future.
Introduction
COVID-19 is a highly contagious viral pneumonia, which has hitherto caused an exceeding 6 million worldwide death toll among over 500 million confirmed cases (1). In addition to fever, dry cough, and shortness of breath, fatigue (38%) and myalgia (15%–44%) are also commonplace in COVID-19 (2). With the prevalence and transmission of the Omicron BA.2 variant virulent strain, influenza-like and cold-like symptoms such as cough, fever, and fatigue/myalgia become more prevalent (3). Even though many patients with COVID-19 have received treatment, approximately one in three patients still develop persisting symptoms within 12 weeks after onset (4). Regardless of the severity of severe acute respiratory syndrome coronavirus 2 (SARS-CoV-2) infection, Long COVID, typically manifested as fatigue or muscle weakness similar to the symptoms of myalgic encephalomyelitis/chronic fatigue syndrome (ME/CFS) (5, 6), can be experienced by all age groups (7, 8). Fatigue being the most common symptom of Long COVID could be a potential threat to public health and the economy due to restriction of daily working abilities and social participation (7, 9).
Similar to Long COVID with fatigue, ME/CFS, an intractable heterogeneous disease, has posed a non-negligible influence on millions of people globally, although a recent study shows the worrisome fact that up to 91% of patients remain undiagnosed or misdiagnosed owing to other clinical conditions (10–12). A prospective observational study presents that almost half of the patients with Long COVID at 6 months after SARS-CoV-2 infection fulfilled the diagnostic criteria of ME/CFS (13). If left ignored, cases of ME/CFS could double in the United States on account of the unprecedented COVID-19 pandemic (14).
People with acute COVID-19 and with ME/CFS have similar pathological mechanisms such as redox imbalance, systemic inflammation and neuroinflammation, impaired energy metabolism, and a hypometabolic state (15). Research shows that during the acute phase of COVID-19, the activation of immune-inflammatory pathways due to lung lesions and hypoxemia may lead to chronic fatigue syndrome-like symptoms such as fatigue and myalgia (16), while the pathophysiological mechanisms of Long COVID and ME/CFS remain ambiguous/elusive by far. Previous studies have indicated that autonomic dysfunction after a viral illness, tissue scarring, organ damage, immune system dysregulation, and autoantibodies could potentially be the underlying mechanisms that explain the development of Long COVID (17–20). ME/CFS gradually manifests as abnormalities of the central and autonomic nervous system including general downregulation of the hypothalamic–pituitary–adrenal axis, cognitive impairment manifested by slowed information processing speed and impaired memory and attention, abnormal signals in magnetic resonance imaging, a widespread state of neuroinflammation, and changes in systemic and cerebral hemodynamics that correlate with symptoms (21). Aside from the mechanisms mentioned above, infection, sleep disturbance, energy metabolism impairment, and impaired immune function are also involved in the progression of ME/CFS (21–25). Of particular concern is that compared with uninfected individuals, patients with COVID-19 exhibit increasing autoantibody reactivities (26), which may help to explain that patients suffering from Long COVID could manifest characteristics typically found in ME/CFS.
Bioinformatics analysis refers to the integration and analysis of biological data through a variety of bioinformatics tools, which is one of the important means of life science research. To exemplify, Hasan Mahmud et al. identified 10 hub genes related to SARS-CoV-2, idiopathic pulmonary fibrosis, and chronic obstructive pulmonary disease via bioinformatics and systems biology methods, which provides new perspectives for further in-depth research on comorbidity mechanisms of COVID-19, idiopathic pulmonary fibrosis (IPF), and chronic obstructive pulmonary disease (COPD); meanwhile, they predicted 10 possible drugs for clinical reference accordingly (27). A more accurate prediction could be performed by utilizing bioinformatics analysis for the purpose of understanding (physio)pathological molecular mechanisms of diseases and expediting the implementation of precision medicine (28).
Aiming to discover the mechanisms of ME/CFS associated with Long COVID, common genes of aforementioned diseases were located from acknowledged databases, the basis on which the common molecular pathogenesis and potential therapeutic drugs were predicted. The workflow of our research is presented in Figure 1.
Materials and methods
Collection of Long COVID and Myalgic Encephalomyelitis/Chronic Fatigue Syndrome-related genes
By searching CTD (http://ctdbase.org/) (29), GeneCards (https://www.genecards.org/) (30), and DisGeNET (https://www.disgenet.org/) (31) databases, we collected the genes related to COVID-19 and ME/CFS. In addition, we selected a relevant dataset, acquired from the Gene Expression Omnibus (GEO) database (https://www.ncbi.nlm.nih.gov/geo/ ) (32), for supplementary validation of disease genes. Dataset (GSE169687) (33) provides the total RNA sequenced from recovered COVID-19 patients’ blood several weeks post-infection. By performing differential gene analysis separately, we obtained the expression of differential genes in convalescent individuals at 12, 16, and 24 weeks post-infection compared with healthy controls and then merged and deduplicated the differentially expressed genes (DEGs). In the expression profiling datasets related to ME/CFS (including GSE130353, GSE128078, GSE14577, and GSE16059) (34–37), principal component analysis (PCA) shows that the heterogeneity between the disease group and the healthy control group was small after adjusting for batch effect. We found that the differential genetic results between the disease and normal control groups were disappointing, which may be attributed to the limitations of previous study designs on the one hand and the nature of the disease itself on the other.
According to the scoring rules of each database, we gathered the top 500 genes of each database. We analyzed the dataset by R programming language, DESeq2 (38), and limma (39) package. The adjusted p-value (false discovery rate (FDR)) <0.05 and fold change > 1.5-fold are used as cutoff criteria for DEGs. Subsequently, we obtained the related genes of Long COVID by taking the intersection of the two parts of the genes. We then overlapped the related genes of Long COVID and ME/CFS to obtain common genes for further analysis. We accessed to these websites on 27 April 2022.
Gene ontology and pathway enrichment analyses
To understand a functional characteristic of the common genes in Long COVID and ME/CFS, a series of enrichment analyses were conducted utilizing Enrichr, a comprehensive gene set enrichment web tool (https://maayanlab.cloud/Enrichr/) (40), to gain detailed information on characterizing biological mechanisms and signaling pathways. Gene ontology (GO) (41, 42) includes three terms: biological process, molecular function, and cellular component. The Kyoto Encyclopedia of Genes and Genomes (KEGG) pathway (43) was used to recognize a metabolic pathway. For a more comprehensive understanding of the relevant signaling pathways, WikiPathways (44), Reactome (45), and BioCarta (46) databases were also used alongside the KEGG pathway.
Protein–protein interaction analysis and network construction
In cellular as well as systems biology, understanding the interaction of an intracellular protein with another protein through the assessment and analysis of protein–protein interaction (PPI) networks can contribute to improved comprehension of protein function. Common genes were used to construct a PPI network by STRING (47) with a confidence score (0.4) as the minimum required interaction score, and all other parameters were set to their default. The PPI results were analyzed and visualized via Cytoscape (V3.8.2) (48–50). Through the cytoHubba (51), a plug-in of Cytoscape, the five hub proteins with the highest degree values were obtained by using the degree topological algorithm.
Transcription factor-gene interactions
Transcription factors (TFs) are proteins that can bind to specific DNA sequences and regulate the expression of genes. NetworkAnalyst 3.0 (https://www.networkanalyst.ca/) (52) was used to analyze the interaction of the common genes and transcription factors and to assess the impact of the TF on the expression and functional pathways of the common genes. Transcription factor and gene target data were derived from the ENCODE ChIP-seq data (53–55). Only peak intensity signal <500 and the predicted regulatory potential score <1 are used (using the BETA Minus algorithm). TF–gene regulatory network was constructed and visualized by Cytoscape.
Transcription factor–miRNA coregulatory network
MicroRNAs (miRNAs), which mediate target mRNA degradation or translation inhibition, are one class of endogenous short non-coding RNAs (56). We must understand the deregulation of gene expression in different physiological and disease conditions by comprehending transcriptional networks of regulation between TFs and miRNAs (56–60). The common genes were submitted to NetworkAnalyst 3.0 to generate a TF–miRNA coregulatory network. The literature-curated regulatory interaction information was collected from RegNetwork (http://www.regnetworkweb.org/) (61). Relevant results were also visualized by Cytoscape.
Prediction of candidate drugs
Evaluating protein–drug interactions is important for understanding the structural features recommended for receptor sensitivity. The common genes were uploaded to the Drug Signatures Database (DSigDB, http://dsigdb.tanlab.org/DSigDBv1.0/) (62), which consists of 22,527 gene sets for further candidate drug prediction. Access to DSigDB is acquired through the Enrichr platform. The candidate drugs were sorted by adjusted p-value from small to large, and the adjusted p-value <0.01 was considered statistically significant.
Result
Collection of Long COVID and Myalgic Encephalomyelitis/Chronic Fatigue Syndrome-related genes
By searching CTD, GeneCards, and DisGeNET databases, we collected the genes related to COVID-19 and ME/CFS. To improve the credibility of the data, we gathered the top 500 genes of each database according to the scoring rules of each database. If the raw data are less than 500, we included all retrieved data. On this basis, we obtained 500, 500, and 118 ME/CFS-related genes from CTD, GeneCards, and DisGeNET, respectively. After that, we gained 1,023 ME/CFS-related genes by merging and deduplicating the results collected from three databases.
Analogously, we gained 1,233 COVID-19-related genes by CTD, GeneCards, and DisGeNET. At the same time, we collected 1,186 Long COVID-related genes using the cutoff criteria (FDR < 0.05 and fold change > 1.5-fold). By taking the intersection of the results of the database and the dataset, we included 49 Long COVID-related genes. Details on genes collected from above can be found in the Supplementary Material.
Finally, we obtained nine common genes of ME/CFS and Long COVID by cross-processing the related genes of the two diseases. The screening process and results are shown in Table 1 and Figure 2.
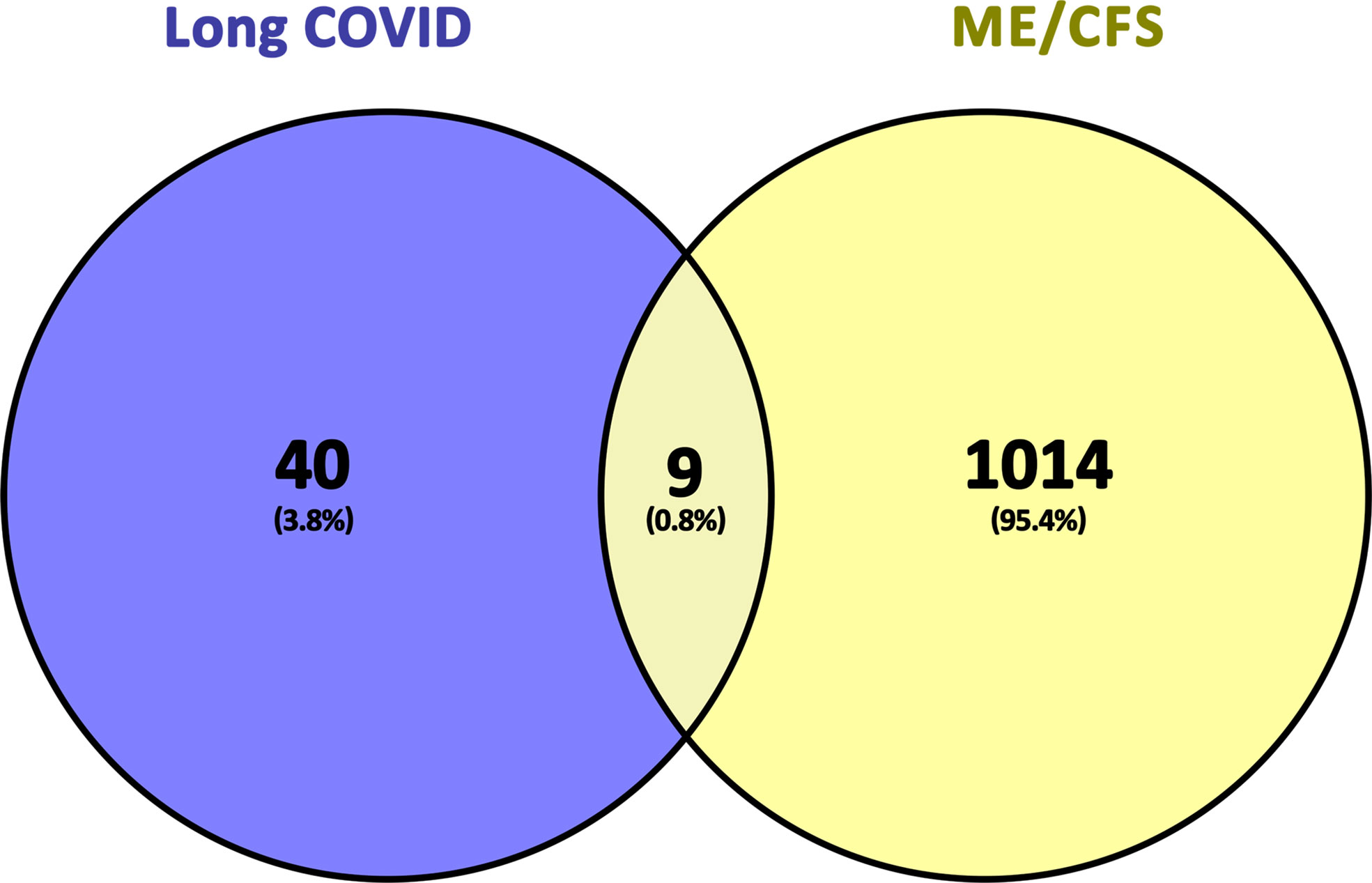
Figure 2 Common genes representation through a Venn diagram. 9 genes were found as common genes from 49 related genes of long COVID and 1023 related genes of ME/CFS.
Gene ontology and pathway enrichment analyses
Gene ontology, pathway enrichment analysis, and the visualization of results were executed by Enrichr. A combined score was performed by the Enrichr web tool, which is determined by the log of the p-value and Z-score. The gene ontology analysis of common genes (CXCL8, B2M, SOD1, BCL2, EGF, SERPINE1, S100A8, S100A9, and HMGB1), using the GO database as an annotation source, was acquired within three categories (biological process, cellular component, and molecular function). The most impacted pathways of the common genes among ME/CFS and Long COVID were gathered from four global databases, including KEGG, WikiPathways, Reactome, and BioCarta. The top 10 GO terms and pathways are summarized in Tables 2, 3, respectively, and presented in the form of bar graphs in Figures 3, 4.
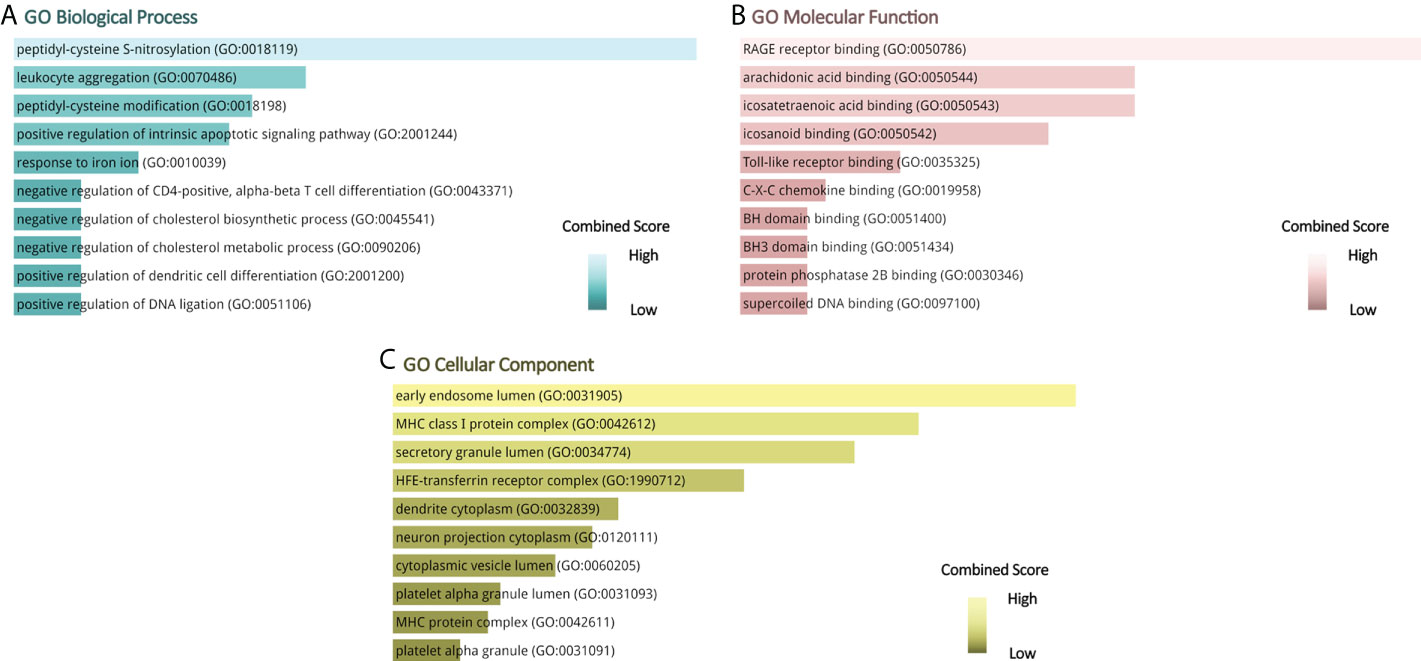
Figure 3 GO terms of common genes between long COVID and ME/CFS. (A) Biological Processes, (B) Molecular Function, (C) Cellular Component.
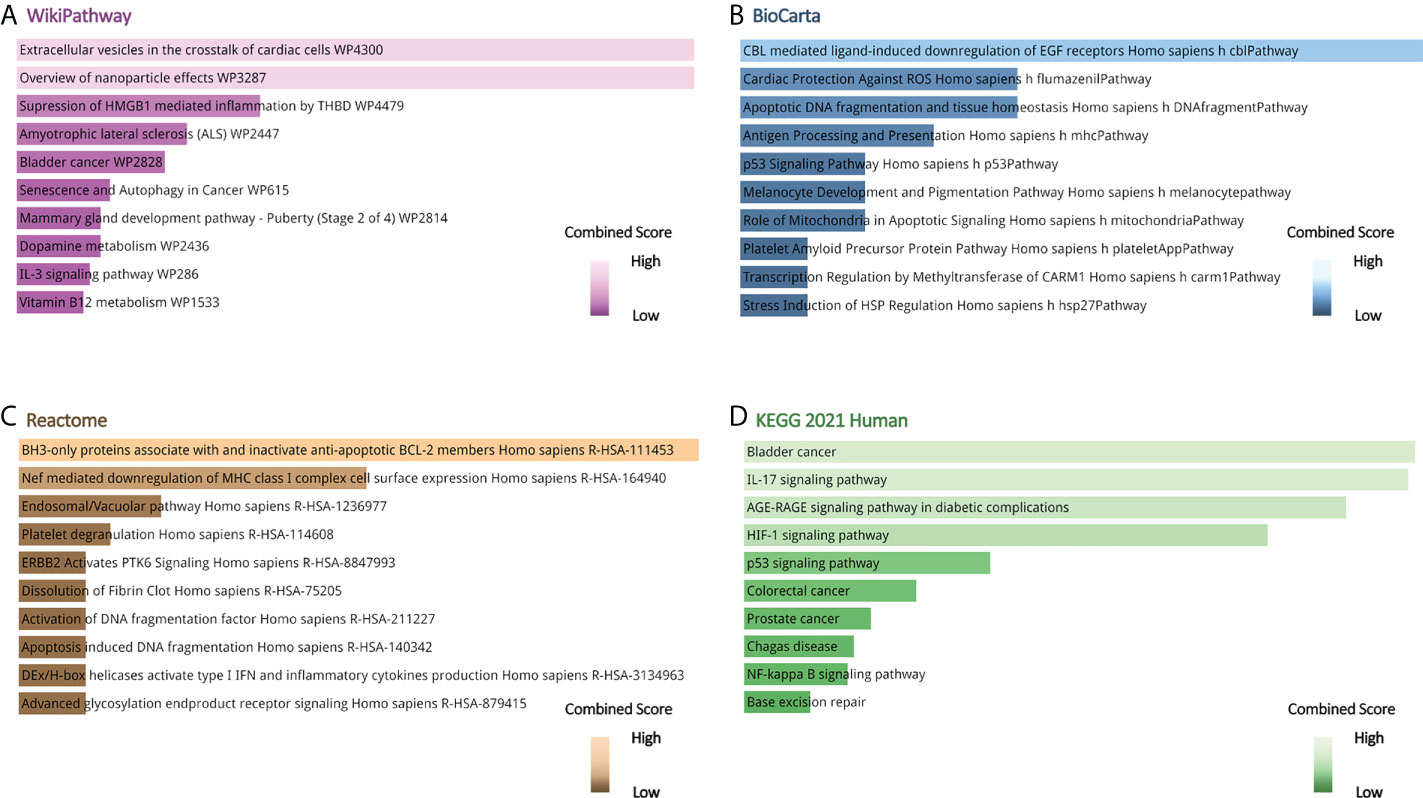
Figure 4 Pathway enrichment analysis of common genes between long COVID and ME/CFS. (A) Wikipathway, (B) BioCarta Pathway, (C) Reactome Pathway, (D) KEGG Human Pathway.
Protein–protein interaction analysis and network construction
The nine common genes were provided as input in STRING, and the file generated from the analysis is reintroduced into Cytoscape for visual representation. As shown in Figure 5, the PPI network of common genes consists of 50 nodes and 375 edges. According to the degree in the PPI network, the top 5 hub proteins as IL-6, IL-1B, CD8A, TP53, and CXCL8 were listed through the cytoHubba plugin. The interaction of hub proteins with other proteins in the PPI network is demonstrated in Figure 6.
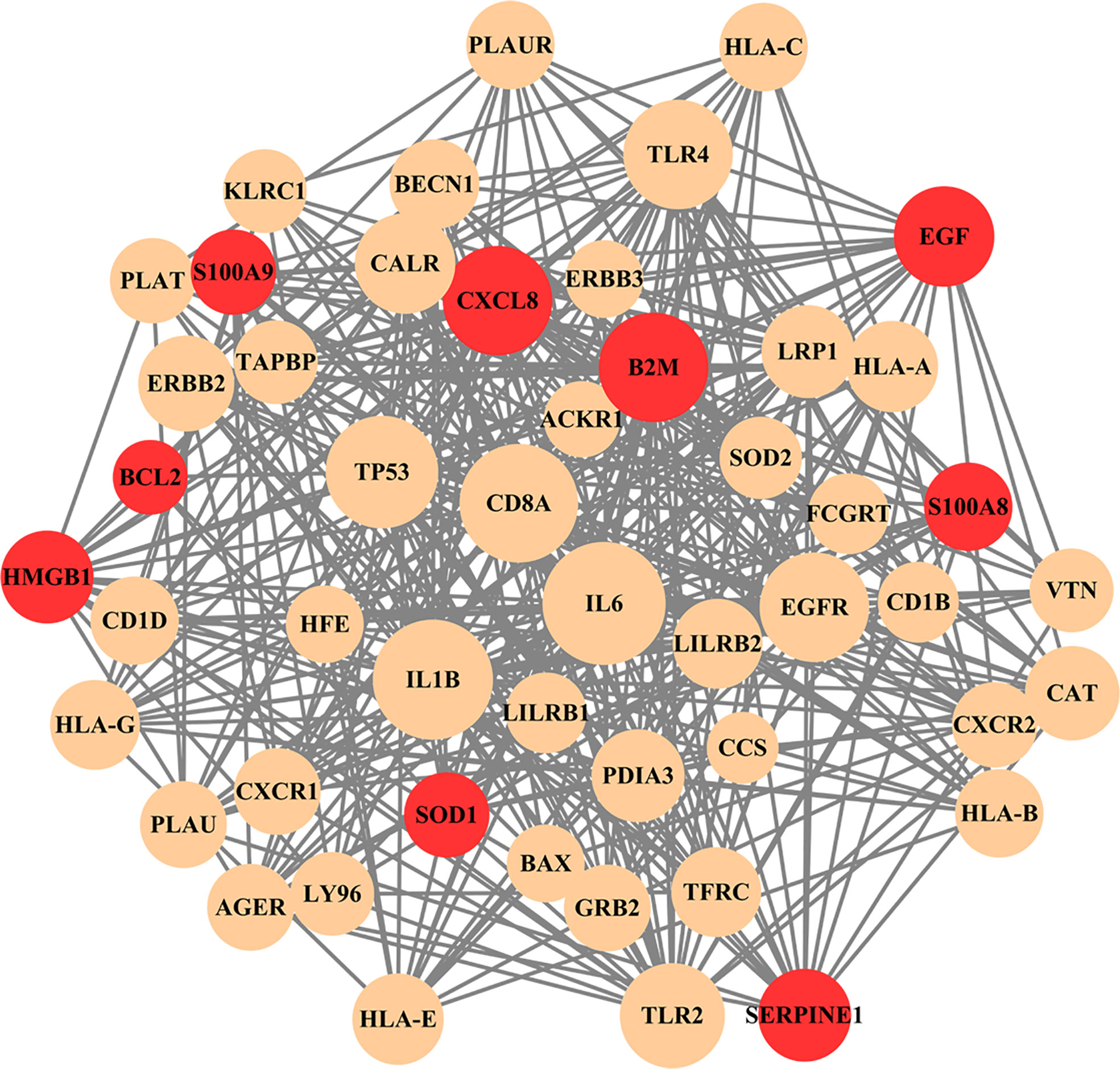
Figure 5 PPI network of common genes among long COVID and ME/CFS. In the figure, nodes in red color represent common genes and edges represent the interactions between nodes. The analyzed network holds 50 nodes and 375 edges.
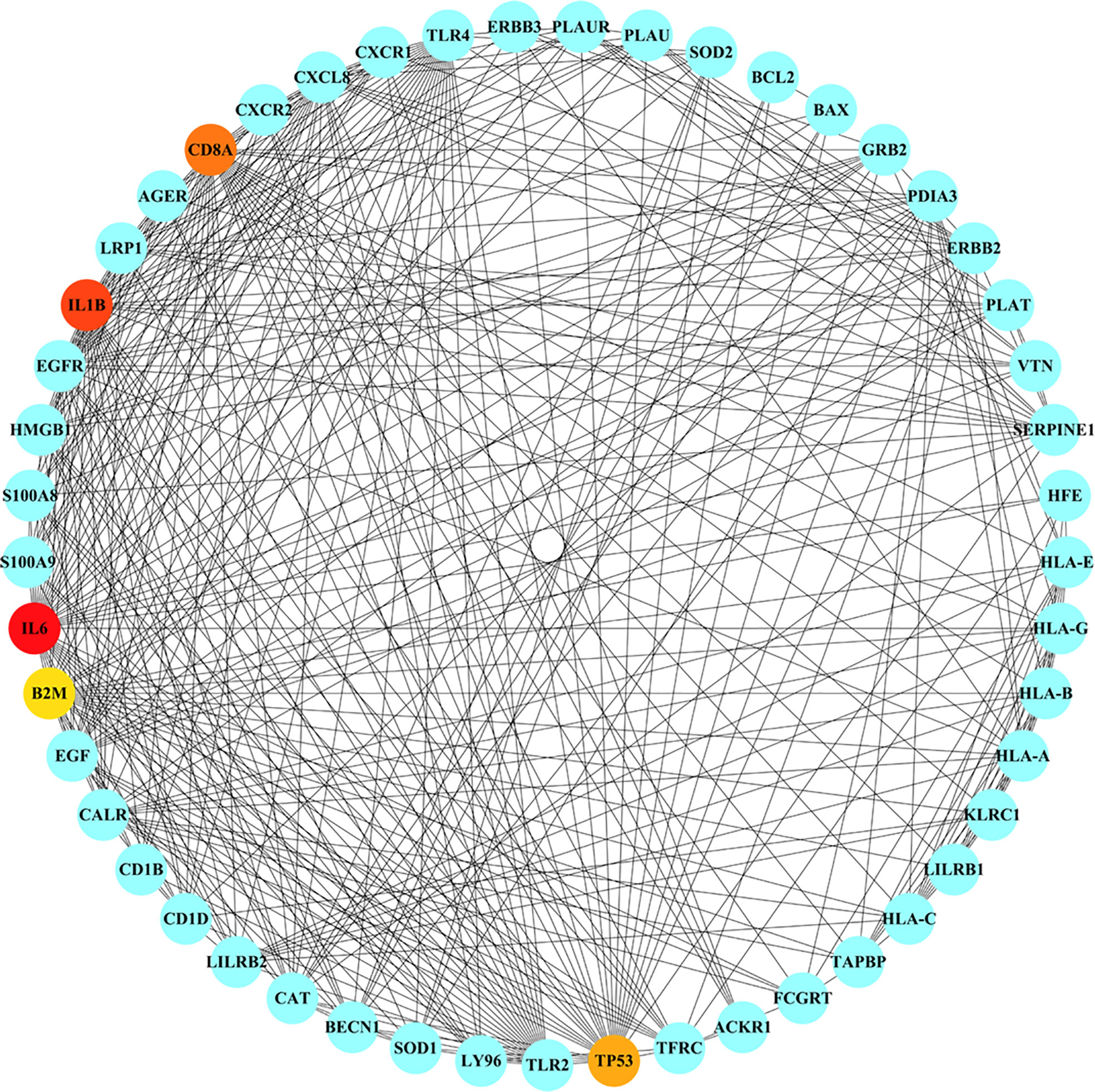
Figure 6 Detection of hub genes from the PPIs network of common genes. The highlighted 5 hub genes, based on their degree, are IL6, IL1B, CD8A, TP53, CXCL8. The network has 49 nodes and 373 edges.
Transcription factor–gene interactions
TF–gene interactions showed the interaction of nine common genes and TF genes by using NetworkAnalyst. The TF–gene interaction network consists of 136 nodes and 156 edges (Figure 7). Among them, SERPINE1 is regulated by 57 TF genes, and B2M is regulated by 54 genes (Table 4). The degree values of transcription factors CEBPG, KLF8, and WRNIP1 in the TF–gene interaction network were all 3.
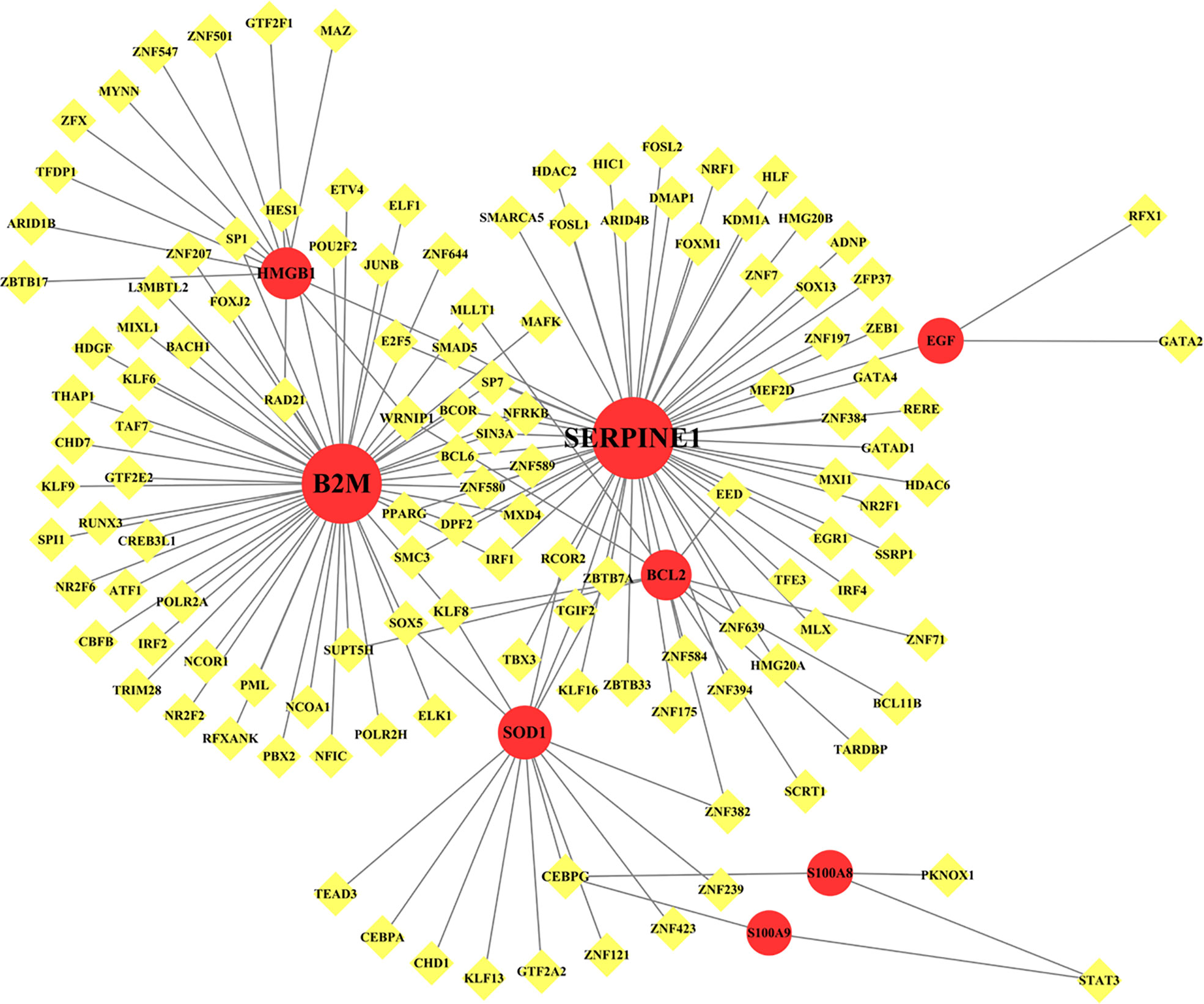
Figure 7 Network for TF-gene interaction with common differentially expressed genes. The highlighted red color node represents the common genes and other nodes represent TF-genes. The network consists of 136 nodes and 156 edges.
Transcription factor–miRNA coregulatory network
TF–miRNA coregulatory network is also generated using NetworkAnalyst 3.0. The TF–miRNA coregulatory network comprises 240 nodes and 327 edges (Figure 8). A total of 130 TF genes and 102 miRNAs have interacted with the nine common genes (Table 5).
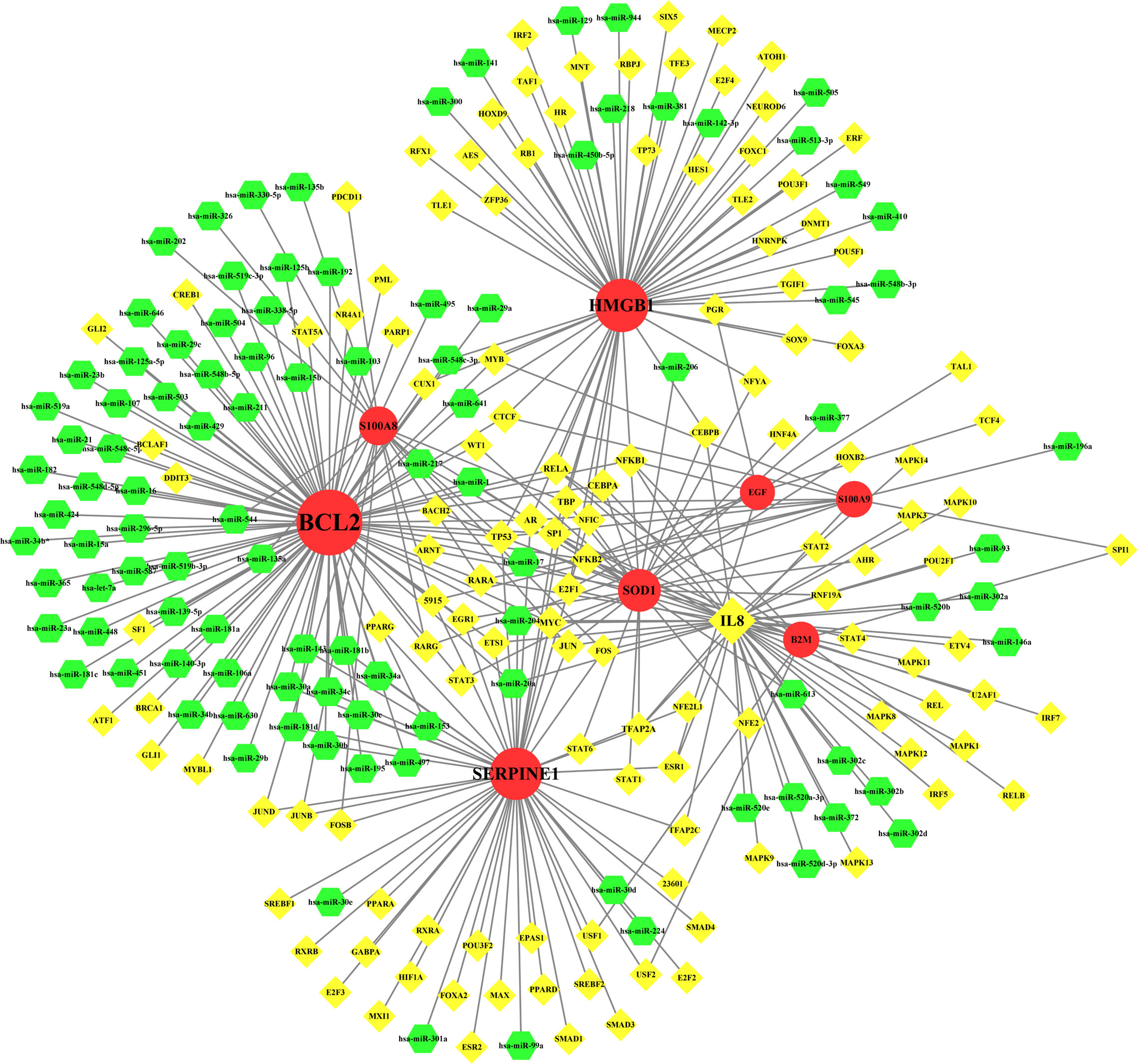
Figure 8 The network presents the TF-miRNA coregulatory network. The network consists of 240 nodes and 327 edges including 130 TF-genes, 102 miRNA and 9 common genes. The nodes in red color are the common genes, yellow nodes represent miRNA and green nodes indicate TF-genes.
Prediction of candidate drugs
We made predictions about possible effective intervention drugs with the use of the Enrichr platform, which is based on the DSigDB database. The top 10 potential chemical compounds are extracted based on their adjusted p-value (Table 6). The results showed that phorbol 12-myristate 13-acetate (CTD 00006852) and dexamethasone (CTD 00005779) are the two drug molecules that interacted with most genes.
Discussion
Several studies have shown that a large proportion of patients with SARS-CoV-2 infection will experience a series of symptoms such as fatigue, dyspnea, and sleep difficulties after acute onset, which is called Long COVID (5, 63–66). Cardinal clinical features manifested by individuals with Long COVID present analogously to ME/CFS, which is also known as a post-infectious syndrome caused by many types of infectious agents (67). Given the substantial public health burden that Long COVID and ME/CFS could impose, increasing investment and proactive advances in potential mechanisms are in urgent need considering that the optimal therapeutic regimen’s current status is undetermined (68–73). In this study, we identified genes associated with Long COVID and ME/CFS. Afterward, we performed a series of bioinformatics analyses grounding on nine common genes that we found between Long COVID and ME/CFS.
The nine identified common genes were applied for detecting GO terms. Regarding the GO biological process terms, peptidyl-cysteine S-nitrosylation, leukocyte aggregation, peptidyl-cysteine modification, positive regulation of intrinsic apoptotic signaling pathway, and response to iron ion are the most significant. Among them, leukocyte aggregation is intimately related to microvascular plugging (74). Leukocyte aggregation to platelets has been demonstrated by post-mortem studies of deceased patients with COVID-19 (75). However, whether the widespread vascular dysfunction is a potential cause of neurological deterioration in COVID-19 patients remains uncertain. As for the molecular function, the top GO terms are as follows: RAGE receptor binding, arachidonic acid binding, and icosatetraenoic acid binding. RAGE, presenting on the surface of various cell types in atherosclerotic lesions, is a multiligand transmembrane receptor in the immunoglobulin superfamily (76–78). The binding of RAGE to advanced glycation end products (AGEs) plays an important role in the development of late atherosclerosis complications in diabetes (79–81). The dense linkage and underlying mechanisms among COVID-19, atherosclerosis, and diabetes have been shown in the relevant literature (82–84). In terms of cellular components, the early endosome lumen and MHC class I protein complex rank the top 2. β2-Microglobulin is the essential conformation of the MHC class I protein complex, the level of which acts as an early indicator for disease severity and outcome prediction of COVID-19 (85).
The KEGG pathway enrichment analysis was performed to identify the common pathway of Long COVID and ME/CFS. The top 10 KEGG Human pathways include bladder cancer, IL-17 signaling pathway, AGE-RAGE signaling pathway in diabetic complications, HIF-1 signaling pathway, p53 signaling pathway, colorectal cancer, prostate cancer, Chagas disease, NF-kappa B signaling pathway, and base excision repair. IL-17, a member of the multifunctional cytokine family, serves as both a severity biomarker of COVID-19 and a promising therapeutic target to mitigate the lung damage of COVID-19 (86–88). Intriguingly, as a key modulator of upstream inflammatory pathways, IL-17 contributes to the production of IL-6, the elevated peripheral levels of which may initiate ME/CFS through neuroinflammation (6). Meanwhile, results from WikiPathways show that most interacted gene pathways are extracellular vesicles in the crosstalk of cardiac cells and the overview of nanoparticle effects. Results from BioCarta and Reactome separately produce CBL-mediated ligand-induced downregulation of EGF receptors Homo sapiens h-cbl Pathway and BH3-only proteins associate with and inactivate anti-apoptotic BCL-2 members Homo sapiens R-HSA-111453.
According to the PPI network, IL-6, IL-1B, CD8A, TP53, and CXCL8 were declared as hub proteins on account of their high degrees. On the one hand, IL-6, as a multifunctional molecule, plays a crucial role in COVID-19-related hyperinflammation (89), which may lead to ME/CFS through neuroinflammation (90, 91). On the other hand, as an energy distributor in muscle tissue (92), IL-6 may get involved in the occurrence of ME/CFS through related pathways of energy metabolism (93). The results of the study showed that the levels of inflammatory cytokines such as IL-6, IL-10, and TNF-α increased in ME/CFS patients, suggesting that ME/CFS patients were in a low-grade systemic inflammatory state for a long time (94). In infection-triggered ME/CFS, IL-1b release is inversely correlated with sCD26 expression (95), while the expression level of sCD26 has been linked to health-related quality of life (96). IL-1b was associated with the altered regulation of several genes involved in the myogenic processes, elucidating the mechanism of muscle loss in COVID-19 (97). A substantial reduction of CD8A was found in spleen autopsy specimens from patients who died of COVID-19 (98). In addition to being known as a tumor suppressor, the P53 protein, encoded by the TP53 gene, is a crucial component of the body’s antiviral response (99). The higher expression level of CXCL8, a chemokine also known as IL-8, was correlated with greater clinical severity of COVID-19 (100). Interestingly, the levels of CXCL8 showed a decrease of 42% in CFS patients, compared with control subjects (101).
Transcription factors leverage a prominent role in regulating gene expression. From the TF–gene interaction network, SERPINE1 and BCL2 showed a high interaction rate with other TF genes. Among them, SERPINE1 is regulated by 57 TF genes, and BCL2 is regulated by 54 TF genes. SERPINE1, a member of the Serpin family of proteins, prevents the formation of plasmin and inhibits fibrinolysis and blood clot dissolution (102), contributing to coagulopathy associated with COVID-19. Evidence suggests that all COVID-19 patients regardless of disease severity have elevated levels of SERPINE1 (103). In addition, SERPINE1 impedes the regeneration of skeletal muscle strength (104). Among the regulators, CEBPG, KLF8, and WRNIP1 were the regulators with the highest degree in the TF–gene interaction network, all of which were involved in the expression of three common genes.
TFs and miRNAs not only can co-regulate the expression of target genes but also can mutually regulate each other, standing out as a pivot in various biological processes and different diseases (105). By complying TF–miRNA coregulatory network, our analysis revealed the relationship between shared genes, TFs, and miRNAs. Among the identified transcription factors, IL-8 showed the highest degree at 43. As a member of the cytokine storm caused by COVID-19 (106), IL-8 is closely related to the severity and prognosis of the disease (107). IL-8 is expressed at high levels for ME/CFS in the recently afflicted, and the adjustment of IL-1α, IL-6, and IL-8 for illness duration may serve as powerful biomarkers for screening ME/CFS (108). Among the identified MicroRNAs, hsa-miR-204 showed the highest degree at 3. As a regulator of gene expression, miR-204 may engage in mediating the expression of neurotransmitter and ion channel-related gene sets by regulating non-coding RNAs (ncRNAs) (109).
By screening the DSigDB database, nine common genes were applied to predict candidate drugs, and the top 10 significant drugs were highlighted. Phorbol 12-myristate 13-acetate (TPA), used for the treatment of various tumors, can induce the differentiation or apoptosis of various cell lines at low concentrations (110). Dexamethasone, a synthetic adrenal corticosteroid that exerts anti-inflammatory and immunosuppressive effects through the glucocorticoid receptor (GR), was demonstrated to relieve inflammation in COVID-19 by pro-resolving lipid mediators (111). Decreased hypothalamic–pituitary–adrenal (HPA) axis function in patients with chronic fatigue syndrome (CFS) suggests that CFS is associated with hypocortisolism (112). Lactacystin, a component of the ubiquitin–proteasome complex degrading unnecessary cellular proteins, shows the influence on mitochondrial metabolism via modulation of reactive oxygen species (ROS) and glutathione (GSH) (113), which also get involved in sleep homeostasis and CFS development (114).
This study included several limitations, which should be acknowledged and taken into consideration. On the one hand, the studies of Long COVID and ME/CFS remained inadequate so far as compared with abundant data in the acute phase of COVID-19, causing the paucity of available datasets for the aforementioned diseases. On the other hand, the differential expression gene about ME/CFS in multiple datasets, including both RNA sequencing data and microarray data, is absent, which may be due to the shortcomings of previous study designs as well as the attribute of the disease itself. All of the abovementioned causes eventually limited the use of the additional datasets in this study. Furthermore, since our study is based on pure bioinformatics analysis without clinical substantiation, the result including the biological functions and the enrichment analysis of hub genes as well as in vivo safety and efficacy of candidate drugs needs to be validated by further experimental exploration and clinical trials.
Conclusion
Our study found the common genes between Long COVID and ME/CFS. Long COVID and ME/CFS show relative similarities in infection, neuroinflammation, energetic metabolic dysfunction, and impaired immune function by a series of bioinformatics analyses. The long-term health consequences of COVID-19 are not to be neglected, and dexamethasone may treat patients with Long COVID and ME/CFS by modulating the HPA axis, although the predicted results still need to be rigorously validated by experiments.
Data availability statement
Publicly available datasets were analyzed in this study. This data can be found here: https://www.ncbi.nlm.nih.gov/geo/query/acc.cgi?acc=GSE169687.
Author contributions
YL, TZ conceived and designed this research. YL carried out the data analysis and data interpretation. TZ is responsible for literature searching on the background of this disease and the image processing. JC and SZ wrote most of the article. CH annotated the picture and wrote the conclusion. JL reviewed and revised the manuscript. All authors contributed to the article and approved the submitted version.
Funding
This research was funded by grants from the Guangzhou Municipal Science and Technology Project, Guangdong, China (Grant No. 201803010053).
Acknowledgments
We are grateful to the researchers who built the public database and shared an enormous amount of research data, which made our study possible through their generous contributions.
Conflict of interest
The authors declare that the research was conducted in the absence of any commercial or financial relationships that could be construed as a potential conflict of interest.
Publisher’s note
All claims expressed in this article are solely those of the authors and do not necessarily represent those of their affiliated organizations, or those of the publisher, the editors and the reviewers. Any product that may be evaluated in this article, or claim that may be made by its manufacturer, is not guaranteed or endorsed by the publisher.
Supplementary material
The Supplementary Material for this article can be found online at: https://www.frontiersin.org/articles/10.3389/fimmu.2022.952987/full#supplementary-material
References
1. Organization WH. Who coronavirus (Covid-19) dashboard (2022). Available at: https://covid19.who.int/.
2. Wiersinga WJ, Rhodes A, Cheng AC, Peacock SJ, Prescott HC. Pathophysiology, transmission, diagnosis, and treatment of coronavirus disease 2019 (Covid-19): A review. JAMA (2020) 324(8):782–93. doi: 10.1001/jama.2020.12839
3. Whitaker M, Elliott J, Bodinier B, Barclay W, Ward H, Cooke G, et al. Variant-specific symptoms of covid-19 among 1,542,510 people in England. medRxiv (2022) 2022.05.21.22275368. doi: 10.1101/2022.05.21.22275368
4. Whitaker M, Elliott J, Chadeau-Hyam M, Riley S, Darzi A, Cooke G, et al. Persistent symptoms following sars-Cov-2 infection in a random community sample of 508,707 people. medRxiv (2021) 13(1):1957. doi: 10.1101/2021.06.28.21259452
5. Huang C, Huang L, Wang Y, Li X, Ren L, Gu X, et al. 6-month consequences of covid-19 in patients discharged from hospital: A cohort study. Lancet (2021) 397(10270):220–32. doi: 10.1016/s0140-6736(20)32656-8
6. Komaroff AL, Lipkin WI. Insights from myalgic Encephalomyelitis/Chronic fatigue syndrome may help unravel the pathogenesis of postacute covid-19 syndrome. Trends Mol Med (2021) 27(9):895–906. doi: 10.1016/j.molmed.2021.06.002
7. Statistics OfN. Prevalence of ongoing symptoms following coronavirus (Covid-19) infection in the uk (2022). Available at: https://www.ons.gov.uk/peoplepopulationandcommunity/healthandsocialcare/conditionsanddiseases/bulletins/prevalenceofongoingsymptomsfollowingcoronaviruscovid19infectionintheuk/6may2022.
8. Statistics OfN. The prevalence of long covid symptoms and covid-19 complications (2020). Available at: https://www.ons.gov.uk/news/statementsandletters/theprevalenceoflongcovidsymptomsandcovid19complications.
9. Ziauddeen N, Gurdasani D, O’Hara ME, Hastie C, Roderick P, Yao G, et al. Characteristics of long covid: Findings from a social media survey. medRxiv (2021) 2021.03.21.21253968. doi: 10.1101/2021.03.21.21253968
10. Solomon L, Reeves WC. Factors influencing the diagnosis of chronic fatigue syndrome. Arch Internal Med (2004) 164(20):2241–5. doi: 10.1001/archinte.164.20.2241
11. Committee on the Diagnostic Criteria for Myalgic Encephalomyelitis/Chronic Fatigue S, Board on the Health of Select P. Institute of M. The National Academies Collection: Reports Funded by National Institutes of Health Beyond myalgic Encephalomyelitis/Chronic fatigue syndrome: Redefining an illness. Washington (DC): National Academies Press (2015).
12. Bateman L, Bested AC, Bonilla HF, Chheda BV, Chu L, Curtin JM, et al. Myalgic Encephalomyelitis/Chronic fatigue syndrome: Essentials of diagnosis and management. Mayo Clinic Proc (2021) 96(11):2861–78. doi: 10.1016/j.mayocp.2021.07.004
13. Kedor C, Freitag H, Meyer-Arndt L, Wittke K, Zoller T, Steinbeis F, et al. Chronic covid-19 syndrome and chronic fatigue syndrome (Me/Cfs) following the first pandemic wave in Germany – a first analysis of a prospective observational study. medRxiv (2021) 22(5):bbab115. doi: 10.1101/2021.02.06.21249256
14. Komaroff AL, Bateman L. Will covid-19 lead to myalgic Encephalomyelitis/Chronic fatigue syndrome? Front Med (2021) 7:606824. doi: 10.3389/fmed.2020.606824
15. Paul BD, Lemle MD, Komaroff AL, Snyder SH. Redox imbalance links covid-19 and myalgic Encephalomyelitis/Chronic fatigue syndrome. Proc Natl Acad Sci U.S.A. (2021) 118(34):e2024358118. doi: 10.1073/pnas.2024358118
16. Al-Jassas HK, Al-Hakeim HK, Maes M. Intersections between pneumonia, lowered oxygen saturation percentage and immune activation mediate depression, anxiety, and chronic fatigue syndrome-like symptoms due to covid-19: A nomothetic network approach. J Affect Disord (2022) 297:233–45. doi: 10.1016/j.jad.2021.10.039
17. Alwan NA. The road to addressing long covid. Science (2021) 373(6554):491–3. doi: 10.1126/science.abg7113
18. Nalbandian A, Sehgal K, Gupta A, Madhavan MV, McGroder C, Stevens JS, et al. Post-acute covid-19 syndrome. Nat Med (2021) 27(4):601–15. doi: 10.1038/s41591-021-01283-z
19. Phetsouphanh C, Darley DR, Wilson DB, Howe A, Munier CML, Patel SK, et al. Immunological dysfunction persists for 8 months following initial mild-to-Moderate sars-Cov-2 infection. Nat Immunol (2022) 23(2):210–6. doi: 10.1038/s41590-021-01113-x
20. Cheung CCL, Goh D, Lim X, Tien TZ, Lim JCT, Lee JN, et al. Residual sars-Cov-2 viral antigens detected in gi and hepatic tissues from five recovered patients with covid-19. Gut (2022) 71(1):226–9. doi: 10.1136/gutjnl-2021-324280
21. Komaroff AL. Advances in understanding the pathophysiology of chronic fatigue syndrome. Jama (2019) 322(6):499–500. doi: 10.1001/jama.2019.8312
22. Mariman AN, Vogelaers DP, Tobback E, Delesie LM, Hanoulle IP, Pevernagie DA. Sleep in the chronic fatigue syndrome. Sleep Med Rev (2013) 17(3):193–9. doi: 10.1016/j.smrv.2012.06.003
23. Keller BA, Pryor JL, Giloteaux L. Inability of myalgic Encephalomyelitis/Chronic fatigue syndrome patients to reproduce Vo2Peak indicates functional impairment. J Transl Med (2014) 12:104. doi: 10.1186/1479-5876-12-104
24. Choutka J, Jansari V, Hornig M, Iwasaki A. Unexplained post-acute infection syndromes. Nat Med (2022) 28(5):911–23. doi: 10.1038/s41591-022-01810-6
25. Jammes Y, Steinberg JG, Delliaux S. Chronic fatigue syndrome: Acute infection and history of physical activity affect resting levels and response to exercise of plasma Oxidant/Antioxidant status and heat shock proteins. J Intern Med (2012) 272(1):74–84. doi: 10.1111/j.1365-2796.2011.02488.x
26. Wang EY, Mao T, Klein J, Dai Y, Huck JD, Jaycox JR, et al. Diverse functional autoantibodies in patients with covid-19. Nature (2021) 595(7866):283–8. doi: 10.1038/s41586-021-03631-y
27. Mahmud SMH, Al-Mustanjid M, Akter F, Rahman MS, Ahmed K, Rahman MH, et al. Bioinformatics and system biology approach to identify the influences of sars-Cov-2 infections to idiopathic pulmonary fibrosis and chronic obstructive pulmonary disease patients. Briefings Bioinf (2021) 22(5):bbab115. doi: 10.1093/bib/bbab115
28. van Kampen AHC, Moerland PD. Taking bioinformatics to systems medicine. In: Schmitz U, Wolkenhauer O, editors. Systems medicine. New York, NY: Humana Press (2016). p. 17–41.
29. Davis AP, Grondin CJ, Johnson RJ, Sciaky D, Wiegers J, Wiegers TC, et al. Comparative toxicogenomics database (Ctd): Update 2021. Nucleic Acids Res (2021) 49(D1):D1138–d43. doi: 10.1093/nar/gkaa891
30. Safran M, Rosen N, Twik M, BarShir R, Stein TI, Dahary D, et al. The genecards suite. In: Practical guide to life science databases. Springer Nature Singapore: Springer (2021). p. 27–56.
31. Piñero J, Ramírez-Anguita JM, Saüch-Pitarch J, Ronzano F, Centeno E, Sanz F, et al. The disgenet knowledge platform for disease genomics: 2019 update. Nucleic Acids Res (2019) 48(D1):D845–D55. doi: 10.1093/nar/gkz1021
32. Barrett T, Wilhite SE, Ledoux P, Evangelista C, Kim IF, Tomashevsky M, et al. Ncbi geo: Archive for functional genomics data sets–update. Nucleic Acids Res (2013) 41(Database issue):D991–5. doi: 10.1093/nar/gks1193
33. Ryan FJ, Hope CM, Masavuli MG, Lynn MA, Mekonnen ZA, Yeow AEL, et al. Long-term perturbation of the peripheral immune system months after sars-Cov-2 infection. BMC Med (2022) 20(1):26. doi: 10.1186/s12916-021-02228-6
34. Byrnes A, Jacks A, Dahlman-Wright K, Evengard B, Wright FA, Pedersen NL, et al. Gene expression in peripheral blood leukocytes in monozygotic twins discordant for chronic fatigue: No evidence of a biomarker. PloS One (2009) 4(6):e5805. doi: 10.1371/journal.pone.0005805
35. Gow JW, Hagan S, Herzyk P, Cannon C, Behan PO, Chaudhuri A. A gene signature for post-infectious chronic fatigue syndrome. BMC Med Genomics (2009) 2:38. doi: 10.1186/1755-8794-2-38
36. Raijmakers RPH, Jansen AFM, Keijmel SP, Ter Horst R, Roerink ME, Novakovic B, et al. A possible role for mitochondrial-derived peptides humanin and mots-c in patients with q fever fatigue syndrome and chronic fatigue syndrome. J Transl Med (2019) 17(1):157. doi: 10.1186/s12967-019-1906-3
37. Bouquet J, Li T, Gardy JL, Kang X, Stevens S, Stevens J, et al. Whole blood human transcriptome and virome analysis of Me/Cfs patients experiencing post-exertional malaise following cardiopulmonary exercise testing. PloS One (2019) 14(3):e0212193. doi: 10.1371/journal.pone.0212193
38. Love MI, Huber W, Anders S. Moderated estimation of fold change and dispersion for rna-seq data with Deseq2. Genome Biol (2014) 15(12):550. doi: 10.1186/s13059-014-0550-8
39. Ritchie ME, Phipson B, Wu D, Hu Y, Law CW, Shi W, et al. Limma powers differential expression analyses for rna-sequencing and microarray studies. Nucleic Acids Res (2015) 43(7):e47. doi: 10.1093/nar/gkv007
40. Kuleshov MV, Jones MR, Rouillard AD, Fernandez NF, Duan Q, Wang Z, et al. Enrichr: A comprehensive gene set enrichment analysis web server 2016 update. Nucleic Acids Res (2016) 44(W1):W90–7. doi: 10.1093/nar/gkw377
41. Ashburner M, Ball CA, Blake JA, Botstein D, Butler H, Cherry JM, et al. Gene ontology: Tool for the unification of biology. the gene ontology consortium. Nat Genet (2000) 25(1):25–9. doi: 10.1038/75556
42. Consortium GO. The gene ontology resource: Enriching a gold mine. Nucleic Acids Res (2021) 49(D1):D325–d34. doi: 10.1093/nar/gkaa1113
43. Kanehisa M, Furumichi M, Sato Y, Ishiguro-Watanabe M, Tanabe M. Kegg: Integrating viruses and cellular organisms. Nucleic Acids Res (2020) 49(D1):D545–D51. doi: 10.1093/nar/gkaa970
44. Martens M, Ammar A, Riutta A, Waagmeester A, Slenter DN, Hanspers K, et al. Wikipathways: Connecting communities. Nucleic Acids Res (2021) 49(D1):D613–d21. doi: 10.1093/nar/gkaa1024
45. Jassal B, Matthews L, Viteri G, Gong C, Lorente P, Fabregat A, et al. The reactome pathway knowledgebase. Nucleic Acids Res (2020) 48(D1):D498–d503. doi: 10.1093/nar/gkz1031
46. Nishimura D. Biocarta. Biotech Softw Internet Rep (2001) 2:117–20. doi: 10.1089/152791601750294344
47. Szklarczyk D, Gable AL, Nastou KC, Lyon D, Kirsch R, Pyysalo S, et al. The string database in 2021: Customizable protein-protein networks, and functional characterization of user-uploaded Gene/Measurement sets. Nucleic Acids Res (2021) 49(D1):D605–d12. doi: 10.1093/nar/gkaa1074
48. Shannon P, Markiel A, Ozier O, Baliga NS, Wang JT, Ramage D, et al. Cytoscape: A software environment for integrated models of biomolecular interaction networks. Genome Res (2003) 13(11):2498–504. doi: 10.1101/gr.1239303
49. Cline MS, Smoot M, Cerami E, Kuchinsky A, Landys N, Workman C, et al. Integration of biological networks and gene expression data using cytoscape. Nat Protoc (2007) 2(10):2366–82. doi: 10.1038/nprot.2007.324
50. Otasek D, Morris JH, Bouças J, Pico AR, Demchak B. Cytoscape automation: Empowering workflow-based network analysis. Genome Biol (2019) 20(1):185. doi: 10.1186/s13059-019-1758-4
51. Chin CH, Chen SH, Wu HH, Ho CW, Ko MT, Lin CY. Cytohubba: Identifying hub objects and Sub-networks from complex interactome. BMC Syst Biol (2014) 8 Suppl 4(Suppl 4):S11. doi: 10.1186/1752-0509-8-s4-s11
52. Zhou G, Soufan O, Ewald J, Hancock REW, Basu N, Xia J. Networkanalyst 3.0: A visual analytics platform for comprehensive gene expression profiling and meta-analysis. Nucleic Acids Res (2019) 47(W1):W234–w41. doi: 10.1093/nar/gkz240
54. Davis CA, Hitz BC, Sloan CA, Chan ET, Davidson JM, Gabdank I, et al. The encyclopedia of DNA elements (Encode): Data portal update. Nucleic Acids Res (2018) 46(D1):D794–d801. doi: 10.1093/nar/gkx1081
55. Consortium EP. An integrated encyclopedia of DNA elements in the human genome. Nature (2012) 489(7414):57–74. doi: 10.1038/nature11247
56. Bartel DP. Micrornas: Genomics, biogenesis, mechanism, and function. Cell (2004) 116(2):281–97. doi: 10.1016/s0092-8674(04)00045-5
57. Hobert O. Common logic of transcription factor and microrna action. Trends Biochem Sci (2004) 29(9):462–8. doi: 10.1016/j.tibs.2004.07.001
58. Zaborowski AB, Walther D. Determinants of correlated expression of transcription factors and their target genes. Nucleic Acids Res (2020) 48(20):11347–69. doi: 10.1093/nar/gkaa927
59. Zhou Y, Ferguson J, Chang JT, Kluger Y. Inter- and intra-combinatorial regulation by transcription factors and micrornas. BMC Genomics (2007) 8:396. doi: 10.1186/1471-2164-8-396
60. Iwama H, Murao K, Imachi H, Ishida T. Microrna networks alter to conform to transcription factor networks adding redundancy and reducing the repertoire of target genes for coordinated regulation. Mol Biol Evol (2011) 28(1):639–46. doi: 10.1093/molbev/msq231
61. Liu ZP, Wu C, Miao H, Wu H. Regnetwork: An integrated database of transcriptional and post-transcriptional regulatory networks in human and mouse. Database (Oxford) (2015) 2015:bav095. doi: 10.1093/database/bav095
62. Yoo M, Shin J, Kim J, Ryall KA, Lee K, Lee S, et al. Dsigdb: Drug signatures database for gene set analysis. Bioinformatics (2015) 31(18):3069–71. doi: 10.1093/bioinformatics/btv313
63. Carfì A, Bernabei R, Landi F, Group ftGAC-P-ACS. Persistent symptoms in patients after acute covid-19. JAMA (2020) 324(6):603–5. doi: 10.1001/jama.2020.12603
64. Garrigues E, Janvier P, Kherabi Y, Le Bot A, Hamon A, Gouze H, et al. Post-discharge persistent symptoms and health-related quality of life after hospitalization for covid-19. J Infect (2020) 81(6):e4–6. doi: 10.1016/j.jinf.2020.08.029
65. Davis HE, Assaf GS, McCorkell L, Wei H, Low RJ, Re'em Y, et al. Characterizing long covid in an international cohort: 7 months of symptoms and their impact. EClinicalMedicine (2021) 38:101019. doi: 10.1016/j.eclinm.2021.101019
66. Blomberg B, Mohn KG, Brokstad KA, Zhou F, Linchausen DW, Hansen BA, et al. Long covid in a prospective cohort of home-isolated patients. Nat Med (2021) 27(9):1607–13. doi: 10.1038/s41591-021-01433-3
67. Spudich S, Nath A. Nervous system consequences of covid-19. Science (2022) 375(6578):267–9. doi: 10.1126/science.abm2052
68. White P. Long covid: Don't consign Me/Cfs to history. Nature (2020) 587(7833):197. doi: 10.1038/d41586-020-03136-0
69. Subbaraman N. Us health agency will invest $1 billion to investigate 'Long covid'. Nature (2021) 591(7850):356. doi: 10.1038/d41586-021-00586-y
70. Hunt J, Blease C, Geraghty KJ. Long covid at the crossroads: Comparisons and lessons from the treatment of patients with myalgic Encephalomyelitis/Chronic fatigue syndrome (Me/Cfs). J Health Psychol (2022) 13591053221084494. doi: 10.1177/13591053221084494
71. Routen A, O’Mahoney L, Ayoubkhani D, Banerjee A, Brightling C, Calvert M, et al. Understanding and tracking the impact of long covid in the united kingdom. Nat Med (2022) 28(1):11–5. doi: 10.1038/s41591-021-01591-4
72. Banerjee A. Long covid: New wine in need of new bottles. BMJ (2021) 375:n2736. doi: 10.1136/bmj.n2736
74. Meisel SR, Shapiro H, Radnay J, Neuman Y, Khaskia AR, Gruener N, et al. Increased expression of neutrophil and monocyte adhesion molecules lfa-1 and mac-1 and their ligand icam-1 and vla-4 throughout the acute phase of myocardial infarction: Possible implications for leukocyte aggregation and microvascular plugging. J Am Coll Cardiol (1998) 31(1):120–5. doi: 10.1016/s0735-1097(97)00424-5
75. Fox SE, Akmatbekov A, Harbert JL, Li G, Quincy Brown J, Vander Heide RS. Pulmonary and cardiac pathology in African American patients with covid-19: An autopsy series from new Orleans. Lancet Respir Med (2020) 8(7):681–6. doi: 10.1016/s2213-2600(20)30243-5
76. Neeper M, Schmidt AM, Brett J, Yan SD, Wang F, Pan YC, et al. Cloning and expression of a cell surface receptor for advanced glycosylation end products of proteins. J Biol Chem (1992) 267(21):14998–5004. doi: 10.1016/S0021-9258(18)42138-2
77. Bierhaus A, Ritz E, Nawroth PP. Expression of receptors for advanced glycation end-products in occlusive vascular and renal disease. Nephrol Dial Transplant (1996) 11 Suppl 5:87–90. doi: 10.1093/ndt/11.supp5.87
78. Soulis T, Thallas V, Youssef S, Gilbert RE, McWilliam BG, Murray-McIntosh RP, et al. Advanced glycation end products and their receptors Co-localise in rat organs susceptible to diabetic microvascular injury. Diabetologia (1997) 40(6):619–28. doi: 10.1007/s001250050725
79. Basta G, Schmidt AM, De Caterina R. Advanced glycation end products and vascular inflammation: Implications for accelerated atherosclerosis in diabetes. Cardiovasc Res (2004) 63(4):582–92. doi: 10.1016/j.cardiores.2004.05.001
80. Kajikawa M, Nakashima A, Fujimura N, Maruhashi T, Iwamoto Y, Iwamoto A, et al. Ratio of serum levels of ages to soluble form of rage is a predictor of endothelial function. Diabetes Care (2015) 38(1):119–25. doi: 10.2337/dc14-1435
81. Heier M, Margeirsdottir HD, Gaarder M, Stensæth KH, Brunborg C, Torjesen PA, et al. Soluble rage and atherosclerosis in youth with type 1 diabetes: A 5-year follow-up study. Cardiovasc Diabetol (2015) 14:126. doi: 10.1186/s12933-015-0292-2
82. Sagris M, Theofilis P, Antonopoulos AS, Tsioufis C, Oikonomou E, Antoniades C, et al. Inflammatory mechanisms in covid-19 and atherosclerosis: Current pharmaceutical perspectives. Int J Mol Sci (2021) 22(12):6607. doi: 10.3390/ijms22126607
83. Erener S. Diabetes, infection risk and covid-19. Mol Metab (2020) 39:101044. doi: 10.1016/j.molmet.2020.101044
84. Chiappalupi S, Salvadori L, Vukasinovic A, Donato R, Sorci G, Riuzzi F. Targeting rage to prevent sars-Cov-2-Mediated multiple organ failure: Hypotheses and perspectives. Life Sci (2021) 272:119251. doi: 10.1016/j.lfs.2021.119251
85. Conca W, Alabdely M, Albaiz F, Foster MW, Alamri M, Alkaff M, et al. Serum β2-microglobulin levels in coronavirus disease 2019 (Covid-19): Another prognosticator of disease severity? PloS One (2021) 16(3):e0247758. doi: 10.1371/journal.pone.0247758
86. Pacha O, Sallman MA, Evans SE. Covid-19: A case for inhibiting il-17? Nat Rev Immunol (2020) 20(6):345–6. doi: 10.1038/s41577-020-0328-z
87. Raucci F, Mansour AA, Casillo GM, Saviano A, Caso F, Scarpa R, et al. Interleukin-17a (Il-17a), a key molecule of innate and adaptive immunity, and its potential involvement in covid-19-Related thrombotic and vascular mechanisms. Autoimmun Rev (2020) 19(7):102572. doi: 10.1016/j.autrev.2020.102572
88. Azevedo MLV, Zanchettin AC, Vaz de Paula CB, Motta Júnior JDS, Malaquias MAS, Raboni SM, et al. Lung neutrophilic recruitment and il-8/Il-17a tissue expression in covid-19. Front Immunol (2021) 12:656350. doi: 10.3389/fimmu.2021.656350
89. Gong B, Huang L, He Y, Xie W, Yin Y, Shi Y, et al. A genetic variant in il-6 lowering its expression is protective for critical patients with covid-19. Signal Transduct Target Ther (2022) 7(1):112. doi: 10.1038/s41392-022-00923-1
90. Pan R, Zhang Q, Anthony SM, Zhou Y, Zou X, Cassell M, et al. Oligodendrocytes that survive acute coronavirus infection induce prolonged inflammatory responses in the cns. Proc Natl Acad Sci USA (2020) 117(27):15902–10. doi: 10.1073/pnas.2003432117
91. Nakatomi Y, Mizuno K, Ishii A, Wada Y, Tanaka M, Tazawa S, et al. Neuroinflammation in patients with chronic fatigue Syndrome/Myalgic encephalomyelitis: An ¹¹c-(R)-Pk11195 pet study. J Nucl Med (2014) 55(6):945–50. doi: 10.2967/jnumed.113.131045
92. Kistner TM, Pedersen BK, Lieberman DE. Interleukin 6 as an energy allocator in muscle tissue. Nat Metab (2022) 4(2):170–9. doi: 10.1038/s42255-022-00538-4
93. Naviaux RK, Naviaux JC, Li K, Bright AT, Alaynick WA, Wang L, et al. Metabolic features of chronic fatigue syndrome. Proc Natl Acad Sci USA (2016) 113(37):E5472–80. doi: 10.1073/pnas.1607571113
94. Domingo JC, Cordobilla B, Ferrer R, Giralt M, Alegre-Martín J, Castro-Marrero J. Are circulating fibroblast growth factor 21 and n-terminal prohormone of brain natriuretic peptide promising novel biomarkers in myalgic Encephalomyelitis/Chronic fatigue syndrome? Antioxid Redox Signal (2021) 34(18):1420–7. doi: 10.1089/ars.2020.8230
95. Szklarski M, Freitag H, Lorenz S, Becker SC, Sotzny F, Bauer S, et al. Delineating the association between soluble Cd26 and autoantibodies against G-protein coupled receptors, immunological and cardiovascular parameters identifies distinct patterns in post-infectious vs. non-Infection-Triggered myalgic Encephalomyelitis/Chronic fatigue syndrome. Front Immunol (2021) 12:644548. doi: 10.3389/fimmu.2021.644548
96. Fenouillet E, Vigouroux A, Steinberg JG, Chagvardieff A, Retornaz F, Guieu R, et al. Association of biomarkers with health-related quality of life and history of stressors in myalgic Encephalomyelitis/Chronic fatigue syndrome patients. J Transl Med (2016) 14(1):251. doi: 10.1186/s12967-016-1010-x
97. Cantu N, Vyavahare S, Kumar S, Chen J, Kolhe R, Isales CM, et al. Synergistic effects of multiple factors involved in covid-19-Dependent muscle loss. Aging Dis (2022) 13(2):344–52. doi: 10.14336/ad.2021.0817
98. Russell CD, Valanciute A, Gachanja NN, Stephen J, Penrice-Randal R, Armstrong SD, et al. Tissue proteomic analysis identifies mechanisms and stages of immunopathology in fatal covid-19. Am J Respir Cell Mol Biol (2022) 66(2):196–205. doi: 10.1165/rcmb.2021-0358OC
99. Harford JB, Kim SS, Pirollo KF, Chang EH. Tp53 gene therapy as a potential treatment for patients with covid-19. Viruses (2022) 14(4):739. doi: 10.3390/v14040739
100. Kesmez Can F, Özkurt Z, Öztürk N, Sezen S. Effect of il-6, il-8/Cxcl8, ip-10/Cxcl 10 levels on the severity in covid 19 infection. Int J Clin Pract (2021) 75(12):e14970. doi: 10.1111/ijcp.14970
101. Fletcher MA, Zeng XR, Barnes Z, Levis S, Klimas NG. Plasma cytokines in women with chronic fatigue syndrome. J Transl Med (2009) 7:96. doi: 10.1186/1479-5876-7-96
102. Urano T, Suzuki Y, Iwaki T, Sano H, Honkura N, Castellino FJ. Recognition of plasminogen activator inhibitor type 1 as the primary regulator of fibrinolysis. Curr Drug Targets (2019) 20(16):1695–701. doi: 10.2174/1389450120666190715102510
103. Goshua G, Pine AB, Meizlish ML, Chang CH, Zhang H, Bahel P, et al. Endotheliopathy in covid-19-Associated coagulopathy: Evidence from a single-centre, cross-sectional study. Lancet Haematol (2020) 7(8):e575–e82. doi: 10.1016/s2352-3026(20)30216-7
104. Koh TJ, Bryer SC, Pucci AM, Sisson TH. Mice deficient in plasminogen activator inhibitor-1 have improved skeletal muscle regeneration. Am J Physiol Cell Physiol (2005) 289(1):C217–23. doi: 10.1152/ajpcell.00555.2004
105. Zhang HM, Kuang S, Xiong X, Gao T, Liu C, Guo AY. Transcription factor and microrna Co-regulatory loops: Important regulatory motifs in biological processes and diseases. Brief Bioinform (2015) 16(1):45–58. doi: 10.1093/bib/bbt085
106. Wilson JG, Simpson LJ, Ferreira AM, Rustagi A, Roque J, Asuni A, et al. Cytokine profile in plasma of severe covid-19 does not differ from Ards and sepsis. JCI Insight (2020) 5(17):e140289. doi: 10.1172/jci.insight.140289
107. Dorgham K, Quentric P, Gökkaya M, Marot S, Parizot C, Sauce D, et al. Distinct cytokine profiles associated with covid-19 severity and mortality. J Allergy Clin Immunol (2021) 147(6):2098–107. doi: 10.1016/j.jaci.2021.03.047
108. Russell L, Broderick G, Taylor R, Fernandes H, Harvey J, Barnes Z, et al. Illness progression in chronic fatigue syndrome: A shifting immune baseline. BMC Immunol (2016) 17:3. doi: 10.1186/s12865-016-0142-3
109. Cammaerts S, Strazisar M, Smets B, Weckhuysen S, Nordin A, De Jonghe P, et al. Schizophrenia-associated Mir204 regulates noncoding rnas and affects neurotransmitter and ion channel gene sets. PloS One (2015) 10(12):e0144428. doi: 10.1371/journal.pone.0144428
110. Schaar D, Goodell L, Aisner J, Cui XX, Han ZT, Chang R, et al. A phase I clinical trial of 12- O-Tetradecanoylphorbol-13-Acetate for patients with Relapsed/Refractory malignancies. Cancer Chemother Pharmacol (2006) 57(6):789–95. doi: 10.1007/s00280-005-0125-1
111. Andreakos E, Papadaki M, Serhan CN. Dexamethasone, pro-resolving lipid mediators and resolution of inflammation in covid-19. Allergy (2021) 76(3):626–8. doi: 10.1111/all.14595
112. Papadopoulos A, Ebrecht M, Roberts AD, Poon L, Rohleder N, Cleare AJ. Glucocorticoid receptor mediated negative feedback in chronic fatigue syndrome using the low dose (0.5 mg) dexamethasone suppression test. J Affect Disord (2009) 112(1-3):289–94. doi: 10.1016/j.jad.2008.05.001
113. Perez-Alvarez S, Solesio ME, Manzanares J, Jordán J, Galindo MF. Lactacystin requires reactive oxygen species and bax redistribution to induce mitochondria-mediated cell death. Br J Pharmacol (2009) 158(4):1121–30. doi: 10.1111/j.1476-5381.2009.00388.x
114. Morris G, Stubbs B, Köhler CA, Walder K, Slyepchenko A, Berk M, et al. The putative role of oxidative stress and inflammation in the pathophysiology of sleep dysfunction across neuropsychiatric disorders: Focus on chronic fatigue syndrome, bipolar disorder and multiple sclerosis. Sleep Med Rev (2018) 41:255–65. doi: 10.1016/j.smrv.2018.03.007
Keywords: Long COVID, myalgic encephalomyelitis/chronic fatigue syndrome, ME/CFS, systems biology, bioinformatics analyses, protein–protein interaction network
Citation: Lv Y, Zhang T, Cai J, Huang C, Zhan S and Liu J (2022) Bioinformatics and systems biology approach to identify the pathogenetic link of Long COVID and Myalgic Encephalomyelitis/Chronic Fatigue Syndrome. Front. Immunol. 13:952987. doi: 10.3389/fimmu.2022.952987
Received: 25 May 2022; Accepted: 18 August 2022;
Published: 16 September 2022.
Edited by:
Danuta Radzioch, McGill University, CanadaReviewed by:
Jose Alegre-Martin, Vall d’Hebron University Hospital, SpainHussein Kadhem Al-Hakeim, University of Kufa, Iraq
Copyright © 2022 Lv, Zhang, Cai, Huang, Zhan and Liu. This is an open-access article distributed under the terms of the Creative Commons Attribution License (CC BY). The use, distribution or reproduction in other forums is permitted, provided the original author(s) and the copyright owner(s) are credited and that the original publication in this journal is cited, in accordance with accepted academic practice. No use, distribution or reproduction is permitted which does not comply with these terms.
*Correspondence: Jianbo Liu, 13538759071@163.com
†These authors have contributed equally to this work and share first authorship